- Wageningen University & Research Animal Breeding and Genomics, Wageningen, Netherlands
Resilience is the capacity of an animal to be minimally affected by disturbances or to rapidly return to the state pertained before exposure to a disturbance. Less resilient animals are expected to be more susceptible to environmental perturbations, such as diseases, and will consequently show more and/or greater fluctuations in production than more resilient animals. Natural antibodies (NAb) are antibodies recognizing antigens without previous exposure to these, and are hypothesized to be an indication of general disease resistance. The objective of this research was to investigate genetic parameters of resilience indicators based on standardized body weight (BW) deviations and to investigate its relation with immunity (i.e. NAb) and disease resistance. Keyhole limpet hemocyanin-binding NAb were measured in layer chickens, which were selectively bred for high and low keyhole limpet hemocyanin-binding NAb levels during six generations. In addition, BW data of these layers were collected on a four-weekly interval from 4 weeks of age until 32 weeks of age. Standardized deviations of BW from an individual were compared to lines’ average BW (i.e. across individuals), and these were used to calculate resilience indicators: natural logarithm-transformed variance [ln(variance)], skewness, and lag-one autocorrelation of deviations (i.e. all within an individual). Heritabilities of resilience indicators were between 0.09 and 0.11. Genetic correlations between the three resilience indicators were between -0.20 and 0.40 (with high SE), which might suggest that the resilience indicators capture different aspects of resilience. Genetic correlations between resilience indicators and NAb were close to zero, which suggests that the resilience indicators and NAb capture different aspects of immunity. This might indicate that, in this dataset, environmental perturbations are only to a small extent affected by disease incidence, possibly due to a lack of disease occurrence. However, a lower estimated breeding value for ln(variance) was predictive for lower lesion scores after an avian pathogenic Escherichia coli inoculation and vice versa. In conclusion, this study shows that there is genetic variation in resilience indicators based on BW deviations in layer chickens, which opens up possibilities to improve resilience by means of selective breeding.
Introduction
Resilience (or in other work referred to as robustness) is the capacity of an animal to be minimally affected by disturbances or to rapidly return to the state pertained before exposure to a disturbance (Colditz and Hine, 2016; Berghof et al., 2019b). Resilient livestock are essential for future livestock production as resilient livestock are easy-to-manage and require less individual treatment (Elgersma et al., 2018), which results in a greater production without negative effects on animals and farmers (Berghof et al., 2019b). Resilience can be of different nature based on the disturbance (e.g. disease, heat stress) (Colditz and Hine, 2016). Thus, “general” resilience is a composite trait consisting of different resilience types, such as disease-resilience and heat stress-resilience (Colditz and Hine, 2016; Friggens et al., 2017; Berghof et al., 2019b).
Improving general resilience on livestock animals can be achieved via different complementary strategies, of which one is breeding. In a recent paper, Berghof et al. (2019b) showed that economic values for resilience can be derived based on reduced labor and health costs. Scenarios for pig and dairy cattle breeding schemes showed a greater selection response when information on resilience was used in selection (Berghof et al., 2019b). These simulations suggest that resilience should be included in total merit indices of livestock. However, these simulations assumed the availability of suitable indicators for resilience, which is not the case in practice.
Resilience indicators for individual animals have been proposed based on longitudinal data by calculating deviations of observed from expected production (Knap, 2009; Doeschl-Wilson et al., 2012; Colditz and Hine, 2016; Friggens et al., 2017). Deviations and their patterns in production traits have been shown before as indicative for health-related traits, although these approaches use only relatively-large, short-time deviations (e.g. De Haas et al., 2004; Codrea et al., 2011; Fischer et al., 2018; Nguyen-Ba et al., 2019; Revilla et al., 2019; Byrd et al., 2019). Berghof et al. (2019b), however, used a different approach to define three resilience indicators based on all production deviations due to unknown disturbances during a production cycle using concepts to study resilience of ecosystems (Scheffer et al., 2009; Scheffer et al., 2015; Scheffer et al., 2018): natural logarithm-transformed variance [ln(variance)] of deviations, skewness of deviations, and autocorrelation of deviations (Berghof et al., 2019b). These resilience indicators were proposed to have potential, because they are easy to obtain in livestock production data, cover the whole production period, and are expected to represent different aspects of resilience (Berghof et al., 2019b). More resilient animals are expected to show few(er) and smaller deviations compared to less resilient animals, because they are less influenced by disturbances. This is assumed to be independent of the nature and degree of the disturbances (i.e. no re-ranking), which are often unknown and never completely constant anyway. Figure 1 illustrates a more resilient sire-family and a less resilient sire-family based on the sire's estimated breeding values (EBV) for ln(variance) of body weight (BW) deviations. More resilient animals are expected to have a smaller ln(variance) (i.e. closer to zero), and a skewness and an autocorrelation around zero compared to the population average (Berghof et al., 2019b).
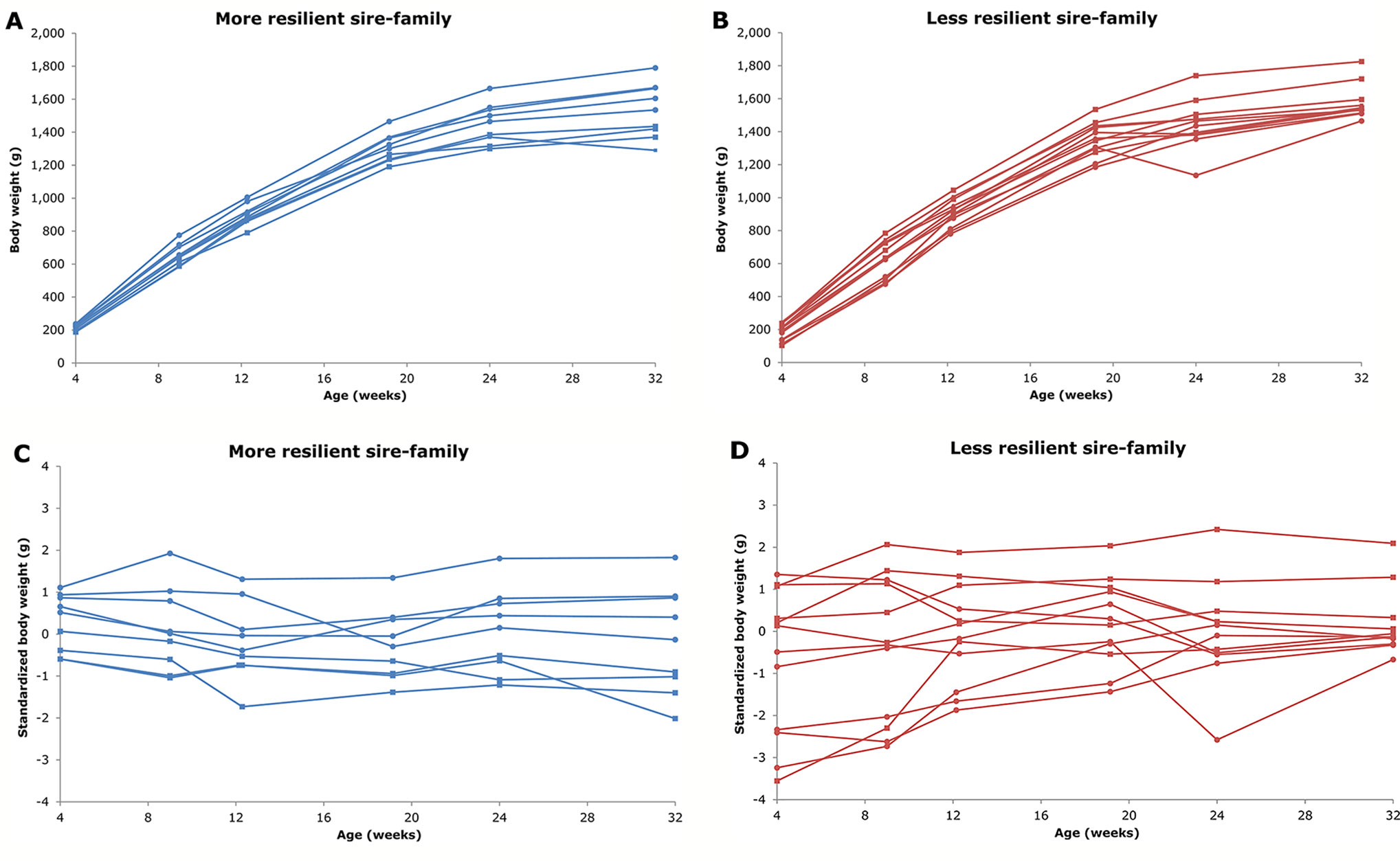
Figure 1 Examples of a more resilient sire-family (A and C; in blue) and a less resilient sire-family (B and D; in red) based on the sire's estimated breeding values for natural logarithm-transformed variance [ln(variance)]. A and B show body weights (BW) over time. C and D show standardized BW over time. C and D illustrate the difference in ln(variance) most easily: a more horizontal standardized BW line over time indicates a lower ln(variance) and thus a greater (hypothesized) resilience, and vice versa. The sire-families were obtained by mating the sire with two dams. The two families within the sire-family are indicated with different markers (round or square). Only BW of offspring with estimates for the resilience indicators (5 or more BW observations) are shown. The sire-families in this figure are families of generation 5 of the high line of the natural antibody (NAb)-selection experiment (i.e. the sires were generation 4).
Three studies have investigated the genetic potential of the variance of deviations of longitudinal data as an indicator for resilience in livestock and found favorable relations with health: Elgersma et al. (2018) and Poppe et al. (2020) found that fluctuations in daily milk yield in dairy cows are heritable, and are favorably and negatively genetically correlated to health and longevity traits (Elgersma et al., 2018; Poppe et al., 2020). Putz et al. (2019) found that fluctuations in daily feed intake and daily duration at the feeder in pigs are heritable, and favorably and negatively genetically correlated to mortality and treatment rate (Putz et al., 2019). In addition, many studies found heritable variation in uniformity in livestock species, which is the same as genetic heterogeneity of residual variance (see Hill and Mulder, 2010 and Elgersma et al., 2018 for overviews; e.g. Rowe et al., 2006; Mulder et al., 2007; Mulder et al., 2008; Ibáñez-Escriche et al., 2008a; Ibáñez-Escriche et al., 2008b; Mulder et al., 2009; Wolc et al., 2009; Neves et al., 2011; Wolc et al., 2012; Janhunen et al., 2012; Sae-Lim et al., 2015; Mulder et al., 2016; Sae-Lim et al., 2016; Sae-Lim et al., 2017). Thus, variance of production traits is heritable and seems to indicate resilience, however skewness and autocorrelation of production traits have not previously been investigated as potential indicators for resilience in livestock.
Natural antibodies (NAb) are antigen binding antibodies present in individuals without a (known) previous exposure to this antigen (Baumgarth et al., 2005). Previous studies showed that commercial layer chickens with high NAb levels binding keyhole limpet hemocyanin (KLH) were associated with lower mortality (Star et al., 2007; Sun et al., 2011; Wondmeneh et al., 2015). A NAb-selection experiment divergently selected layer chickens on total keyhole limpet hemocyanin (KLH)-binding NAb levels (IgTotal), resulting in a line with high NAb levels (High line) and a line with low NAb levels (low line) (Berghof, 2018). The High line showed increased antibody response to some antigens (Berghof et al., 2018a). Moreover, the High line had a 3.0 times reduced risk to die during an avian pathogenic Escherichia coli (APEC)-inoculation compared to chickens of the low line. Surviving High line chickens also had lower total lesion scores (i.e. morbidity) compared to surviving low line chickens at the end of the experiment (Berghof et al., 2019a). It was hypothesized that the NAb-selection lines differ in general (bacterial) disease resistance (Berghof, 2018). We hypothesize that the NAb-selection lines also differ in disease resilience and consequentially differ in general resilience (i.e. the resilience indicators).
The objectives of this study were:
1. to investigate the genetic parameters of resilience indicators based on four-weekly standardized BW deviations of layer chickens divergently selected for total KLH-binding NAb levels;
2. to investigate the relationship between resilience indicators and immunity by estimating the (genetic) correlation between resilience indicators and NAb levels; and
3. to investigate the relationship between resilience indicators and disease resistance by investigating the predictive ability of resilience indicators on APEC-resistance.
Given the current developments in the field of sensor technology, it is expected that large amounts of longitudinal data (“big data”) will become available for investigation of resilience based on deviations. This study explores the potential of resilience indicators based on longitudinal data. Moreover, this study is, as far as we know, the first to investigate genetic parameters of skewness and autocorrelation, and the first to investigate a direct relationship between resilience, immunity and disease resistance.
Materials and Methods
Study Populations
The study populations consisted of the high and low NAb-selection lines in the selection experiment (including base population) and the NAb-selection lines in the infection experiments. Figure 2 gives an overview of the study populations.
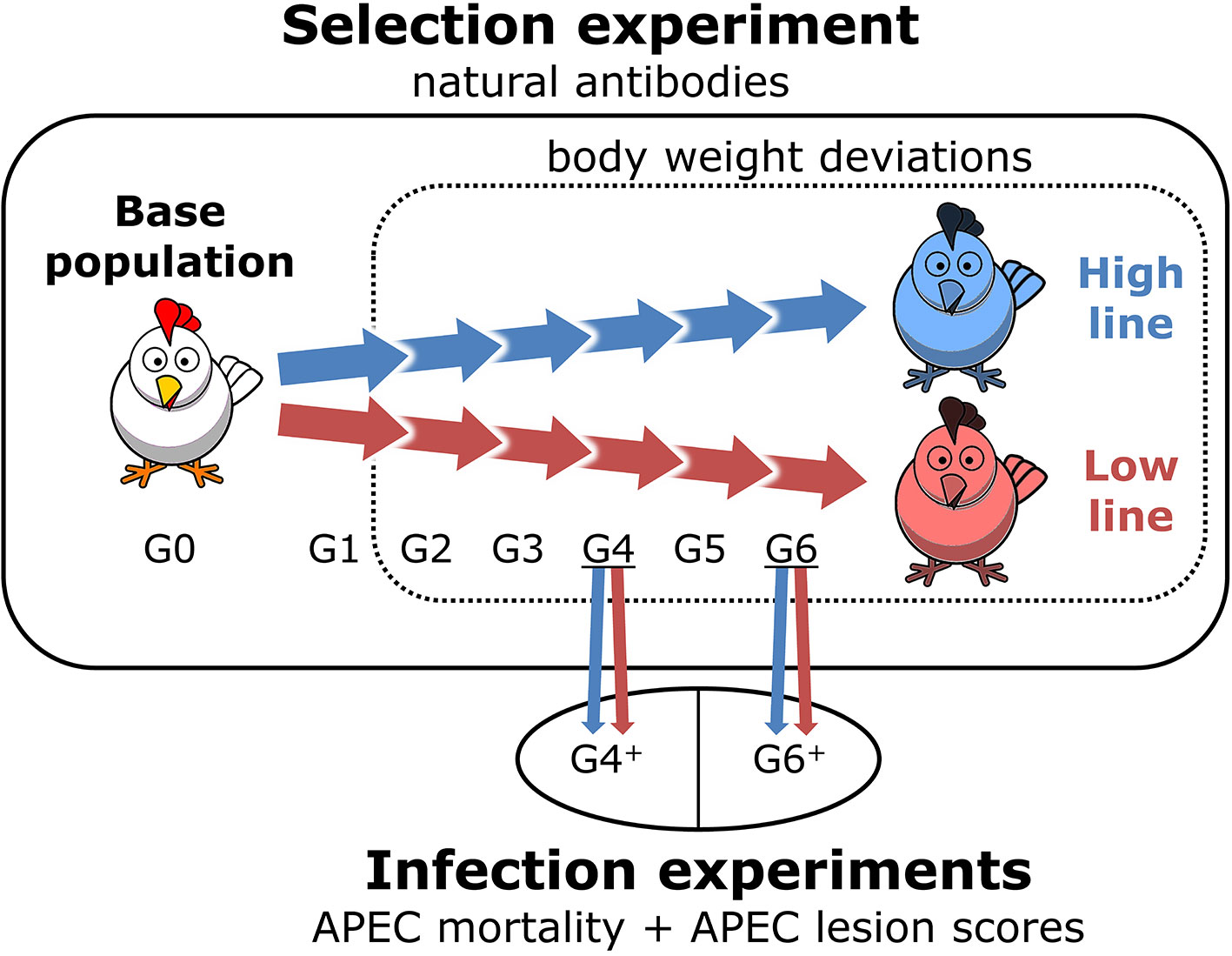
Figure 2 Overview of the study populations. Body weight deviations and natural antibody (NAb) were measured during the selection experiment. Avian pathogenic Escherichia coli (APEC) mortality and APEC lesion scores were measured during the infection experiments. Base population is the unselected population (G0) and the NAb-selection lines are generation (G)1 to G6. High line and Low line are the selected lines for high and low total keyhole limpet hemocyanin-binding NAb titers at 16 weeks of age, respectively. G4+ and G6+ are the additionally bred chickens for the infection experiments and are similar to G4 and G6 of the selection experiment.
Selection Experiment
For objective 1 and objective 2, genetic parameters of resilience indicators based on BW deviations and their relations with NAb were investigated based on data of the NAb-selection experiment. The selection experiment-population consisted of an unselected (i.e. not for NAb selected) base population [generation (G)0] and six generations of NAb-selection lines [generation 1–6 (G1–G6)]. A steady increase in phenotypic differences in NAb and NAb EBV was observed over six generations between the high and low NAb-selection line, see Berghof (2018).
Briefly, the selection process was performed as follows:
● The G0 consisted of almost 3,700 purebred White Leghorn chickens (approximately 2,400 females and 1,300 males) from the “WA” line of Hendrix Genetics. Plasma samples of the studied chicken population were collected at 15 weeks of age (males) or 19 weeks of age (females). Selection of the breeding candidates was based on total KLH-binding NAb (IgTotal) titer (see below). For the high and low line, 25 males and 50 females with the highest and lowest titers were selected to breed G1. Each male was mated to 2 females and each female was mated to 1 male (adjusted from Berghof et al., 2018a).
● Incubation of eggs and housing of chickens for the selection lines (from G1 onwards) was at research facility “Carus” from Wageningen University & Research according to standard production practices. Each generation consisted of approximately 600 chickens per line. Plasma samples were collected at 16 weeks of age. Selection of the breeding candidates was based on total KLH-binding NAb (IgTotal) titer (see below).Within a line, the 25 best males and the 50 best females were selected to breed the next generation. Selected males and females were group housed from 0 to 16 weeks of age and individually housed from 16 weeks of age to 32 weeks of age. Non-selected males were culled after 16 weeks of age and non-selected females were group-housed from 0 weeks of age to 32 weeks of age. Chickens were not exchanged between lines. Each male was mated to 2 unrelated females (i.e. not mated with siblings or half-siblings) and each female was mated to 1 unrelated male (i.e. not mated with sibling or half-sibling) (adjusted from Berghof et al., 2018a).
More information about G0 can be found in Van Der Klein et al. (2015), and more information about the NAb-selection experiment can be found in Berghof et al. (2018a).
Infection Experiments
For objective 3, data of infection experiments of the NAb-selection lines were used to investigate the relationship between resilience and disease resistance. An additional G4 and G6 were bred during the NAb-selection experiment, and inoculated with APEC, see Disease Resistance: APEC Mortality and APEC Lesion Scores and Berghof et al. (2019a) for more details.
Resilience Indicators Based on Body Weight Deviations
BW were collected approximately every 4 weeks from 4 weeks of age to approximately 32 weeks of age for hens in the selection experiment, except at 28 weeks of age. Roosters were not included in the dataset, because only roosters selected for breeding the next generation of the selection experiment were kept until approximately 32 weeks of age.
BW records were visually checked for reliability across individuals of their respective cohort of line*generation*weighing moment with the Interactive Data Analysis tool from SAS® software (SAS software V9.3 TS Level 1M2): 4 BW observations of different individuals were considered to be unreliable and were removed from the dataset. For these individuals, BW doubled between time point 1 and time point 2 and was subsequently reduced with more than 25% between time point 2 and time point 3. Time point 2 was then considered to be an unreliable BW observation and was removed.
BW were standardized to an average of zero and a standard deviation of one across individuals of their respective cohort of line*generation*weighing moment to correct for scaling effects during life and known cohort differences in BW (Berghof, 2018) (see Supplementary Table 1 for descriptive statistics of the cohorts). As a consequence, the standardized BW records are equal to standardized deviations. Thus, deviations were calculated as:
where BWyz is BW y of the zth cohort, is the average BW of the zth cohort, and sz is the standard deviation of BW of the zth cohort.
The resilience indicators used in this study are the ln-transformed variance of deviations within an individual, the skewness of deviations within an individual, and the lag-one autocorrelation of deviations within an individual (Berghof et al., 2019b). The variance was ln-transformed with a natural logarithm (ln), because this is the commonly used scale to express the variance (also known as uniformity) in other studies and allows direct comparison of the additive genetic variance between studies (Hill and Mulder, 2010; Sell-Kubiak et al., 2015). The resilience indicators were calculated for each individual with 5 or more BW observations, which resulted in the removal of all individuals in G0 and G1 and removal of G2–G6 individuals without sufficient observations. The dataset contained 4,966 individuals before editing. A total of 3,393 individuals were removed (G0: 2,392 individuals, G1: 458 individuals, and G2–G6: 543 individuals). The final dataset for ln(variance) and skewness consisted of 1,593 individuals. Autocorrelation was only kept in the dataset if an individual had five or more subsequent observations in order to obtain at least 4 pairs of data points to estimate autocorrelation. This resulted in an additional removal of 110 individuals, which resulted in 1,463 individuals in the final dataset for autocorrelation.
The ln(variance) of deviations of the jth individual was calculated as:
where xij is deviation i of the jth individual, is the mean of deviations of the jth individual, and nj is the number of deviation observations of the jth individual.
The skewness of deviations of the jth individual was calculated as:
where nj is the number of deviation observations of the jth individual, xij is deviation i of the jth individual, is the mean of deviations of the jth individual, and is the variance of deviations of the jth individual being calculated as: , where xij is deviation i of the jth individual, is the mean of deviations of the jth individual, and nj is the number of deviation observations of the jth individual.
The autocorrelation of deviations of the jth individual was calculated as:
where nj is the number of pairs of subsequent deviations of the jth individual, xij is deviation i of the jth individual, is the mean of deviations of the jth individual, and x(i+1)j is the subsequent deviation of deviation i of the jth individual.
Table 1 shows an overview of the number of observations for the three resilience indicators per line and generation.
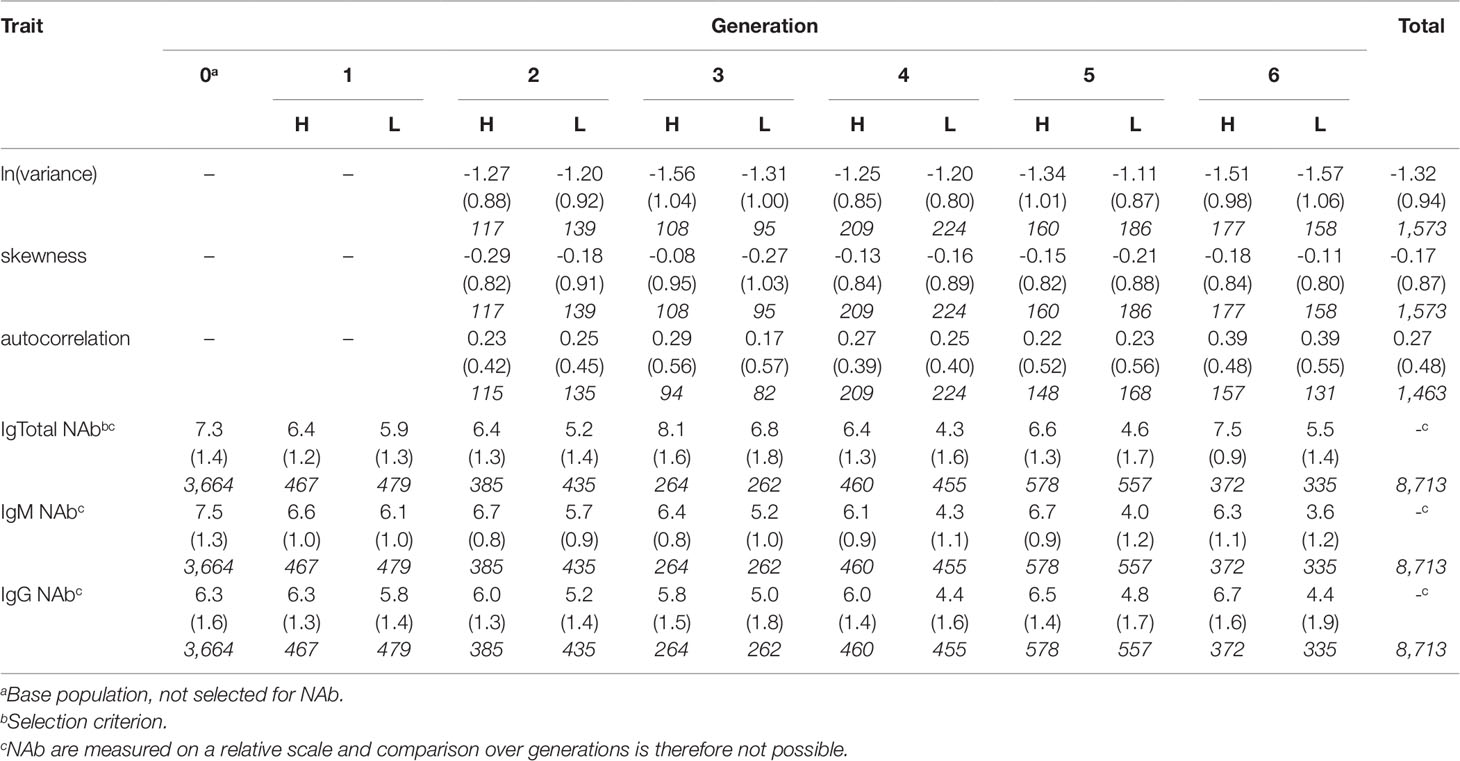
Table 1 Average, standard deviation (in parentheses) and number of observations (in italic) for the three resilience indicators and keyhole-limpet hemocyanin-binding natural antibody (NAb) titers at 16 weeks of age per generation per line (H, high NAb-selection line; L, low NAb-selection line) and total.
Immunity: Natural Antibodies
NAb optical densities (OD) were determined in individual plasma samples by an indirect two-step ELISA and calculated as described by Berghof et al. (2018c).
Briefly, plasma samples were 1:10 pre-diluted with dilution buffer. Flat-bottomed, 96-well medium binding plates were coated with 2 µg/ml KLH in 100 µl coating buffer and incubated at 4°C overnight. After washing for 6 s with tap water containing Tween® 20, plates were tapped dry. The 1:10 pre-dilution of the samples were further diluted with dilution buffer to 1:40, 1:160, 1:640, and 1:2,560 test dilutions. Duplicate standard positive plasma samples were stepwise 1:1 diluted with dilution buffer. The plates were incubated for 1.5 h at room temperature (20–25°C). After washing, plates were incubated with 1:20,000-diluted anti-chicken IgG heavy and light chain (IgTotal) labeled with horse radish peroxidase (PO), or 1:20,000-diluted anti-chicken IgM labeled with PO, or 1:40,000-diluted anti-chicken IgG(Fc) labeled with PO, and incubated for 1.5 h at room temperature (20–25°C). After washing, binding of the antibodies to KLH was visualized by adding 100 µl substrate buffer at room temperature (20–25°C). After 15 min the reaction was stopped with 50 µl of 1.25 M H2SO4. OD were measured at 450 nm [adjusted from Berghof et al. (2018c)].
Antibody titers were calculated as described by Berghof et al. (2018c) (based on Frankena, 1987). Briefly, the OD of the duplicate standard positive plasma samples were averaged for each plate. Logit values of the OD per plate were calculated, and a linear regression line of the logit OD against the respective log2-dilution values of the averaged duplicate standard positive plasma samples was fitted. Titers of the plasma samples per plate were calculated using the linear regression line (adjusted from Berghof et al., 2018c). Table 1 shows an overview of the number of observations for NAb titers per line and generation.
Disease Resistance: Avian Pathogenic Escherichia coli Mortality and Avian Pathogenic Escherichia coli Lesion Scores
Two inoculation experiments were performed with additionally bred G4 and G6 chickens, as described by Berghof et al. (2019a). Care was taken to select one male and one female of each family (where possible) per treatment to have a balanced representation of both NAb-selection lines.
Briefly, at 8 days of age, chickens of both selection lines received an intratracheal inoculation of 0.2 ml phosphate buffered saline containing 108.20 colony-forming units (CFU)/milliliter APEC in the additional G4 (197 individuals in total), or 106.64 CFU/ml APEC in the additional G6 (180 individuals in total) (adjusted from Berghof et al., 2019a). These treatments were selected, because they had the largest variation in mortality (108.20 CFU/ml) and lesion scores (106.64 CFU/ml) (Berghof et al., 2019a, see Berghof et al., 2019a for all treatments).
After inoculation, chickens were checked every 2 h for the first 4 days post inoculation, and subsequently every 8 h until the end of the experiment (approximately 170 h post inoculation). Mortality was scored as the percentage of chickens alive at a certain moment divided by the total number of chickens at the start of the experiment. At 15 days of age (7 days post inoculation), all surviving chickens were euthanized [adjusted from Berghof et al. (2019a)].
Lesion scores (i.e. morbidity) were macroscopically assessed on the surviving chickens at the end of the experiment based on Van Eck and Goren (1991). Lesion scoring was performed on the left thoracic air sac, the right thoracic air sac, the pericardium, and the serosal surface of the liver, always in the same order. Severity of the lesion scores were defined as follows: 0: no lesions, 0.5: one single pinhead-sized inflammatory spot, 1: two or more pinhead-sized spots, 2: fibrinous patches on various locations, and 3: extensive fibrinous patches (Van Eck and Goren, 1991). The total lesion score is the sum of scores for the four individual locations [adjusted from Berghof et al. (2019a)].
Statistical Analyses
Genetic Parameters of Resilience Indicators
Genetic parameters for the resilience indicators based on four-weekly BW deviations of layer chickens were estimated.
The following linear animal model was used for estimating variance components for the resilience indicators:
where yabcj is the resilience indicator ln(variance), skewness, or autocorrelation, µ is the overall mean, nObsa is the fixed effect of the number of observations for the resilience indicator (a = 5–7 for ln(variance) and skewness, and a = 4–6 for autocorrelation), Genb is the fixed effect of generation (b = 2–6), Selc is the fixed effect of individual housing (after 16 weeks of age) of the females selected for producing the next generation within the selection experiment (with c being not selected or being selected), (Gen *Sel)bc is the fixed effect of the interaction term between Genb and Selc, aj is the random additive genetic effect of the jth animal assumed to be , eabcj is the residual term assumed to be . Assumed (co)variance structures of the random model terms are and , in which A is the additive genetic relationship matrix based on the pedigree consisting of 11,360 individuals from in total 13 generations, is the additive genetic variance, I is an identity matrix, and is the residual variance.
Heritabilities were calculated as
where is the additive genetic variance, and is the phenotypic variance being calculated as: , where is the additive genetic variance and is the residual variance. The likelihood ratio test was used to test whether estimated heritabilities were significantly different from zero, comparing the tested model to a model in which the additive genetic variance was fixed at a value of 0.000001. The likelihood ratio test was -2ln(Λ(x)) with , where L0 is the likelihood under the null hypothesis with the additive genetic variance fixed at 0.000001, L1 is the likelihood under the alternative hypothesis without variance components constrained, and x is the given dataset. Significance was assessed with the likelihood-ratio test assuming that the likelihood ratio follows a -distribution.
The genetic coefficient of variation (GCV) was calculated as:
where is the additive genetic variance of the resilience indicator skewness or autocorrelation, and µ is the overall mean of the resilience indicator. For ln(variance), the GCV is calculated as , because the ln-transformation implicitly assumes an exponential model. Therefore is without units and division by µ is redundant (Mulder et al., 2007; Hill and Mulder, 2010).
To estimate the similarity between resilience indicators, phenotypic and genetic correlations between resilience indicators were estimated based on bivariate analyses using the linear animal models for the resilience indicators described above.
Resilience Indicators and Immunity
To investigate the relationship between resilience indicators and immunity, the phenotypic and genetic correlations between resilience indicators and NAb, and the correlated selection response of resilience indicators in the NAb-selection lines were estimated.
Phenotypic and genetic correlations between resilience indicators and NAb were estimated based on bivariate analyses using the linear animal models for the resilience indicators described above and the following linear animal model for NAb as described by Berghof (2018):
where ydjk is the IgTotal, IgM, or IgG titer, µ is the overall mean, Plated is the fixed effect of plate d on which a sample was analyzed (d = 1-422), aj is the random additive genetic effect of the jth animal assumed to be , damk is the random effect of the kth dam assumed to be , and edjk is the residual term assumed to be . Assumed (co)variance structures of the random model terms are , , and , in which A is the additive genetic relationship matrix based on the pedigree consisting of 11,360 individuals from in total 13 generations, is the additive genetic variance, I is an identity matrix, is the maternal variance, and is the residual variance. Note that the plate effect accounts for confounded effects on the samples, such as sex, storage, and analysis effects.
The correlated selection response of resilience indicators was investigated by plotting the average EBV of the resilience indicators (obtained from the univariate linear animal models) for each line and generation.
Resilience Indicators and Disease Resistance
To investigate the relationship between resilience indicators and disease resistance, the predictive ability of the resilience indicators on APEC mortality and APEC lesion scores was investigated.
EBV of the resilience indicators and IgTotal (obtained from the univariate linear animal models) were used as predictors for mortality in infection experiment 1 (G4) and total lesion scores in infection experiment 2 (G6). Because individuals in the challenge experiments were not part of the NAb-selection lines (i.e. additional G4 and G6), the EBV basically represent parents' EBV averages.
The statistical model used for performing the survival analysis to investigate the relationship between resilience indicators and APEC mortality in the additional G4 was a Cox proportional hazards model (Cox, 1972; Kleinbaum and Klein, 2012):
where hj(t) is a hazard function describing the probability at time t (t = 0–170 h post inoculation) for death to occur on the jth APEC-inoculated chicken (j = 1–197), h0(t) is an unspecified baseline hazard function at time t, exp is the natural exponential function (i.e. ex with x being Xβ), X is a design matrix containing predictors with β being the vector with parameters. The investigated predictors were , , or for the jth APEC-inoculated chicken. To account for the effect of selection on IgTotal NAb, the model was also extended with for the jth APEC-inoculated chicken.
Conditional probabilities were calculated for chickens that died at the same moment (i.e. ties), according to Kalbfleisch and Prentice (2011). Chickens that were euthanized at the end of the experiment were censored (adjusted from Berghof et al., 2019a).
The statistical model used for performing the analysis to investigate the relationship between resilience indicators and APEC lesion scores in the additional G6 was an analysis of covariance (i.e. a general linear model):
where yj is the total lesion score on the jth surviving APEC-inoculated chicken (j = 1-180), µ is the overall mean, X is a design matrix containing predictors with β being the vector with parameters, and ej is the residual term assumed to be where I is an identity matrix and is the residual variance. The investigated predictors were , , or for the jth APEC-inoculated chicken. To account for the effect of selection on IgTotal NAb, the model was also extended with for the jth APEC-inoculated chicken.
Miscellaneous
Genetic parameters of NAb have already been reported based on (part of) this data elsewhere, and will therefore not be reported here (Berghof et al., 2015; Berghof, 2018).
Statistical analyses were performed using ASReml 4.1 (Gilmour et al., 2014) for “Genetic Parameters of Resilience Indicators” and “Resilience Indicators and Immunity,” and by using SAS® software (SAS software V9.3 TS Level 1M2) for “Resilience Indicators and Disease Resistance.”
Significance was declared for p-values ≤ 0.05 and tendency to significance was declared for p-values ≤ 0.10.
Results
Genetic Parameters of Resilience Indicators
Variance components, heritabilities, and GCV of the resilience indicators ln(variance), skewness, and autocorrelation are reported in Table 2. The additive genetic variance () for ln(variance) was 0.09, for skewness was 0.07, and for the autocorrelation was 0.02. Heritabilities were estimated to be 0.10 for ln(variance) and 0.09 for skewness, and were significantly different from zero. However, the heritability for autocorrelation was estimated to be 0.11 and tended to significance (p = 0.053). GCV was 0.30 for ln(variance), 1.56 for skewness, and 0.52 for autocorrelation. Note that the means of skewness and autocorrelation were close to zero, which may inflate their GCV. Thus, resilience indicators based on BW deviations are heritable and show high genetic variability.
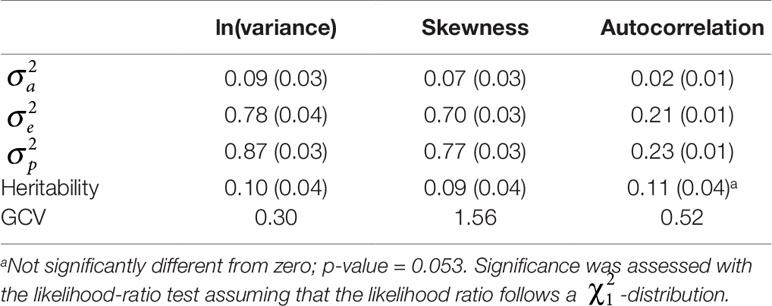
Table 2 Variance components and SE (in parentheses), heritability and SE (in parentheses), and the genetic coefficient of variation (GCV) of the three resilience indicators {i.e. natural logarithm-transformed variance [ln(variance)], skewness, and autocorrelation}.
Phenotypic and genetic correlations among resilience indicators are reported in Table 3. Phenotypic correlations (rp) among resilience indicators were all weak (-0.10 ≤ rp ≤ 0.32). The genetic correlations (rg) between the resilience indicators were low to moderate: the genetic correlation was -0.20 between ln(variance) and skewness, 0.40 between ln(variance) and autocorrelation, and 0.07 between skewness and autocorrelation, all with high SE. Thus, the weak correlations suggest that the resilience indicators capture different aspects of BW deviations.
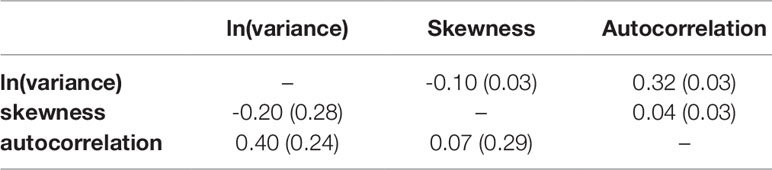
Table 3 Phenotypic (above diagonal) and genetic correlations (below diagonal) and SE (in parentheses) of the three resilience indicators from the linear animal model {natural logarithm-transformed variance [ln(variance)], skewness, and autocorrelation}.
Resilience Indicators and Immunity
Phenotypic and genetic correlations of the resilience indicators and IgTotal, IgM, and IgG NAb are reported in Table 4. The phenotypic and genetic correlations between the resilience indicators and NAb were low: -0.03 ≤ rp ≤ 0.02 and -0.09 ≤ rg ≤ 0.08, all with high SE. Thus, the resilience indicators are genetically different from NAb, i.e. they contain hardly any common genetic variation.

Table 4 Phenotypic and genetic correlations and SE (in parentheses) of the three resilience indicators {natural logarithm-transformed variance [ln(variance)], skewness, and autocorrelation} and the natural antibodies (NAb).
Average EBV of the two NAb-selection lines for the three resilience indicators are shown in Figure 3. In agreement with the estimated genetic correlation, the High line showed a negative trend in average EBVln(variance) and the low line showed a positive trend. EBVskewness and EBVautocorrelation averages did not show clear line differences and remained around zero. Thus, even though the genetic correlations between resilience indicators and NAb were low, EBV of ln(variance) showed a small correlated selection response for IgTotal NAb.
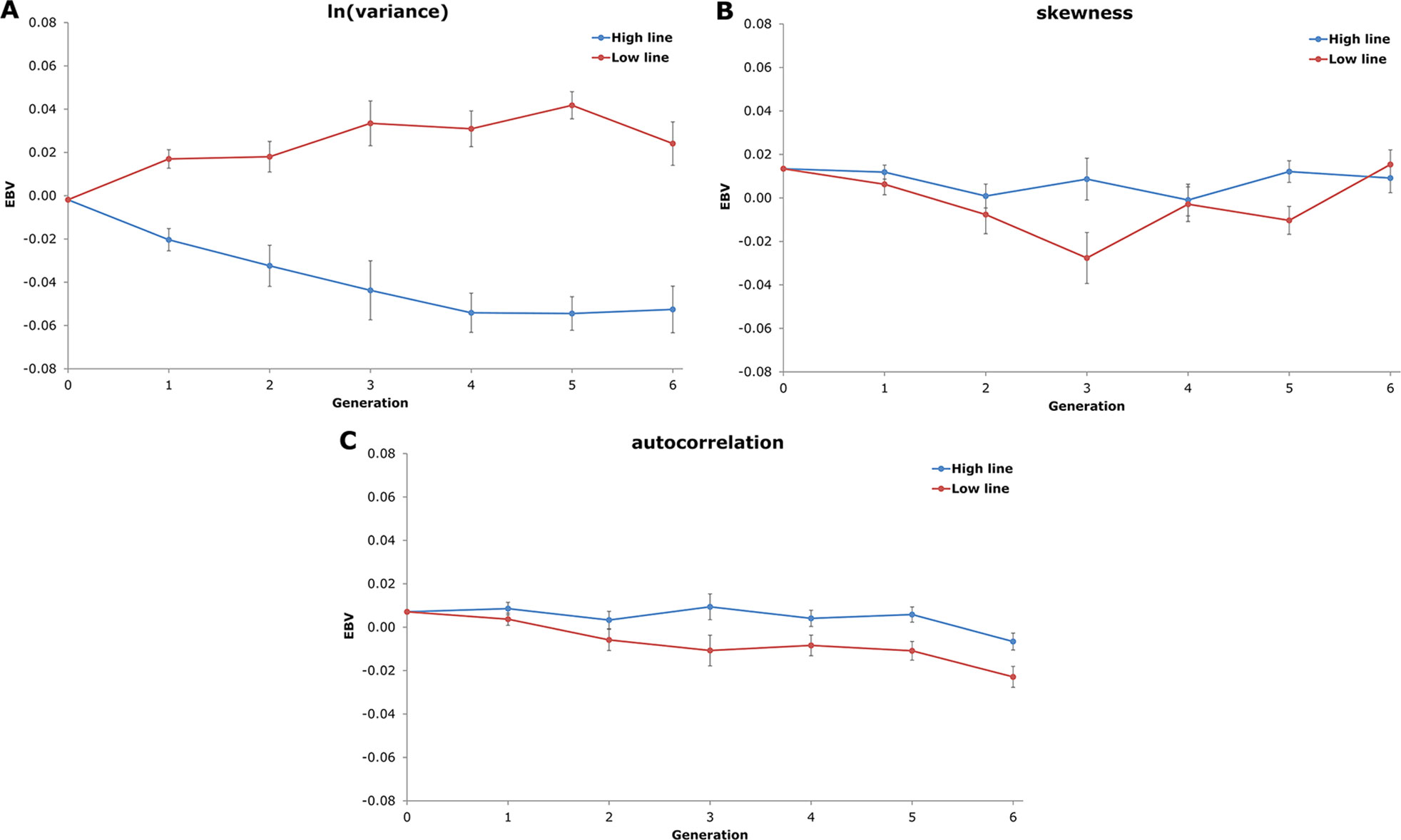
Figure 3 Average estimated breeding values (EBV) and standard errors of the two natural antibody (NAb)-selection lines (High line in blue and Low line in red) over six generations of selection for the three resilience indicators from the linear animal model (A: natural logarithm-transformed variance; B: skewness; and C: autocorrelation). Only EBV of females are shown. EBV of generation 0 and generation 1 are based on offspring phenotypes (i.e. no own phenotypes).
Resilience Indicators and Disease Resistance
The predictive ability of EBV of the resilience indicators and IgTotal NAb for APEC mortality is shown in Table 5. EBVIgTotal significantly predicted mortality (hazard ratio = 0.59; p = 0.01). None of the EBV of the resilience indicators did significantly predicted mortality, neither as single predictor, nor in combination with EBVIgTotal. Thus, the resilience indicators do not predict APEC-induced mortality.
The predictive ability of EBV of the resilience indicator and IgTotal NAb for APEC morbidity is shown in Table 6. EBVIgTotal tended to significantly predict mortality (β = -0.50; p = 0.09). EBVln(variance) significantly predicted lesion scores (p = 0.03). The regression coefficient was positive (β = 6.20) and therefore favorable: greater EBVln(variance) (i.e. lower resilience) was associated with greater lesion scores (i.e. larger disease impact). The EBVln(variance) difference (minimum = -0.33, maximum = 0.19) accounted for a maximal total lesion score difference of 3.2 (out of 12), while EBVIgTotal difference (minimum = -1.45, maximum = 1.41) accounted for a maximal total lesion score difference of 1.4. EBVln(variance) was significant when solely included as a predictor in the model, but only tended to significance (p = 0.07) when EBVIgTotal was included. This suggests that EBVln(variance) and EBVIgTotal partly compete to explain the same variance by the model. EBVskewness and EBVautocorrelation did not significantly predict lesion scores. Thus, EBV of the resilience indicator ln(variance) had a favorable relationship to APEC-induced lesion scores.
Discussion
Breeding for improved resilience seems a promising strategy to obtain trouble-free livestock which is easy-to-manage, and has a greater welfare and health. Berghof et al. (2019b) proposed three resilience indicators to measure resilience based on deviations between expected production and observed production: ln(variance) of deviations, skewness of deviations, and autocorrelation of deviations (Berghof et al., 2019b). Recent studies suggest that fluctuations of longitudinally observed production traits might indeed be related to improved (disease) resilience in livestock (Elgersma et al., 2018); Putz et al., 2019; Poppe et al., 2020). Alternative resilience indicators like skewness and autocorrelation, however, have not been reported before (but is investigated in dairy cattle by Poppe et al., 2020). Breeding for greater NAb levels has been shown to improve APEC-resistance in chickens (Berghof et al., 2019a) and greater NAb levels have been hypothesized to improve or to be indicative for improved general disease resistance (Berghof, 2018). This study is the first to investigate heritabilities of ln(variance), skewness, and autocorrelation of longitudinal-observed deviations, and to relate these resilience indicators with immunity and disease resistance. The obtained results increase our understanding of the biological mechanisms underlying resilience in animals.
Genetic Parameters of Resilience Indicators
The additive genetic variance of ln(variance) was within the range of previously reported estimates based on variation of different traits in different livestock species (e.g. Rönnegård et al., 2013; Vandenplas et al., 2013; Sell-Kubiak et al., 2015; Mulder et al., 2016; Iung et al., 2017; Elgersma et al., 2018), including BW variation in chickens (Rowe et al., 2006; Mulder et al., 2009; Wolc et al., 2009). Although reported heritabilities for ln(variance) are mostly low (0.00–0.10), GVC are generally considerable (0.15–0.30), which was observed for ln(variance) in this study as well. This indicates that there are good prospects for the genetic improvement of ln(variance) and thus for genetic improvement of resilience.
This study is the first study to investigate skewness and autocorrelation of deviations. Heritability estimates of skewness and autocorrelation were similar to the heritability of ln(variance). Poppe et al. (2020) estimated a heritability for autocorrelation based on daily milk deviations similar to this study, although the GCV was considerably lower (0.07–0.17) due to a much lower additive genetic variance. In contrast to our study, they estimated a very low heritability of 0.01 for skewness based on daily milk deviations and a low GCV (0.05–0.10) due to a much lower additive genetic variance compared to this study (factor 10 lower). Three major differences between Poppe et al.'s study and this study might underlie these differences observed for skewness and autocorrelation: 1. Poppe et al. used daily observations with a minimum of 50 observations per individual in contrast to this study's four-weekly observations with a minimum of five observations per individual. Poppe et al.'s approach captures (smaller, shorter) disturbances more accurately and is therefore expected to be a more accurate representation of resilience (as discussed in Berghof et al., 2019b); 2. Poppe et al. used milk yield deviations as an indicator. Milk yield is known to respond fast to environmental factors (i.e. disturbances) and changes in milk yield can be observed between days. In contrast, BW responds slowly to environmental factors, except for enteric diseases, and, generally, changes in BW only become apparent after several days. Thus, daily milk yield deviations can be a “fast resilience indicator,” while BW deviations (even if taken daily) are a “slow” resilience indicator. Therefore, it is expected that resilience indicators based on daily milk yield deviations are different from resilient indicators based on BW deviations; and 3. Poppe et al. based the expected production of an individual on the individual's lactation curve in contrast to this study's cohort average (line*generation*weighing moment). To approximate this method, deviations based on Gompertz curves for growth of each individual were used to estimate resilience indicators (results not shown). However, due to the small number of BW observations, deviations were almost completely absorbed into the Gompertz curve parameters, which resulted in very small resilience indicators. Therefore, fitting the Gompertz curve or fitting any other growth curve seems not appropriate when the number of observations is small, which effectively results in a low number of degrees of freedom. On the contrary, when using deviations from cohort averages to calculate resilience indicators, genetic differences in individual growth curves might end up as deviations from the cohort averages. As a consequence, resilience and growth curves are confounded. To investigate this potential confounding, the genetic correlations between the resilience indicators and the Gompertz curve's parameter a (estimated BW after the growth phase), parameter b (estimated BW before the growth phase), and parameter c (the estimated growth during the growth phase) were estimated (results not shown). The correlations were around zero between the resilience indicators and parameters a and b, which indicates that the resilience indicators are independent of the maximum BW and the initial BW. This is expected, because all resilience indicators are (mathematically) independent of averages. However, from the definitions of the three parameters, it is most likely that parameter c would yield non-zero genetic correlations with the resilience indicators. Indeed, the estimated genetic correlation between skewness and parameter c was -0.58 (SE = 0.17), while the genetic correlations between parameter c and ln(variance), and between parameter c and autocorrelations were close to zero. This suggests that skewness based on cohort averages captures partly individual differences in growth rate, and this might explain the differences observed for skewness between the study of Poppe et al. (2020), who used individual lactation curves, and this study. This finding does not per se exclude skewness based on BW deviations as a resilience indicator, because the individual growth differences might be a consequence of differences in resilience. It can be concluded that using cohort averages to calculate deviations is useful when the number of observations is low, but skewness of deviations based on cohort averages may also capture individual genetic differences in trait curves.
Genetic correlations between resilience indicators were low. This might indicate that some of the resilience indicators are not predictive or that the different resilience indicators capture different aspects of resilience (as argued above). However, it cannot be excluded that the genetic correlations are actually greater than estimated in this study and consequently that the resilience indicators are more alike, because of the large SE of the estimations. The large SE might be a consequence of the low frequency and impact of (disease) disturbances in the study population's environment. However, similar results were observed in the study of Poppe et al. (2020) under commercial settings, supporting the hypothesis of a different genetic makeup and different information of the resilience indicators (Berghof et al., 2019b), even though these indicators are based on the same deviations. In order to genetically improve resilience, the different resilience indicators need to be combined in a selection index, if such an index indeed predicts resilience better than any of the individual indicators alone.
In previous studies, ln(variance) was investigated by using double-hierarchical generalized linear models (DHGLM), which accounts for the mean and variance of deviations simultaneously (Rönnegård et al., 2010; Felleki et al., 2012; Mulder et al., 2013). DHGLM analyses are computationally and mathematically more challenging to perform and to achieve convergence, which makes implementations of these models into breeding programs also practically challenging. Nevertheless, a DHGLM was used to analyze the BW data and results were compared to the simpler approach reported here (results not shown). Interestingly, the additive genetic variance of the DHGLM-ln(variance) was equal to the additive genetic variance of ln(variance) with a high genetic correlation (0.995). This shows that the more complex DHGLM approach, though theoretically more accurate, can be approximated very well by a more simplistic approach (similar to Sell-Kubiak et al., 2015), which can be more easily implemented in practice.
Possible factors influencing the BW deviations are permanent environmental effects and maternal environmental effects. Permanent environmental effects exist on BW, because of the repeated measurements. However, we condensed the BW information to one estimate of a resilience indicator per animal, and therefore permanent environmental effects are not identifiable. Maternal environmental effects could not be estimated (results not shown), because for most of the dams too few (i.e. <5) offspring were present in the dataset. Not accounting for maternal effects might result in overestimation of the genetic variance. Genetic analyses of BW at different time points did have significant maternal environmental effects at all time points (Berghof et al., in preparation). When maternal environmental effects were estimated for the resilience indicators, a significant maternal environmental effect was found for autocorrelation. However, this also completely absorbed the genetic variance (i.e. heritability was not significantly different from zero), confirming difficulties to disentangle genetic variance and maternal variance with a low number of offspring per dam in this dataset. Nevertheless, this indicates that environmental effects may influence resilience indicators and should be considered in future studies whenever possible.
The proposed resilience indicators by Berghof et al. (2019b) are defined based on longitudinal data on a commonly-measured production trait. This study used 5 to 7 BW measurements for estimating the heritability of ln(variance). The ln(variance) heritability in this study was clearly greater than ln(variance) heritability estimates based on one observation per individual (e.g. Janhunen et al., 2012; Sae-Lim et al., 2015; Iung et al., 2017; see also Hill and Mulder, 2010 for a review). This fits within the proposed idea of high-frequency BW measurements (Friedman et al., 2012; Revilla et al., 2019) and the strong increase in heritability estimates with the addition of a few observations for an individual (Figure 2 from Berghof et al., 2019b). Moreover, the current technological developments are expected to allow more frequent (i.e. daily) measurements on different phenotypes in all livestock species in the near future, including chickens (Friggens et al., 2017; Mulder, 2017; Berghof et al., 2019b). This study is therefore also an exploratory study to investigate the potential of different resilience indicators based on longitudinal data.
Resilience Indicators and Immunity
To investigate the relationship between resilience and immunity, the genetic correlations between the three resilience indicators and NAb were investigated in chickens divergently selected for NAb levels (see Berghof, 2018 for more information). High levels of KLH-binding NAb were previously associated with lower mortality, improved immunity, and increased disease resistance in chickens (Lammers et al., 2004; Star et al., 2007; Sun et al., 2011; Wondmeneh et al., 2015; Berghof, 2018; Berghof et al., 2018a; Berghof et al., 2019a). Thus, NAb are expected to influence both the resistance (i.e. “minimally affected”) and the recovery (i.e. “rapidly return”), which are both intrinsic parts of (the definition of) resilience (Berghof et al., 2019b). We hypothesized that chickens selectively bred for greater NAb levels have a greater resilience compared to chickens selectively bred for lower Nab levels.
Genetic correlations between the resilience indicators and NAb were low, and thus, in contrast to our hypothesis, shared common mechanisms seem not to be present. This means that the resilience indicators do not account for disease resistance, or, more likely, do not cover disease resistance in this study population due to a too low level of disease pressure to exploit genetic differences under the standardized conditions of the research facility. It is well-known that in high challenge environment more genetic variation in resistance or resilience is observed compared to “normal” environments (Drangsholt et al., 2011; LaFrentz et al., 2016; Putz et al., 2019). For example, Putz et al. (2019) investigated resilience indicators in pigs housed in a “natural disease challenge model” (i.e. an environment with a high multifactorial disease pressure). They found relatively high heritabilities for variation (0.15–0.26), and favorable correlations between variation and mortality, and variation and number of treatments (0.37–0.85) (Putz et al., 2019). In the same study population, NAb (measured before entering the high challenge environment) were predictive for fewer medical treatments and a greater resilience (i.e. decreased day-to-day fluctuations in feed intake) (Tibbs et al., 2018). Although the phenotypic correlations were weak (< |0.09|; Tibbs et al., 2018), they are similar to the phenotypic correlations in this study. We are currently investigating the relation between resilience indicators and NAb in cross-bred populations housed in conventional production system. This will give more insight in their relationship under more challenging conditions. Anyhow, we hypothesize for now that NAb are informative for disease resilience before disturbances (i.e. diseases) are present, while the resilience indicators are informative for disease resilience after disturbances.
Remarkably, in preliminary work on the resilience indicators in animals up to G5, larger favorable genetic correlations (approximately -0.3) were found between ln(variance) and NAb (Berghof et al., 2018b). The preliminary study (G0–G5) and this study (G0–G6) have similar variance components, which suggests that G6 is similar to G0–G5. However, reported SE of both studies are large (around 0.2) due to a small study population, and consequently the difference in genetic correlations might be due to sampling. In addition, the discrepancy between G0–G5 and G0–G6 can also indicate that G6 added different information to the dataset regarding the relationship between NAb and resilience. G6 did have two notable differences compared to the other generations: 1. an E. coli infection was present in the flock during the first two weeks of life and the animals were treated with antibiotics. It can be expected that such an event would actually increase BW variation. However, that seems not the case. Alternatively, NAb levels and NAb level development during early life might have been influenced by this event; and 2. G6 was bred from parents of approximately 50 weeks of age due to other experimental work, while parents of the other generations were between 30 and 35 weeks of age. Such an age difference can result in for example differences of maternal antibody transfer to offspring, which can permanently influence humoral immunity (Klipper et al., 2004; Lammers et al., 2004; Gharaibeh et al., 2008; Hasselquist and Nilsson, 2009; Ulmer-Franco et al., 2012). However, maternal effects on NAb levels did not seem to be different in G6 (results not shown). Nevertheless, this remains speculative and future studies will have to give more insight in the genetic relationship between resilience and NAb, and the influence of maternal effects (e.g. dam age).
Resilience Indicators and Disease Resistance
As stated above, a possible lack of disease challenges in the study population can explain an apparent weak relationship between resilience indicators and NAb. Berghof et al. (2019a) performed two APEC-challenge experiments to measure disease resistance in the NAb-selection lines. Although the experiments are too short to measure BW deviations to obtain resilience indicators on the challenged individuals, EBV for resilience indicators for the challenged individuals were obtained from the genetic parameter estimation. The predictive ability of EBV of resilience indicators on APEC-induced mortality and total lesion scores in the infection experiments was investigated to obtain insights in the relationships between resilience indicators and the response to diseases, i.e. APEC.
EBVskewness and EBVautocorrelation were not predictive for mortality or lesion scores. This is in line with Poppe et al. (2020), who concluded that skewness and autocorrelation of deviations in milk yield seem less useful as resilience indicators in dairy cows. Therefore, their potential use as resilience indicators seems to be limited, but needs further investigation in other study populations.
EBVln(variance) was predictive for lesion scores, but not for mortality. Possibly, lesion scores represent the capacity to deal with infections (i.e. not dying) and thus might be closer to the definition of resilience (i.e. recovery). Chickens with lower EBVln(variance) (i.e. a greater resilience) had lower lesion scores compared to chickens with greater EBVln(variance) (i.e. a lower resilience). EBVln(variance) predicted up to 25% of the total lesion score, which was twice as much as the EBV for IgTotal NAb, i.e. the selection criterion. Compared to ln(variance), this difference might be due to: 1. the protective function of NAb being more related to disease resistance (and mortality) rather than recovery, which is circumvented in this experiment by applying a standardized dose of an infectious agent; 2. the immunological function of NAb being more related to the initiation of the (humoral) adaptive immune response, which is barely initiated within the experimental period (i.e. one week is too short) (Berghof et al., in preparation); or 3. APEC-resistance being not solely dependent on NAb levels. Nevertheless, some common background in resilience and immunity/disease resistance seems to be present, since EBV of ln(variance) and NAb accounted for the same model variance. Thus, in line with previous studies (Elgersma et al., 2018; Putz et al., 2019; Poppe et al., 2020), ln(variance) seems to have predictive capacity for disease resilience.
Conclusion
This study described three proposed resilience indicators in chickens: ln(variance), skewness, and autocorrelation of standardized BW deviations. All three resilience indicators were heritable, and suggest to capture different parts of resilience. In contrast to our hypothesis, NAb (as a measure of immunity) were not or only weakly genetically correlated with resilience indicators in this study population. Thus, resilience indicators and NAb seem not to be under common genetic control, suggesting that diseases cause only a small (negligible?) part of day-to-day disturbances. However, this might be a consequence of a lack of disease challenges during life. In addition, hints of a small common genetic background between ln(variance) and NAb were found: 1. the high NAb-selection line had a greater resilience compared to the low NAb-selection line based on EBV of ln(variance); and 2. individuals with a lower EBV for ln(variance) had lower APEC-induced lesion scores. Thus, ln(variance) might be an indicator for disease resilience and can, together with NAb, be included in breeding indices to improve resilience and immunity.
This study is, as far as we know, the first to investigate genetic parameters of skewness and autocorrelation, and the first to investigate a direct relationship between resilience phenotypes (as defined by Berghof et al., 2019b) and the immune system. Overall, this study suggests that ln(variance) is a promising resilience indicator, because it shows a (weak) relationship to immunity and disease resistance in a relatively high hygiene environment. More studies are needed to investigate the potential for skewness and autocorrelation, but these parameters seem less promising. Anyhow, the genetic variation found for the proposed resilience indicators gives ample opportunity to genetically improve resilience in chickens.
Data Availability Statement
Data are available upon request, after approval of both the authors and Hendrix Genetics. Contact the corresponding author by e-mail.
Ethics Statement
Ethical review and approval was not required for the animal study because Collection of samples and data of the base population was done according to Hendrix Genetics protocols, under the supervision of Hendrix Genetics employees. Samples and data were collected as part of routine data collection in a commercial breeding program for layer chickens in The Netherlands. Samples and data were collected on a breeding nucleus of Hendrix Genetics for breeding purposes and are a non-experimental, agricultural practice, regulated by the Act Animals and the Royal Decree on Procedures. The Dutch Experiments on Animals Act does not apply to non-experimental, agricultural practices. An ethical review by the 'Dierexperimentencommissie’ (Animal Experiment Committee) was therefore not required. No extra discomfort was caused by sample collection for the purpose of this part of the study. The selection and infection experiments were approved by the ‘Dierexperimentencommissie’ (Animal Experiment Committee) of Wageningen University & Research according to Dutch law (Experiment Codes Selection experiment: 2012105, 2013091, 2014058, and 2014093; Experiment Codes Infection experiments: 2014126 and 2016057) and the ‘Centrale Commissie Dierproeven’ (Central Committee Animal Experiments) of the Dutch government (numbers Infection experiments: 201521, and 2015357). Written informed consent was obtained from the owners for the participation of their animals in this study.
Author Contributions
TB, HB, and HM designed the research. TB performed the research, collected the data, analyzed the data, and wrote the manuscript. HM assisted with the genetic analyses and interpretation of results. HB and HM revised the manuscript. All authors approved the final version.
Funding
This work is part of the research programme “Divergent selection for natural antibodies in poultry” with project number 12208, which is financially supported by the Netherlands Organisation for Scientific Research. This work is financially supported by Netherlands Organisation for Scientific Research Earth and Life Sciences (NWO-ALW; ALWSA.2016.4). This work is in kind supported by Hendrix Genetics.
Conflict of Interest
This research was in kind supported by Hendrix Genetics. Except for this support, no other shared interests (e.g. employment, consultancy, patents, products) exist between Hendrix Genetics and the authors.
All authors declare that the research was conducted in the absence of any commercial or financial relationships that could be construed as a potential conflict of interest.
The reviewer AD-W declared a past co-authorship with one of the authors HM to the handling editor.
Acknowledgments
We thank Marieke Poppe of Wageningen University & Research Animal Breeding and Genomics for her help on defining and interpreting the resilience indicators and for critically reading the manuscript. We thank Michael N. Aldridge of Wageningen University & Research Animal Breeding and Genomics for his help on estimating the Gompertz parameters in this study. We thank Henk Parmentier of Wageningen University & Research Adaptation Physiology and Jan van der Poel of Wageningen University & Research Animal Breeding and Genomics for their role in the experimental design of the NAb-selection experiment. We thank Joop Arts of Wageningen University & Research Adaptation Physiology and all involved animal caretakers of research facility “Carus” from Wageningen University & Research for their role during the experimental period. We thank Komarudin and Francois Karangali of Wageningen University for their help in organizing the data. We thank the Netherlands Organisation for Scientific Research for financial support of this work through the research programme “Divergent selection for NAb in poultry” with project number 12208. We thank Netherlands Organisation for Scientific Research Earth and Life Sciences (NWO-ALW) for financial support of this work (ALWSA.2016.4). We thank Hendrix Genetics for their in kind support of this work.
Supplementary Material
The Supplementary Material for this article can be found online at: https://www.frontiersin.org/articles/10.3389/fgene.2019.01216/full#supplementary-material
References
Baumgarth, N., Tung, J. W., Herzenberg, L. A. (2005). Inherent specificities in natural antibodies: a key to immune defense against pathogen invasion. Semin. Immunopathol. 26, 347–362. doi: 10.1007/s00281-004-0182-2
Berghof, T. V. L., Van Der Klein, S. A. S., Arts, J. A. J., Parmentier, H. K., Van Der Poel, J. J., Bovenhuis, H. (2015). Genetic and non-genetic inheritance of natural antibodies binding keyhole limpet hemocyanin in a purebred layer chicken line. PloS One 10, e0131088. doi: 10.1371/journal.pone.0131088
Berghof, T. V. L., Arts, J. A. J., Bovenhuis, H., Lammers, A., Van Der Poel, J. J., Parmentier, H. K. (2018a). Antigen-dependent effects of divergent selective breeding based on natural antibodies on specific humoral immune responses in chickens. Vaccine 36, 1444–1452. doi: 10.1016/j.vaccine.2018.01.063
Berghof, T. V. L., Bovenhuis, H., Arts, J. A. J., Karangali, F., Van Der Poel, J. J., Parmentier, H. K., et al (2018b). "Body weight variation as indicator for resilience in chickens," in Book of abstracts of the 69th annual meeting of the European federation of animal science (Wageningen, NL: Wageningen Academic Publishers) vol. 24, 577. doi: 10.3920/978-90-8686-871-1
Berghof, T. V. L., Visker, M. H. P. W., Arts, J. A. J., Parmentier, H. K., Van Der Poel, J. J., Vereijken, A. L. J., et al. (2018c). Genomic region containing Toll-like receptor genes has a major impact on total IgM antibodies including KLH-binding IgM natural antibodies in chickens. Front. Immunol. 8, 1879. doi: 10.3389/fimmu.2017.01879
Berghof, T. V. L., Matthijs, M. G. R., Arts, J. A. J., Bovenhuis, H., Dwars, R. M., Van Der Poel, J. J., et al. (2019a). Selective breeding for high natural antibody level increases resistance to avian pathogenic Escherichia coli (APEC) in chickens. Dev. Comp. Immunol. 93, 45–57. doi: 10.1016/j.dci.2018.12.007
Berghof, T. V. L., Poppe, M., Mulder, H. A. (2019b). Opportunities to improve resilience in animal breeding programs. Front. Genet. 9, 692. doi: 10.3389/fgene.2018.00692
Berghof, T. V. L. (2018). "Selective breeding on natural antibodies in chickens." PhD dissertation. (Wageningen, NL: Wageningen University). doi: 10.18174/426278
Byrd, C. J., Johnson, J. S., Radcliffe, J. S., Craig, B. A., Eicher, S. D., Lay, D. C. (2019). Nonlinear analysis of heart rate variability for evaluating the growing pig stress response to an acute heat episode. Animal. doi: 10.1017/S1751731119001630
Codrea, M. C., Højsgaard, S., Friggens, N. C. (2011). Differential smoothing of time-series measurements to identify disturbances in performance and quantify animal response characteristics: an example using milk yield profiles in dairy cows. J. Anim. Sci. 89, 3089–3098. doi: 10.2527/jas.2010-3753
Colditz, I. G., Hine, B. C. (2016). Resilience in farm animals: biology, management, breeding and implications for animal welfare. Anim. Prod. Sci. 56, 1961–1983. doi: 10.1071/AN15297
Cox, D. R. (1972). Regression models and life-tables. J. R. Stat. Soc. Ser. B Stat. Methodol. 34, 187–220.
De Haas, Y., Veerkamp, R. F., Barkema, H. W., Gröhn, Y. T., Schukken, Y. H. (2004). Associations between pathogen-specific cases of clinical mastitis and somatic cell count patterns. J. Dairy Sci. 87, 95–105. doi: 10.3168/jds.S0022-0302(04)73146-X
Doeschl-Wilson, A. B., Villanueva, B., Kyriazakis, I. (2012). The first step toward genetic selection for host tolerance to infectious pathogens: obtaining the tolerance phenotype through group estimates. Front. Genet. 3, 265. doi: 10.3389/fgene.2012.00265
Drangsholt, T. M., Gjerde, B., Odegard, J., Finne-Fridell, F., Evensen, O., Bentsen, H. B. (2011). Quantitative genetics of disease resistance in vaccinated and unvaccinated Atlantic salmon (Salmo salar L.). Heredity 107, 471–477. doi: 10.1038/hdy.2011.34
Elgersma, G. G., De Jong, G., Van Der Linde, R., Mulder, H. A. (2018). Fluctuations in milk yield are heritable and can be used as a resilience indicator to breed healthy cows. J. Dairy Sci. 101, 1240–1250. doi: 10.3168/jds.2017-13270
Felleki, M., Lee, D., Lee, Y., Gilmour, A. R., Rönnegård, L. (2012). Estimation of breeding values for mean and dispersion, their variance and correlation using double hierarchical generalized linear models. Genet. Res. 94, 307–317. doi: 10.1017/s0016672312000766
Fischer, A., Friggens, N. C., Berry, D. P., Faverdin, P. (2018). Isolating the cow-specific part of residual energy intake in lactating dairy cows using random regressions. Animal 12, 1396–1404. doi: 10.1017/S1751731117003214
Frankena, K. (1987). "The interaction between Cooperia spp. and Ostertagia spp. (Nematoda: Trichostrongylidae) in cattle." PhD dissertation. (Wageningen, NL: Agricultural University Wageningen).
Friedman, A., Elad, O., Cohen, I., Bar-Shira, E. (2012). The gut associated lymphoid system in the post-hatch chick: dynamics of maternal IgA. Isr. J. Vet. Med. 67, 75–81.
Friggens, N. C., Blanc, F., Berry, D. P., Puillet, L. (2017). Review: deciphering animal robustness. A synthesis to facilitate its use in livestock breeding and management. Animal 11, 2237–2251. doi: 10.1017/S175173111700088X
Gharaibeh, S., Mahmoud, K., Al-Natour, M. (2008). Field evaluation of maternal antibody transfer to a group of pathogens in meat-type chickens. Poult. Sci. 87, 1550–1555. doi: 10.3382/ps.2008-00119
Gilmour, A. R., Gogel, B. J., Cullis, B. R., Thompson, R. (2014). ASReml user guide, release 4.1. Hemel Hempstead, UK: VSN International Ltd.
Hasselquist, D., Nilsson, J.-Å. (2009). Maternal transfer of antibodies in vertebrates: trans-generational effects on offspring immunity. Phil. Trans. R. Soc. B 364, 51–60. doi: 10.1098/rstb.2008.0137
Hill, W. G., Mulder, H. A. (2010). Genetic analysis of environmental variation. Genet. Res. 92, 381–395. doi: 10.1017/s0016672310000546
Ibáñez-Escriche, N., Sorensen, D., Waagepetersen, R., Blasco, A. (2008a). Selection for environmental variation: a statistical analysis and power calculations to detect response. Genetics 180, 2209–2226. doi: 10.1534/genetics.108.091678
Ibáñez-Escriche, N., Varona, L., Sorensen, D., Noguera, J. L. (2008b). A study of heterogeneity of environmental variance for slaughter weight in pigs. Animal 2, 19–26. doi: 10.1017/S1751731107001000
Iung, L. H. S., Neves, H. H. R., Mulder, H. A., Carvalheiro, R. (2017). Genetic control of residual variance of yearling weight in Nellore beef cattle. J. Anim. Sci. 95, 1425–1433. doi: 10.2527/jas.2016.1326
Janhunen, M., Kause, A., Vehviläinen, H., Järvisalo, O. (2012). Genetics of microenvironmental sensitivity of body weight in rainbow trout (Oncorhynchus mykiss) selected for improved growth. PloS One 7, e38766. doi: 10.1371/journal.pone.0038766
Kalbfleisch, J. D., Prentice, R. L. (2011). The statistical analysis of failure time data. Hoboken (NJ), US: John Wiley & Sons. doi: 10.1002/9781118032985
Kleinbaum, D. G., Klein, M. (2012). Survival analysis - A self-learning text (third edition). New York (NY), US: Springer. doi: 10.1007/978-1-4419-6646-9
Klipper, E., Sklan, D., Friedman, A. (2004). Maternal antibodies block induction of oral tolerance in newly hatched chicks. Vaccine 22, 493–502. doi: 10.1016/j.vaccine.2003.07.011
Knap, P. W. (2009). "Voluntary feed intake and pig breeding," in Voluntary feed intake in pigs. Eds. D. Torrallardona, and E. A. Roura (Wageningen, NL: Wageningen Academic Publishers). doi: 10.3920/978-90-8686-689-2
LaFrentz, B. R., Lozano, C. A., Shoemaker, C. A., García, J. C., Xu, D.-H., Løvoll, M., et al. (2016). Controlled challenge experiment demonstrates substantial additive genetic variation in resistance of Nile tilapia (Oreochromis niloticus) to Streptococcus iniae. Aquaculture 458, 134–139. doi: 10.1016/j.aquaculture.2016.02.034
Lammers, A., Klomp, M. E. V., Nieuwland, M. G. B., Savelkoul, H. F. J., Parmentier, H. K. (2004). Adoptive transfer of natural antibodies to non-immunized chickens affects subsequent antigen-specific humoral and cellular immune responses. Dev. Comp. Immunol. 28, 51–60. doi: 10.1016/S0145-305X(03)00102-2
Mulder, H. A., Bijma, P., Hill, W. G. (2007). Prediction of breeding values and selection responses with genetic heterogeneity of environmental variance. Genetics 175, 1895–1910. doi: 10.1534/genetics.106.063743
Mulder, H. A., Bijma, P., Hill, W. G. (2008). Selection for uniformity in livestock by exploiting genetic heterogeneity of residual variance. Genet. Sel. Evol. 40, 37–59. doi: 10.1051/gse:2007034
Mulder, H. A., Hill, W. G., Vereijken, A., Veerkamp, R. F. (2009). Estimation of genetic variation in residual variance in female and male broiler chickens. Animal 3, 1673–1680. doi: 10.1017/s1751731109990668
Mulder, H. A., Rönnegård, L., Fikse, W. F., Veerkamp, R. F., Strandberg, E. (2013). Estimation of genetic variance for macro- and micro-environmental sensitivity using double hierarchical generalized linear models. Genet. Sel. Evol. 45, 23. doi: 10.1186/1297-9686-45-23
Mulder, H. A., Visscher, J., Fablet, J. (2016). Estimating the purebred-crossbred genetic correlation for uniformity of eggshell color in laying hens. Genet. Sel. Evol. 48, 39. doi: 10.1186/s12711-016-0212-2
Mulder, H. A. (2017). Is GxE a burden or a blessing? Opportunities for genomic selection and big data. J. Anim. Breed. Genet. 134, 435–436. doi: 10.1111/jbg.12303
Neves, H. H. R., Carvalheiro, R., Roso, V. M., Queiroz, S. A. (2011). Genetic variability of residual variance of production traits in Nellore beef cattle. Livest. Sci. 142, 164–169. doi: 10.1016/j.livsci.2011.07.010
Nguyen-Ba, H., Van Milgen, J., Taghipoor, M. (2019). A procedure to quantify the feed intake response of growing pigs to perturbations. Animal. doi: 10.1017/S1751731119001976
Poppe, M., Veerkamp, R. F., Van Pelt, M. L., Mulder, H. A. (2020). Exploration of variance, autocorrelation, and skewness of deviations from lactation curves as resilience indicators for breeding. J. Dairy Sci. doi: 10.3168/jds.2019-17290
Putz, A. M., Harding, J. C. S., Dyck, M. K., Fortin, F., Plastow, G. S., Dekkers, J. C. M. (2019). Novel resilience phenotypes using feed intake data from a natural disease challenge model in wean-to-finish pigs. Front. Genet. 9, 660. doi: 10.3389/fgene.2018.00660
Rönnegård, L., Felleki, M., Fikse, F., Mulder, H. A., Strandberg, E. (2010). Genetic heterogeneity of residual variance - estimation of variance components using double hierarchical generalized linear models. Genet. Sel. Evol. 42, 8. doi: 10.1186/1297-9686-42-8
Rönnegård, L., Felleki, M., Fikse, W. F., Mulder, H. A., Strandberg, E. (2013). Variance component and breeding value estimation for genetic heterogeneity of residual variance in Swedish Holstein dairy cattle. J. Dairy Sci. 96, 2627–2636. doi: 10.3168/jds.2012-6198
Revilla, M., Friggens, N. C., Broudiscou, L. P., Lemonnier, G., Blanc, F., Ravon, L., et al. (2019). Towards the quantitative characterisation of piglets’ robustness to weaning: a modelling approach. Animal 13, 2536–2546. doi: 10.1017/S1751731119000843
Rowe, S. J., White, I. M., Avendaño, S., Hill, W. G. (2006). Genetic heterogeneity of residual variance in broiler chickens. Genet. Sel. Evol. 38, 617. doi: 10.1186/1297-9686-38-6-617
Sae-Lim, P., Kause, A., Janhunen, M., Vehviläinen, H., Koskinen, H., Gjerde, B., et al. (2015). Genetic (co)variance of rainbow trout (Oncorhynchus mykiss) body weight and its uniformity across production environments. Genet. Sel. Evol. 47, 46. doi: 10.1186/s12711-015-0122-8
Sae-Lim, P., Gjerde, B., Nielsen, H. M., Mulder, H., Kause, A. (2016). A review of genotype-by-environment interaction and micro-environmental sensitivity in aquaculture species. Rev. Aquac. 8, 369–393. doi: 10.1111/raq.12098
Sae-Lim, P., Kause, A., Lillehammer, M., Mulder, H. A. (2017). Estimation of breeding values for uniformity of growth in Atlantic salmon (Salmo salar) using pedigree relationships or single-step genomic evaluation. Genet. Sel. Evol. 49, 33. doi: 10.1186/s12711-017-0308-3
Scheffer, M., Bascompte, J., Brock, W. A., Brovkin, V., Carpenter, S. R., Dakos, V., et al. (2009). Early-warning signals for critical transitions. Nature 461, 53. doi: 10.1038/nature08227
Scheffer, M., Carpenter, S. R., Dakos, V., Van Nes, E. H. (2015). Generic indicators of ecological resilience: inferring the chance of a critical transition. Annu. Rev. Ecol. Evol. Syst. 46, 145–167. doi: 10.1146/annurev-ecolsys-112414-054242
Scheffer, M., Bolhuis, J. E., Borsboom, D., Buchman, T. G., Gijzel, S. M. W., Goulson, D., et al. (2018). Quantifying resilience of humans and other animals. Proc. Natl. Acad. Sci. U. S. A. 115, 11883–11890. doi: 10.1073/pnas.1810630115
Sell-Kubiak, E., Bijma, P., Knol, E. F., Mulder, H. A. (2015). Comparison of methods to study uniformity of traits: application to birth weight in pigs. J. Anim. Sci. 93, 900–911. doi: 10.2527/jas.2014-8313
Star, L., Frankena, K., Kemp, B., Nieuwland, M. G. B., Parmentier, H. K. (2007). Natural humoral immune competence and survival in layers. Poult. Sci. 86, 1090–1099. doi: 10.1093/ps/86.6.1090
Sun, Y., Parmentier, H. K., Frankena, K., Van Der Poel, J. J. (2011). Natural antibody isotypes as predictors of survival in laying hens. Poult. Sci. 90, 2263–2274. doi: 10.3382/ps.2011-01613
Tibbs, L. E., Ashley, C., Putz, A., Lim, K.-S., Dyck, M. K., Fontin, F., et al. (2018). Selection for increased natural antibody levels to improve disease resilience in pigs. Animal Industry Report AS 664, ASL R3271. doi: 10.31274/ans_air-180814-285
Ulmer-Franco, A. M., Cherian, G., Quezada, N., Fasenko, G. M., Mcmullen, L. M. (2012). Hatching egg and newly hatched chick yolk sac total IgY content at 3 broiler breeder flock ages. Poult. Sci. 91, 758–764. doi: 10.3382/ps.2011-01757
Van Eck, J. H. H., Goren, E. (1991). An Ulster 2C strain-derived Newcastle disease vaccine: vaccinal reaction in comparison with other lentogenic Newcastle disease vaccines. Avian Pathol. 20, 497–507. doi: 10.1080/03079459108418787
Van Der Klein, S. A. S., Berghof, T. V. L., Arts, J. A. J., Parmentier, H. K., Van Der Poel, J. J., Bovenhuis, H. (2015). Genetic relations between natural antibodies binding keyhole limpet hemocyanin and production traits in a purebred layer chicken line. Poult. Sci. 94, 875–882. doi: 10.3382/ps/pev052.
Vandenplas, J., Bastin, C., Gengler, N., Mulder, H. A. (2013). Genetic variance in micro-environmental sensitivity for milk and milk quality in Walloon Holstein cattle. J. Dairy Sci. 96, 5977–5990. doi: 10.3168/jds.2012-6521
Wolc, A., White, I. M. S., Avendano, S., Hill, W. G. (2009). Genetic variability in residual variation of body weight and conformation scores in broiler chickens. Poult. Sci. 88, 1156–1161. doi: 10.3382/ps.2008-00547
Wolc, A., Arango, J., Settar, P., Fulton, J. E., O’sullivan, N. P., Preisinger, R., et al. (2012). Genome-wide association analysis and genetic architecture of egg weight and egg uniformity in layer chickens. Anim. Genet. 43, 87–96. doi: 10.1111/j.1365-2052.2012.02381.x
Keywords: resilience, immunity, disease resistance, natural antibody, production, body weight, deviation, micro-environment
Citation: Berghof TVL, Bovenhuis H and Mulder HA (2019) Body Weight Deviations as Indicator for Resilience in Layer Chickens. Front. Genet. 10:1216. doi: 10.3389/fgene.2019.01216
Received: 03 July 2019; Accepted: 04 November 2019;
Published: 13 December 2019.
Edited by:
Guilherme J. M. Rosa, University of Wisconsin-Madison, United StatesReviewed by:
Allan Schinckel, Purdue University, United StatesAndrea B. Doeschl-Wilson, University of Edinburgh, United Kingdom
Copyright © 2019 Berghof, Bovenhuis and Mulder. This is an open-access article distributed under the terms of the Creative Commons Attribution License (CC BY). The use, distribution or reproduction in other forums is permitted, provided the original author(s) and the copyright owner(s) are credited and that the original publication in this journal is cited, in accordance with accepted academic practice. No use, distribution or reproduction is permitted which does not comply with these terms.
*Correspondence: Tom V.L. Berghof, tom.berghof@wur.nl