- Computational Epigenomics, Center for Genomic Science of IIT@SEMM, Fondazione Istituto Italiano di Tecnologia, Milan, Italy
A multi-layered set of epigenetic marks, including post-translational modifications of histones and methylation of DNA, is finely tuned to define the epigenetic state of chromatin in any given cell type under specific conditions. Recently, the knowledge about the combinations of epigenetic marks occurring in the genome of different cell types under various conditions is rapidly increasing. Computational methods were developed for the identification of these states, unraveling the combinatorial nature of epigenetic marks and their association to genomic functional elements and transcriptional states. Nevertheless, the precise rules defining the interplay between all these marks remain poorly characterized. In this perspective we review the current state of this research field, illustrating the power and the limitations of current approaches. Finally, we sketch future avenues of research illustrating how the adoption of specific experimental designs coupled with available experimental approaches could be critical for a significant progress in this area.
Introduction
Histone post-translational modifications (PTMs; Kouzarides, 2007) and DNA methylation (Pelizzola and Ecker, 2011) are the main constituent of the epigenome, which greatly contributes to the definition of cells’ identity through the instruction of specific transcriptional and regulatory programs (Rivera and Ren, 2013). Histone PTMs mostly occur on the amino-terminal tails of the core histone proteins, which protrude through the DNA backbone and are exposed on the nucleosomal surface where they are subjected to a wide range of enzyme-catalyzed modifications (Rothbart and Strahl, 2014). These include acetylation of lysine, methylation of both lysine and arginine and phosphorylation of serine, and threonine residues. It is also possible to distinguish these PTMs based on the number of such modifications (e.g., mono-, di-, or tri-methylation of lysines), and based on the symmetry (or lack of) of the modification over the two copies of each core histone (Voigt et al., 2012). Histone PTMs are recognized by specific binding proteins, acting as docking point for chromatin regulators (CRs), which in turn could trigger further modifications in a chain process (Schreiber and Bernstein, 2002).
Assigning a clear and distinct functional role to each histone PTM has proven to be elusive. For example, phosphorylation of H3 at serine 10 is associated with both chromosome condensation during mitosis (Hendzel et al., 1997; Wei et al., 1999) and transcriptional activation following mitogenic stimulation (Barratt et al., 1994). Similarly, histone acetylation, which is commonly associated to active chromatin, can also be linked to gene repression (Braunstein et al., 1996; De Rubertis et al., 1996). Moreover, DNA methylation can be an important feature in either repressed or actively transcribed genes, depending on the localization at the level of promoter or gene-body, respectively (Pelizzola and Ecker, 2011; Baubec and Schübeler, 2014).
These observations led to the formulation of the histone code hypothesis (Strahl and Allis, 2000) which, including DNA methylation, can be extended to the more general epigenetic code. The main idea behind the histone code is that the functional role of histone PTMs is better defined taking into account multiple histone modifications acting in a combinatorial or sequential fashion, specifying unique downstream functions. The compliance of the histone code to the semiotic definition of a code was discussed by Turner (2007). In that context, a code is a system made of signs, to which a meaning is assigned by the rules of the code itself. The rules, in biology are the “readers” of the code: for the genetic code tRNAs, for the epigenetic code CRs. Following the semiotic definition, no causal relationships should exist between the sign and its meaning. However, as in the cases of lysine acetylation and serine phosphorylation, some modifications, or signs, physically predispose the local environment to the fulfillment of their meaning. Probably many real codes break semiotic rules in order to reduce reading errors and increase robustness. For example, in the traffic-light code it was decided to rely on red, a color associated with fear, to convey the action of stopping. A good code is a code that works, at the end.
The existence of the epigenetic code is supported by the observation that CRs are often more specific for peptides marked by multiple modifications (Morinière et al., 2009; Ruthenburg et al., 2011). Additionally, CRs can be multimeric complexes containing multiple recognition sites (Lindroth et al., 2004). Genome-wide mapping studies found limited combinatorial complexity of the marks (Huff et al., 2010; Ernst et al., 2011) compared to the number of theoretically possible combinations, but in our opinion, this does not represent an argument against the existence of the epigenetic code. Nevertheless, the emergence of the histone code hypothesis was followed by a series of criticisms. First, Rando (2012) commented that the histone code hypothesis does not provide any additional insight into the reason why a given combination of histone modifications, that globally co-occur, only affects a small subset of genes. We note here that typically only a subset of the known marks is actually profiled and that the complete set of modifications has likely not been identified yet. Thus, it is conceivable that the inclusion of the missing marks could shed light on those discrepancies. Second, limited complexity at the level of the transcriptional response is associated to mutations of lysines in the N-terminal tails of core histones in yeast (Martin et al., 2004). This could either suggest relevant redundancy in the functional role of these PTMs, or the fact that these mutations could result in other types of phenotypic responses. Finally, the transcriptional consequences of combinations of histone mutations affecting their PTMs could be in some cases predicted by the linear combination of individual mutations, suggesting that some of these modification could be read separately (Dion et al., 2005; Yuan et al., 2006). Eventually, despite debate about the complexity and prevalence of this code, this is an active area of research in both experimental and computational genomics.
Computational Methods for the Identification of Chromatin States
Following the histone code hypothesis, computational tools were developed for the identification of recurrent combinations of histone PTMs. Combinations associated to transcribed regions, active promoters, and enhancers were recognized and used to identify new occurrences of the same regions, allowing the prediction of new non-coding transcripts (Guttman et al., 2009; Fatica and Bozzoni, 2013) and enhancers sites (Heintzman et al., 2007; Wang et al., 2013).
Conversely, computational tools were developed for the unsupervised identification of recurrent combinations of histone PTMs, showing that the chromatin can be described by a limited number of chromatin states that are specifically enriched in functional genomic regions. The most widely used unsupervised tools are ChromaSig (Hon et al., 2008) and ChromHMM (Ernst and Kellis, 2012). ChromaSig was applied for the identification of 16 clusters of histone modifications using genome-wide maps of 21 chromatin marks from ChIP-chip experiments in HeLa cells. ChromHMM was applied for the analysis of 38 histone modifications, H2AZ, RNA polymerase II, and CTCF in human CD4 T-cells and allowed the identification of 51 distinct chromatin states using multivariate Hidden Markov Models. These states could be subsequently matched to functional genomic elements and distinguished into six broad classes of chromatin states: promoter, enhancer, insulator, transcribed, repressed, and inactive states. ChromHMM is also able to classify chromatin in regions strongly depleted of histone modifications. Overall, the results of the two methods applied to the same dataset are highly correlated (Ernst and Kellis, 2010). These tools are currently the reference for a holistic view of the chromatin and for associating complex combinatorial signatures of histone PTMs with critical functional elements of the genome (Figure 1A).
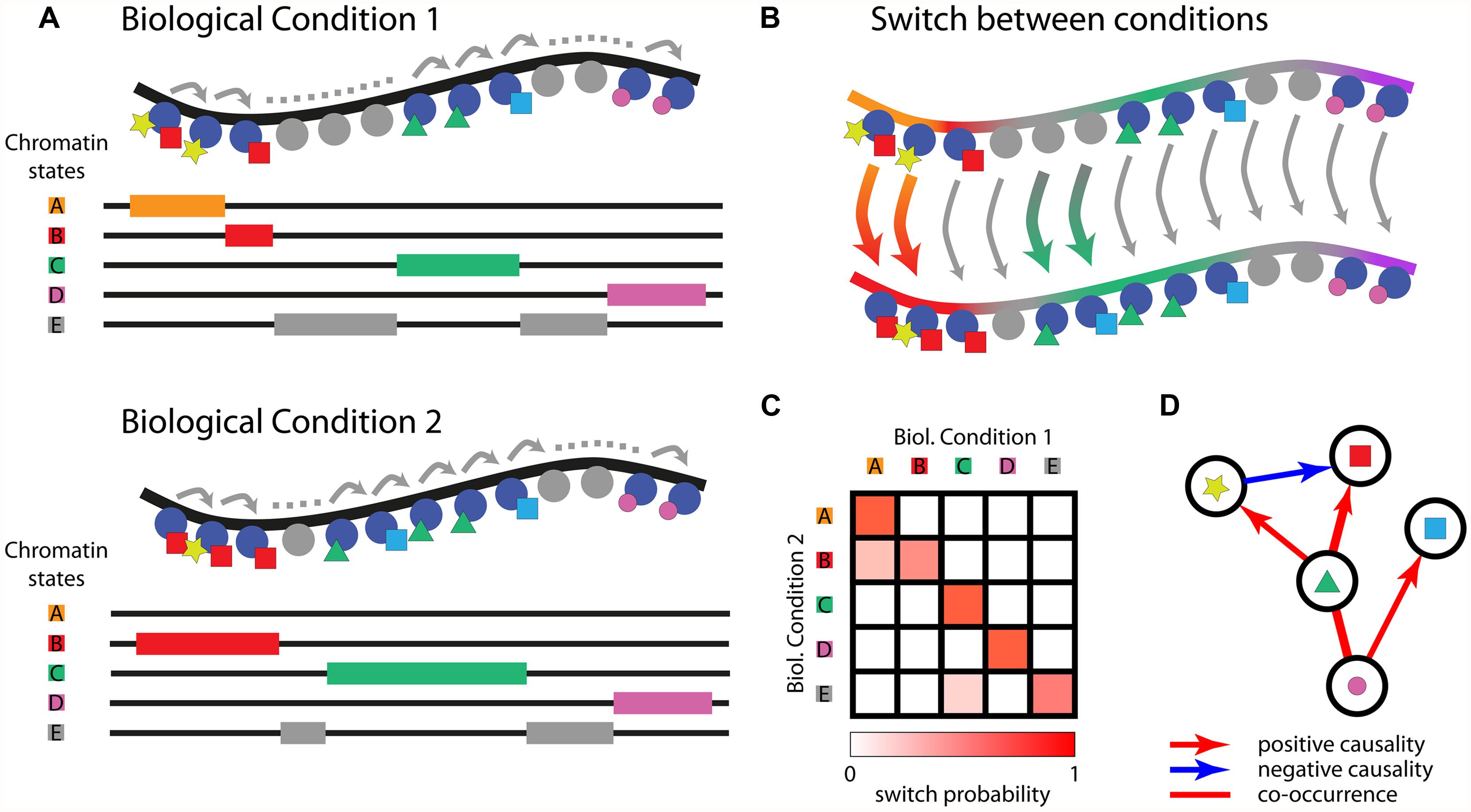
FIGURE 1. Adopting chromatin states to decipher the interplay between epigenetic marks across multiple biological conditions. HMM-based learning of chromatin states; DNA is depicted in black, histones as blue or gray circles, and different histone’s PTMs as colored shapes. Chromatin states identifying relevant combinations of histones PTMs are drawn in the underlying diagram (A). Chromatin states can be compared over different cell types or biological conditions; arrows represent the switch between different states (B). Heatmap displaying the probability of switching between chromatin states in different biological conditions (C). Graph depicting causal relationships among epigenetic marks determined based on (C,D).
It is now clear that out of the enormous theoretical combinatorial complexity of histone PTMs, only a subset of these combinations seem to occur in nature. Histone modifications are highly related to each other, some of them are highly co-occurring while other are clearly anti-correlated, greatly reducing the combinatorial complexity. Taking a step ahead in the study of the correlation structure among these marks, a couple of studies was recently published adopting approaches based on (i) partial correlations and (ii) maximum entropy modeling. On one hand, partial correlations were used to discriminate the cases where two modifications are both strongly related to a third one, prompting the possibility that their correlation is only an indirect effect. Partial correlation between marks A and B is determined as the correlation of the residuals arising from the linear modeling of the individual marks (A and B) with an additional factor (mark C). If the residuals are not correlated it means that the correlation between A and B is likely due to the similarity to the mark C (Lasserre et al., 2013). On the other hand, a framework based on maximum entropy modeling was adopted to decipher pairwise and higher-order interactions between chromatin factors (Zhou and Troyanskaya, 2014). Approaches like these are critical to obtain more meaningful network relating different marks, disentangling direct from indirect similarities.
Dynamics of Chromatin States
While important progress was recently made to increase the likelihood of identifying biologically relevant similarities and interactions among epigenetic marks (Lasserre et al., 2013; Zhou and Troyanskaya, 2014), new computational tools and carefully designed experiments are needed to progress from correlative to causal analyses. Most of the studies in this area are conducted using data collected under static conditions, or steady state, and are limited to the identification of networks of epigenetic marks that are necessarily undirected and lack causality. Indeed, after the formalization of the histone code hypothesis, numerous experimental and computational efforts have been carried over to chart chromatin states in a plethora of biological conditions and cell types. These efforts raised the consciousness that similar chromatin states exist in different cell types but they are in some cases displaced (Ernst et al., 2011; Ernst and Kellis, 2013). To this purpose, a non-parametric method for the analysis of differential chromatin modifications (dCMD) was developed for the identification of cell-type specific regulatory elements (Chen et al., 2013), based on the same data used in (Ernst et al., 2011). The comprehension on the mechanism of this displacement, which leads specific portions of the chromatin to change their configuration and possibly the expression pattern, requires following these processes step by step. It is now time for a better understanding on the mechanisms that are responsible for the establishment of a given chromatin state and for its subsequent modification. Given a chromatin state composed of marks A, B, and C in a biological condition X, is there a specific order in their deposition that determined the emergence of the combinatorial pattern as a stable and relevant state? Given a biological condition Y derived from X, which mechanisms determine the establishment of differential patterns of chromatin states (Figure 1B)? Using Bayesian statistics, recent works tried to infer causality among different marks using only data from single steady state conditions (Yu et al., 2008). We believe that this task would be greatly facilitated by the study of chromatin state dynamics in subsequent biological conditions, such as in the course of consecutive differentiation stages or in general following biological responses over time. This would be critical to dissect which histone marks are more important in positioning other marks in subsequent conditions (Figures 1C,D). While highly relevant, efforts made to explore the variation of chromatin states between different cell types (Ernst et al., 2011) are limited in terms of improving our comprehension about the mechanisms that could have brought to those alternative differentiation end points. Multiple differentiation steps could have been missed, which could be critical for comprehending the mechanisms bringing from one chromatin state to the following. Choosing the right experimental design, or conditions to compare, is not straightforward. Even comparing consecutive differentiation stages can be uninformative, because not every differentiation step involves chromatin remodeling. During erythroid differentiation, for example, chromatin states are established at the stage of lineage commitment and extensive changes in gene expression follow a different recruitment of the master transcription factor GATA1, while the chromatin state profiles and accessibility remain largely unchanged (Wu et al., 2011).
Epigenetic Code: The Role of the Chromatin Regulators
As previously noted, a code is made essentially by three components: the sign, the meaning, and the reader of the sign (Turner, 2007). According to that, the same sign could convey a different meaning given different readers, meaning that the expression of a different set of CRs could determine an alternative readout of the same epigenetic marks, while leaving the signs (the marks) unchanged. As discussed above, the same histone modifications could have different functional roles in different moments of the cell cycle, as in the case of the H3S10 phosphorylation during mitosis and mitogenic stimulation (Strahl and Allis, 2000). The two most likely explanations are that additional modifications have to be taken into account to confer the right meaning to that shared sign (mark), or that there is some difference at the level of the readers. Strahl and Allis (2000) in their pioneer article on the histone code, hypothesized that “part of the solution to this paradox may be in having unique histone codes read by distinct sets of proteins that then bring about different downstream responses. If correct, it may be that mitosis-specific HATs, HDACs, and HMTs act during chromosome condensation and that distinct sets of histone-modifying enzymes mark chromatin for decondensation during gene activation” (Strahl and Allis, 2000). Indeed different cell types have been described to express different sets of CRs (Ho and Crabtree, 2010) among the 100s encoded by the human genome (Kouzarides, 2007; Ruthenburg et al., 2007), leading to a possible context dependent interpretation of the marks. During development, for example, different combinations of the vertebrate SWI/SNF complexes undergo progressive changes in subunit composition, from pluripotent stem cells to multipotent neuronal progenitor cells to a committed neuron (Lessard et al., 2007; Yan et al., 2008). Alterations in the composition of these complexes at the level of specific subunits can influence the ability of these cell types to self-renew or differentiate (Lessard et al., 2007; Ho et al., 2009), suggesting that the cell specific reader is critical to determine cell identity. CRs have been identified as a new set of driver genes in different types of tumors (Elsässer et al., 2011) confirming their relevance.
Chromatin regulators not only need to be correctly expressed in the specific cell type, but also need gain access to the locus where the target histone mark is located in order to exploit their function. In a recent study, chromatin localization of 29 CRs was profiled genome-wide in K562 cells and human ESCs (Ram et al., 2011). Very recently, a powerful experimental methodology was developed to study the substrate specificity of CRs, based on the semi-synthesis of nucleosome libraries with distinct combinations of PTMs (Nguyen et al., 2014). Similar approaches can help elucidating the interaction between chromatin states and CRs, being able to properly assign distinct functions to each of these ensembles.
Turner (2007) suggested the following definition for the epigenetic code: it “describes the way in which the potential for expression of genes in a particular cell type is specified by chromatin modifications put in place at an earlier stage of differentiation.” This definition particularly fits in the context described above, in which the presence of a specific reader could eventually put into effect the meaning of a previously set epigenetic mark.
Experimental Methods for Investigating the Epigenetic Code
The success in deciphering the epigenetic code depends on the availability of new computational methods and the generation of suitable datasets. The latter have to be as informative as possible on the combinatorial combinations in which the epigenetic marks could occur. The highest is the coverage of any possible occurring combination, the highest is the likelihood for the computational tools to capture it. Two types of datasets are most suited for these analyses: (i) large-scale public datasets, and (ii) ad hoc perturbation datasets.
Large-scale datasets such as ChIP-chip and ChIP-seq samples stored in public repositories currently account for 1000s of samples in various species, tissues, and conditions, representing a formidable resource covering numerous epigenetic combinatorial states. Still, while numerous, these datasets do not guarantee complete covering of all possible combinations among known epigenetic marks. To overcome this issue, it is nowadays possible to take advantage of experimental methods to build perturbation experiments, providing medium- or high-throughput datasets where the combinations among epigenetic marks are explored through direct perturbation of a baseline combinatorial state. These experiments naturally provide important hints on the causal mechanisms determining the occurrence of these combinatorial states. Among these experimental procedures (i) precision epigenetic engineering and (ii) high-throughput screening methods are emerging as powerful tools to rewire the epigenome. Examples of the former are epigenome-editing tools such as transcription activator-like effector repeat arrays (TALE) and Zinc fingers, which can be coupled with enzymes modifying a given epigenetic mark in targeted genomic regions. Specifically, engineered TALE were fused to the TET1 hydroxylase catalytic domain to obtain targeted demethylation of endogenous promoters in human cells, determining increased transcription of the downstream genes (Maeder et al., 2013). Similarly, another group was able to fuse TALE with the LSD1 histone de-methylase to remove H3K4me3 at the level of enhancer sites, driving transcriptional repression of the target gene (Mendenhall et al., 2013; Figures 2A,B). Using zinc finger proteins (ZFPs), 223 CRs were fused to ZFPs in a remarkable effort to study the transcriptional logic resulting from combinatorial recruitment of CRs in yeast (Keung et al., 2014). Finally, coupling of the DNMT3a catalytic domain with ZFPs allowed targeted methylation of the promoter of the tumor suppressor Maspin determining its stable transcriptional repression over multiple cell generations, even in absence of sustained presence of the ZFPs (Rivenbark et al., 2012). Ongoing research is focused on the development of similar tools adopting a more flexible methodology based on clustered regularly interspaced short palindromic repeats (CRISPR). CRISPR constructs associated to catalytic domains of enzymes targeting the epigenome could conveniently be directed to specific genomic loci through the simple transfection of guide-RNA molecules complementary to the target region.
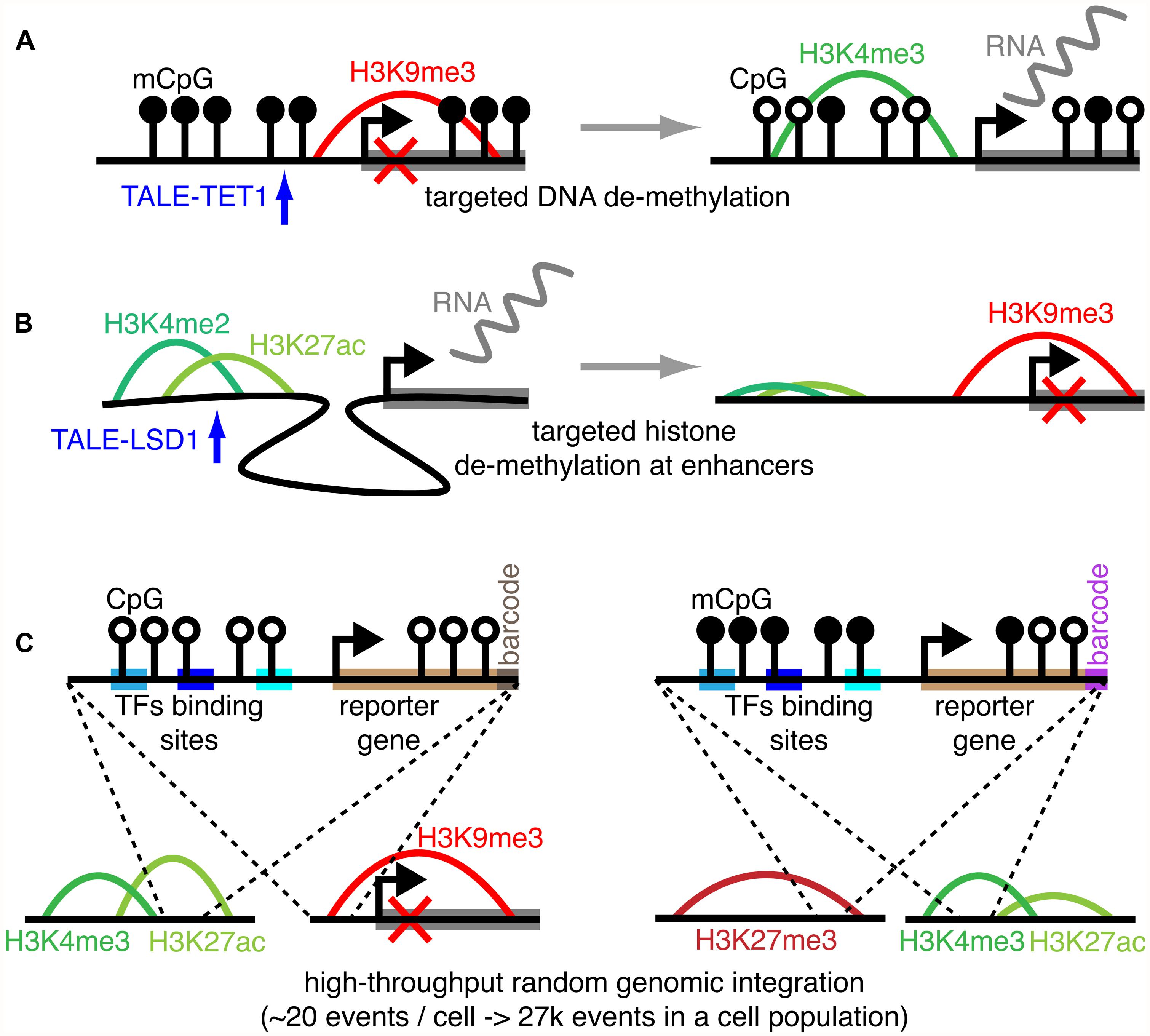
FIGURE 2. Experimental methods to probe the epigenetic code. Using a TALE-TET1 construct to determine TET1-mediated DNA de-methylation of a specific promoter, resulting in the transcriptional induction of the downstream gene (A). Using a TALE-LSD1 construct to determine LSD1-mediated histone de-methylation of a specific active enhancer, resulting in enhancer inactivation and the transcriptional repression of the target gene (B). Simplified representation of the thousands of reporters integrated in parallel (TRIP) method that can be used to probe the epigenome with reporter genes set under control of specific regulatory and epigenetic input, here depicted as transcription factor (TFs) binding sites and DNA methylation (C).
Importantly these methods are suitable for multiplexing, allowing in principle to target various enzymatic activities of interest in multiple genomic regions. These methods are still under development, including a better quantification and minimization of off-target effects. Nevertheless, published proof of concept experiments suggest that these tools offer a unique opportunity for interfering with the epigenetic code, injecting controlled, and targeted epigenetic alterations and opening new avenues of research in rewiring epigenome code in normal and cancer cells (Blancafort et al., 2013). Finally, matching these epigenetic perturbations with the consequent alteration in the binding of regulatory proteins and gene transcriptional activity will provide data suitable for reverse engineering the interplay among epigenetic, regulatory, and transcriptional layers.
On the other hand, high-throughput screening methods were developed to probe the epigenome by measuring how the local epigenetic state influences the expression of reporter genes randomly integrated in the genome. In particular, the thousands of reporters integrated in parallel (TRIP) method was recently developed to target 1000s of random genomic loci in a cell population with a gene reporter. The promoters controlling the reporter expression can be designed to contain specific transcriptional factor binding sites and/or to host methylated cytosines (Figure 2C). This would allow us to associate the reporter gene activity to both a specific regulatory/epigenetic input and the epigenetic state of the insertion region. Indeed, both the location of the insertion events and the normalized expression of the reporter gene can be determined through simple high-throughput sequencing of barcoded sequences, without cloning, greatly simplifying the experimental setting and accelerating the acquisition of the data (Akhtar et al., 2013, 2014).
Conclusion
The comprehension on the mechanism regulating the intricate networks of epigenetic modifications represents a formidable while exciting area of research. Significant progress is currently being made thanks to the advent of high-throughput sequencing technologies, which are allowing an unprecedented accumulation of data and the development of computational tools developed to characterize the combinatorial nature of the epigenome. It is now time to design experiments which are directly intended to challenge and deepen our knowledge in this field, taking advantage of powerful epigenome-editing and high-throughput screening experimental methodologies which are currently being made available.
Conflict of Interest Statement
The authors declare that the research was conducted in the absence of any commercial or financial relationships that could be construed as a potential conflict of interest.
Acknowledgments
Stefano de Pretis and Mattia Pelizzola acknowledge funding from the European Community’s Seventh Framework Program (FP7/2007-2013), project RADIANT (grant agreement no. 305626).
References
Akhtar, W., de Jong, J., Pindyurin, A. V., Pagie, L., Meuleman, W., de Ridder, J.,et al. (2013). Chromatin position effects assayed by thousands of reporters integrated in parallel. Cell 154, 914–927. doi: 10.1016/j.cell.2013.07.018
Akhtar, W., Pindyurin, A. V., de Jong, J., Pagie, L., Hoeve ten, J., Berns, A.,et al. (2014). Using TRIP for genome-wide position effect analysis in cultured cells. Nat. Protoc. 9, 1255–1281. doi: 10.1038/nprot.2014.072
Barratt, M. J., Hazzalin, C. A., Cano, E., and Mahadevan, L. C. (1994). Mitogen-stimulated phosphorylation of histone H3 is targeted to a small hyperacetylation-sensitive fraction. Proc. Natl. Acad. Sci. U.S.A. 91, 4781–4785. doi: 10.1073/pnas.91.11.4781
Baubec, T., and Schübeler, D. (2014). Genomic patterns and context specific interpretation of DNA methylation. Curr. Opin. Genet. Dev. 25, 85–92. doi: 10.1016/j.gde.2013.11.015
Blancafort, P., Jin, J., and Frye, S. (2013). Writing and rewriting the epigenetic code of cancer cells: from engineered proteins to small molecules. Mol. Pharmacol. 83, 563–576. doi: 10.1124/mol.112.080697
Braunstein, M., Sobel, R. E., Allis, C. D., Turner, B. M., and Broach, J. R. (1996). Efficient transcriptional silencing in Saccharomyces cerevisiae requires a heterochromatin histone acetylation pattern. Mol. Cell. Biol. 16, 4349–4356.
Chen, C., Zhang, S., and Zhang, X. S. (2013). Discovery of cell-type specific regulatory elements in the human genome using differential chromatin modification analysis. Nucleic Acids Res. 41, 9230–9242. doi: 10.1093/nar/gkt712
De Rubertis, F., Kadosh, D., Henchoz, S., Pauli, D., Reuter, G., Struhl, K.,et al. (1996). The histone deacetylase RPD3 counteracts genomic silencing in Drosophila and yeast. Nature 384, 589–591. doi: 10.1038/384589a0
Dion, M. F., Altschuler, S. J., Wu, L. F., and Rando, O. J. (2005). Genomic characterization reveals a simple histone H4 acetylation code. Proc. Natl. Acad. Sci. U.S.A. 102, 5501–5506. doi: 10.1073/pnas.0500136102
Elsässer, S. J., Allis, C. D., and Lewis, P. W. (2011). Cancer. New epigenetic drivers of cancers. Science 331, 1145–1146. doi: 10.1126/science.1203280
Ernst, J., and Kellis, M. (2010). Discovery and characterization of chromatin states for systematic annotation of the human genome. Nat. Biotechnol. 28, 817–825. doi: 10.1038/nbt.1662
Ernst, J., and Kellis, M. (2012). ChromHMM: automating chromatin-state discovery and characterization. Nat. Methods 9, 215–216. doi: 10.1038/nmeth.1906
Ernst, J., and Kellis, M. (2013). Interplay between chromatin state, regulator binding, and regulatory motifs in six human cell types. Genome Res. 23, 1142–1154. doi: 10.1101/gr.144840.112
Ernst, J., Kheradpour, P., Mikkelsen, T. S., Shoresh, N., Ward, L. D., Epstein, C. B.,et al. (2011). Mapping and analysis of chromatin state dynamics in nine human cell types. Nature 473, 43–49. doi: 10.1038/nature09906
Fatica, A., and Bozzoni, I. (2013). Long non-coding RNAs: new players in cell differentiation and development. Nat. Rev. Genet. 15, 7–21. doi: 10.1038/nrg3606
Guttman, M., Amit, I., Garber, M., French, C., Lin, M. F., Feldser, D.,et al. (2009). Chromatin signature reveals over a thousand highly conserved large non-coding RNAs in mammals. Nature 457, 223–227. doi: 10.1038/nature07672
Heintzman, N. D., Stuart, R. K., Hon, G., Fu, Y., Ching, C. W., Hawkins, R. D.,et al. (2007). Distinct and predictive chromatin signatures of transcriptional promoters and enhancers in the human genome. Nat. Genet. 39, 311–318. doi: 10.1038/ng1966
Hendzel, M. J., Wei, Y., Mancini, M. A., Van Hooser, A., Ranalli, T., Brinkley, B. R.,et al. (1997). Mitosis-specific phosphorylation of histone H3 initiates primarily within pericentromeric heterochromatin during G2 and spreads in an ordered fashion coincident with mitotic chromosome condensation. Chromosoma 106, 348–360. doi: 10.1007/s004120050256
Ho, L., and Crabtree, G. R. (2010). Chromatin remodelling during development. Nature 463, 474–484. doi: 10.1038/nature08911
Ho, L., Ronan, J. L., Wu, J., Staahl, B. T., Chen, L., Kuo, A.,et al. (2009). An embryonic stem cell chromatin remodeling complex, esBAF, is essential for embryonic stem cell self-renewal and pluripotency. Proc. Natl. Acad. Sci. U.S.A. 106, 5181–5186. doi: 10.1073/pnas.0812889106
Hon, G., Ren, B., and Wang, W. (2008). ChromaSig: a probabilistic approach to finding common chromatin signatures in the human genome. PLoS Comput. Biol. 4:e1000201. doi: 10.1371/journal.pcbi.1000201
Huff, J. T., Plocik, A. M., Guthrie, C., and Yamamoto, K. R. (2010). Reciprocal intronic and exonic histone modification regions in humans. Nat. Struct. Mol. Biol. 17, 1495–1499. doi: 10.1038/nsmb.1924
Keung, A. J., Bashor, C. J., Kiriakov, S., Collins, J. J., and Khalil, A. S. (2014). Using targeted chromatin regulators to engineer combinatorialand spatial transcriptional regulation. Cell 158, 110–120. doi: 10.1016/j.cell.2014.04.047
Kouzarides, T. (2007). Chromatin modifications and their function. Cell 128, 693–705. doi: 10.1016/j.cell.2007.02.005
Lasserre, J., Chung, H.-R., and Vingron, M. (2013). Finding associations among histone modifications using sparse partial correlation networks. PLoS Comput. Biol. 9:e1003168. doi: 10.1371/journal.pcbi.1003168
Lessard, J., Wu, J. I., Ranish, J. A., Wan, M., Winslow, M. M., Staahl, B. T.,et al. (2007). An essential switch in subunit composition of a chromatin remodeling complex during neural development. Neuron 55, 201–215. doi: 10.1016/j.neuron.2007.06.019
Lindroth, A. M., Shultis, D., Jasencakova, Z., Fuchs, J., Johnson, L., Schubert, D.,et al. (2004). Dual histone H3 methylation marks at lysines 9 and 27 required for interaction with CHROMOMETHYLASE3. EMBO J. 23, 4286–4296. doi: 10.1038/sj.emboj.7600430
Maeder, M. L., Angstman, J. F., Richardson, M. E., Linder, S. J., Cascio, V. M., Tsai, S. Q.,et al. (2013). Targeted DNA demethylation and activation of endogenous genes using programmable TALE-TET1 fusion proteins. Nat. Biotechnol. 31, 1137–1142. doi: 10.1038/nbt.2726
Martin, A. M., Pouchnik, D. J., Walker, J. L., and Wyrick, J. J. (2004). Redundant roles for histone H3 N-terminal lysine residues in subtelomeric gene repression in Saccharomyces cerevisiae. Genetics 167, 1123–1132. doi: 10.1534/genetics.104.026674
Mendenhall, E. M., Williamson, K. E., Reyon, D., Zou, J. Y., Ram, O., Joung, J. K.,et al. (2013). Locus-specific editing of histone modifications at endogenous enhancers. Nat. Biotechnol. 31, 1133–1136. doi: 10.1038/nbt.2701
Morinière, J., Rousseaux, S., Steuerwald, U., Soler-López, M., Curtet, S., Vitte, A.-L.,et al. (2009). Cooperative binding of two acetylation marks on a histone tail by a single bromodomain. Nature 461, 664–668. doi: 10.1038/nature08397
Nguyen, U. T. T., Bittova, L., Muller, M. M., Fierz, B., David, Y., Houck-Loomis, B.,et al. (2014). Accelerated chromatin biochemistry using DNA- barcoded nucleosome libraries. Nat. Methods 11, 834–840. doi: 10.1038/nmeth.3022
Pelizzola, M., and Ecker, J. R. (2011). The DNA methylome. FEBS Lett. 585, 1994–2000. doi: 10.1016/j.febslet.2010.10.061
Ram, O., Goren, A., Amit, I., Shoresh, N., Yosef, N., Ernst, J.,et al. (2011). Combinatorial patterning of chromatin regulators uncovered by genome-wide location analysis in human cells. Cell 147, 1628–1639. doi: 10.1016/j.cell.2011.09.057
Rando, O. J. (2012). Combinatorial complexity in chromatin structure and function: revisiting the histone code. Curr. Opin. Genet. Dev. 22, 148–155. doi: 10.1016/j.gde.2012.02.013
Rivenbark, A. G., Stolzenburg, S., Beltran, A. S., Yuan, X., Rots, M. G., Strahl, B. D.,et al. (2012). Epigenetic reprogramming of cancer cells via targeted DNA methylation. Epigenetics 7, 350–360. doi: 10.4161/epi.19507
Rivera, C. M., and Ren, B. (2013). Mapping human epigenomes. Cell 155, 39–55. doi: 10.1016/j.cell.2013.09.011
Rothbart, S. B., and Strahl, B. D. (2014). Interpreting the language of histone and DNA modifications. Biochim. Biophys. Acta 1839, 627–643. doi: 10.1016/j.bbagrm.2014.03.001
Ruthenburg, A. J., Li, H., Milne, T. A., Dewell, S., McGinty, R. K., Yuen, M.,et al. (2011). Recognition of a mononucleosomal histone modification pattern by BPTF via multivalent interactions. Cell 145, 692–706. doi: 10.1016/j.cell.2011.03.053
Ruthenburg, A. J., Li, H., Patel, D. J., and Allis, C. D. (2007). Multivalent engagement of chromatin modifications by linked binding modules. Nat. Rev. Mol. Cell Biol. 8, 983–994. doi: 10.1038/nrm2298
Schreiber, S. L., and Bernstein, B. E. (2002). Signaling network model of chromatin. Cell 111, 771–778. doi: 10.1016/S0092-8674(02)01196-0
Strahl, B. D., and Allis, C. D. (2000). The language of covalent histone modifications. Nature 403, 41–45. doi: 10.1038/47412
Voigt, P., Leroy, G., Drury, W. J. III., Zee, B. M., Son, J., Beck, D. B.,et al. (2012). Asymmetrically modified nucleosomes. Cell 151, 181–193. doi: 10.1016/j.cell.2012.09.002
Wang, C., Zhang, M. Q., and Zhang, Z. (2013). Computational identification of active enhancers in model organisms. Genomics Proteomics Bioinformatics 11, 142–150. doi: 10.1016/j.gpb.2013.04.002
Wei, Y., Yu, L., Bowen, J., Gorovsky, M. A., and Allis, C. D. (1999). Phosphorylation of histone H3 is required for proper chromosome condensation and segregation. Cell 97, 99–109. doi: 10.1016/S0092-8674(00)80718-7
Wu, W., Cheng, Y., Keller, C. A., Ernst, J., Kumar, S. A., Mishra, T.,et al. (2011). Dynamics of the epigenetic landscape during erythroid differentiation after GATA1 restoration. Genome Res. 21, 1659–1671. doi: 10.1101/gr.125088.111
Yan, Z., Wang, Z., Sharova, L., Sharov, A. A., Ling, C., Piao, Y.,et al. (2008). BAF250B-associated SWI/SNF chromatin-remodeling complex is required to maintain undifferentiated mouse embryonic stem cells. Stem Cells 26, 1155–1165. doi: 10.1634/stemcells.2007-0846
Yu, H., Zhu, S., Zhou, B., Xue, H., and Han, J.-D. J. (2008). Inferring causal relationships among different histone modifications and gene expression. Genome Res. 18, 1314–1324. doi: 10.1101/gr.073080.107
Yuan, G.-C., Ma, P., Zhong, W., and Liu, J. S. (2006). Statistical assessment of the global regulatory role of histone acetylation in Saccharomyces cerevisiae. Genome Biol. 7, R70. doi: 10.1186/gb-2006-7-8-r70
Keywords: epigenetic code, histone code, chromatin state, histone mark, epigenome editing, chromatin regulators
Citation: de Pretis S and Pelizzola M (2014) Computational and experimental methods to decipher the epigenetic code. Front. Genet. 5:335. doi: 10.3389/fgene.2014.00335
Received: 11 July 2014; Paper pending published: 15 August 2014;
Accepted: 05 September 2014; Published online: 23 September 2014.
Edited by:
Shihua Zhang, Academy of Mathematics and Systems Science – Chinese Academy of Science, ChinaReviewed by:
Ping Ma, University of Georgia, USAYang Dai, University of Illinois at Chicago, USA
Jingyi Jessica Li, University of California, Los Angeles, USA
Copyright © 2014 de Pretis and Pelizzola. This is an open-access article distributed under the terms of the Creative Commons Attribution License (CC BY). The use, distribution or reproduction in other forums is permitted, provided the original author(s) or licensor are credited and that the original publication in this journal is cited, in accordance with accepted academic practice. No use, distribution or reproduction is permitted which does not comply with these terms.
*Correspondence: Mattia Pelizzola, Computational Epigenomics, Center for Genomic Science of IIT@SEMM, Fondazione Istituto Italiano di Tecnologia, Via Adamello 16, Milan 20139, Italy e-mail: mattia.pelizzola@iit.it