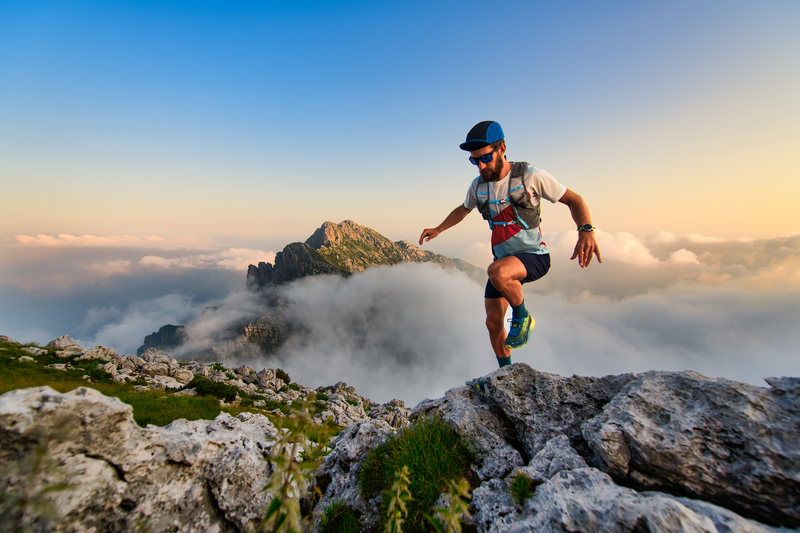
95% of researchers rate our articles as excellent or good
Learn more about the work of our research integrity team to safeguard the quality of each article we publish.
Find out more
MINI REVIEW article
Front. Genet. , 16 September 2014
Sec. Computational Genomics
Volume 5 - 2014 | https://doi.org/10.3389/fgene.2014.00324
This article is part of the Research Topic Computational epigenomics: challenges and opportunities View all 12 articles
DNA methylation, the reversible addition of methyl groups at CpG dinucleotides, represents an important regulatory layer associated with gene expression. Changed methylation status has been noted across diverse pathological states, including cancer. The rapid development and uptake of microarrays and large scale DNA sequencing has prompted an explosion of data analytic methods for processing and discovering changes in DNA methylation across varied data types. In this mini-review, we present a compact and accessible discussion of many of the salient challenges, such as experimental design, statistical methods for differential methylation detection, critical considerations such as cell type composition and the potential confounding that can arise from batch effects. From a statistical perspective, our main interests include the use of empirical Bayes or hierarchical models, which have proved immensely powerful in genomics, and the procedures by which false discovery control is achieved.
Epigenomics can be defined as the genome-wide investigation of stably heritable phenotypes resulting from changes in a chromosome without alterations in the DNA sequence (Berger et al., 2009). DNA methylation is the most well-studied epigenetic mark and notably, the enzymatic mechanism for mitotically copying methylation status is well understood (Bird, 2002), unlike the mechanism for maintaining chromatin state (Moazed, 2011). In this review, we focus on differential methylation (DM) for methyl groups added to cytosines in the CpG dinucleotide context, since this is the predominant form observed in differentiated mammalian cells (Varley et al., 2013). However, some of the statistical methods and technologies discussed here can be applied more generally.
In the last decade, considerable progress has been made in (observationally) characterizing epigenetic phenomena across a wide spectrum of normal and disease states, predominantly due to large-scale explorative studies using emerging disruptive technologies, such as microarrays and large-scale sequencing of DNA (Satterlee et al., 2010; Ziller et al., 2013). These studies have highlighted the important causal associations of DNA methylation with gene regulation and its potential in diagnosing or stratifying patients according to their combined genomic/epigenomic molecular state (e.g., Szyf, 2012). Robust and efficient statistical and computational frameworks must be developed to facilitate interpretation of the growing masses of data. For CpG methylation, the main workhorse is treatment of DNA with sodium bisulphite (Clark et al., 2006), which preserves methylated cytosines while converting unmethylated cytosines to uracil. This transformation can allow high-throughput readouts, whether by microarray hybridization or DNA sequencing, to quantify the (relative) level of methylation.
While an individual's (pathologically normal) genome is almost completely static in all cells, the epigenome is highly dynamic both in time (e.g., through development) and across cell types. Since the epigenome is a combinatorial assembly of regulatory factors (e.g., DNA methylation, histone modifications, non-coding RNAs, etc.), comprehensively profiling the epigenome is orders of magnitude more difficult than genome sequencing. Therefore, accurately measuring DNA methylation or other layers of the epigenome requires additional considerations to ensure that detected changes are not confounded with external factors, such as cell type.
Not surprisingly, the community has embraced consortium science to scale up data collection efforts. Prominent projects that involve large-scale profiling of DNA methylation include the ENCODE Roadmap Epigenomics Consortium (Bernstein et al., 2010), The Cancer Genome Atlas and International Cancer Genome Consortium (Hudson et al., 2010; Chin et al., 2011), the BLUEPRINT project (Martens and Stunnenberg, 2013) and the International Human Epigenome Consortium (Bae, 2013). See Table 1 for further description.
Present techniques for interrogating methylation fall into three categories: methylation-specific enzyme digestion, affinity enrichment, and chemical treatment with bisulphite (BS). Techniques have been used in combination (e.g., enzyme digestion then BS, commonly known as RRBS; see Laird, 2010), and with high-throughput readout. Early demonstrations were able to distinguish methylcytosine from cytosine with third-generation technologies (Flusberg et al., 2010), but no commercially viable offering has yet appeared. Methylation profiling techniques vary in resolution from low (~100–200 base pair) to high (individual CpG sites) and their costs vary widely. Each platform has its own limitations related to cost, resolution, scalability, and the amount of starting DNA required (Laird, 2010; Robinson et al., 2010; Bock, 2012). For example, enzyme digestion studies remain dependent on the location and frequency of enzyme restriction sites; the prominent BS-based microarray platform is only available for human; the sensitivity of enrichment approaches depends on CpG density, while genome-scale sequencing-based BS methods are costly and require considerable computing resources (Riebler et al., 2014). Depending on the biological question and resources available, a platform may be selected based on these tradeoffs.
Notably, BS-based methods cannot distinguish between methylcytosine and other variants, such as hydroxymethylcytosine (Huang et al., 2010), although additional treatment steps can readily allow this (Booth et al., 2012). The methods we discuss below are agnostic to this technicality, aside from specific biological questions regarding the interplay between methylation states. Another biological phenomenon we sidestep in this review is methylation in non-CpG contexts, shown to be prominent in pluripotent cells (Varley et al., 2013). Interestingly, a recent report using whole genome BS-seq across various cell types found that CpG methylation is only “dynamic” in approximately 20% of sites (Ziller et al., 2013), suggesting that BS-seq could be more directed. Enzyme digestion (and size selection) with BS-seq is already a favored method to reduce sequencing depth, but is difficult to tailor to specific genomic regions. An alternative reduced complexity strategy is to first capture fragments of interest, for example by using the Agilent SureSelect system (Borno et al., 2012).
A popular, cost-efficient and scalable technology for profiling DNA methylation on a “genome-scale” is the Illumina 450k microarray. The platform can be thought of as genotyping BS-treated DNA to reveal the relative proportion of methylated and unmethylated alleles (Pidsley et al., 2013). For every CpG site, measurements are either made with two separate physical beads (Type I) or through a single bead across two fluorescence channels (Type II); properties of these probe types are vastly different and require careful normalization (Maksimovic et al., 2012; Aryee et al., 2014).
With the decreasing costs of single-base resolution DNA methylation data, enrichment techniques that capture methylated DNA fragments appear to have gone somewhat out of favor. Methylated DNA immunoprecipitation (MeDIP) or methyl-binding domain enrichments share many features of chromatin immunoprecipitation experiments. However, they are plagued by enrichment biases associated with CpG density, some of which can be fixed in silico (Down et al., 2008; Pelizzola et al., 2008; Riebler et al., 2014). Recently, a combination approach of MeDIP-seq and methylation-sensitive restriction enzyme sequencing (MRE-seq) has become available, promising to quickly compare methylomes at lower cost (Zhang et al., 2013).
Table 2 summarizes the methods reviewed and gives brief details on some of the important features: (i) data type; (ii) ability to define regions; (iii) support for covariate adjustment; (iv) statistical tests used.
Ultimately, the same experimental design concepts that apply broadly to any scientific investigation, such as sampling, randomization, and blocking, are assumed. Excepting single-cell DNA methylation studies (e.g., Guo et al., 2013), it is crucial to remember that every experimental unit represents a population of cells. This implies that a consensus methylation estimate of 50% could mean 50% of the alleles are methylated in all cells (e.g., allele-specific methylation) or 50% of the cells are fully methylated (e.g., mixtures of cell types), or any combination thereof. Only BS-seq data can properly decompose this information, and at the same time infer allele-specific patterns, using the methylation status from individual DNA fragments (Fang et al., 2012; Statham et al., 2012; Song et al., 2013). But, there are limits: since small fragments are observed, it remains challenging to relate the allelic methylation status at one loci to another genomically distant loci without additional haplotype information (Kuleshov et al., 2014).
In contrast to genome sequencing studies, collecting relevant populations of cell types is important for epigenome profiling projects. Many population-scale profiling studies may consider using readily accessible bodily fluids such as blood, which represents a rich milieu of cell types that may vary in composition across the experimental units being studied. If the cell types and cell surface markers of interest are known, it may be beneficial to first sort cells into subpopulations and profile each individually (Houseman et al., 2012). Doing so will give a more focused interrogation of methylation and improved signal over noise. However, there are many situations where pre-sorting is not possible. Importantly, profiling mixtures of cell types and looking for changes in DNA methylation can be misleading when the cell composition is associated with an external factor, such as age of the patient (Jaffe and Irizarry, 2014). However, there are now various emerging computational techniques to deconvolute the cell composition signals in silico (see Section 6).
Another design consideration for BS-seq experiments is whether money is better allocated toward deeper sequencing or additional replicates. Because of the local smoothing frameworks available for methylation measurements (e.g., BSmooth, Hansen et al., 2012b), it is considered better to sequence additional replicates than to gather deep information on fewer samples.
We first focus on the methodology for discovering individual differentially methylated CpG sites for single-base resolution assays. BS-seq data can be summarized as counts of methylated and unmethylated reads at any given site. Many early BS-seq studies profiled cells without collecting replicates and used Fisher's exact test (FET) to discern DM (Lister et al., 2009). While this strategy may be sufficient for comparing cell lines, we stress that the use of FET should be generally avoided; most systems have inherent biological variation and FET does not account for it. For example, in a two-condition comparison, FET requires the data to be condensed to counts for each condition, completely ignoring the within-condition variability. This will underestimate variability and overstate differences, leading to a high false positive rate. Likewise, using the binomial distribution, e.g., within a logistic regression framework (e.g., methylKit; Akalin et al., 2012), does not facilitate estimation of biological variability unless an overdispersion term is used. While BSmooth uses a “signal-to-noise” statistic to quantify DM evidence at individual CpG sites, it is not used directly for inference of differential sites (more details in Section 5).
The most natural statistical model for replicated BS-seq DNA methylation measurements is beta-binomial. Conditional on the methylation proportion at a particular site, the observations are binomial distributed, while the methylation proportion itself can vary across experimental units (e.g., patients), according to a beta distribution. It is therefore no surprise that beta-binomial assumptions are made in several recent packages, such as BiSeq (Hebestreit et al., 2013), MOABS (Sun et al., 2014), DSS (Feng et al., 2014), RADMeth (Dolzhenko and Smith, 2014), and methylSig (Park et al., 2014). Similarly, empirical Bayes (EB) methods fit naturally for modeling and inference across many types of genomic data, including DNA methylation assays. MOABS and DSS both implement hierarchical models and use the full dataset to estimate the hyperparameters of the beta distribution; RADMeth, BiSeq and methylSig use standard maximum likelihood without any moderation. While BiSeq and RADMeth do not moderate parameter estimates, they provide facilities for complex designs through design matrices, which MOABS, DSS and methylSig do not currently offer. Inference for parameters of interest (i.e., changes in methylation) are conducted using standard techniques, such as Wald tests (DSS, BiSeq) and likelihood ratio tests (RADMeth, methylSig). Notably, MOABS introduces a new metric, called credible methylation difference, which is a conservative estimate of the true methylation difference, calibrated by the statistical evidence available.
DNA methylation arrays, such as Illumina's 27k or 450k array, give fluorescence intensities that quantify relative abundance of methylated and unmethylated loci, in contrast to the count-based modeling assumptions for BS-seq based profiling. In particular, the data used for downstream analyses can be either (i) log-ratios of methylated to unmethylated intensities, or (ii) the beta-value, which gives the ratio of the methylated to the total of methylated and unmethylated intensities. Previous comparisons suggest that statistical inferences based on log-ratios are preferred (Du et al., 2010), perhaps not surprisingly since they can rely on earlier successful moderated statistical testing strategies (e.g., limma; Smyth, 2004). Much of the recent effort for the 450k array has been dedicated to normalization and filtering (e.g., Price et al., 2013; Aryee et al., 2014) and various options for inferring DM sites from 27k/450k array data have been proposed. To test for DM, IMA proposes Wilcoxon rank-sum tests on beta-values (Wang et al., 2012). COHCAP operates either on methylation array data or BS-seq data, using beta-values or methylation proportions as input; they offer FET (see comment above), t-tests and ANOVA analyses (without moderation), depending on the study design (Warden et al., 2013). Ultimately, we speculate that moderated t/F-statistics on the normalized log-ratios of intensities should perform well.
Although there are occasions when researchers are interested in relating single CpG sites to a phenotype (e.g., Weaver et al., 2004), often differentially methylated regions (DMRs) are a more predictive feature. Another advantage is that while differences at any individual site may be small, if they are persistent across a region, statistical power to detect them may be greater. Methods that operate on predefined regions must be distinguished from those that define regions of DM. The latter is considerably more difficult because ensuring control of the false discovery rate (FDR) at the region-level is non-trivial; in particular, FDR control at the site-level does not give a direct way to region-level control when the region itself is also to be defined (Lun and Smyth, 2014).
Therefore, the most straightforward approach is to use predefined regions, such as CpG islands, CpG shores, UTRs, and so on; statistical testing can be conducted fairly routinely at a region-level. Many of the packages mentioned above, such as IMA, COHCAP, DMAP, methylSig, and methylKit, do exactly this. A special case is DMAP, which can operate on fragments (using the sampled MspI-digested fragments as the region of interest) or according to predefined regions (Stockwell et al., 2014).
There are now many approaches for defining DMRs. For example, Bumphunter can be applied quite generally across data types (Jaffe et al., 2012), perhaps after transformation in the case of count data. Notably, it also integrates a surrogate variable analysis (Leek and Storey, 2007) to simultaneously account for potential batch effects while permutation tests are used to assign FDR at the region-level; users should set a smoothing window size and a threshold on the percentile of the smoothed effect sizes (or t-statistics) (Jaffe et al., 2012). Similarly, BSmooth searches for runs of smoothed absolute t-like scores beyond a threshold, however, does not suggest a permutation strategy to control region-level FDR. From the same authors, minfi wraps bumphunting into the suite of methods available for Illumina 450k arrays; in addition, they provide a module for block finding, which is essentially bumphunting with a much greater window size (e.g., 250kbp) (Aryee et al., 2014). BiSeq proposes, via a Wald test statistic from the beta-binomial regression fit, a hierarchical testing strategy that first considers target regions and controls error using a cluster-wise weighted FDR strategy (Hebestreit et al., 2013); secondly, the differential clusters are trimmed using a second stage of testing, analogous to methods used for spatial signals (Benjamini and Heller, 2007). A clustering method, A-clust, proposes first to cluster CpG sites according to correlation in methylation signal across samples; within the clusters, associations can be modeled with correlated error and fit using a generalized estimating equation framework (Sofer et al., 2013). The DSS authors simply set some thresholds on the P-values, number of CpG sites and length of regions, but they do not pursue FDR control (Feng et al., 2014). The MOABS authors suggest grouping DM sites into DMRs using a hidden Markov model or alternatively testing of predefined regions, but no specific details are given. RADMeth proposes a transformation of P-values (from a likelihood ratio test) into a weighted Z-test that builds in the correlation of neighboring probes (Kechris et al., 2010; Dolzhenko and Smith, 2014); the same adjustment, also known as the Stouffer-Liptak test, is used in the eDMR tool (Li et al., 2013). Also used in the context of DMR detection for combining spatially correlated P-values, but applicable more generally, is a tool called comb-p (Pedersen et al., 2012).
Enrichment assays, such as MeDIP-seq, are by their very nature of capturing fragments, only capable of finding regions of DM. Packages for considering enrichment data, including MEDIPS (Chavez et al., 2010; Lienhard et al., 2014), ABCD-DNA (Robinson et al., 2012) and DiffBind (Ross-Innes et al., 2012), compare relative abundance of fragment counts by repackaging RNA sequencing statistical frameworks. In a related assay, the M&M algorithm models normalized bin-wise methylated counts (MeDIP-seq) and unmethylated counts (MRE-seq) as jointly Poisson distributed with a shared parameter (Zhang et al., 2013). Analogous to the FET, the Poisson model does not account for biological variability (Zhang et al., 2013).
Researchers need to carefully design studies that associate phenotypes with DNA methylation. Some aspects, such as cell type composition, cannot be readily controlled by design; patients and therefore individual DNA samples simply differ in their cell type composition. A recent report has highlighted that many of the DNA methylation markers that have been associated with age are actually driven by age-related changes in cell composition (Jaffe and Irizarry, 2014). Whole blood is a mixture of several cell types; using an independent dataset of methylation profiles of the dominant cell types (Monocytes, CD4+ and CD8+ T cells, Granulocytes, B cells, natural killer cells) from flow sorting, patient profiles were deconvoluted using a reimplementation of the Houseman algorithm (Houseman et al., 2012; Jaffe and Irizarry, 2014). From the methylation profiles of pure cell populations, cell-type-specific markers are selected and then used to “calibrate” a regression model that associates methylation observations to a response of interest (Carroll, 2006; Houseman et al., 2012). Of course, this approach requires advance knowledge of the dominant cell types and methylation profiles for them, preferably across multiple replicates to seed the deconvolution algorithm with appropriate methylation markers. However, a recent study has highlighted that advanced statistical modeling can correct for cell type composition without the need for pure-cell profiles; starting from uncorrected standard model fits, the method regresses principal components within a linear mixed model until control for the inflation of the test statistics (e.g., relative to a uniform distribution of P-values) is achieved (Zou et al., 2014).
In this review, we briefly explored the various methodologies available for deciphering DMRs across the main data types and highlighted some of the common themes and current challenges. The tradeoffs made by method developers are apparent. In fact, it's a lot to ask of a single statistical framework to do everything: moderate parameter estimates using genome-wide information or accurately and robustly smooth local estimates, accommodate low coverage data, account for batch effects and cell type composition, allow complex experimental designs and accurately control FDR at the site- and/or region-level. In addition, identification of DMRs is only the discovery step; validating these detections, perhaps by associating them with other biological outcomes in silico requires additional frameworks, some of which have already been integrated alongside the packages reviewed here.
On the statistical and computational side, the field is moving fast and several advanced methods have been proposed. One of the next challenges will be to comprehensively compare method performance, in terms of statistical power, ability to control FDR, robustness, and scalability to large datasets and large studies. Representative simulation frameworks will be fundamental for this task. Given the large number of methods available, this will already be a large undertaking. To avoid bias, these comparisons should be done either independently of the method development process, or collectively with all method developers. Advanced deconvolution algorithms and batch effect removal strategies are, at present, targeted at 450k array data. The development and vetting of similar techniques that can be readily applied to count data, such as BS-seq data, are well underway (Leek, 2014; Risso et al., 2014).
Mark D. Robinson drafted the text with contributions from all co-authors: Abdullah Kahraman, Charity W. Law, Helen Lindsay, Malgorzata Nowicka, Lukas M. Weber and Xiaobei Zhou. All authors read and approved the final manuscript.
Mark D. Robinson acknowledges financial support from SNSF (grant 143883) and from the European Commission's RADIANT project (Grant Number: 305626).
The authors declare that the research was conducted in the absence of any commercial or financial relationships that could be construed as a potential conflict of interest.
We would like to thank followers on Twitter (@timtriche, @PeteHaitch, @brent_p) and especially Peter Hickey for suggesting additional citations and/or carefully reading an earlier draft.
Akalin, A., Kormaksson, M., Li, S., Garrett-Bakelman, F. E., Figueroa, M. E., Melnick, A., et al. (2012). methylKit: a comprehensive R package for the analysis of genome-wide DNA methylation profiles. Genome Biol. 13, R87. doi: 10.1186/gb-2012-13-10-r87
Aryee, M. J., Jaffe, A. E., Corrada-Bravo, H., Ladd-Acosta, C., Feinberg, A. P., Hansen, K. D., et al. (2014). Minfi: a flexible and comprehensive Bioconductor package for the analysis of Infinium DNA Methylation microarrays. Bioinformatics 30, 1363–1369. doi: 10.1093/bioinformatics/btu049
Bae, J. B. (2013). Perspectives of international human epigenome consortium. Genom. Inform. 11, 7–14. doi: 10.5808/GI.2013.11.1.7
Benjamini, Y., and Heller, R. (2007). False discovery rates for spatial signals. J. Am. Stat. Assoc. 102, 1272–1281. doi: 10.1198/016214507000000941
Berger, S. L., Kouzarides, T., Shiekhattar, R., and Shilatifard, A. (2009). An operational definition of epigenetics. Genes Dev. 23, 781–783. doi: 10.1101/gad.1787609
Bernstein, B. E., Stamatoyannopoulos, J. A., Costello, J. F., Ren, B., Milosavljevic, A., Meissner, A., et al. (2010). The NIH roadmap epigenomics mapping consortium. Nat. Biotechnol. 28, 1045–1048. doi: 10.1038/nbt1010-1045
Bird, A. (2002). DNA methylation patterns and epigenetic memory. Genes Dev. 16, 6–21. doi: 10.1101/gad.947102
Bock, C. (2012). Analysing and interpreting DNA methylation data. Nat. Rev. Genet. 13, 705–719. doi: 10.1038/nrg3273
Booth, M. J., Branco, M. R., Ficz, G., Oxley, D., Krueger, F., Reik, W., et al. (2012). Quantitative sequencing of 5-Methylcytosine and 5-Hydroxymethylcytosine at single-base resolution. Science 336, 934–937. doi: 10.1126/science.1220671
Borno, S. T., Fischer, A., Kerick, M., Falth, M., Laible, M., Brase, J. C., et al. (2012). Genome-wide DNA Methylation Events in TMPRSS2-ERG fusion-negative prostate cancers implicate an EZH2-Dependent mechanism with miR-26a Hypermethylation. Cancer Discov. 2, 1024–1035. doi: 10.1158/2159-8290.CD-12-0041
Carroll, R. J. (2006). Measurement Error in Nonlinear Models: A Modern Perspective. Boca Raton, FL: Chapman & Hall/CRC. doi: 10.1201/9781420010138
Chavez, L., Jozefczuk, J., Grimm, C., Dietrich, J., Timmermann, B., Lehrach, H., et al. (2010). Computational analysis of genome-wide DNA methylation during the differentiation of human embryonic stem cells along the endodermal lineage. Genome Res. 20, 1441–1450. doi: 10.1101/gr.110114.110
Chin, L., Andersen, J. N., and Futreal, P. A. (2011). Cancer genomics: from discovery science to personalized medicine. Nat. Med. 17, 297–303. doi: 10.1038/nm.2323
Clark, S. J., Statham, A., Stirzaker, C., Molloy, P. L., and Frommer, M. (2006). DNA methylation: bisulphite modification and analysis. Nat. Protoc. 1, 2353–2364. doi: 10.1038/nprot.2006.324
Dolzhenko, E., and Smith, A. D. (2014). Using beta-binomial regression for high-precision differential methylation analysis in multifactor whole-genome bisulfite sequencing experiments. BMC Bioinformatics 15:215. doi: 10.1186/1471-2105-15-215
Down, T. A., Rakyan, V. K., Turner, D. J., Flicek, P., Li, H., Kulesha, E., et al. (2008). A Bayesian deconvolution strategy for immunoprecipitation-based DNA methylome analysis. Nat. Biotechnol. 26, 779–785. doi: 10.1038/nbt1414
Du, P., Zhang, X., Huang, C.-C., Jafari, N., Kibbe, W. A., Hou, L., et al. (2010). Comparison of Beta-value and M-value methods for quantifying methylation levels by microarray analysis. BMC Bioinformatics 11:587. doi: 10.1186/1471-2105-11-587
Fang, F., Hodges, E., Molaro, A., Dean, M., Hannon, G. J., and Smith, A. D. (2012). Genomic landscape of human allele-specific DNA methylation. Proc. Natl. Acad. Sci. U.S.A. 109, 7332–7337. doi: 10.1073/pnas.1201310109
Feng, H., Conneely, K. N., and Wu, H. (2014). A Bayesian hierarchical model to detect differentially methylated loci from single nucleotide resolution sequencing data. Nucleic Acids Res. 42, e69. doi: 10.1093/nar/gku154
Flusberg, B. A., Webster, D. R., Lee, J. H., Travers, K. J., Olivares, E. C., Clark, T. A., et al. (2010). Direct detection of DNA methylation during single-molecule, real-time sequencing. Nat. Methods 7, 461–465. doi: 10.1038/nmeth.1459
Guo, H., Zhu, P., Wu, X., Li, X., Wen, L., and Tang, F. (2013). Single-cell methylome landscapes of mouse embryonic stem cells and early embryos analyzed using reduced representation bisulfite sequencing. Genome Res. 23, 2126–2135. doi: 10.1101/gr.161679.113
Hansen, K. D., Irizarry, R. A., and Wu, Z. (2012a). Removing technical variability in RNA-seq data using conditional quantile normalization. Biostatistics 13, 204–216. doi: 10.1093/biostatistics/kxr054
Hansen, K. D., Langmead, B., and Irizarry, R. A. (2012b). BSmooth: from whole genome bisulfite sequencing reads to differentially methylated regions. Genome Biol. 13:R83. doi: 10.1186/gb-2012-13-10-r83
Hebestreit, K., Dugas, M., and Klein, H. U. (2013). Detection of significantly differentially methylated regions in targeted bisulfite sequencing data. Bioinformatics 29, 1647–1653. doi: 10.1093/bioinformatics/btt263
Houseman, E., Accomando, W., Koestler, D., Christensen, B., Marsit, C., Nelson, H., et al. (2012). DNA methylation arrays as surrogate measures of cell mixture distribution. BMC Bioinformatics 13:86. doi: 10.1186/1471-2105-13-86
Huang, Y., Pastor, W. A., Shen, Y., Tahiliani, M., Liu, D. R., and Rao, A. (2010). The behaviour of 5-hydroxymethylcytosine in bisulfite sequencing. PLoS ONE 5:e8888. doi: 10.1371/journal.pone.0008888
Hudson, T. J., Anderson, W., Artez, A., Barker, A. D., Bell, C., Bernabé, R. R., et al. (2010). International network of cancer genome projects. Nature 464, 993–998. doi: 10.1038/nature08987
Jaffe, A. E., and Irizarry, R. A. (2014). Accounting for cellular heterogeneity is critical in epigenome-wide association studies. Genome Biol. 15, R31. doi: 10.1186/gb-2014-15-2-r31
Jaffe, A. E., Murakami, P., Lee, H., Leek, J. T., Fallin, M. D., Feinberg, A. P., et al. (2012). Bump hunting to identify differentially methylated regions in epigenetic epidemiology studies. Int. J. Epidemiol. 41, 200–209. doi: 10.1093/ije/dyr238
Kechris, K. J., Biehs, B., and Kornberg, T. B. (2010). Generalizing moving averages for tiling arrays using combined p-value statistics. Stat. Appl. Genet. Mol. Biol. 9:29. doi: 10.2202/1544-6115.1434
Kuleshov, V., Xie, D., Chen, R., Pushkarev, D., Ma, Z., Blauwkamp, T., et al. (2014). Whole-genome haplotyping using long reads and statistical methods. Nat. Biotechnol. 32, 261–266. doi: 10.1038/nbt.2833
Laird, P. W. (2010). Principles and challenges of genome-wide DNA methylation analysis. Nat. Rev. Genet. 11, 191–203. doi: 10.1038/nrg2732
Leek, J. (2014). svaseq: Removing Batch Effects and Other Unwanted Noise from Sequencing Data. bioRxiv. Available online at: http://dx.doi.org/10.1101/006585
Leek, J. T., and Storey, J. D. (2007). Capturing heterogeneity in gene expression studies by surrogate variable analysis. PLoS Genet. 3:e161. doi: 10.1371/journal.pgen.0030161
Li, S., Garrett-Bakelman, F. E., Akalin, A., Zumbo, P., Levine, R., To, B. L., et al. (2013). An optimized algorithm for detecting and annotating regional differential methylation. BMC Bioinformatics 14(Suppl. 5):S10. doi: 10.1186/1471-2105-14-S5-S10
Lienhard, M., Grimm, C., Morkel, M., Herwig, R., and Chavez, L. (2014). MEDIPS: Genome-wide differential coverage analysis of sequencing data derived from DNA enrichment experiments. Bioinformatics 30, 284–286. doi: 10.1093/bioinformatics/btt650
Lister, R., Pelizzola, M., Dowen, R. H., Hawkins, R. D., Hon, G., Tonti-Filippini, J., et al. (2009). Human DNA methylomes at base resolution show widespread epigenomic differences. Nature 462, 315–322. doi: 10.1038/nature08514
Lun, A. T., and Smyth, G. K. (2014). De novo detection of differentially bound regions for ChIP-seq data using peaks and windows: controlling error rates correctly. Nucleic Acids Res. 42, e95. doi: 10.1093/nar/gku351
Maksimovic, J., Gordon, L., Oshlack, A., and Makismovic, J. (2012). SWAN: Subset-quantile within array normalization for illumina infinium HumanMethylation450 BeadChips. Genome Biol. 13, R44. doi: 10.1186/gb-2012-13-6-r44
Martens, J. H. H., and Stunnenberg, H. G. (2013). BLUEPRINT: mapping human blood cell epigenomes. Haematologica 98, 1487–1489. doi: 10.3324/haematol.2013.094243
Moazed, D. (2011). Mechanisms for the inheritance of chromatin states. Cell 146, 510–518. doi: 10.1016/j.cell.2011.07.013
Park, Y., Figueroa, M. E., Rozek, L. S., and Sartor, M. A. (2014). MethylSig: a whole genome DNA methylation analysis pipeline. Bioinformatics 30, 2414–2422. doi: 10.1093/bioinformatics/btu339
Pedersen, B. S., Schwartz, D. A., Yang, I. V., and Kechris, K. J. (2012). Comb-p: Software for combining, analyzing, grouping and correcting spatially correlated P-values. Bioinformatics 28, 2986–2988. doi: 10.1093/bioinformatics/bts545
Pelizzola, M., Koga, Y., Urban, A. E., Krauthammer, M., Weissman, S., Halaban, R., et al. (2008). MEDME: an experimental and analytical methodology for the estimation of DNA methylation levels based on microarray derived MeDIP-enrichment. Genome Res. 18, 1652–1659. doi: 10.1101/gr.080721.108
Pidsley, R., Y Wong, C. C., Volta, M., Lunnon, K., Mill, J., Schalkwyk, L. C., et al. (2013). A data-driven approach to preprocessing Illumina 450K methylation array data. BMC Genomics 14:293. doi: 10.1186/1471-2164-14-293
Price, M. E., Cotton, A. M., Lam, L. L., Farré, P., Emberly, E., Brown, C. J., et al. (2013). Additional annotation enhances potential for biologically-relevant analysis of the Illumina Infinium HumanMethylation450 BeadChip array. Epigenetics Chromatin 6, 4. doi: 10.1186/1756-8935-6-4
Riebler, A., Menigatti, M., Song, J. Z., Statham, A. L., Stirzaker, C., Mahmud, N., et al. (2014). BayMeth: improved DNA methylation quantification for affinity capture sequencing data using a flexible Bayesian approach. Genome Biol. 15, R35. doi: 10.1186/gb-2014-15-2-r35
Risso, D., Ngai, J., Speed, T. P., and Sandrine, D. (2014). Normalization of RNA-seq data using factor analysis of control genes or samples. Nat. Biotechnol. 32, 896–902. doi: 10.1038/nbt.2931.
Robinson, M. D., Statham, A. L., Speed, T. P., and Clark, S. J. (2010). Protocol matters : which methylome are you actually studying? Epigenomics 2, 587–598. doi: 10.2217/epi.10.36
Robinson, M. D., Strbenac, D., Stirzaker, C., Statham, A. L., Song, J., Speed, T. P., et al. (2012). Copy-number-aware differential analysis of quantitative DNA sequencing data. Genome Res. 22, 2489–2496. doi: 10.1101/gr.139055.112
Ross-Innes, C. S., Stark, R., Teschendorff, A. E., Holmes, K. A., Ali, H. R., Dunning, M. J., et al. (2012). Differential oestrogen receptor binding is associated with clinical outcome in breast cancer. Nature 481, 389–393. doi: 10.1038/nature10730
Satterlee, J. S., Schübeler, D., and Ng, H.-H. (2010). Tackling the epigenome: challenges and opportunities for collaboration. Nat. Biotechnol. 28, 1039–1044. doi: 10.1038/nbt1010-1039
Smyth, G. K. (2004). Linear models and empirical Bayes methods for assessing differential expression in microarray experiments. Stat. Appl. Genet. Mol. Biol. 3:3. doi: 10.2202/1544-6115.1027
Sofer, T., Schifano, E. D., Hoppin, J. A., Hou, L., and Baccarelli, A. A. (2013). A-clustering: a novel method for the detection of co-regulated methylation regions, and regions associated with exposure. Bioinformatics 29, 2884–2891. doi: 10.1093/bioinformatics/btt498
Song, Q., Decato, B., Hong, E. E., Zhou, M., Fang, F., Qu, J., et al. (2013). A reference methylome database and analysis pipeline to facilitate integrative and comparative epigenomics. PLoS ONE 8:e81148. doi: 10.1371/journal.pone.0081148
Statham, A. L., Robinson, M. D., Song, J. Z., Coolen, M. W., Stirzaker, C., and Clark, S. J. (2012). Bisulfite sequencing of chromatin immunoprecipitated DNA (BisChIP-seq) directly informs methylation status of histone-modified DNA. Genome Res. 22, 1120–1127. doi: 10.1101/gr.132076.111
Stockwell, P. A., Chatterjee, A., Rodger, E. J., and Morison, I. M. (2014). DMAP: differential methylation analysis package for RRBS and WGBS data. Bioinformatics 30, 1814–1822. doi: 10.1093/bioinformatics/btu126
Sun, D., Xi, Y., Rodriguez, B., Park, H. J., Tong, P., Meong, M., et al. (2014). MOABS: model based analysis of bisulfite sequencing data. Genome Biol. 15, R38. doi: 10.1186/gb-2014-15-2-r38
Szyf, M. (2012). DNA methylation signatures for breast cancer classification and prognosis. Genome Med. 4:26. doi: 10.1186/gm325
Varley, K. E., Gertz, J., Bowling, K. M., Parker, S. L., Reddy, T. E., Pauli-Behn, F., et al. (2013). Dynamic DNA methylation across diverse human cell lines and tissues. Genome Res. 23, 555–567. doi: 10.1101/gr.147942.112
Wang, D., Yan, L., Hu, Q., Sucheston, L. E., Higgins, M. J., Ambrosone, C. B., et al. (2012). IMA: an R package for high-throughput analysis of Illumina's 450K Infinium methylation data. Bioinformatics 28, 729–730. doi: 10.1093/bioinformatics/bts013
Warden, C. D., Lee, H., Tompkins, J. D., Li, X., Wang, C., Riggs, A. D., et al. (2013). COHCAP: an integrative genomic pipeline for single-nucleotide resolution DNA methylation analysis. Nucleic Acids Res. 41:e117. doi: 10.1093/nar/gkt242
Weaver, I. C. G., Cervoni, N., Champagne, F. A., D'Alessio, A. C., Sharma, S., Seckl, J. R., et al. (2004). Epigenetic programming by maternal behavior. Nat. Neurosci. 7, 847–854. doi: 10.1038/nn1276
Zhang, B., Zhou, Y., Lin, N., Lowdon, R. F., Hong, C., Nagarajan, R. P., et al. (2013). Functional DNA methylation differences between tissues, cell types, and across individuals discovered using the M&M algorithm. Genome Res. 23, 1522–1540. doi: 10.1101/gr.156539.113
Ziller, M. J., Gu, H., Müller, F., Donaghey, J., Tsai, L. T.-Y., Kohlbacher, O., et al. (2013). Charting a dynamic DNA methylation landscape of the human genome. Nature 500, 477–481. doi: 10.1038/nature12433
Keywords: differential methylation, bisulphite sequencing, cell type composition, beta-binomial
Citation: Robinson MD, Kahraman A, Law CW, Lindsay H, Nowicka M, Weber LM and Zhou X (2014) Statistical methods for detecting differentially methylated loci and regions. Front. Genet. 5:324. doi: 10.3389/fgene.2014.00324
Received: 14 July 2014; Accepted: 29 August 2014;
Published online: 16 September 2014.
Edited by:
Shihua Zhang, Chinese Academy of Science, ChinaReviewed by:
Helder I. Nakaya, Emory University, USACopyright © 2014 Robinson, Kahraman, Law, Lindsay, Nowicka, Weber and Zhou. This is an open-access article distributed under the terms of the Creative Commons Attribution License (CC BY). The use, distribution or reproduction in other forums is permitted, provided the original author(s) or licensor are credited and that the original publication in this journal is cited, in accordance with accepted academic practice. No use, distribution or reproduction is permitted which does not comply with these terms.
*Correspondence: Mark D. Robinson, Institute of Molecular Life Sciences, University of Zurich, Winterthurerstrasse 190, CH-8057 Zurich, Switzerland e-mail:bWFyay5yb2JpbnNvbkBpbWxzLnV6aC5jaA==
Disclaimer: All claims expressed in this article are solely those of the authors and do not necessarily represent those of their affiliated organizations, or those of the publisher, the editors and the reviewers. Any product that may be evaluated in this article or claim that may be made by its manufacturer is not guaranteed or endorsed by the publisher.
Research integrity at Frontiers
Learn more about the work of our research integrity team to safeguard the quality of each article we publish.