- 1Department of Genomics, Branch for Northwest & West region, Agricultural Biotechnology Research Institute of Iran (ABRII), Agricultural Research, Education and Extension Organization (AREEO), Tabriz, Iran
- 2Department of Animal Science, Faculty of Agriculture, University of Tabriz, Tabriz, Iran
- 3Department of Plant and Soil Sciences, University of Delaware, Newark, DE, USA
- 4Department of Food Biotechnology, Branch for Northwest & West region, Agricultural Biotechnology Research Institute of Iran (ABRII), Agricultural Research, Education and Extension Organization (AREEO), Tabriz, Iran
Photosynthetic microalgae are potentially yielding sources of different high-value secondary metabolites. Salinity is a complex stress that influences various metabolite-related pathways in microalgae. To obtain a clear view of the underlying metabolic pathways and resolve contradictory information concerning the transcriptional regulation of Dunaliella species in salt stress conditions, RNA-seq meta-analysis along with systems levels analysis was conducted. A p-value combination technique with Fisher method was used for cross species meta-analysis on the transcriptomes of two Dunaliella salina and Dunaliellatertiolecta species. The potential functional impacts of core meta-genes were surveyed based on gene ontology and network analysis. In the current study, the integration of supervised machine-learning algorithms with RNA-seq meta-analysis was performed. The analysis shows that the lipid and nitrogen metabolism, structural proteins of photosynthesis apparatus, chaperone-mediated autophagy, and ROS-related genes are the keys and core elements of the Dunaliella salt stress response system. Cross-talk between Ca2+ signal transduction, lipid accumulation, and ROS signaling network in salt stress conditions are also proposed. Our novel approach opens new avenues for better understanding of microalgae stress response mechanisms and for selection of candidate gene targets for metabolite production in microalgae.
Introduction
Microalgae are photosynthetic organisms that are considered potential sources of different secondary metabolites such as β-carotene and lipid (Alcantara et al., 2013; Klein et al., 2013). Microalgae produce these metabolites by harvesting sunlight and subsequently fixing CO2 using this energy. It has been proposed that efficiency of CO2 fixation and consequently the production rate of lipids and secondary metabolites are affected by different stresses such as salt, light, temperature, pH, and nutrient starvation (Takagi, 2006; Devi and Venkata, 2012). These are common stresses found in industrial production of microalgae and are usually considered to hamper production. In general, stress decreases the microalgae growth rate and biomass production, although it is well known that several stresses can be used to increase lipid and/starch accumulation; however, the increased accumulation per cells does not often make up for the lost cellular growth. Although attempts have been made to manipulate the stress response; however, progress has been limited due to the lack of understanding of the basic metabolism of algae and how the different stresses impact metabolic pathways (Shin et al., 2015).
It has been reported that salt stress induce the glycerol metabolism enzymes such as glycerol-3-phosphate phosphatase (GPP), glycerol 2-dehydrogenase (NADP+) (DHAR), and dihydroxyacetone kinase (DHAK) activity in Dunaliella salina (Breuer et al., 2013). Similar results have been obtained on enzymatic activities of fructose-bisphosphate aldolase (FBPA) involved in starch metabolisms (Klok et al., 2013). It has been noted that the enzymatic activities of ribulose-5-phosphate kinase (RuPK), ribulose-bisphosphate carboxylase (RuBisCO), phosphoglycerate kinase (PGK), and glyceraldehyde-3-phosphae dehydrogenase (GAPDH) involved in photosynthetic carbon fixation increase in stress condition (Ben‐Amotz, 1975; Beardall et al., 1976; Johnson et al., 1976;Wegmann, 1979).
Moreover, transcriptional regulation of metabolic enzymes is closely associated with the growth rate and physiological conditions (Brauer et al., 2008). So, stress-responsive transcripts can be populating with the slow growth and metabolite production.
It has been proposed that the transcription of enzymes involved in glycerol metabolisms and its potential carbon sources increases under salinity stress condition. Moreover, correlated transcriptional regulation of enzymes involved in glycerol metabolisms with the flow of pathways has been proposed (Fang et al., 2017). Transcriptomic study of Klebsormidium crenulatum has showed increase of sucrose synthase, sucrose phosphate synthase, and several enzymes involved in the biosynthesis of the raffinose family of oligosaccharides after desiccation stress (Holzinger et al., 2014).
However, literatures have showed contradictory findings about transcriptional regulation (Alkayal et al., 2010; Cui et al., 2010; Kim et al., 2010). These incongruences are mostly related to differences in severity, time range of treatments, and sample size (Farhadian et al., 2018b).
Due to the extensive application of RNA-seq technology for global expression analysis, the amount of deposited transcriptome data in stress condition is exponentially increasing. With the considerable increasing of deposited transcriptome data for the various physiological conditions, generalization of the major transcriptome regulatory mechanism is essential to provide meaningful and precise biological conclusions.
It has been proposed that combining the results of independent studies with meta-analysis can bypass the challenges associated with individual transcriptome studies (Sharifi et al., 2018). In the previous meta-analysis studies, differentially expressed genes (DEGs) involved in multiple stresses were identified (Ashrafi-Dehkordi et al., 2018). Kong et al. (2019) investigated a common transcriptional response to salt stress in different rice genotypes at the seedling stage. Wang et al. (2018a), Wang et al. (2018b) also identified the salt stress responding genes using transcriptome analysis in green algae Chlamydomonas reinhardtii and Dunaliella salina, respectively.
In the current study, for the first time, we integrated RNA-seq meta-analysis and supervised machine-learning models to detect and prioritize the salt stress responding genes and pathways which held common between two Dunaliella tertiolecta and D. salina species. Machine learning is the term of computer science in which computational statistics and information theory employ to construct algorithms that can learn from data (Wang et al., 2018a). The learning process refers to knowledge discovery that translate the features in the existing data sets into pattern (Yu et al., 2018). Machine learning has attracted wide attention for its various applications in modern biology such as cancer study (Akay, 2009), robust phenotyping (Platt, 1999), and transcriptome data analysis (Ebrahimi et al., 2014). Guo et al. (2016) applied the MinReg algorithm to infer the global gene regulatory networks in Fusarium graminearum on transcriptome datasets. Moreover, machine learning–based differential network analysis has been applied to predict stress-responsive genes (Wang et al., 2018a). Moreover, feasibility of supervised machine-learning models on bio-signature identification has been confirmed by Farhadian et al. (2018a) and Sharifi et al. (2018). We used various feature selection algorithms for modeling and ranking of common stress responding genes and proposed some important salt stress–responsive genes and pathways in two species of Dunaliella microalga.
Methods and Materials
Data Set Collection
RNA-seq raw reads were retrieved from the European Nucleotide Archive database. One D. salina and two D. tertiolecta datasets were selected for meta-analysis. The first dataset from D. tertiolecta (PRJNA385719) contains six biological samples which were grown in 0.08 M NaCl–treated ATCC media, harvested during stationary phase, and sequenced using Illumina MiSeq platform. The second dataset from D. tertiolecta (PRJNA51835) had five biological samples that were grown in 0.5 M NaCl were sequenced using Illumina GAIIx platform. The third dataset (PRJNA295823) contains reads from 18 salt–treated samples of D. salina. In this dataset, cells were grown in 0.5 M and harvested during stationary phase of growth for sequencing with Illumina HiSeq 2000 platform. In this work, samples that were treated with high salinity were included in our analysis.
RNA-seq and Differential Gene Expression Analysis
FastQC v0.11.5 (http://www.bioinformatics.babraham.ac.uk/projects/fastqc/) was used to assess quality of datasets, and reads were trimmed using Trimmomatic v0.32 (Bolger et al., 2014). The filtered reads were de novo assembled using Trinity v2.4.0 (Haas, 2013). The Trinity was run-in strand-specific mode (using the “—SS_lib_type RF” and “—SS_lib_type FR” options for D. tertiolecta and D. salina detests, respectively). Filtered reads from each biological sample were aligned to the de novo assembled transcripts using Kallisto (v0.44.0) with default parameters. Reads abundant per each transcript were normalized using fragment per kilo bases per million (FPKM), and the deferentially expressed genes (assembled transcripts) between treated and untreated samples were captured using Fisher model in edgeR package (Robinson et al., 2010). Significant differential expression was defined as a fold change ≥ |2| and a false discovery rate (FDR) corrected p-value ≤ 0.05 (Benjamin and Hochberg, 1995).
Orthology Definition and Meta-Analysis
Protein orthology was determined using Blastx (cutoff value of 6) against C. reinhardtii, Volvox carteri, and D. salina (https://phytozome.jgi.doe.gov/). The best hits were extracted with an in-house python scripts (Supplementary script S1). A meta-analysis was carried out on the integrated dataset to find the DEGs between two species. First, to reduce number of statistical tests and control of false positives, 10% of genes that have low expression levels and variance were excluded. A comparison between two classes for each species designed and moderated t-statistic with 1,000 random permutations carried out to define the genes with significant expression. The adjusted p-value (FDR <0.05) (Benjamini and Hochberg, 1995) were considered significant. P-value of DEGs in the each of the datasets was merged. To combine p-values of DEGs between two conditions, Fisher method was used. The log ratio of means (ROM) was applied to measure the gene expression values by following formula:
where represent ROM, mean expression level for each gene in dataset, respectively. The preprocessing and analysis were performed with the metaRNASeq package (Rau et al., 2014) of R software. A Venn diagram was generated using the ggplot2 package in R (Wickham, 2016).
Gene Ontology Enrichment and Functional Analysis
GO enrichment analysis of biological process (BP), molecular function (MF), and cellular component (CC) categories with p-value < 0.05 cutoff was performed using the Algal Functional Annotation tools (Lopez et al., 2011). Pathway enrichment of DEGs and meta-analysis results were visualized in MapMan software (Thimm, 2004).
Protein–Protein Network Analysis
Protein function information is critical for the elucidation of dynamics in complex processes (Panahi et al., 2014b; Panahi et al., 2015). This study used STRING database version 11.0 (https://string-db.org/) to predict protein–protein interactions networks from the DEGs. The k-means clustering algorithm was used for the functional module identification. Finally, identified modules were enriched using the KEGG database version 88.2.
Supervised Machine-Learning Models
Data cleaning on the merged dataset was conducted with useless and correlated attribute-removing approaches. The useless and correlated attributes (genes) were defined for genes with expression variation lower than 0.1 and correlation higher than 95%, respectively. Cleaned data subsequently were normalized, and the results from different weighting algorithms were presented as values between 0 and 1. Different attribute weighting algorithms including the information gain, information gain ratio, chi-squared, deviation, rule, SVM, Gini index, uncertainty, and relief were used as supervised machine-learning models to repeat ably investigation of the discrimination genes between the control and stress conditions in the Dunaliella spp. Two approaches were used to survey the species dependency or independency of identified meta-genes. For the first approach, models were run for each separate species while the stress treatment status was defined as a label variable. Discriminating genes that were shared by both species were defined as species-independent salt stress–responsive genes. In the second approach, the expression value (count data) and type of species (D. salina and D. tertiolecta) were set as features for attribute weighting while stress treatment status was defined as a label variable. The importance value of each feature calculates as (1-p) where p was the p-value of the feature selection test between the candidate predictor and the stress condition.
Results
De Novo Assembly
Strand-specific RNA sequencing data from each condition were pooled together for de novo assembly and subsequent gene expression analysis. In PRJNA385719 data set, 17,312 transcripts were matched to proteins based on our criteria. Moreover, transcript length ranged from 110 bps to 15,458 bps. Detailed assembly information of three data sets was provided in Table 1.
Metabolic Overview of Differentially Expressed Genes
The MapMan annotation tool was used to display potential metabolic impacts from DEGs the three different data sets (Figure 1 and Tables S1–S3). DEGs were annotated as minor carbohydrate, light reactions, sucrose and starch, lipid, amino acid, and TCA metabolism. The three experiments showed similar expression patterns for the metabolic genes although the amount of expression was different. For example, a putative PfkB-type carbohydrate kinase which participate in minor carbohydrate metabolism showed severe (fold change > 3), moderate (2 < fold change < 3), and lower (2 > fold change) down-regulation in PRJNA385719, PRJNA51835, and PRJNA295823, respectively. Of all the lipid metabolism genes, an acyl carrier protein thioesterase was dramatically up-regulated in all experiments. This is contrast to majority of lipid metabolism genes that were moderately down-regulated in the salt stress condition. Species-specific patterns were observed for the light reaction genes. In D. tertiolecta datasets, the moderate up- and down-regulated genes were uniformly observed whereas in D. salina dataset; most of light reactions underlying genes were moderately down-regulated in salt stress condition.
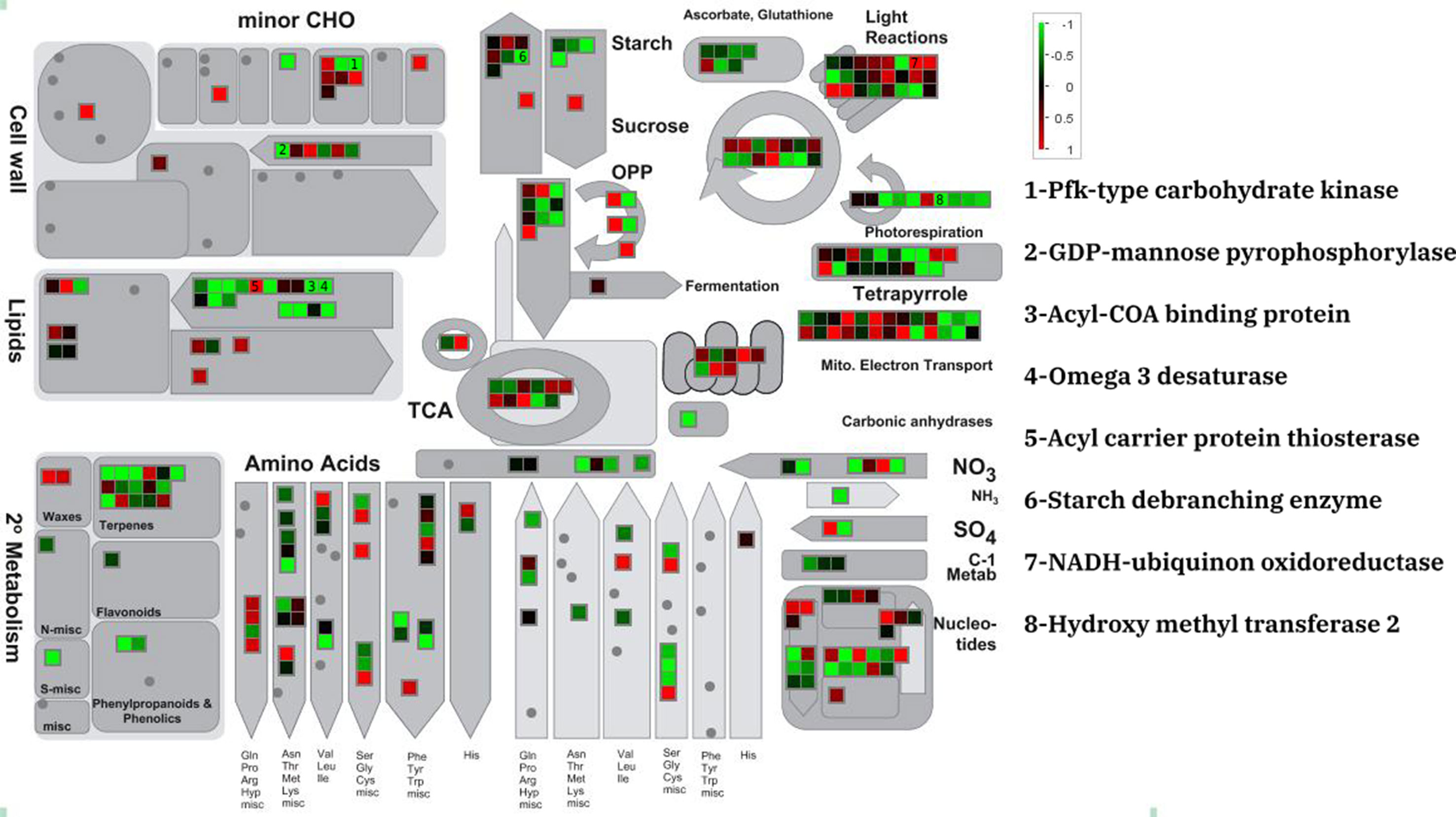
Figure 1 Metabolic overview of differentially expressed genes of D. tertiolecta (PRJNA51835) in responses to salt stress.
RNA-seq Meta-Analysis
Fisher method defined 49 differentially expressed transcripts representatives of 41 meta-genes (Figure 2). Details of identified meta genes and annotations were presented in Table 2. Of the 41 meta-genes, AMT1A, CLPD, and CLPB1, which encode ammonium transporter, chloroplast ClpD chaperone, and cytosolic ClpB chaperone, respectively, were up-regulated in salt stress conditions (Figure 3).
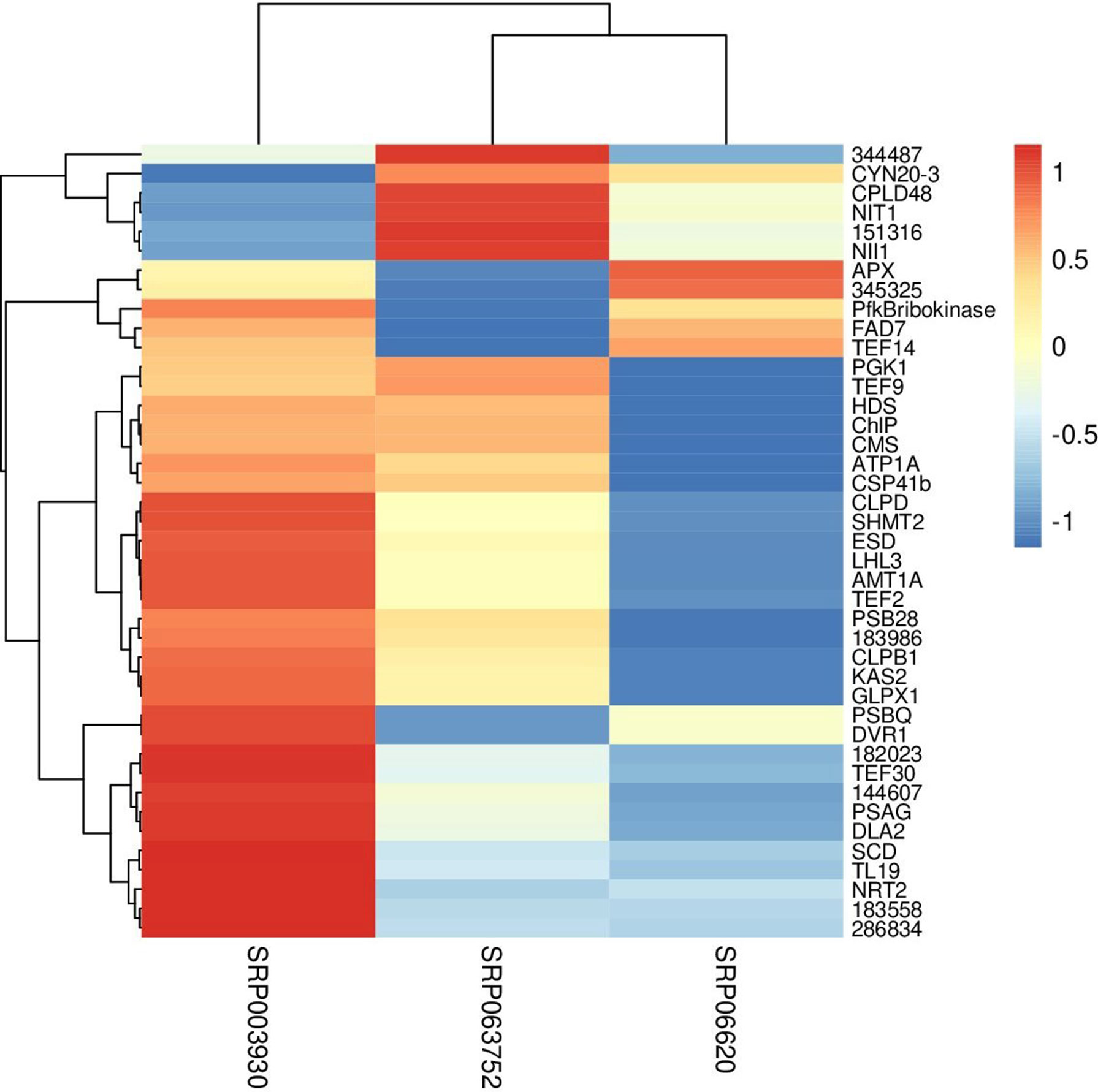
Figure 3 Clustering of metagenes based on expression patterns in three data sets. The fold changes were used as the expression value in constructing heatmap.
Functional Impacts of Meta-Genes Based on Gene Ontology and Network Analysis
Functional gene ontology analysis of identified meta-genes was conducted in three categories including biological process (BP), MF, and CCs (Table 3). In the biological process, fatty acid and carboxylic acid biosynthetic processes were enriched (Table 3). Regarding the MF categories, oxidoreductase activity was most prevalent, even though different functions such as CoA desaturase, fatty acid synthase, omega-3 fatty acid desaturase, stearoyl-CoA 9-desaturase, nitrate reductase (NADH), ferredoxin-nitrite reductase, and geranylgeranyl reductase activities were also enriched (Table 3).
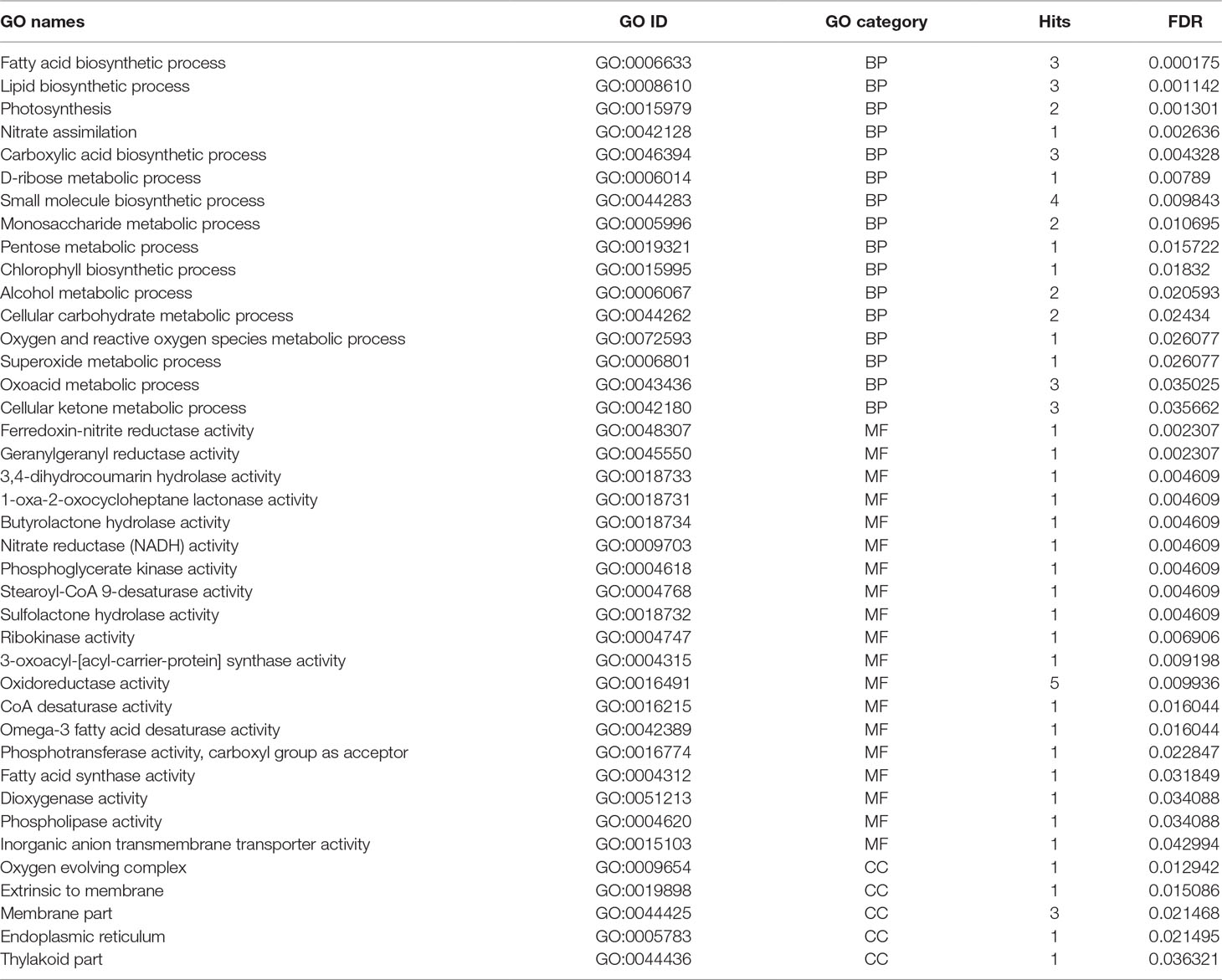
Table 3 Gene ontology enrichments of meta genes in three categories including BP (biological process), MF (molecular functions) and CC (cellular components), number of hits, and corresponding FDR value.
Protein–protein network of meta-genes based on co-expression and experimentally verified knowledge showed that 60% of identified meta-genes had a significant interaction with important functional modules, and remaining meta-genes had no other connections in the network (these nodes were removed from constructed network). Nitrogen metabolism, photosynthesis, oxidative phosphorylation, and splicing were the most important modules in the constructed network (Figure 4). We used a network modules analysis to investigate the core molecular networks that may be participating in biosynthesis of secondary metabolisms. Closer inspection of constructed networks revealed some important finding in Dunaliella responses to salt stress including 1) SHMT2 as important coordinator between nitrogen and carbon metabolism, photosynthesis, and secondary metabolite biosynthesis; 2) crosstalk between identified functional modules and splicing as a transcriptome plasticity mechanism; 3) anterograde-/retrograde-signaling networks importance in Dunaliella responses to salt stress condition; and 4) crosstalk between tetrapyrrole and secondary metabolite biosynthesis.
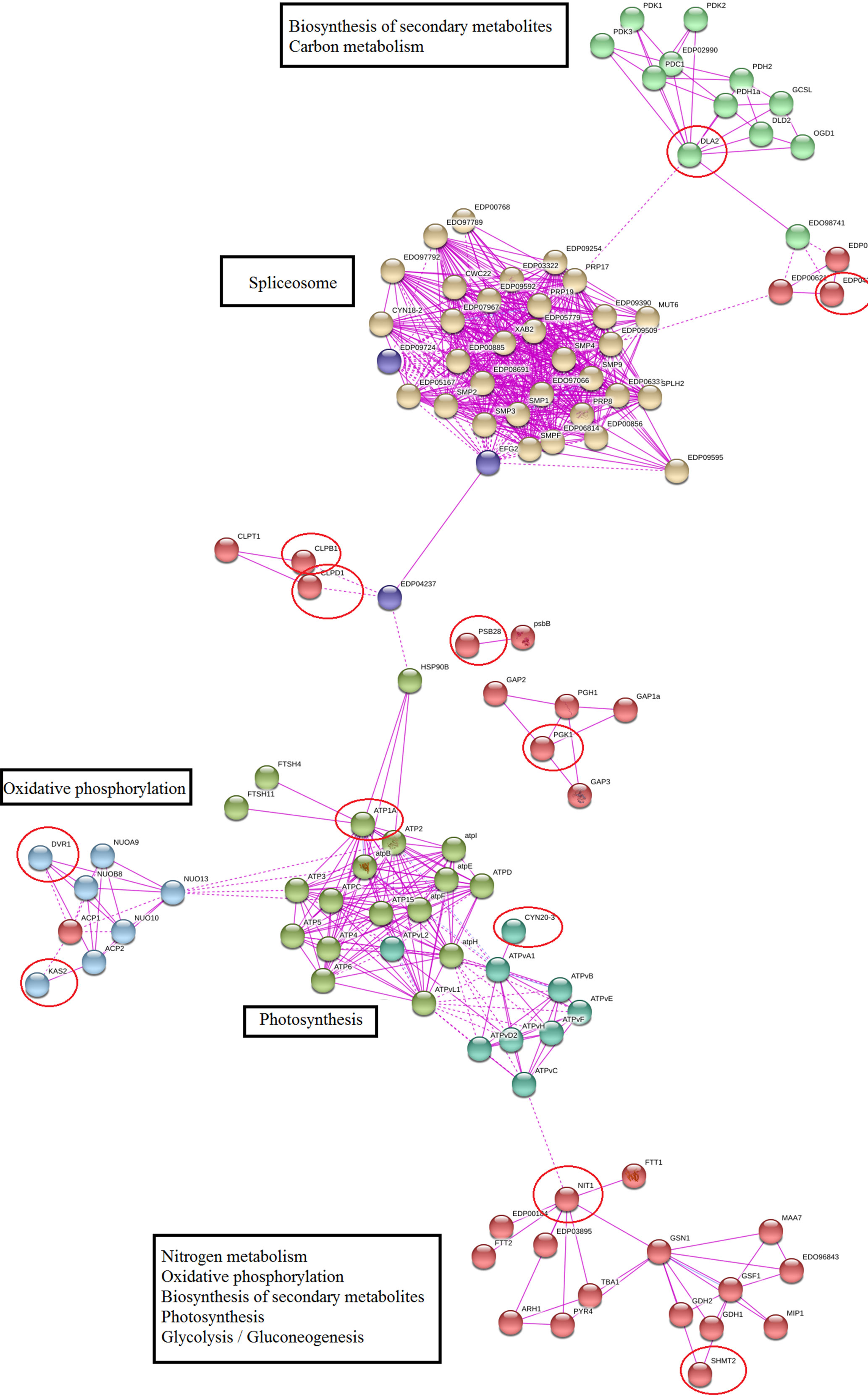
Figure 4 Protein–protein interaction network of meta-genes. The unconnected meta-genes were removed from constructed network. Meta-genes were signed by red circles.
Data Mining
Two hundred ninety-six attributes were selected from 2,900 common genes of merged file after data cleaning steps. The attributes with weight values higher than 0.5 were selected (Table S4). Results of species-specific analysis were also presented in Tables S5 and S6. Of the 41 meta-genes, 16 genes were selected by more than three weighting algorithms (Table 4). The verified meta-genes were related to photosynthesis (PSBQ, LHL3), lipid metabolism (ESD, KAS2), nitrogen metabolism (NIT1), ROS detoxification (APX, SHMT2), and retrograde-signaling network (DVR1, LHL3). Thereafter, the verified genes and pathways were defined as core and key salt stress–responsive genes and pathways in Dunaliella.
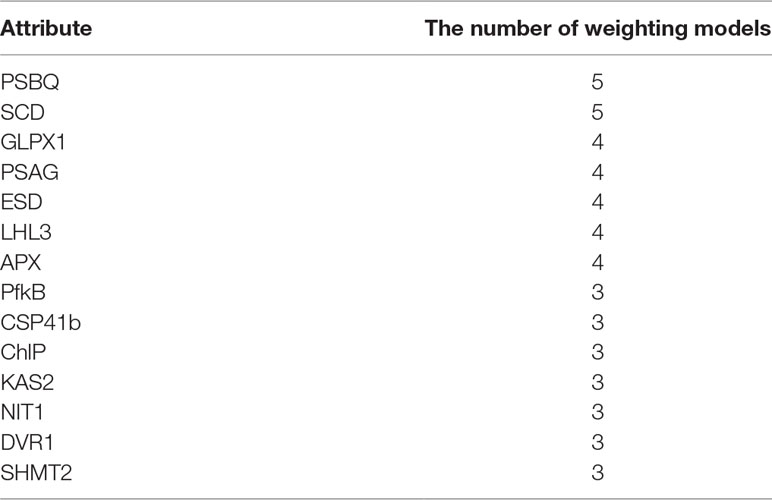
Table 4 Machine learning models based on attribute weighting algorithms demonstrated the most important salt stress responsive genes (species independent).
Discussion
Recently, high-throughput transciptomics data has helped increase the elucidation of the complexity of gene regulation in various abiotic stress conditions (Panahi et al., 2014b; Panahi et al., 2015). However, the complex interaction between genes and environment is not yet well understood. It has been proposed that integrative analysis of global gene expression data is effective approach for identification of key regulatory networks (Panahi et al., 2013; Shahriari Ahmadi et al., 2013; Farhadian et al., 2018a; Panahi et al., 2019). To our knowledge, this is the first study where multiple transcriptomic datasets under salt stress condition were used to probe the genetic response of the Dunaliella spp. In the current study, integration of supervised machine-learning algorithms with RNA-seq meta-analysis was proposed that lipid and nitrogen metabolism, structural proteins of photosynthesis apparatus, signaling, and ROS-related genes are the key and core elements of the Dunaliella salt stress response system.
Photosynthesis Machinery Structural Proteins as Important Salt Stress–Responsive Genes
Photosynthesis–related structural and functional proteins such as chloroplast stem-loop–binding protein (CSP41b), oxygen-evolving enhancer protein (PSBQ), photosystem II reaction center protein (PSB28), photosystem I reaction center subunit V (PSAG), thylakoid luminal protein (TEF14), and photosystem I chlorophyll a–/b–binding protein 2 (LHCA2) were all defined as meta-genes. These findings of the current study are consistent with those of Ji et al. (2018) who found that photosystem II (PSII) is one of the most sensitive components of the electron transport chain under stress condition (Ji et al., 2018). So, the presence of several photosystem structural genes as meta-genes in salt stress is not unsurprising; more importantly, some of these genes (PSBQ and PSB28) were defined as key salt stress–responsive genes (Table 2). The PSBQ protein is an extrinsic subunit of the PSII and is necessary for the regulation of both activity and assembly of PSII (Thornton et al., 2004; Summerfield et al., 2005). The down-regulation of PSBQ during salt stress in the three datasets also agrees with a previous study done on other Dunaliella spp. (Suorsa et al., 2006). The importance of PSBQ transcriptional in response to salt stress in Dunaliella was also confirmed by five different machine-learning algorithms (Table 4). Another PSII-related gene, PSB28 was also an important meta-genes for Dunaliella spp. PSB28 is involved in the biogenesis of PSII inner antenna CP47 (PsbB) and is essential for the protection of the reaction-center against high-light stress (Weisz et al., 2017). Our data suggests that PSB28 may also play a role in the salt stress response. The down-regulations of PSBQ and PSB28 may be an important adaptation response for microalgae against salt stress. In addition to PSII, photosystem I (PSI) was also affected by salt stress. PSI is composed of chlorophyll-binding core complex and a chlorophyll a–/b–binding peripheral antenna called light harvesting complex (LHCs). The results of transcriptome meta-analysis along with machine-learning weighting confirmed the importance of PSAG and LHCA2 in adaptation responses to salt stress condition (Table 2). It has been proposed that salt stress weakens the connection between LHCs and PSI and consequently reduces the conversion of light energy to chemical energy (Gupta et al., 2017). Our hypothesis has been also confirmed by recent study (Wang et al., 2019). Wang et al. (2019) found that salt stress induce protein interactions between FTSY-RPL13a-RPL18-EIF3A and chlL-chlN-rbcL-psaB-psaA-LHCB4-ATPvL1-atpI-cox1. The downregulation of rbcL, HSP90A, and LHC in the PPI network was also consistent with previous findings (Wang et al., 2019). It has also been found that chlorophyll a–/b–binding proteins such as LHCA2 are affected by light, oxidative stress, and chlorophyll retrograde signaling (Gupta et al., 2017). Downregulation of LHC under the stress condition corroborates these earlier finding that downregulation of the LHC under stress conditions is an attempt to minimize energy utilization by lowering photosynthetic demands (Xu et al., 2012). It has been proposed that these down regulations are attempting to minimize energy utilization by lowering photosynthetic demands. Additionally, decreased levels of chlorophyll a–/b–binding proteins were correlated with accumulation of ROS (Xu et al., 2012). Our data also confirms the coordinate response of chlorophyll a–/b–binding proteins, signaling, and ROS detoxification system–related genes (Tables 2 and 4 and Figure 4).
Contribution of ROS Scavenging and Signaling Pathways in Adaptation Network
In the present study, several meta-genes (APX, CLPB1, CLPD, LHL3, SHMT2, DVR1, and WD40, which encode ascorbate peroxidase, chaperone protein ClpB1 chaperone protein ClpD, Lhc-like protein, serine hydroxyl methyl transferase, protein DVR-1, and WD40 repeat-containing protein, respectively) were found as the main backbone of ROS and signaling network. Although different scavenging enzymes were up-regulated in response to salt stress, APX was the only enzyme selected as meta-genes in Dunaliella (Figure 1 and Table 2) and also verified by four machine learning–based weighting algorithms (Table 4). This may indicate that APX is more effective than other scavenging enzymes. Although there are no published reports comparing the efficiency of different algal scavenging enzymes in salt stress conditions, it has been reported that APX activity in halophyte plants is more important than other scavenging enzymes (Shalata et al., 2001; Sekmen et al., 2007; Panahi et al., 2014a). Due to the dual roles of ROS in toxicity and as signal molecules, Dunaliella species seems to have developed complex strategies to regulate and detoxify ROS in salt stress conditions. Meta-analysis and machine learning–based weighting algorithms analysis proposed that chaperone-mediated autophagy (CMA) is another important system for Dunaliella spp. to cope with salt stress conditions (Xiong et al., 2007).
CLPB1 and CLPD and DVR1 are other groups of important salt stress–responsive genes in Dunaliella. These chaperones are proposed to be involved in plastid protein quality control and degradation of oxidized proteins (Ramundo et al., 2014).
SHMT1 (serine hydroxyl methyl transferase 1), which regulates ROS generation by controlling photorespiratory pathways, was another important ROS signaling–related genes (Moreno et al., 2005). SHMT1 is known to influence resistance to different stress conditions and mutation of SHMT1 resulted in increased cell damage due to strong accumulation of H2O2 (Moreno et al., 2005). LHL3 (low molecular mass early light-induced protein) is proposed as an ROS protection system against oxidative damage and was identified as a meta-gene for Dunaliella spp. (Hutin et al., 2003). Additionally, the presence of spliceosome components and ROS signaling cascades in the meta-genes suggests cross-talk between these pathways (Figure 4), and this is reflected in a recent investigation showing that spliceosomal protein mutants are related with ROS accumulation (Gu et al., 2018).
Cross-Talk Between ROS Signaling Pathways, Lipid Biosynthesis, and Calcium Signal Transduction
Multiple studies reported that stress-induced lipid accumulation always correlates with an increase in antioxidant defenses systems (Hu et al., 2008; Zhao et al., 2015). In addition to their function in carbon and energy storage, lipids may act as antioxidants or protective defense molecules as part of the stress response (Hu et al., 2008). Our data also suggests this, since lipid metabolism–related genes responded transcriptionally to salt stress treatments in both species of Dunaliella (Figure 1). Particularly, KAS2 (3-ketoacyl-ACP-synthase) and FAD7 (chloroplast glycerol lipid omega-3-fatty acid desaturase) are implicated in the salt-induced response of lipid metabolism plasticity (Tables 2 and 4).
TEF2 which encodes a rhodanese-like Ca-sensing receptor was determined as another important gene in Dunaliella spp. responses to salt stress conditions (Table 2). It has been proposed that calcium-sensing receptors are important regulators of extracellular calcium content in which increases cytosolic Ca2+ concentration in stress conditions (Zhao et al., 2015). The co-occurrence in the meta-gene list as well as verification by machine-learning algorithms and network analysis of the calcium signal transduction gene TEF2 and lipid biosynthesis–related genes suggests that there may be potential cross-talking between Ca2+ signal transduction, lipid accumulation, and ROS signaling pathways in salt stress conditions. Similar cross-talking has been proposed for nitrogen starvation; so, it is feasible that similar pathways could be used for the salt stress responses also (Chen et al., 2014).
Transport and Assimilation of Nitrogen Are Important Coordinators for Adaptation Network
Excessive cytosolic NaH4+ concentration can induce the accumulation of ROS, oxidative damages, and subsequent membrane disruption in different eukaryotic cells (Shahriari Ahmadi et al., 2013). Flexibility in NaH4+ uptake mechanisms was proposed as one of the important acclimatization approaches in salt stress conditions (Abouelsaad et al., 2016). Among the different NaH4+ transporters and assimilation-related genes that were differentially expressed in that salt stress condition (Figure 1), AMT1A, NIT1, NII1, NAR3, NAR4, encoding ammonium transporter, nitrate reductase, nitrite reductase, and high-affinity nitrate transporter, respectively, were selected as meta-genes (Table 2). Based on expression profiles, the ammonium transporter was up-regulated while the nitrate transporters and nitrate reduction genes were downregulated. A recent transcriptome from Dunaliella viridis shows the same expression pattern when cells are grown with NH4+ as a nitrogen source (Dums et al., 2018), which might suggests a difference in nitrogen source between the different datasets used. However, the study done with salt tolerance in tomato shows ammonium transporter up-regulation and nitrate transporter down-regulation under salt stress (Abouelsaad et al., 2016). This equally reflects that data in this study. Regulation of inorganic nitrogen metabolism genes seems to contribute to the salt stress response and possibly could be tied into crosstalk with aforementioned pathways.
Conclusion
In conclusion, we identified a number of genes whose expression was putatively altered in the response to salt stress in two species of Dunaliella. The importance of identified responsive genes was validated with machine-learning algorithms, which mainly involved in ROS scavenging and signaling, chaperone-mediated autophagy, calcium signal transduction, and nitrogen metabolism. Furthermore, coordinate responses of chlorophyll a–/b–binding proteins, signaling, and ROS detoxification systems were highlighted by machine-learning and network analysis. PPI network analysis suggested the cross-talk between Ca2+ signal transduction, lipid accumulation, and ROS signaling pathways in salt stress conditions. Exploration of these signaling networks and additional knowledge about the identified meta-genes could provide new avenue for engineering of Dunaliella spp. for the production of a variety of secondary metabolites.
Data Availability
All datasets analyzed for this study are included in the manuscript and the supplementary files.
Author Contributions
Concept and design of the experiment: BP; Data analysis: BP and MF; Writing the manuscript: BP, JTD, and MAH.
Conflict of Interest Statement
The authors declare that the research was conducted in the absence of any commercial or financial relationships that could be construed as a potential conflict of interest.
Supplementary Material
The Supplementary Material for this article can be found online at: https://www.frontiersin.org/articles/10.3389/fgene.2019.00752/full#supplementary-material
References
Abouelsaad, I., Weihrauch, D., Renault, S. (2016). Effects of salt stress on the expression of key genes related to nitrogen assimilation and transport in the roots of the cultivated tomato and its wild salt-tolerant relative. Sci. Hortic. 211, 9–78. doi: 10.1016/j.scienta.2016.08.005
Alcantara, C., García-Encina, P. A., Munoz, R. (2013). Evaluation of mass and energy balances in the integrated microalgae growth-anaerobic digestion process. Chem. Eng. J. 221, 238–246. doi: 10.1016/j.cej.2013.01.100
Akay, M. F. (2009). Support vector machines combined with feature selection for breast cancer diagnosis. Expert. Syst. Appl. 36, 3240–3247.
Alkayal, F., Albion, R. L., Tillett, R. L., Hathwaik, L. T., Lemos, M. S., Cushman, J. C. (2010). Expressed sequence tag (EST) profiling in hyper saline shocked Dunaliella salina reveals high expression of protein synthetic apparatus components. Plant Sci. 179, 437–449. doi: 10.1016/j.plantsci.2010.07.001
Ashrafi-Dehkordi, E., Alemzadeh, A., Tanaka, N., Razi, H. (2018). Meta-analysis of transcriptomic responses to biotic and abiotic stress in tomato. PeerJ 6, e4631. doi: 10.7717/peerj.4631
Beardall, D., Mukerji, J., Glover, H. E., Morris, I. (1976). The path of carbon in photosynthesis by marine phytoplankton. J. Phycol. 11, 50–54. doi: 10.1111/j.1529-8817.1976.tb02864.x
Ben-Amotz, A. (1975). Adaptations of the unicellular alga Dunaliella parva to a saline environment. J. Phycol. 11, 50–54. doi: 10.1111/j.1529-8817.1975.tb02747.x
Benjamin, Y., Hochberg, Y. (1995). Controlling the false discovery rate: a practical and powerful approach to multiple testing. J. R. Stat. Soc. Ser. B Methodol. 57 (1), 289–300.
Bolger, A. M., Lohse, M., Usadel, B. (2014). Trimmomatic: a flexible trimmer for Illumina sequence data. Bioinformatics 30 (15), 2114-2120.
Brauer, M. J., Huttenhower, C., Airoldi, E. M., Rosenstein, R., Matese, J. C., Gresham, D., et al. (2008). Coordination of growth rate, cell cycle, stress response, and metabolic activity in yeast. Mol. Biol. Cell. 19, 352–367. doi: 10.1091/mbc.e07-08-0779
Breuer, G., Evers, W. A., de Vree, J. H., Kleinegris, D. M., Martens, D. E. (2013). Analysis of fatty acid content and composition in microalgae. J. Vis. Exp. 80, 1–9. doi: 10.3791/50628
Chen, H., Zhang, Y. M., He, C. L., Wang, Q. (2014). Ca2C signal transduction related to neutral lipid synthesis in an oil-producing green alga Chlorella sp. C2. Plant Cell Physiol. 55, 634–644. doi: 10.1093/pcp/pcu015
Cui, L., Xue, L., Li, J., Zhang, L., Yan, H. (2010). Characterization of the glucose-6-phosphate isomerase (GPI) gene from the halotolerant alga Dunaliella salina. Mol. Biol. Rep. 37, 911–916. doi: 10.1007/s11033-009-9717-x
Devi, M. P., Venkata, M. (2012). CO2 supplementation to domestic wastewater enhances microalgae lipid accumulate ion under mixotrophic microenvironment: effect of sparging period and interval. Bioresour. Technol. 112, 116–123. doi: 10.1016/j.biortech.2012.02.095
Dums, J., Murphree, C., Vasani, N., Young, D., Sederoff, H. (2018). Metabolic and transcriptional profiles of Dunaliella viridis supplemented with ammonium derived from glutamine. Front. Mar. Sci. 5, 311. doi: 10.3389/fmars.2018.00311
Ebrahimi, M., Aghagolzadeh, P., Shamabadi, N., Tahmasebi, A., Alsharifi, M., Adelson, D. L., et al. (2014). Understanding the underlying mechanism of HA-subtyping in the level of physic-chemical characteristics of protein. PLoS One 9, e96984.
Fang, L., Qi, S., Xu, Z., Wang, W., He, J., Chen, X., et al. (2017). De novo transcriptomic profiling of Dunaliella salina reveals concordant flows of glycerol metabolic pathways upon reciprocal salinity changes. Algal Res. 23, 135–149. doi: 10.1016/j.algal.2017.01.017
Farhadian, M., Rafat, S., Hasanpur, K., Ebrahimie, E. (2018a). Transcriptome signature of the lactation process, identified by meta-analysis of microarray and RNA-Seq data. BioTechnologia 99 (2), 153–163. doi: 10.5114/bta.2018.75659
Farhadian, M., Rafat, S. A., Hasanpur, K., Ebrahimi, M., Ebrahimie, E. (2018b). Cross-species meta-analysis of transcriptomic data in combination with supervised machine learning models identifies the common gene signature of lactation process. Front. Genet. 9, 235. doi: 10.3389/fgene.2018.00235
Gu, J., Xia, Z., Luo, Y., Jiang, X., Qian, B., Xie, H. (2018). Spliceosomal protein U1A is involved in alternative splicing and salt stress tolerance in Arabidopsis thaliana. Nucleic Acids Res. 46 (4), 1777–1792. doi: 10.1093/nar/gkx1229
Guo, L., Zhao, G., Xu, J. R., Kistler, H. C., Gao, L., Ma, L. J. (2016). Compartmentalized gene regulatory network of the pathogenic fungus Fusarium graminearum. New Phytol. 211, 527–541. doi: 10.1111/nph.13912
Gupta, S., Bhar, A., Chatterjee, M., Ghosh, A., Das, S. (2017). Transcriptomic dissection reveals wide spread differential expression in chickpea during early time points of Fusarium oxysporum f. sp. ciceri Race 1 attack. PLoS One 12 (5), e0178164. doi: 10.1371/journal.pone.0178164
Haas, B. J. (2013). De novo transcript sequence reconstruction from RNA-Seq: reference generation and analysis with Trinity. Nat. Protoc. 8, 1494–1512. doi: 10.1038/nprot.2013.084
Holzinger, A., Kaplan, F., Blaas, K., Zechmann, B., Komsic-Buchmann, K., Becker, B. (2014). Transcriptomics of desiccation tolerance in the streptophyte green alga Klebsormidium reveal a land plant-like defense reaction. PLoS One 9 (10), e110630. doi: 10.1371/journal.pone.0110630
Hu, Q., Sommerfeld, M., Jarvis, E., Ghirardi, M., Posewitz, M., Seibert, M. (2008). Microalgal triacylglycerols as feedstocks for biofuel production: perspectives and advances. Plant J. 54, 621–639. doi: 10.1111/j.1365-313X.2008.03492.x
Hutin, C., Nussaume, L., Moise, N., Moya, I., Kloppstech, K., Havaux, M. (2003). Early light-induced proteins protect Arabidopsis from photooxidative stress. Proc. Natl. Acad. Sci. 100, 4921. doi: 10.1073/pnas.0736939100
Ji, X., Cheng, J., Gong, D., Zhao, X., Qi, Y., Su, Y. (2018). The effect of NaCl stress on photosynthetic efficiency and lipid production in freshwater microalga Scenedesmus obliquus XJ002 . Sci. Total Environ. 633, 593–599. doi: 10.1016/j.scitotenv.2018.03.240
Johnson, M. K., Johnson, E. J., MacElroy, R. D., Speer, L., Bruff, B. (1976). Effects of salts on the halophilic alga Dunaliella viridis. J. Bacteriol. 95, 1461–1468.
Kim, M., Park, S., Polle, J. E., Jin, E. (2010). Gene expression profiling of Dunaliella sp. acclimated to different salinities. Phycol. Res. 58, 17–28. doi: 10.1111/j.1440-1835.2009.00554.x
Klein, M. D., Chisti, Y., Benemann, J. R., Lewis, D. (2013). A matter of detail: assessing the true potential of microalgal biofuels. Biotechnol. Bioeng. 110, 2317–2322. doi: 10.1002/bit.24967
Klok, A. J., Martens, D. E., Wijffels, R. H., Lamers, P. P. (2013). Simultaneous growth and neutral lipid accumulation in microalgae. Bioresour. Technol. 134, 233–243. doi: 10.1016/j.biortech.2013.02.006
Kong, W., Zhong, H., Gong, Z., Fang, X., Sun, T., Deng, X., et al. (2019). Meta-analysis of salt stress transcriptome responses in different rice genotypes at the seedling stage. Plants (Basel) 8 (3), 64. doi: 10.3390/plants8030064
Lopez, D., Casero, D., Cokus, S. J., Merchant, S. S., Pellegrini, M. (2011). Algal functional annotation tool: a web-based analysis suite to functionally interpret large gene lists using integrated annotation and expression data. BMC Bioinformatics 12, 282. doi: 10.1186/1471-2105-12-282
Moreno, J. I., MartõÂn, R., Castresana, C. (2005). Arabidopsis SHMT1, a serine hydroxymethyltransferase that functions in the photorespiratory pathway influences resistance to biotic and abiotic stress. Plant J. 41, 451–463. doi: 10.1111/j.1365-313X.2004.02311.x
Panahi, B., Abbaszadeh, B., Taghizadeghan, M., Ebrahimi, E. (2014b). Genome-wide survey of alternative splicing in Sorghum Bicolor. Physiol. Mol. Biol. Plants 20 (3), 323–329. doi: 10.1007/s12298-014-0245-3
Panahi, B., Mohammadi, S., Ebrahimie, E. (2014a). Identification of miRNAs and their potential targets in halophyte plant Thellungiella halophile. BioTechnologia 94 (3), 285–290. doi: 10.5114/bta.2013.46422
Panahi, B., Mohammadi, S. A., Khaksefidi, R. E., Mehrabadi, J. F., Ebrahimie, E. (2015). Genome-wide analysis of alternative splicing events in Hordeum vulgare: highlighting retention of intron-based splicing and its possible function through network analysis. FEBS Lett. 589, 3564–3575. doi: 10.1016/j.febslet.2015.09.023
Panahi, B., Mohammadi, S. A., Ruzicka, K., Abbasi Holaso, H., Zare Mehrjerdi, M., (2019). Genome-wide identification and co-expression network analysis of nuclear factor-Y in barley revealed potential functions in salt stress. Physiol. Mol. Biol. Plants 25 (2), 485–495. doi: 10.1007/s12298-018-00637-1
Panahi, B., Shahriari Ahmadi, F., Zare Mehrjerdi, M., Moshtaghi, N. (2013). Molecular cloning and the expression of the Na+/H+ antiporter in the monocot halophyte Leptochloa fusca (L). Kunth. NJAS-Wageningen J. Life Sci. 64, 87–93. doi: 10.1016/j.njas.2013.05.002
Platt, J. C. (1999). “Probabilistic outputs for support vector machines and comparisons to regularized likelihood methods” in Advances in large margin classifiers. Eds Smola, A. J., Bartlett, P., Schölkopf, B., Schuurmans, O. (Cambridge, MA: MIT Press), pp. 61–74.
Ramundo, S., Casero, D., Muhlhaus, T., Hemme, D., Sommer, F., Crevecoeur, M. (2014). Conditional depletion of the Chlamydomonas chloroplast ClpP protease activates nuclear genes involved in autophagy and plastid protein quality control. Plant Cell 26 (5), 2201–2222. doi: 10.1105/tpc.114.124842
Rau, A., Marot, G., Jaffrézic, F. J. B. B. (2014). Differential meta-analysis of RNA-seq data from multiple studies. BMC Bioinformatics 15, 91. doi: 10.1186/1471-2105-15-91
Robinson, M. D., McCarthy, D. J., Smyth, G. K. (2010). edgeR: a bioconductor package for differential expression analysis of digital gene expression data. Bioinformatics 26, 139–140. doi: 10.1093/bioinformatics/btp616
Sekmen, A. H., Turkana, I., Takiob, S. (2007). Differential responses of antioxidative enzymes and lipid peroxidation to salt stress in salt-tolerant Plantago maritime and salt-sensitive Plantago media. Physiol. Plant 131, 399–411. doi: 10.1111/j.1399-3054.2007.00970.x
Shahriari Ahmadi, F., Panahi, B., Marashi, H., Moshtaghi, N., Mirshamsi Kakhki, A. (2013). Coordinate up-regulation of vacuolar Na+/H+ antiporter and V-PPase to early time salt stress in monocot halophyte Leptochloa fusca roots. J. Agric. Sci. Technol. 15, 369–376.
Shalata, A., Mittova, V., Volokita, M., Guy, M., Tal, M. (2001). Response of the cultivated tomato and its wild salt-tolerant relative Lycopersicon pennellii to salt-dependent oxidative stress: the root antioxidative system. Physiol. Plant 112, 487–494. doi: 10.1034/j.1399-3054.2001.1120405.x
Sharifi, S., Pakdel, A., Ebrahimi, M., Reecy, J. M., Fazeli Farsani, S., Ebrahimie, E. (2018). Integration of machine learning and meta-analysis identifies the transcriptomic bio-signature of mastitis disease in cattle. PLoS One 13 (2), e0191227. doi: 10.1371/journal.pone.0191227
Shin, H., Hong, S. J., Kim, H., Yoo, C., Lee, H., Choi, H. K., et al. (2015). Elucidation of the growth delimitation of Dunaliella tertiolecta under nitrogen stress by integrating transcriptome and peptidome analysis. Bioresour. Technol. 194, 57–66. doi: 10.1016/j.biortech.2015.07.002
Summerfield, T. C., Shand, J. A., Bentley, F. K., Eaton-Rye, J. J. (2005). PsbQ (Sll1638) in Synechocystis sp. PCC 6803 is required for photosystem II activity in specific mutants and in nutrient-limiting conditions. Biochemistry 44 (2), 805–815. doi: 10.1021/bi048394k
Suorsa, M., Sirpiö, S., Allahverdiyeva, Y., Paakkarinen, V., Mamedov, F., Styring, S. (2006). PsbR, a missing link in the assembly of the oxygen-evolving complex of plant photosystem II. J. Biol. Chem. 281 (1), 145–150. doi: 10.1074/jbc.M510600200
Takagi, M. (2006). Effect of salt concentration on intracellular accumulation of lipids and triacylglycerides in marine microalgae Dunaliella cells. J. Biosci. Bioeng. 101, 223–226. doi: 10.1263/jbb.101.223
Thimm, O. (2004). MAPMAN: a user-driven tool to display genomics data sets onto diagrams of metabolic pathways and other biological processes. Plant J. 37, 914–939. doi: 10.1111/j.1365-313X.2004.02016.x
Thornton, L. E., Ohkawa, H., Roose, J. L., Kashino, Y., Keren, N., Pakrasi, H. B. (2004). Homologs of plant PsbP and PsbQ proteins are necessary for regulation of photosystem II activity in the Cyanobacterium synechocystis 6803. Plant Cell 16 (8), 2164–2175. doi: 10.1105/tpc.104.023515
Wang, L., Xi, Y., Sung, S., Qiao, H. (2018a). RNA-seq assistant: machine learning based methods to identify more transcriptional regulated genes. BMC Genomics 19 (1), 546. doi: 10.1186/s12864-018-4932-2
Wang, N., Qian, Z., Luo, M., Fan, S., Zhang, X., Zhang, L. (2018b). Identification of salt stress responding genes using transcriptome analysis in green alga Chlamydomonas reinhardtii. Int. J. Mol. Sci. 19 (11), 3359. doi: 10.3390/ijms19113359
Wang, Y., Cong, Y., Wang, Y., Guo, Z., Yue, J., Xing, Z., et al. (2019). Identification of early salinity stress-responsive proteins in Dunaliella salina by isobaric tags for relative and absolute quantitation (iTRAQ)-based quantitative proteomic analysis. Int. J. Mol. Sci. 20 (3), 599. doi: 10.3390/ijms20030599
Wegmann, K. (1979). Biochemical adaption of Dunaliella tertiolecta to salinity and temperature changes. Ber Dtsch. Bot. Ges. 92, 43–54.
Weisz, D. A., Liu, H., Zhang, H., Thangapandian, S., Tajkhorshid, E. (2017). Mass spectrometry-based cross-linking study shows that the Psb28 protein binds to cytochrome b559 in photosystem II. Proc. Natl. Acad. Sci. U.S.A. 114 (9), 2224–2229. doi: 10.1073/pnas.1620360114
Wickham, H. (2016). ggplot2: Elegant graphics for data analysis. New York: Springer-Verlag. ISBN: 978-3-319-24277-4. doi: 10.1007/978-3-319-24277-4
Xiong, Y., Contento, A. L., Nguyen, P. Q., Bassham, D. C. (2007). Degradation of oxidized proteins by autophagy during oxidative stress in Arabidopsis. Plant Physiol. 143 (1), 291–299. doi: 10.1104/pp.106.092106
Xu, Y.-H., Liu, R., Yan, L., Liu, Z.-Q., Jiang, S.-C., Shen, Y.-Y. (2012). Light-harvesting chlorophyll a/b-binding proteins are required for stomatal response to abscisic acid in Arabidopsis. J. Exp. Bot. 63, 1095–1106. doi: 10.1093/jxb/err315
Yu, Y., Wang, J., Ng, C. W., Ma, Y., Mo, S., Fong, E. L. S., et al. (2018). Deep learning enables automated scoring of liver fibrosis stages. Sci. Rep. 8 (1), 16016.
Keywords: Dunaliella, RNA-seq meta-analysis, machine learning, network, retrograde signaling, ROS, tetrapyrrole
Citation: Panahi B, Frahadian M, Dums JT and Hejazi MA (2019) Integration of Cross Species RNA-seq Meta-Analysis and Machine-Learning Models Identifies the Most Important Salt Stress–Responsive Pathways in Microalga Dunaliella. Front. Genet. 10:752. doi: 10.3389/fgene.2019.00752
Received: 06 April 2019; Accepted: 17 July 2019;
Published: 29 August 2019.
Edited by:
Juan Caballero, Universidad Autónoma de Querétaro, MexicoReviewed by:
Hamed Bostan, North Carolina State University, United StatesHemant Ritturaj Kushwaha, Jawaharlal Nehru University, India
Copyright © 2019 Panahi, Frahadian, Dums and Hejazi. This is an open-access article distributed under the terms of the Creative Commons Attribution License (CC BY). The use, distribution or reproduction in other forums is permitted, provided the original author(s) and the copyright owner(s) are credited and that the original publication in this journal is cited, in accordance with accepted academic practice. No use, distribution or reproduction is permitted which does not comply with these terms.
*Correspondence: Bahman Panahi, panahibahman@ymail.com; b.panahi@abrii.ac.ir