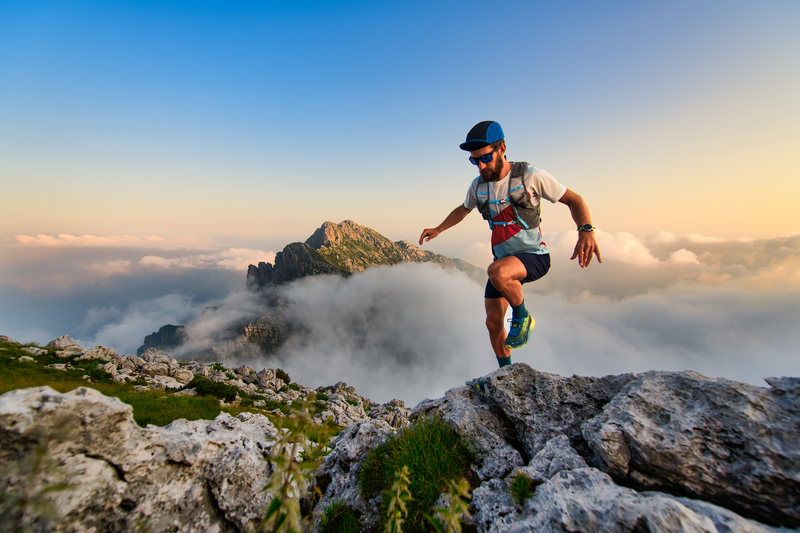
94% of researchers rate our articles as excellent or good
Learn more about the work of our research integrity team to safeguard the quality of each article we publish.
Find out more
ORIGINAL RESEARCH article
Front. Genet. , 21 March 2019
Sec. Epigenomics and Epigenetics
Volume 10 - 2019 | https://doi.org/10.3389/fgene.2019.00220
DNA methylation is an important form of epigenetic regulation that can regulate the expression of genes and the development of tissues. Muscle satellite cells play an important role in skeletal muscle development and regeneration. Therefore, the DNA methylation status of genes in satellite cells is important in the regulation of the development of skeletal muscle. This study systematically investigated the changes of genome-wide DNA methylation in satellite cells during skeletal muscle development. According to the MeDIP-Seq data, 52,809–123,317 peaks were obtained for each sample, covering 0.70–1.79% of the genome. The number of reads and peaks was highest in the intron regions followed by the CDS regions. A total of 96,609 DMRs were identified between any two time points. Among them 6198 DMRs were annotated into the gene promoter regions, corresponding to 4726 DMGs. By combining the MeDIP-Seq and RNA-Seq data, a total of 202 overlap genes were obtained between DMGs and DEGs. GO and Pathway analysis revealed that the overlap genes were mainly involved in 128 biological processes and 23 pathways. Among the biological processes, terms related to regulation of cell proliferation and Wnt signaling pathway were significantly different. Gene–gene interaction analysis showed that Wnt5a, Wnt9a, and Tgfβ1 were the key nodes in the network. Furthermore, the expression level of Wnt5a, Wnt9a, and Tgfβ1 genes could be influenced by the methylation status of promoter region during skeletal muscle development. These results indicated that the Wnt and Tgfβ signaling pathways may play an important role in functional regulation of satellite cells, and the DNA methylation status of Wnt and Tgfβ signals is a key regulatory factor during skeletal muscle development. This study provided new insights into the effects of genome-wide methylation on the function of satellite cells.
Skeletal muscle satellite cells are a kind of mononuclear cells with a flattened projection, which locate between the muscle fiber basement membrane and the muscle cell membrane in skeletal muscle (Mauro, 1961). Satellite cells play an important role in skeletal muscle development and regeneration (Ono et al., 2015; Gurevich et al., 2016; Angelino et al., 2018; Guitart et al., 2018). Satellite cells can undergo myogenic differentiation, thereby providing the nucleus for the attached muscle fibers and participating in the development of muscle fibers (Alameddine et al., 1989). In adult skeletal muscle, satellite cells can be activated, and then undergo myogenic differentiation to form new muscle fibers or to fuse with myotubes to repair damaged areas, when muscle fibers are damaged (Collins et al., 2005; Guitart et al., 2018).
Researches showed that the activation, proliferation, and differentiation of satellite cells play a critical role in skeletal muscle development, which require the involvement of a variety of signaling molecules, including TGF pathway (Delaney et al., 2017), FGF pathway (Han et al., 2012), and Wnt pathway (Girardi and Le Grand, 2018). Studies showed that TGFβ1 could inhibit the proliferation of myoblasts and the regeneration of necrotic muscle fibers, and Wnt signaling could regulate the differentiation of myoblast by interacting with TGFβ signaling (Melone et al., 1999; Rudolf et al., 2016). In addition, our previous study showed that TGFβ and WNT9a could inhibit the differentiation of satellite cell, and the synergistic effects of TGFβ2, WNT9a, and FGFR4 signals could be involved in regulating the differentiation of satellite cells during skeletal muscle development (Zhang et al., 2018). However, the epigenetic regulatory mechanism affecting the expression level of these key factors remain largely unknown.
Methylation is a common form of epigenetic regulation affecting the expression of related genes and the development of tissue (Gilsbach et al., 2014). The hypermethylation of promoter regions can lead to gene silencing, but the hypomethylated regions are usually an open area (Bird, 1986; Bernstein et al., 2007). In recent years, many studies have shown that methylation plays an important role in skeletal muscle development, in which satellite cells are involved. Histone methylation and DNA methylation are the two most common forms of methylation modification, and these two forms of methylation modification could effectively affect the function of satellite cells in skeletal muscle development (Liu et al., 2013; Miyata et al., 2017; Robinson and Dilworth, 2018). Methylated Pax7 could directly bind to MLL1/2 protein and then recruited histone H3K4 methyltransferase complex to regulate the expression of Myf5 (McKinnell et al., 2008; Kawabe et al., 2012). The expression level of Myogenin was up-regulated during the differentiation of satellite cells on account of the decrease of the 5′-flanking methylation level (Fuso et al., 2010). Methyl-CpG-binding protein, named CIBZ, could affect the methylation status of the promoter-proximal region of Myogenin gene and then inhibited its transcription level (Oikawa et al., 2011). Although many studies have shown that methylation plays an important role in regulating the functions of satellite cells, the systematic exposition of the methylation profile of satellite cells in the process of skeletal muscle development is still very limited.
Our group studied the transcriptome and differentiation characteristics of satellite cells at different developmental stages. We confirmed that the Wnt, Tgfβ, and Fgfr signals were responsible for the attenuation of satellite cell differentiation with development, but the mechanisms of expression change of key factors were unknown (Zhang et al., 2018). Hence, in this study, mouse satellite cells from four time points were obtained. Furthermore, MeDIP-Seq analysis was performed to investigate the genome-wide temporal dynamic changes of DNA methylation during skeletal muscle development. This study systematically analyzed the DNA methylation profile of satellite cells in skeletal muscle development, and identified the potential effects of DNA methylation on skeletal muscle development.
In our previous study, the skeletal muscle development of mice postnatal can be divided into four stages according to cluster analysis, namely, Weeks 2, 4 & 6, 8, and 10 & 12 (Zhang et al., 2018). Therefore, the leg muscle of four different time points (Weeks 2, 6, 8, and 12) was obtained to isolate satellite cells. Then the MeDIP-Seq analysis was performed. Firstly, we investigated the global DNA methylation status at different developmental stages. According to our data, 21 million clean reads per sample were obtained through filtering the raw reads. Furthermore, the clean reads were mapped to the mouse reference genome, and the mapping rates ranged from 95.74 to 96.34% with uniquely mapped rates ranging from 36.05 to 45.36% (Supplementary Table S1). The uniquely mapped reads were considered during further analysis. The result demonstrating that the global distribution of uniquely mapped reads (chromosomes 1–19 and X) was investigated for each sample (Supplementary Figure S1). Furthermore, the coverage of different methylated models CG, CHG, and CHH were analyzed, demonstrating that the CG, CHG, and CHH sites with high methylation level account for a small proportion in genome-wide (Figure 1 and Supplementary Figures S2–S4).
Figure 1. Proportion of the methylated CG, CHG, and CHH sites under different enrichment level of reads in different samples. The average value of each stage was considered.
Usually, methylation peak is an important index for the study of differential methylation sites genome-wide. In our study, 52,809–123,317 peaks were obtained for each sample, covering 0.70–1.79% of the genome (Supplementary Table S2). To further investigate the genome-wide distribution of methylation data, the proportion of reads peaks in different genome components was calculated in six different regions. The result showed that the distribution of reads and peaks in CDS regions was second only to intron regions (Figures 2A,B). Furthermore, the specific pattern of gene body was investigated. We divided each gene into 60 equal windows, and the upstream and downstream 2 kb regions were split into 20 non-overlapping windows. The result showed that the reads at the transcription start site (TSS) obviously decreased in all samples but gradually increased in the intragenic region (Figure 2C). Moreover, the proportion of reads and peaks in CpG island was counted. The result showed that the distribution of reads and peaks in CpG island was lower than that outside CpG island regions (Figures 2D,E and Supplementary Figure S5).
Figure 2. Distribution of unique mapped reads and peaks in different genomic regions. (A) The proportion of unique mapped reads in different gene regions. (B) The proportion of peaks in different gene regions. (C) The distribution of unique mapped reads in 2 kb region upstream of the transcription start site (TSS), the gene body from the TSS to the transcription termination site (TTS), and a 2 kb region downstream of the TTS. (D) The proportion of unique mapped reads in CpG island region and outside CpG regions. (E) The proportion of peaks in CpG island region and outside CpG regions.
The methylation data was further explored to compare the differential methylation regions (DMRs) between any two time points (Week 2 versus Week 6, Week 2 versus Week 8, Week 2 versus Week 12, Week 6 versus Week 8, Week 6 versus Week 12, and Week 8 versus Week 12). According to the data, a total of 96,609 DMRs were identified (p < 0.01). Among them, 6198 DMRs were annotated into the gene promoter regions (Supplementary Table S3). In this study, we considered the genes with methylation peaks in the promoter regions as the methylated genes. A total of 4726 differential methylation genes (DMGs) were identified (Supplementary Table S4), and then clustering analysis was performed. The result showed that the methylation status of genes in satellite cells was a dynamic process with the development of skeletal muscle (Supplementary Figure S6).
Studies showed that the methylation of promoter regions could affect gene expression (Niemann et al., 2008; Laurent et al., 2010). To further understand the effects of methylation on gene function and expression, the differentially expressed genes (DEGs) were compared between any two time points, and the union set of DEGs in any comparison group were considered. A total of 1717 DEGs were identified (FDR < 0.05) (Supplementary Table S4). Furthermore, overlap analysis was performed between DEGs and DMGs, and a total of 202 genes were obtained (Figure 3A and Supplementary Table S4). The result indicated that changes in the expression levels of these genes might be influenced by methylation. Furthermore, the cluster analysis result showed that most genes had a lower methylation level at Week 2, and the methylation level of overlap genes was most significantly different between Weeks 2 and 12 (Figure 3B).
Figure 3. (A) The number of overlap genes between DMGs and DEGs is shown by a Venn diagram. (B) Cluster analysis of overlap genes between DMGs and DEGs according to the methylation levels of genes. Red indicated hypermethylation levels and purple indicated hypomethylation levels.
In addition, Gene Ontology (GO) analysis was performed using the DAVID program. The result showed that these overlap genes were mainly involved in 128 biological processes (p < 0.05). The top 30 GO terms were showed in Figure 4A, including positive regulation of cell migration, positive regulation of smooth muscle cell proliferation, Wnt signaling pathway, calcium modulating pathway, and regulation of cell proliferation. To further understand the role of DMGs in the functional regulation of satellite cells, signaling pathways were analyzed using the Kyoto Encyclopedia of Genes and Genomes database (KEGG). The result showed that the overlap genes were enriched in 23 signaling pathways (p < 0.05), some of which were related to muscle development, including Calcium signaling pathway, Wnt signaling pathway, and Insulin secretion (Figure 4B). These results indicated that the DNA methylation status in satellite cells plays an important role in the development of skeletal muscle.
Figure 4. (A) Significantly enriched GO terms (top 30) of the overlap genes. (B) Significantly enriched signaling pathways of the overlap genes.
To identify the potential key genes that can regulate the function of satellite cells with skeletal muscle development, overlap genes between DMGs and DEGs were selected to study the interaction using String software. A total of 52 genes were considered in the network after filtering genes with weak interaction strength (confidence >0.7) (Figure 5). The network revealed that Wnt5a, Wnt9a, Tgfβ1, Cxcl12, Mmp14, Cdh5, and Egr1 were the core nodes. In addition, Tgfβ1 had the largest number of interactive genes in the network. Moreover, Wnt signaling pathway, including Wnt5a, Wnt9a, Fzd2, and Ror2 genes, was located in the upstream of the interaction network and could regulate Mmp4, Cdh5, and Egr1 indirectly through the Cxcl12 gene. This result indicated that Wnt5a, Wnt9a, and Tgfβ1 were the key nodes in the gene interaction network and that the methylation status of Wnt and Tgfβ signaling pathways may play an important role in functional regulation of satellite cells during skeletal muscle development.
Figure 5. Analysis of the interaction between overlap genes using String software according to interplay index (confidence >0.7). Interplay index between genes was represented by width and transparency of edges. Dark and wide edge indicated high confidence.
In order to study the effect of DNA methylation on gene expression level, the trend of gene expression with that of methylation was compared, using FPKM of RNA-seq data and MeDIP-seq data, respectively. The result showed that, the expression trends of Wnt5a and Tgfβ1 genes were consistent with those of DNA methylation level, which were gradually up-regulated with development (Figures 6A,C). Furthermore, Q-PCR result showed that the expression level of Wnt5a and Tgfβ1 genes were up-regulated with development (Figures 6B,D). However, the DNA methylation level in the promoter region of Wnt9a gene was down-regulated at Week 6, but significantly up-regulated at Weeks 8 and 12, contrary to the change trend of expression level (Figure 6E). Furthermore, Q-PCR result showed that the expression level of Wnt9a gene was significantly up-regulated at Week 6, but down-regulated from Weeks 8 to 12 (Figure 6F). These results indicated that the expression level of Wnt5a, Wnt9a, and Tgfβ1 genes could be influenced by the methylation state of promoter region during skeletal muscle development.
Figure 6. (A) FPKM data were used to compare the variation trend of expression level and methylation level of Tgfβ1 gene. (B) Q-PCR was performed to detect the expression level of Tgfβ1 gene in satellite cells. (C) FPKM data were used to compare the variation trend of expression level and methylation level of Wnt5a gene. (D) Q-PCR was performed to detect the expression level of Wnt5a gene in satellite cells. (E) FPKM data were used to compare the variation trend of expression level and methylation level of Wnt9a gene. (F) Q-PCR was performed to detect the expression level of Wnt9a gene in satellite cells. In Q-PCR experiments, Tubulin was used as the internal control, and the relative fold change was compared to the expression in Week 2 satellite cells. Triplicate samples were analyzed for each treatment, and the results were presented as the mean ± SEM. ∗P < 0.05, ∗∗P < 0.01.
Skeletal muscle satellite cells are a class of stem cells located between the myofibril membrane and the myofibril basement membrane, and they can participate in the development and regeneration of muscle fibers (Charge and Rudnicki, 2004; Lepper et al., 2011). In our previous studies, the capacity of the differentiation of satellite cells decreased with age, and Weeks 4 and 6 were two similar stages of development, as well as Weeks 10 and 12. Moreover, we confirmed that many genes and pathways related to growth and development were involved in this process through transcriptome analysis (Zhang et al., 2018). Therefore, we isolated skeletal muscle satellite cells from mice of different ages for MeDIP-Seq analysis to study the expression change mechanism of related genes during skeletal muscle development. In this study, only four time points were selected to represent the development of skeletal muscle postnatal for the law of skeletal muscle development shown in our previous article. In addition, from Day 1 to Week 2 are the early stages of growth and development after birth. Considering the operability, satellite cells of Week 2 mice were selected as the representative of early stages.
According to the MeDIP-Seq data, 21 million clean reads per sample were obtained by filtering the raw reads. The rate of uniquely mapped ranged from 36.05 to 45.36% (Supplementary Table S1). Studies have shown that methylation peak was an important index of the methylation level (Li et al., 2010; Su et al., 2016). Generally, promoter regions have lower methylation levels than other regions (Laurent et al., 2010; Li et al., 2014). In our study, the genome-wide coverage of peaks was 0.70–1.79%, and the promoter region had a lower methylation level than other regions (Figure 2). Moreover, the proportion of peaks in CpG island regions was lower than that outside CpG island regions due to the small proportion of CpG island regions in genome-wide.
Researches showed that DNA methylation in promoter regions could inhibit the gene expression, namely high methylation levels correspond to low expression levels (Wagner et al., 2014; Wu and Zhang, 2014). In the present study, a total of 96,609 DMRs were annotated into the gene promoter regions, and these corresponded to 4726 DMGs. Moreover, the 202 overlap genes were identified by comparing DMGs and DEGs. Most of the genes were related to satellite cell function and muscle development, including positive regulation of cell migration, positive regulation of smooth muscle cell proliferation, Wnt signaling pathway, calcium modulating pathway, and regulation of cell proliferation. Cluster analysis revealed that many of the overlap genes had a lower methylation levels at Week 2 (Figure 3B). This result indicated that these genes were transcriptionally activated at early stages due to related to the growth and development processes of tissues. Studies showed that dynamics in DNA methylation persisted during development and cell differentiation (Stelzer et al., 2016; Li et al., 2018). Interestingly, the changes of methylation levels of the overlap genes was not linear in these four developmental stages, indicating that these genes may be involved in different biological processes due to the developmental characteristics at the different stages of skeletal muscle development.
Furthermore, signaling pathway analysis was performed. As expected, we found that Wnt signaling pathway was significantly enriched. Researches have shown that Wnt and Tgfβ signaling pathway play important roles in skeletal muscle development regulation (Biressi et al., 2014; Zhu et al., 2017; Girardi and Le Grand, 2018; Zhang et al., 2018). Gene–gene interaction analysis showed that Wnt5a, Wnt9a, and Tgfβ1 were the key nodes in the network. Previous study showed that WNT5a could promote the proliferation of skeletal muscle satellite cells (Otto et al., 2008). Studies showed that TGFβ1 could inhibit the proliferation of myoblasts and the regeneration of myofiber (Melone et al., 1999; Delaney et al., 2017). In addition, our previous study has shown that Wnt and Tgfβ signals could regulate the differentiation of satellite cells. The synergistic effects of TGFβ2, WNT9a, and FGFR4 signals could regulate the differentiation of satellite cells, and WNT9a acted as an intermediate regulator in this equilibrium system (Zhang et al., 2018). Moreover, we also found that Acvrl1 and Mstn, which are members of Tgfβ superfamily (McPherron et al., 1997; Humbert, 2002), also occurred in the interaction network (Figure 5). Studies showed that MSTN could inhibit the differentiation of myoblasts (Guardiola et al., 2012; Rossi et al., 2016). Combining results from our study and those in these researches, we concluded that DNA methylation could affect the function of satellite cells during skeletal muscle development process in mice.
Researches have shown that DNA methylation in promoter regions was related to the silence of gene, while the effect of DNA methylation in gene body regions on gene expression was variable (Gelfman et al., 2013; Wu and Zhang, 2014; Yang et al., 2014). In this study, we focused on the DNA methylation of promoter regions. Study showed that hypermethylation of promoter regions could inhibit the expression of Wnt9a gene, which consisted with the result in this study (Figure 6) (Shu et al., 2006). Interestingly, the methylation level in the promoter region of Wnt5a and Tgfβ1 genes showed positive correlation with their expression level. Therefore, we speculated that the methylation of promoter regions cooperated with that of the gene body regions to regulate the expression of Wnt5a and Tgfβ1 genes. Hence, we concluded that Wnt and Tgfβ signaling pathways were the key regulators during skeletal muscle development, and their DNA methylation status may be the key factor on functional regulation of satellite cell.
The DNA methylation in satellite cells dynamically changed during skeletal muscle development, which may affect the expression of genes and regulate the function of satellite cells. The DNA methylation status of Wnt and Tgfβ signals is a key factor on functional regulation of skeletal muscle satellite cell development. This study provides evidence for epigenetic studies of skeletal muscle development. Such information is potentially useful for improving the muscle growth of livestock.
All C57BL/6 mice used in this study were obtained from Hubei Center for Disease Control and Prevention (Wuhan, China). All experiments were performed in accordance with the Guide for the Care and Use of Laboratory Animals (Institute of Laboratory Animal Resources, Commission on Life Sciences, National Research Council, 1996). The protocols were approved by the Hubei Province Committee on Laboratory Animal Care (HZAUMU2013-0005).
Satellite cells were isolated from the hind-limb muscle tissues at four different time points (postnatal Weeks 2, 6, 8, and 12), and 8 ∼ 12 mice (8 mice for Weeks 2 and Week 6, 10 mice for Week 8, and 12 mice for Week 12) were used for each cell isolation experiment. Satellite cell isolation method referred that described in previous study (Lu et al., 2008; Zhang et al., 2018). The muscle tissues were digested for 60–90 min with collagenase I (2 mg/mL) (Sigma, United States, C1639) at 37°C. The dissociated suspension was sifted through 100, 200, and 400 mesh sieves. Then, the suspension was washed with RPMI 1640 medium, re-suspended by growth medium with 15% fetal calf serum (Gibco, United States, 10082-147), chick embryo extract (GEMINI, United States, 100-163p), basic fibroblast growth factor (Life, United States, 13256-029) (0.25 μg/100 mL), and RPMI 1640 medium. The suspension was plated on a normal dish and then transferred to a dish coated with matrigel (BD, United States, 356234) after 2.5 h. The satellite cells were cultured at 37°C in a cell incubator with 5% CO2 until they converged to 60%. Then, the second differential attachment experiment was performed.
The sequencing process and analysis methods used were in accordance with those used in our previous research (Zhang et al., 2018). The total RNA was extracted from isolated satellite cells at six different time points using RNeasy Mini Kit (Qiagen, Germany, 74106) in accordance with the manufacturer’s instructions. Qualified total RNA was further purified by using the RNAClean XP Kit (Beckman Coulter, Inc., Kraemer Boulevard Brea, CA, United States, A63987) and the RNase-Free DNase Set (QIAGEN, Germany, 79254). RNA and the library preparation integrity were verified with an Agilent Bioanalyzer 2100 (Agilent Technologies, Santa Clara, CA, United States). We accomplished the cluster and first dimension sequencing primer hybridization on cBot of Illumina sequencing machine in accordance with the cBot User Guide. Sequencing was performed by Shanghai Biotechnology Corporation (P.R.C). Edger, which is an R package, was used to screen the DEGs.
DNA was isolated from cultured satellite cells using OMEGA TISSUE DNA Kit (2000) (n = 3 for each time point). The concentration and quality of DNA were assessed by NanoDrop® ND-1000 and agarose gel electrophoresis. The library was prepared according to MeDIP library development flow. Sequencing was performed by SHANGHAI BIOTECHNOLOGY CORPORATION. The amount of DNA used for sequencing was about 2 μg. The sequencing process included the following: (1) Library construction was performed and the integrity was verified with Agilent 2100 (Agilent Technologies, Santa Clara, CA, United States). (2) Cluster generation and primer hybridization were completed on the cBot equipped with the Illumina HiSeq sequencing instrument according to the corresponding process shown in the cBot User Guide. (3) The sequencing process was controlled by data collection software provided by Illumina, and real-time data analysis was carried out.
Raw sequencing data were first filtered with the following five steps: (a) Reads with >50% bases having phred quality < 20 were removed; (b) Removal of the bases with phred quality < 20 in 3′ end; (c) Removal of adapter contained in reads; (d) Removal of reads with length < 20; (e) Removal of reads that contain ‘N’ base.
Clean reads were aligned to the Ensembl mouse reference genome (GRCm381) using BWA (Version 0.7.17) with default parameters (Li and Durbin, 2009). To investigate the general model of methylation, we analyzed the distribution of aligned reads on the whole reference genome, CGIs, and gene regions, as well as the genome coverage of CG, CHG, and CHH regions with different sequencing depths in each sample and group. The peak distribution was analyzed using MACS (version 2.1.1) software in different genome components, including upstream 2 kb, 5′ UTR, CDS, intron, 3′ UTR, downstream 2 kb, and CpG islands (Zhang et al., 2008).
EdgeR integrated in the R packages MEDIPS with 200 bp window size was used to identify DMRs between different groups (Lienhard et al., 2014). MEDIPS identified DMRs between groups by calculating Wilcoxon rank tests for the reads per million (rpm) values of each window (cite MEDIPS). DMRs were filtered for windows with p < 0.01. Further, ChIPseeker was performed to annotate the DMRs in gene level, and only the genes with promoter annotated by DMRs were defined as differential methylated genes (DMGs) (Yu et al., 2015). All DMGs were subsequently analyzed with GO and KEGG pathway of the DAVID web server (Huang da et al., 2009).
Reverse transcription was performed to initiate cDNA synthesis by using the Prime ScriptTM RT Reagent Kit with gDNA Eraser (TAKARA BIO INC, Otsu, Shiga, Japan). THUNDERBIRD SYBR qPCR Mix (TOYOBO, Japan) was used for Q-PCR, and the results were monitored using a CFX384 Real-Time PCR Detection System (Bio-Rad, United States). The sequences for Q-PCR primer were as follow: Wnt9a-F, 5′GATTTGCGAGCCCGAGTG3′, Wnt9a-R, 5′GTCTCATATTTGTGTTTTAGGTGCTT3′; Wnt5a-F, 5′CTCACCCCCACAGGCAAC3′, Wnt5a-R, 5′TGCCCTACCAGCAGTGAGTG3′; Tgfβ1-F, 5′GGCGGTGCTCGCTTTGTA3′, Tgfβ1-R, 5′TCCCGAATGTCTGACGTATTGA3′.
The data sets supporting the results of this article were included within the article and additional files. Raw sequencing reads are available at the Short Read Archive (SRA) database of NCBI (bio-project accession PRJNA510174, study SRP173522, MeDIP-seq accessions SRR8325050-SRR8325061, and RNA-seq accessions SRR8453587-SRR8453598).
WZ conducted the experiments and prepared the materials involved in this study. SaZ and YX performed the bioinformatics analysis. ShZ and XL conceived this study. ShZ, XL, and WZ participated in its design and coordination. XL, WZ, and SaZ contributed to the analysis and interpretation of the data. WZ drafted the manuscript. ShZ, XL, YM, and DZ helped to revise the manuscript. All authors read and approved the final manuscript.
This work was supported by the National Natural Science Foundation of China (31361140365 and 31672391), National Swine Industry Technology System (CARS-35), and the Major Project of National Natural Science Foundation of China (31790414).
The authors declare that the research was conducted in the absence of any commercial or financial relationships that could be construed as a potential conflict of interest.
The Supplementary Material for this article can be found online at: https://www.frontiersin.org/articles/10.3389/fgene.2019.00220/full#supplementary-material
Alameddine, H. S., Dehaupas, M., and Fardeau, M. (1989). Regeneration of skeletal muscle fibers from autologous satellite cells multiplied in vitro. an experimental model for testing cultured cell myogenicity. Muscle Nerve 12, 544–555. doi: 10.1002/mus.880120705
Angelino, E., Reano, S., Bollo, A., Ferrara, M., De Feudis, M., Sustova, H., et al. (2018). Ghrelin knockout mice display defective skeletal muscle regeneration and impaired satellite cell self-renewal. Endocrine 62, 129–135. doi: 10.1007/s12020-018-1606-4
Bernstein, B. E., Meissner, A., and Lander, E. S. (2007). The mammalian epigenome. Cell 128, 669–681. doi: 10.1016/j.cell.2007.01.033
Bird, A. P. (1986). CpG-rich islands and the function of DNA methylation. Nature 321, 209–213. doi: 10.1038/321209a0
Biressi, S., Miyabara, E. H., Gopinath, S. D., Carlig, P. M., and Rando, T. A. (2014). A Wnt-TGFbeta2 axis induces a fibrogenic program in muscle stem cells from dystrophic mice. Sci. Transl. Med. 6:267ra176. doi: 10.1126/scitranslmed.3008411
Charge, S. B., and Rudnicki, M. A. (2004). Cellular and molecular regulation of muscle regeneration. Physiol. Rev. 84, 209–238. doi: 10.1152/physrev.00019.2003
Collins, C. A., Olsen, I., Zammit, P. S., Heslop, L., Petrie, A., Partridge, T. A., et al. (2005). Stem cell function, self-renewal, and behavioral heterogeneity of cells from the adult muscle satellite cell niche. Cell 122, 289–301. doi: 10.1016/j.cell.2005.05.010
Delaney, K., Kasprzycka, P., Ciemerych, M. A., and Zimowska, M. (2017). The role of TGF-beta1 during skeletal muscle regeneration. Cell Biol. Int. 41, 706–715. doi: 10.1002/cbin.10725
Fuso, A., Ferraguti, G., Grandoni, F., Ruggeri, R., Scarpa, S., Strom, R., et al. (2010). Early demethylation of non-CpG, CpC-rich, elements in the myogenin 5′-flanking region: a priming effect on the spreading of active demethylation. Cell Cycle 9, 3965–3976. doi: 10.4161/cc.9.19.13193
Gelfman, S., Cohen, N., Yearim, A., and Ast, G. (2013). DNA-methylation effect on cotranscriptional splicing is dependent on GC architecture of the exon-intron structure. Genome Res. 23, 789–799. doi: 10.1101/gr.143503.112
Gilsbach, R., Preissl, S., Gruning, B. A., Schnick, T., Burger, L., Benes, V., et al. (2014). Dynamic DNA methylation orchestrates cardiomyocyte development, maturation and disease. Nat. Commun. 5:5288. doi: 10.1038/ncomms6288
Girardi, F., and Le Grand, F. (2018). Wnt signaling in skeletal muscle development and regeneration. Prog. Mol. Biol. Transl. Sci. 153, 157–179. doi: 10.1016/bs.pmbts.2017.11.026
Guardiola, O., Lafuste, P., Brunelli, S., Iaconis, S., Touvier, T., Mourikis, P., et al. (2012). Cripto regulates skeletal muscle regeneration and modulates satellite cell determination by antagonizing myostatin. Proc. Natl. Acad. Sci. U.S.A. 109, E3231–E3240. doi: 10.1073/pnas.1204017109
Guitart, M., Lloreta, J., Manas-Garcia, L., and Barreiro, E. (2018). Muscle regeneration potential and satellite cell activation profile during recovery following hindlimb immobilization in mice. J. Cell. Physiol. 233, 4360–4372. doi: 10.1002/jcp.26282
Gurevich, D. B., Nguyen, P. D., Siegel, A. L., Ehrlich, O. V., Sonntag, C., Phan, J. M., et al. (2016). Asymmetric division of clonal muscle stem cells coordinates muscle regeneration in vivo. Science 353:aad9969. doi: 10.1126/science.aad9969
Han, D., Zhao, H., Parada, C., Hacia, J. G., Bringas, P. Jr., Chai, Y., et al. (2012). A TGFbeta-Smad4-Fgf6 signaling cascade controls myogenic differentiation and myoblast fusion during tongue development. Development 139, 1640–1650. doi: 10.1242/dev.076653
Huang da, W., Sherman, B. T., and Lempicki, R. A. (2009). Bioinformatics enrichment tools: paths toward the comprehensive functional analysis of large gene lists. Nucleic Acids Res. 37, 1–13. doi: 10.1093/nar/gkn923
Humbert, M. (2002). [Mutations of genes coding for TGF-beta receptors (BMPR2 and ALK-1) in primary pulmonary arterial hypertension]. J. De La Soc. De Biol. 196, 53–58. doi: 10.1051/jbio/2002196010053
Kawabe, Y., Wang, Y. X., McKinnell, I. W., Bedford, M. T., and Rudnicki, M. A. (2012). Carm1 regulates Pax7 transcriptional activity through MLL1/2 recruitment during asymmetric satellite stem cell divisions. Cell Stem Cell 11, 333–345. doi: 10.1016/j.stem.2012.07.001
Laurent, L., Wong, E., Li, G., Huynh, T., Tsirigos, A., Ong, C. T., et al. (2010). Dynamic changes in the human methylome during differentiation. Genome Res. 20, 320–331. doi: 10.1101/gr.101907.109
Lepper, C., Partridge, T. A., and Fan, C. M. (2011). An absolute requirement for Pax7-positive satellite cells in acute injury-induced skeletal muscle regeneration. Development 138, 3639–3646. doi: 10.1242/dev.067595
Li, G., Jia, Q., Zhao, J., Li, X., Yu, M., Samuel, M. S., et al. (2014). Dysregulation of genome-wide gene expression and DNA methylation in abnormal cloned piglets. BMC Genomics 15:811. doi: 10.1186/1471-2164-15-811
Li, H., and Durbin, R. (2009). Fast and accurate short read alignment with burrows-wheeler transform. Bioinformatics 25, 1754–1760. doi: 10.1093/bioinformatics/btp324
Li, J., Wu, X., Zhou, Y., Lee, M., Guo, L., Han, W., et al. (2018). Decoding the dynamic DNA methylation and hydroxymethylation landscapes in endodermal lineage intermediates during pancreatic differentiation of hESC. Nucleic Acids Res. 46, 2883–2900. doi: 10.1093/nar/gky063
Li, N., Ye, M., Li, Y., Yan, Z., Butcher, L. M., Sun, J., et al. (2010). Whole genome DNA methylation analysis based on high throughput sequencing technology. Methods 52, 203–212. doi: 10.1016/j.ymeth.2010.04.009
Lienhard, M., Grimm, C., Morkel, M., Herwig, R., and Chavez, L. (2014). MEDIPS: genome-wide differential coverage analysis of sequencing data derived from DNA enrichment experiments. Bioinformatics 30, 284–286. doi: 10.1093/bioinformatics/btt650
Liu, L., Cheung, T. H., Charville, G. W., Hurgo, B. M., Leavitt, T., Shih, J., et al. (2013). Chromatin modifications as determinants of muscle stem cell quiescence and chronological aging. Cell Rep. 4, 189–204. doi: 10.1016/j.celrep.2013.05.043
Lu, A., Cummins, J. H., Pollett, J. B., Cao, B., Sun, B., Rudnicki, M. A., et al. (2008). Isolation of myogenic progenitor populations from Pax7-deficient skeletal muscle based on adhesion characteristics. Gene Ther. 15, 1116–1125. doi: 10.1038/gt.2008.86
Mauro, A. (1961). Satellite cell of skeletal muscle fibers. J. Biophys. Biochem. Cytol. 9, 493–495. doi: 10.1083/jcb.9.2.493
McKinnell, I. W., Ishibashi, J., Le Grand, F., Punch, V. G., Addicks, G. C., Greenblatt, J. F., et al. (2008). Pax7 activates myogenic genes by recruitment of a histone methyltransferase complex. Nat. Cell Biol. 10, 77–84. doi: 10.1038/ncb1671
McPherron, A. C., Lawler, A. M., and Lee, S. J. (1997). Regulation of skeletal muscle mass in mice by a new TGF-beta superfamily member. Nature 387, 83–90. doi: 10.1038/387083a0
Melone, M. A., Peluso, G., Petillo, O., Galderisi, U., and Cotrufo, R. (1999). Defective growth in vitro of duchenne muscular dystrophy myoblasts: the molecular and biochemical basis. J. Cell. Biochem. 76, 118–132. doi: 10.1002/(SICI)1097-4644(20000101)76:1<118::AID-JCB12>3.0.CO;2-F
Miyata, K., Naito, M., Miyata, T., Mokuda, S., and Asahara, H. (2017). Bisulfite sequencing for DNA methylation analysis of primary muscle stem cells. Methods Mol. Biol. 1668, 3–13. doi: 10.1007/978-1-4939-7283-8_1
Niemann, H., Tian, X. C., King, W. A., and Lee, R. S. (2008). Epigenetic reprogramming in embryonic and foetal development upon somatic cell nuclear transfer cloning. Reproduction 135, 151–163. doi: 10.1530/REP-07-0397
Oikawa, Y., Omori, R., Nishii, T., Ishida, Y., Kawaichi, M., and Matsuda, E. (2011). The methyl-CpG-binding protein CIBZ suppresses myogenic differentiation by directly inhibiting myogenin expression. Cell Res. 21, 1578–1590. doi: 10.1038/cr.2011.90
Ono, Y., Urata, Y., Goto, S., Nakagawa, S., Humbert, P. O., Li, T. S., et al. (2015). Muscle stem cell fate is controlled by the cell-polarity protein scrib. Cell Rep. 10, 1135–1148. doi: 10.1016/j.celrep.2015.01.045
Otto, A., Schmidt, C., Luke, G., Allen, S., Valasek, P., Muntoni, F., et al. (2008). Canonical Wnt signalling induces satellite-cell proliferation during adult skeletal muscle regeneration. J. Cell Sci. 121, 2939–2950. doi: 10.1242/jcs.026534
Robinson, D. C. L., and Dilworth, F. J. (2018). Epigenetic regulation of adult myogenesis. Curr. Top. Dev. Biol 126, 235–284. doi: 10.1016/bs.ctdb.2017.08.002
Rossi, G., Antonini, S., Bonfanti, C., Monteverde, S., Vezzali, C., Tajbakhsh, S., et al. (2016). Nfix regulates temporal progression of muscle regeneration through modulation of myostatin expression. Cell Rep. 14, 2238–2249. doi: 10.1016/j.celrep.2016.02.014
Rudolf, A., Schirwis, E., Giordani, L., Parisi, A., Lepper, C., Taketo, M. M., et al. (2016). Beta-catenin activation in muscle progenitor cells regulates tissue repair. Cell Rep. 15, 1277–1290. doi: 10.1016/j.celrep.2016.04.022
Shu, J., Jelinek, J., Chang, H., Shen, L., Qin, T., Chung, W., et al. (2006). Silencing of bidirectional promoters by DNA methylation in tumorigenesis. Cancer Res. 66, 5077–5084. doi: 10.1158/0008-5472.CAN-05-2629
Stelzer, Y., Wu, H., Song, Y., Shivalila, C. S., Markoulaki, S., and Jaenisch, R. (2016). Parent-of-origin DNA methylation dynamics during mouse development. Cell Rep. 16, 3167–3180. doi: 10.1016/j.celrep.2016.08.066
Su, Y., Fan, Z., Wu, X., Li, Y., Wang, F., Zhang, C., et al. (2016). Genome-wide DNA methylation profile of developing deciduous tooth germ in miniature pigs. BMC Genomics 17:134. doi: 10.1186/s12864-016-2485-9
Wagner, J. R., Busche, S., Ge, B., Kwan, T., Pastinen, T., and Blanchette, M. (2014). The relationship between DNA methylation, genetic and expression inter-individual variation in untransformed human fibroblasts. Genome Biol. 15:R37. doi: 10.1186/gb-2014-15-2-r37
Wu, H., and Zhang, Y. (2014). Reversing DNA methylation: mechanisms, genomics, and biological functions. Cell 156, 45–68. doi: 10.1016/j.cell.2013.12.019
Yang, X., Han, H., De Carvalho, D. D., Lay, F. D., Jones, P. A., and Liang, G. (2014). Gene body methylation can alter gene expression and is a therapeutic target in cancer. Cancer Cell 26, 577–590. doi: 10.1016/j.ccr.2014.07.028
Yu, G., Wang, L. G., and He, Q. Y. (2015). ChIPseeker: an R/Bioconductor package for ChIP peak annotation, comparison and visualization. Bioinformatics 31, 2382–2383. doi: 10.1093/bioinformatics/btv145
Zhang, W., Xu, Y., Zhang, L., Wang, S., Yin, B., Zhao, S., et al. (2018). Synergistic effects of TGFbeta2, WNT9a, and FGFR4 signals attenuate satellite cell differentiation during skeletal muscle development. Aging Cell 17:e12788. doi: 10.1111/acel.12788
Zhang, Y., Liu, T., Meyer, C. A., Eeckhoute, J., Johnson, D. S., Bernstein, B. E., et al. (2008). Model-based analysis of ChIP-Seq (MACS). Genome Biol. 9:R137. doi: 10.1186/gb-2008-9-9-r137
Keywords: MeDIP-Seq, DNA methylation, Wnt, Tgfβ, satellite cells, skeletal muscle development
Citation: Zhang W, Zhang S, Xu Y, Ma Y, Zhang D, Li X and Zhao S (2019) The DNA Methylation Status of Wnt and Tgfβ Signals Is a Key Factor on Functional Regulation of Skeletal Muscle Satellite Cell Development. Front. Genet. 10:220. doi: 10.3389/fgene.2019.00220
Received: 11 November 2018; Accepted: 28 February 2019;
Published: 21 March 2019.
Edited by:
Amrendra Kumar Ajay, Harvard Medical School, United StatesReviewed by:
Rajni Kumar, Michigan State University, United StatesCopyright © 2019 Zhang, Zhang, Xu, Ma, Zhang, Li and Zhao. This is an open-access article distributed under the terms of the Creative Commons Attribution License (CC BY). The use, distribution or reproduction in other forums is permitted, provided the original author(s) and the copyright owner(s) are credited and that the original publication in this journal is cited, in accordance with accepted academic practice. No use, distribution or reproduction is permitted which does not comply with these terms.
*Correspondence: Shuhong Zhao, c2h6aGFvQG1haWwuaHphdS5lZHUuY24=
†These authors have contributed equally to this work as co-first authors
Disclaimer: All claims expressed in this article are solely those of the authors and do not necessarily represent those of their affiliated organizations, or those of the publisher, the editors and the reviewers. Any product that may be evaluated in this article or claim that may be made by its manufacturer is not guaranteed or endorsed by the publisher.
Research integrity at Frontiers
Learn more about the work of our research integrity team to safeguard the quality of each article we publish.