- 1Medical Genomics Research Department, King Abdullah International Medical Research Center, Riyadh, Saudi Arabia
- 2King Saud Bin Abdulaziz University for Health Sciences, Riyadh, Saudi Arabia
- 3Division Heart and Lungs, Department of Cardiology, University Medical Center Utrecht, Utrecht University, Utrecht, Netherlands
- 4Department of Clinical Pharmacy, University Medical Center Utrecht, Utrecht, Netherlands
- 5Faculty of Population Health Sciences, Institute of Cardiovascular Science, University College London, London, United Kingdom
- 6Health Data Research UK and Institute of Health Informatics, University College London, London, United Kingdom
Introduction: The Dutch Pharmacogenetics Working Group (DPWG) indicated a list of actionable genotypes that affect patients’ response to more 50 drugs; these drugs which show variable effects based on patients’ genetic traits were named as pharmacogenetics (PGX) drugs. Preemptive genetic testing before using these drugs may protect certain patients from serious adverse reactions and could help in avoiding treatment failures. The objectives of this study include identifying the rate of PGX drug usage among Dutch population, estimating the level of users who carry the actionable genotypes and determining the main genes involved in drug’s effect variability.
Methods; Usage of PGX drugs over 2011–2017 by the insured population (an average of 11.4 million) in outpatient clinics in Netherlands was obtained from the publically available GIP databank. The data of 45 drugs were analyzed and their interactions with selected pharmacogenes were estimated. Frequency of actionable genotypes of 249 Dutch parents was obtained from the public database: Genome of Netherlands (GoNL), to identify the pattern of genetic characteristics of Dutch population.
Results: Over a 7 year period, 51.3 million exposures of patients to PGX drugs were reported with an average of 5.3 exposures per each drug user. One quarterof the exposures (12.4 million) are predicted to be experienced by individuals with actionable genotypes (risky exposures). Up to 60% of the risky exposures (around 7.5 million) were related to drugs metabolized by CYP2D6. SLCO1B1, and CYP2C19 were also identified among the top genes affecting response of drugs users (involved in about 22 and 12.4% of the risky exposures, respectively). Cardiovascular medications were the top prescribed PGX drug class (43%), followed by gastroenterology (29%) and psychiatry/neurology medications (15%). Women use more PGX drugs than men (55.8 vs. 44.2%, respectively) with the majority (84%) of users in both sexes are above 45 years.
Conclusion: PGX drugs are commonly used in Netherlands. Preemptive panel testing for CYP2D6, SLCO1B1, and CYP2C19 only could be useful to predict 95% of vulnerable patients’ exposures to PGX drugs. Future studies to assess the economic impact of preemptive panel testing on patients of older age are suggested.
Introduction
Variability in patients’ response to drug treatment represents a major challenge to medical researchers, clinical practitioners and drug regulatory agencies (Ingelman-Sundberg et al., 2018). The variability may occur as a result of a carriage of different genetic traits that interact with the administered drugs; this interaction may lead to significant drug toxicities and/or lack of drug efficacy (Roses, 2000). Genotypes with potential interactions with certain drugs that necessitate therapeutic intervention or modification such as increasing or decreasing drug dose, stopping the drug or using an alternative are titled as actionable genotypes (Bain et al., 2018). These medications which show variable effects based on patients’ genetic traits can be described as pharmacogenetic (PGX) drugs (Carpenter et al., 2016). Several PGX tests are currently available which allow clinicians to predict a patient’s response to many drug therapies including central nervous system (CNS) agents, cardiovascular, endocrinology agents and many others (Vivot et al., 2015). Action of important therapeutic classes of drugs such as anticoagulants, anti-infective agents, and some pain relievers was confirmed to be affected by multiple interactions with numerous genetic variations. Carriage of such variations may put patients at risk of developing adverse drug reactions (ADRs) (Collins et al., 2016).
The majority of known ADRs, 60% approximately, are triggered via exposure to drugs that are metabolized by enzymes with altered functions induced by genetic mutations (Alagoz et al., 2016). Such types of ADRs which may initiate or extend hospital stays are potentially avoidable. Previous studies have investigated the genotypes frequency of a number of pharmacogenes including CYP2C9, CYP2C19, CYP2D6, SLCO1B1, TPMT, and VKORC1 which showed that more than 90% of individuals carry at least one actionable genotype (Van Driest et al., 2014; Ji et al., 2016). Therefore, implementation of PGX testing has the potential to prevent occurrence of serious drug related events; in some cases leading to death. Prior genetic testing may reduce the episodes of hospitalization as a result of ADR, and minimize the cost of treating avoidable medical conditions (Wong et al., 2010).
The international Clinical Pharmacogenetics Implementation Consortium (CPIC) reported 358 interactions between 127 genes and 226 drugs, and established the needed therapeutic guidelines for 52 drugs up to present1. Similarly, the clinical guidelines established by Dutch Pharmacogenetics Working Group (DPWG) identified significant actionable genotypes that affect patients’ response to more than 50 drugs (Samwald et al., 2016). Specific genotyping tests can now be carried out for the reported drugs to protect highly susceptible individuals from serious adverse drug toxicities, to identify low or non-responders and choose appropriate genetic based doses. This is known as personalized medicine where health care providers could tailor medical treatment based on patient’s genetic characteristics (Relling and Klein, 2011; Swen et al., 2011).
Prior knowledge of drug-gene interactions can help in gaining a better understanding of drugs’ mechanism of actions and provide insights for pharmaceutical manufacturer to develop new safer and more effective drugs (Karczewski et al., 2012). Physicians’ compliance with the PGX guidelines and their adherence to the recommendations suggested for each genetic test result is expected to impact treatment effectiveness which may reduce the burden of overall treatment cost. The extent of cost reduction related to ordering PGX tests depends on how common polymorphisms in pharmacogenes exist in certain patient populations, the pattern of diseases and the pattern of drug prescribing in each community (Verbelen et al., 2017).
Despite the determination of important drug-gene interactions, implementation of these findings into clinical practice is still in its early stage; therefore, more efforts are needed to illustrate the benefits of using such PGX tests. Well-designed studies are required to show the potential return of PGX testing prior to drug prescribing and provide detailed therapeutic and cost-effective advantages based on variable demographic data (Cecchin et al., 2017).
This study aimed to determine (1) the major genes involved in drug-gene interactions, (2) the percentage of Dutch population using the drugs listed by DPWG and frequency of genetic variants that affect PGX drugs, in addition to (3) identifying the main prescribed PGX drug category.
Methodology
The following steps have been taken to estimate actionable genotypes and PGX drug consumption:
(1) Frequency of risk alleles involved in drug interactions among Dutch population was obtained from the public database: (Genome of Netherlands, 2014) (GoNL)2. This was used to identify the portion of people at high risk of response alteration when exposed to PGX drugs. Four Dutch biobanks in Amsterdam, Groningen, Leiden, and Rotterdam provided the blood samples for genetic screening. Detailed demographic data of the participants are described by Boomsma et al. (2014). The genetic characteristics available in GoNL database are limited to the Dutch and those with European ancestry only. They represent 85% of the population living in Netherlands (79.3% Dutch and 5.7% Europeans) (Netherlands Population, 2018). 769 Dutch individuals (250 trios) took part in the GoNL project but the genetic dataset shown in this study involved only the genetic profiles of the parents only (n = 498; 249 fathers and 249 mothers [average age 63.8 (46–87) and 61.7 (43–86), respectively]. Children data was excluded to avoid the possible confounding effect (bias); since they inherit their genes from their parents. Whole-genome sequencing was performed by BGI Hong Kong. To ensure that GoNL provided accurate estimates of Dutch genotyping data, the results reported in GoNL will be compared to a recent study (Mizzi et al., 2016) that used different genotyping platform (affymetrix DMETTM Plus) and validated its results through using TaqMan SNP Genotyping Assays and/or Sanger sequencing. Mizzi and co-authors investigated selected pharmacogenes among European population, of them 349 were Dutch. GoNL data will also be compared to the Royal Dutch Pharmacists Association (KNMP) genetic database3 for variants not reported in Mizzi’s study.
(2) GIP databank4, was used to identify the figures of PGX drug usage over the period 2011–2017 by the insured population (an average of 11.4 million) in primary care in Netherlands. Since part of the resident in Netherlands (15%) is not Caucasian (Netherlands Population, 2018), this percentage will be deducted out of the net drug consumption results. Drug consumption data are classified per items issued and per drug users. Repetitive use of a PGX drug by each user was counted as one exposure even though the patient used multiple prescriptions of the same drug.
(3) The consumption data of 45 PGX drugs, described in Table 3, were analyzed and estimations of their interactions with most common genes were made. The PGX drugs listed by DPWG are more than 45 but some of them were excluded due to:
(a) Restricted to hospital use, e.g., irinotecan (our data focused on outpatient care only).
(b) Marketed recently (after 2011) in Netherlands, e.g., eliglustat, marketed in 2015.
(c) Not marketed in Netherlands, e.g., desipramine and warfarin.
(4) Consumption during 2015 and 2017 was further stratified per patient’s sex and different age groups to determine variances in PGX drug consumption between different patients’ categories. The stratified data of other years are not available so we used the 2-year data as an example of the pattern of drug usage rate per different age groups. This has enabled us to pay more attention on the age group of patients with high use of PGX drugs.
Genotypes were translated into predicted phenotypes, e.g., Normal (N), Intermediate (I), Poor (P), or Ultrarapid (U) according to the DPWG guidelines (see text footnote 2).
Results
Genotyping data of 498 Dutch parents for the common genes, predicted to influence drug response, extracted from the GoNL database are shown in Table 1. The reported results are in line with the Mizzi et al. (2016) findings of 349 Dutch healthy individuals. The screened numbers in both studies may not considered to be representative of the whole population. However, consistency of genetic datasets between both studies provides confidence about the trends of pharmacogenes among the Dutch population. Mizzi et al. (2016) examined only seven genes described in this project. Of which, frequency distribution of 18 SNPs were provided; all showed similar results to GoNL data except CYP2C9*3 [Minimum allele frequency (MAF) = 0.20 in Dutch population in Mizzi’s study versus 0.07 in GoNL, P = 0.0001]. The average MAF of CYP2C9*3 among the tested samples for the other 17 European countries was 0.08 which is consistent to MAF found in GoNL. Also, The frequency of CYP2C9*3 shown in KNMP database is in line with the GoNL data, although it is slightly higher (MAF = 0.09 vs. 0.07, respectively).
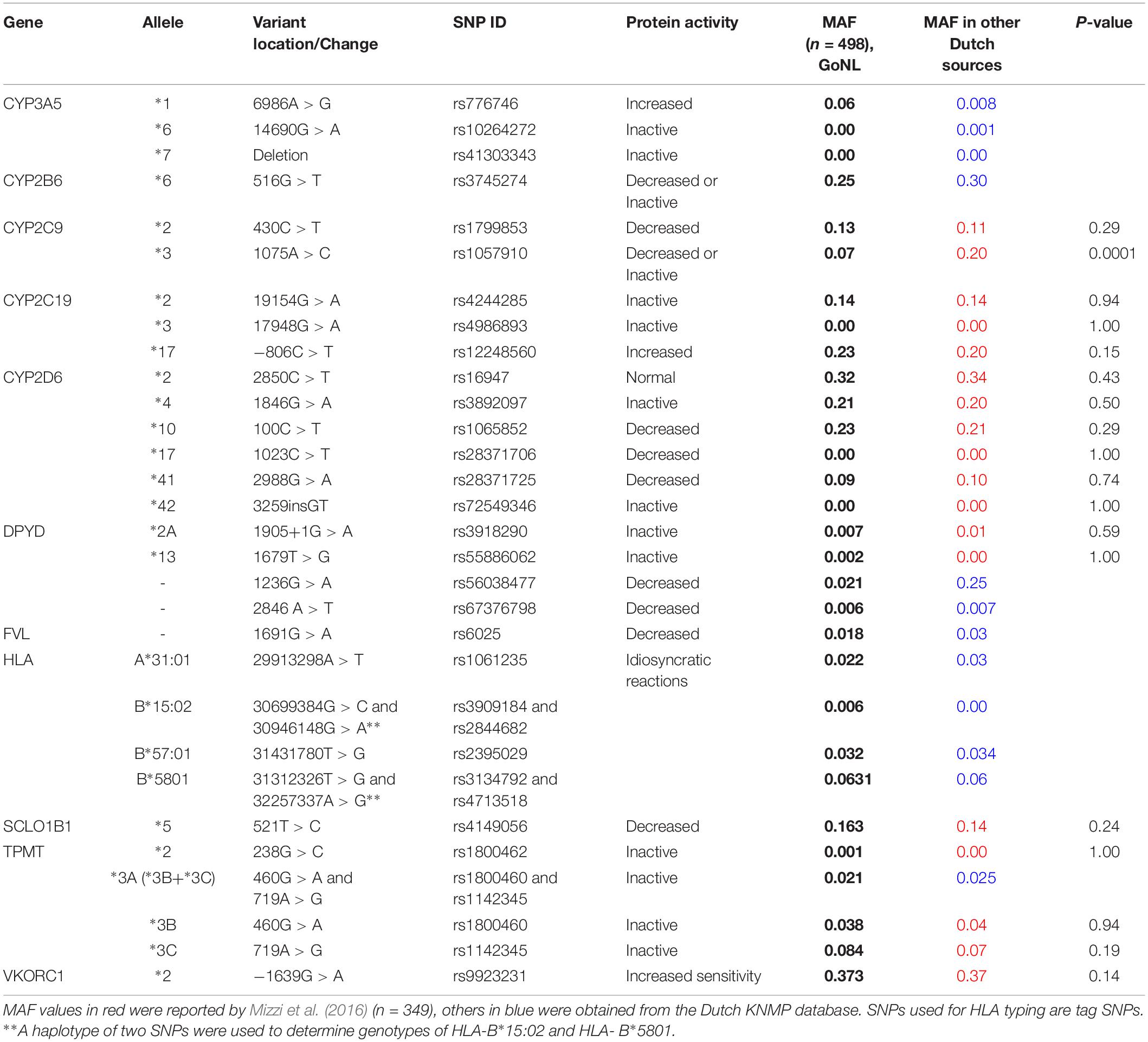
Table 1. Frequency of the common variants located in pharmacogenes among Duch population: GoNL data versus other Dutch genetic databases.
The levels of genes’ phenotypes were predicted based on the genotypes frequency extracted from GoNL, Table 2. To determine the ultrarapid phenotype of CYP2D6, further knowledge of the copy number of the gene is needed but this was not provided by GoNL; therefore, the frequency of this important phenotype was obtained from KNMP database. MAF of the variants in the pharmacogenes shown in KNMP dataset match the data seen in GoNL. Only 3 out of the tested participants (0.6%) in GoNL were negative for all actionable variants while the vast majority were found carrying at least one or more risky genotypes. Among them, positivity to two, three, four or five genotypes were 18.5, 30, 25, and 16%, Figure 1.
Over a 7 year period, prescribing of PGX drugs, in total, represented around one quarter (24%) of the total drugs used (384.4 million PGX items issued out of 1,600.2 million prescriptions used), Table 3. Usage of these quantities resulted in 51.3 million exposures of 9.7 million patients [individuals with European ancestry only (85%)] to PGX drugs; an average of 5.3 exposures are estimated per each drug user, Table 4. Of those exposures, one quarter (12.38 million, 24.1%) have clinical significance that may result in reduced drug efficacy or possibly lead to ADRs. Among the screened pharmacogenes interacting with PGX drugs, CYP2D6 was determined as the top gene influencing patients’ response. More than seven million exposures are predicted to involve users with CYP2D6 actionable genotypes which represent 60% of the total risky exposures over 7-year interval. Also, the polymorphisms in SLCO1B1, which codes for statin transporter protein, and CYP2C19, that encode a metabolizing enzyme of multiple drugs, have shown high rates of interaction with used drugs in Netherlands (about 22% and 12.35% of the significant exposures, respectively).
Cardiovascular drugs such as statins, e.g., simvastatin and atorvastatin were the top PGX drug class prescribed (43%), followed by gastroenterology agents, in particular proton pump inhibitors (PPI), e.g., omeprazole and pantoprazole, and psychiatry/neurology medications (29% and 15%, respectively), Figure 2. Use of analgesic/anesthetics medications were rated as fourth class (7%). Metoprolol and simvastatin were the most issued PGX drugs, followed by omeprazole and pantoprazole (16, 15, 14, and 10%, respectively).
Further analysis of combined use in 2015 and 2017 based on different sex showed higher level of PGX drugs usage by women than men (55.8% for women vs. 44.2% for men), Table 5. Intake of these medications was found to be more common in older age groups than younger age groups. The top drug users in both sexes were the group aged between 45 and 64 years (percentage of usage was 36 and 31% for men and women, respectively). In total, more than 84% of PGX drug prescriptions were issued to patients older than 45 years.
Discussion
Usage of PGX drugs may expose patients to increased risk for ADR (Wang, 2010). In addition, treatment failures are expected when drugs are used without prior identification of the exact patients’ genetic characteristics (Blakey and Hall, 2011). Ordering genetic testing to all patients prior to usage of PGX drugs might be the ideal approach to avoid preventable drug toxicities and to improve drug efficacy, therefore, its implementation is the ultimate mean to accelerate practicing precision medicine. Although, preemptive genetic testing is highly recommended (Caudle et al., 2014; Bank et al., 2018b) to predict patient safety, its high cost may prevent health care authorities to propose it as a standard practice in health institutes. The burden on health budget will be huge when genotyping of every patient is requested. Therefore, at this stage, determining the level of consumption of these specific drugs could guide the prioritization process of patients who are of utmost need for genetic testing.
This study is intended to assess the frequency of actionable genotypes among Dutch population and identify the rate of usage of PGX drugs and further identify the frequent users based on their disease type, age group, and sex. The findings of the study were used to set up a recommendation that focuses on certain age groups. A matter of utmost importance, this study shortlisted the main genes of interest which affect the majority of used PGX drugs based on actual large scale drug usage in Netherlands. In contrast to our approach, the previous studies conducted by Van Driest et al. (2014) and Ji et al. (2016) suggested a panel of genes based on their understanding of gene functions and their knowledge of interaction between gene products and the selected PGX drugs. Familiarity with functional consequences of actionable genotypes of different pharmacogenes is needed to identify the most important genes for preemptive testing, however, incorporating this knowledge with the information of PGXs drugs usage rate represents a better method of guided selection of gene panels since it reflects the real need for drug-related genetic testing in each unique population. As a result of different type of diseases and drug prescribing patterns in other countries, such project is suggested among population with different ethnicities to determine the best pharmacogenes for preemptive testing.
Previous surveillance in the United States revealed that 91% of European Americans (EA) and 96% of African Americans (AA) (average age is 64 and 60, respectively) carry genetic variations that could put them at risk of an ADR upon receiving PGX drugs (Van Driest et al., 2014), these risks are predictable and can be prevented when patients being tested for prior to drug administration. In addition, a more recent study that involved 1013 non-Hispanic white United States individuals indicated that 99% of the participants (median age = 56 yeas) inherit at least one actionable variant and 58% of them were found positive for three or more drug affecting variants (Ji et al., 2016). These results were supported by our findings where 99.4% of the genotyped Dutch individuals carried one or more actionable variants. Our data showed that 76% of the participants were positive for three or more risky genotypes. In line with adult findings, pharmacogenes’ screening of 98 pediatric patients revealed that 95% of them had at least one pharmacogenomic variant (Cohn et al., 2017).
Results of this study indicated that exposure of patients to PGX drugs is common in Netherlands with an average of five exposures per patient over 7-year period among users who have actionable genotypes. This finding is similar to the results reported by an American study where half of the participants had one incident experience while one quarter to one third of them had two or more serious PGX exposures over a 4 year period (Samwald et al., 2016). In another study that involved an approximate 53,000 United States patients, 64.8% of them were exposed to one PGX drug within 5 years, whereas 5.9% of the individuals had five exposures (Schildcrout et al., 2012). In the Vanderbilt study which recruited 10,000 patients over 3 years in the so called PREDICT program, 42% of the participants had evidences of exposures to PGX drugs (Van Driest et al., 2014). Although our study covered a longer period (7 years) than PREDICT program, the estimated level of exposure was lower in the Dutch patients (24.1%), but this level is predicted to be raised over time. Each of these exposure episodes to PGXs drugs represents a chance to minimize related ADRs or optimize therapeutic plan.
Selection of the 45 PGX drugs included in this study was guided by the DPWG recommendations. Although CPIC and DPWG share consistent guidelines for the majority of reported interactions between actionable genotypes and PGX drugs, the criteria and methodology for ranking are different (Bank et al., 2018a). Therefore, differences in ranking between both groups may exist in term of level of evidence that support each interaction pairs. For example the claimed interaction between metoprolol with CYP2D6 was classified by DPWG as level 4, this is the highest rank of evidence based on published controlled studies of good quality (Deneer and van Schaik, 2013). In contrast, CPIC classified the evidence of metoprolol-CYP2D6 interaction as level C (A and B levels are the top ranking, (CPIC web page, see text footnote 1). In the case of atorvastatin- SCLO1B1 interaction, limited evidence is available that support an impact of 521T > C variant on atorvastatin-induced myopathy (Pasanen et al., 2007) and therefore was ranked by CPIC as level D, however, DPWG guidelines recommended genetic testing if additional risk factors exist (KNMP genetic database). Patients with high risk involve females, elderly people, those receiving high doses or with lower hepatic or renal function, concomitantly using medications that inhibit CYP3A4 (e.g., clarithromycin) or SLCO1B1 (e.g., gemfibrozil) (Ramsey et al., 2014).
Our study showed high consumption of PGX drugs that belongs to certain therapeutic classes, in particular, cardiovascular agents (metoprolol and statins) and the PPIs. Cardiovascular medications were also found to be the most commonly used therapeutic agents among patients aged more than 65 in the study conducted by Samwald et al. (2016). They also reported PPI group (omeprazole and pantoprazole) as the second drug category used in their study which is similar to our findings. Extra caution need to be taken when prescribing these medications through ordering the appropriate genetic tests and following the recommendations stated by DPWG guidelines. In younger patients, analgesic drugs were used more frequently in both Samwald et al. (2016) and Ji et al. (2016) studies which involved United States population. The latter study found that 75% of participants had an exposure to both tramadol and codeine or at least to one of them.
As identified in this project, CYP2D6, SLCO1B1, and CYP2C19 were the most commonly genes predicted to affect response of Dutch population to the used PGX drugs, hence, genotyping of these nominated genes before drug intake (preemptive testing) may significantly reduce emergence of ADRs and possibly optimize therapeutic outcomes. Similar to our findings, a recent study in the United States reported these three genes proposed in this study in addition to those affecting warfarin metabolism (VKORC1/CYP2C9) to be part of the suggested gene panels to be tested prior to cardiac angiography intervention (Dong et al., 2018). These five genes were exclusively studied by Ji et al. (2016) on 1013 subjects in the United States and emphasized that 79% of the participants carried actionable CYP2D6 genotypes. In contrast, our findings showed that 40% of Dutch population carry one or more CYP2D6 actionable variants. Similarly, the frequency of SLCO1B1*5 allele in the Dutch and the United States individuals involved in both studies was exactly the same (30%). Slightly lower frequency (26%) of this allele was noted in the study that involved larger number (10,000) of United States individuals (Van Driest et al., 2014). On the other hand, CYP2C19 actionable genotypes were more prominent in the United States individuals than that seen in Dutch population (28.5 vs. 10%, respectively). Warfarin is not used in Netherlands, however, testing for VKORC1 gene is advisable for both of the warfarin alternatives (acenocoumarol and phenprocoumon) commonly used by Dutch patients. In Netherlands, testing for CYP2C9 is sometimes suggested for patients on phenytoin; in particular, in patients with high phenytoin levels. Genotyping patients for CYP2D6, SLCO1B1, and CYP2C19 only provide the necessary information of possible interactions with 95% (60.31% + 22.00% + 12.35%, respectively) of the prescribed drugs in Netherlands. This information is essential to intervene before exposing patients to unnecessary risk. Knowing the enzymatic level of function based on characterization of gene activity as in the case of CYP2D6 would allow physicians to initiate proper medication and dose at an early stage of drug therapy. For example, CYP2D6 poor metabolizers are advised to use an alternative to amitriptyline (TCAs) or to use half of the recommended dose to avoid risk of adverse reaction which may result due to the elevated drug concentration in blood stream; it could be increased up to 2–3 times the normal level, even though normal doses are used in patients with low gene function (Halling et al., 2008); therefore, selective serotonin reuptake inhibitors (SSRIs), e.g., sertraline and citalopram are suggested to be used instead (Swen et al., 2011). Similarly, patients with poor CYP2D6 gene activity who are using codeine need to be instructed to use alternatives medications such as acetaminophen, non-steroidal anti-inflammatory drugs (NSAID) or morphine to achieve adequate pain relief (VanderVaart et al., 2011). Currently, a number of United States health institutes including universities (in Vanderbilt, Florida and Illinois), medical centers (in Vanderbilt, Mount Sinai and Mayo Clinic) and several hospitals (e.g., St. Jude Children’s Research Hospital and Shands Hospital) started reactive or preemptive testing for certain gene panels (Pulley et al., 2012; Crews et al., 2012; Nutescu et al., 2013; Hoffman et al., 2014; Weitzel et al., 2014; Shuldiner et al., 2014; Dunnenberger et al., 2015); of which the five genes described above are the commonest recommended for testing. Other suggested genes for testing were TPMT, CYP3A5, DPYD, UGT1A1, HLA-B IFNL3, and CYP4F2. The latter two genes were not included in our study as their actionable variants suggested by CPIC were not considered by DPWG guidelines which we adhered to.
Although, our data showed limited rate of usage of antineoplastic agents and chemotherapy (>1%), it is crucial to do genetic testing to avoid extreme toxicities related to some of these drugs, e.g., capecitabine. In addition, genetic profiling may help in tailoring therapies of cancer patients which possibly provide a better chance to treat them successfully (Patel, 2016). Our data indicated that females are using PGX drugs more than males (55.8% vs 44.2%, respectively). Identical results were reported to women (55.7%) in Samwald et al. (2016) the study which analyzed 72 million United States participants.
The highest level of exposure to PGx drug seen in our study was in patients aged 45 or above, who consumed the majority of PGX medications (84%). Of which, the patients aged 45–64 were the top users (33.5%). This finding is consistent with the results reported by Samwald et al. (2016) who emphasized that patients with age group 40–64 had the highest PGX drugs usage rate (37.9%). This may support offering the genetic tests to this targeted group at the meantime to customize health budget until the cost of genetic testing get reduced. However, mandatory tests must continue to be offered for selected younger individuals who may use drugs of serious toxicities, e.g., Abacavir, Capecitabine. The limitation of age breakdown analysis in this study is that the given age groups were divided into 7 groups only (0–4, 5–14, 15–24, 25–44, 45–64, 65–74, and <75) similar to the age distribution available on GIP database. It might be more helpful to further breakdown PGX drug usage over short age periods such as 5 or 10-year periods rather than 20 year age groups as in the case of 25–44 and 45–74 year subgroups.
In conclusion, the level of patients’ exposures to PGXs drugs reported here may encourage Dutch health institutes to offer panel-based preemptive testing with possible wider implementation of DPWG guidelines in the future. Future studies should evaluate cost-effectiveness of preemptive testing in routine clinical care, preferably in selected age groups using limited number of pharmacogenes.
Author Contributions
MA, FA, and VD worked together in setting the study objectives, designing the project, and analyzing the data. FA suggested the main concept about the project and highlighted its importance. MA took the lead in collecting the data related to drug consumption and identifying the frequency of SNPs in pharmacogenes that characterize Dutch population. VD did a great job in providing the currentDPWGguidelines and deciding the actionable drugs. AK participated in the final approval of the version to be published. The principal author wrote the manuscript which was reviewed and modified by all co-authors.
Funding
FA was financially supported by the UCL Hospitals NIHR Biomedical Research Centre.
Conflict of Interest Statement
The authors declare that the research was conducted in the absence of any commercial or financial relationships that could be construed as a potential conflict of interest.
Acknowledgments
We are grateful to Vinicius Tragante, Jessica Van Setten, and Abdalrhman A. Alswaji for their help in accessing and extracting genotyping data from GoNL database.
Footnotes
- ^ https://cpicpgx.org/genes-drugs/
- ^ http://www.nlgenome.nl/
- ^ https://www.knmp.nl/
- ^ https://www.gipdatabank.nl/databank.asp
References
Alagoz, O., Durham, D., and Kasirajan, K. (2016). Cost-effectiveness of one-time genetic testing to minimize lifetime adverse drug reactions. Pharmacogenomics J. 16, 129–136. doi: 10.1038/tpj.2015.39
Bain, K. T., Schwartz, E. J., Knowlton, O. V., Knowlton, C. H., and Turgeon, J. (2018). implementation of a pharmacist-led pharmacogenomics service for the program of all-inclusive care for the elderly (PHARM-GENOME-PACE). J. Am. Pharm. Assoc. 58:e1. doi: 10.1016/j.japh.2018.02.011
Bank, P. C. D., Caudle, K. E., Swen, J. J., Gammal, R. S., Whirl-Carrillo, M., Klein, T. E., et al. (2018a). Comparison of the guidelines of the clinical pharmacogenetics implementation consortium and the dutch pharmacogenetics working group. Clin. Pharmacol. Ther. 103, 599–618. doi: 10.1002/cpt.762
Bank, P. C. D., Swen, J. J., and Guchelaar, H. J. (2018b). Implementation of pharmacogenomics in everyday clinical settings. Adv. Pharmacol. 83, 219–246. doi: 10.1016/bs.apha.2018.04.003
Boomsma, D. I., Wijmenga, C., Slagboom, E. P., Swertz, M. A., Karssen, L. C., Abdellaoui, A., et al. (2014). The Genome of the Netherlands: design, and project goals. Eur. J. Hum. Genet. 22, 221–227. doi: 10.1038/ejhg.2013.118
Blakey, J. D., and Hall, I. P. (2011). Current progress in pharmacogenetics. Br. J. Clin. Pharmacol. 71, 824–831. doi: 10.1111/j.1365-2125.2011.03912.x
Carpenter, J. S., Rosenman, M. B., Knisely, M. R., Decker, B. S., Levy, K. D., and Flockhart, D. A. (2016). Pharmacogenomically actionable medications in a safety net health care system. SAGE Open Med. 4:2050312115624333. doi: 10.1177/2050312115624333
Caudle, K. E., Klein, T. E., Hoffman, J. M., Muller, D. J., Whirl-Carrillo, M., Gong, L., et al. (2014). Incorporation of pharmacogenomics into routine clinical practice: the clinical pharmacogenetics implementation consortium (CPIC) guideline development process. Curr. Drug Metab. 15, 209–217. doi: 10.2174/1389200215666140130124910
Cecchin, E., Roncato, R., Guchelaar, H. J., and Toffoli, G. Ubiquitous Pharmacogenomics Consortium (2017). Ubiquitous pharmacogenomics (U-PGx): the time for implementation is now. An Horizon2020 program to drive pharmacogenomics into clinical practice. Curr. Pharm. Biotechnol. 18, 204–209. doi: 10.2174/1389201018666170103103619
Cohn, I., Paton, T. A., Marshall, C. R., Basran, R., Stavropoulos, D. J., Ray, P. N., et al. (2017). Genome sequencing as a platform for pharmacogenetic genotyping: a pediatric cohort study. NPJ Genom. Med. 2:19. doi: 10.1038/s41525-017-0021-8
Collins, S. L., Carr, D. F., and Pirmohamed, M. (2016). Advances in the pharmacogenomics of adverse drug reactions. Drug Saf. 39, 15–27. doi: 10.1007/s40264-015-0367-8
Crews, K. R., Hicks, J. K., Pui, C. H., Relling, M. V., and Evans, W. E. (2012). Pharmacogenomics and individualized medicine: translating science into practice. Clin. Pharmacol. Ther. 92, 467–475. doi: 10.1038/clpt.2012.120
Deneer, V. H., and van Schaik, R. H. (2013). Evidence based drug dosing and pharmacotherapeutic recommendations per genotype. Methods Mol. Biol. 1015, 345–354. doi: 10.1007/978-1-62703-435-7_23
Dong, O. M., Li, A., Suzuki, O., Oni-Orisan, A., Gonzalez, R., Stouffer, G. A., et al. (2018). Projected impact of a multigene pharmacogenetic test to optimize medication prescribing in cardiovascular patients. Pharmacogenomics 19, 771–782. doi: 10.2217/pgs-2018-0049
Dunnenberger, H. M., Crews, K. R., Hoffman, J. M., Caudle, K. E., Broeckel, U., Howard, S. C., et al. (2015). Preemptive clinical pharmacogenetics implementation: current programs in five US medical centers. Annu. Rev. Pharmacol. Toxicol. 55, 89–106. doi: 10.1146/annurev-pharmtox-010814-124835
Halling, J., Weihe, P., and Brosen, K. (2008). The CYP2D6 polymorphism in relation to the metabolism of amitriptyline and nortriptyline in the faroese population. Br. J. Clin. Pharmacol. 65, 134–138. doi: 10.1111/j.1365-2125.2007.02969.x
Hoffman, J. M., Haidar, C. E., Wilkinson, M. R., Crews, K. R., Baker, D. K., Kornegay, N. M., et al. (2014). PG4KDS: a model for the clinical implementation of pre-emptive pharmacogenetics. Am. J. Med. Genet. C Semin. Med. Genet. 166C, 45–55. doi: 10.1002/ajmg.c.31391
Ingelman-Sundberg, M., Mkrtchian, S., Zhou, Y., and Lauschke, V. M. (2018). Integrating rare genetic variants into pharmacogenetic drug response predictions. Hum. Genomics 12:26. doi: 10.1186/s40246-018-0157-3
Ji, Y., Skierka, J. M., Blommel, J. H., Moore, B. E., Vancuyk, D. L., Bruflat, J. K., et al. (2016). Preemptive pharmacogenomic testing for precision medicine: a comprehensive analysis of five actionable pharmacogenomic genes using next-generation DNA sequencing and a customized CYP2D6 genotyping cascade. J. Mol. Diagn. 18, 438–445. doi: 10.1016/j.jmoldx.2016.01.003
Karczewski, K. J., Daneshjou, R., and Altman, R. B. (2012). Pharmacogenomics. PLoS Comput. Biol. 8:e1002817. doi: 10.1371/journal.pcbi.1002817
Mizzi, C., Dalabira, E., Kumuthini, J., Dzimiri, N., Balogh, I., Basak, N., et al. (2016). A european spectrum of pharmacogenomic biomarkers: implications for clinical pharmacogenomics. PLoS One 11:e0162866. doi: 10.1371/journal.pone.0162866
Netherlands Population (2018). Netherlands Population World Population Review. Available at: http://worldpopulationreview.com/countries/netherlands-population/ (accessed December 12, 2018).
Nutescu, E. A., Drozda, K., Bress, A. P., Galanter, W. L., Stevenson, J., Stamos, T. D., et al. (2013). Feasibility of implementing a comprehensive warfarin pharmacogenetics service. Pharmacotherapy 33, 1156–1164. doi: 10.1002/phar.1329
Pasanen, M. K., Fredrikson, H., Neuvonen, P. J., and Niemi, M. (2007). Different effects of SLCO1B1 polymorphism on the pharmacokinetics of atorvastatin and rosuvastatin. Clin. Pharmacol. Ther. 82, 726–733. doi: 10.1038/sj.clpt.6100220
Patel, J. N. (2016). Cancer pharmacogenomics, challenges in implementation, and patient-focused perspectives. Pharmacogenomics Pers. Med. 9:65. doi: 10.2147/PGPM.S62918
Pulley, J. M., Denny, J. C., Peterson, J. F., Bernard, G. R., Vnencak-Jones, C. L., Ramirez, A. H., et al. (2012). Operational implementation of prospective genotyping for personalized medicine: the design of the vanderbilt PREDICT project. Clin. Pharmacol. Ther. 92, 87–95. doi: 10.1038/clpt.2011.371
Ramsey, L. B., Johnson, S. G., Caudle, K. E., Haidar, C. E., Voora, D., Wilke, R. A., et al. (2014). The clinical pharmacogenetics implementation consortium guideline for SLCO1B1 and simvastatin-induced myopathy: 2014 update. Clin. Pharmacol. Ther. 96, 423–428. doi: 10.1038/clpt.2014.125
Relling, M., and Klein, T. (2011). CPIC: clinical pharmacogenetics implementation consortium of the pharmacogenomics research network. Clin. Pharmacol. Ther. 89, 464–467. doi: 10.1002/cpt.630
Roses, A. D. (2000). Pharmacogenetics and the practice of medicine. Nature 405, 857–865. doi: 10.1038/35015728
Samwald, M., Xu, H., Blagec, K., Empey, P. E., Malone, D. C., Ahmed, S. M., et al. (2016). Incidence of exposure of patients in the united states to multiple drugs for which pharmacogenomic guidelines are available. PLoS One 11:e0164972. doi: 10.1371/journal.pone.0164972
Schildcrout, J. S., Denny, J. C., Bowton, E., Gregg, W., Pulley, J. M., Basford, M. A., et al. (2012). Optimizing drug outcomes through pharmacogenetics: a case for preemptive genotyping. Clin. Pharmacol. Ther. 92, 235–242. doi: 10.1038/clpt.2012.66
Shuldiner, A. R., Palmer, K., Pakyz, R. E., Alestock, T. D., Maloney, K. A., O’neill, C., et al. (2014). Implementation of pharmacogenetics: the university of maryland personalized anti-platelet pharmacogenetics program. Am. J. Med. Genet. C Semin. Med. Genet. 166C, 76–84. doi: 10.1002/ajmg.c.31396
Swen, J., Nijenhuis, M., Boer, A. D., Grandia, L., Maitland-Van Der Zee, A.-H., and Mulder, H. (2011). Pharmacogenetics: from bench to byte—an update of guidelines. Clin. Pharmacol. Ther. 89, 662–673. doi: 10.1038/clpt.2011.34
Van Driest, S. L., Shi, Y., Bowton, E. A., Schildcrout, J. S., Peterson, J. F., Pulley, J., et al. (2014). Clinically actionable genotypes among 10,000 patients with preemptive pharmacogenomic testing. Clin. Pharmacol. Ther. 95, 423–431. doi: 10.1038/clpt.2013.229
VanderVaart, S., Berger, H., Sistonen, J., Madadi, P., Matok, I., Gijsen, V. M., et al. (2011). CYP2D6 polymorphisms and codeine analgesia in postpartum pain management: a pilot study. Ther. Drug Monit. 33, 425–432. doi: 10.1097/FTD.0b013e3182272b10
Verbelen, M., Weale, M. E., and Lewis, C. M. (2017). Cost-effectiveness of pharmacogenetic-guided treatment: are we there yet? Pharmacogenomics J. 17, 395–402. doi: 10.1038/tpj.2017.21
Vivot, A., Boutron, I., Ravaud, P., and Porcher, R. (2015). Guidance for pharmacogenomic biomarker testing in labels of FDA-approved drugs. Genet. Med. 17, 733–738. doi: 10.1038/gim.2014.181
Wang, L. (2010). Pharmacogenomics: a systems approach. Wiley Interdiscip. Rev. Syst. Biol. Med. 2, 3–22. doi: 10.1002/wsbm.42
Weitzel, K. W., Elsey, A. R., Langaee, T. Y., Burkley, B., Nessl, D. R., Obeng, A. O., et al. (2014). Clinical pharmacogenetics implementation: approaches, successes, and challenges. Am. J. Med. Genet. C Semin. Med. Genet. 166C, 56–67. doi: 10.1002/ajmg.c.31390
Keywords: pharmacogenetics, preemptive genetic testing, ADRs, CYP2D6, SLCO1B1, CYP2C19
Citation: Alshabeeb MA, Deneer VHM, Khan A and Asselbergs FW (2019) Use of Pharmacogenetic Drugs by the Dutch Population. Front. Genet. 10:567. doi: 10.3389/fgene.2019.00567
Received: 14 January 2019; Accepted: 29 May 2019;
Published: 02 July 2019.
Edited by:
Marcelo Rizzatti Luizon, Federal University of Minas Gerais, BrazilReviewed by:
Vanessa Fontana, University of Liverpool, United KingdomTodd Skaar, Indiana University Hospital, United States
Copyright © 2019 Alshabeeb, Deneer, Khan and Asselbergs. This is an open-access article distributed under the terms of the Creative Commons Attribution License (CC BY). The use, distribution or reproduction in other forums is permitted, provided the original author(s) and the copyright owner(s) are credited and that the original publication in this journal is cited, in accordance with accepted academic practice. No use, distribution or reproduction is permitted which does not comply with these terms.
*Correspondence: Mohammad A. Alshabeeb, shabeebonline@hotmail.com; Folkert W. Asselbergs, F.W.Asselbergs@umcutrecht.nl