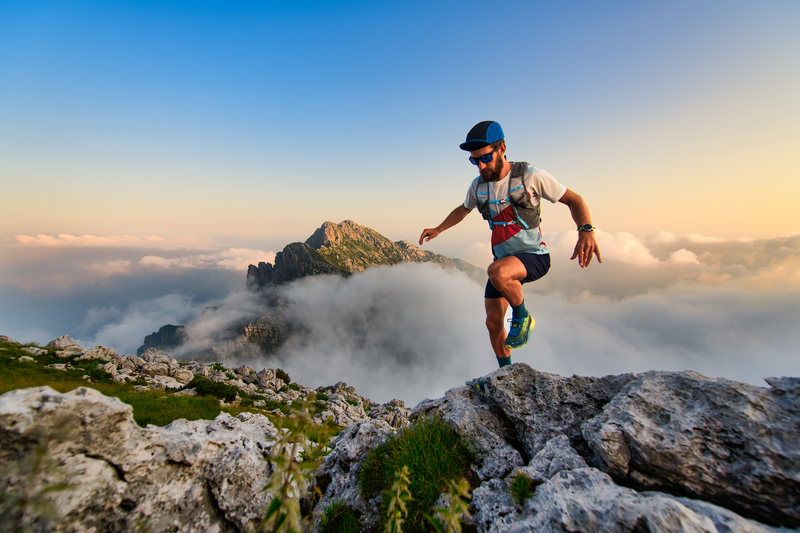
94% of researchers rate our articles as excellent or good
Learn more about the work of our research integrity team to safeguard the quality of each article we publish.
Find out more
ORIGINAL RESEARCH article
Front. Genet. , 04 June 2014
Sec. Pharmacogenetics and Pharmacogenomics
Volume 5 - 2014 | https://doi.org/10.3389/fgene.2014.00167
Introduction: Pharmacogenomics research has concentrated on variation in genes coding for drug metabolizing enzymes, transporters and nuclear receptors. However, variation affecting microRNA could also play a role in drug response. This project set out to investigate potential microRNA target sites in 11 genes and the extent of variation in the 3′-UTR of six selected genes; CYP1A2, CYP2B6, CYP2D6, CYP3A4, NR1I2, and UGT2B7.
Methods: Fifteen microRNA target prediction algorithms were used to identify microRNAs predicted to regulate 11 genes. The 3′-UTR of the 6 genes which topped the list of potential microRNA targets was sequenced in 30 black South Africans. In addition, genetic variants within these genes were investigated for interference with mRNA-microRNA interactions. Potential effects of observed variants were determined using in silico prediction tools.
Results: The 11 genes coding for DMEs, transporters and nuclear receptors were predicted to be targets of microRNAs with CYP2B6, NR1I2 (PXR), CYP3A4, and CYP1A2, interacting with the most microRNAs. The majority of identified genetic variants were predicted to interfere with microRNA regulation. For example, the variant, rs1054190C in NR1I2 was predicted to result in the presence of a binding site for the microRNA miR-1250-5p, while the variant rs1054191G was predicted to result in the absence of a recognition site for miR-371b-3p, miR-4258 and miR-4707-3p. Fifteen of the seventeen, novel variants occurred within microRNA target sequences.
Conclusion: The 3′-UTR harbors variation that is likely to influence regulation of specific genes by microRNA. In silico prediction followed by functional validation could aid in decoding the contribution of variation in the 3′-UTR, to some unexplained heritability that affects drug response. Understanding the specific role of each microRNA may lead to identification of markers for targeted therapy and therefore improve personalized drug treatment.
Drug metabolizing enzymes (DMEs), drug transporters and nuclear receptors, play an important role in response outcomes to therapeutically prescribed drugs. For example, the analgesic drug codeine is dependent on CYP2D6 metabolism to the more active morphine metabolite (Armstrong and Cozza, 2003). However, CYP2D6 exhibits extensive genetic polymorphism resulting in individuals being broadly classified as poor metabolizers (PMs), extensive metabolizers (EMs), and ultrarapid metabolizers, phenotypes that vary across world populations. Another example is that of the disposition of efavirenz and nevirapine used in treatment of HIV/AIDS, both of which are metabolized by CYP2B6. The genetic variants CYP2B6 c.516T, and c.983C have been linked to high efavirenz or nevirapine plasma levels and the development of central nervous system side effects (Marzolini et al., 2001; Ciccacci et al., 2013; Swart et al., 2013; Vardhanabhuti et al., 2013). In general, many pharmacogenetics studies have concentrated on the effects of genetic variation in exons, introns, and promoters of pharmacogenetically relevant genes (Batt et al., 1994; Commandeur et al., 1995; Tukey and Strassburg, 2000; McCarver and Hines, 2002; Hayes et al., 2005; Petzinger and Geyer, 2006; Alnouti and Klaassen, 2008; Guengerich, 2008). However, it is now apparent that genetic variation in the 3′-UTR, which is a target for microRNA regulation, could also play a role in pharmacogenomics (Xu et al., 2005).
Thus, in order to get a complete picture of the pharmacogenetic determinants of drug response, genetic variation in the 3′-UTR region of DMEs, drug transporters and nuclear receptors, should also be evaluated as this is the region targeted by microRNAs. MicroRNAs are evolutionary conserved (Lagos-Quintana et al., 2001) and predicted to regulate more than 30% of protein coding genes in the human genome (Yu, 2009; Breving and Esquela-Kerscher, 2010; Shomron, 2010; Rodrigues et al., 2011) which include genes with pharmacogenomics relevance. MicroRNAs regulate genes post-transcriptionally by binding to the 3′-UTR of mRNA target sequences and facilitating degradation or translational repression (Baek et al., 2008; Mishra and Bertino, 2009; Marin and Vanicek, 2012). MicroRNAs have been implicated in regulation of the following pharmacogenomically relevant genes; CYP1B1 (Tsuchiya et al., 2006), CYP2E1 (Mohri et al., 2010), CYP3A4 (Pan et al., 2009), and PXR (Takagi et al., 2008). Pan et al. (2009) showed that microRNA miR-27b regulates expression of CYP3A4 directly, while miR-148a regulates CYP3A4 indirectly through regulation of PXR (Takagi et al., 2008). SULT1A1 has also been shown to be regulated directly by miR-631 in an allele-specific manner (Yu et al., 2010). Genes coding for CYP1A2 and CYP2B6, are predicted to be regulated by microRNAs based solely on the length of their 3′-UTRs, but these predictions are yet to be confirmed through functional studies (Ingelman-Sundberg et al., 2007; Ramamoorthy and Skaar, 2011; Singh et al., 2011; Mishra, 2012).
Genetic variation that occurs either in the sequence coding for microRNAs or in the sequence targeted by microRNA on the mRNA, is referred to as miRSNPs. MiRSNPs potentially alter microRNA function or interfere with microRNA-mRNA interaction (Mishra et al., 2008; Liu et al., 2012). MiRSNPs located in the mRNA can result in the presence or absence of microRNA target sites and to date only a few pharmacogenomics studies have included genetic variation in the 3′-UTR of pharmacogenomically-relevant genes as possible contributors to interindividual variability observed in drug response. In order to evaluate the role of microRNA in drug response, prediction of likely microRNA target sequences should be followed by functional validation.
There are several algorithms to predict microRNA targets and these algorithms are designed based on a number of different criteria (Witkos et al., 2011). To increase the precision and power of microRNA target prediction as well as reduce false positives, the use of a combination of prediction algorithms is advised (Mishra and Bertino, 2009; Marin and Vanicek, 2012). Recently, a study by Ramamoorthy and Skaar (2011) used six algorithms to predict microRNAs that potentially target 12 major drug metabolizing CYPs. With more than 10 algorithms available, the challenge is which combinations or how many algorithms should be used to predict microRNA targets. The use of a combination of microRNA target prediction, using different features including; sequence complementarity, target site accessibility, binding energy, and conservation of target sites, in a complementary manner may improve sensitivity, specificity, and consistency of microRNA target prediction (Zhang and Verbeek, 2010). In this study, we set out to identify microRNAs predicted to regulate genes associated with drug metabolism and disposition using 15 in silico prediction algorithms that are available online to evaluate the spectrum of microRNA target sequences in the 3′-UTR of 11 genes. Variation in the 3′-UTR of six genes was evaluated by sequencing resulting in the identification of microRNA SNPs (miRSNPs) which were predicted to potentially affect microRNA binding.
Fifteen different web-based bioinformatics algorithms (DIANA-microT-CDS, DIANA-microT, MicroCosm, miR2Gene, miRanda-MirSVR, miRBRIDGE, miRSystem, miRTar, PACCMIT, PicTar, PITA, RegRNA, RNA22, TargetScan, and TargetSpy) that were freely-available online were used to predict microRNA targeting (John et al., 2004; Krek et al., 2005; Griffiths-Jones et al., 2006; Huang et al., 2006; Miranda et al., 2006; Kertesz et al., 2007; Sturm et al., 2010; Tsang et al., 2010; Hsu et al., 2011; Qiu et al., 2011; Lu et al., 2012; Vlachos et al., 2012) (Supplementary Table S1). These bioinformatics algorithms were selected to predict microRNA targeting based on a number of different features including; sequence complementarity, target site accessibility, binding energy, and conservation of target sites in order to increase precision and power.
The total number of unique microRNAs were calculated for each gene and the percentage overlap was estimated based on the number of microRNAs predicted by at least two algorithms. Analysis using these prediction algorithms was performed using the most recent version and respective default settings. Eight datasets containing microRNA expression profiles in liver tissue was obtained from mimiRNA (http://mimirna.centenary.org.au/mep/formulaire.html) (Ritchie et al., 2010) and compared to microRNAs predicted by the different algorithms and microRNAs with targeting predicted to be affected by miRSNPs, to determine overlap between predicted and functionally relevant microRNAs.
After prediction of likely microRNAs targeting genes coding for DMEs, the top six genes (with the longest 3′-UTR, most microRNA targets as well genes of interest in our research group) were chosen for sequencing of the 3′-UTR among 30 Bantu-speaking South Africans, and these genes included CYP1A2, CYP2B6, CYP2D6, CYP3A4, NR1I2, and UGT2B7. The assumption for selection of the six genes was based on the prediction that a gene with a longer 3′-UTR is more likely to be regulated by a larger number of microRNAs.
Participants were South African HIV/AIDS patients receiving efavirenz-based treatment for at least 6 months and recruited to participate in pharmacogenomics research. All subjects were of Bantu origin from Gauteng Province, South Africa. Participants gave information on their ethnicity, age, health status (including self-reported adherence to treatment or pill counts), dietary, and smoking habits. Ethics and study approval (HREC REF 103/2009) was given by the University of Cape Town, Faculty of Health Sciences Research Ethics Committee. Written informed consent was obtained from participants as part of a study focussing on pharmacogenetics of HIV therapy. Patients were mostly female (90%) and had an average age of 42 years. The research was performed in accordance with guidelines of the Helsinki Declaration of 2008.
DNA was isolated from a 5 mL blood sample using the method adapted from Gustafson et al. (1987) or the GenEluteTM Blood Genomic DNA kit (Sigma-Aldrich, St Louis, Missouri, USA). Primers for each 3′-UTR were designed to flank the 3′-UTR and were specific to the gene of interest (designed by aligning the gene sequence with that of pseudogenes to select a region that is unique to the gene of interest). NCBI Primer-BLAST (Altschul et al., 1990) (http://www.ncbi.nlm.nih.gov/tools/primer-blast/), and IDT Oligo Analyzer from Integrated DNA Technologies (http://www.idtdna.com/analyzer/Applications/OligoAnalyzer/) were used for primer design while synthesis was done by Integrated DNA Technologies, Inc. (Coralville, Iowa, USA). Primers for each gene's 3′-UTR were as follows: CYP1A2 (two sets), F1: 5'-CGACCTGACCCCCATCTAC-3′/R2: 5'-AAGAATGTAAGTTAGGCTGGATGTG-3′ and F2: 5'-CGCAGGTTCAAGCAATCC-3′/R1: 5'-AGGACTCAAGCACCAAGAGC-3′; CYP2B6 (two sets), F1: 5'-GTGGTGCCATCTCTGTCCA-3′/R1: 5'-AGAGTTGGCATTGAGGTGAGAG-3′ and F2: 5'-GGCAAAATACCCCCAACATA-3′/R2: 5'-GCCTGTGATACCAGCTCCTC-3′; CYP2D6, F: 5'-GCCACCATGGTGTCTTTGCTTTCCTGG-3′/R: 5'-ACTGAGCCCTGGGAGGTAGGTAG-3′; CYP3A4, F: 5'-CACTGAAGGCGTGTCTCACTCACT-3′/R: 5'-CTTCTCTACCTTAATGTGAGGGCACCA-3′; NR1I2, F: 5'-CCAGGACATACACCCCTTTG-3′/R: 5'-TATTTCCACACCCCCACATT-3′; and UGT2B7, F: 5'-AGAGAGGAGTCTTGCCGATG-3′/R: 5'-GAGAATAAAGTCAACCAGATGT-3′, respectively.
PCR amplification was performed using the following conditions: initial denaturation at 94°C for 3 min, followed by 40 cycles of denaturation at 94°C for 30 s, annealing at 61°C (CYP1A2 and CYP2B6), 60°C (CYP3A4 and NR1I2), 58°C (UGT2B7), or 70°C (CYP2D6) for 30 s, primer extension at 72°C for 2 min and final extension at 72°C for 10 min. A “T100™ Thermal cycler” (Bio-Rad, Hercules, USA) was used and the PCR reaction contained the following reagents; 50–100 ng genomic DNA, 1X Green GoTaq Reaction Buffer (Promega Corporation, Madison, USA), 0.2 mM of each of the deoxynucleotide triphosphates (dNTPs) (Bioline, London, UK), 1.5 mM MgCl2 (except for CYP1A2 fragment 1 where 2 mM MgCl2 was used), 40 pmol of the forward and reverse primers (Integrated DNA Technologies, Inc., Coralville, USA) and 2U of GoTaq DNA Polymerase (Promega Corporation, Madison, USA).
PCR product was cleaned using FastAP (Fermentas Life Sciences, Burlington, Canada) and ExoI (Fermentas Life Sciences, Burlington, Canada). The reaction conditions were as reported previously (Swart et al., 2013). The cleaned PCR product was sequenced on a “GeneAmp® PCR System 9700 version 3.08 (Applied Biosystems, Carlsbad, CA, USA) and capillary electrophoresis was performed on an ABI3130xl Genetic Analyzer (Applied Biosystems, Carlsbad, CA, USA). Analysis of the sequences was performed using DNAstar Lasergene Sequence Alignment Editor V.10 (DNASTAR, Inc., Madison, WI, USA).
The PolymiRTS database 3.0 (Bao et al., 2007; Ziebarth et al., 2012; Bhattacharya et al., 2014) (http://compbio.uthsc.edu/miRSNP/) was used to predict if SNPs located in the 3′-UTR of genes would affect mRNA-microRNA interaction. A search in the database was performed using the GeneID obtained from the NCBI database (http://www.ncbi.nlm.nih.gov/) and default settings. Pre-computed context+ scores were included in a recent update of TargetScan as a measure of predicted efficacy of microRNA targeting and down regulation of the target mRNA (log2 fold change in mRNA abundance after microRNA transfection assays) (Grimson et al., 2007) by summing up contributions made by individual sites using information on the site-type contribution, 3′-pairing contribution, local AU contribution, position contribution, target-site abundance contribution and seed-pairing stability contribution. The PolymiRTS database use the context+ scores from TargetScan to calculate the difference in context+ scores between the reference and derived alleles for each SNP. Differences in context+ scores caused by a SNP in the microRNA target site have been included in this study and a more negative difference in context+ scores indicates an increased likelihood that the target sites are either absent or present due to the variant allele, aiding in prioritizing miRSNPs with potential effect instead of showing miRSNPs that significantly alter microRNA binding (Bhattacharya et al., 2014).
To assess the potential effect of novel SNPs, the mrSNP software was used by selecting assembly hg19 of the human genome, using default cut-offs and inserting the chromosome position and two alleles. The prediction method applied is adapted from Diana-microT (Maragkakis et al., 2009; Deveci et al., 2014) (http://mrsnp.osu.edu/). Prediction is based on the ratio of binding energy and maximum binding energy of a microRNA (maximum MFE in Supplementary Table S3). The identified SNPs need to be further characterized in terms of their differential tissue expression as well as strength in achieving the predicted effects.
Minor allele frequencies (MAF) for SNPs in the 3′-UTR of the 6 genes sequenced among the 30 black South Africans, were compared to those of other world population groups, obtained from the dbSNP database (http://www.ncbi.nlm.nih.gov/SNP/), the HapMap project (http://hapmap.ncbi.nlm.nih.gov/), and the 1000 genomes project (http://www.1000genomes.org/). Statistical analyses were performed using the Graphpad Prism statistical program (Version 5, GraphPad Software Inc., San Diego, CA). Pearson's χ2-test and Fisher's exact test were used to examine differences between the population groups with regards to distribution of minor alleles. For all statistical tests significance was defined as P < 0.05. The novel SNPs identified in the 3′-UTR of the six genes could not be compared to other world population groups as they have not been reported before.
CYP1A2, CYP2B6, CYP2D6, CYP3A4, CYP3A5, GSTP1, UGT1A1, UGT2B7, SULT1A1, NR1I2, and NR1I3 were all predicted to be targets of microRNAs. The genes with the highest number of hits for microRNA target sites were CYP2B6, NR1I2 (PXR), CYP1A2, and CYP3A4, respectively (Table 1). CYP2B6, CYP1A2, and CYP3A4 were also predicted to be targets of many microRNAs by Ramamoorthy and Skaar (2011). Extensive variability in the number of microRNAs predicted by each algorithm was observed as expected. For example, the program miRTar estimated only four microRNA targets in CYP1A2, whereas the program PITA, estimated 78 microRNA targets in the same gene. The algorithm TargetScan predicted the highest number of microRNA target sites while the algorithms, PICTAR, and miRTar predicted the least number of target sites. The overlap in the number of microRNAs predicted by 15 algorithms ranged from 14 to 30% and the four genes predicted to be targets of the highest number of microRNAs (CYP1A2, CYP2B6, CYP3A4, and NR1I2) had at least 93% of their microRNAs predicted by seven algorithms (miR2Gene—DIANA-microT v.3, miRanda-MirSVR, miRSystem, PACCMIT, PITA, TargetScan, and TargetSpy). The specific microRNAs predicted to target CYP1A2, CYP2B6, CYP3A4, and NR1I2 by most algorithms are miR-542-3p (9 algorithms), miR-542-3p (10 algorithms), miR-548c-5p (11 algorithms), and miR-133a (9 algorithms), respectively (Table 2). The microRNAs predicted to target the most genes were miR-216a, miR-548a, miR-508-5p, miR-510, miR-671-5p, miR-142-3p, miR-608, miR-548b-5p, miR-548c-5p, miR-548d-5p, miR-548i, miR-548j, miR-514, miR-548a-3p, miR-548a-5p, miR-548h, miR-152, miR-148b, miR-214-3p, miR-520h, miR-520g, miR-330-5p, and miR-326. Comparison between the number of microRNAs predicted by the 15 algorithms and microRNAs expressed in liver tissue, showed an overlap of about 16% (240 microRNAs both expressed and predicted/1537 predicted microRNAs). Additionally, the length of the 3′-UTR correlated significantly (r2 = 0.764, P-value = 0.0062) with the number of microRNAs predicted to target the specific gene. The correlation of number of microRNAs predicted to target and the length of the 3′-UTR is similar to previous reports (Ramamoorthy and Skaar, 2011).
Table 2. MicroRNAs predicted to target the 3′-UTR of CYP1A2, CYP2B6, CYP3A4, and NR1I2 by seven or more algorithms.
Based on the total microRNAs predicted to target the mRNA, the top four genes CYP1A2, CYP2B6, CYP3A4, and NR1I2 were selected for further analysis. CYP2D6 and UGT2B7 were included as they form part of our ongoing pharmacogenomics studies. In total six genes had their 3′-UTR sequenced among 30 individuals. A total of 52 genetic variants were identified, including 17 novel and 35 previously reported SNPs. Novel SNPs identified, despite comprehensive re-sequencing of various population groups, are potentially rare and population specific or this may be an indication of the exclusion of populations from this region in the re-sequencing projects. CYP2B6 had the most genetic variants in the 3′-UTR accounting for 26 of the 52. No SNPs were identified within the 75bp 3′-UTR of CYP2D6 (Table 3). Forty of the 52 genetic variants were predicted to potentially affect regulation of 212 microRNAs by creating or abolishing microRNA target sites depending on which of the variants is the ancestral variant (Supplementary Table S2). Based on predictions by mrSNP, 15 of the 17 novel SNPs were located within microRNA target sites and potentially result in the creation of microRNA target sites (Supplementary Table S3). Comparison between the number of microRNAs potentially affected by miRSNPs and microRNAs expressed in liver tissue, showed an overlap of about 3% (10 microRNAs both expressed and predicted to potentially be affected by miRSNPs/363 expressed microRNAs).
Table 3. Genetic variation identified in the 3′-UTR of CYP1A2, CYP2B6, CYP2D6, CYP3A4, NR1I2, and UGT2B7 after sequencing of 30 black South Africans.
Of the 52 variants identified in sequencing the 3′-UTR of selected genes among South African black participants, 18 SNPs which also had information for other population groups (i.e., through the HapMap and 1000 genomes projects) were compared to other African groups, African-Americans, Asian, and European population groups (Table 4). Statistically significant differences were observed when the South African group was compared to Asian, Caucasian and other Africa groups. For example, NR1I2 rs1054190T allele occurring at a frequency of 0.18 in the South African group, was absent among Asians, significantly lower among another African group the Yoruba (0.02), yet comparable to that reported among Caucasians (0.15). For example, the variant, rs1054190C in NR1I2 was predicted to result in the presence of a binding site for the microRNA miR-1250-5p, while the variant rs1054191G was predicted to result in the absence of a recognition site for miR-371b-3p, miR-4258, and miR-4707-3p. A second SNP in NR1I2, rs3732359A allele showed no significant differences when the South African group (0.56) was compared to Asians (0.47), but showed statistically significant differences when compared to the Caucasians (0.84) and Yoruba (0.24). As expected, differences in allele frequencies of miRSNPs were observed when comparing the South African Bantu population group to both Caucasian and Asian populations as highlighted by the CYP2B6 rs28969420T allele (20, 4, 3%, respectively).
Table 4. Comparison of minor allele frequencies for selected genetic variants in the 3′-UTR of pharmacogenomically-relevant genes among different world populations.
Pharmacogenomics studies have focussed largely on the effects of genetic variation in genes coding for DMEs (Ingelman-Sundberg et al., 2007) and very few have investigated the role of variation at the level of microRNA target sites. MicroRNAs affect global gene expression, thus, including miRSNPs in pharmacogenomic tests, could potentially explain some of the genetics-associated differences in drug response (Mishra and Bertino, 2009). Computational prediction analysis carried out in this study has highlighted microRNAs that regulate some of the genes with pharmacogenomics relevance, further confirming observations by Ramamoorthy and Skaar (2011) with respect to CYP1A2, CYP2B6, and CYP3A4.
At least 10% more microRNAs were predicted to target DMEs, for example the genes CYP1A2, CYP2B6, CYP2D6, CYP3A4, and CYP3A5 were reported to be targeted by 436, 515, 196, 429, and 176 potential microRNAs using the 15 prediction algorithms, compared to 386, 416, 40, 333, and 32 using six algorithms as reported by Ramamoorthy and Skaar (2011). In addition, the number of microRNAs predicted using TargetScan v.6.2 in the current study were more than double those reported by Ramamoorthy, using an earlier version (Ramamoorthy and Skaar, 2011). The higher number of microRNAs predicted, is potentially a result of using newer versions of the algorithms compared to those used by Ramamoorthy and Skaar (2011). The use of more algorithms was justified by the low percentage (14–30%) overlap between microRNAs predicted by two or more algorithms. However, the question still remains as to what is the appropriate minimum number or combination of algorithms to pick most microRNAs. MicroRNA prediction using 7 of the 15 algorithms (miR2Gene—DIANA-microT v.3, miRanda-MirSVR, miRSystem, PACCMIT, PITA, TargetScan, and TargetSpy), showed on average 83% (range 43–98%) of all unique microRNAs, pointing toward the major contribution of these seven algorithms (especially TargetScan) compared to the remaining eight algorithms. The appropriate combination of algorithms will only be apparent after confirmatory functional validation of the predicted binding targets.
A few microRNAs have been experimentally shown to regulate expression of DMEs. For example, Pan et al. (2009) reported that CYP3A4 is regulated by miR-27b directly, and by miR-148a indirectly through the regulation of PXR (Takagi et al., 2008). This observation is confirmed by our prediction analysis as five algorithms predicted miR-27b to target CYP3A4, while four algorithms predicted miR-148a to target NR1I2 (coding for PXR). Two microRNAs namely; miR-590 and miR-27a have been associated with either targeting CYP2B6 (miR-590) or CYP3A4 (miR-27a) (Ramamoorthy et al., 2013). The finding of CYP2B6, CYP3A4, and UGT1A1 being targets of miR-590, miR-27a, miR-491-3p, respectively (Dluzen et al., 2014), is supported by our prediction analysis. However, our observations on SULT1A1 mRNA are contradictory to those of Yu et al. (2010), who reported targeting by miR-631, yet none of the 15 computational algorithms picked it. MiR-26b-5p is reportedly associated with CYP2D6 expression (Gennarino et al., 2009) and miR-335-5p with CYP3A5, but none of the two microRNAs was picked by the 15 algorithms used in the current prediction analysis. It appears that the prediction algorithms might have inherent weaknesses, for example, GSTP1 has been experimentally validated to be affected by miR-513a-3p, miR-133a-3p, miR-26b-5p, and miR-92a-3p, but only miR-133a-3p was picked by the use of 15 algorithms. It is important to note that computational prediction algorithms are thought to predict microRNA-mRNA targeting with a fraction of false positives estimated at 31% and it is, thus, of importance to prioritize microRNAs for further studies (Lewis et al., 2003). The overlap between microRNAs predicted by the 15 algorithms and microRNAs potentially affected by miRSNPs with microRNAs expressed in liver tissue is very low. Prediction algorithms use different criteria to rank microRNAs that potentially target mRNA of a gene of interest, and are likely to be more lenient in the prediction as it is mostly based on sequence complementarity, target site accessibility, binding energy and conservation of target sites. Also on the other hand, expression is dependent on many other factors including export and tissues involved. Further comprehensive microRNA expression profiling in liver as well as extrahepatic tissues would contribute toward identifying microRNAs that are potentially involved in regulation of DMEs. There is also a strong need for experimental validation of predicted microRNA targets.
A total of 52 SNPs were identified within the 3′-UTR of CYP1A2, CYP2B6, CYP3A4, NR1I2, and UGT2B7 including 17 novel SNPs, which is an indication of the extensive degree of genetic diversity characteristic to African and specifically South African populations (Matimba et al., 2009; Warnich et al., 2011; Dandara et al., 2014). SNPs (miRSNPs) identified in the South African group and already available in the PolymiRTS database, were predicted to alter the microRNA targeting of more than 200 microRNAs (Supplementary Table S2). As expected, differences in allele frequencies of miRSNPs were observed when comparing the South African Bantu population group to both Caucasian and Asian populations as highlighted by the CYP2B6 rs28969420T allele (20% compared to 4 and 3%, respectively) and the NR1I2 rs3732359A-allele (59% compared to 84 and 47%, respectively). Differences in the frequency of miRSNPs among population groups as highlighted by the NR1I2 rs37372359A allele, which results in the presence of a target site for several microRNAs including miR-362-5p, miR-500b-5p, and miR-501-5p, could translate into population differences in drug disposition and response. These qualitative and quantitative differences in allele frequencies of SNPs further support previous studies arguing against directly inferring effects of therapeutic drugs from one population to another (Swart et al., 2012a,b,c; Dandara et al., 2014). The 3′-UTR variation identified in the current study contributes to further genetic characterizing of South Africans. However, the observed variation should be functionally validated in order to deduce its contribution to the observed variability in drug response.
Response to therapeutic treatment is a complex phenotype determined by genetic variation in DMEs, nuclear receptors, drug transporters, miRSNPs, and pharmacoepigenetics (Zhang et al., 2010). MicroRNAs have the potential to be used as therapeutic targets through their ability to regulate expression of pharmacogenomically relevant genes (Singh et al., 2011; Van Rooij et al., 2012). Creating a microRNA recognition site leads to down regulation of mRNA expression of specific genes, while disruption of a site causes loss of down regulation of mRNA expression (Mishra and Bertino, 2009). Caution should however be exercised when developing microRNA-based therapeutics because of unpredictable off-target effects due to microRNAs having broad mRNA targets (Singh et al., 2011). A study by Mishra et al. (2007) demonstrated the effect of a SNP near the miR-24 binding site in the 3′-UTR of the dihydrofolate reductase (DHFR) gene, that interferes with miR-24 binding and resulting in DHFR overexpression and methotrexate resistance. Resistance to the cancer therapeutics cisplatin and 5-fluorouracil has been linked to induced miR-148a expression and targeting of the gene KIT (Hummel et al., 2011). MiR-148a has been shown by Takagi et al. (2008) to regulate PXR and affect downstream expression of CYP3A4, resulting in reduced drug metabolism. Presence of the NR1I2 rs1054190T allele results in the absence of a miR-148a target site, potentially leading to increased CYP3A4 expression. It is important that future studies investigate the functional significance of microRNAs and miRSNPs. The recently developed Clustered Regularly Interspaced Short Palindromic Repeats (CRISPR) technology could be used to create knockout cell lines for specific microRNAs to validate their role in targeting of genes with pharmacogenomics relevance (Dandara et al., 2014).
The integration of microRNA variation and epigenetics into pharmacogenomics studies may provide additional insights into the mechanisms of drug response and advance individualized therapy (Welsh et al., 2009; Zhang and Dolan, 2010). Use of in silico methods should be complimented by functional validation to confirm the predicted effects. It is therefore possible that some of the observed non-reproducibility of pharmacogenomics studies could partially be due to the unexplained variation in the 3′-UTR, thus, functional validation of microRNA targets should be another area of research to advance pharmacogenomics.
Marelize Swart carried out the in silico prediction and sequencing analysis and drafted the manuscript. Collet Dandara conceived of the study, designed, coordinated the study, and also assisted with statistical data analysis, helped to draft the manuscript and approved the final version. Both authors read and approved the final manuscript.
The authors declare that the research was conducted in the absence of any commercial or financial relationships that could be construed as a potential conflict of interest.
We thank the South African Medical Research Council (MRC), the National Research Foundation of South Africa (NRF), and the University of Cape Town for funding.
The Supplementary Material for this article can be found online at: http://www.frontiersin.org/journal/10.3389/fgene.2014.00167/abstract
Alnouti, Y., and Klaassen, C. D. (2008). Regulation of sulfotransferase enzymes by prototypical microsomal enzyme inducers in mice. J. Pharmacol. Exp. Ther. 324, 612–621. doi: 10.1124/jpet.107.129650
Altschul, S. F., Gish, W., Miller, W., Myers, E. W., and Lipman, D. J. (1990). Basic local alignment search tool. J. Mol. Biol. 215, 403–410. doi: 10.1016/S0022-2836(05)80360-2
Armstrong, S. C., and Cozza, K. L. (2003). Pharmacokinetic drug interactions of morphine, codeine, and their derivatives: theory and clinical reality, Part II. Psychosomatics 44, 515–520. doi: 10.1176/appi.psy.44.6.515
Baek, D., Villen, J., Shin, C., Camargo, F. D., Gygi, S. P., and Bartel, D. P. (2008). The impact of microRNAs on protein output. Nature 455, 64–71. doi: 10.1038/nature07242
Bao, L., Zhou, M., Wu, L., Lu, L., Goldowitz, D., Williams, R. W., et al. (2007). PolymiRTS Database: linking polymorphisms in microRNA target sites with complex traits. Nucleic Acids Res. 35, D51–D54. doi: 10.1093/nar/gkl797
Batt, A. M., Magdalou, J., Vincent-Viry, M., Ouzzine, M., Fournel-Gigleux, S., Galteau, M. M., et al. (1994). Drug metabolizing enzymes related to laboratory medicine: cytochromes P-450 and UDP-glucuronosyltransferases. Clin. Chim. Acta 226, 171–190. doi: 10.1016/0009-8981(94)90214-3
Bhattacharya, A., Ziebarth, J. D., and Cui, Y. (2014). PolymiRTS Database 3.0: linking polymorphisms in microRNAs and their target sites with human diseases and biological pathways. Nucleic Acids Res. 42, D86–D91. doi: 10.1093/nar/gkt1028
Breving, K., and Esquela-Kerscher, A. (2010). The complexities of microRNA regulation: mirandering around the rules. Int. J. Biochem. Cell Biol. 42, 1316–1329. doi: 10.1016/j.biocel.2009.09.016
Ciccacci, C., Di Fusco, D., Marazzi, M. C., Zimba, I., Erba, F., Novelli, G., et al. (2013). Association between CYP2B6 polymorphisms and Nevirapine-induced SJS/TEN: a pharmacogenetics study. Eur. J. Clin. Pharmacol. 69, 1909–1916. doi: 10.1007/s00228-013-1549-x
Commandeur, J. N., Stijntjes, G. J., and Vermeulen, N. P. (1995). Enzymes and transport systems involved in the formation and disposition of glutathione S-conjugates. Role in bioactivation and detoxication mechanisms of xenobiotics. Pharmacol. Rev. 47, 271–330.
Dandara, C., Swart, M., Mpeta, B., Wonkam, A., and Masimirembwa, C. (2014). Cytochrome P450 pharmacogenetics in African populations: implications for public health. Expert Opin. Drug Metab. Toxicol. 10, 769–785. doi: 10.1517/17425255.2014.894020
Deveci, M., Catalyurek, U. V., and Toland, A. E. (2014). mrSNP: software to detect SNP effects on microRNA binding. BMC Bioinformatics 15:73. doi: 10.1186/1471-2105-15-73
Dluzen, D. F., Sun, D., Salzberg, A. C., Jones, N., Bushey, R. T., Robertson, G. P., et al. (2014). Regulation of UDP-glucuronosyltransferase 1A1 expression and activity by microRNA 491-3p. J. Pharmacol. Exp. Ther. 348, 465–477. doi: 10.1124/jpet.113.210658
Gennarino, V. A., Sardiello, M., Avellino, R., Meola, N., Maselli, V., Anand, S., et al. (2009). MicroRNA target prediction by expression analysis of host genes. Genome Res. 19, 481–490. doi: 10.1101/gr.084129.108
Griffiths-Jones, S., Grocock, R. J., Van Dongen, S., Bateman, A., and Enright, A. J. (2006). miRBase: microRNA sequences, targets and gene nomenclature. Nucleic Acids Res. 34, D140–D144. doi: 10.1093/nar/gkj112
Grimson, A., Farh, K. K., Johnston, W. K., Garrett-Engele, P., Lim, L. P., and Bartel, D. P. (2007). MicroRNA targeting specificity in mammals: determinants beyond seed pairing. Mol. Cell 27, 91–105. doi: 10.1016/j.molcel.2007.06.017
Guengerich, F. P. (2008). Cytochrome p450 and chemical toxicology. Chem. Res. Toxicol. 21, 70–83. doi: 10.1021/tx700079z
Gustafson, S., Proper, J. A., Bowie, E. J., and Sommer, S. S. (1987). Parameters affecting the yield of DNA from human blood. Anal. Biochem. 165, 294–299. doi: 10.1016/0003-2697(87)90272-7
Hayes, J. D., Flanagan, J. U., and Jowsey, I. R. (2005). Glutathione transferases. Annu. Rev. Pharmacol. Toxicol. 45, 51–88. doi: 10.1146/annurev.pharmtox.45.120403.095857
Hsu, J. B., Chiu, C. M., Hsu, S. D., Huang, W. Y., Chien, C. H., Lee, T. Y., et al. (2011). miRTar: an integrated system for identifying miRNA-target interactions in human. BMC Bioinformatics 12:300. doi: 10.1186/1471-2105-12-300
Huang, H. Y., Chien, C. H., Jen, K. H., and Huang, H. D. (2006). RegRNA: an integrated web server for identifying regulatory RNA motifs and elements. Nucleic Acids Res. 34, W429–W434. doi: 10.1093/nar/gkl333
Hummel, R., Wang, T., Watson, D. I., Michael, M. Z., Van Der Hoek, M., Haier, J., et al. (2011). Chemotherapy-induced modification of microRNA expression in esophageal cancer. Oncol. Rep. 26, 1011–1017. doi: 10.3892/or.2011.1381
Ingelman-Sundberg, M., Sim, S. C., Gomez, A., and Rodriguez-Antona, C. (2007). Influence of cytochrome P450 polymorphisms on drug therapies: pharmacogenetic, pharmacoepigenetic and clinical aspects. Pharmacol. Ther. 116, 496–526. doi: 10.1016/j.pharmthera.2007.09.004
John, B., Enright, A. J., Aravin, A., Tuschl, T., Sander, C., and Marks, D. S. (2004). Human microRNA targets. PLoS Biol. 2:e363. doi: 10.1371/journal.pbio.0020363
Kertesz, M., Iovino, N., Unnerstall, U., Gaul, U., and Segal, E. (2007). The role of site accessibility in microRNA target recognition. Nat. Genet. 39, 1278–1284. doi: 10.1038/ng2135
Krek, A., Grun, D., Poy, M. N., Wolf, R., Rosenberg, L., Epstein, E. J., et al. (2005). Combinatorial microRNA target predictions. Nat. Genet. 37, 495–500. doi: 10.1038/ng1536
Lagos-Quintana, M., Rauhut, R., Lendeckel, W., and Tuschl, T. (2001). Identification of novel genes coding for small expressed RNAs. Science 294, 853–858. doi: 10.1126/science.1064921
Lewis, B. P., Shih, I. H., Jones-Rhoades, M. W., Bartel, D. P., and Burge, C. B. (2003). Prediction of mammalian microRNA targets. Cell 115, 787–798. doi: 10.1016/S0092-8674(03)01018-3
Liu, C., Zhang, F., Li, T., Lu, M., Wang, L., Yue, W., et al. (2012). MirSNP, a database of polymorphisms altering miRNA target sites, identifies miRNA-related SNPs in GWAS SNPs and eQTLs. BMC Genomics 13:661. doi: 10.1186/1471-2164-13-661
Lu, T. P., Lee, C. Y., Tsai, M. H., Chiu, Y. C., Hsiao, C. K., Lai, L. C., et al. (2012). miRSystem: an integrated system for characterizing enriched functions and pathways of microRNA targets. PLoS ONE 7:e42390. doi: 10.1371/journal.pone.0042390
Maragkakis, M., Reczko, M., Simossis, V. A., Alexiou, P., Papadopoulos, G. L., Dalamagas, T., et al. (2009). DIANA-microT web server: elucidating microRNA functions through target prediction. Nucleic Acids Res. 37, W273–W276. doi: 10.1093/nar/gkp292
Marin, R. M., and Vanicek, J. (2012). Optimal use of conservation and accessibility filters in microRNA target prediction. PLoS ONE 7:e32208. doi: 10.1371/journal.pone.0032208
Marzolini, C., Telenti, A., Decosterd, L. A., Greub, G., Biollaz, J., and Buclin, T. (2001). Efavirenz plasma levels can predict treatment failure and central nervous system side effects in HIV-1-infected patients. AIDS 15, 71–75. doi: 10.1097/00002030-200101050-00011
Matimba, A., Del-Favero, J., Van Broeckhoven, C., and Masimirembwa, C. (2009). Novel variants of major drug-metabolising enzyme genes in diverse African populations and their predicted functional effects. Hum. Genomics 3, 169–190. doi: 10.1186/1479-7364-3-2-169
McCarver, D. G., and Hines, R. N. (2002). The ontogeny of human drug-metabolizing enzymes: phase II conjugation enzymes and regulatory mechanisms. J. Pharmacol. Exp. Ther. 300, 361–366. doi: 10.1124/jpet.300.2.361
Miranda, K. C., Huynh, T., Tay, Y., Ang, Y. S., Tam, W. L., Thomson, A. M., et al. (2006). A pattern-based method for the identification of MicroRNA binding sites and their corresponding heteroduplexes. Cell 126, 1203–1217. doi: 10.1016/j.cell.2006.07.031
Mishra, P. J. (2012). The miRNA-drug resistance connection: a new era of personalized medicine using noncoding RNA begins. Pharmacogenomics 13, 1321–1324. doi: 10.2217/pgs.12.121
Mishra, P. J., and Bertino, J. R. (2009). MicroRNA polymorphisms: the future of pharmacogenomics, molecular epidemiology and individualized medicine. Pharmacogenomics 10, 399–416. doi: 10.2217/14622416.10.3.399
Mishra, P. J., Humeniuk, R., Mishra, P. J., Longo-Sorbello, G. S., Banerjee, D., and Bertino, J. R. (2007). A miR-24 microRNA binding-site polymorphism in dihydrofolate reductase gene leads to methotrexate resistance. Proc. Natl. Acad. Sci. U.S.A. 104, 13513–13518. doi: 10.1073/pnas.0706217104
Mishra, P. J., Mishra, P. J., Banerjee, D., and Bertino, J. R. (2008). MiRSNPs or MiR-polymorphisms, new players in microRNA mediated regulation of the cell: introducing microRNA pharmacogenomics. Cell Cycle 7, 853–858. doi: 10.4161/cc.7.7.5666
Mohri, T., Nakajima, M., Fukami, T., Takamiya, M., Aoki, Y., and Yokoi, T. (2010). Human CYP2E1 is regulated by miR-378. Biochem. Pharmacol. 79, 1045–1052. doi: 10.1016/j.bcp.2009.11.015
Pan, Y. Z., Gao, W., and Yu, A. M. (2009). MicroRNAs regulate CYP3A4 expression via direct and indirect targeting. Drug Metab. Dispos. 37, 2112–2117. doi: 10.1124/dmd.109.027680
Petzinger, E., and Geyer, J. (2006). Drug transporters in pharmacokinetics. Naunyn Schmiedebergs Arch. Pharmacol. 372, 465–475. doi: 10.1007/s00210-006-0042-9
Qiu, C., Wang, J., and Cui, Q. (2011). miR2Gene: pattern discovery of single gene, multiple genes, and pathways by enrichment analysis of their microRNA regulators. BMC Syst. Biol. 5(Suppl. 2):S9. doi: 10.1186/1752-0509-5-S2-S9
Ramamoorthy, A., Liu, Y., Philips, S., Desta, Z., Lin, H., Goswami, C., et al. (2013). Regulation of microRNA expression by rifampin in human hepatocytes. Drug Metab. Dispos. 41, 1763–1768. doi: 10.1124/dmd.113.052886
Ramamoorthy, A., and Skaar, T. C. (2011). In silico identification of microRNAs predicted to regulate the drug metabolizing cytochrome P450 genes. Drug Metab. Lett. 5, 126–131. doi: 10.2174/187231211795305258
Ritchie, W., Flamant, S., and Rasko, J. E. (2010). mimiRNA: a microRNA expression profiler and classification resource designed to identify functional correlations between microRNAs and their targets. Bioinformatics 26, 223–227. doi: 10.1093/bioinformatics/btp649
Rodrigues, A. C., Li, X., Radecki, L., Pan, Y. Z., Winter, J. C., Huang, M., et al. (2011). MicroRNA expression is differentially altered by xenobiotic drugs in different human cell lines. Biopharm. Drug Dispos. 32, 355–367. doi: 10.1002/bdd.764
Shomron, N. (2010). MicroRNAs and pharmacogenomics. Pharmacogenomics 11, 629–632. doi: 10.2217/pgs.10.26
Singh, D., Kashyap, A., Pandey, R. V., and Saini, K. S. (2011). Novel advances in cytochrome P450 research. Drug Discov. Today 16, 793–799. doi: 10.1016/j.drudis.2011.08.003
Sturm, M., Hackenberg, M., Langenberger, D., and Frishman, D. (2010). TargetSpy: a supervised machine learning approach for microRNA target prediction. BMC Bioinformatics 11:292. doi: 10.1186/1471-2105-11-292
Swart, M., Ren, Y., Smith, P., and Dandara, C. (2012a). ABCB1 4036A>G and 1236C>T polymorphisms affect plasma efavirenz levels in South African HIV/AIDS patients. Front. Genet. 3:236. doi: 10.3389/fgene.2012.00236
Swart, M., Skelton, M., Ren, Y., Smith, P., Takuva, S., and Dandara, C. (2013). High predictive value of CYP2B6 SNPs for steady-state plasma efavirenz levels in South African HIV/AIDS patients. Pharmacogenet. Genomics 23, 415–427. doi: 10.1097/FPC.0b013e328363176f
Swart, M., Skelton, M., Wonkam, A., Kannemeyer, L., Chin'ombe, N., and Dandara, C. (2012b). CYP1A2, CYP2A6, CYP2B6, CYP3A4 and CYP3A5 polymorphisms in two bantu-speaking populations from Cameroon and South Africa: implications for global pharmacogenetics. Curr. Pharmacogenomics Person. Med. 10, 43–53. doi: 10.2174/1875692111201010043
Swart, M., Whitehorn, H., Ren, Y., Smith, P., Ramesar, R. S., and Dandara, C. (2012c). PXR and CAR single nucleotide polymorphisms influence plasma efavirenz levels in South African HIV/AIDS patients. BMC Med. Genet. 13:112. doi: 10.1186/1471-2350-13-112
Takagi, S., Nakajima, M., Mohri, T., and Yokoi, T. (2008). Post-transcriptional regulation of human pregnane X receptor by micro-RNA affects the expression of cytochrome P450 3A4. J. Biol. Chem. 283, 9674–9680. doi: 10.1074/jbc.M709382200
Tsang, J. S., Ebert, M. S., and Van Oudenaarden, A. (2010). Genome-wide dissection of microRNA functions and cotargeting networks using gene set signatures. Mol. Cell 38, 140–153. doi: 10.1016/j.molcel.2010.03.007
Tsuchiya, Y., Nakajima, M., Takagi, S., Taniya, T., and Yokoi, T. (2006). MicroRNA regulates the expression of human cytochrome P450 1B1. Cancer Res. 66, 9090–9098. doi: 10.1158/0008-5472.CAN-06-1403
Tukey, R. H., and Strassburg, C. P. (2000). Human UDP-glucuronosyltransferases: metabolism, expression, and disease. Annu. Rev. Pharmacol. Toxicol. 40, 581–616. doi: 10.1146/annurev.pharmtox.40.1.581
Van Rooij, E., Purcell, A. L., and Levin, A. A. (2012). Developing microRNA therapeutics. Circ. Res. 110, 496–507. doi: 10.1161/CIRCRESAHA.111.247916
Vardhanabhuti, S., Acosta, E. P., Ribaudo, H. J., Severe, P., Lalloo, U., Kumarasamy, N., et al. (2013). Clinical and genetic determinants of plasma nevirapine exposure following an intrapartum dose to prevent mother-to-child HIV transmission. J. Infect. Dis. 208, 662–671. doi: 10.1093/infdis/jit223
Vlachos, I. S., Kostoulas, N., Vergoulis, T., Georgakilas, G., Reczko, M., Maragkakis, M., et al. (2012). DIANA miRPath v.2.0: investigating the combinatorial effect of microRNAs in pathways. Nucleic Acids Res. 40, W498–W504. doi: 10.1093/nar/gks494
Warnich, L., Drogemoller, B. I., Pepper, M. S., Dandara, C., and Wright, G. E. (2011). Pharmacogenomic research in South Africa: lessons learned and future opportunities in the rainbow nation. Curr. Pharmacogenomics Person. Med. 9, 191–207. doi: 10.2174/187569211796957575
Welsh, M., Mangravite, L., Medina, M. W., Tantisira, K., Zhang, W., Huang, R. S., et al. (2009). Pharmacogenomic discovery using cell-based models. Pharmacol. Rev. 61, 413–429. doi: 10.1124/pr.109.001461
Witkos, T. M., Koscianska, E., and Krzyzosiak, W. J. (2011). Practical aspects of microRNA target prediction. Curr. Mol. Med. 11, 93–109. doi: 10.2174/156652411794859250
Xu, C., Li, C. Y., and Kong, A. N. (2005). Induction of phase I, II and III drug metabolism/transport by xenobiotics. Arch. Pharm. Res. 28, 249–268. doi: 10.1007/BF02977789
Yu, A. M. (2009). Role of microRNAs in the regulation of drug metabolism and disposition. Expert Opin. Drug Metab. Toxicol. 5, 1513–1528. doi: 10.1517/17425250903307448
Yu, X., Dhakal, I. B., Beggs, M., Edavana, V. K., Williams, S., Zhang, X., et al. (2010). Functional genetic variants in the 3′-untranslated region of sulfotransferase isoform 1A1 (SULT1A1) and their effect on enzymatic activity. Toxicol. Sci. 118, 391–403. doi: 10.1093/toxsci/kfq296
Zhang, G., Wang, Q., and Xu, R. (2010). Therapeutics based on microRNA: a new approach for liver cancer. Curr. Genomics 11, 311–325. doi: 10.2174/138920210791616671
Zhang, W., and Dolan, M. E. (2010). The emerging role of microRNAs in drug responses. Curr. Opin. Mol. Ther. 12, 695–702.
Zhang, Y., and Verbeek, F. J. (2010). Comparison and integration of target prediction algorithms for microRNA studies. J. Integr. Bioinform. 7. doi: 10.2390/biecoll-jib-2010-127
Keywords: bioinformatics prediction, drug metabolizing enzymes, microRNA, miRSNPs, pharmacogenomics, polymorphisms
Citation: Swart M and Dandara C (2014) Genetic variation in the 3′-UTR of CYP1A2, CYP2B6, CYP2D6, CYP3A4, NR1I2, and UGT2B7: potential effects on regulation by microRNA and pharmacogenomics relevance. Front. Genet. 5:167. doi: 10.3389/fgene.2014.00167
Received: 06 January 2014; Accepted: 19 May 2014;
Published online: 04 June 2014.
Edited by:
Guilherme Suarez-Kurtz, Instituto Nacional de Cancer, BrazilReviewed by:
Todd Skaar, Indiana University Medical Center, USACopyright © 2014 Swart and Dandara. This is an open-access article distributed under the terms of the Creative Commons Attribution License (CC BY). The use, distribution or reproduction in other forums is permitted, provided the original author(s) or licensor are credited and that the original publication in this journal is cited, in accordance with accepted academic practice. No use, distribution or reproduction is permitted which does not comply with these terms.
*Correspondence: Collet Dandara, Pharmacogenetics and Cancer Research Group, Division of Human Genetics, Department of Clinical Laboratory Science, Faculty of Health Sciences, University of Cape Town, Room N3.18.3, Wernher Beit North Building, Anzio Rd., Observatory, 7925 Cape Town, South Africa e-mail:Y29sbGV0LmRhbmRhcmFAdWN0LmFjLnph
Disclaimer: All claims expressed in this article are solely those of the authors and do not necessarily represent those of their affiliated organizations, or those of the publisher, the editors and the reviewers. Any product that may be evaluated in this article or claim that may be made by its manufacturer is not guaranteed or endorsed by the publisher.
Research integrity at Frontiers
Learn more about the work of our research integrity team to safeguard the quality of each article we publish.