- Department of Veterinary Clinical and Animal Sciences, Faculty of Health and Medical Sciences, University of Copenhagen, Frederiksberg, Denmark
With unprecedented increase in next generation sequencing technologies, there has been a persistent interest on transcript profiles of long non-coding RNAs (lncRNAs) and protein-coding genes forming an interaction network. Apart from protein–protein interaction (PPI), gene network models such as Weighted Gene Co-expression Network Analysis (WGCNA) are used to functionally annotate lncRNAs in identifying their potential disease associations. To address this, studies have led to characterizing transcript structures and understanding expression profiles mediating regulatory roles. In the current exploratory analysis, we show how a lncRNA – cyp2c91 contributes to the transcriptional regulation localized to cytoplasm thereby making refractory environment for transcription. By applying network methods and pathway analyses on genes related to a disease such as obesity and systemic lupus erythematosus, we show that we can gain deeper insight in biological processes such as the perturbances in immune system, and get a better understanding of the systems biology of diseases.
Introduction
The long non-coding RNAs (lncRNAs) are known to be involved in several biological roles including epigenetic regulation, immune signaling, aberrant methylation of imprinted genes and cell cycle (Li et al., 2015). Distinct lncRNA expression profiles are associated with recurrent mutations linked to cancer and therapy related diseases (Garzon et al., 2014). With unprecedented increase in next generation sequencing (NGS) technologies, there has been a persistent interest on transcript profiles of lncRNAs and protein-coding genes forming an interaction network. Apart from protein–protein interaction (PPI), co-expression models such as Weighted Gene Co-expression Network Analysis (WGCNA; Xue et al., 2013) are used to functionally annotate lncRNAs in identifying their potential disease associations (Cogill and Wang, 2014). To address this, studies have led to characterizing transcript structures and understanding expression profiles mediating regulatory roles and comparing them with the ENCODE project (ENCODE Project Consortium, 2004). Recent reports show how lncRNAs contribute toward regulatory interactions with their non-coding peers like miRNAs (Jalali et al., 2013). It is not well-known whether lncRNA-protein networks restrain interactions. How such regulatory interactions between classes of lncRNAs and proteins would have a significant influence on the organism remains a challenge.
Earlier, we have shown three regulatory genes, viz. chemokine (C–C motif) receptor 1 (CCR1), macrophage scavenger receptor 1 (MSR1) and spleen focus forming virus proviral integration oncogene (SPI1) associated with diseases like obesity and osteoporosis using gene network algorithms WGCNA and Lemon-Tree (Kogelman et al., 2014) applied to NGS-based RNA-Seq datasets from porcine model for obesity (see RNA-Seq web reference1). These clusters of highly co-expressed genes were ranked as highly significant based on their association with obesity-related phenotypes. With a wide range of biological processes effectively used as regulatory molecules, we anticipate (a) if the co-expressed genes have interacting partners with any lncRNAs, (b) if so, whether they affect the co-expression, further changing the networks and influencing the organismal phenotype or disease outcomes, or (c) if not, what would be the outcome of such lncRNA-dependent transcription. From a putative interaction network visualized using Cytoscape (Lopes et al., 2010), we have established functional classes based on several different methods, explicitly focusing on the betweenness centrality, closeness centrality and presence of subcellular location signals (see Figure 1). These resilient methods would distinguish probability of lncRNA to show association/disassociation paradigm, RNA binding protein-lncRNA interactivity and importantly disease association, if any.
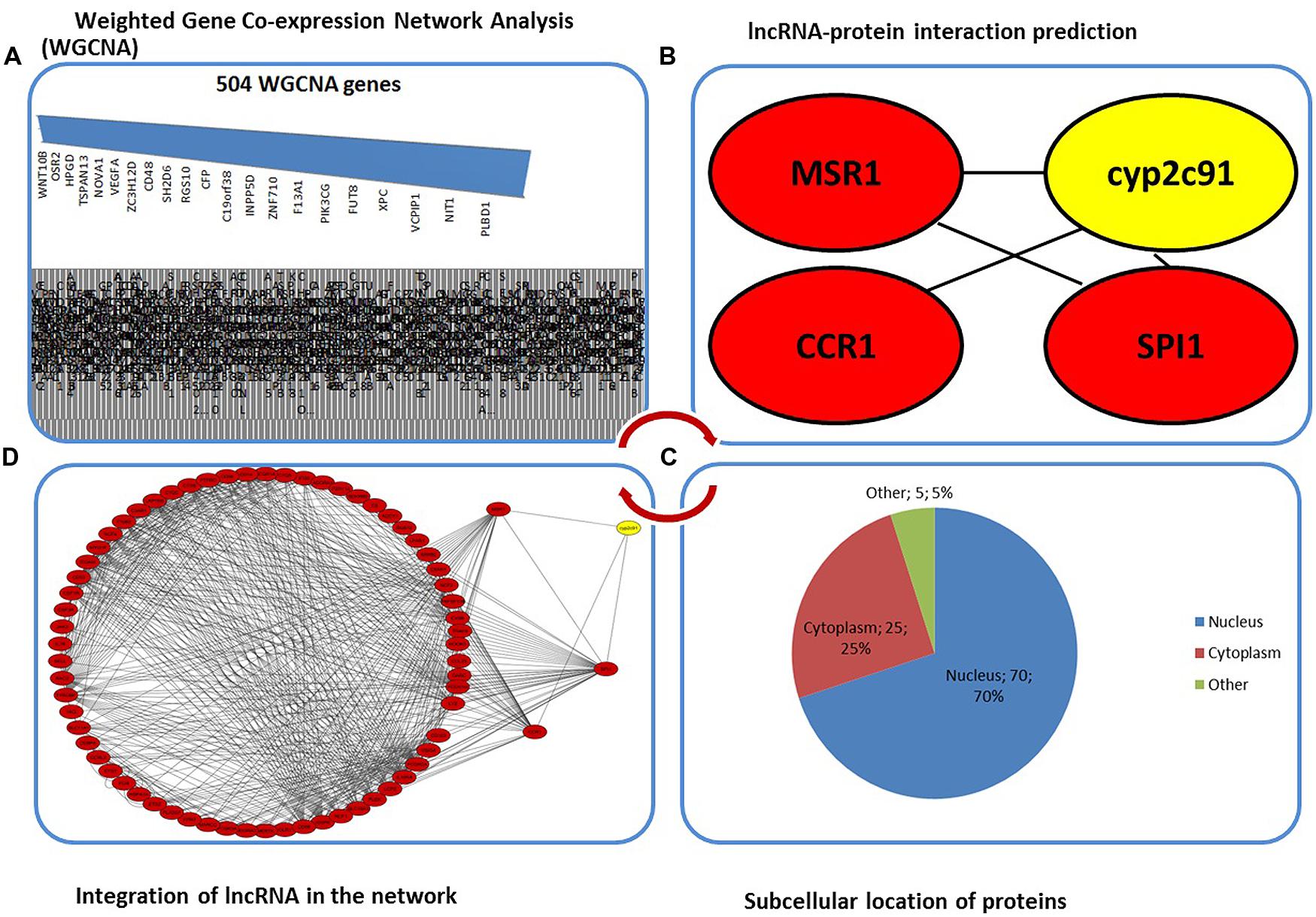
FIGURE 1. (A) The 504 genes from WGCNA across different modules linked to diseases not limited to obesity and immune response. (B) Representative lncRNA cyp2c91 gene (in yellow) shown to be interacting with three regulatory protein-coding genes predicted from RPI-pred. (C) Subcellular location of the genes associated in the network analyzed from TargetP and Cello predictions (Emanuelsson et al., 2000; Yu et al., 2014). (D) The network topology showing the profiled expression across the regulatory genes associated with cyp2c91. This is indicative of global protein-RNA interaction data.
Computational Methods for lncRNA cyp2c91–Protein Interaction
In the current study, we made a human concordant network after previously published WGCNA result from an animal model (Kogelman et al., 2014) and found that 340 of 504 porcine genes have ortholog peers in humans (Figure 1A). The 504 genes were extracted from a summated list of different modules containing clusters of highly co-expressed genes (see Supplementary Table S2). The absence of orthologs in human is in agreement with the homology data available from the Pig Analysis Database (PAD), which specifies that about 73% of the sequences are covered by the both genomes (see PAD web reference2). With the hypothesis that lncRNA–protein interactions play an important role in regulating post-transcriptional changes and subsequent localization of the transcript, we used RNA–protein interaction predictor (RPI-pred) to predict whether or not the proteins encoded by these genes and the RNA form interaction pairs (Suresh et al., 2015). From these networks and GenBank annotation, we observed that cyp2c91, a lncRNA interacts with a host of regulatory genes. The betweenness and closeness centralities were computed using Cytoscape. The topological parameters containing betweenness and closeness centralities were taken from a host of parameters (average clustering coefficient, betweenness centrality, closeness centrality, neighborhood connectivity, node degree distribution, shared neighbors, shortest length, stress centrality, topological coefficients) that are ideally calculated by Cytoscape by default. Computing these centrality indices would accomplish identifying the relationship between the nodes, understanding node-by-node quantification (Su et al., 2014). Furthermore, a classification of such nodes would allow us to understand their capability to influence the function of nodes/genes in the network, where in this case, lncRNA–protein interactions. As the betweenness centrality is computed for the interaction networks that do not contain multiple edges, closeness centrality was also added as an indicative which is reciprocal of the average shortest path length between the nodes (see Supplementary Table S1). The betweenness centrality of cyp2c91 with the three regulator genes linked to obesity (CCR1, MSR1, and SPI1) was found to be between 0 to 1 (Figure 1B). Considering the fact that these small molecules enter the nucleus without regulation, we asked if any gene products are localized to nucleus. From the subcellular prediction tools (Emanuelsson et al., 2000; Yu et al., 2014), we observed that among the three regulator genes, CCR1 was found to be localized to cytoplasm (Figure 1C). Encouraged by the outcome that the three have a plausible role of interaction with cyp2c91, we made a reliable interaction network with the mean disassociation based on the betweenness centrality (Figure 1D). We found that MSR1 and SPI1 form interacting pairs with each other while CCR1 was a lone gene. Nonetheless, the lncRNA–protein interactions were extended with the CCR1-cyp2c91 association mapped from network genes. The study suggests two ways forward. First, the fold change can be attributed to lncRNA-dependent transcription. Second, CCR1-cyp2c91 association is significant when compared to MSR1-cyp2c91 and SPI1-cyp2c91 where the genes are regulatory in nature forming diseased network. The three regulatory genes are associated with obesity and immune system, possibly linking them to Lupus. This is evident by the fact that several genes present in the WGCNA modules of Kogelman et al. (2014; TNIP1, GPSM3, TFEC, TES, KCP, IRF5, TNPO3, ELF1, ITGAM and TNXB, KLF6, AKR1E2) are related to nuclear factor-kappaB (NF-κB) signaling pathways classified to immune system and systemic lupus erythematosus (SLE; Cen et al., 2013). This might allow us to use this network as a model for immune response or obesity.
Conclusion
The genome is lengthily transcribed in eukaryotes and it has been known that many transcripts have larger proportion of non-coding components. Although about 66–73% of the porcine genome (including ESTs, genes etc.) is conserved across humans, a considerable set of genes regulate interactions with lncRNAs. Further, a range of transcribed regions might tend to be regulatory and indicative of enhancing non-functional activity. Moving to a broader spectrum of calling them as junk, we ask for evidences on their regulatory potential based on their association with protein-coding genes. Consistent with the interaction networks, subcellular localization of the products of the three protein-coding genes revealed that two are nuclear while one, CCR1 was found to be in cytoplasm. This is again in agreement with the fact that the subcellular fractions of lncRNA differ significantly from each other, with a majority enriched in the nucleus, cytoplasm, and ribosomes (van Heesch et al., 2014). These results show that lncRNA–protein interactions are self-regulating and may not be influenced by organellar specificity. Our exploratory analysis suggests that CCR1-cyp2c91 contributes to the transcriptional regulation localized to cytoplasm thereby making refractory environment for transcription. By applying network methods and pathway analyses on genes related to a disease such as obesity and SLE, we show that we can gain deeper insight in biological processes such as the perturbances in immune system, and get a better understanding of the systems biology of diseases. This stresses the possible need of finding genes linked to lncRNA-protein networks and further use them as potential diagnostic markers.
Conflict of Interest Statement
The authors declare that the research was conducted in the absence of any commercial or financial relationships that could be construed as a potential conflict of interest.
Supplementary Material
The Supplementary Material for this article can be found online at: http://journal.frontiersin.org/article/10.3389/fgene.2015.00255
Footnotes
References
Cen, H., Zhou, M., Leng, R. X., Wang, W., Feng, C. C., Li, B. Z., et al. (2013). Genetic interaction between genes involved in NF-κB signaling pathway in systemic lupus erythematosus. Mol. Immunol. 56, 643–648. doi: 10.1016/j.molimm.2013.07.006
Cogill, S. B., and Wang, L. (2014). Co-expression network analysis of human lncRNAs and cancer genes. Cancer Inform. 13(Suppl. 5), 49–59.
Emanuelsson, O., Nielsen, H., Brunak, S., and von Heijne, G. (2000). Predicting subcellular localization of proteins based on their N-terminal amino acid sequence. J. Mol. Biol. 300, 1005–1016. doi: 10.1006/jmbi.2000.3903
ENCODE Project Consortium. (2004). The ENCODE (ENCyclopedia Of DNA Elements) project. Science 306, 636–640.
Garzon, R., Volinia, S., Papaioannou, D., Nicolet, D., Kohlschmidt, J., Yan, P. S., et al. (2014). Expression and prognostic impact of lncRNAs in acute myeloid leukemia. Proc. Natl. Acad. Sci. U.S.A. 111, 18679–18684. doi: 10.1073/pnas.1422050112
Jalali, S., Bhartiya, D., Lalwani, M. K., Sivasubbu, S., and Scaria, V. (2013). Systematic Transcriptome wide analysis of lncRNA-miRNA interactions. PLoS ONE 8:e53823. doi: 10.1371/journal.pone.0053823
Kogelman, L. J. A., Cirera, S., Zhernakova, D. V., Fredholm, M., Franke, L., and Kadarmideen, H. N. (2014). Identification of co-expression gene networks, regulatory genes and pathways for obesity based on adipose tissue RNA Sequencing in a porcine model. BMC Med. Genomics 7:57. doi: 10.1186/1755-8794-7-57
Li, Y., Zhang, Y., Li, S., Lu, J., Chen, J., Wang, Y., et al. (2015). Genome-wide DNA methylome analysis reveals epigenetically dysregulated non-coding RNAs in human breast cancer. Sci. Rep. 5:8790. doi: 10.1038/srep08790
Lopes, C. T., Franz, M., Kazi, F., Donaldson, S. L., Morris, Q., and Bader, G. D. (2010). Cytoscape Web: an interactive web-based network browser. Bioinformatics 26, 2347–2348. doi: 10.1093/bioinformatics/btq430
Su, G., Morris, J. H., Demchak, B., and Bader, G. D. (2014). Biological network exploration with cytoscape 3. Curr. Protoc. Bioinformatics 47, 8.13.1–8.13.24. doi: 10.1002/0471250953.bi0813s47
Suresh, V., Liu, L., Adjeroh, D., and Zhou, X. (2015). RPI-Pred: predicting ncRNA-protein interaction using sequence and structural information. Nucleic Acids Res. 43, 1370–1379. doi: 10.1093/nar/gkv020
van Heesch, S., van Iterson, M., Jacobi, J., Boymans, S., Essers, P. B., de Bruijn, E., et al. (2014). Extensive localization of long noncoding RNAs to the cytosol and mono- and polyribosomal complexes. Genome Biol. 15:R6. doi: 10.1186/gb-2014-15-1-r6
Xue, Z., Huang, K., Cai, C., Cai, L., Jiang, C. Y., Feng, Y., et al. (2013). Genetic programs in human and mouse early embryos revealed by single-cell RNA sequencing. Nature 500, 593–597. doi: 10.1038/nature12364
Keywords: long non-coding RNA, systems biology, protein interactions, disease association, WGCNA
Citation: Suravajhala P, Kogelman LJA, Mazzoni G and Kadarmideen HN (2015) Potential role of lncRNA cyp2c91–protein interactions on diseases of the immune system. Front. Genet. 6:255. doi: 10.3389/fgene.2015.00255
Received: 03 April 2015; Accepted: 15 July 2015;
Published: 28 July 2015.
Edited by:
Daniel Shriner, National Human Genome Research Institute, USACopyright © 2015 Suravajhala, Kogelman, Mazzoni and Kadarmideen. This is an open-access article distributed under the terms of the Creative Commons Attribution License (CC BY). The use, distribution or reproduction in other forums is permitted, provided the original author(s) or licensor are credited and that the original publication in this journal is cited, in accordance with accepted academic practice. No use, distribution or reproduction is permitted which does not comply with these terms.
*Correspondence: Haja N. Kadarmideen, Department of Veterinary Clinical and Animal Sciences, Faculty of Health and Medical Sciences, University of Copenhagen, Grønnegårdsvej 7, 1870 Frederiksberg, Denmark,aGFqYWtAc3VuZC5rdS5kaw==