- 1Department of Epidemiology, College of Public Health and Health Professions and College of Medicine, University of Florida, Gainesville, FL, United States
- 2Department of Epidemiology, School of Public Health, University of Washington, Seattle, WA, United States
- 3Department of Epidemiology, Rollins School of Public Health, Emory University, Atlanta, GA, United States
The timing of daily fluctuations in blood glucose is tightly controlled by the circadian rhythm. DNA methylation accompanies the circadian clock, and aberrant DNA methylation has been associated with circadian disruption and hyperglycemia. However, the precise role of circadian genes methylation in glucose metabolism is unknown. Using a gene-set approach in monozygotic (MZ) twin pairs, we examined the joint effect of 77 CpGs in five core circadian genes (CLOCK, BMAL1, PER1, PER2, PER3) on glucose-related traits in 138 middle-aged, male-male MZ twins (69 pairs). DNA methylation was quantified by bisulfite pyrosequencing. We first conducted matched twin pair analysis to examine the association of single CpG methylation with glucose metabolism. We then performed gene-based and gene-set analyses by the truncated product method to examine the combined effect of DNA methylation at multiple CpGs in a gene or all five circadian genes as a pathway on glucose metabolism. Of the 77 assayed CpGs, only one site was individually associated with insulin resistance at FDR < 0.05. However, the joint effect of DNA methylation in all five circadian genes together showed a significant association with glucose metabolism. Our results may unravel a biological mechanism through which circadian rhythm regulates blood glucose, and highlight the importance of testing the joint effect of multiple CpGs in epigenetic analysis.
Introduction
Circadian rhythms regulate many vital biological processes including glucose metabolism and insulin secretion (Qian and Scheer, 2016). Dysregulation of the circadian system has been associated with metabolic disorders, e.g., hyperglycemia (Leproult et al., 2014), insulin resistance (Rao et al., 2015), obesity (Krueger et al., 2015), dyslipidemia (Chua et al., 2013), metabolic syndrome (Wang et al., 2014), and atherosclerosis (Jankowiak et al., 2016). However, the molecular mechanisms underlying the circadian control of glucose metabolism are incompletely understood.
The circadian clock is under the tight control of an endogenous transcription-translation feedback loop, which is regulated by many clock genes. Among these, the clock circadian regulator (CLOCK), brain and muscle aryl hydrocarbon receptor nuclear translocator-like 1 (BMAL1), and period circadian clock (PER1/PER2/PER3) represent the core circadian genes in peripheral or central tissues (Kalsbeek et al., 2014). Genetic polymorphisms in these clock genes may also be related to fasting glucose (Bouatia-Naji et al., 2009; Englund et al., 2009; Dupuis et al., 2010; Dashti et al., 2015), insulin resistance (Lyssenko et al., 2009; Dashti et al., 2015), obesity (Garaulet et al., 2014), and type 2 diabetes (Bouatia-Naji et al., 2009; Voight et al., 2010; Bonnefond et al., 2012; Gaulton et al., 2015). While these studies highlight the importance of genetic variants in the circadian control of glucose metabolism, DNA sequence variation only explains a small fraction of inter-individual variability in blood glucose. Epigenetic factors such as DNA methylation provide an interface between the fixed genome and dynamic environment, representing a mechanism through which our changing environment can affect glucose metabolism. In fact, dysregulation of DNA methylation has previously been related to various metabolic disorders such as diabetes (Chambers et al., 2015; Kulkarni et al., 2015; Al Muftah et al., 2016; Florath et al., 2016; Kriebel et al., 2016; Soriano-Tarraga et al., 2016), obesity (Demerath et al., 2015; Ding et al., 2015; Al Muftah et al., 2016; Kriebel et al., 2016), and insulin resistance (Dayeh et al., 2014; Hidalgo et al., 2014; Kriebel et al., 2016). Moreover, the circadian clock is epigenetically controlled and circadian disruption causes changes in DNA methylation of circadian genes (Zhu et al., 2011; Bhatti et al., 2015). For example, in a weight loss intervention trial including 60 normal weight and obese women, DNA methylation variation in promoter regions of the CLOCK, BMAL1, and PER2 genes was associated with BMI, adiposity, weight loss, and metabolic syndrome (Milagro et al., 2012). An EWAS in subcutaneous adipose tissue from five pairs of MZ twins discordant for diabetes also revealed a significant enrichment of CpGs in genes involved in the circadian pathway, although no single CpG methylation was individually associated with diabetes (Ribel-Madsen et al., 2012). These results suggest that altered DNA methylation of circadian genes may play an important role in regulating glucose metabolism.
Previous epigenetic studies have largely focused on testing the individual effect of single CpG site, but as the effect of DNA methylation at a single CpG site could be very small, testing the individual effect of a single CpG site on a complex trait such as glucose metabolism may not be efficient. Statistical methods that can test the combined effects of multiple CpGs in multiple genes in a biological pathway may be a preferred approach in unraveling the genetic basis of complex traits.
Using a gene-set approach, the goal of this study is to examine the joint effect of DNA methylation at 77 CpG sites in five core circadian genes (CLOCK, BMAL1, PER1, PER2, PER3) on glucose metabolism in 69 MZ twin pairs participating in THS. Because MZ twin pairs share identical genetic materials and early life familial environment, a MZ co-twin control design rules out potential confounding by these factors and represents an ideal model for epigenetic analysis of human complex traits such as blood glucose.
Materials and Methods
Twin Pairs
The THS was designed to investigate the role of psychological, behavioral, and biological risk factors in subclinical cardiovascular disease in twins (Zhao et al., 2012b). Briefly, the study enrolled 180 middle-aged, male-male twin pairs who were born between 1946 and 1956 from VETR. All twins were free of overt cardiovascular disease at the time of enrollment and were examined in pairs at the Emory University General Clinical Research Center between 2002 and 2010. Venous blood samples were drawn by trained nurse in the morning (6:30∼7:00) after an overnight fast and stored at -80°C until laboratory tests. Zygosity was determined by DNA typing. All twins provided written informed consent. The protocols for both the THS and the current study were approved by the Emory University Institutional Review Board. Of the 84 MZ twin pairs with available DNA sample and clinical data for both members of a twin pair, we removed 15 twin pairs who were either concordant or discordant on overt type 2 diabetes in order to eliminate the potential impact of hypoglycemic drugs on the association between DNA methylation and glucose metabolism. The final data analysis included 69 complete MZ pairs.
DNA Methylation Measurement
DNA methylation levels in the promoter regions of five key circadian genes including CLOCK, BAML1, PER1, PER2, and PER3 were quantified by bisulfite pyrosequencing using genomic DNA isolated from peripheral blood leukocytes, as previously described (Zhao et al., 2013). In brief, genomic DNA was bisulfite treated using the EZ DNA Methylation Kit (Zymo Research, Inc., CA, United States) according to the manufacturer’s protocol, which converts un-methylated cytosines into uracil, and leaves methylated cytosines unchanged. Pyrosequencing was done on the PSQ96 HS System (Pyrosequencing, Qiagen). The targeted CpG sites in each gene were chosen based on: (a) CpG probes in the Illumina 450K array and RRBS databases; (b) regulatory elements such as promoter, enhancer, transcriptional binding sites, and (c) genomic sequences from UCSC genome browser. Genomic coordinates in Genome Reference Consortium Human Build 37 (GRCh37). The genomic coordinates and targeted sequences for pyrosequencing were shown in Supplementary Table S1 and schematically illustrated in Supplementary Figure S1. Methylation level at each CpG site was calculated as the percentage of the methylated alleles over the sum of methylated and un-methylated alleles. For quality control, each experiment included non-CpG cytosines as internal control to verify completion of sodium bisulfite DNA conversion. Un-methylated and methylated DNAs were also included as controls in each run. Pyrosequencing assay was run in duplicates, with a high correlation (≥99.8%) of two runs for a same sample.
Measurement of Glucose Metabolism
Levels of blood glucose, insulin, and HbA1c in fasting plasma were measured on the Beckman CX7 chemistry auto analyzer (Beckman Coulter Diagnostics). Insulin resistance was assessed by the homeostasis model assessment (HOMA-IR) based on fasting glucose and fasting insulin (Duncan et al., 1995). All biochemical assays for each twin pair were processed in the same analytical run.
Measurement of Risk Factors
Body weight (kg) and height (cm) were measured when twin participants wore light clothes and no shoes by trained research staff. BMI was calculated by dividing weight in kilograms by the square of height in meters (kg/m2). Systolic (SBP) and DBP were measured by a mercury sphygmomanometer on the right arm in sitting position after at least 10 min of rest. Two separate blood pressure measurements, taken 5 min apart, were averaged for analysis. Cigarette smoking was classified into current smoker (any number of cigarettes) versus never or past smoker. Pack-years of smoking were calculated as the number of packs of cigarettes smoked per day times the number of years smoked. Information on alcoholic (wine, beer, or cocktail) beverages consumed within a typical week was obtained and alcohol consumption (g/week) was estimated as previously described (Zhao et al., 2012a). Physical activity was assessed by means of the Baecke physical activity score, which summarizes activity related to work, sports, and leisure (Richardson et al., 1995). HDL-c and LDL-c were measured with homogeneous assays (Equal Diagnostics, Exton, Pennsylvania). Triglycerides were determined by enzymatic methods (Beckman Coulter Diagnostics, Fullerton, CA, United States). Depressive symptoms were measured with BDI-II (Beck et al., 1996).
Statistical Analysis
Monozygotic twin pairs share 100% of their genes and early life environment including uterine environment, all of which could influence the epigenome and metabolic risk (Gluckman et al., 2009). To eliminate the potential confounding by shared gene and environment, we conducted a co-twin control matched pair analysis using within-pair differences (Zhao et al., 2015). To achieve this, we first calculated the intra-pair difference in DNA methylation, defined as the difference in methylation level of each CpG site between members of a twin pair. The intra-pair differences in HOMA-IR, fasting glucose, and HbA1c as well as continuous covariates including cigarette smoking (pack-year), alcohol consumption (g/week), physical activity level, BMI, lipids (HDL-c, LDL-c), SBP, and depressive symptoms (BDI-II score) were similarly calculated. To examine whether DNA methylation of circadian genes influences glucose metabolism, we simultaneously conducted single CpG analysis, gene-based and gene-set association analyses. All statistical analyses were performed using SAS statistical software (version 9.4, Cary, NC, United States).
Single CpG Association Analysis
To examine the association of DNA methylation at each single CpG site with glucose metabolism, we constructed a linear regression model (Carlin et al., 2005), in which intra-pair difference in a glucose trait (e.g., HOMA-IR, fasting glucose, or HbA1c) was the dependent variable and intra-pair difference in DNA methylation level at each CpG site was the independent variable, adjusting for twin age and intra-pair differences in covariates listed above. Multiple testing was controlled by adjusting for the total number of CpG sites using FDR, and a FDR-adjusted P-value (i.e., q-value) less than 0.05 was considered statistically significant.
Gene-Based and Gene-Set Association Analyses
To examine the joint effect of DNA methylation at multiple CpG sites in a circadian gene or all five circadian genes as a pathway on glucose metabolism, we employed a wTPM as previously described (Zaykin et al., 2002). This method tests the joint effects of multiple CpG sites in a gene or multiple genes in a pathway by combining P-values of all CpGs in a chosen gene or all genes in a selected pathway. The effect size of DNA methylation at each CpG site (i.e., regression coefficient) was included as weight in the TPM statistic. Multiple testing for gene-based analysis was controlled by adjusting for total number of genes. This method has been evaluated extensively by simulation studies (Sheng and Yang, 2013), and our group has used this approach to test the joint associations of genetic variants in the smoking gene pathway with diabetes (Yang et al., 2012), subclinical atherosclerosis (Yang et al., 2013), kidney function (Zhu et al., 2014a), and obesity (Zhu et al., 2014b). Here we extended this approach to epigenetic analysis.
Results
The current analysis included 69 male-male MZ twin pairs (mean age 55 years). All twins are European Caucasians and were free of overt diabetes and cardiovascular disease at the time of blood draw. Clinical characteristics of the twins are presented in Table 1.
Single CpG Association Analysis
Of the 77 assayed CpG sites in the promoter regions of the five circadian genes, methylation variation at 6 CpG sites (3 in CLOCK, 2 in BMAL1, 1 in PER2) was significantly associated with insulin resistance, methylation at 8 CpG sites (4 in CLOCK, 2 in BMAL1, 2 in PER2) was associated with fasting glucose, and methylation at 3 CpG sites (2 in BMAL1, 1 in PER2) was associated with HbA1c (all P < 0.05), after correction for covariates. After further adjusting for multiple testing, the association between DNA methylation at only 1 CpG site in the BMAL1 gene remained to be statistically significant in relation to insulin resistance at q < 0.05. Table 2 presents the results of single CpG association analysis. Supplementary Table S2 shows the mean intra-pair differences in DNA methylation at each of the assayed CpG sites.
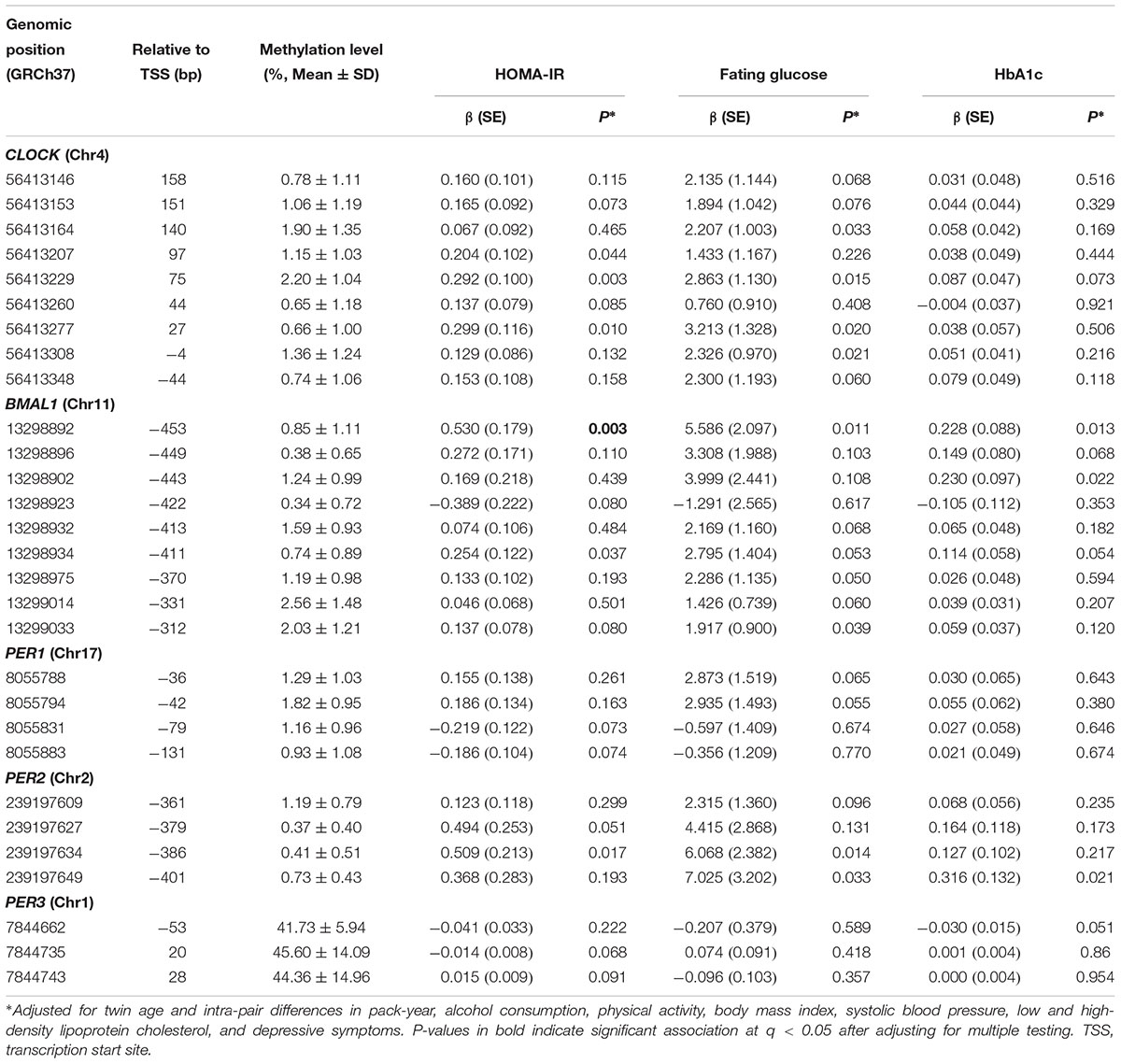
Table 2. Association of single CpG methylation in five core circadian genes with glucose metabolism (raw P ≤ 0.1).
Gene-Based and Gene-Set Association Analyses
At the level of q < 0.05, gene-based analysis detected significant associations of DNA methylation in four circadian genes (CLOCK, BMAL1, PER1, and PER2) with insulin resistance, fasting glucose, or HbA1c. Gene-set analysis revealed that DNA methylation in all five circadian genes as a pathway jointly contributed to insulin resistance (P = 0.0001), fasting glucose (P = 0.0001), and HbA1c (P = 0.0005). Results for gene-based and gene-set association analyses are shown in Table 3.
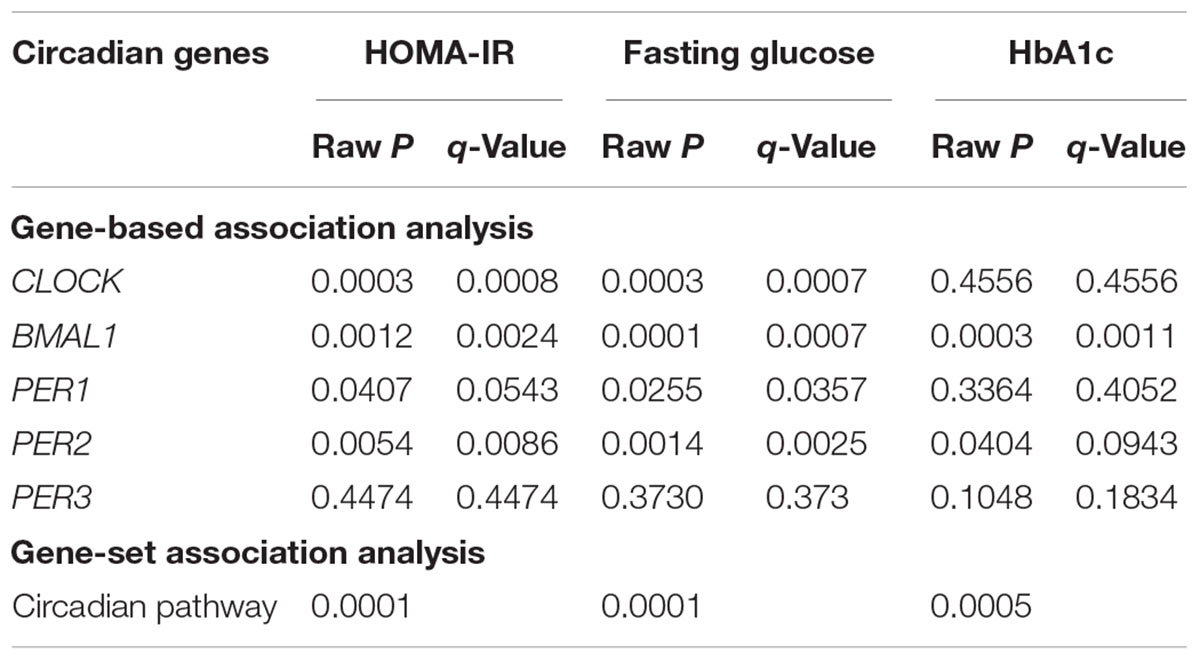
Table 3. Gene-based and gene-set associations of DNA methylation in five core circadian genes with glucose metabolism.
Discussion
Using a gene-family approach, we demonstrated that altered DNA methylation in five circadian genes jointly contributed to glucose metabolism in a well-matched MZ twin sample. Our results may unravel a molecular mechanism through which circadian rhythms affect glucose metabolism, and suggest that simultaneously testing the joint effects of multiple CpG sites in a gene or multiple genes in a pathway is a powerful approach in epigenetic analysis of human complex traits.
Several aspects of our results merit discussion. First, in line with previous studies,(Shah et al., 2015) we also found that individual effect of a single CpG methylation on glucose metabolism is small. Of the 77 CpG sites assayed in our sample, DNA methylation at 10 CpG was nominally associated with one or more glucose traits (raw P < 0.05), with only one site survived multiple testing (q < 0.05). However, the combined effects of multiple CpG sites could be large. For example, in our single CpG analysis, DNA methylation at two out of the nine assayed CpG sites in the PER2 gene showed marginal association with fasting glucose, but both disappeared after multiple testing correction. In contrast, gene-based test revealed a significant association of all nine CpG sites in this gene with fasting glucose. Similarly, of the 30 CpG sites measured in the promoter region of the CLOCK gene, only 4 CpG sites showed nominal individual associations with either blood glucose or insulin resistance, but gene-based approach detected a significant association of all 30 CpG sites in this gene with both blood glucose and insulin resistance. This highlights the importance of testing the joint epigenetic effect of multiple CpGs on human complex traits. Second, a complex trait such as glucose metabolism is most likely regulated by many genes in one or more biological pathways. Testing the individual effect of each individual gene may not be efficient and also does not reflect the true biology. We thus examined the combined effect of five core circadian genes as a pathway on glucose metabolism. It shows that, although not all genes showed significant individual association, the circadian pathway as a whole was significantly associated with glucose measures. Together, our findings suggest that testing the joint epigenetic effects of multiple CpG sites in a gene or a pathway represents a preferred approach for epigenetic analysis of human complex traits. Finally, although several previous studies reported associations of individual CpG methylation in the CLOCK, BMAL1, and PER2 genes with glucose-related traits, e.g., insulin resistance, obesity (Milagro et al., 2012), no study has systematically tested the joint effect of multiple CpGs in a candidate gene or the circadian pathway on glucose metabolism.
Our study has several limitations. First, given that DNA methylation is tissue- or cell-type specific, it is unclear whether or to what extent the results derived from peripheral blood could reflect methylation changes in the target organs of glucose metabolism, e.g., pancreas, liver, fat and muscle. However, accumulating evidence indicated that epimutations may not be limited to the affected tissue but could also be detected in peripheral blood (Menke and Binder, 2014). Second, as the original THS was designed to examine the role of psychosocial factors in subclinical atherosclerosis, the study included multiple twin pairs discordant on major depression (37 MZ discordant pairs were included in the current analysis). It is unclear whether and how such bias will affect our results. However, we believe this should not be a major concern because we controlled for depressive symptoms in all statistical analyses. Third, as all observational studies, we cannot establish the causal role of DNA methylation in glucose metabolism. Moreover, as all studies using MZ twins, the sample size of our study is small. We cannot rule out the possibility of false positive or false negative findings, and thus our results need further replication. Fourth, the present study is a secondary data analysis based on genes assayed in a previous candidate gene-based study that did not include all the circadian genes (e.g., CRY2). Fifth, there is a time interval (about 5–9 years) between the biochemical and methylation assays. However, we believe that this should not be major concern because it has been shown that methylation profiles are stable in DNA or blood samples archived for up to 20 years under appropriate storage conditions (Li et al., 2018). Finally, as all twins included in the current analysis are European Caucasians, the generalizability of our results to other racial/ethnic groups is uncertain.
Nonetheless, our study has several strengths. First, as MZ twin pairs share identical genotypes, age, and sex, as well as many other socioenvironmental factors including in utero and familial environment, the use of a MZ co-twin control design minimizes or eliminates potential confounding by these factors. Moreover, our analyses also controlled for many lifestyles and metabolic factors. Second, we used innovative statistical approaches to test the combined effects of multiple CpG sites in a gene or multiple genes in the circadian pathway in well-matched MZ twins. To the best of our knowledge, we are the first to systematically test the joint epigenetic effect of multiple CpGs in the circadian pathway on glucose traits in MZ twins. Our results provide initial evidence that altered DNA methylation of key circadian genes may play an important role in glucose metabolism.
In summary, our results demonstrated that DNA methylation of the five core circadian genes jointly contributed to blood glucose metabolism. These findings also highlight the importance of testing the effect of DNA methylation jointly rather than separately on human complex traits in epigenetic analysis.
Author Contributions
HP and YZ conducted the analysis. HP drafted the manuscript. JG and VV provided the critical comments. JZ designed the study and finalized the manuscript. All authors read and approved the final manuscript.
Funding
This study was supported by NIH grants R01MH097018, R01DK091369, R21HL092363, and K01AG034259. The United States Department of Veterans Affairs has provided financial support for the development and maintenance of the Vietnam Era Twin Registry (VETR).
Conflict of Interest Statement
The authors declare that the research was conducted in the absence of any commercial or financial relationships that could be construed as a potential conflict of interest.
Acknowledgments
Numerous organizations have provided invaluable assistance in the conduct of this study, including: Department of Defense; National Personnel Records Center, National Archives and Records Administration; the Internal Revenue Service; National Institutes of Health; National Opinion Research Center; National Research Council, National Academy of Sciences; and the Institute for Survey Research, Temple University. Most importantly, the authors gratefully acknowledge the continued cooperation and participation of the members of the VET Registry and their families. Without their contribution this research would not have been possible.
Supplementary Material
The Supplementary Material for this article can be found online at: https://www.frontiersin.org/articles/10.3389/fgene.2019.00329/full#supplementary-material
Abbreviation
BDI-II, the Beck Depression Inventory-II; BMI, body mass index; DBP, diastolic blood pressures; EWAS, epigenome-wide association study; FDR, false discovery rate; HbA1c, hemoglobin A1c; HDL-c, high-density lipoprotein cholesterol; HOMA-IR, the homeostasis model assessment of insulin resistance; LDL-c, low-density lipoprotein cholesterol; MZ, monozygotic; SBP, systolic blood pressure; THS, the Twins Heart Study; VETR, the Vietnam Era Twin Registry; wTPM, weighted truncated product method.
References
Al Muftah, W. A., Al-Shafai, M., Zaghlool, S. B., Visconti, A., Tsai, P. C., Kumar, P., et al. (2016). Epigenetic associations of type 2 diabetes and BMI in an Arab population. Clin. Epigenetics 8:13. doi: 10.1186/s13148-016-0177-6
Beck, A. T., Steer, R. A., and Brown, G. K. (1996). Beck Depression Inventory-II. San Antonio, TX: Psychological Corporation.
Bhatti, P., Zhang, Y., Song, X., Makar, K. W., Sather, C. L., Kelsey, K. T., et al. (2015). Nightshift work and genome-wide DNA methylation. Chronobiol. Int. 32, 103–112. doi: 10.3109/07420528.2014.956362
Bonnefond, A., Clement, N., Fawcett, K., Yengo, L., Vaillant, E., Guillaume, J. L., et al. (2012). Rare MTNR1B variants impairing melatonin receptor 1B function contribute to type 2 diabetes. Nat. Genet. 44, 297–301. doi: 10.1038/ng.1053
Bouatia-Naji, N., Bonnefond, A., Cavalcanti-Proenca, C., Sparso, T., Holmkvist, J., Marchand, M., et al. (2009). A variant near MTNR1B is associated with increased fasting plasma glucose levels and type 2 diabetes risk. Nat. Genet. 41, 89–94. doi: 10.1038/ng.277
Carlin, J. B., Gurrin, L. C., Sterne, J. A., Morley, R., and Dwyer, T. (2005). Regression models for twin studies: a critical review. Int. J. Epidemiol. 34, 1089–1099. doi: 10.1093/ije/dyi153
Chambers, J. C., Loh, M., Lehne, B., Drong, A., Kriebel, J., Motta, V., et al. (2015). Epigenome-wide association of DNA methylation markers in peripheral blood from indian asians and europeans with incident type 2 diabetes: a nested case-control study. Lancet Diabetes Endocrinol. 3, 526–534. doi: 10.1016/S2213-8587(15)00127-8
Chua, E. C., Shui, G., Lee, I. T., Lau, P., Tan, L. C., Yeo, S. C., et al. (2013). Extensive diversity in circadian regulation of plasma lipids and evidence for different circadian metabolic phenotypes in humans. Proc. Natl. Acad. Sci. U.S.A. 110, 14468–14473. doi: 10.1073/pnas.1222647110
Dashti, H. S., Follis, J. L., Smith, C. E., Tanaka, T., Garaulet, M., Gottlieb, D. J., et al. (2015). Gene-Environment interactions of circadian-related genes for cardiometabolic traits. Diabetes Care 38, 1456–1466. doi: 10.2337/dc14-2709
Dayeh, T., Volkov, P., Salo, S., Hall, E., Nilsson, E., Olsson, A. H., et al. (2014). Genome-wide DNA methylation analysis of human pancreatic islets from type 2 diabetic and non-diabetic donors identifies candidate genes that influence insulin secretion. PLoS Genet. 10:e1004160. doi: 10.1371/journal.pgen.1004160
Demerath, E. W., Guan, W., Grove, M. L., Aslibekyan, S., Mendelson, M., Zhou, Y. H., et al. (2015). Epigenome-wide association study (EWAS) of BMI, BMI change and waist circumference in African American adults identifies multiple replicated loci. Hum. Mol. Genet. 24, 4464–4479. doi: 10.1093/hmg/ddv161
Ding, X., Zheng, D., Fan, C., Liu, Z., Dong, H., Lu, Y., et al. (2015). Genome-wide screen of DNA methylation identifies novel markers in childhood obesity. Gene 566, 74–83. doi: 10.1016/j.gene.2015.04.032
Duncan, M. H., Singh, B. M., Wise, P. H., Carter, G., and Alaghband-Zadeh, J. (1995). A simple measure of insulin resistance. Lancet 346, 120–121. doi: 10.1016/S0140-6736(95)92143-5
Dupuis, J., Langenberg, C., Prokopenko, I., Saxena, R., Soranzo, N., Jackson, A. U., et al. (2010). New genetic loci implicated in fasting glucose homeostasis and their impact on type 2 diabetes risk. Nat. Genet. 42, 105–116. doi: 10.1038/ng.520
Englund, A., Kovanen, L., Saarikoski, S. T., Haukka, J., Reunanen, A., Aromaa, A., et al. (2009). NPAS2 and PER2 are linked to risk factors of the metabolic syndrome. J. Circadian Rhythms 7:5. doi: 10.1186/1740-3391-7-5
Florath, I., Butterbach, K., Heiss, J., Bewerunge-Hudler, M., Zhang, Y., Schottker, B., et al. (2016). Type 2 diabetes and leucocyte DNA methylation: an epigenome-wide association study in over 1,500 older adults. Diabetologia 59, 130–138. doi: 10.1007/s00125-015-3773-7
Garaulet, M., Smith, C. E., Gomez-Abellan, P., Ordovas-Montanes, M., Lee, Y. C., Parnell, L. D., et al. (2014). REV-ERB-ALPHA circadian gene variant associates with obesity in two independent populations: mediterranean and North American. Mol. Nutr. Food Res. 58, 821–829. doi: 10.1002/mnfr.201300361
Gaulton, K. J., Ferreira, T., Lee, Y., Raimondo, A., Magi, R., Reschen, M. E., et al. (2015). Genetic fine mapping and genomic annotation defines causal mechanisms at type 2 diabetes susceptibility loci. Nat. Genet. 47, 1415–1425. doi: 10.1038/ng.3437
Gluckman, P. D., Hanson, M. A., Buklijas, T., Low, F. M., and Beedle, A. S. (2009). Epigenetic mechanisms that underpin metabolic and cardiovascular diseases. Nat. Rev. Endocrinol. 5, 401–408. doi: 10.1038/nrendo.2009.102
Hidalgo, B., Irvin, M. R., Sha, J., Zhi, D., Aslibekyan, S., Absher, D., et al. (2014). Epigenome-wide association study of fasting measures of glucose, insulin, and HOMA-IR in the genetics of lipid lowering drugs and diet network study. Diabetes 63, 801–807. doi: 10.2337/db13-1100
Jankowiak, S., Backe, E., Liebers, F., Schulz, A., Hegewald, J., Garthus-Niegel, S., et al. (2016). Current and cumulative night shift work and subclinical atherosclerosis: results of the gutenberg health study. Int. Arch. Occup. Environ. Health 89, 1169–1182. doi: 10.1007/s00420-016-1150-6
Kalsbeek, A., La Fleur, S., and Fliers, E. (2014). Circadian control of glucose metabolism. Mol. Metab. 3, 372–383. doi: 10.1016/j.molmet.2014.03.002
Kriebel, J., Herder, C., Rathmann, W., Wahl, S., Kunze, S., Molnos, S., et al. (2016). Association between DNA methylation in whole blood and measures of glucose metabolism: KORA F4 study. PLoS One 11:e0152314. doi: 10.1371/journal.pone.0152314
Krueger, P. M., Reither, E. N., Peppard, P. E., Burger, A. E., and Hale, L. (2015). Cumulative exposure to short sleep and body mass outcomes: a prospective study. J. Sleep Res. 24, 629–638. doi: 10.1111/jsr.12327
Kulkarni, H., Kos, M. Z., Neary, J., Dyer, T. D., Kent, J. W. Jr., Göring, H. H., et al. (2015). Novel epigenetic determinants of type 2 diabetes in Mexican-American families. Hum. Mol. Genet. 24, 5330–5344. doi: 10.1093/hmg/ddv232
Leproult, R., Holmback, U., and Van Cauter, E. (2014). Circadian misalignment augments markers of insulin resistance and inflammation, independently of sleep loss. Diabetes 63, 1860–1869. doi: 10.2337/db13-1546
Li, Y., Pan, X., Roberts, M. L., Liu, P., Kotchen, T. A., Cowley, A. W., et al. (2018). Stability of global methylation profiles of whole blood and extracted DNA under different storage durations and conditions. Epigenomics 10, 797–811. doi: 10.2217/epi-2018-0025
Lyssenko, V., Nagorny, C. L., Erdos, M. R., Wierup, N., Jonsson, A., Spegel, P., et al. (2009). Common variant in MTNR1B associated with increased risk of type 2 diabetes and impaired early insulin secretion. Nat. Genet. 41, 82–88. doi: 10.1038/ng.288
Menke, A., and Binder, E. B. (2014). Epigenetic alterations in depression and antidepressant treatment. Dialogues Clin. Neurosci. 16, 395–404.
Milagro, F. I., Gomez-Abellan, P., Campion, J., Martinez, J. A., Ordovas, J. M., and Garaulet, M. (2012). CLOCK, PER2 and BMAL1 DNA methylation: association with obesity and metabolic syndrome characteristics and monounsaturated fat intake. Chronobiol. Int. 29, 1180–1194. doi: 10.3109/07420528.2012.719967
Qian, J., and Scheer, F. A. (2016). Circadian system and glucose metabolism: implications for physiology and disease. Trends Endocrinol. Metab. 27, 282–293. doi: 10.1016/j.tem.2016.03.005
Rao, M. N., Neylan, T. C., Grunfeld, C., Mulligan, K., Schambelan, M., and Schwarz, J. M. (2015). Subchronic sleep restriction causes tissue-specific insulin resistance. J. Clin. Endocrinol. Metab. 100, 1664–1671. doi: 10.1210/jc.2014-3911
Ribel-Madsen, R., Fraga, M. F., Jacobsen, S., Bork-Jensen, J., Lara, E., Calvanese, V., et al. (2012). Genome-wide analysis of DNA methylation differences in muscle and fat from monozygotic twins discordant for type 2 diabetes. PLoS One 7:e51302. doi: 10.1371/journal.pone.0051302
Richardson, M. T., Ainsworth, B. E., Wu, H. C., Jacobs, D. R. Jr., and Leon, A. S. (1995). Ability of the atherosclerosis risk in communities (ARIC)/baecke questionnaire to assess leisure-time physical activity. Int. J. Epidemiol. 24, 685–693. doi: 10.1093/ije/24.4.685
Shah, S., Bonder, M. J., Marioni, R. E., Zhu, Z., Mcrae, A. F., Zhernakova, A., et al. (2015). Improving phenotypic prediction by combining genetic and epigenetic associations. Am. J. Hum. Genet. 97, 75–85. doi: 10.1016/j.ajhg.2015.05.014
Sheng, X., and Yang, J. (2013). Truncated product methods for panel unit root tests. Oxf. Bull. Econ. Stat. 75, 624–636. doi: 10.1111/j.1468-0084.2012.00705.x
Soriano-Tarraga, C., Jimenez-Conde, J., Giralt-Steinhauer, E., Mola-Caminal, M., Vivanco-Hidalgo, R. M., Ois, A., et al. (2016). Epigenome-wide association study identifies TXNIP gene associated with type 2 diabetes mellitus and sustained hyperglycemia. Hum. Mol. Genet. 25, 609–619. doi: 10.1093/hmg/ddv493
Voight, B. F., Scott, L. J., Steinthorsdottir, V., Morris, A. P., Dina, C., Welch, R. P., et al. (2010). Twelve type 2 diabetes susceptibility loci identified through large-scale association analysis. Nat. Genet. 42, 579–589. doi: 10.1038/ng.609
Wang, F., Zhang, L., Zhang, Y., Zhang, B., He, Y., Xie, S., et al. (2014). Meta-analysis on night shift work and risk of metabolic syndrome. Obes. Rev. 15, 709–720. doi: 10.1111/obr.12194
Yang, J., Zhu, Y., Cole, S. A., Haack, K., Zhang, Y., Beebe, L. A., et al. (2012). A gene-family analysis of 61 genetic variants in the nicotinic acetylcholine receptor genes for insulin resistance and type 2 diabetes in American Indians. Diabetes 61, 1888–1894. doi: 10.2337/db11-1393
Yang, J., Zhu, Y., Lee, E. T., Zhang, Y., Cole, S. A., Haack, K., et al. (2013). Joint associations of 61 genetic variants in the nicotinic acetylcholine receptor genes with subclinical atherosclerosis in American Indians: a gene-family analysis. Circ. Cardiovasc. Genet. 6, 89–96. doi: 10.1161/CIRCGENETICS.112.963967
Zaykin, D. V., Zhivotovsky, L. A., Westfall, P. H., and Weir, B. S. (2002). Truncated product method for combining P-values. Genet. Epidemiol. 22, 170–185. doi: 10.1002/gepi.0042
Zhao, J., An, Q., Goldberg, J., Quyyumi, A. A., and Vaccarino, V. (2015). Promoter methylation of glucocorticoid receptor gene is associated with subclinical atherosclerosis: a monozygotic twin study. Atherosclerosis 242, 71–76. doi: 10.1016/j.atherosclerosis.2015.07.007
Zhao, J., Forsberg, C. W., Goldberg, J., Smith, N. L., and Vaccarino, V. (2012a). MAOA promoter methylation and susceptibility to carotid atherosclerosis: role of familial factors in a monozygotic twin sample. BMC Med. Genet. 13:100. doi: 10.1186/1471-2350-13-100
Zhao, J., Goldberg, J., Bremner, J. D., and Vaccarino, V. (2012b). Global DNA methylation is associated with insulin resistance: a monozygotic twin study. Diabetes 61, 542–546. doi: 10.2337/db11-1048
Zhao, J., Goldberg, J., and Vaccarino, V. (2013). Promoter methylation of serotonin transporter gene is associated with obesity measures: a monozygotic twin study. Int. J. Obes. 37, 140–145. doi: 10.1038/ijo.2012.8
Zhu, Y., Stevens, R. G., Hoffman, A. E., Tjonneland, A., Vogel, U. B., Zheng, T., et al. (2011). Epigenetic impact of long-term shiftwork: pilot evidence from circadian genes and whole-genome methylation analysis. Chronobiol. Int. 28, 852–861. doi: 10.3109/07420528.2011.618896
Zhu, Y., Yang, J., Li, S., Cole, S. A., Haack, K., Umans, J. G., et al. (2014a). Genetic variants in nicotinic acetylcholine receptor genes jointly contribute to kidney function in American Indians: the strong heart family study. J. Hypertens 32, 1042–1048; discussion 1049. doi: 10.1097/HJH.0000000000000151
Keywords: circadian rhythm, DNA methylation, monozygotic twins, glucose metabolism, epigenetics
Citation: Peng H, Zhu Y, Goldberg J, Vaccarino V and Zhao J (2019) DNA Methylation of Five Core Circadian Genes Jointly Contributes to Glucose Metabolism: A Gene-Set Analysis in Monozygotic Twins. Front. Genet. 10:329. doi: 10.3389/fgene.2019.00329
Received: 10 December 2018; Accepted: 28 March 2019;
Published: 12 April 2019.
Edited by:
Tina Bianco-Miotto, The University of Adelaide, AustraliaReviewed by:
Susan Kay Murphy, Duke University, United StatesNejat Dalay, Istanbul University, Turkey
Copyright © 2019 Peng, Zhu, Goldberg, Vaccarino and Zhao. This is an open-access article distributed under the terms of the Creative Commons Attribution License (CC BY). The use, distribution or reproduction in other forums is permitted, provided the original author(s) and the copyright owner(s) are credited and that the original publication in this journal is cited, in accordance with accepted academic practice. No use, distribution or reproduction is permitted which does not comply with these terms.
*Correspondence: Jinying Zhao, anpoYW82NkB1ZmwuZWR1