- Department of Animal and Poultry Science, College of Aburaihan, University of Tehran, Tehran, Iran
Objective: Mastitis is defined as the inflammation of the mammary gland, which impact directly on the production performance and welfare of dairy cattle. Since, mastitis is a multifactorial complex disease and the molecular pathways underlying this disorder have not been clearly understood yet, a system biology approach was used in this study to a better understanding of the molecular mechanisms behind mastitis.
Methods: Publicly available RNA-Seq data containing samples from milk of five infected and five healthy Holstein cows at five time points were retrieved. Gene Co-expression network analysis (WGCNA) approach and functional enrichment analysis were then applied with the aim to find the non-preserved module of genes that their connectivity were altered under infected condition. Hub genes were identified in the non-preserved modules and were subjected to protein-protein interactions (PPI) network construction.
Results: Among the 25 modules identified, eight modules were non-preserved and were also biologically associated with inflammation, immune response and mastitis development. Interestingly most of the hub genes in the eight modules were also densely connected in the PPI network. Of the hub genes, 250 genes were hubs in both co-expression and PPI networks and most of them were reported to play important roles in immune response or inflammatory pathways. The blue module was highly enriched in inflammatory responses and STAT1 was suggested to play an important role in mastitis development by regulating the immune related genes in this module. Moreover, a set of highly connected genes were identified such as BIRC3, PSMA6, FYN, F11R, NFKBIZ, NFKBIA, GRO1, PHB, CD3E, IL16, GSN, SOCS2, HCK, VAV1 and TLR6, which have been established to be critical for mastitis pathogenesis.
Conclusion: This study improved the understanding of the mechanisms underlying bovine mastitis and suggested eight non-preserved modules along with several most important genes with promising potential in etiology of mastitis.
Introduction
Mastitis, inflammation of the mammary gland, is one of the most prevalent diseases in dairy cattle around the world (Munro et al., 1984; Biggs, 2009). Mastitis is mainly caused by pathogens, which can be divided into contagious, non-contagious (Staphylococcus aureus, Streptococcus agalactiae, Corynebacterium bovis, Mycoplasma bovis) and peripheral (E. coli, Streptococcus dysgalactiae, Streptococcus uberis) (Zhao and Lacasse, 2008). Mastitis has been considered as one of the most economically important disorders due to its negative effects on quantity (Schukken et al., 2009) and quality of milk (Hortet and Seegers, 1998), animal welfare (Peters et al., 2015) reproductive performance (Kumar et al., 2017), increased use of antibiotics (Groot and van’t Hooft, 2016) and the need for the treatment and premature culling of dairy cows (Heikkilä et al., 2012). Streptococcus uberis enters the udder via the teat canal and produces clinical and subclinical cases of mastitis (e.g., Hogan et al., 1989; Williamson et al., 1995). In this regard, environmental streptococci (Streptococcus uberis) are responsible for one third of clinical mastitis cases (Hillerton and Berry, 2003) and considers as the most prevalent mastitis causing pathogens throughout Europe and North America (Ward et al., 2009; Reinoso et al., 2011). In comparison to Escherichia, Streptococcus uberis induces a delayed mRNA expression of interleukin-8 by epithelial cells (Wellnitz et al., 2006). This cytokine is involved in the recruitment of neutrophils, which play roles in the healing of intramammary infections (Barber and Yang, 1998). Previous studies reported that a wide variety of strains can infect the mammary gland with different intensity (Leigh et al., 1990; Phuektes et al., 2001). This constitutes a major obstacle in the effective treatment and development of strategies to control this important mastitis pathogen. Hence, a more precise identification of dynamics of infection and new candidate genes in the development of mastitis induced by Streptococcus uberis would be useful.
Several studies have been conducted on different aspects of the topic such as nutrition (Heinrichs et al., 2009), management (Neave et al., 1969), or genetic (De Vliegher et al., 2012) to prevent or alleviate the consequences of bovine mastitis. The previous studies have been reported some differentially expressed genes (DEGs) as potential candidates in both inflammatory responses (Lutzow et al., 2008) and overall metabolism (Mitterhuemer et al., 2010) including TLR2, TLR4, S100A12, IL8, CD14, IL-1β, IL-6, IL-8, and TNFα. For example, Lawless et al. (2014) used RNA sequencing to identify mRNA and miRNA genes involved in bovine mastitis and reported more than 3700 DEGs, which were significantly enriched in inflammatory and non-glycolytic metabolic pathways. However, it is well-known that differential expression analysis merely focuses on the effect of individual genes rather than considering the effect of clusters of genes. Therefore, individual assessment of gene expression may fail to explain the complex etiology of diseases or traits of interest.
On the other hand, gene expression data can also be used for constructing the gene regulatory networks (like co-expression gene networks), using a system biology approach, to better understand molecular mechanisms behind the complex diseases such as mastitis (Nielen et al., 1995). Moyes et al. (2009) used a gene regulatory approach to understand the most affected gene networks in bovine mammary tissue in response to infection. They found some pro-inflammatory pathways associated with a marked inhibition of lipid synthesis, stress-activated kinase signaling cascades and PPAR signaling were activated (Moyes et al., 2009). In the study of Han (2019) by using gene regulatory network approach, discovered that differential expressed genes in the E. coli-inoculated and the S. aureus-inoculated groups, were associated with the RIG-I-like receptor signaling pathway and lysosome pathway, respectively. The main assumption underlying gene co-expression networks states that highly co-expressed genes are likely to be functionally associated. In this regard, a well-known and widely used method for constructing the gene co-expression networks is weighted gene co-expression network analysis (WGCNA) (Langfelder and Horvath, 2008). WGCNA considers the differences in the response of the samples at different time points by clustering the genes into the specified modules based on the expression correlation patterns among genes across the samples. Potential of this approach for grouping genes into the functional modules and revealing regulatory mechanisms underlying the complex traits have been highlighted in many recent studies (Bakhtiarizadeh et al., 2018). Using WGCNA, highly connected genes (called hub genes) can also be screened within the modules, based on intramodular gene connectivity. Moreover, WGCNA provides a unique network-based strategy to access whether the network density and topology pattern of a module, obtained from a given set of samples (normal samples), are preserved in another set of samples (disease samples) (Langfelder and Horvath, 2008). Thus, some modules and their hub genes that are not preserved between these situations may potentially be involved in the biological processes of interest (Botía et al., 2017).
It is of great significance to understand the mechanisms of disease. High-throughput technologies combined with novel computational systems biology approach have provided new opportunities for a better understanding of the molecular regulatory mechanisms that mastitis can be developed (Sharifi et al., 2019). Hence, in the present study, RNA-Seq data was obtained from a previous study (Lawless et al., 2014) and was used to construct the modules with biologically related genes in healthy bovine samples by WGCNA method. Then, module preservation functionality in WGCNA was served to discover non-preserved modules in infected samples and further functional analysis were carried out. The main assumption was that non-preserved modules may contain potential functionally related genes or possibly share common biological regulatory functions in pathological processes related to mastitis. This effort can accelerate discovery of genes as well as molecular mechanisms responsible for immune response to mastitis in cattle.
Materials and Methods
Gene Expression Dataset
RNA-Seq data of healthy and infected bovine samples were obtained from the Gene Expression Omnibus (GEO) database at the National Center for Biotechnology Information (NCBI) under accession number of GSE51858. The data included samples from milk of five infected (via the teat canal of right-front quarter with 500 units of Streptococcus uberis (S. uberis0140) colonies at 0, 12, 24, 36, 48 h after infection), as well as five healthy Holstein cows, at the same time points. More details of the data can be found in the original paper (Lawless et al., 2014). Briefly, in Lawless et al., study Milk-derived CD14 + monocytes (CD14 is a receptor that binds to LPS and mediates the LPS-induced activation of host cells) were isolated by fluorescence-activated cell sorting. These cells were then labeled with monoclonal anti-bovine CD14 and a PE-conjugated anti-mouse IgG1 antibody. Labeled cells were separated based on fluorescence intensity and the cells with more than 95% purity were isolated from the milk of each animal. The infection was monitored using recorded milk bacterial counts (CFU/ml) and somatic cell counts (per ml) at each of the five time points for each animal (control and infected). An Illumina HiSeq 2000 tool was used to generate 50-bp single-end reads and totally 50 samples were created (five biological replications for each time point). After obtaining the data, five samples (including GSM1254091, GSM1254117, GSM1254119, GSM1254120, and GSM1254121) were removed due to low quality reads (Q < 20 and low number of reads) and the remaining 45 samples (24 healthy and 21 infected samples) were kept for further analysis.
RNA-Seq Data Analysis and Preprocessing
Quality control of the raw data was evaluated using FastQC (version 0.10.1) (Andrews, 2010). Trimmomatic software (version 0.32) (Bolger et al., 2014) was used to filter out the adapter sequences and low quality bases/reads with trimming criteria: LEADING:20, ILLUMINACLIP: Adapters.fa:2:30:10, and MINLEN:25. The clean reads were checked again using FastQC. The clean reads were then aligned to the reference bovine genome using Tophat software (version 2.1.0) (Trapnell et al., 2009). The bovine genome was downloaded from the Ensembl database (version UMD_3.1). The reads were mapped according to the genomic annotations provided in the bovine Ensembl annotation in gene transfer format (GTF). HTSeq-count software (Python package HTSeq, version 2.7.3) (Anders et al., 2015) was applied to count aligned reads that overlapped with all bovine gene using the bovine GTF file. All the count files were then merged into a count table containing read-count information for all samples. Since WGCNA approach was originally developed for microarray data, raw counts data have to be normalized to be suitable for WGCNA analysis. Hence, the raw counts data were normalized to log-counts per million (log-cpm), using the voom normalization function of the limma package (version 3.40.2) (Smyth, 2005). Taking into account that genes with very low expression are less reliable and indistinguishable from sampling noise, the genes with less than one cpm (count per million) in at least five samples and standard deviation lower than 0.25 were filtered out.
WGCNA Network Analysis
Network analysis was performed according to the protocol of the WGCNA R-package (version 1.68) (Langfelder and Horvath, 2008). Firstly, in order to remove outlier samples, distance-based adjacency matrices of samples were estimated and sample network connectivity according to the distances was standardized. Samples with connectivity less than -2.5 were considered as outliers and were excluded (Supplementary File S1). Then, reliability of samples and genes for WGCNA analysis was inspected to exclude the samples and genes with excessive missing entries and genes with zero variance. Based on the assumption that non-preserved modules between healthy and infected groups may be functionally related to mastitis, healthy samples were considered as the reference set for module detection. Here, a signed weighted gene co-expression network was constructed, which only consider positively correlated genes and genes with negative correlation are considered unconnected. Generally, signed networks are preferred over unsigned networks and appeared to be more robust by identifying modules with more significant enrichment of functional terms (Langfelder and Horvath, 2008; Smita et al., 2013). Also, biweight midcorrelation was used instead of the Pearson or Spearman correlation, because it is robust and resistant to outliers (Song et al., 2012). To be sure that the constructed network satisfies the scale-free topology (a fundamental property of biological gene networks in which some genes are more connected than others), an appropriate soft-thresholding power was calculated by plotting the R2 (scale-free topology fitting index) against soft thresholds. At β = 23, network created by WGNCA showed >90% scale free topology in healthy samples. Supplementary File S2 shows the relationship between the β and scale free topology fitting index in healthy samples. Then, the co-expression modules were constructed using automatic module detection function blockwiseModules of WGCNA and four important steps were performed including (1) create weighted adjacency matrix by calculating biweight midcorrelation between each gene pairs to determine connection strengths between them, (2) transform weighted adjacency matrix into a topological overlap matrix (TOM), which summarizes the network connectivity of genes, (3) identify the modules by average linkage hierarchical clustering analysis through a dynamic hybrid tree cutting algorithm and using TOM graphs as input (by defining a dissimilarity matrix, 1-TOM), (4) merge the modules with highly correlated eigengenes, which have extremely similar expression profiles. The following parameters were used; power = 23, corType = “bicor,” minModuleSize = 30, mergeCutHeight = 0.25, maxBlockSize = 17,000, reassignThreshold = 0, networkType = “signed,” and TOMType = “signed.”
Preservation Analysis
Module preservation analysis allowed us to evaluate how well the modular structure of the healthy samples are preserved in the infected samples. To do this, the function “module Preservation” of WGCNA package was used and a permutation test (based on the generation of 200 random permutations) was calculated, which assesses the preservation of the connectivity and density of each network module. In this study, a combination of two widely used network-based module-preservation statistics including Zsummary and medianRank scores were applied to determine the modules which are preserved, semi-preserved or non-preserved. Zsummary is a composite module preservation statistic that simultaneously assess whether the genes in a defined module in the healthy samples remain highly connected in the infected samples as well as investigate whether the connectivity patterns between the genes in the healthy samples remain similar, compared with the infected samples (Langfelder et al., 2011). Zsummary allows for significance thresholds but shows a strong dependence on module size and tends to increase with module size. On the other hand, medianRank is the mean of median ranks computed for connectivity and density measures of each module and is independent of module size. Hence, medianRank is more robust and always apply to confirm the Zsummary results. Totally, a module with lower medianRank or higher Zsummary tends to exhibit stronger preservations. Here, the modules with Zsummary >10 and medianRank <8 were interpreted as highly preserved, the modules with 2< Zsummary ≤8 and medianRank <8 were defined as semi-conserved and the modules with Zsummary <2 and medianRank ≥8 were considered to be non-preserved.
Functional Enrichment Analysis
In order to better understand the potential mechanisms of how module genes can affect mastitis, all genes in the modules as well as their hub genes were separately subjected into gene ontology (GO) and KEGG pathways analysis using Enrichr online tool (Chen et al., 2013). Only terms with adjusted p < 0.05 (FDR by Benjamini–Hochberg method) were considered.
Potential Hub Genes Identification and PPI Network Construction
The genes with the highest degree of connectivity in the module are considered as the hub genes and is expected to exhibit higher biologically significance compared with the other gene members of the module. Module membership or kME is defined as the correlation between the gene expression profile and the module eigengene for each gene in the module. In other words, kME measures the connection strength of a gene with the module it has been assigned to and to the other modules as well. We used kME >0.7 to identify the hub genes in the non-preserved modules. Next, the identified hub genes in each module were subjected to PPI network construction using Search Tool for the Retrieval of Interacting Genes (STRING) database and medium stringency settings was set and included all possible interactions (Szklarczyk et al., 2019). This analysis investigates whether co-expressed hub genes in each module are still significantly associated, based on PPIs data, or not. To explore the important nodes and subnetworks in the PPI networks, cytoHubba application (version 0.1), a Cytoscape plugin, was used (Chin et al., 2014). This application suggests 12 topological analysis methods for ranking the important nodes in a biological network including maximum click centrality (MCC), density of maximum neighborhood component (DMNC), maximum neighborhood component (MNC), degree method, edge percolated component (EPC), bottleneck, EcCentricity, closeness, radiality, betweenness, stress, and clustering coefficient (Chin et al., 2014). The top 50 important genes in each PPI network were ranked using all methods. To establish a consensus rank of the important genes, rankAggreg R package (version 0.6.5) (Pihur et al., 2009) was applied based on two methods including cross-entropy Monte Carlo algorithm and Genetic algorithm. Finally, the overlapped genes between these two methods were defined as highly connected genes. Cytoscape (version 3.7.2) (Saito et al., 2012) was used to visualize the gene co-expression network of the important non-preserved modules.
Results
RNA-Seq Data Analysis
A stringent stepwise pipeline was used to construct the co-expression gene network (Figure 1). A total of 1,935,472,920 reads related to 45 RNA-Seq samples (24 healthy and 21 infected bovine samples) were analyzed. After trimming the raw data, a total of 1,690,493,091clean reads were obtained. The average sample sizes were 43 and 37 million reads before and after quality control, respectively. On average 93% of the clean reads were aligned to reference genome (ranged from 84 to 94%). The summary of the RNA-Seq data and mapping of all samples are provided in Supplementary File S3. To remove the genes with very low expression in most of the samples, different filtering parameters were used and a total of 9,721 genes were remained for network construction. The complete list of these genes along with their log-transformed expressions are provided in Supplementary File S4.
Weighted Co-expression Network Construction
To avoid the influence of potential outlier samples, one outlier sample (GSM1254086, from healthy samples) whose connectivity was less than -2.5 was removed. To fulfill the criteria of approximate scale-free topology, the soft threshold power beta was set to 23 in which R2 was >0.9 (Supplementary File S2). Taking healthy bovine samples as the reference set, hierarchic clustering and dynamic branch cutting procedures resulted in identification of 25 modules. Each module as a branch of the resulting clustering tree was labeled by a unique color (Figure 2). The modules inferred showing different sizes, with an average of 398 genes. The turquoise module had the largest number of genes (1,132), while the orange module with 42 genes showed the smallest number of genes (Supplementary File S5). Also, 460 genes were assigned into gray module, which represents the genes that were not co-expressed based on gene dissimilarity measure and were not assigned into any of the modules.
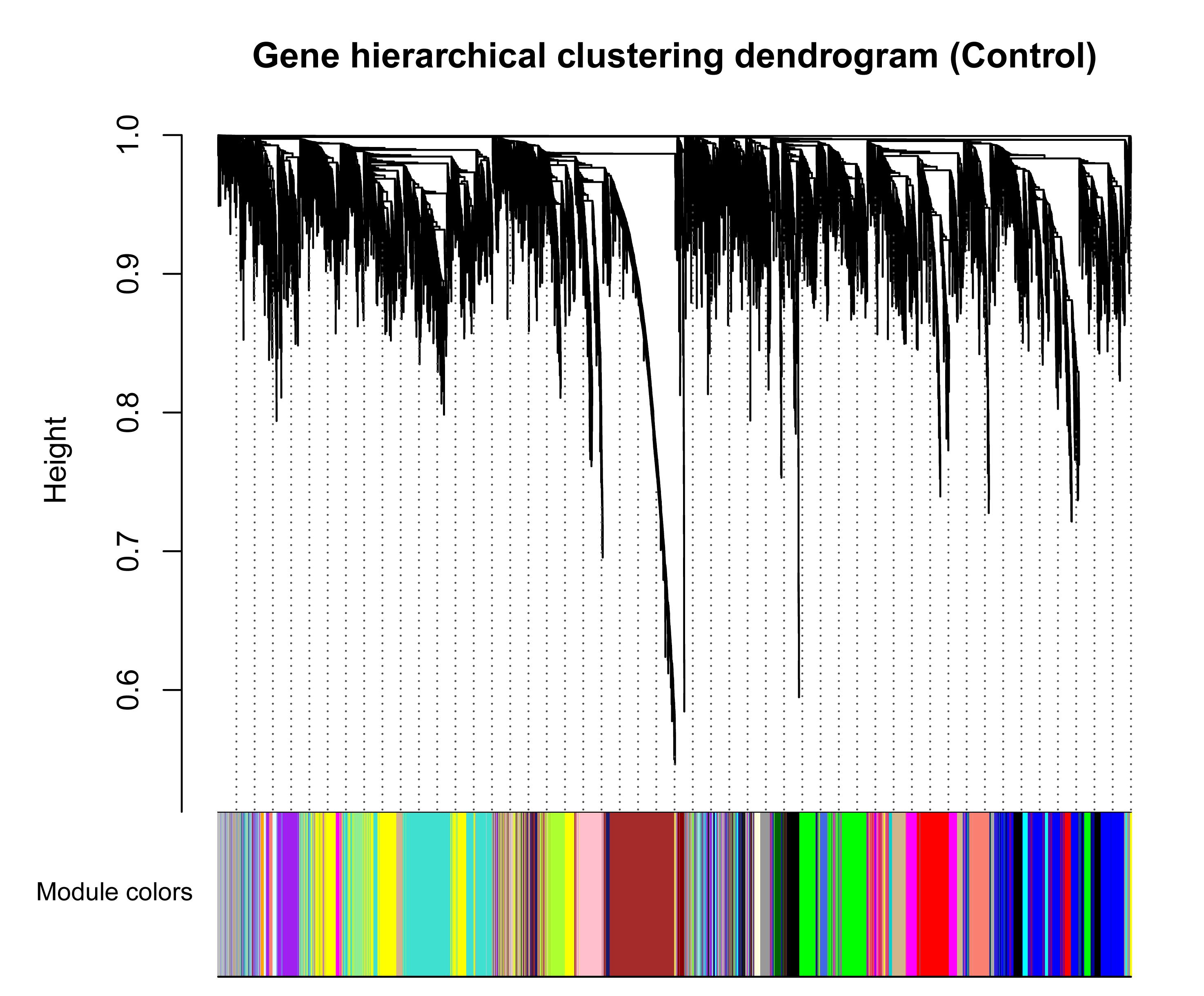
Figure 2. Clustering dendrogram of genes based on a dissimilarity measure (1-TOM), which was then used to group genes into 25 modules in healthy samples. The branches correspond to modules of highly interconnected groups of genes. The height (y-axis) indicates the co-expression distance and the x-axis corresponds to genes. Colors represent the 25 different modules along with gray indicating genes that could not be assigned to any module.
Module Preservation Analysis
Module preservation analysis revealed two highly preserved modules including green and tan modules. Three modules were found to be semi-preserved including pink, grey60, and light-yellow modules. Preservation analysis indicated that expression patterns and network characteristics among the co-expressed genes of 20 modules were changed during the physiological state alteration (healthy state to mastitis state) (Figure 3). Of these, purple and salmon modules were detected as the least preserved modules with 391 and 280 genes in each module, respectively. Their Zsummary were -3.1 and -2.9, respectively, and medianRank score was 24 for both (Supplementary File S6 and Figure 3).
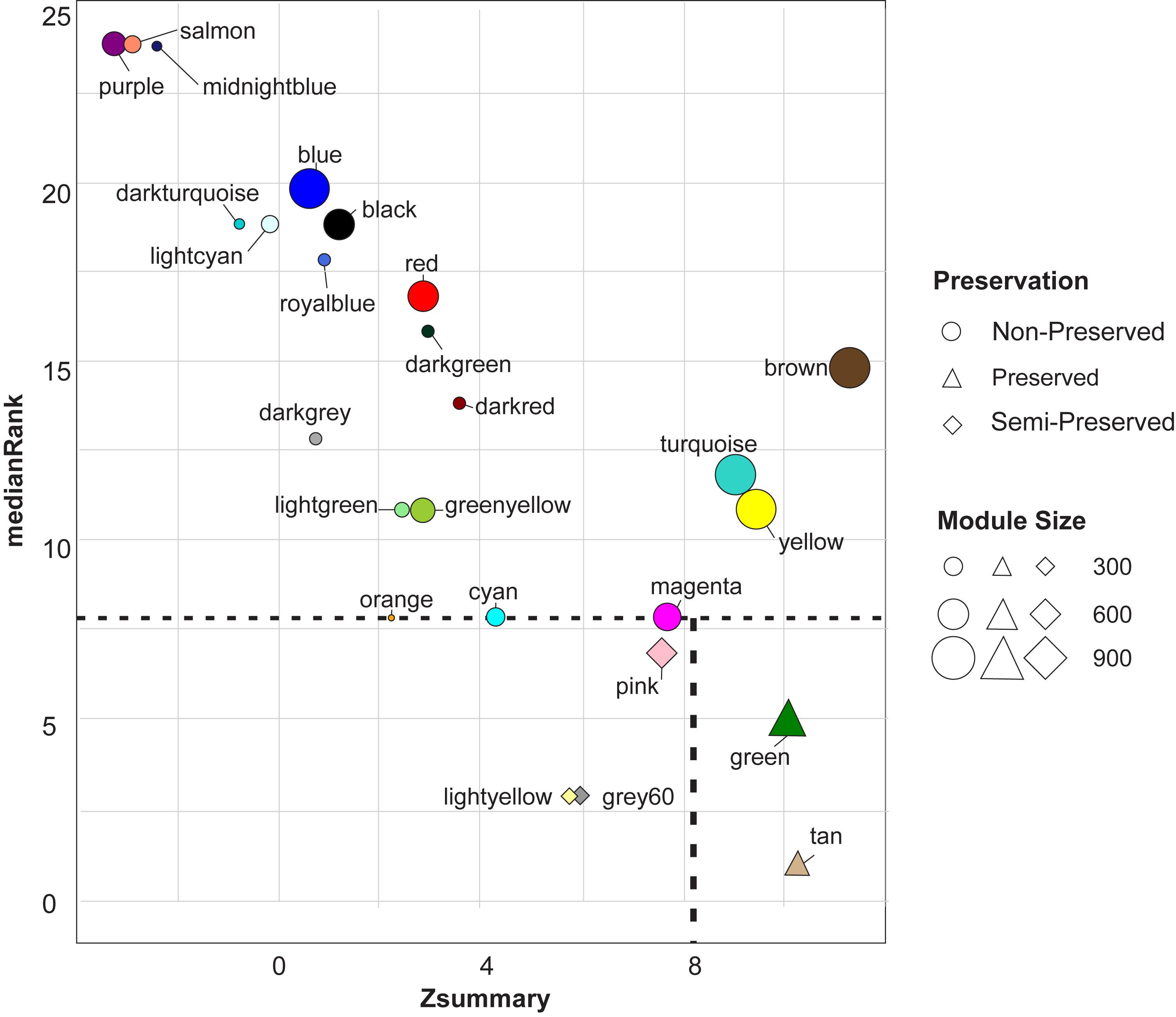
Figure 3. The medianRank (left scatter plot) and Zsummary (right scatter plot) statistics of the module preservation. The medianRank and Zsummary of the modules close to zero indicate the high and low degree of module preservation, respectively. A negative Zsummary value indicates the modules’ disruption.
Functional Enrichment Analysis
To investigate the putative functions associated with the modules, all the identified modules were subjected to functional enrichment analysis. Totally, 408 biological processes and 93 KEGG pathways were significantly enriched in 18 modules. Genes in the green module, as a highly preserved module, were significantly enriched in 49 and six biological processes and KEGG pathways, respectively. Only five KEGG pathways were enriched in the other highly preserved module, tan module. Also, 34, seven and seven biological processes and eight, five and no KEGG pathways were found in pink, lightyellow, and gray60 modules, respectively, as semi-preserved modules. The complete list of the functional enrichment analysis for highly and semi-preserved modules is available in Supplementary File S7. The top 20 significant biological process terms for highly and semi-preserved modules are presented in Figure 4.
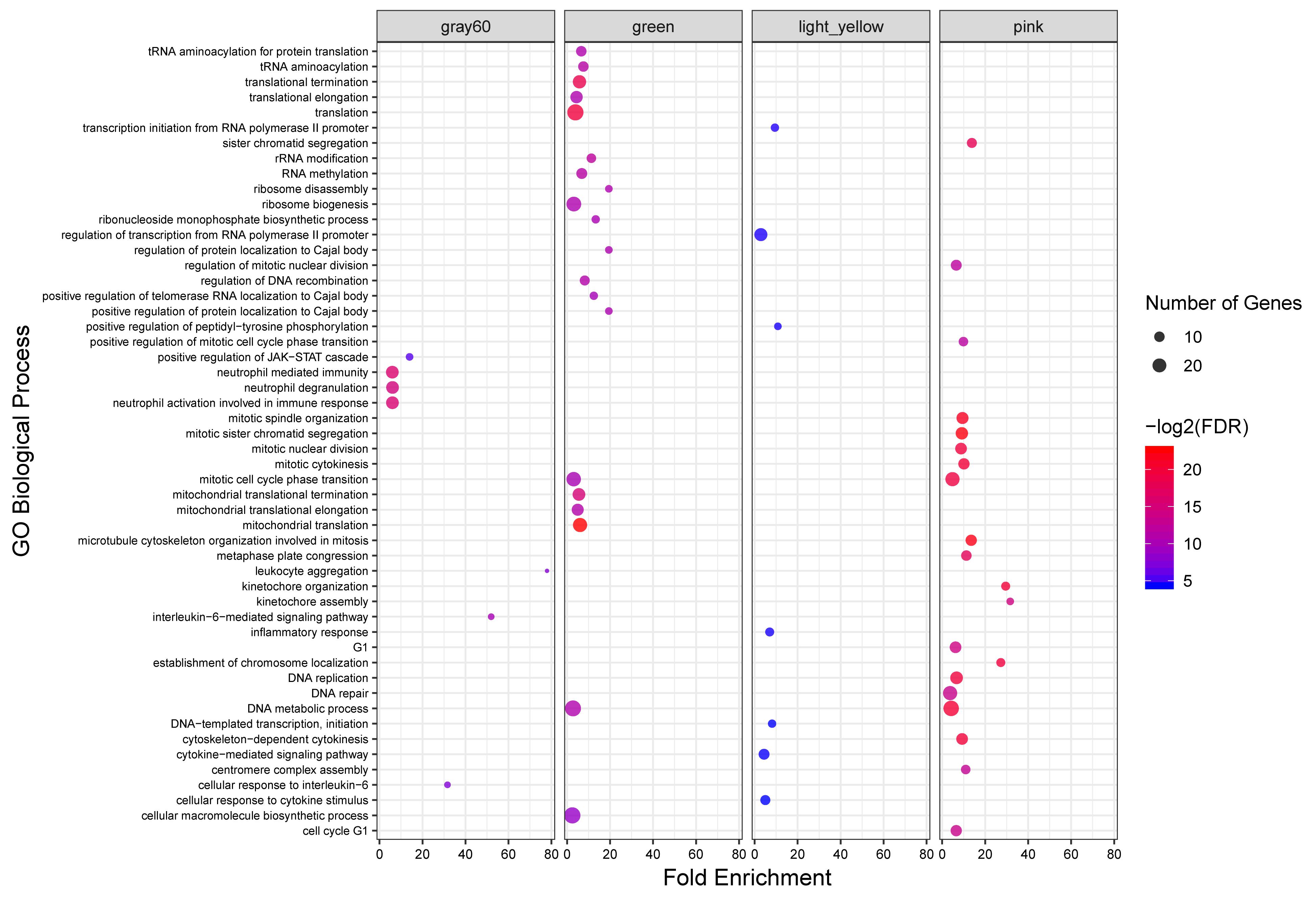
Figure 4. GO analysis results of highly and semi-preserved modules. Owing to the large number of significant GO terms (biological process) in the green and pink modules, only the top 20 significant terms are displayed. Size and color of points represent -Log2 of FDR and number of genes associated with each term, respectively.
No enrichment of biological process and KEGG pathway were detected in seven non-preserved modules including lightcyan, cyan, darkgrey, darkred, darkturquoise, royalblue, and salmon modules. On the other hand, 312 biological processes and 67 KEGG pathways were significantly enriched in the other 13 non-preserved modules. Of these, eight non-preserved modules (including blue, brown, magenta, purple, darkgreen, red, midnightblue, and lightgreen) were associated with immune response functions based on the literature reports or term definition itself. The complete list of the functional enrichment analysis for non-preserved modules is available in Supplementary File S8. Moreover, the top 10 significant biological process terms for the eight non-preserved modules are presented in Figure 5.
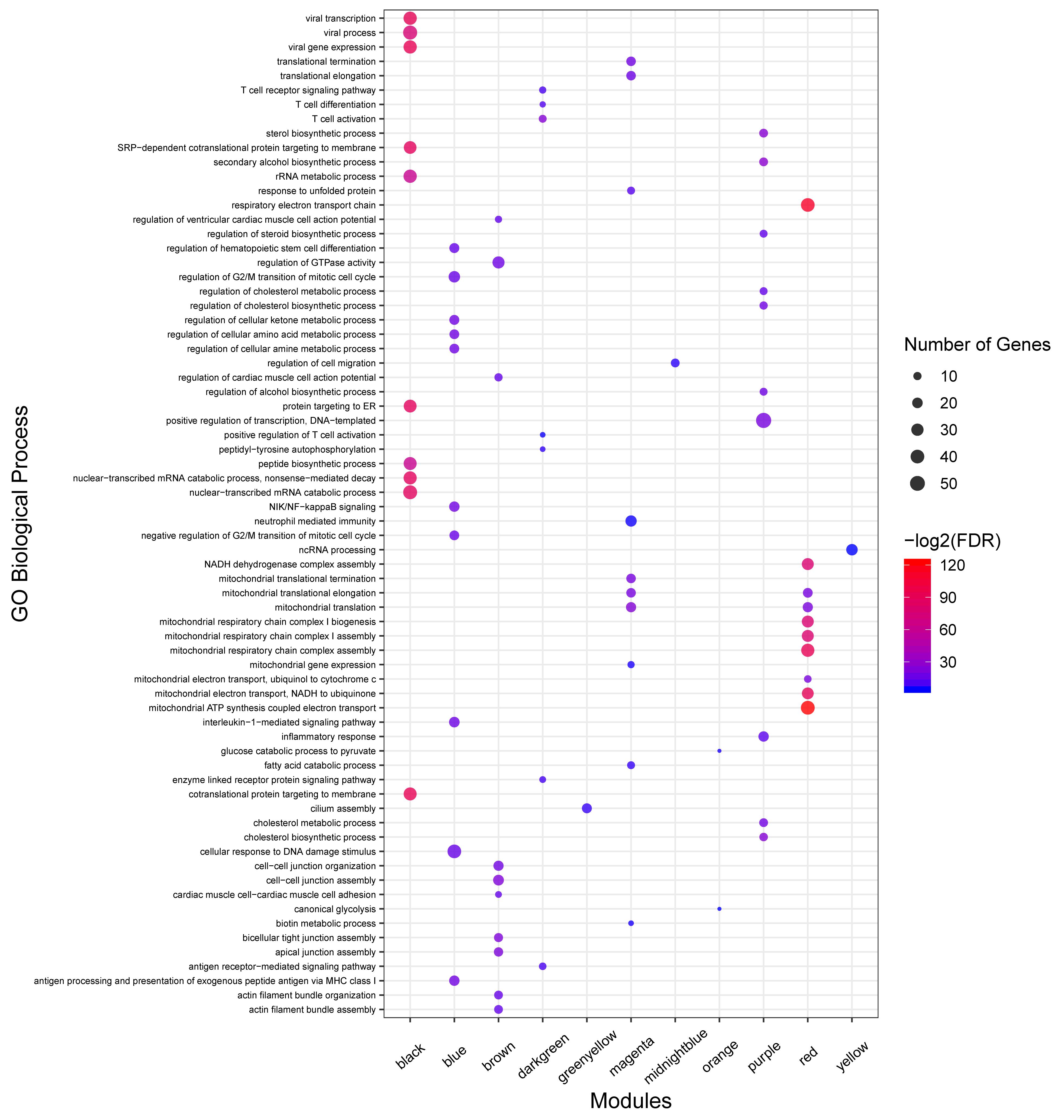
Figure 5. GO analysis results of non-preserved modules. Owing to the large number of significant GO terms (biological process) in blue, brown, red, magenta, black, and purple modules, only the top 10 significant terms are displayed. Size and color of points represent -Log2 of FDR and number of genes associated with each term, respectively. The fold enrichment measure is not shown for better visualization.
Hub Genes Identification in the Non-preserved Modules
Here, the eight non-preserved modules (blue, brown, magenta, purple, darkgreen, red, midnightblue, and lightgreen) were further examined to assess their topological behavior in terms of intra-modular connectivity to identify the hub genes. A total number of 533, 537, 234, 185, 47, 361, 69, and 88 hub genes were found in blue, brown, magenta, purple, darkgreen, red, midnightblue, and lightgreen non-preserved modules, respectively (Supplementary File S9). The identified hub genes in each module were analyzed for detecting significantly enriched biological processes and KEGG pathways. The results revealed 222 GO terms and 59 KEGG pathways similar to the functional enrichment analysis results of the modules where they have been assigned to (Supplementary File S10). In order to analyze the connections from the proteins encoded by the hub genes of each module, PPI networks were constructed for each hub gene sets in accordance with STRING database. The PPI networks of all eight hub gene sets showed significant connectivity. According to the important nodes detection approach described in the method section, 32, 32, 32, 36, 27, 32, 23, and 36 highly connected genes were detected in the constructed PPI networks, based on the hub genes in blue, brown, magenta, purple, darkgreen, red, midnightblue and lightgreen non-preserved modules, respectively (Table 1). These genes were hubs in their respective non-preserved modules and were also centrally located in their respective PPI networks, which make them promising candidates to illustrate the etiology behind bovine mastitis. The PPI network of the blue module is shown in Figure 6. Also, to have an overall view of the genes in the other non-preserved modules, their connections to each other (PPI networks) are provided in Supplementary File S11.
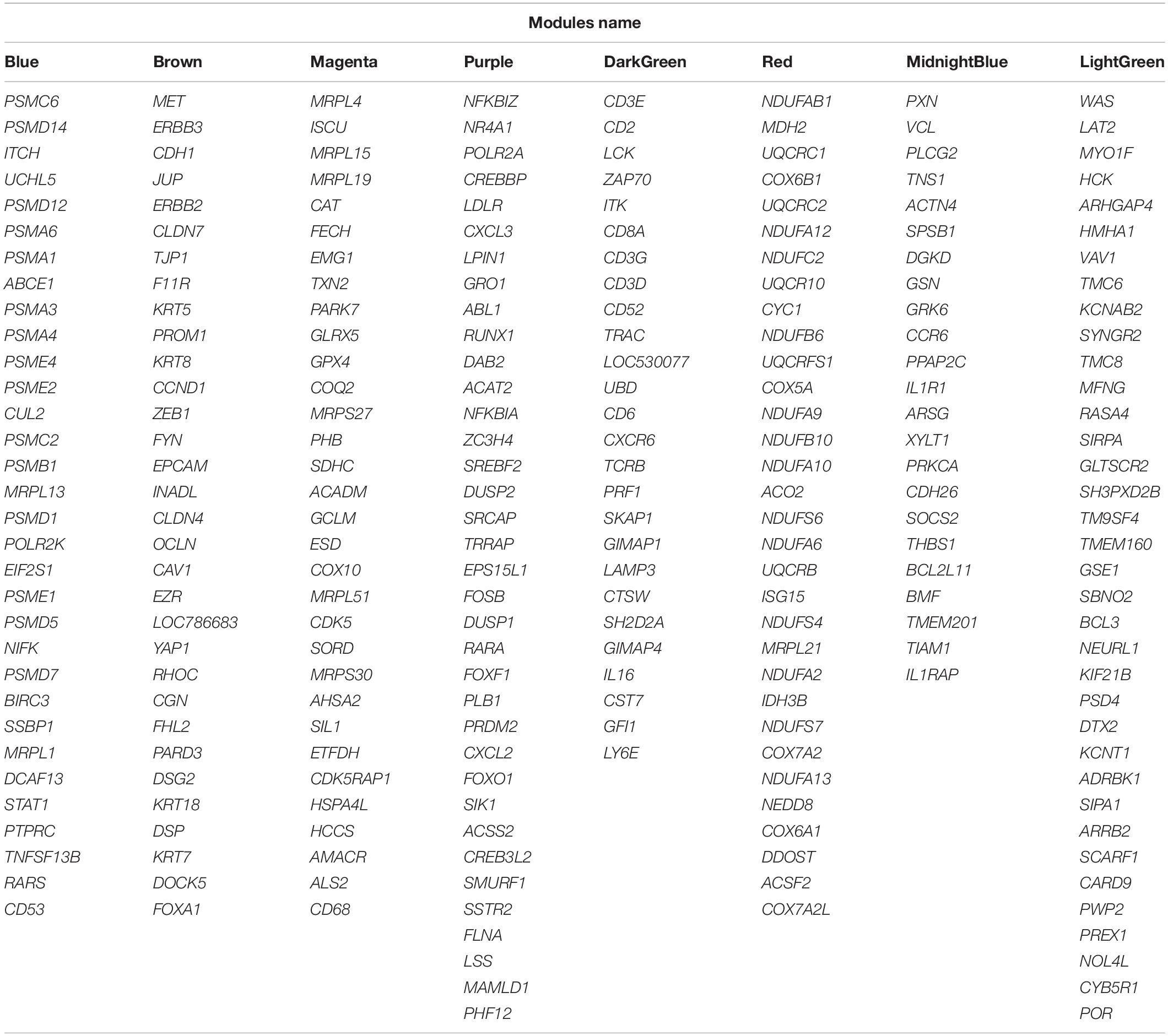
Table 1. List of the highly connected genes in the PPI networks that were constructed based on the hub genes of non-preserved modules.
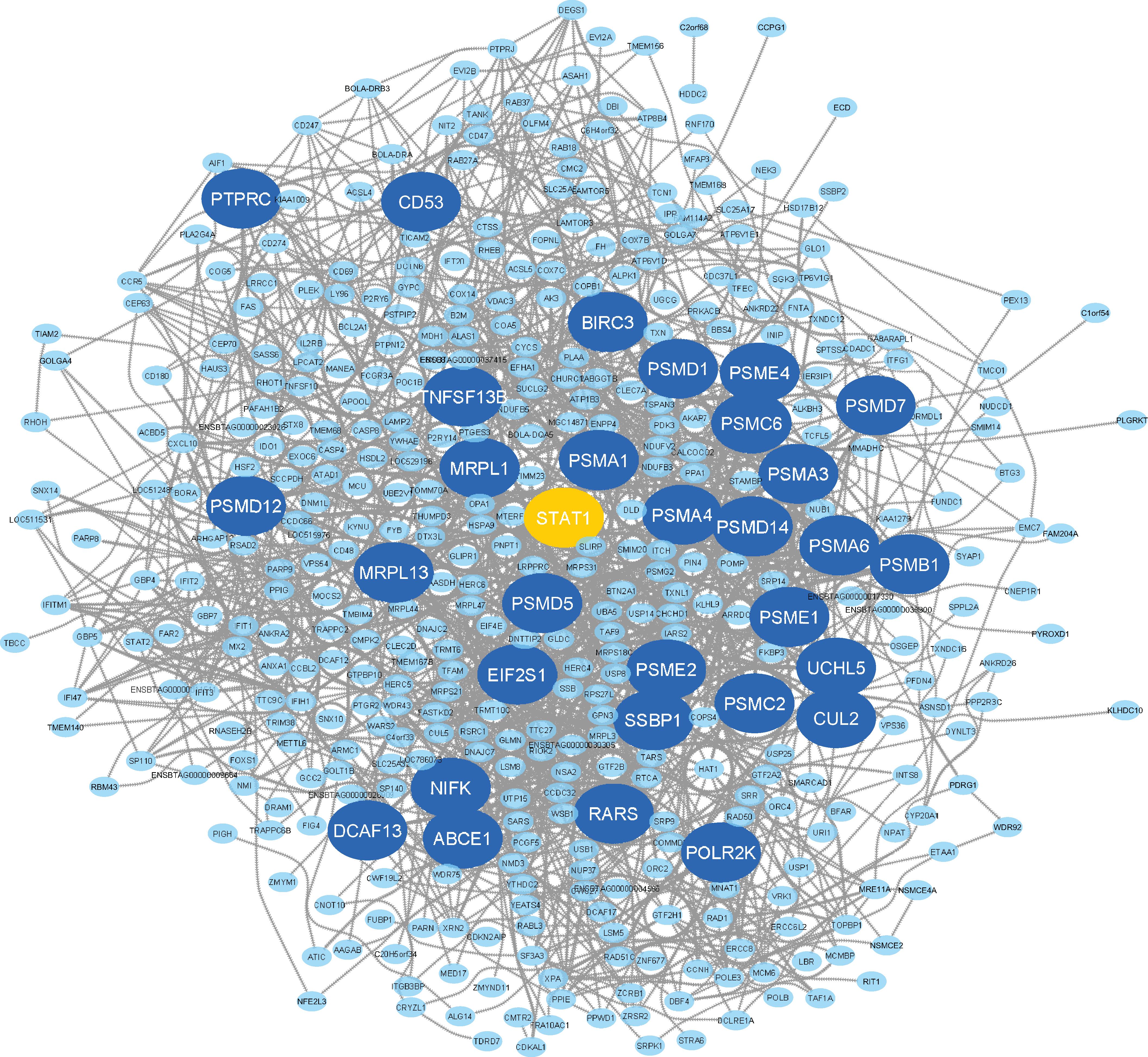
Figure 6. PPI network based on the hub genes of the blue module. The larger circles indicate highly connected genes and the orange circle represents the only TF among the highly connected genes.
Discussion
Mastitis remains among the most challenging disorders in the cattle industry to treat. In this study, WGCNA approach was utilized to improve the efficiency of important genes identification through clustering the genes into modules that are likely enriched for particular biological pathways associated with bovine mastitis. The genes in 72% of the identified modules (18 out of 25) were significantly enriched in at least one GO term or KEGG pathway, which indicated that signed WGCNA effectively clustered the co-regulated and biologically related genes into separate modules. Functional enrichment analysis of the preserved and semi-preserved modules showed enriched terms representing basic biological processes such as “translation,” “rRNA modification,” and “DNA replication.” Therefore, based on the preservation structure and functional enrichment results, these modules cannot potentially distinguish infected from healthy samples. On the other hand, gray60 and lightyellow modules (as semi-preserved) showed some terms that are potentially related to mastitis such as “neutrophil mediated immunity” and “inflammatory response.” However, we focused on the eight non-preserved modules (blue, brown, magenta, purple, darkgreen, red, midnightblue, and lightgreen), which exhibited significantly altered intramodular connectivity in the infected samples as well as showed functional terms related to mastitis or immune responses. It is worth to note that various pathogens, which can cause mastitis, induce different immune responses in the bovine mammary epithelial cells (Wellnitz and Bruckmaier, 2012). Subsequently, we narrowed down the list of genes within the non-preserved modules by focusing on the hub genes. The constructed PPI networks based on the hub genes of all the eight non-preserved modules showed a significant and ideal connectivity, which emphasized effectiveness of our method to organize functional modules that comprises of a set of proteins having similar functions. Hence, these modules might influence the pathogenesis process of mastitis and, therefore, warrant further validation.
Based on the enriched functional terms in the blue module, which were potentially related to mastitis development, this module was determined as one of the most important modules in the present study. Some of the enriched terms included “innate immune response,” “NIK/NF-kappaB signaling,” “interleukin-1-mediated signaling pathway,” “NOD-like receptor signaling pathway,” “toll-like receptor 4 signaling pathway,” “neutrophil mediated immunity,” “T cell receptor signaling pathway,” “cytokine-mediated signaling pathway,” “regulation of defense response,” and “response to cytokine.” Pathogens invades mammary epithelial cells through pattern recognition receptors, which induce different signaling pathways and lead to the establishment of an inflammatory response. Toll-like receptors (TLRs) are one of the most important pattern recognition receptors in various cell types (Dinarello, 2018). TLRs are key participants in the induction of innate immune responses in the mammary gland cells through recognizing various bacterial cell wall components such as lipopolysaccharide (LPS, typical of gram-negative bacteria, e.g., Streptococcus uberis) and lipoteichoic acid (LTA, typical of gram-positive bacteria, e.g., Staphylococcus aureus) (Aderem and Ulevitch, 2000; Taraktsoglou et al., 2011). In this regard, activation of TLR4 is linked to the expression of pro-inflammatory cytokines and the activation of NF-kappaB signaling pathway in mastitis (Wu et al., 2015). Upregulation of TLR4 in bovine macrophages after stimulation with either LTA or LPS has been demonstrated (Franchini et al., 2006). NF-kappaB signaling pathway is a key pathway responsible for the expression of pro-inflammatory cytokines (Wu et al., 2016). This pathway modulates the expression of many inflammation-related genes (such as inflammatory cytokines TNF-α, IL-1β, and IL-6), especially in mastitis (Heyninck et al., 2014). Interestingly, in our study, TLR4 was detected as the hub gene in the blue module. One of the other important pattern recognition receptors are interleukin-1 receptors and their important functions in acute bacterial mastitis have been documented (Filipe et al., 2019). Also, the higher expression of interleukin family members is reported during mastitis infection (Lutzow et al., 2008; Compton et al., 2009). NOD-like receptor signaling pathway is responsible for detecting various pathogens and mediate numerous aspects of innate immunity (dan Xu et al., 2017). This pathway acts in parallel with the Toll-like receptor signaling pathway (Aderem and Ulevitch, 2000). Additionally, NOD-like receptor signaling pathway was observed among the 30 most impacted pathways in the three gene expression-based studies on bovine mastitis (Loor et al., 2011). Neutrophils as a source of small antibacterial peptides, are considered to be a primary defense mechanism to kill a variety of mastitis-causing pathogens. They are the predominant cell types found in the mammary glands during early stage of mastitis and recognize, adhere, and phagocyte invading pathogens (Medina, 2009). T cell receptor signaling pathway is related to adaptive immunity and has been reported as a candidate pathway associated with occurrence and development of mastitis in dairy cow (Luoreng et al., 2018).
Additionally, in terms of individual highly connected genes identified in the blue module, several genes such as BIRC3, PSMA6, PSMB1, PSMD12, PSMD14, PSMD7 (Brand et al., 2011; Loor et al., 2011), EIF2S1 (Appuhamy et al., 2011), PTPRC (Nicholas et al., 2003), and CD53 (Rinaldi et al., 2010) have been reported as important genes in mastitis development. Among the highly connected genes of the blue module, STAT1 was the only TF (Figure 6 and Supplementary File S12). The gene members of the signal transducers and activators of transcription (STAT) family (including STAT1, -2, -3, -4, -5a, -5b, and -6) have been reported as important factors involved in every stage of mammary gland development (Philp et al., 1996; Cobanoglu et al., 2006). Of these, STAT3 and STAT5 have been well-known as important regulators during mammary gland development and tumorigenesis (Philp et al., 1996). The functions of STAT1, STAT3, STAT5, and STAT6 in breast cancer formation, progression, prognosis and prediction have been documented (Haricharan and Li, 2014). Moreover, STAT3 has recently been reported as a potential therapeutic target in mastitis. In this regard, STAT1 has been shown to be important in immune cells in mastitis (Hughes and Wood, 2017). On the other hand, the role of STAT1 as a tumor suppressor has been demonstrated in human breast cancer (Haricharan and Li, 2014). In a good agreement with the recent studies that consider STAT family members as more important candidates in mammary gland development, in the present study, STAT1 was found as the only highly connected TF in the blue module, which reinforce the potential function of this regulator in defense against infection-causing bacteria. Since, co-expressed genes are likely to be functionally related and regulated by the same TF (Behdani and Bakhtiarizadeh, 2017; Bakhtiarizadeh et al., 2018), the other highly connected genes in the blue module might be ideal candidates to better understand molecular mechanisms behind mastitis.
Genes in the brown module showed KEGG pathway enrichment in “Leukocyte transendothelial migration,” “Cell adhesion molecules,” and “Focal adhesion.” Focal adhesion is necessary for cell migration and some important biological processes such as leukocyte transendothelial migration (Jin et al., 2016; Chang et al., 2017). Leukocyte transendothelial migration is an important process in inflammation and the innate immune system that cause the first cellular responders to migrate into infected tissue (Getter et al., 2019). A huge influx of polymorphonuclear leukocytes (the major leukocyte cell type) into the infected mammary glands is an initial inflammatory response to bacterial infection (such as mastitis) (Shah et al., 2018), where they may combat invading pathogens. Cell adhesion molecule is the other important part of the host immune system. Interaction between leukocytes and specific endothelial cell adhesion molecules has been demonstrated, which helps to regulate the migration of leukocytes to the site of inflammation (Kleczkowski et al., 2017). Additionally, some of the highly connected genes belonging to this module have been found by others to be related to mastitis development, immune response or mammary gland development including FYN, CDH11, CAV1, F11R, ZEB1, ERBB2, and ERBB3 (Cohen et al., 2015; Yang et al., 2018). For example, F11R was reported as a candidate gene of mammary gland immune response (Kosciuczuk et al., 2017). These findings supporting the potential functions of the brown module during mastitis infection.
Enrichment of “TNF signaling pathway,” “NF-kappa B signaling pathway,” “Toll-like receptor signaling pathway,” and “MAPK signaling pathway” in the purple module revealed the potential functions of its members in mastitis pathogenesis. Tumor necrosis factor (TNF) is of the cytokine family that coordinates the mammalian immune response and secrete by macrophages in response to endotoxins. TNF signaling pathway is a classical immune system pathway, which has a central role in the control of inflammation, immunity and cell survival (Galvão et al., 2012; Rana et al., 2019). Hence, it is suggested that a potential mechanism to block the development of inflammation, by the effective medicines that used for treating mastitis, is inhibiting TNF signaling pathway through reducing the secretion of TNF (Wu et al., 2019). MAPK signaling pathway plays a key role during inflammatory responses (Kim et al., 2014). It is well-accepted that both of MAPK and NF-kappaB signaling pathways can induce the expressions of various inflammatory mediators and pro-inflammatory cytokines. In a previous study, both of these pathways were activated in LPS-induced mastitis (Liang et al., 2014). Some of the highly connected genes in the purple module have previously been related to mastitis development including NFKBIZ (Compton et al., 2009), NFKBIA, CXCL2, GRO1 (Li et al., 2019), CXCL3 (Rainard et al., 2008), LPIN1 (Moyes et al., 2009), and DAB2 (Banos et al., 2017). For example, NFKBIZ is a regulator of NF-kappaB and gene variants of this gene is introduced as potential markers of mastitis resistance in dairy heifers (Compton et al., 2009). These results suggested that the members of this module may contribute to the pathogenesis of mastitis.
In the magenta module, genes with annotated functions in “neutrophil activation involved in immune response” and “neutrophil mediated immunity” were enriched and are likely to be related to mastitis. Among the highly connected genes in the magenta module, GCLM (Wang K. et al., 2016), PARK7 (Donaldson et al., 2005), SIL1 (Li et al., 2019), PHB (Genini et al., 2011), and CD68 (Bilir et al., 2012) were potentially associated with mastitis or similar biological processes, based on the previous studies. For instance, PARK7 has been shown to play an important role in the bovine immune response (Donaldson et al., 2005).
In agreement with the previous studies (Genini et al., 2011; Luoreng et al., 2018), the functional enrichment results of the darkgreen module reinforced that the “T cell receptor signaling pathway” might be involved in mastitis development. Accordingly, CD3E (Paquette et al., 2015), CD2 (Rivas et al., 2002), LCK (Nie et al., 2012), ZAP70 (Schulman et al., 2009), CD3D (Bonnefont et al., 2011), PRF1 (Twigger et al., 2018), CD8A (Kosciuczuk et al., 2017), and IL16 (Sharmila et al., 2002) were identified as highly connected genes of this module and also have been reported as important genes in immune response or mastitis development.
Genes with biological processes enrichment in “cytokine-mediated signaling pathway” was observed in the red module. It is well-known that the transcription and secretion of proinflammatory/regulatory cytokines occur during the stimulation of bovine macrophages with LPS (in gram-negative bacteria, e.g., Streptococcus uberis) or LTA (in gram-positive bacteria, e.g., Staphylococcus aureus). The inflammatory cytokines can activate the host defense mechanisms during mastitis (Aderem and Ulevitch, 2000; Taraktsoglou et al., 2011). TLR6, as a hub gene in the red module, is an essential component of the recognition complex for LTA (Henneke et al., 2005).
The important enriched pathways in midnightblue module were “Leukocyte transendothelial migration” and “Inflammatory mediator regulation of TRP channels.” Transient receptor potential (TRP) channels are important mediators of sensory signals that have contribution in the detection of physical stimuli including inflammatory stimulations (Levine and Alessandri-Haber, 2007; Zielińska et al., 2015). In the case of the midnightblue module, some of the highly connected genes including GSN (Polgar et al., 2012), CCR6 (Polgar et al., 2012), SOCS2 (Rupp et al., 2015), THBS1 (Wang X.G. et al., 2016), IL1R1, and IL1RAP (Bonnefont et al., 2011) were involved in mastitis defense or immune response. For example, GSN (Marchitelli et al., 2017) has been suggested as indicator of mastitis and many studies have documented important function of SOCS2 in the regulation of cytokines and mastitis (Bonnefont et al., 2011).
In the lightgreen module, KEGG pathway analysis of the genes indicated that the “Chemokine signaling pathway” and “Fc gamma R-mediated phagocytosis” were significantly enriched, which are part of the immune system. These pathways were also found to be significantly enriched in the up-regulated genes in the mammary gland of dairy cattle with E. coli-induced mastitis (Buitenhuis et al., 2011). In this module, the most important highly connected genes were HCK, which encodes two isoforms of a protein-tyrosine kinase involved in mammary gland immune response (Kosciuczuk et al., 2017) and VAV1, which encodes a unique protein involved in tyrosine-mediated signal transduction and immune response (Turner and Billadeau, 2002).
Conclusion
Our results led to identify the eight modules of genes, which were non-preserved between the healthy and infected samples and may play important roles in the pathogenesis of mastitis. Integration of the co-expression network with PPI data enabled us to identify highly connected genes that were hubs in both co-expression and PPI networks. Totally, based on our topological and functional analysis of the eight non-preserved modules, 250 highly connected genes were found, as most of them were directly or indirectly associated with mastitis. These genes can be considered as potential targets for future research aimed at understanding the function of important genes in mastitis pathogenesis. Moreover, the other members of the eight non-preserved modules may potentially play important roles in mastitis development. Therefore, a further analysis and validation of the candidate genes reported here deserve further study, including those that have not yet been associated with mastitis or immune response.
Data Availability Statement
RNA-Seq data of healthy and infected bovine samples were obtained from the Gene Expression Omnibus (GEO) database at the National Center for Biotechnology Information (NCBI) under accession number of GSE51858.
Author Contributions
MB conceived the ideas. MB and SM designed the study and analyzed the data. MB, MN, NS, and MV interpreted the data and wrote the main manuscript text. All authors contributed to the article and approved the submitted version.
Conflict of Interest
The authors declare that the research was conducted in the absence of any commercial or financial relationships that could be construed as a potential conflict of interest.
Acknowledgments
We would like to thank the University of Tehran for the financial support of this work.
Supplementary Material
The Supplementary Material for this article can be found online at: https://www.frontiersin.org/articles/10.3389/fgene.2020.00722/full#supplementary-material
FILE S1 | Sample clustering to detect outliers. All samples (except tc13) were well-clustered.
FILE S2 | Analysis of network topology for a set of soft thresholding powers. The left plot displays the scale of free fit index (y-axis) as a function of the soft thresholding power (x-axis). The right plot shows the mean connectivity (degree, y-axis) as a function of the soft thresholding power (x-axis).
FILE S3 | Descriptive statistics of sequence quality and mapping rate of the RNA-Seq data.
FILE S4 | The gene expression matrix of all samples in normal and mastitis groups.
FILE S5 | The list of genes in each module.
FILE S6 | Summary of module preservation analysis.
FILE S7 | The complete list of the functional enrichment analysis for highly and semi-preserved modules.
FILE S8 | The complete list of the functional enrichment analysis of the identified non-preserved modules.
FILE S9 | The complete list of the hub genes in each module.
FILE S10 | The complete list of the functional enrichment analysis of the hub genes in the eight non-preserved modules.
FILE S11 | Protein-protein interaction networks based on the hub genes of the non-preserved modules of interest.
FILE S12 | The complete list of transcription factors per module. The genes of the modules were aligned with bovine transcription factors extracted from AnimalTFDB database (http://bioinfo.life.hust.edu.cn/AnimalTFDB/).
References
Aderem, A., and Ulevitch, R. J. (2000). Toll-like receptors in the induction of the innate immune response. Nature 406, 782–787. doi: 10.1038/35021228
Anders, S., Pyl, P. T., and Huber, W. (2015). HTSeq-A Python framework to work with high-throughput sequencing data. Bioinformatics 31, 166–169. doi: 10.1093/bioinformatics/btu638
Andrews, S. (2010). FastQC: A Quality Control Tool for High Throughput Sequence Data. Available online at: http://www.bioinformatics.babraham.ac.uk/projects/fastqc/ (accessed March 7, 2020).
Appuhamy, J. A. D. R. N., Bell, A. L., Nayananjalie, W. A. D., Escobar, J., and Hanigan, M. D. (2011). Essential amino acids regulate both initiation and elongation of mRNA translation independent of insulin in MAC-T cells and bovine mammary tissue slices. J. Nutr. 141, 1209–1215. doi: 10.3945/jn.110.136143
Bakhtiarizadeh, M. R., Hosseinpour, B., Shahhoseini, M., Korte, A., and Gifani, P. (2018). Weighted gene co-expression network analysis of endometriosis and identification of functional modules associated with its main hallmarks. Front. Genet. 9:453. doi: 10.3389/fgene.2018.00453
Banos, G., Bramis, G., Bush, S. J., Clark, E. L., McCulloch, M. E. B., Smith, J., et al. (2017). The genomic architecture of mastitis resistance in dairy sheep. BMC Genomics 18:624. doi: 10.1186/s12864-017-3982-1
Barber, M. R., and Yang, T. J. (1998). Chemotactic activities in nonmastitic and mastitic mammary secretions: presence of interleukin-8 in mastitic but not nonmastitic secretions. Clin. Diagn. Lab. Immunol. 5, 82–86. doi: 10.1128/cdli.5.1.82-86.1998
Behdani, E., and Bakhtiarizadeh, M. R. (2017). Construction of an integrated gene regulatory network link to stress-related immune system in cattle. Genetica 145, 441–454. doi: 10.1007/s10709-017-9980-z
Bilir, B. E., Atile, N. S., Bilir, B., Guldiken, S., Tuncbilek, N., Puyan, F. O., et al. (2012). A metabolic syndrome case presenting with lymphocytic mastitis. Breast Care 7, 493–495. doi: 10.1159/000345474
Bolger, A. M., Lohse, M., and Usadel, B. (2014). Trimmomatic: a flexible trimmer for Illumina sequence data. Bioinformatics 30, 2114–2120. doi: 10.1093/bioinformatics/btu170
Bonnefont, C. M. D., Toufeer, M., Caubet, C., Foulon, E., Tasca, C., Aurel, M. R., et al. (2011). Transcriptomic analysis of milk somatic cells in mastitis resistant and susceptible sheep upon challenge with Staphylococcus epidermidis and Staphylococcus aureus. BMC Genomics 12:208. doi: 10.1186/1471-2164-12-208
Botía, J. A., Vandrovcova, J., Forabosco, P., Guelfi, S., D’Sa, K., Hardy, J., et al. (2017). An additional k-means clustering step improves the biological features of WGCNA gene co-expression networks. BMC Syst. Biol. 11:47. doi: 10.1186/s12918-017-0420-6
Brand, B., Hartmann, A., Repsilber, D., Griesbeck-Zilch, B., Wellnitz, O., Kühn, C., et al. (2011). Comparative expression profiling of E. coli and S. aureus inoculated primary mammary gland cells sampled from cows with different genetic predispositions for somatic cell score. Genet. Sel. Evol. 43:24. doi: 10.1186/1297-9686-43-24
Buitenhuis, B., Røntved, C. M., Edwards, S. M., Ingvartsen, K. L., and Sørensen, P. (2011). In depth analysis of genes and pathways of the mammary gland involved in the pathogenesis of bovine Escherichia coli-mastitis. BMC Genomics 12:130. doi: 10.1186/1471-2164-12-130
Chang, S. J., Chen, Y. C., Yang, C. H., Huang, S. C., Huang, H. K., Li, C. C., et al. (2017). Revealing the three dimensional architecture of focal adhesion components to explain Ca2+-mediated turnover of focal adhesions. Biochim. Biophys. Acta 1861, 624–635. doi: 10.1016/j.bbagen.2017.01.002
Chen, E. Y., Tan, C. M., Kou, Y., Duan, Q., Wang, Z., Meirelles, G. V., et al. (2013). Enrichr: interactive and collaborative HTML5 gene list enrichment analysis tool. BMC Bioinformatics 14:128. doi: 10.1186/1471-2105-14-128
Chin, C. H., Chen, S. H., Wu, H. H., Ho, C. W., Ko, M. T., and Lin, C. Y. (2014). cytoHubba: identifying hub objects and sub-networks from complex interactome. BMC Syst. Biol. 8:S11. doi: 10.1186/1752-0509-8-S4-S11
Cobanoglu, O., Zaitoun, I., Chang, Y. M., Shook, G. E., and Khatib, H. (2006). Effects of the signal transducer and activator of transcription 1 (STAT1) gene on milk production traits in Holstein dairy cattle. J. Dairy Sci. 89, 4433–4437. doi: 10.3168/jds.S0022-0302(06)72491-2
Cohen, E. N., Gao, H., Anfossi, S., Mego, M., Reddy, N. G., Debeb, B., et al. (2015). Inflammation mediated metastasis: immune induced epithelial-to-mesenchymal transition in inflammatory breast cancer cells. PLoS One 10:e0132710. doi: 10.1371/journal.pone.0132710
Compton, C. W. R., Cursons, R. T. M., Barnett, C. M. E., and McDougall, S. (2009). Expression of innate resistance factors in mammary secretion from periparturient dairy heifers and their association with subsequent infection status. Vet. Immunol. Immunopathol. 127, 357–364. doi: 10.1016/j.vetimm.2008.10.331
De Vliegher, S., Fox, L. K., Piepers, S., McDougall, S., and Barkema, H. W. (2012). Invited review: mastitis in dairy heifers: nature of the disease, potential impact, prevention, and control. J. Dairy Sci. 95, 1025–1040. doi: 10.3168/jds.2010-4074
Dinarello, C. A. (2018). Overview of the IL-1 family in innate inflammation and acquired immunity. Immunol. Rev. 281, 8–27. doi: 10.1111/imr.12621
Donaldson, L., Vuocolo, T., Gray, C., Strandberg, Y., Reverter, A., McWilliam, S., et al. (2005). Construction and validation of a bovine innate immune microarray. BMC Genomics 6:135. doi: 10.1186/1471-2164-6-135
Filipe, J., Bronzo, V., Curone, G., Castiglioni, B., Vigo, D., Smith, B., et al. (2019). Staphylococcus aureus intra-mammary infection affects the expression pattern of IL-R8 in goat. Comp. Immunol. Microbiol. Infect. Dis. 66:101339. doi: 10.1016/j.cimid.2019.101339
Franchini, M., Schweizer, M., Mätzener, P., Magkouras, I., Sauter, K. S., Mirkovitch, J., et al. (2006). Evidence for dissociation of TLR mRNA expression and TLR agonist-mediated functions in bovine macrophages. Vet. Immunol. Immunopathol. 110, 37–49. doi: 10.1016/j.vetimm.2005.09.002
Galvão, K. N., Felippe, M. J. B., Brittin, S. B., Sper, R., Fraga, M., Galvão, J. S., et al. (2012). Evaluation of cytokine expression by blood monocytes of lactating Holstein cows with or without postpartum uterine disease. Theriogenology 77, 356–372. doi: 10.1016/j.theriogenology.2011.08.008
Genini, S., Badaoui, B., Sclep, G., Bishop, S. C., Waddington, D., Pinard van der Laan, M. H., et al. (2011). Strengthening insights into host responses to mastitis infection in ruminants by combining heterogeneous microarray data sources. BMC Genomics 12:225. doi: 10.1186/1471-2164-12-225
Getter, T., Margalit, R., Kahremany, S., Levy, L., Blum, E., Khazanov, N., et al. (2019). Novel inhibitors of leukocyte transendothelial migration. Bioorg. Chem. 92:103250. doi: 10.1016/j.bioorg.2019.103250
Groot, M. J., and van’t Hooft, K. E. (2016). The hidden effects of dairy farming on public and environmental health in the Netherlands, India, Ethiopia, and Uganda, Considering the Use of Antibiotics and Other Agro-chemicals. Front. Public Heal. 4:12 doi: 10.3389/fpubh.2016.00012
Han, H. (2019). Identification of several key genes by microarray data analysis of bovine mammary gland epithelial cells challenged with Escherichia coli and Staphylococcus aureus. Gene 683, 123–132. doi: 10.1016/j.gene.2018.10.004
Haricharan, S., and Li, Y. (2014). STAT signaling in mammary gland differentiation, cell survival and tumorigenesis. Mol. Cell. Endocrinol. 382, 560–569. doi: 10.1016/j.mce.2013.03.014
Heikkilä, A. M., Nousiainen, J. I., and Pyörälä, S. (2012). Costs of clinical mastitis with special reference to premature culling. J. Dairy Sci. 95, 139–150. doi: 10.3168/jds.2011-4321
Heinrichs, A. J., Costello, S. S., and Jones, C. M. (2009). Control of heifer mastitis by nutrition. Vet. Microbiol. 134, 172–176. doi: 10.1016/j.vetmic.2008.09.025
Henneke, P., Morath, S., Uematsu, S., Weichert, S., Pfitzenmaier, M., Takeuchi, O., et al. (2005). Role of Lipoteichoic Acid in the Phagocyte Response to Group B Streptococcus. J. Immunol. 174, 6449–6455. doi: 10.4049/jimmunol.174.10.6449
Heyninck, K., Lahtela-Kakkonen, M., Van Der Veken, P., Haegeman, G., and Vanden Berghe, W. (2014). Withaferin A inhibits NF-kappaB activation by targeting cysteine 179 in IKKβ. Biochem. Pharmacol. 91, 501–509. doi: 10.1016/j.bcp.2014.08.004
Hillerton, J. E., and Berry, E. A. (2003). The management and treatment of environmental streptococcal mastitis. Vet. Clin. North Am. Food Anim. Pract. 19, 157–169. doi: 10.1016/S0749-0720(02)00069-5
Hogan, J. S., Smith, K. L., Hoblet, K. H., Schoenberger, P. S., Todhunter, D. A., Hueston, W. D., et al. (1989). Field survey of clinical mastitis in low somatic cell count herds. J. Dairy Sci. 72, 1547–1556. doi: 10.3168/jds.S0022-0302(89)79266-3
Hortet, P., and Seegers, H. (1998). Loss in milk yield and related composition changes resulting from clinical mastitis in dairy cows. Prev. Vet. Med. 37, 1–20. doi: 10.1016/S0167-5877(98)00104-4
Hughes, K., and Wood, P. (2017). Is STAT3 a future therapeutic target in ovine and bovine mastitis? Proceedings of the 2017 British Mastitis Conference, Worcester.
Jin, G. H., Xu, W., Shi, Y., and Wang, L. B. (2016). Celecoxib exhibits an anti-gastric cancer effect by targeting focal adhesion and leukocyte transendothelial migration-associated genes. Oncol. Lett. 12, 2345–2350. doi: 10.3892/ol.2016.4976
Kim, S. D., Lee, Y. J., Baik, J. S., Han, J. Y., Lee, C. G., Heo, K., et al. (2014). Baicalein inhibits agonist- and tumor cell-induced platelet aggregation while suppressing pulmonary tumor metastasis via cAMP-mediated VASP phosphorylation along with impaired MAPKs and PI3K-Akt activation. Biochem. Pharmacol. 92, 251–265. doi: 10.1016/j.bcp.2014.09.019
Kleczkowski, M., Kluciński, W., Czerski, M., and Kudyba, E. (2017). Association between acute phase response, oxidative status and mastitis in cows. Vet. Stanica 48, 177–186.
Kosciuczuk, E. M., Lisowski Pawełand Jarczak, J., Majewska, A., Rzewuska, M., Zwierzchowski, L., and Bagnicka, E. (2017). Transcriptome profiling of Staphylococci-infected cow mammary gland parenchyma. BMC Vet. Res. 13:161. doi: 10.1186/s12917-017-1088-2
Kumar, N., Manimaran, A., Kumaresan, A., Jeyakumar, S., Sreela, L., Mooventhan, P., et al. (2017). Mastitis effects on reproductive performance in dairy cattle: a review. Trop. Anim. Health Prod. 49, 663–673. doi: 10.1007/s11250-017-1253-4
Langfelder, P., and Horvath, S. (2008). WGCNA: an R package for weighted correlation network analysis. BMC Bioinformatics 9:559. doi: 10.1186/1471-2105-9-559
Langfelder, P., Luo, R., Oldham, M. C., and Horvath, S. (2011). Is my network module preserved and reproducible? PLoS Comput. Biol. 7:e1001057. doi: 10.1371/journal.pcbi.1001057
Lawless, N., Reinhardt, T. A., Bryan, K., Baker, M., Pesch, B., Zimmerman, D., et al. (2014). Microrna regulation of bovine monocyte inflammatory and metabolic networks in an in Vivo infection model. G3 4, 957–971. doi: 10.1534/g3.113.009936
Leigh, J. A., Field, T. R., and Williams, M. R. (1990). Two strains of Streptococcus uberis, of differing ability to cause clinical mastitis, differ in their ability to resist some host defence factors. Res. Vet. Sci. 49, 85–87. doi: 10.1016/s0034-5288(18)31052-x
Levine, J. D., and Alessandri-Haber, N. (2007). TRP channels: targets for the relief of pain. Biochim. Biophys. Acta 1772, 989–1003. doi: 10.1016/j.bbadis.2007.01.008
Li, Z. F., Xu, W. W., Li, J. D., Tao, F. L., Chen, J. X., and Xu, J. H. (2019). Nucleotide exchange factor SIL1 promotes the progress of breast cancer cells via regulating the cell cycle and apoptosis. Sci. Prog. 103:36850419891046. doi: 10.1177/0036850419891046
Liang, D., Li, F., Fu, Y., Cao, Y., Song, X., Wang, T., et al. (2014). Thymol inhibits LPS-stimulated inflammatory response via down-regulation of NF-κB and MAPK signaling pathways in mouse mammary epithelial cells. Inflammation 37, 214–222. doi: 10.1007/s10753-013-9732-x
Loor, J. J., and Kasey, M. M. Massimo Bionaz. (2011). Functional adaptations of the transcriptome to mastitis-causing pathogens: the mammary gland and beyond. J. Mammary Gland Biol. Neoplasia 16, 305–322. doi: 10.1007/s10911-011-9232-2
Luoreng, Z. M., Wang, X. P., Mei, C. G., and Zan, L. S. (2018). Expression profiling of peripheral blood miRNA using RNAseq technology in dairy cows with Escherichia coli-induced mastitis. Sci. Rep. 8:12693. doi: 10.1038/s41598-018-30518-2
Lutzow, Y. C. S., Donaldson, L., Gray, C. P., Vuocolo, T., Pearson, R. D., Reverter, A., et al. (2008). Identification of immune genes and proteins involved in the response of bovine mammary tissue to Staphylococcus aureus infection. BMC Vet. Res. 4:18. doi: 10.1186/1746-6148-4-18
Marchitelli, C., Signorelli, F., Napolitano, F., Buttazzoni, L., Grelet, C., Vanlierde, A., et al. (2017). “Expression profiles of immune genes in milk somatic cells and MIR predicted mineral contents in milk as indicators of mastitis,” in Proceedings of the 36th International Society for Animal Genetics Conference, Dublin.
Medina, E. (2009). Neutrophil extracellular traps: a strategic tactic to defeat pathogens with potential consequences for the host. J. Innate Immun. 1, 176–180. doi: 10.1159/000203699
Mitterhuemer, S., Petzl, W., Krebs, S., Mehne, D., Klanner, A., Wolf, E., et al. (2010). Escherichia coli infection induces distinct local and systemic transcriptome responses in the mammary gland. BMC Genomics 11:138. doi: 10.1186/1471-2164-11-138
Moyes, K. M., Drackley, J. K., Morin, D. E., Bionaz, M., Rodriguez-Zas, S. L., Everts, R. E., et al. (2009). Gene network and pathway analysis of bovine mammary tissue challenged with Streptococcus uberis reveals induction of cell proliferation and inhibition of PPAR signaling as potential mechanism for the negative relationships between immune response and lipi. BMC Genomics 10:542. doi: 10.1186/1471-2164-10-542
Munro, G. L., Grieve, P. A., and Kitchen, B. J. (1984). Effects of mastitis on milk yield, milk composition, processing properties and yield and quality of milk products. Aust. J. Dairy Technol. 39, 7–16.
Neave, F. K., Dodd, F. H., Kingwill, R. G., and Westgarth, D. R. (1969). Control of mastitis in the dairy herd by hygiene and management. J. Dairy Sci. 52, 696–707. doi: 10.3168/jds.S0022-0302(69)86632-4
Nicholas, R. S., Partridge, J., Donn, R. P., Hawkins, C., and Boggild, M. D. (2003). The role of the PTPRC (CD45) mutation in the development of multiple sclerosis in the North West region of the United Kingdom. J. Neurol. Neurosurg. Psychiatry 74, 944–945. doi: 10.1136/jnnp.74.7.944
Nie, Q., Sandford, E. E., Zhang, X., Nolan, L. K., and Lamont, S. J. (2012). Deep sequencing-based transcriptome analysis of chicken spleen in response to Avian Pathogenic Escherichia coli (APEC) infection. PLoS One 7: e41645. doi: 10.1371/journal.pone.0041645
Nielen, M., Spigt, M. H., Schukken, Y. H., Deluyker, H. A., Maatje, K., and Brand, A. (1995). Application of a neural network to analyse on-line milking parlour data for the detection of clinical mastitis in dairy cows. Prev. Vet. Med. 22, 15–28. doi: 10.1016/0167-5877(94)00405-8
Paquette, S. G., Banner, D., Huang, S. S. H., Almansa, R., Leon, A., Xu, L., et al. (2015). Influenza transmission in the mother-infant dyad leads to severe disease, mammary gland infection, and pathogenesis by regulating host responses. PLoS Pathog. 11:e1005173. doi: 10.1371/journal.ppat.1005173
Peters, M. D. P., Silveira, I. D. B., and Fischer, V. (2015). Impact of subclinical and clinical mastitis on sensitivity to pain of dairy cows. Animal 9, 2024–2028. doi: 10.1017/S1751731115001391
Philp, J. A. C., Burdon, T. G., and Watson, C. J. (1996). Differential activation of STATs 3 and 5 during mammary gland development. FEBS Lett. 396, 77–80. doi: 10.1016/0014-5793(96)01069-1
Phuektes, P., Mansell, P. D., Dyson, R. S., Hopper, N. D., Dick, J. S., and Browning, G. F. (2001). Molecular epidemiology of Streptococcus uberis isolates from dairy cows with mastitis. J. Clin. Microbiol. 39, 1460–1466. doi: 10.1128/JCM.39.4.1460-1466.2001
Pihur, V., Datta, S., and Datta, S. (2009). RankAggreg, an R package for weighted rank aggregation. BMC Bioinformatics 10:62. doi: 10.1186/1471-2105-10-62
Polgar, N., Csongei, V., Szabo, M., Zambo, V., Melegh, B. I., Sumegi, K., et al. (2012). Investigation of JAK2, STAT3 and CCR6 polymorphisms and their gene-gene interactions in inflammatory bowel disease. Int. J. Immunogenet. 39, 247–252. doi: 10.1111/j.1744-313X.2012.01084.x
Rainard, P., Riollet, C., Berthon, P., Cunha, P., Fromageau, A., Rossignol, C., et al. (2008). The chemokine CXCL3 is responsible for the constitutive chemotactic activity of bovine milk for neutrophils. Mol. Immunol. 45, 4020–4027. doi: 10.1016/j.molimm.2008.06.010
Rana, H. K., Akhtar, M. R., Islam, M. B., Ahmed, M. B., Liò, P., Quinn, J. M. W., et al. (2019). Genetic effects of welding fumes on the development of respiratory system diseases. Comput. Biol. Med. 108, 142–149. doi: 10.1016/j.compbiomed.2019.04.004
Reinoso, E. B., Lasagno, M. C., Dieser, S. A., and Odierno, L. M. (2011). Distribution of virulence-associated genes in Streptococcus uberis isolated from bovine mastitis. FEMS Microbiol. Lett. 318, 183–188. doi: 10.1111/j.1574-6968.2011.02258.x
Rinaldi, M., Li, R. W., Bannerman, D. D., Daniels, K. M., Evock-Clover, C., Silva, M. V. B., et al. (2010). A sentinel function for teat tissues in dairy cows: dominant innate immune response elements define early response to E. coli mastitis. Funct. Integr. Genomics 10, 21–38. doi: 10.1007/s10142-009-0133-z
Rivas, A. L., Tadevosyan, R., Quimby, F. W., and Lein, D. H. (2002). Blood and milk cellular immune responses of mastitic non-periparturient cows inoculated with Staphylococcus aureus. Can. J. Vet. Res. 66, 125–131.
Rupp, R., Senin, P., Sarry, J., Allain, C., Tasca, C., Ligat, L., et al. (2015). A point mutation in suppressor of cytokine signalling 2 (Socs2) increases the susceptibility to inflammation of the mammary gland while associated with higher body weight and size and higher milk production in a sheep model. PLoS Genet. 11:e1005629. doi: 10.1371/journal.pgen.1005629
Saito, R., Smoot, M. E., Ono, K., Ruscheinski, J., Wang, P. L., Lotia, S., et al. (2012). A travel guide to Cytoscape plugins. Nat. Methods 9, 1069–1076. doi: 10.1038/nmeth.2212
Schukken, Y. H., Hertl, J., Bar, D., Bennett, G. J., González, R. N., Rauch, B. J., et al. (2009). Effects of repeated gram-positive and gram-negative clinical mastitis episodes on milk yield loss in Holstein dairy cows. J. Dairy Sci. 92, 3091–3105. doi: 10.3168/jds.2008-1557
Schulman, N. F., Sahana, G., Iso-Touru, T., Lund, M. S., Andersson-Eklund, L., Viitala, S. M., et al. (2009). Fine mapping of quantitative trait loci for mastitis resistance on bovine chromosome 11. Anim. Genet. 40, 509–515. doi: 10.1111/j.1365-2052.2009.01872.x
Shah, K. N., Valand, P., Nauriyal, D. S., and Joshi, C. G. (2018). Immunomodulation of IL-1, IL-6 and IL-8 cytokines by Prosopis juliflora alkaloids during bovine sub-clinical mastitis. 3 Biotech 8:409. doi: 10.1007/s13205-018-1438-1
Sharifi, S., Pakdel, A., Ebrahimie, E., Aryan, Y., Ghaderi Zefrehee, M., and Reecy, J. M. (2019). Prediction of key regulators and downstream targets of E. coli induced mastitis. J. Appl. Genet. 60, 367–373. doi: 10.1007/s13353-019-00499-7
Sharmila, C., Williams, J. W., and Reddy, P. G. (2002). Effect of caprine arthritis-encephalitis virus infection on expression of interleukin-16 in goats. Am. J. Vet. Res. 63, 1418–1422. doi: 10.2460/ajvr.2002.63.1418
Smita, S., Katiyar, A., Pandey, D. M., Chinnusamy, V., Archak, S., and Bansal, K. C. (2013). Identification of conserved drought stress responsive gene-network across tissues and developmental stages in rice. Bioinformation 9, 72–78. doi: 10.6026/97320630009072
Smyth, G. K. (2005). Limma: Linear Models for Microarray Data. In Bioinformatics and Computational Biology Solutions Using R and Bioconductor. Available online at: http://citeseerx.ist.psu.edu/viewdoc/summary?doi=10.1.1.363.443 (accessed March 7, 2020).
Song, L., Langfelder, P., and Horvath, S. (2012). Comparison of co-expression measures: mutual information, correlation, and model based indices. BMC Bioinformatics 13:328. doi: 10.1186/1471-2105-13-328
Szklarczyk, D., Gable, A. L., Lyon, D., Junge, A., Wyder, S., Huerta-Cepas, J., et al. (2019). STRING v11: protein-protein association networks with increased coverage, supporting functional discovery in genome-wide experimental datasets. Nucleic Acids Res. 47, D607–D613. doi: 10.1093/nar/gky1131
Taraktsoglou, M., Szalabska, U., Magee, D. A., Browne, J. A., Sweeney, T., Gormley, E., et al. (2011). Transcriptional profiling of immune genes in bovine monocyte-derived macrophages exposed to bacterial antigens. Vet. Immunol. Immunopathol. 140, 130–139. doi: 10.1016/j.vetimm.2010.12.002
Trapnell, C., Pachter, L., and Salzberg, S. L. (2009). TopHat: discovering splice junctions with RNA-Seq. Bioinformatics 25, 1105–1111. doi: 10.1093/bioinformatics/btp120
Turner, M., and Billadeau, D. D. (2002). VAV proteins as signal integrators for multi-subunit immune-recognition receptors. Nat. Rev. Immunol. 2, 476–486. doi: 10.1038/nri840
Twigger, A. J., Küffer, G. K., Geddes, D. T., and Filgueria, L. (2018). Expression of granulisyn, perforin and granzymes in human milk over lactation and in the case of maternal infection. Nutrients 10:1230. doi: 10.3390/nu10091230
Wang, K., Jin, X. L., Shen, X. G., Sun, L. P., Wu, L. M., Wei, J. Q., et al. (2016). Effects of Chinese propolis in protecting bovine mammary epithelial cells against mastitis pathogens-induced cell damage. Mediators Inflamm. 2016:8028291. doi: 10.1155/2016/8028291
Wang, X. G., Ju, Z. H., Hou, M. H., Jiang, Q., Yang, C. H., Zhang, Y., et al. (2016). Deciphering transcriptome and complex alternative splicing transcripts in mammary gland tissues from cows naturally infected with Staphylococcus aureus mastitis. PLoS One 11:e0159719. doi: 10.1371/journal.pone.0159719
Ward, P. N., Holden, M. T. G., Leigh, J. A., Lennard, N., Bignell, A., Barron, A., et al. (2009). Evidence for niche adaptation in the genome of the bovine pathogen Streptococcus uberis. BMC Genomics 10:54. doi: 10.1186/1471-2164-10-54
Wellnitz, O., and Bruckmaier, R. M. (2012). The innate immune response of the bovine mammary gland to bacterial infection. Vet. J. 192, 148–152. doi: 10.1016/j.tvjl.2011.09.013
Wellnitz, O., Reith, P., Haas, S. C., and Meyer, H. H. D. (2006). Immune relevant gene expression of mammary epithelial cells and their influence on leukocyte chemotaxis in response to different mastitis pathogens. Vet. Med. 51, 125–132. doi: 10.17221/5531-VETMED
Williamson, J. H., Woolford, M. W., and Day, A. M. (1995). The prophylactic effect of a dry-cow antibiotic against Streptococcus uberis. N. Z. Vet. J. 43, 228–234. doi: 10.1080/00480169.1995.35898
Wu, D., Zhang, X., Liu, L., and Guo, Y. (2019). Key CMM combinations in prescriptions for treating mastitis and working mechanism analysis based on network pharmacology. Evid. Based Complement. Altern. Med. 2019:8245071. doi: 10.1155/2019/8245071
Wu, H., Zhao, G., Jiang, K., Chen, X., Zhu, Z., Qiu, C., et al. (2016). Puerarin exerts an antiinflammatory effect by inhibiting NF-kB and MAPK activation in Staphylococcus aureus-induced mastitis. Phyther. Res. 1658–1664. doi: 10.1002/ptr.5666
Wu, J., Li, L., Sun, Y., Huang, S., Tang, J., Yu, P., et al. (2015). Altered molecular expression of the TLR4/NF-κB signaling pathway in mammary tissue of Chinese Holstein cattle with mastitis. PLoS One 10:e0118458. doi: 10.1371/journal.pone.0118458
Xu, D.-D., Wang, G., He, X.-J., Wang, J.-F., Yang, B., Sun, Z.-P., et al. (2017). 17β-Estradiol and progesterone decrease MDP induced NOD2 expression in bovine mammary epithelial cells. Vet. Immunol. Immunopathol. 188, 59–64. doi: 10.1016/j.vetimm.2017.04.010
Yang, B., Jiao, B., Ge, W., Zhang, X., Wang, S., Zhao, H., et al. (2018). Transcriptome sequencing to detect the potential role of long non-coding RNAs in bovine mammary gland during the dry and lactation period. BMC Genomics 19:605. doi: 10.1186/s12864-018-4974-5
Zhao, X., and Lacasse, P. (2008). Mammary tissue damage during bovine mastitis: causes and control. J. Anim. Sci. 86, 57–65. doi: 10.2527/jas.2007-0302
Keywords: weighted gene co-expression network, protein-protein interaction, RNA-seq, hub genes, bovine
Citation: Bakhtiarizadeh MR, Mirzaei S, Norouzi M, Sheybani N and Vafaei Sadi MS (2020) Identification of Gene Modules and Hub Genes Involved in Mastitis Development Using a Systems Biology Approach. Front. Genet. 11:722. doi: 10.3389/fgene.2020.00722
Received: 12 March 2020; Accepted: 15 June 2020;
Published: 13 July 2020.
Edited by:
James J. Cai, Texas A&M University, United StatesReviewed by:
Mao Yongjiang, Yangzhou University, ChinaAlessandro Giuliani, Istituto Superiore di Sanità (ISS), Italy
Ying Yu, China Agricultural University, China
Copyright © 2020 Bakhtiarizadeh, Mirzaei, Norouzi, Sheybani and Vafaei Sadi. This is an open-access article distributed under the terms of the Creative Commons Attribution License (CC BY). The use, distribution or reproduction in other forums is permitted, provided the original author(s) and the copyright owner(s) are credited and that the original publication in this journal is cited, in accordance with accepted academic practice. No use, distribution or reproduction is permitted which does not comply with these terms.
*Correspondence: Mohammad Reza Bakhtiarizadeh, mrbakhtiari@ut.ac.ir; mrb20045@gmail.com
†ORCID: Mohammad Reza Bakhtiarizadeh, orcid.org/0000-0001-5336-6987