- 1Institut de Recherche Pour le Développement, UMR DIADE, Montpellier, France
- 2Institut de Recherche Pour le Développement, Niamey, Niger
- 3University Montpellier II, Place Eugène Bataillon, Montpellier, France
- 4University Abdou Moumouni of Niamey, Niamey, Niger
- 5Institut National de Recherche Agronomique, INRA, UMR AGAP, Montpellier, France
- 6Institut de Recherche Pour le Développement, IRD, UMR LTHE, Grenoble, France
Ongoing global climate changes imply new challenges for agriculture. Whether plants and crops can adapt to such rapid changes is still a widely debated question. We previously showed adaptation in the form of earlier flowering in pearl millet at the scale of a whole country over three decades. However, this analysis did not deal with variability of year to year selection. To understand and possibly manage plant and crop adaptation, we need more knowledge of how selection acts in situ. Is selection gradual, abrupt, and does it vary in space and over time? In the present study, we tracked the evolution of allele frequency in two genes associated with pearl millet phenotypic variation in situ. We sampled 17 populations of cultivated pearl millet over a period of 2 years. We tracked changes in allele frequencies in these populations by genotyping more than seven thousand individuals. We demonstrate that several allele frequencies changes are compatible with selection, by correcting allele frequency changes associated with genetic drift. We found marked variation in allele frequencies from year to year, suggesting a variable selection effect in space and over time. We estimated the strength of selection associated with variations in allele frequency. Our results suggest that the polymorphism maintained at the genes we studied is partially explained by the spatial and temporal variability of selection. In response to environmental changes, traditional pearl millet varieties could rapidly adapt thanks to this available functional variability.
Introduction
The general test of the theory of evolution led to the conclusion that most polymorphisms are neutral and are transiently polymorphic due to the effect of mutation and drift (Kimura, 1983). However, it is also postulated that some polymorphism may be maintained by variable selection in space and over time (Hedrick, 1986, 2006). The number of documented genes in which polymorphism has been shown to be maintained by this mechanism is limited (Hedrick, 2006). However to date, very few studies have investigated this question in situ (O’Hara, 2005; Pemberton, 2010; Mojica et al., 2012; Gratten et al., 2012).
In situ studies of selection are rather difficult because variability between years could affect both the strength of selection and the heritability of traits. In sheep, such variability has led to low heritability of birth weight when selection is strong and stronger heritability when selection is weak (Wilson et al., 2006). To assess whether their evolution is simply explained by the effect of drift or whether the effect of selection also plays a role, rather than relying on morphological variation, one can rely directly on the alleles associated with variations in morphological characters.
Studies on pearl millet identified functional polymorphism associated with variations in morphology and as well as in flowering time (Saïdou et al., 2009; Mariac et al., 2011). Some of this polymorphism was associated with the evolution to earlier flowering of traditional varieties over a period of 27 years at a whole country scale (Vigouroux et al., 2011). Pearl millet is an out-breeding crop and traditional varieties sown by farmers from year to year are subjected to selection both imposed by humans and by the environment. The aim of this study was to investigate if selection occurred and varied in the field at the level of varieties. We focused primarily on selection imposed by the environment on varieties and not on that imposed by humans. We consequently did not consider seed selection from one year to the next (Allinne et al., 2008), nor the impact of early thinning, which is known to have a potential selective effect (Couturon et al., 2003). Both of these effects are directly due to human selection. In this paper, we focus on direct environmental effect, in situ. For this reason, we sampled seedlings at a later stage along with seeds at maturity to assess changes in overall allele frequency between these two stages.
We analyzed selection over a period of 2 years in situ by genotyping more than seven thousand individual plants. We examined the evolution of early and late allele frequency of the two genes in 17 populations over two growing seasons to assess whether or not their evolution was compatible with neutral evolution. As we focused on selection imposed by the environment, we studied the evolution of allele frequency at the seedling stage and at harvest.
Materials and Methods
Field Sampling and Plant Material
Sampling was conducted during two rainy seasons in 2008 and 2009. Seventeen different fields were chosen in an area of 100 km × 100 km around Niamey in Niger (Figure 1). The fields were chosen because they were homogenous with respect to soil, their size (mean = 5.7 ha SE = 3.4) and the vegetation. No specific permission was required for these experiments, and the experiments did not involve endangered or protected species.
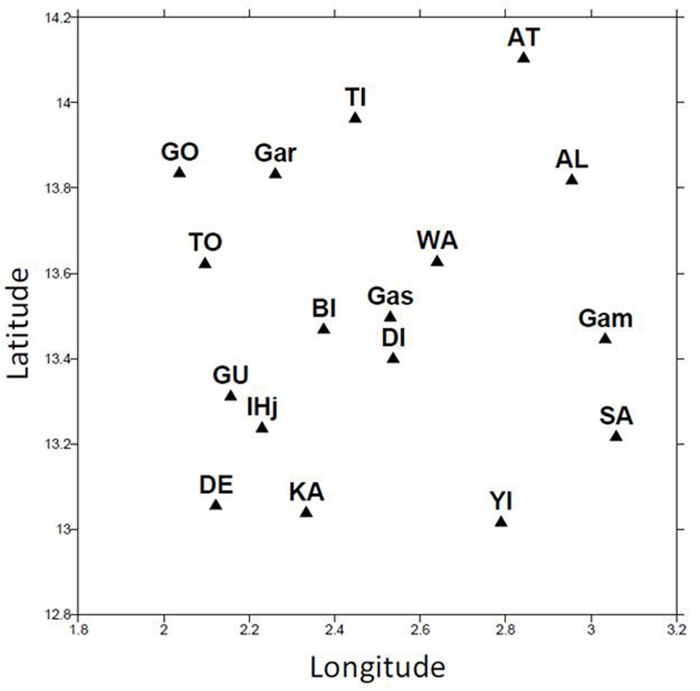
FIGURE 1. Location of sampling sites. A total of 17 different sampling sites were used in the study. These sites were located near Niamey in Niger between longitude 2 and 3.2 and between latitude 13 and 14.2.
At each site in each year, allele frequencies were estimated at the seedling and seed stage.
One hundred and twenty plants were sampled by choosing the eight closest plants around 15 plots randomly located in a 7,500 m2 area chosen in the center of fields.
Seedlings were sampled at the late vegetative stage, in July. Leaf fragments (15 cm2) were collected at 4°C and stored at -20°C until DNA extraction. Seeds were sampled just before harvest in October, spikes were randomly picked following exactly the same protocol. Spikes from the entire sample were mixed and threshed to form a bulk sample of seeds from which a subset was grown in the laboratory for DNA extraction. We did not ask the farmer to collect the different spikes, but sampled them ourselves with a trained technician.
The average yield of each farmer’s field was evaluated for each year by counting the number of bundles of spikes harvested. Rainfall data (Supplementary Figure S1) were recorded by pluviographs located close to each field (mean distance from the field = 406 m, SE = 551).
DNA Extraction and Genotyping
DNA was extracted using a modified high-throughput method (Xin et al., 2003; Saïdou et al., 2014). Briefly, 30 mm2 of leaves were transferred in a 96-well plate, only very slight crushed with a small plastic stick, and mixed with 50 μl extraction buffer (100 mM NaOH, 2% Tween 20, 10% chelex, pH 10). The plate was then heated at 95°C for 10 min and immediately cooled to 4°C. Then 50 μl of neutralization buffer (100 mM tris, 2 mM EDTA pH 2) was added, mixed and left overnight at 4°C. For PCR amplification, 2 μl of the supernatant was used.
We genotyped either an SNP at the PgPHYC gene using a cleaved amplified polymorphic sequence fragment of the gene (see Saïdou et al., 2009 for primer sequences, PCR and digestion conditions and genotype scoring) or a 3 bp indel using a new set of primers for direct PCR amplification and genotyping on an LI-COR sequencer (PgPHYC-F5′GCTCTGTTGCGTCACTTG3′; PgPHYC-R 5′CTGCTGATCACTCCCAGTAT3′). Two alleles were observed: one at 80 bp and one at 83 bp. According to previous observations, the restriction of size variation polymorphisms is perfectly linked and associated with phenotypic variation (Saïdou et al., 2009, 2014). We also genotyped a 24 bp indel in the PgMADS11 gene (Mariac et al., 2011) using a simple PCR reaction (PgMADS11 Forward 5′CCAAAACCAAACCCTAGCAA3′, PgMADS11 Reverse 5′GTTCAAGAAGGCGGAGGAG 3′). Two alleles were observed, one of 363 bp and one of 387 bp.
The forward primers of PgPHYC and PgMADS11 were synthesized with an M13 forward primer sequence at the 5′-end (5′-ACGACGTTGTAAAACGAC-3′). The PCR of the two genes were performed simultaneously including an M13 labeled primer. The PCR primer concentrations were adjusted to obtain amplification of similar intensity for the two genes (the final concentration of primers in PCR reaction was 0.13 μM of PgMADS11-F, 0.36 μM of PgMADS11-R, 0.2 μM of PHYC- F, 0.2 μM PHYC-R, and 0.1 μM of IRDye-700 labeled M13 Primer). The two genes were scored using a LI-COR system using the migration conditions previously described in Allinne et al. (2008). Allele scoring was checked manually by two different people.
A set of nine microsatellite markers was chosen according to their high polymorphism levels and their distribution among the seven linkage groups of pearl millet: PSMP2085, PSMP2202, PSMP2218, PSMP2220, PSMP2237, PSMP2263, PSMP2270, PSMP2271, and PSMP2273 (Mariac et al., 2006). Primers and PCR conditions are described in (Mariac et al., 2006). Microsatellites were genotyped using a LI-COR sequencer (see Allinne et al., 2008 for details). We used Flexibin (Amos et al., 2007) to differentiate alleles.
Statistical Analysis and FST Based Test of Selection
For each population, we calculated the genotype and allele frequency of each allele for PgPHYC and PgMADS11. Differences in allele frequency were assessed using a G-test (Sokal and Rohlf, 1981). We calculated the changes in allele frequencies: a difference in a positive value is associated with an increase in the earlier flowering allele, while a negative value is associated with a decrease in the earlier flowering allele.
If selection is acting on a particular functional allele, we expect its allele to show stronger allele frequency changes than a neutral allele. To assess the strength of the change relative to a neutral allele, we need to take into account both the effect of drift and sampling. To do so, we built two FST distributions describing a neutral expected distribution using a previously described approach (Vigouroux et al., 2011). The first distribution of the FST value was built based on the FST value calculated for each allele of the 173 alleles of the nine microsatellite loci. This distribution is hereafter referred to as empirical distribution. We built a second model based on FST distribution (hereafter referred to as simulated FST distribution) taking the sampling effect and drift into account. Drift is the direct product of the effective size of the population; consequently, we needed to estimate this parameter. Differences in the allele frequency of microsatellite loci between the seedling sample and the seed sample were used to estimate this effective size using the pseudo-likelihood method (Wang, 2001, 2005). This method makes it possible to derive an expected effective size (Ne) as well as a confidence interval. To be effective, the sampling size needs to be of the same order of magnitude as the unknown effective size. If the sampling size is much lower than the effective size of the population, the method only makes it possible to define the lower bound of the 95% confidence interval, and the estimated effective size or higher bound will be very inaccurate. However, this lower bound leads to over correction for the drift effect and is consequently sufficient to build a conservative distribution.
For the simulation of drift, we used a standard Wright–Fisher model; and considered a single nucleotide polymorphism (SNP) with a frequency p drawn from a uniform distribution [0,1]. We simulated variation in allele frequency by simulating the allele frequency after drift associated with an effective size Ne by drawing a binomial law. We then simulated a sample of size n (less than the effective size Ne) from the initial population and the next generation population. From these two samples, we then derived the estimated initial allele frequency, and the estimated final allele frequency. Consequently, this simulation took into account the effect of drift (shaped by Ne) and the effect of sampling (shaped by n). Using the two samples, we were able to easily estimate the differentiation using FST (Weir, 1996). This simulation was performed using R1 and we performed 100,000 simulations. The FST distribution was then treated as a null distribution against which allele variation on the two genes was tested. We calculated the rank of the differentiation observed for PgMADS11 or PgPHYC in the empirical or the simulation based FST distribution. This rank, divided by the number of simulations, was used as a p-value (Vigouroux et al., 2011). The effective size was estimated for two populations in 2008. For these two populations, the lower bound of the 95% confidence interval for the effective size was used to derive the simulation based FST distribution. For the other population, we used the smaller lower bound of the two populations to simulate the FST distribution for the other population. We estimated the effective size of two populations in 2009 and used the same procedure to derive FST distribution.
Microsatellites have a high mutation rate (Vigouroux et al., 2002), and in this particular case, FST is not independent of the mutation rate (Vigouroux et al., 2005). But for the generation time considered, the microsatellite mutation rate does not affect FST estimation (see Vigouroux et al., 2011 for a simulation study).
Estimation of Selection
The previous analyses proved change in allele frequency beyond drift. We were consequently also able to estimate a selection coefficient using an approximate Bayesian computation approach (Csilléry et al., 2010). In this particular case, we considered that a particular observed allele frequency change is shaped by drift (Ne), sampling (n), and selection (s). We used changes in microsatellite allele frequency to estimate Ne and n is perfectly known, consequently we were able to estimates.
We simulated three genotypes, AA, Aa, and aa, with a frequency q for a. We calculated the genotype frequency associated with a coefficient of selection s and a dominance h: AA: (1-q)2/(2hsq(q-1)+1-sq2); Aa: 2pq(1-hs)/ (2hsq(q-1)+1-sq2); aa q2(1-s)/(2hsq(q-1)+1-sq2). We simulated the effect of drift based on a multinomial distribution with Ne individuals. We then simulated the effect of sampling with a multinomial distribution with Ns individuals (Ns < Ne). We used a fixed value for Ne, choosing either the average previously reported Ne value or the lower bound of the Ne distribution. ABC approaches are simulation based approaches used to obtain a posterior distribution of a parameter, in this case, s. The prior distribution of s was chosen as a uniform distribution, (0,1). The method was only applied when the FST approach classified the loci as outliers, i.e., selected. So we only considered loci with s≠0. Based on observed allele frequency q, the genotypes AA, Aa, aa were simulated for a given value of s, Ne, Ns, h. The simulated genotype frequencies were compared to the observed genotype frequency using a khi-square test. The p value of the test was used as the selection criterion. All simulations with a p-value of 0.5 or higher were kept. The posterior distribution of the s distribution was based on the simulations that were kept. The median value and the 95% interval were calculated based on this distribution. For validation, the retrieved median value of s was then used to simulate the average frequency of the genotype, and the difference between the simulated frequency and the observed frequency were tested using a G-test.
We assessed the ability of the ABC approach to estimate s. We ran a simulation in which we simulated a selection effect (s), a drift effect (Ne), and a sampling size (n). We then estimated a genotype frequency knowing s. We then used the ABC approach to estimate the known value of s. For this simulation, we simulated different values of s: 0.02, 0.05, 0.1, 0.2, 0.3, 0.4, 0.5, 0.7, 0.9. The allele frequency q was set at 0.5. The effective size Ne was set at 100,000 or 300. The sampling size varied from n = 100, 200, Ne. The dominance coefficient h was set at 0 or 1. The median value of s estimated using the ABC approach was then compared to the true exact value.
Field Trial
A field trial was conducted in 2009 using the seeds collected in 2008. The experiment was performed at the International Center of Research for the Semi-Arid Tropics (ICRISAT) station, Sadore, Niger with the agreement of this institution. Two repetitions (plots) were performed at each planting date; and two different planting dates were used: June 15 and July 15, 2009. Each sample in each field trial contained 20 plants, i.e., a total of 340 randomly distributed plants. We recorded flowering time (time from sowing to female flowering) and size of the spike at maturity of each individual plant. An overall measure for each sample (including all 20 individuals) was the weight of the spike, the total seed weight, total dry weight, and 100 seed weight. We performed an analysis of variance using the aov function in R (R Development Core Team, 2008) considering both the effect of samples, date of planting, and replicates. For the analysis of correlation, we used cor.test in R (R Development Core Team, 2008).
Results
Changes in Allele Frequency
We retrieved genotypes on 1,659 seedlings for PgPHYC and 1,455 seedlings for PgMADS11 at the 17 sampling sites in 2008. At the seed stage, a total of 1,968 genotypes were obtained for PgPHYC and 1,512 for PgMADS11. In 2009, 1,904 individual genotypes were obtained for PgPHYC and 1,863 for PgMADS. Finally, at the seed stage, 1,539 PgPHYC genotypes and 1,439 for PgMADS phenotype were obtained. A grand total of 7,070 individuals were genotyped for PgPHYC, and 6,310 individuals were genotyped for PgMADS11. An average 98.4 genotypes were finally obtained for each sampling site, at each stage for each gene.
Allele frequencies between seedlings and seeds (Figure 2) differed significantly in the PgPHYC gene in seven populations in 2008 (DE +0.088, p ≤ 0.015; DI +0.093, p ≤ 0.016; Gak +0.078, p ≤ 0.029; Ihj -0.061, p ≤ 0.036; SA +0.072, p ≤ 0.044; TO +0.094, p ≤ 0.031; WA +0.085, p ≤ 0.031). In 2009, three populations differed significantly in allele frequency in PgPHYC (AT +0.085, p ≤ 0.014; DI +0.068, p ≤ 0.029; Gak -0.075, p ≤ 0.044). In PgMADS, three populations were significantly different in 2008 (AL +0.123, p ≤ 0.007; TI +0.129, p ≤ 0.004; TO -0.08, p ≤ 0.047,), and two populations were significantly different in 2009 (BI -0.0904, p ≤ 0.038; WA +0.086, p < 0.046).
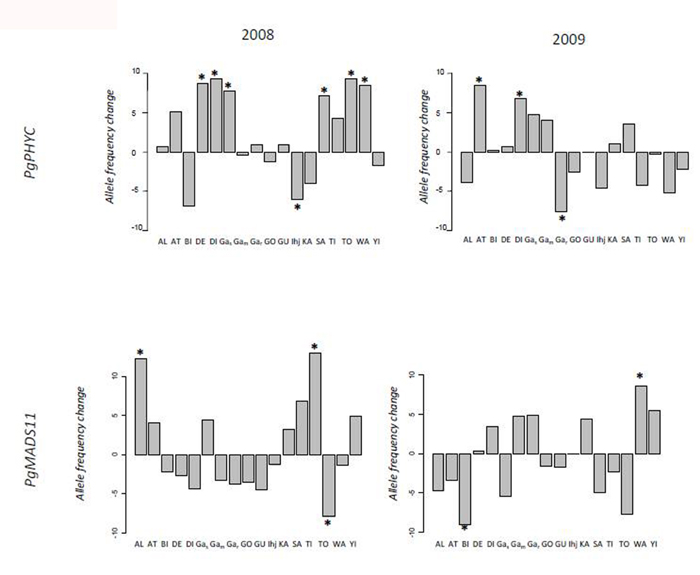
FIGURE 2. Differences in allele frequency between the late seedling stage and seed stage of two flowering time genes. The difference in allele frequency between seedlings and seeds was estimated at 17 sampling sites. Differences in the two flowering time genes PgPHYC and PgMADS11 were assessed during two seasons, 2008 and 2009. A different positive value is associated with an increase in the earlier flowering allele, a negative value with a decrease in the earlier flowering allele. The significance of the difference in frequency was assessed using a G-test (*P < 0.05). The 17 different sampling points were Alkama (AL), Ataloga (AT), Billingol (BI), DeberiGati (DE), Diribangou (DI), Gassan Kournie (Gas), Gamonzon (Gam), Gardama Kouara (Gar), Gorougoussa (GO), Guilahle (GU), IHjachere (IHj), Kare (KA), Sandileye (SA), Tiloa Kaina (TI), Tondibia Gorou (TO), Wankama (WA), Yillade (YI).
The probability of obtaining two or more significant tests out of 17 at a 5% level is 5%, and the probability is 1% with three or more significant tests, and 6.3 10-7 with seven or more significant tests. These results suggest that the significant difference observed in 2008 and 2009 in PgPHYC and in 2008 in PgMADS11 is not simply associated with the number of populations investigated.
However, these different allele frequencies would be expected as the effect of drift if the effective population size is small. We consequently estimated the effective population size (Figure 3A; Supplementary Figure S2) using microsatellite datasets for two populations in 2009 and 2008: Diribangou and Tondibia Gorou. In 2008, we estimated the effective size at 296 (95% lower bound: 130) for the Tondibia Gorou population, and 378 (95% lower bound: 160) for the Diribangou population. In 2009, using the same sampling design, we were able to estimate the lower bound of the 95% CI with 216 for Tondibia Gorou and 239 for Diribangou with confidence. The estimated effective size is rather imprecise but was 1,115 for Tondibia Gorou and 8,986 for Diribangou. In 2009, our sample (~100 plants) was much smaller than the lower bound, meaning that, with our present design, the approach based on the change in allele frequency was not powerful enough to assess the average effective size. A bigger sample would be needed to accurately estimate the average effective size. However, these results are sufficiently precise to evaluate the effect of drift on the difference in allele frequency between seedling and seeds. We based our simulation of drift on the lower bound of the confidence interval, leading to conservative tests of significance. The test applied to the population from Tondibia Gorou in 2008 (Figure 3B) showed that the observed FST in PgPHYC was extreme in the distribution of the empirical or simulated FST distribution (PgPHYC FST = 0.0201, p < 0.05). No difference was observed in PgMADS11 (Figures 2 and 3). The same results were obtained at the Diribangou site (PgPHYC FST = 0.0201, p < 0.05). Using the 95% lower bound interval of the effective size estimated in 2008 and 2009, we built a FST simulated distribution for the other sites. These analyses confirmed the significant difference in allele frequency at 13 of the 15 sites with a significant G-test. The two non-significant FST-tests were observed in 2008 in the IHjachere (IHj) sample for PgPHYC and in the Tondibia Gorou sample for PgMADS11.
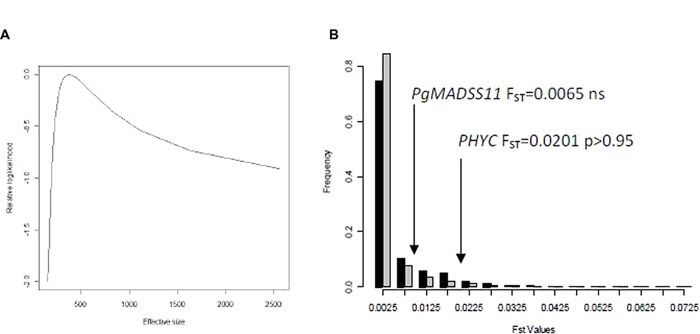
FIGURE 3. Estimation of effective size, observed and expected FST values. The effective size from seedling to seed was estimated using Wang methods [9] for the Diribangou samples (A). In 2008, nine microsatellites markers were genotyped on 93 seedlings and 144 seeds at this site. The graph shows changes in the relative log likelihood as a function of effective size, the highest likelihood being set to 0. The average estimation was 378, and the lowest 95% interval was 160. The lower interval value was used to simulate a FST distribution (B). The FST distribution based on empirical FST (black) or simulated FST (gray) are very similar. The significance of the FST p-value was assessed using the simulated FST distribution for each gene.
Estimation of Selection
We developed an ABC approach to estimate the selection coefficient s. This method was effective (Supplementary Figure S3) for a high selection coefficient (s > 0.3) for the size of the sample used in this study (Ns~100) and the effective size observed (Ne~300). A bigger sample and higher effective size make it possible to estimate a lower selection coefficient. We then applied this approach at the two original sites (Tondibia Gorou and Diribangou). The selection coefficient estimated for h = 0 at the Tondibia Gorou site was 0.49 (95%CI 0.06–0.75). The selection coefficient estimated at the Diribangou site was 0.44 (95%CI 0.06–0.69). These estimated coefficients were then used to calculate the expected frequency of the genotype and to compare these frequencies with the observed class of genotypes. The selection coefficients were in agreement with the frequency observed at Tondibia Gorou (χ2 = 0.30, dof = 2, p = 0.85) and at Diribangou (χ2 = 1.69, dof = 2, p = 0.43).
Morphological Analyses
Field trail analyses revealed a significant difference (Table 1) between varieties in flowering time (June 15, F16,600 = 13.9, p < 0.001; July 15 F16,595 = 9.3, p < 0.001) and in spike length (June 15, F16,601 = 7.3, p < 0.001; July 15 F16,595 = 9.0, p < 0.001). As only aggregated data were available for yield, biomass and 100-seed weight, we calculated the correlation between allele frequency and each of these morphological characters as well as for flowering time and spike length. Significant correlations were found for yield with PgPHYC allele frequency at both sowing dates (June 15, R = -0.69, p < 0.006; July 15, R = -0.69, p < 0.02), but only for the earlier seedling for PgMADS11 (June 15, R = -0.60, p < 0.012; July 15, R = -0.34, p = 0.19). The correlation with biomass tended to be only significant for PgPHYC and at the early planting date (June 15, R = -0.51, p < 0.05). A significant correlation for PgMADSS11 and flowering time was only found at the late planting date (R = -0.48, p < 0.05). The other correlations were not significant.
Finally, in 2008 and 2009, we recorded the yield of all the populations in the field. We used this estimation of in situ yield to check whether or not the variation in allele frequency was linked with yield. We only found (Figure 4) a significant negative correlation for PgPHYC in 2008 (R = -0.76, p < 0.001). No significant correlation was found for 2009 or PgMADS11.
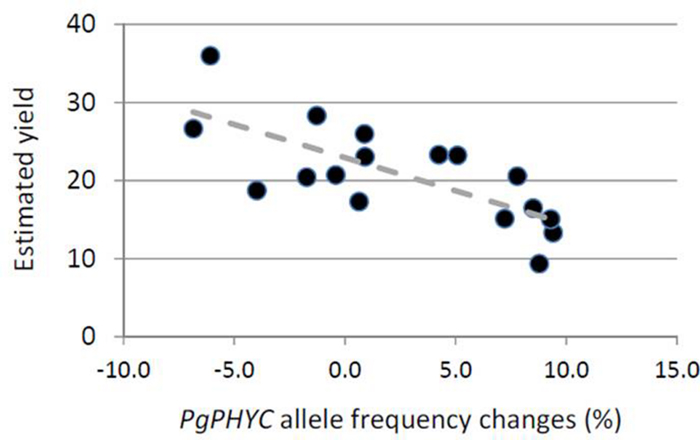
FIGURE 4. Relationship between changes in PgPHYC allele frequency and estimated yield. For each sampling site, we plotted the change in the PgPHYC allele frequency and estimated yield. Yield was estimated in situ based on the number of bundles of spikes per hectare. The relationship is significant (R = -0.76, p < 0.001).
Discussion
The two genes PgMADS11 and PgPHYC have previously been shown to have an effect on several traits including flowering time and spike length (Saïdou et al., 2009; Mariac et al., 2011; Vigouroux et al., 2011). A recent study found also an association between PgPHYC and panicle harvest index (Sehgal et al., 2015). For both genes, we detected a significant effect on yield in the field trials. However, one of the effects was not significant for PgMADS11 at the later planting date (July 15). These correlations (here and in Sehgal et al., 2015) suggest that variations in these genes may have a direct impact on fitness (yield in an agricultural setting). However, this effect could be influenced by other parameters, for example, planting date. In this particular case, depending on the emergence of the plant, polymorphism could be associated with fitness or not.
In our study, effective size strongly varied between years. This result is not surprising, since in the Sahel, crop failure is frequently observed and climate variability is very high. Total crop failure will actually lead to an effective size of zero, and the maximum value one could expect is the total number of individuals randomly breeding in the field. Taking the effect of drift into account, our study suggests a variation in the selection of genetic markers associated with quantitative traits. Our results suggest that this variation varied from positive selection in 1 year to neutral or negative selection in the other. If variation in allele frequency is associated with selection, then one might expect a correlation between what will measure the strength of selection (difference in allele frequency between seedlings and seeds) and the overall fitness of the population. We actually found a significant correlation between variation in PgPHYC allele frequency and yield in the field in 2008 (Figure 4). Such a correlation would be detected if and only if a large number of sampling sites had significant changes in allele frequency, i.e., is associated with selective events. We actually only observed such a situation for PgPHYC in 2008. In the other year for PgMADS11, few sampling sites showed significant changes in allele frequency. It should be noted that majority of the field studies/gene studies over 2 years (53/68) showed no significant change in PgPHYC and PgMADS11 allele frequencies. And using the FST based test of selection, 81% (55/68) of the field studies/gene pairs were considered to have changes in allele frequencies that did not differ from a neutral pattern.
In our study, the point estimated selection coefficient is high (~0.44–0.49). However, since the confidence interval is relatively high, our results are compatible with a lower selection coefficient. A high coefficient of selection was also observed in situ in a field study on mice (Linnen et al., 2013). Such a high selection coefficient could rapidly lead to the fixation of an allele in a given population. However, several factors certainly helped maintain polymorphism in the present study: the variability of selection (in space and over time), the effect of gene flow (Allinne et al., 2008), and, in our context, human counter selection. One of the first variability factors to be considered is the variability of the environmental conditions. The Sahelian climate is known to vary considerably from year to year, as actually observed in our field study (Supplementary Figure S1). Moreover, as previously discussed, there is a complex interaction between allele frequency, environment and fitness. Again this interaction could play a significant role in maintaining polymorphism: polymorphisms with such interaction do not always appear to be associated with fitness, and in our study mostly appeared to be “neutral”. Another factor that certainly plays a role is human selection. We need to underline that our study was performed during a short period of time during which humans cannot not have a direct effect on changes in allele frequency, i.e., between the late seedling stage and seed maturity. In a previous study, we demonstrated that PgPHYC is associated with slightly shorter spikes (Saïdou et al., 2009, 2014). Human selection after harvest might favor longer spikes, which are associated with higher yields, and with what farmers consider to be the normal morphology of their varieties. It that case, human interaction might also counter environmentally imposed selection. Moreover, when crop failure is high, farmers import new seeds sometimes from a distance (Allinne et al., 2008), which might also provide an opportunity for environmental selection. To decipher the role of these different types of selection, future experiments should include selection imposed by the environment and that imposed by humans. Here we only focused on selection imposed by the environment.
In this study, one factor that was possibly not controlled was pollen gene flow from neighboring fields. Few pollen gene flow studies have been conducted in pearl millet but data are available on maize, a wind pollinated crop that closely resembles pearl millet. Field studies on maize suggest that gene flow is as low as 1% at a distance of 60 m (Bateman, 1947). Based on this figure, if we considered the biggest difference between populations for PgPHYC frequency (24%), a gene flow of 1% would lead to a 0.24% change in allele frequency. It is thus unlikely that pollen gene flow between neighboring fields would change PgPHYC or PgMADS11 allele frequency to the level observed in this study.
The study of selection in an ecological context requires the fulfillment of several conditions. Selection can only be assessed if it is high enough to outperform the effect of drift. So a large population is needed. The size of the sample needs to be adjusted so that the effect of selection can be identified. In this study, we used a sample of around 100 plants, i.e., 200 chromosomes. A bigger sample would be needed to detect small changes in allele frequency and a lower selection coefficient. It also certainly means that the detection of selection in field conditions will be particularly difficult unless either the selection coefficient is very high and/or the population size (and hence the sample) is sufficiently large. This requirement may explain why observations of selection maintained polymorphism are so rare.
Conclusion
The results of study suggest variability of selection on alleles associated with phenotypic variation in situ. Such selection could certainly lead to the ultimate fixation of an allele but the variability of the selection in space and over time could certainly maintain such functional variation. Selection for these genes could be rapid in response to environmental changes. In addition, we only considered environmentally imposed selection and in the present case, human selection may also have had a major impact on the allele frequency of the two genes. A better understanding of the dynamic constraints of such a system needs to incorporate environmental as well as human selection pressures.
Author Contributions
CM, J-LP, LD, IO, and YV designed the research. CM, IO, HJ, KX, JR, GB performed the research, contributed reagent and feedback, CM and YV performed the statistical analysis, IO and YV developed the ABC model for s estimation, CM, IO, and YV wrote the paper.
Funding
This project was funded by the Institut de Recherche pour le developpement core grant (Action incitative IRD). YV was supported by a grant of the Agence Nationale de la Recherche (ANR-07-JCJC-0116-01) and the Agropolis Fondation (ARCAD project). IO was partly funded by a grant from the ARCAD project, by a Ph.D. grant from the French Embassy in Niger and an IRD Ph.D. grant (ARTS). The funders had no role in study design, data collection, and analysis, decision to publish, or preparation of the manuscript.
Conflict of Interest Statement
The authors declare that the research was conducted in the absence of any commercial or financial relationships that could be construed as a potential conflict of interest.
Acknowledgments
We thank D. Moussa and M. Tidjani for their help during the field studies and laboratory experiments. We thank the farmers in Niger who participated in this study.
Supplementary Material
The Supplementary Material for this article can be found online at: http://journal.frontiersin.org/article/10.3389/fgene.2016.00130
Footnotes
References
Allinne, C., Mariac, C., Vigouroux, Y., Bezançon, G., Couturon, E., Moussa, D., et al. (2008). Role of seed flow on the pattern and dynamics of pearl millet (Pennisetum glaucum [L.] R. Br.) genetic diversity assessed by AFLP markers: a study in south-western Niger. Genetica 133, 167–178. doi: 10.1007/s10709-007-9197-7
Amos, W., Hoffman, J. I., Frodsham, A., Zhang, L., Best, S., and Hill, A. V. S. (2007). Automated binning of microsatellite alleles: problems and solutions Mol. Ecol. Notes 7, 10–14. doi: 10.1111/j.1471-8286.2006.01560.x
Bateman, A. J. (1947). Contamination in seed crops. II. wind pollination. Heredity 1, 235–246. doi: 10.1038/hdy.1947.15
Couturon, E., Mariac, C., Bezançon, G., Lauga, J., and Renno, J.-F. (2003). Impact of natural and human selection on the frequency of the F1 hybrid between cultivated and wild pearl millet (Pennisetum glaucum (L.) R. Br.). Euphytica 133, 329–337. doi: 10.1023/A:1025773313096
Csilléry, K., Blum, M. G., Gaggiotti, O. E., and François, O. (2010). Approximate bayesian computation (ABC) in practice. Trends Ecol. Evol. 25, 410–418. doi: 10.1016/j.tree.2010.04.001
Gratten, J., Pilkington, J. G., Brown, E. A., Clutton-Brock, T. H., Pemberton, J. M., and Slate, J. (2012). Selection and microevolution of coat pattern are cryptic in a wild population of sheep. Mol. Ecol. 21, 2977–2990. doi: 10.1111/j.1365-294X.2012.05536.x
Hedrick, P. W. (1986). Genetic polymorphism in heterogeneous environments: a decade later. Annu. Rev. Ecol. Syst. 17, 535–566. doi: 10.1146/annurev.es.17.110186.002535
Hedrick, P. W. (2006). Genetic polymorphism in heterogeneous environments: the age of genomics. Annu. Rev. Ecol. Evol. Syst. 37, 67–93. doi: 10.1146/annurev.ecolsys.37.091305.110132
Kimura, M. (1983). The Neutral Theory of Molecular Evolution. Cambridge: Cambridge University Press, 367. doi: 10.1017/CBO9780511623486
Linnen, C. R., Poh, Y. P., Peterson, B. K., Barrett, R. D., Larson, J. G., Jensen, J. D., et al. (2013). Adaptive evolution of multiple traits through multiple mutations at a single gene. Science 339, 1312–1326. doi: 10.1126/science.1233213
Mariac, C., Jehin, L., Saïdou, A. A., Thuillet, A. C., Couderc, M., Sire, P., et al. (2011). Genetic basis of pearl millet adaptation along an environmental gradient investigated by a combination of genome scan and association mapping. Mol. Ecol. 20, 80–91. doi: 10.1111/j.1365-294X.2010.04893.x
Mariac, C., Luong, V., Kapran, I., Mamadou, A., Sagnard, F., Deu, M., et al. (2006). Diversity of wild and cultivated pearl millet accessions (Pennisetum glaucum [L.] R. Br.) in Niger assessed by microsatellite markers. Theor. Appl. Genet. 114, 49–58. doi: 10.1007/s00122-006-0409-9
Mojica, J. P., Lee, Y. W., Willis, J. H., and Kelly, J. K. (2012). Spatially and temporally varying selection on intrapopulation quantitative trait loci for a life history trade-off in Mimulus guttatus. Mol. Ecol. 21, 3718–3728. doi: 10.1111/j.1365-294X.2012.05662.x
O’Hara, R. B. (2005). Comparing the effects of genetic drift and fluctuating selection on genotype frequency changes in the scarlet tiger moth. Proc. Biol. Sci. 272, 211–217. doi: 10.1098/rspb.2004.2929
Pemberton, J. M. (2010). Evolution of quantitative traits in the wild: mind the ecology. Philos. Trans. R. Soc. Lond. B Biol. Sci. 365, 2431–2438. doi: 10.1098/rstb.2010.0108
R Development Core Team (2008). R: A Language and Environment for Statistical Computing. Vienna: R Foundation for Statistical Computing.
Saïdou, A. A., Clotault, J., Couderc, M., Mariac, C., Devos, K. M., Thuillet, A. C., et al. (2014). Association mapping, patterns of linkage disequilibrium and selection in the vicinity of the PHYTOCHROME C gene in pearl millet. Theor. Appl. Genet. 127, 19–32. doi: 10.1007/s00122-013-2197-3
Saïdou, A. A., Mariac, C., Luong, V., Pham, J. L., Bezançon, G., and Vigouroux, Y. (2009). Association studies identify natural variation at PHYC linked to flowering time and morphological variation in Pennisetum glaucum [(L.) R. Br.]. Genetics 182, 899–910. doi: 10.1534/genetics.109.102756
Sehgal, D., Skot, L., Singh, R., Srivastava, R. K., Das, S. P., Taunk, J., et al. (2015). Exploring potential of pearl millet germplasm association panel for association mapping of drought tolerance traits. PLoS ONE 10:e0122165. doi: 10.1371/journal.pone.0122165
Vigouroux, Y., Jaqueth, J. S., Matsuoka, Y., Smith, O. S., Beavis, W. D., Smith, J. S., et al. (2002). Rate and pattern of mutation at microsatellite loci in maize. Mol. Biol. Evol. 19, 1251–1260. doi: 10.1093/oxfordjournals.molbev.a004186
Vigouroux, Y., Mariac, C., De Mita, S., Pham, J. L., Gérard, B., Kapran, I., et al. (2011). Selection for earlier flowering crop associated with climatic variations in the Sahel. PLoS ONE 6:e19563. doi: 10.1371/journal.pone.0019563
Vigouroux, Y., Mitchell, S., Matsuoka, Y., Hamblin, M., Kresovich, S., Smith, J. S., et al. (2005). An analysis of genetic diversity across the maize genome using microsatellites. Genetics 169, 1617–1630. doi: 10.1534/genetics.104.032086
Wang, J. (2001). A pseudo-likelihood method for estimating effective population size from temporally spaced samples. Genet. Res. 78, 243–257. doi: 10.1017/S0016672301005286
Wang, J. (2005). Estimation of effective population sizes from data on genetic markers. Philos. Trans. R. Soc. Lond. B Biol. Sci. 360, 1395–1409. doi: 10.1098/rstb.2005.1682
Wilson, A. J., Pemberton, J. M., Pilkington, J. G., Coltman, D. W., Mifsud, D. V., Clutton-Brock, T. H., et al. (2006). Environmental coupling of selection and heritability limits evolution. PLoS Biol. 4:e216. doi: 10.1371/journal.pbio.0040216
Keywords: selection, temporal and spatial variability, functional diversity, pearl millet, adaptation to climate variation
Citation: Mariac C, Ousseini IS, Alio A-K, Jugdé H, Pham J-L, Bezançon G, Ronfort J, Descroix L and Vigouroux Y (2016) Spatial and Temporal Variation in Selection of Genes Associated with Pearl Millet Varietal Quantitative Traits In situ. Front. Genet. 7:130. doi: 10.3389/fgene.2016.00130
Received: 01 April 2016; Accepted: 07 July 2016;
Published: 26 July 2016.
Edited by:
Manoj Prasad, National Institute of Plant Genome Research, IndiaReviewed by:
Dongying Gao, University of Georgia, USADeepmala Sehgal, International Maize and Wheat Improvement Center, Mexico
Copyright © 2016 Mariac, Ousseini, Alio, Jugdé, Pham, Bezançon, Ronfort, Descroix and Vigouroux. This is an open-access article distributed under the terms of the Creative Commons Attribution License (CC BY). The use, distribution or reproduction in other forums is permitted, provided the original author(s) or licensor are credited and that the original publication in this journal is cited, in accordance with accepted academic practice. No use, distribution or reproduction is permitted which does not comply with these terms.
*Correspondence: Yves Vigouroux, yves.vigouroux@ird.fr
†Co-first authors