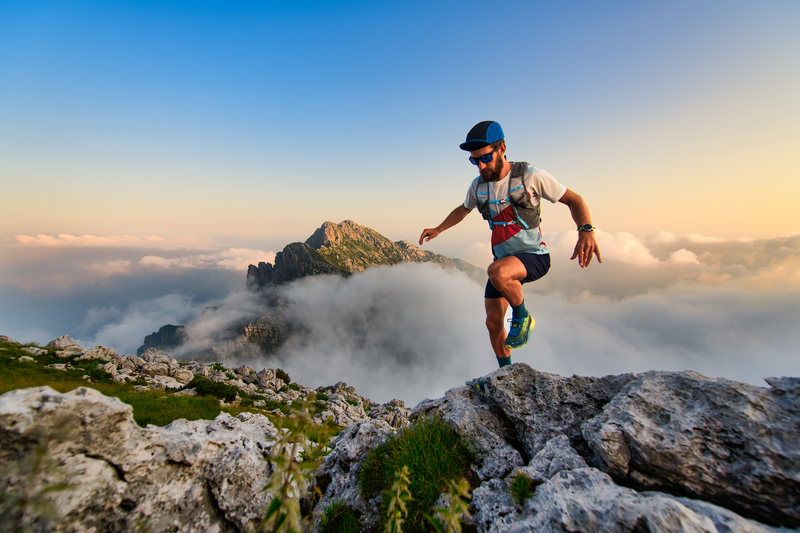
95% of researchers rate our articles as excellent or good
Learn more about the work of our research integrity team to safeguard the quality of each article we publish.
Find out more
ORIGINAL RESEARCH article
Front. Genet. , 28 September 2018
Sec. Computational Genomics
Volume 9 - 2018 | https://doi.org/10.3389/fgene.2018.00434
This article is part of the Research Topic Machine Learning Advanced Dynamic Omics Data Analysis for Precision Medicine View all 40 articles
Hundreds of genomic loci have been identified with the recent advances of schizophrenia in genome-wide association studies (GWAS) and sequencing studies. However, the functional interactions among those genes remain largely unknown. We developed a network-based approach to integrate multiple genetic risk factors, which lead to the discovery of new susceptibility genes and causal sub-networks, or pathways in schizophrenia. We identified significantly and consistently over-represented pathways in the largest schizophrenia GWA studies, which are highly relevant to synaptic plasticity, neural development and signaling transduction, such as long-term potentiation, neurotrophin signaling pathway, and the ERBB signaling pathway. We also demonstrated that genes targeted by common SNPs are more likely to interact with genes harboring de novo mutations (DNMs) in the protein-protein interaction (PPI) network, suggesting a mutual interplay of both common and rare variants in schizophrenia. We further developed an edge-based search algorithm to identify the top-ranked gene modules associated with schizophrenia risk. Our results suggest that the N-methyl-D-aspartate receptor (NMDAR) interactome may play a leading role in the pathology of schizophrenia, as it is highly targeted by multiple types of genetic risk factors.
Schizophrenia is a psychiatric disorder with profound genetic heterogeneity. Genetic risk factors of schizophrenia range in frequency from common to rare, including common single nucleotide polymorphisms (SNPs), recurrent rare copy number variants (CNVs) and de novo mutations (DNMs) (Friedman et al., 2008; International Schizophrenia Consortium, 2008; Vrijenhoek et al., 2008; Walsh et al., 2008; Xu et al., 2008; Glessner et al., 2010; Mulle et al., 2010; Girard et al., 2011; Levinson et al., 2011; Vacic et al., 2011; Kirov et al., 2012; Xu et al., 2012; Ripke et al., 2013; Sleiman et al., 2013; Fromer et al., 2014; Schizophrenia Working Group of the Psychiatric Genomics Consortium, 2014). Current genome-wide association studies (GWAS) in schizophrenia have reported 108 genome-wide significant loci, each of small effect size (Schizophrenia Working Group of the Psychiatric Genomics Consortium, 2014). It has also been reported that at least a quarter of the genetic contribution to schizophrenia risk can be explained by common SNPs (Lee et al., 2012; Ripke et al., 2013; Schizophrenia Working Group of the Psychiatric Genomics Consortium, 2014). On the other hand, multiple case-control studies have identified rare CNVs of strong effect to the risk of schizophrenia (International Schizophrenia Consortium, 2008; Vrijenhoek et al., 2008; Walsh et al., 2008; Xu et al., 2008; Glessner et al., 2010; Mulle et al., 2010; Levinson et al., 2011; Bergen et al., 2012; Kirov et al., 2012; Szatkiewicz et al., 2014). In addition, recent sequencing studies have shed new light on the genetic basis of schizophrenia that DNMs play a prominent part in the sporadic form of schizophrenia (Xu et al., 2012; Gulsuner et al., 2013; Fromer et al., 2014; McCarthy et al., 2014).
In these studies, multiple pieces of evidence show that genetic susceptibility of schizophrenia displays disruption across a group of functionally related genes implying a complex genetic network underlying schizophrenia (Glessner et al., 2010; Gulsuner et al., 2013; Fromer et al., 2014). To explore the network structure of schizophrenia, many network-based approaches have been applied to different types of genetic variations (Bullmore and Sporns, 2009; Gilman et al., 2012; Jia et al., 2012; Luo et al., 2014a,b). Among the different types of gene networks, protein-protein interaction (PPI) networks have been shown to be a powerful tool to identify the disease-associated modules and pathways, and reveal the biological significance of diverse genetic variations (Barabasi et al., 2011; Jia et al., 2011; Chang et al., 2013; Han et al., 2013; International Multiple Sclerosis Genetics Consortium, 2013; Leiserson et al., 2013; Luo et al., 2014b; Zhou et al., 2014). For example, instead of pursuing genome-wide significance, two GWA studies have successfully identified disease-associated gene modules, which are comprised of many closely interacting genes showing nominal significance, by integrating PPI networks analysis into GWAS (Han et al., 2013; International Multiple Sclerosis Genetics Consortium, 2013). However, it is still a challenge to conduct a comprehensive PPI network analysis, in particular by incorporating different types of genetic factors from different tissue types.
In the present study, we established a network-based approach to investigate the gene modules and pathways underlying schizophrenia, and to explore the inherent associations among multiple genetic risk factors. Our analysis uncovered significantly enriched association signals in pathways relevant to synaptic plasticity, neural development and signaling transduction such as long-term potentiation, neurotrophin signaling pathway, ERBB signaling pathway and MAPK signaling pathway, suggesting those play contributory roles in the pathophysiology of schizophrenia. We also demonstrated that genes targeted by common SNPs are more likely to interact with genes carrying DNMs. Finally, we identified a group of interacting genes showing a significant combined effect to the genetic susceptibility of schizophrenia.
Gene-level P values were calculated based on SNP P values from the largest GWAS conducted by Schizophrenia Psychiatric Genome-Wide Association Study Consortium (PGC), which recruited 36,989 cases and 113,075 controls (PGC phase 2, abbreviated as PGC2) (Schizophrenia Working Group of the Psychiatric Genomics Consortium, 2014). The association results were downloaded from the website of PGC1. As a control, we used the GWAS data of Crohn’s disease (CD) from the International IBD Genetics Consortium2 including a total of 3,685 cases and 5,968 controls (Jostins et al., 2012).
Gene-level associations were calculated by VEGAS (Liu et al., 2010). VEGAS performs Monte-Carlo simulations from the multivariate normal distribution based on the LD pattern from reference populations and assigns an estimated P value to each gene. SNPs located within 50 kb upstream and 50 kb down stream of gene boundaries are used in the analysis in order to capture regulatory regions and SNPs in LD. Previous studies suggested P-value < 0.05 as the threshold of gene-level significance (Liu et al., 2010; International Multiple Sclerosis Genetics Consortium, 2013). However, since the number of genome-wide significant loci from the PGC2 study are much more than from the previous studies as a result of study size differences, the gene-level significance at both P-value < 0.01 (2501 significant genes) and P-value < 0.05 (4698 significant genes) was evaluated in this study. Genes located in the MHC region (25–34 mb on chr6) were excluded in the analysis.
In this study, we used the sequencing results from previous studies (Xu et al., 2012; Gulsuner et al., 2013; Fromer et al., 2014) and annotated the variants by wANNOVAR3 (Chang and Wang, 2012). We used SIFT and Polyphen2 (HDIV) scores compiled by dbNSFP2 database as well as the AVSIFT score based on annotations at http://sift.bii.a-star.edu.sg to assess whether the missense variants are benign or damaging (Supplementary Table S1).
For the CNVs, we collected the genes disrupted by CNVs reported in large case-control studies of schizophrenia (Supplementary Table S2).
Schematic overview of the network analysis pipeline in this study was provided in Supplementary Figure S2.
The PPI Network was constructed based on the database iRefindex, which collected the protein interactions from a number of primary interaction databases (Razick et al., 2008). In order to control the rate of false positive interactions, we selected only those interactions that were supported by at least two independent PubMed literatures. A high-confidence network with 9,090 proteins (nodes) and 25,864 interactions (edges) was subsequently built for downstream analyses.
We next mapped the significant genes (P < 0.05) identified by VEGAS to the PPI network, and obtained a sub-network comprised of the significant genes and the interactions among them. The sub-network contains several connected components and many singletons. We then extracted the largest connected component (LCC) of the sub-network for downstream analysis.
To test whether the size of the LCC is larger than what would be expected by chance, we randomly assigned P values of the same network and generated the simulated LCCs. We repeated this procedure 10,000 times, and use these simulations as background to estimate the significance of the LCCs generated from the real data (Figure 1 and Supplementary Table S3). To investigate the biological significance of the genes in the LCC, we carried out a gene function enrichment analysis against the KEGG database using DAVID (Supplementary Table S4) (Huang et al., 2007).
FIGURE 1. (A,B) Comparison of the number of nodes between the real network and random networks. Connectedness of the LCC based on gene-wise significant genes (Pgene < 0.01) from PGC2 study. The background distributions are generated by the number of nodes and edges of LCCs from 10,000 random simulations. P values are estimated by the proportion of LCCs from 10,000 random networks with more nodes or edges than the real network. Both node and edge numbers of the real data are significantly larger than random simulations (Pnode = 0.0012; Pedge = 0.0003).
Gens algorithm is modified based on a previously published node-based network search method (Ideker et al., 2002; Chuang et al., 2007; Jia et al., 2011).
Gens first assigns a weight to each edge of the network calculated by the gene-wise P values and mRNA expression correlations of interacting gene pairs (Supplementary Data Sheet 1). The weight of each edge is defined as
where Cij denotes the Pearson Correlation Coefficient of interacting gene pairs, gene i and gene j. Pi is the P value of Gene i, Pj is the P value of Gene j.
The gene mRNA expression data were downloaded from Allen Brain Atlas4
The weight of each edge was then converted into a Z score
where ϕ−1 represents the inverse normal cumulative distribution function.
The score of gene module is defined as
where k is the number of edges in the module.
The search procedure starts from the seed edge, neighborhood interactors are added into the module if they can yield an increment greater than Zm×r, r is set to 0.05 in this study.
To evaluate the likelihood of the detected modules were identified by chance, Gens creates a background distribution by scoring 100,000 randomly generated modules with the same number of genes as the detected module. The significance is calculated as the proportion of those random generated modules whose Zm are larger than or equal to that of the identified module. Gens also adjusted the identified module size by defining a normalized module score
where Zm(π) represents the distribution of Zm generated by 100,000 simulations.
We first used VEGAS to convert the SNP associations into gene-level P values (Supplementary Figure S1). We next extracted the sub-networks by genes with a significant gene-level P value. The identified sub-networks are comprised of connected components and singletons. Among the connected components, the LCC contains most of the nodes and edges in the sub-network, which may participate in potential pathways underlying schizophrenia. To investigate the biological significance of the LCCs, we carried out a gene function enrichment analysis on the gene set of LCCs. We found significantly over-represented KEGG pathways, which are highly relevant to synaptic plasticity, neural development and signaling transduction such as long-term potentiation, neurotrophin signaling pathway, ERBB signaling pathway, MAPK signaling pathway, and T cell receptor signaling pathway. Other enriched pathways include proteasome, ubiquitin mediated proteolysis pathway and multiple cancers associated pathways (Supplementary Table S4).
We further confirmed that the sizes of LCCs are significantly larger than the LCCs generated by simulated random networks (Figure 1 and Supplementary Table S3). For comparison, we performed the same analysis on a CD cohort, the LCC size is also larger than random simulations (Supplementary Table S3). This result is consistent with a previous study pointing to a biological plausibility that a set of genes coherently contribute to disease risk through interactive co-function and co-regulation (International Multiple Sclerosis Genetics Consortium, 2013).
To examine whether genes belonging to the LCC network and identified by GWAS data are more likely to interact with genes harboring DNMs, We added the genes carrying potential DNMs (frameshift insertions/deletions, missense variants, or nonsense variants) and extracted the LCC based on the merged gene set. The size of the LCC significantly increased, larger than 10,000 simulations of the above procedure based on the same number of randomly selected genes. As a control, we tested the same number of top significant genes from CD GWAS. The size of the resulting LCC was not significantly different from random simulations. Furthermore, we also found the size of LCC did not increase significantly than random simulations if genes with silent de novo variants in schizophrenia cases were included (Figure 2 and Supplementary Table S5).
FIGURE 2. (A,B) Comparison of the number of nodes between the real network (damaging events) and random networks. (C,D) Comparison of the number of nodes between the real network (benign events) and random networks. Connectedness of the LCC based on gene-level significant genes (Pgene < 0.01) from PGC2 study and genes harboring DNMs. Original size of LCC based on gene-wise significant genes constituting 402 nodes and 620 edges. 635 genes harboring DNMs are added to generate the new LCC. The background distribution is generated by 10,000 LCCs based on adding 635 random selected genes. P values are estimated by the proportion of LCCs from random networks with more nodes or edges than the real network. As a control, we use the LCC generated by adding top 635 gene-level significant genes from Crohn’s disease as control. Dash line denotes the size of LCC generated by adding DNMs. Solid line denotes the size of LCC generated by adding CD top genes. Adding DNMs significantly increased the size of LCCs (DNMs: Pnode = 0.0022, Pedge = 0.0032; CD: Pnode = 0.1941, Pedge = 0.0678), while adding top CD genes did not. For comparison, we also added the synonymous and non-frameshift substitutions to generate the new LCC. The size of new LCC is not significantly larger than random simulations (Benign substitutions: Pnode = 0.698, Pedge = 0.0571; CD: Pnode = 0.1922, Pedge = 0.1900).
In an attempt to add some more understanding to the schizophrenia genetic puzzle, we collected evidence for literature reported genes that are known to be disrupted by CNVs in schizophrenia patients (Supplementary Table S2), and added them to the PPI network analysis. We subsequently derived the LCC from genes targeted by SNPs, DNMs, and CNVs.
To pinpoint a small group of interactive genes with significant combined/additive effect to schizophrenia, we developed an edge-based network search algorithm (Gens) for detecting causal gene modules in PPI networks (Supplementary Figure S2). The results from gene-level significance at both 0.05 and 0.01 were highly consistent with each other demonstrating that the top-ranked gene modules overlapped considerably in their gene content. The shared genes between top-ranked modules significantly pointed to the interactome of N-methyl-D-aspartate receptor (NMDAR) genes including DLG1, DLG2, DLG4, ERBB4, GRIN2A, and GRIN2B (Supplementary Figure S3). All of those genes exhibited strong associations with schizophrenia susceptibility (DLG1, rs436564, P = 8.97 × 10−4; DLG2, rs12294291, P = 4.90 × 10−7; DLG4, rs222854, P = 3.76 × 10−5; ERBB4, rs16846200, P = 1.62 × 10−5; GRIN2A, rs9922678, P = 6.72 × 10−9; GRIN2B, rs11757887, P = 8.81 × 10−7; Supplementary Figure S4) with GRIN2A, reaching genome-wide significance in the PGC2 study.
Some of the NMDAR genes are also targeted by rare variations. For example, DLG1 and GRIN2A have been reported to be targeted by DNMs; DLG1, DLG2, and ERBB4 have been reported to be targeted by CNVs. To further explore the risk genes from the PPI network, we next select all the gene modules with P < 0.05 (P value calculated by random simulation, see Methods) and calculated the frequency of genes occurring in the selected modules. Genes with the frequency above the upper quartiles were defined as ‘top genes’. The ‘top genes’ was used to construct a new PPI network of 152 nodes and 324 edges (Figure 3), which reflects the most significant gene module derived from the network analysis.
FIGURE 3. PPI network visualization of the most significant gene module derived from the network analysis. Gene-level P values (<0.05) are colored from green to red. Genes harboring DNMs and CNVs are shown as circles, and triangles respectively. Genes harboring both DNMs and CNVs are diamond shaped. Edges width reflects the gene co-expression correlation between two connected nodes. Solid and dash line denote positive, and negative correlations respectively.
Enrichment analysis indicated that they are enriched in the neurotrophin signaling pathway (P = 7.27 × 10−13), ERBB signaling pathway (P = 1.84 × 10−7), long-term potentiation (P = 5.37 × 10−5), MAPK signaling pathway (P = 3.16 × 10−5), T cell receptor signaling pathway (P = 1.17 × 10−5), and pathways in cancer (P = 4.87 × 10−8) to name a few (Supplementary Table S6). Moreover, in this network, we found multiple genes are connected with the core members of NMDAR interactome, such as ATP2B2, DLGAP, MAP1A, NOS1, PTK2B, PTPRG and PRKCA. Among them, ATP2B2 (rs9879311, P = 2.77 × 10−6) and NOS1 (rs2293052, P = 1.24 × 10−6) exhibited strong associations with schizophrenia risk in the PGC2 GWAS.
Beside the NMDAR interactome, we also found candidate genes showing strong associations with schizophrenia risk in the network, such as ANKS1B (rs10745841, P = 1.28 × 10−6), CHUK (rs975752, P = 2.52 × 10−6), CNTN2 (rs16937, P = 8.69 × 10−7), CNTNAP2 (rs6961013, P = 4.80 × 10−5), CREB1 (rs2709410, P = 4.07 × 10−6), CREB5 (rs4722797, P = 7.58 × 10−6; rs887622, P = 8.79 × 10−6), CUL3 (rs11685299, P = 1.11 × 10−8), EP300 (rs9607782, P = 6.76 × 10−12), GABBR2 (rs2304389, P = 3.81 × 10−7), GNA13 (rs11868185, P = 4.44 × 10−5), NCOR2 (rs2229840, P = 2.90 × 10−4), NTRK3 (rs146797905, P = 3.35 × 10−7; rs8042993, P = 7.84 × 10−6), PAK2 (rs10446497, P = 5.30 × 10−6), PTK2 (rs4961278, P = 1.86 × 10−5), PTK2B (rs2565065, P = 1.94 × 10−7), PTN (rs3735025, P = 7.75 × 10−9), PTPRF (rs11210892, P = 4.97 × 10−10), STK4 (rs6065777, P = 5.92 × 10−6), TCF4 (rs9636107, P = 9.09 × 10−13). Among them, CUL3, EP300, NCOR2, PTK2B, and PTPRF were targeted by DNMs, and PAK2, PARK2 and PTK2 were targeted by CNVs.
Given the heterogeneity and complexity of the genomic landscape in schizophrenia, we employed multiple network-based methods to reveal the instinct associations among different types of genetic risk variants, resulting in the discovery of novel gene modules and pathways underlying schizophrenia (Supplementary Figure S2).
With the recent GWAS success measures in schizophrenia uncovering 108 genome-wide significant loci (Schizophrenia Working Group of the Psychiatric Genomics Consortium, 2014), the genetic underpinning of this complex disease have begun to unravel. However, a considerable number of nominally significant loci are likely to be identified in future studies through the analysis of larger sample sizes or the application of new and innovative methods. For example, the schizophrenia susceptibility gene CAMKK2 showing nominal significance (rs1063843, P = 2.32 × 10−5) in the PGC2 study was successfully identified by integrative analysis of gene expression and PPI (Luo X.J. et al., 2014).
We hypothesize that a group of functionally related genes with nominal significance could jointly contribute to schizophrenia susceptibility. We further performed a PPI network-based pathway analysis on two GWA studies of schizophrenia and identified significantly enriched KEGG pathways in both studies. Some pathways have been strongly associated with schizophrenia, such as the long-term potentiation, ERBB signaling pathway and MAPK signaling pathway (Fazzari et al., 2010; Pitcher et al., 2011; Funk et al., 2012; Savanthrapadian et al., 2013; Salavati et al., 2015). Interestingly, we found both the proteasome pathway and the ubiquitin mediated proteolysis pathway to be significantly enriched (Supplementary Table S4). Dysfunction of the ubiquitin-proteasome pathway (UPP) has been implicated in the pathology of various neurodegenerative conditions, and has been linked to several late-onset neurodegenerative diseases caused by aggregate-prone proteins such as Alzheimer’s disease Parkinson’s disease and Huntington’s disease (Rubinsztein, 2006; Hegde and Upadhya, 2011). Cumulative evidence also suggests that schizophrenia patients have aberrant gene expression patterns and protein expression disruptions in the UPP suggesting the UPP may also contribute to the deficits in schizophrenia (Vawter et al., 2001; Aston et al., 2004; Altar et al., 2005; Bousman et al., 2010; Rubio et al., 2013). Our results are consistent with these findings and provide new evidence in support of the association between the UPP and the pathogenesis of schizophrenia.
Cumulative evidence suggests that DNMs are an important cause of mental disorders such as schizophrenia, autism and intellectual disability (Veltman and Brunner, 2012). DNMs occur in different genes of different patients may be collectively responsible for a portion of sporadic schizophrenia cases. However, unlike CNVs, genes recurrently mutated by SNVs are rare and the overlap of genes disrupted by DNMs from recent studies is also small (Supplementary Figure S5). Thus, we naturally raise the question if genes targeted by common SNPs are more likely to be targeted by DNMs, and if genes targeted by common SNPs are more likely to interact with genes carrying DNMs? For the first question, the PGC2 study unveiled significant overlap between genes in the schizophrenia GWAS associated intervals and those with DNMs in schizophrenia (P = 0.0061) (Schizophrenia Working Group of the Psychiatric Genomics Consortium, 2014). For the second question, our analysis provides new evidence suggesting that genes targeted by common SNPs or DNMs are likely to interact with each other or participant in the same pathway. Collectively, these results suggest that schizophrenia susceptibility involves a mutual interplay of both common and rare genetic risk factors.
We additionally developed an edge-based network search algorithm to identify the leading disease associated modules underlying schizophrenia. The network search method was initially node-based, and developed in order to detect a group of interactive genes which show significantly changes in mRNA expression (Ideker et al., 2002). Later, this method was successfully applied on the post-GWAS network analysis (Jia et al., 2011; Jia et al., 2012; Han et al., 2013; International Multiple Sclerosis Genetics Consortium, 2013). Here, the advantage of Gens is that the edge-based method can utilize not only the node P values for the node but also the gene co-expression information as edge weights to score and rank the detected modules (Methods).
Using this approach, we found the top-ranked modules were significantly enriched in the NMDAR pathway associated genes including DLG1, DLG2, DLG4, ERBB4, GRIN2A, and GRIN2B. All of those genes show strong association with schizophrenia from GWAS. DLG1, DLG2, ERBB4, and GRIN2A were also targeted by DNMs or CNVs. In addition to GRIN2A, which has surpassed genome-wide significance (rs9922678, P = 6.72 × 10−9) in the PGC2 study, DLG2 (rs12294291, P = 4.90 × 10−7), GRIN2B (rs11757887, P = 8.81 × 10−7) also showed strong associations nearly reaching genome-wide significance. These results suggested that the dysfunction of the NMDAR complex plays a leading role in the pathology of schizophrenia and is highly impacted by multiple genetic risk factors.
We further pinpointed two genes ATP2B2 (rs9879311, P = 2.77 × 10−6) and NOS1 (rs2293052, P = 1.24 × 10−6), which were closely connected to the NMDAR interactome and showed strong associations with schizophrenia risk. ATP2B2 encodes the plasma membrane calcium-transporting ATPase 2 which plays an important role in intracellular calcium homeostasis and extrudes Ca2+ from cytosol into extracellular space. Family-based association studies suggested ATP2B2 as a risk gene for autism in multiple ethnicities (Carayol et al., 2011; Prandini et al., 2012; Yang et al., 2013). A previous study also suggested ATP2B2 could confer risk to schizophrenia (Ikeda et al., 2010). NOS1 encodes a member of nitric oxide synthases, which functions as a biologic mediator in neurotransmission. Previous studies also provided evidence of the associations between NOS1 and schizophrenia risk (Shinkai et al., 2002; Reif et al., 2011; Zhang et al., 2014).
Besides the NMDAR interactome, CUL3, EP300, PTN, PTPRF, TCF4 reached genome-wide significance in the PGC2 study. CUL3, EP300, and PTPRF were also targeted by DNMs. EP300 servers as an important hub in the network which directly interacted with 14 genes (TCF4, EGR1, SREBF1, and SREBF2 located in genome-wide significant regions; AKT1 and SMAD7 targeted by DNMs). The product of EP300 functions as histone acetyltransferase and regulates transcription via chromatin remodeling. Defects of EP300 can cause Rubinstein-Taybi syndrome (a disease with short stature and intellectual disability) and may result in the formation of tumors (Tillinghast et al., 2003; Roelfsema et al., 2005; Negri et al., 2015). Interestingly, the DNM (NM_001429, exon14, c.C2656G, p.P886A) found in EP300 is not predicted as damaging by either SIFT nor PolyPhen2, and a common missense variant in EP300 is also strongly associated with schizophrenia (rs20551, P = 1.38 × 10−8; NM_001429, exon15, c.A2989G, p.I997V), which suggest that slight changes in the protein conformation of EP300 may confer risk to schizophrenia. EP300 is also interacted and co-expressed with CREB1 in the network. It is reported that EP300 can mediate cAMP-gene regulation through phosphorylated CREB proteins. CREB1 also showed strong association (rs2709410, P = 4.07 × 10−6) in the PGC2 study. CREB1 has been linked to drug addiction, memory disorders and neurodegenerative diseases (Bilecki and Przewlocki, 2000; Nestler, 2002; Josselyn and Nguyen, 2005; Lee et al., 2005). There is also some evidence of the association between CREB1 and schizophrenia (Li et al., 2013; Ma et al., 2014; Kumar et al., 2015). PTN is another important hub, which interacted with eight genes (NCAN, PSMB10, and SGSM2 located in genome-wide significant regions; NCAN, PSMD2, and SGSM2 targeted by DNMs). PTN encodes pleiotrophin, which may suppress long-term potentiation induction (Pavlov et al., 2002).
In the network, candidate genes with nominal significance such as ANKS1B, CNTN2, CNTNAP2, GABBR2, NCOR2, and NTRK3 also may be involved in the pathology of schizophrenia. The product of ANKS1B is predominantly expressed in brain tissue and interacted with amyloid beta protein precursor, which may play a role in brain development. A recent study demonstrated that ANKS1B product regulates synaptic GluN2B levels and further influence the NMDAR function. Multiple pieces of evidence have linked CNTN2, CNTNAP2, GABBR2, and NTRK3 to neuropsychiatric disorders, including schizophrenia (Weickert et al., 2005; Friedman et al., 2008; Otnaess et al., 2009; Fazzari et al., 2010; Fatemi et al., 2011; Roussos et al., 2012; Bormuth et al., 2013; Fatemi et al., 2013; Karayannis et al., 2014). SNPs in NCOR2 are associated with cocaine dependence in a recent GWAS (Gelernter et al., 2014).
In conclusion, the heterogeneity and complexity of the genetic landscape in schizophrenia is high. Here, we demonstrate that common and rare genetic risk factors converge on PPI networks that are enriched for schizophrenia candidate genes involved in synaptic plasticity and neural development. We also provide new evidence demonstrating that the NMDAR interactome is highly targeted by multiple types of genetic risk factors and may play a leading role in the risk of schizophrenia. Furthermore, we pinpointed many nominally significant genes in GWAS showing strong evidence to influence schizophrenia risk according to their network properties. These genes may reach genome-wide significance or carry DNMs to be unveiled in further genetic studies with more samples.
XC and HH designed the research. XC, LL, YL, and JL performed the analysis. QL and PS provided guidance for the analysis. XC and HH wrote and finalized the paper.
This study is funded by an Institutional Development Award to the Center for Applied Genomics from The Children’s Hospital of Philadelphia; Adele and Daniel Kubert donation; MH096891-03S1 (NIMH); RC2 MH089924-02 (NIMH); R01 MH097284-03 (NIMH); U01-HG008684 (NIH).
QL was employed by company Janssen Research & Development, LCC.
The remaining authors declare that the research was conducted in the absence of any commercial or financial relationships that could be construed as a potential conflict of interest.
The Supplementary Material for this article can be found online at: https://www.frontiersin.org/articles/10.3389/fgene.2018.00434/full#supplementary-material
Altar, C. A., Jurata, L. W., Charles, V., Lemire, A., Liu, P., Bukhman, Y., et al. (2005). Deficient hippocampal neuron expression of proteasome, ubiquitin, and mitochondrial genes in multiple schizophrenia cohorts. Biol. Psychiatry 58, 85–96. doi: 10.1016/j.biopsych.2005.03.031
Aston, C., Jiang, L., and Sokolov, B. P. (2004). Microarray analysis of postmortem temporal cortex from patients with schizophrenia. J. Neurosci. Res. 77, 858–866. doi: 10.1002/jnr.20208
Barabasi, A. L., Gulbahce, N., and Loscalzo, J. (2011). Network medicine: a network-based approach to human disease. Nat. Rev. Genet. 12, 56–68. doi: 10.1038/nrg2918
Bergen, S. E., O’dushlaine, C. T., Ripke, S., Lee, P. H., Ruderfer, D. M., Akterin, S., et al. (2012). Genome-wide association study in a Swedish population yields support for greater CNV and MHC involvement in schizophrenia compared with bipolar disorder. Mol. Psychiatry 17, 880–886. doi: 10.1038/mp.2012.73
Bilecki, W., and Przewlocki, R. (2000). Effect of opioids on Ca2 + /cAMP responsive element binding protein. Acta Neurobiol. Exp. 60, 557–567.
Bormuth, I., Yan, K., Yonemasu, T., Gummert, M., Zhang, M., Wichert, S., et al. (2013). Neuronal basic helix-loop-helix proteins Neurod2/6 regulate cortical commissure formation before midline interactions. J. Neurosci. 33, 641–651. doi: 10.1523/JNEUROSCI.0899-12.2013
Bousman, C. A., Chana, G., Glatt, S. J., Chandler, S. D., Lucero, G. R., Tatro, E., et al. (2010). Preliminary evidence of ubiquitin proteasome system dysregulation in schizophrenia and bipolar disorder: convergent pathway analysis findings from two independent samples. Am. J. Med. Genet. B Neuropsychiatr. Genet. 153B, 494–502. doi: 10.1002/ajmg.b.31006
Bullmore, E., and Sporns, O. (2009). Complex brain networks: graph theoretical analysis of structural and functional systems. Nat. Rev. Neurosci. 10, 186–198. doi: 10.1038/nrn2575
Carayol, J., Sacco, R., Tores, F., Rousseau, F., Lewin, P., Hager, J., et al. (2011). Converging evidence for an association of ATP2B2 allelic variants with autism in male subjects. Biol. Psychiatry 70, 880–887. doi: 10.1016/j.biopsych.2011.05.020
Chang, X., and Wang, K. (2012). wANNOVAR: annotating genetic variants for personal genomes via the web. J. Med. Genet. 49, 433–436. doi: 10.1136/jmedgenet-2012-100918
Chang, X., Xu, T., Li, Y., and Wang, K. (2013). Dynamic modular architecture of protein-protein interaction networks beyond the dichotomy of ‘date’ and ‘party’ hubs. Sci. Rep. 3:1691. doi: 10.1038/srep01691
Chuang, H. Y., Lee, E., Liu, Y. T., Lee, D., and Ideker, T. (2007). Network-based classification of breast cancer metastasis. Mol. Syst. Biol. 3:140.
Fatemi, S. H., Folsom, T. D., Rooney, R. J., and Thuras, P. D. (2013). Expression of GABAA alpha2-, beta1- and epsilon-receptors are altered significantly in the lateral cerebellum of subjects with schizophrenia, major depression and bipolar disorder. Transl. Psychiatry 3:e303. doi: 10.1038/tp.2013.64
Fatemi, S. H., Folsom, T. D., and Thuras, P. D. (2011). Deficits in GABA(B) receptor system in schizophrenia and mood disorders: a postmortem study. Schizophr. Res. 128, 37–43. doi: 10.1016/j.schres.2010.12.025
Fazzari, P., Paternain, A. V., Valiente, M., Pla, R., Lujan, R., Lloyd, K., et al. (2010). Control of cortical GABA circuitry development by Nrg1 and ErbB4 signalling. Nature 464, 1376–1380. doi: 10.1038/nature08928
Friedman, J. I., Vrijenhoek, T., Markx, S., Janssen, I. M., Van Der Vliet, W. A., Faas, B. H., et al. (2008). CNTNAP2 gene dosage variation is associated with schizophrenia and epilepsy. Mol. Psychiatry 13, 261–266. doi: 10.1038/sj.mp.4002049
Fromer, M., Pocklington, A. J., Kavanagh, D. H., Williams, H. J., Dwyer, S., Gormley, P., et al. (2014). De novo mutations in schizophrenia implicate synaptic networks. Nature 506, 179–184. doi: 10.1038/nature12929
Funk, A. J., Mccullumsmith, R. E., Haroutunian, V., and Meador-Woodruff, J. H. (2012). Abnormal activity of the MAPK- and cAMP-associated signaling pathways in frontal cortical areas in postmortem brain in schizophrenia. Neuropsychopharmacology 37, 896–905. doi: 10.1038/npp.2011.267
Gelernter, J., Sherva, R., Koesterer, R., Almasy, L., Zhao, H., Kranzler, H. R., et al. (2014). Genome-wide association study of cocaine dependence and related traits: FAM53B identified as a risk gene. Mol. Psychiatry 19, 717–723. doi: 10.1038/mp.2013.99
Gilman, S. R., Chang, J., Xu, B., Bawa, T. S., Gogos, J. A., Karayiorgou, M., et al. (2012). Diverse types of genetic variation converge on functional gene networks involved in schizophrenia. Nat. Neurosci. 15, 1723–1728. doi: 10.1038/nn.3261
Girard, S. L., Gauthier, J., Noreau, A., Xiong, L., Zhou, S., Jouan, L., et al. (2011). Increased exonic de novo mutation rate in individuals with schizophrenia. Nat. Genet. 43, 860–863. doi: 10.1038/ng.886
Glessner, J. T., Reilly, M. P., Kim, C. E., Takahashi, N., Albano, A., Hou, C., et al. (2010). Strong synaptic transmission impact by copy number variations in schizophrenia. Proc. Natl. Acad. Sci. U.S.A. 107, 10584–10589. doi: 10.1073/pnas.1000274107
Gulsuner, S., Walsh, T., Watts, A. C., Lee, M. K., Thornton, A. M., Casadei, S., et al. (2013). Spatial and temporal mapping of de novo mutations in schizophrenia to a fetal prefrontal cortical network. Cell 154, 518–529. doi: 10.1016/j.cell.2013.06.049
Han, S., Yang, B. Z., Kranzler, H. R., Liu, X., Zhao, H., Farrer, L. A., et al. (2013). Integrating GWASs and human protein interaction networks identifies a gene subnetwork underlying alcohol dependence. Am. J. Hum. Genet. 93, 1027–1034. doi: 10.1016/j.ajhg.2013.10.021
Hegde, A. N., and Upadhya, S. C. (2011). Role of ubiquitin-proteasome-mediated proteolysis in nervous system disease. Biochim. Biophys. Acta 1809, 128–140. doi: 10.1016/j.bbagrm.2010.07.006
Huang, D. W., Sherman, B. T., Tan, Q., Kir, J., Liu, D., Bryant, D., et al. (2007). DAVID bioinformatics resources: expanded annotation database and novel algorithms to better extract biology from large gene lists. Nucleic Acids Res. 35, W169–W175. doi: 10.1093/nar/gkm415
Ideker, T., Ozier, O., Schwikowski, B., and Siegel, A. F. (2002). Discovering regulatory and signalling circuits in molecular interaction networks. Bioinformatics 18(Suppl. 1), S233–S240. doi: 10.1093/bioinformatics/18.suppl_1.S233
Ikeda, M., Tomita, Y., Mouri, A., Koga, M., Okochi, T., Yoshimura, R., et al. (2010). Identification of novel candidate genes for treatment response to risperidone and susceptibility for schizophrenia: integrated analysis among pharmacogenomics, mouse expression, and genetic case-control association approaches. Biol. Psychiatry 67, 263–269. doi: 10.1016/j.biopsych.2009.08.030
International Multiple Sclerosis Genetics Consortium (2013). Network-based multiple sclerosis pathway analysis with GWAS data from 15,000 cases and 30,000 controls. Am. J. Hum. Genet. 92, 854–865. doi: 10.1016/j.ajhg.2013.04.019
International Schizophrenia Consortium (2008). Rare chromosomal deletions and duplications increase risk of schizophrenia. Nature 455, 237–241. doi: 10.1038/nature07239
Jia, P., Wang, L., Fanous, A. H., Pato, C. N., Edwards, T. L., International Schizophrenia Consortium., et al. (2012). Network-assisted investigation of combined causal signals from genome-wide association studies in schizophrenia. PLoS Comput. Biol. 8:e1002587. doi: 10.1371/journal.pcbi.1002587
Jia, P., Zheng, S., Long, J., Zheng, W., and Zhao, Z. (2011). dmGWAS: dense module searching for genome-wide association studies in protein-protein interaction networks. Bioinformatics 27, 95–102. doi: 10.1093/bioinformatics/btq615
Josselyn, S. A., and Nguyen, P. V. (2005). CREB, synapses and memory disorders: past progress and future challenges. Curr. Drug Targets CNS Neurol. Disord. 4, 481–497. doi: 10.2174/156800705774322058
Jostins, L., Ripke, S., Weersma, R. K., Duerr, R. H., Mcgovern, D. P., Hui, K. Y., et al. (2012). Host-microbe interactions have shaped the genetic architecture of inflammatory bowel disease. Nature 491, 119–124. doi: 10.1038/nature11582
Karayannis, T., Au, E., Patel, J. C., Kruglikov, I., Markx, S., Delorme, R., et al. (2014). Cntnap4 differentially contributes to GABAergic and dopaminergic synaptic transmission. Nature 511, 236–240. doi: 10.1038/nature13248
Kirov, G., Pocklington, A. J., Holmans, P., Ivanov, D., Ikeda, M., Ruderfer, D., et al. (2012). De novo CNV analysis implicates specific abnormalities of postsynaptic signalling complexes in the pathogenesis of schizophrenia. Mol. Psychiatry 17, 142–153. doi: 10.1038/mp.2011.154
Kumar, G., Clark, S. L., Mcclay, J. L., Shabalin, A. A., Adkins, D. E., Xie, L., et al. (2015). Refinement of schizophrenia GWAS loci using methylome-wide association data. Hum. Genet. 134, 77–87. doi: 10.1007/s00439-014-1494-5
Lee, J., Kim, C. H., Simon, D. K., Aminova, L. R., Andreyev, A. Y., Kushnareva, Y. E., et al. (2005). Mitochondrial cyclic AMP response element-binding protein (CREB) mediates mitochondrial gene expression and neuronal survival. J. Biol. Chem. 280, 40398–40401. doi: 10.1074/jbc.C500140200
Lee, S. H., Decandia, T. R., Ripke, S., Yang, J., Schizophrenia Psychiatric Genome-Wide Association Study Consortium, International Schizophrenia Consortium, et al. (2012). Estimating the proportion of variation in susceptibility to schizophrenia captured by common SNPs. Nat. Genet. 44, 247–250. doi: 10.1038/ng.1108
Leiserson, M. D., Eldridge, J. V., Ramachandran, S., and Raphael, B. J. (2013). Network analysis of GWAS data. Curr. Opin. Genet. Dev. 23, 602–610. doi: 10.1016/j.gde.2013.09.003
Levinson, D. F., Duan, J., Oh, S., Wang, K., Sanders, A. R., Shi, J., et al. (2011). Copy number variants in schizophrenia: confirmation of five previous findings and new evidence for 3q29 microdeletions and VIPR2 duplications. Am. J. Psychiatry 168, 302–316. doi: 10.1176/appi.ajp.2010.10060876
Li, S., Liu, Z. C., Yin, S. J., Chen, Y. T., Yu, H. L., Zeng, J., et al. (2013). Human endogenous retrovirus W family envelope gene activates the small conductance Ca2 + -activated K + channel in human neuroblastoma cells through CREB. Neuroscience 247, 164–174. doi: 10.1016/j.neuroscience.2013.05.033
Liu, J. Z., Mcrae, A. F., Nyholt, D. R., Medland, S. E., Wray, N. R., Brown, K. M., et al. (2010). A versatile gene-based test for genome-wide association studies. Am. J. Hum. Genet. 87, 139–145. doi: 10.1016/j.ajhg.2010.06.009
Luo, X., Huang, L., Han, L., Luo, Z., Hu, F., Tieu, R., et al. (2014a). Systematic prioritization and integrative analysis of copy number variations in schizophrenia reveal key schizophrenia susceptibility genes. Schizophr. Bull. 40, 1285–1299. doi: 10.1093/schbul/sbu045
Luo, X., Huang, L., Jia, P., Li, M., Su, B., Zhao, Z., et al. (2014b). Protein-protein interaction and pathway analyses of top schizophrenia genes reveal schizophrenia susceptibility genes converge on common molecular networks and enrichment of nucleosome (chromatin) assembly genes in schizophrenia susceptibility loci. Schizophr. Bull. 40, 39–49. doi: 10.1093/schbul/sbt066
Luo, X. J., Li, M., Huang, L., Steinberg, S., Mattheisen, M., Liang, G., et al. (2014). Convergent lines of evidence support CAMKK2 as a schizophrenia susceptibility gene. Mol. Psychiatry 19, 774–783. doi: 10.1038/mp.2013.103
Ma, L., Wu, D. D., Ma, S. L., Tan, L., Chen, X., Tang, N. L., et al. (2014). Molecular evolution in the CREB1 signal pathway and a rare haplotype in CREB1 with genetic predisposition to schizophrenia. J. Psychiatr. Res. 57, 84–89. doi: 10.1016/j.jpsychires.2014.06.008
McCarthy, S. E., Gillis, J., Kramer, M., Lihm, J., Yoon, S., Berstein, Y., et al. (2014). De novo mutations in schizophrenia implicate chromatin remodeling and support a genetic overlap with autism and intellectual disability. Mol. Psychiatry 19, 652–658. doi: 10.1038/mp.2014.29
Mulle, J. G., Dodd, A. F., Mcgrath, J. A., Wolyniec, P. S., Mitchell, A. A., Shetty, A. C., et al. (2010). Microdeletions of 3q29 confer high risk for schizophrenia. Am. J. Hum. Genet. 87, 229–236. doi: 10.1016/j.ajhg.2010.07.013
Negri, G., Milani, D., Colapietro, P., Forzano, F., Della Monica, M., Rusconi, D., et al. (2015). Clinical and molecular characterization of Rubinstein-Taybi syndrome patients carrying distinct novel mutations of the EP300 gene. Clin. Genet. 87, 148–154. doi: 10.1111/cge.12348
Nestler, E. J. (2002). Common molecular and cellular substrates of addiction and memory. Neurobiol. Learn. Mem. 78, 637–647. doi: 10.1006/nlme.2002.4084
Otnaess, M. K., Djurovic, S., Rimol, L. M., Kulle, B., Kahler, A. K., Jonsson, E. G., et al. (2009). Evidence for a possible association of neurotrophin receptor (NTRK-3) gene polymorphisms with hippocampal function and schizophrenia. Neurobiol. Dis. 34, 518–524. doi: 10.1016/j.nbd.2009.03.011
Pavlov, I., Voikar, V., Kaksonen, M., Lauri, S. E., Hienola, A., Taira, T., et al. (2002). Role of heparin-binding growth-associated molecule (HB-GAM) in hippocampal LTP and spatial learning revealed by studies on overexpressing and knockout mice. Mol. Cell. Neurosci. 20, 330–342. doi: 10.1006/mcne.2002.1104
Pitcher, G. M., Kalia, L. V., Ng, D., Goodfellow, N. M., Yee, K. T., Lambe, E. K., et al. (2011). Schizophrenia susceptibility pathway neuregulin 1-ErbB4 suppresses Src upregulation of NMDA receptors. Nat. Med. 17, 470–478. doi: 10.1038/nm.2315
Prandini, P., Pasquali, A., Malerba, G., Marostica, A., Zusi, C., Xumerle, L., et al. (2012). The association of rs4307059 and rs35678 markers with autism spectrum disorders is replicated in Italian families. Psychiatr. Genet. 22, 177–181. doi: 10.1097/YPG.0b013e32835185c9
Razick, S., Magklaras, G., and Donaldson, I. M. (2008). iRefIndex: a consolidated protein interaction database with provenance. BMC Bioinformatics 9:405. doi: 10.1186/1471-2105-9-405
Reif, A., Schecklmann, M., Eirich, E., Jacob, C. P., Jarczok, T. A., Kittel-Schneider, S., et al. (2011). A functional promoter polymorphism of neuronal nitric oxide synthase moderates prefrontal functioning in schizophrenia. Int. J. Neuropsychopharmacol. 14, 887–897. doi: 10.1017/S1461145710001677
Ripke, S., O’dushlaine, C., Chambert, K., Moran, J. L., Kahler, A. K., Akterin, S., et al. (2013). Genome-wide association analysis identifies 13 new risk loci for schizophrenia. Nat. Genet. 45, 1150–1159. doi: 10.1038/ng.2742
Roelfsema, J. H., White, S. J., Ariyurek, Y., Bartholdi, D., Niedrist, D., Papadia, F., et al. (2005). Genetic heterogeneity in Rubinstein-Taybi syndrome: mutations in both the CBP and EP300 genes cause disease. Am. J. Hum. Genet. 76, 572–580. doi: 10.1086/429130
Roussos, P., Katsel, P., Davis, K. L., Bitsios, P., Giakoumaki, S. G., Jogia, J., et al. (2012). Molecular and genetic evidence for abnormalities in the nodes of Ranvier in schizophrenia. Arch. Gen. Psychiatry 69, 7–15. doi: 10.1001/archgenpsychiatry.2011.110
Rubinsztein, D. C. (2006). The roles of intracellular protein-degradation pathways in neurodegeneration. Nature 443, 780–786. doi: 10.1038/nature05291
Rubio, M. D., Wood, K., Haroutunian, V., and Meador-Woodruff, J. H. (2013). Dysfunction of the ubiquitin proteasome and ubiquitin-like systems in schizophrenia. Neuropsychopharmacology 38, 1910–1920. doi: 10.1038/npp.2013.84
Salavati, B., Rajji, T. K., Price, R., Sun, Y., Graff-Guerrero, A., and Daskalakis, Z. J. (2015). Imaging-based neurochemistry in schizophrenia: a systematic review and implications for dysfunctional long-term potentiation. Schizophr. Bull. 41, 44–56. doi: 10.1093/schbul/sbu132
Savanthrapadian, S., Wolff, A. R., Logan, B. J., Eckert, M. J., Bilkey, D. K., and Abraham, W. C. (2013). Enhanced hippocampal neuronal excitability and LTP persistence associated with reduced behavioral flexibility in the maternal immune activation model of schizophrenia. Hippocampus 23, 1395–1409. doi: 10.1002/hipo.22193
Schizophrenia Working Group of the Psychiatric Genomics Consortium (2014). Biological insights from 108 schizophrenia-associated genetic loci. Nature 511, 421–427. doi: 10.1038/nature13595
Shinkai, T., Ohmori, O., Hori, H., and Nakamura, J. (2002). Allelic association of the neuronal nitric oxide synthase (NOS1) gene with schizophrenia. Mol. Psychiatry 7, 560–563. doi: 10.1038/sj.mp.4001041
Sleiman, P., Wang, D., Glessner, J., Hadley, D., Gur, R. E., Cohen, N., et al. (2013). GWAS meta analysis identifies TSNARE1 as a novel Schizophrenia / Bipolar susceptibility locus. Sci. Rep. 3:3075. doi: 10.1038/srep03075
Szatkiewicz, J. P., O’dushlaine, C., Chen, G., Chambert, K., Moran, J. L., Neale, B. M., et al. (2014). Copy number variation in schizophrenia in Sweden. Mol. Psychiatry 19, 762–773. doi: 10.1038/mp.2014.40
Tillinghast, G. W., Partee, J., Albert, P., Kelley, J. M., Burtow, K. H., and Kelly, K. (2003). Analysis of genetic stability at the EP300 and CREBBP loci in a panel of cancer cell lines. Genes Chromosomes Cancer 37, 121–131. doi: 10.1002/gcc.10195
Vacic, V., Mccarthy, S., Malhotra, D., Murray, F., Chou, H. H., Peoples, A., et al. (2011). Duplications of the neuropeptide receptor gene VIPR2 confer significant risk for schizophrenia. Nature 471, 499–503. doi: 10.1038/nature09884
Vawter, M. P., Barrett, T., Cheadle, C., Sokolov, B. P., Wood, W. H. III, Donovan, D. M., et al. (2001). Application of cDNA microarrays to examine gene expression differences in schizophrenia. Brain Res. Bull. 55, 641–650. doi: 10.1016/S0361-9230(01)00522-6
Veltman, J. A., and Brunner, H. G. (2012). De novo mutations in human genetic disease. Nat. Rev. Genet. 13, 565–575. doi: 10.1038/nrg3241
Vrijenhoek, T., Buizer-Voskamp, J. E., Van Der Stelt, I., Strengman, E., Sabatti, C., Geurts van Kessel, A., et al. (2008). Recurrent CNVs disrupt three candidate genes in schizophrenia patients. Am. J. Hum. Genet. 83, 504–510. doi: 10.1016/j.ajhg.2008.09.011
Walsh, T., Mcclellan, J. M., Mccarthy, S. E., Addington, A. M., Pierce, S. B., Cooper, G. M., et al. (2008). Rare structural variants disrupt multiple genes in neurodevelopmental pathways in schizophrenia. Science 320, 539–543. doi: 10.1126/science.1155174
Weickert, C. S., Ligons, D. L., Romanczyk, T., Ungaro, G., Hyde, T. M., Herman, M. M., et al. (2005). Reductions in neurotrophin receptor mRNAs in the prefrontal cortex of patients with schizophrenia. Mol. Psychiatry 10, 637–650. doi: 10.1038/sj.mp.4001678
Xu, B., Ionita-Laza, I., Roos, J. L., Boone, B., Woodrick, S., Sun, Y., et al. (2012). De novo gene mutations highlight patterns of genetic and neural complexity in schizophrenia. Nat. Genet. 44, 1365–1369. doi: 10.1038/ng.2446
Xu, B., Roos, J. L., Levy, S., Van Rensburg, E. J., Gogos, J. A., and Karayiorgou, M. (2008). Strong association of de novo copy number mutations with sporadic schizophrenia. Nat. Genet. 40, 880–885. doi: 10.1038/ng.162
Yang, W., Liu, J., Zheng, F., Jia, M., Zhao, L., Lu, T., et al. (2013). The evidence for association of ATP2B2 polymorphisms with autism in Chinese Han population. PLoS One 8:e61021. doi: 10.1371/journal.pone.0061021
Zhang, Z., Chen, X., Yu, P., Zhang, Q., Sun, X., Gu, H., et al. (2014). Evidence for the contribution of NOS1 gene polymorphism (rs3782206) to prefrontal function in schizophrenia patients and healthy controls. Neuropsychopharmacology 40, 1383–1394. doi: 10.1038/npp.2014.323
Keywords: schizophrenia, GWAS, PPI Network, copy number variation (CNV), gene modules
Citation: Chang X, Lima LdA, Liu Y, Li J, Li Q, Sleiman PMA and Hakonarson H (2018) Common and Rare Genetic Risk Factors Converge in Protein Interaction Networks Underlying Schizophrenia. Front. Genet. 9:434. doi: 10.3389/fgene.2018.00434
Received: 05 August 2018; Accepted: 12 September 2018;
Published: 28 September 2018.
Edited by:
Tao Huang, Shanghai Institutes for Biological Sciences (CAS), ChinaReviewed by:
Wei Wang, Rockefeller University, United StatesCopyright © 2018 Chang, Lima, Liu, Li, Li, Sleiman and Hakonarson. This is an open-access article distributed under the terms of the Creative Commons Attribution License (CC BY). The use, distribution or reproduction in other forums is permitted, provided the original author(s) and the copyright owner(s) are credited and that the original publication in this journal is cited, in accordance with accepted academic practice. No use, distribution or reproduction is permitted which does not comply with these terms.
*Correspondence: Xiao Chang, Y2hhbmd4QGVtYWlsLmNob3AuZWR1 Hakon Hakonarson, aGFrb25hcnNvbkBlbWFpbC5jaG9wLmVkdQ==
Disclaimer: All claims expressed in this article are solely those of the authors and do not necessarily represent those of their affiliated organizations, or those of the publisher, the editors and the reviewers. Any product that may be evaluated in this article or claim that may be made by its manufacturer is not guaranteed or endorsed by the publisher.
Research integrity at Frontiers
Learn more about the work of our research integrity team to safeguard the quality of each article we publish.