- Cancer Epigenetics and Biology Program, Bellvitge Biomedical Research Institute, Barcelona, Spain
With rapid advances in sequencing technologies, we are undergoing a paradigm shift from hypothesis- to data-driven research. Genome-wide profiling efforts have given informative insights into biological processes; however, considering the wealth of variation, the major challenge still remains in their meaningful interpretation. In particular sequence variation in non-coding contexts is often challenging to interpret. Here, data integration approaches for the identification of functional genetic variability represent a possible solution. Exemplary, functional linkage analysis integrating genotype and expression data determined regulatory quantitative trait loci and proposed causal relationships. In addition to gene expression, epigenetic regulation and specifically DNA methylation was established as highly valuable surrogate mark for functional variance of the genetic code. Epigenetic modification has served as powerful mediator trait to elucidate mechanisms forming phenotypes in health and disease. Particularly, integrative studies of genetic and DNA methylation data have been able to guide interpretation strategies of risk genotypes, but also proved their value for physiological traits, such as natural human variation and aging. This Review seeks to illustrate the power of data integration in the genomic era exemplified by DNA methylation quantitative trait loci. However, the model is further extendable to virtually all traceable molecular traits.
Introduction
Owing to the boost in detection technologies, numerous genetic and epigenetic variations have been related to phenotypic variability, including human diseases. Their interpretation has given an informative insight into aberrant biological processes and has led to the identification of novel targets for therapeutic interventions (Garraway and Lander, 2013). However, considering the wealth of alterations detectable by genome-wide studies, the major challenge still remains in the discrimination between driving events and those that are functionally silent or mere consequence of the disease. Furthermore, alterations frequently occur in a non-coding context, complicating their functional interpretation (Hindorff et al., 2011).
The comprehensive landmarking of functional elements in the human genome provided a measure of regional transcriptional activity and regulatory potential (Consortium, 2012). Latter regions were categorized by representative histone marks, DNA methylation levels, and chromatin conformations. However, their annotation does not immediately provide functional insights, as most of regulatory loci cannot be clearly assigned to target genes, especially those outside the promoter context. Nevertheless, various studies reported a significant overlap between phenotype-associated polymorphisms with regulatory histone marks (Hnisz et al., 2013), differentially methylated regions (Ziller et al., 2013) or open chromatin formations (Paul et al., 2013), suggesting their implication in functional downstream cascades and phenotype formation.
However, despite the comprehensive functional annotation of the human genome, we largely lack mechanistic interpretations for the majority of genetic variations and genotype–phenotype associations. To improve our understanding of causal relationships, we need to undergo a paradigm shift from analyzing loci function to loci effects; and shifting from annotation toward functional linkage studies. These are in particular straightforward to interpret if a component of the associations can be directly connected to gene function or activity. An illustrative and well-established example of functional linkage analysis is represented by expression quantitative trait loci (eQTL), wherein cis- or trans-located genetic polymorphisms present significant associations to gene activity, determined by transcript abundance and even transcript variants (Grundberg et al., 2012; Lappalainen et al., 2013). The identification of direct impacts of polymorphisms on transcriptional activity suggests causality, with the genetic variant and altered transcription being the cause and consequence, respectively. Particularly valuable for intergenic and intronic polymorphisms that are otherwise challenging to interpret, eQTL directly point to gene targets and hence facilitate the identification of disease driving mechanisms (Li et al., 2010, 2013).
Epigenetic Mediator Function in Gene Regulation
Functionally, the linkage model can be further extended including mediator traits that regulate gene expression. In this regard, virtually all regulatory events can be assigned as mediator, including histone modifications, DNA methylation, non-coding RNAs, or chromatin structure. Intriguingly, although the intense interplay between different layers of epigenetic regulation is accepted knowledge (Bernstein et al., 2012), it is poorly understood how the genetic and epigenetic code interact (Schübeler, 2012).
In this respect, computational strategies linking the genome to the epigenome, suggest a profound intrinsic association between epigenetic marks and genetic sequence, with cumulative effects of many weak interactions eventually forming the epigenetic code (Yuan, 2012). Consistently, to a certain extend epigenetic landscapes could be predicted in silico and assembled in vitro, suggesting the epigenome to be partly encoded in the genome (Kaplan et al., 2009). Herein, specifically DNA methylation patterns present strong genetic associations (Weber et al., 2005; Lienert et al., 2011). However, the genetic blueprint is likely to permit certain epigenetic states without precisely defining shape or timing. Certainly, the linkage between genetic variance and gene regulation could improve our knowledge about phenotype biology and the underlying mechanistic chain of events. Furthermore, an integrative analysis of genetic variation with the gene regulome could facilitate the interpretation of non-coding genetic variance and could further support strategies to reliably identify phenotype driving events.
Technically, it is important to distinguish between two concepts of functional linkage studies: the analysis of overlapping coordinates, defining direct mechanistic effects (Kasowski et al., 2010; McDaniell et al., 2010) and the definition cis-acting connections of distal elements, suggesting their regulatory relationships (Gibbs et al., 2010; Zhang et al., 2010). While the first is identifying additional layers of regulation controlling the activity of a specific locus (possibly being directly affected by the genetic variation; McDaniell et al., 2010; Boyle et al., 2012; Schaub et al., 2012; Paul et al., 2013), the latter is defining a sequential chain of events, strongly suggesting functional importance. This Review will focus on the relationship between cis-associated distal elements, as particularly these interactions provide superior information compared to on-site variation. Indeed, cis-associations not only define local activity, but determine functionality in downstream processes.
Despite the wealth of regulatory mechanisms, this Review focuses on DNA methylation, as the covalent modification of cytosines in a CpG context presents a stable and reliably detectable epigenetic mark with high impact on gene regulation (Jones, 2012). Generally, the DNA hypermethylation, in particular in promoter regions, was associated to transcriptional silencing, whereas promoter hypomethylation favors gene expression. In contrary, the DNA methylation levels in the gene body often correlate positively with transcriptional activity (Jones, 2012; Kulis et al., 2012). Importantly from an integrative perspective, DNA methylation levels were shown to be partially dependant on the underlying genetic sequence defined as DNA methylation quantitative trait loci (meQTL; Kerkel et al., 2008; Shoemaker et al., 2010). Herein, CpG methylation levels present high correlations with the genotype of non-overlapping nucleotides. Specifically, DNA methylation levels appear to segregate with genotypes in their proximity (cis-acting) rather than being farther distributed, suggesting the contribution of the local genomic environment to establish the connections (Shoemaker et al., 2010; Grundberg et al., 2013; Heyn et al., 2013). Although specific mechanisms establishing the association between the genetic and epigenetic code are widely unknown, their identification suggested causal connections between genetic and epigenetic information. From a genomic perspective, meQTL present a powerful surrogate mark to elucidate functional and mechanistic implementations of phenotype-associated genetic variations.
A Cascade of Genetic and Epigenetic Regulation
The fact that meQTL are mainly cis-acting and located in proximity to their related epigenetic variants, suggests that local regulatory mechanisms participate to establish the associations. A possible scenario involves distal regulative features, such as enhancer regions, whose proximity to gene promoters is exhibited through chromatin looping (Figure 1). Enhancer activity triggers measurable downstream events such as changes in transcriptional activity or in interconnected regulatory mechanisms, including DNA methylation. Consequently, genetic variants affecting enhancer activity, by altering the affinity of DNA binding factors (Kasowski et al., 2010) or chromatin formation (McDaniell et al., 2010), alter downstream cascades, detectable as epigenetic or eQTL. Importantly, these connections by themselves might define a functional relevance of both components, determined by a traceable chain of events. In this respect, meQTL are unlikely to represent secondary events, but rather display an important mechanism in a causal chain that provokes variability. Consistently, meQTL were suggested to present an intermediate regulator that mediates phenotypic plasticity and providing a powerful mechanism for evolutionary adaptation in changing environments (Feinberg and Irizarry, 2010).
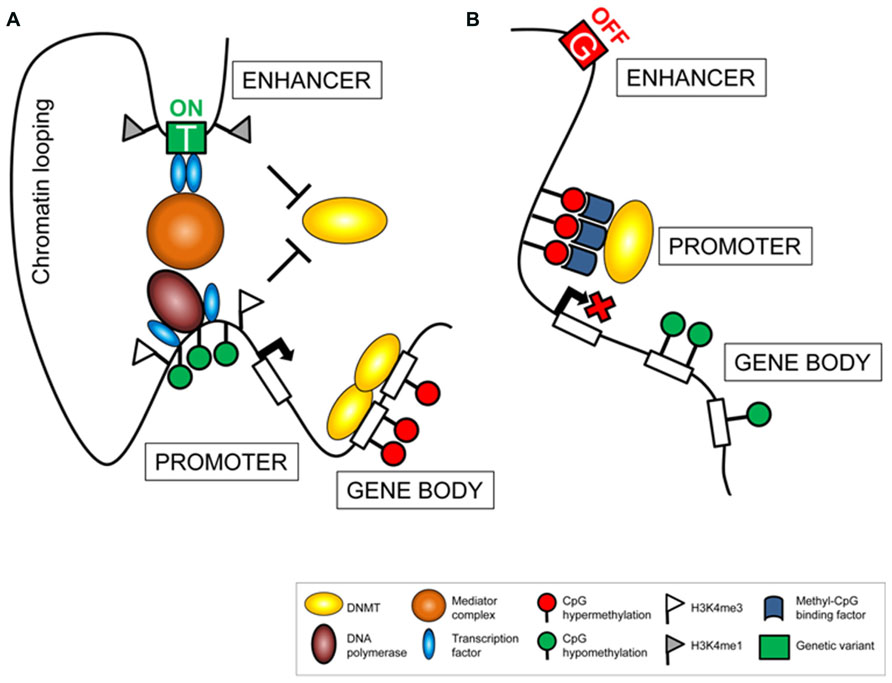
FIGURE 1. Genetic variance affects the regulatory machinery. Polymorphic alleles located in cis-regulating elements (e.g., enhancer sites) can be associated to variation in downstream cascades resulting in transcriptional activation (A) or silencing (B). Changes in gene regulation can be assessed measuring variance in gene expression and related regulatory factors, such as epigenetic modifications (e.g., DNA methylation) or occupancy of regulatory proteins (e.g. transcription factors).
The concept of meQTL defined by genome-scale data integration was initially introduced in two independent studies, profiling matched genotype, epitype, and gene expression information of brain tissue samples. Both studies, analyzed samples from different brain regions (Gibbs et al., 2010) and cerebellum (Zhang et al., 2010), respectively, determining genetically variable loci significantly associated to DNA methylation levels at distal gene promoters. Ergo, the authors suggested a functional and causal relationship between both levels of information with the genetic sequence influencing DNA methylation levels in cis or trans. Surprisingly, meQTL rarely overlapped with eQTLs, wherein transcriptional activity correlated with the underlying genotypes, suggesting meQTL and eQTLs as independent features. This observation was confirmed in studies of different tissue-types that concluded a frequent dependence of DNA methylation levels on the genetic background, however, with the majority not directly associated to expression changes (Grundberg et al., 2013). In this regard, we suggest interconnected DNA methylation and genetic sequence to provide a basal regulatory setting, poising genes and loci for activation. The actual gene activity, however, can be limited to specific cellular contexts. Consistently, a recent study integrating gene expression, DNA methylation and genotype data from three different tissue-types observed a complex relationship between the three features and questioning a strict linear cause-mediator-consequence relationship (Gutierrez-Arcelus et al., 2013).
Epigenetic Variance Facilitates GWAS Interpretation
In light of genome-wide association studies (GWAS), meQTL facilitate the interpretation of non-coding genetic variability and their association to phenotypic differences (Freedman et al., 2011; Hernandez and Singleton, 2012; Kilpinen and Dermitzakis, 2012). Recent studies have given an outlook of the potential of integrative genome-epigenome studies for the meaningful interpretation of genetic risk alleles (Gamazon et al., 2013; Scherf et al., 2013). Further, genotype–epitype associations guided the interpretation of physiological traits, such as natural human variation (Heyn et al., 2013) or aging (Bell et al., 2012). Moreover, in addition to guiding the interpretation of the genetic code, functional linkage could, vice versa, identify epigenetic driver events from often numerous differentially methylated regions (Liu et al., 2013). This symbiotic liaison highlights the power of functional linkage analysis for the meaningful mining of genetic and epigenetic variance in physiological and pathological contexts. Summarizing the current knowledge from integrative genotype–epitype analyses, the following examples also underscore the use of multidimensional datasets and their power to identify phenotype driving events.
Brain associated meQTL were shown to be implemented in neurological disease pathology and enabled the interpretation of GWAS results derived from bipolar disorders (Gamazon et al., 2013). Briefly, polymorphic risk alleles obtained from different genetic studies, revealed enrichment in cis-acting brain meQTL, presenting prior unrecognized disease-related genes. Importantly, incorporating a priori information about meQTL increased the power of detecting genotype–phenotype associations. By restricting the analysis to cis-acting meQTL, the authors detected a significant association between rs12618769 and bipolar disorder, which was not significant in a genome-wide screening. Intriguingly, the polymorphism was associated to differences in promoter methylation of inositol polyphosphate phosphatase 4A (INPP4A), a gene related to the functional integrity of the brain. Thus, a data set reduction to functionally connected variants enabled the identification of aberrant gene regulation with possible implication in the biology of bipolar disorders. The study presents a paradigm of how the complexity observed in genome- and epigenome-wide association studies can be reduced to loci with likely functional relevance in the given context. As initial filter steps reduce the number of required tests (which need to be corrected for in multiple hypotheses testing), functionality-driven approaches might be applicable with substantial lower sample numbers.
Following the objective to interpret GWAS, defined risk variants through epigenetic data integration, another recent study revealed differences in DNA methylation in a region previous defined as lung cancer risk locus (Scherf et al., 2013). In particular, the authors identified a significant association of the risk genotype with differential promoter methylation of the nicotinic acetylcholine receptor subunit gene CHRNA4, suggesting a mechanistic disease-related cascade induced by the genetic variant. Most importantly, following the validation of a direct association to transcriptional regulation, the authors confirmed the impact of increased CHRNA4 expression on oncogenesis in lung tumor cells. Conclusively, the epigenetic activation of the CHRNB4 correlated with the presence of genetically defined lung cancer susceptibility variants, suggesting a causal relationship between different layers of regulation in the risk allele biology.
Combining comprehensive geno- and epityping screening efforts, an adipose tissue based study detected a relationship between a metabolic disease risk loci and variant DNA methylation (Grundberg et al., 2013). Particularly, they determined the significant correlation between a SNP (rs713586) associated with body mass index and DNA methylation in an enhancer region upstream of the adenylate cyclase 3 (ADCY3), a gene previously linked to obesity. Intriguingly, a SNP in perfect linkage disequilibrium directly flanking the altered CpG overlapped transcription factor binding sites, presenting potential mechanistic consequences of the genetic variant. Consistently, transcription factor binding was previously shown to influence DNA methylation levels at distal regulatory elements (Stadler et al., 2011).
Epigenetic Variance Guides Interpretation of Natural Human Variation
Interestingly, the strategy of functional linkage analysis was also proven to be informative outside the disease context using variability observed between different individuals or human populations (Bell et al., 2011; Fraser et al., 2012; Heyn et al., 2013; Moen et al., 2013). Comparing three different human populations, a blood based study determined differentially methylated CpG sites between populations and confirmed the genetic blueprint to be the major factor determining epigenetic differences (Heyn et al., 2013). In particular, the study detected variance in DNA methylation between African, European, and Asian individuals with potential consequences on distinct phenotypes, including differences in drug response or susceptibility to pathogen infections. Although the variance is likely to be transmitted genetically, epigenetically defined phenotypes are exposed to the natural selection process. Accordingly, a subset of differentially methylated genes revealed evidence of local selection pressure. Most interestingly from a integrative perspective, the study determined genetic variants, associated to population-specific DNA methylation, to be enriched for SNPs previously identified as risk loci for the infection with the hepatitis B virus (HBV). Consistently, the risk SNPs were more abundant in Asian and African individuals, ethnicities for which the HBV infection presents an endemic disease. By integrating DNA methylation data, the study determined a chain of genetic and epigenetic events leading to variant HLA-DPA1 expression, likely to drive differences in HBV infection risk between the populations.
Similarly, another study analyzing blood samples from donors with African or European ancestry determined meQTL associated with complex traits such as racial disparities (Moen et al., 2013). In particular, an association between risk alleles for cardiovascular diseases and high cholesterol levels with differential promoter methylation of the apolipoprotein A-V (APOA5) illustrated the implication of meQTL in the biology of human diseases.
Conclusively, ethnicity based studies suggested DNA methylation to be an important intermediate regulator in the translational process from geno- to phenotypes. Furthermore, it represents a valuable information to meaningful interpret variability observed between populations, however, also proving its value to explain inter-individual variation.
Genetic Linkage Identifies Epigenetic Driver Events
Likely, the genetic code vice versa serves as an anchor point to extract functional important epigenetic variation from highly variant epigenomes. Considering the numerous epigenetic events in cancer, wherein multiple genes simultaneously gain or lose DNA methylation, the identification of epigenetic drivers presents an extremely difficult task. However, connecting DNA methylation data to additional layers of information, such as genotype or gene expression, suggests interconnected epigenetic events that are more likely to be of functional importance than unconnected alterations. In this regard, genetic connections could be of value to separate epigenetic driver from passenger events, and to simultaneously define novel risk genotypes and functional cancer genes. Herein, epitype–genotype associations represent a role-model for the interplay between different cellular mechanisms, whose interpretation will certainly provide a rich resource for disease biomarkers and strategic nodes for therapeutic intervention.
The concept of data integration for the meaningful interpretation of genome-scale DNA methylation data was successfully applied using meQTL to determine functional epigenetic events and novel genetic risk loci in rheumatoid arthritis patients (Liu et al., 2013). Specifically, the study determined differentially methylated CpG sites between patients and controls, and subsequently assessed potential genetic risk loci based on their association to aberrantly methylated CpG sites. Defining genotype as causal factor, DNA methylation as mediator and the disease as outcome, the authors assumed direct relationships between these features, which enabled the identification of genetic risk polymorphism and their underlying chain of events leading to rheumatoid arthritis. In particular, the authors defined several significant associations located within the major histocompatibility complex (MHC), genes previously related to disease risk to rheumatoid arthritis. In addition, the study identified DNA methylation levels of the glutathione S-transferase alpha 2 (GSTA2) promoter region to be under genetic control. In line with the results, genetic variance in GSTA2 family members, such as GSTT1, GSTM1 and GSTP1, have been previously reported to predict arthritis risk and severity.
Using an integrative approach, the authors identified novel genetic risk variants. The strategy also enabled them to distinguish disease driving epigenetic events from those that might have been a consequence of the disease itself. Thus, assuming a causal relationship between the genetic and epigenetic code, functional linkage analysis is applicable for the simultaneous identification of genetic and epigenetic disease-driving events.
Integrative Production Pipelines in International Consortia
Highlighting the informative value of associations between the genotype and epigenetic regulation, this Review aimed to illustrate the power of data integration in the genomic era. Although presenting important information itself, genotype data only reveals its real potential after determining its interaction within the cellular context. Herein, phenotype relationships detected in GWA studies only define the endpoint in a network of intensively cross-linked cascades of events; and it is in particular their identification that enables a meaningful interpretation of significant statistical connections. Hence, considering currently ongoing massive data production efforts, we suggest a high value for the simultaneous screening of multiple layers of cellular information. In addition to providing important information for their respective research fields, their integration in multidimensional analysis pipelines will further improve meaningful readouts. Eventually, combined data from different sources of information will provide more value than the sum of its individual components.
In view of the presented examples, we encourage international multidisciplinary research consortia to continue their strategies aiming to comprehensively profile all aspects of the human genome. Although the need for simultaneous production of different data types is widely accepted and moved forward in consortia like the International Cancer Genome Consortium (ICGC, icgc.org), integrative analysis pipelines are still immature and standards still remain elusive. However, first computational tools for the integration of genotype data are developed and freely available to the broad research community (He et al., 2013; Zhang et al., 2014).
Moreover, significant associations between different layers of information need to be annotated in common portals with open access for the community. While this has been partially achieved for genotype-expression associations (He et al., 2013), novel components could amplify the information provided, in order to improve our understanding of complex cellular connections and disease biology.
The association of DNA methylation and genetic sequence displays an exemplary application, which is further extendable to virtually all traceable cellular features, including proteomics or metabolomics, among many others. It will be the knowledge of these complex relationships that will drive future efforts to resolve the conundrum of human variation in physiological and pathological contexts.
Conflict of Interest Statement
The author declares that the research was conducted in the absence of any commercial or financial relationships that could be construed as a potential conflict of interest.
Acknowledgments
The research leading to these results has received funding from the European Community’s Seventh Framework Programme (FP7/2007–2013) under grant agreement no. HEALTH-F5-2011-282510 – BLUEPRINT project and the Sandra Ibarra Foundation under IV ghd Grants for breast cancer research. Holger Heyn is a Juan de la Cierva Researcher (MINECO ref. JCI-2012-15312).
References
Bell, J. T., Pai, A. A., Pickrell, J. K., Gaffney, D. J., Pique-Regi, R., Degner, J. F., et al. (2011). DNA methylation patterns associate with genetic and gene expression variation in HapMap cell lines. Genome Biol. 12:R10. doi: 10.1186/gb-2011-12-1-r10
Bell, J. T., Tsai, P.-C., Yang, T.-P., Pidsley, R., Nisbet, J., Glass, D., et al. (2012). Epigenome-wide scans identify differentially methylated regions for age and age-related phenotypes in a healthy ageing population. PLoS Genet. 8:e1002629. doi: 10.1371/journal.pgen.1002629
Bernstein, B. E., Birney, E., Dunham, I., Green, E. D., Gunter, C., and Snyder, M. (2012). An integrated encyclopedia of DNA elements in the human genome. Nature 489, 57–74. doi: 10.1038/nature11247
Boyle, A. P., Hong, E. L., Hariharan, M., Cheng, Y., Schaub, M. A., Kasowski, M., et al. (2012). Annotation of functional variation in personal genomes using RegulomeDB. Genome Res. 22, 1790–1797. doi: 10.1101/gr.137323.112
Consortium, T. E. P. (2012). An integrated encyclopedia of DNA elements in the human genome. Nature 489, 57–74. doi: 10.1038/nature11247
Feinberg, A. P., and Irizarry, R. A. (2010). Evolution in health and medicine Sackler colloquium: stochastic epigenetic variation as a driving force of development, evolutionary adaptation, and disease. Proc. Natl. Acad. Sci. U.S.A. 107(Suppl. 1), 1757–1764. doi: 10.1073/pnas.0906183107
Fraser, H. B., Lam, L., Neumann, S., and Kobor, M. S. (2012). Population-specificity of human DNA methylation. Genome Biol. 13:R8. doi: 10.1186/gb-2012-13-2-r8
Freedman, M. L., Monteiro, A. N. A., Gayther, S. A., Coetzee, G. A., Risch, A., Plass, C., et al. (2011). Principles for the post-GWAS functional characterization of cancer risk loci. Nat. Genet. 43, 513–518. doi: 10.1038/ng.840
Gamazon, E. R., Badner, J. A., Cheng, L., Zhang, C., Zhang, D., Cox, N. J., et al. (2013). Enrichment of cis-regulatory gene expression SNPs and methylation quantitative trait loci among bipolar disorder susceptibility variants. Mol. Psychiatry 18, 340–346. doi: 10.1038/mp.2011.174
Garraway, L. A., and Lander, E. S. (2013). Lessons from the cancer genome. Cell 153, 17–37. doi: 10.1016/j.cell.2013.03.002
Gibbs, J. R., van der Brug, M. P., Hernandez, D. G., Traynor, B. J., Nalls, M. A., Lai, S.-L., et al. (2010). Abundant quantitative trait loci exist for DNA methylation and gene expression in human brain. PLoS Genet. 6:e1000952. doi: 10.1371/journal.pgen.1000952
Grundberg, E., Meduri, E., Sandling, J. K., Hedman, A. K., Keildson, S., Buil, A., et al. (2013). Global analysis of DNA methylation variation in adipose tissue from twins reveals links to disease-associated variants in distal regulatory elements. Am. J. Hum. Genet. 93, 876–890. doi: 10.1016/j.ajhg.2013.10.004
Grundberg, E., Small, K. S., Hedman, Å. K., Nica, A. C., Buil, A., Keildson, S., et al. (2012). Mapping cis- and trans-regulatory effects across multiple tissues in twins. Nat. Genet. 44, 1084–1089. doi: 10.1038/ng.2394
Gutierrez-Arcelus, M., Lappalainen, T., Montgomery, S. B., Buil, A., Ongen, H., Yurovsky, A., et al. (2013). Passive and active DNA methylation and the interplay with genetic variation in gene regulation. Elife 2:e00523. doi: 10.7554/eLife.00523
He, X., Fuller, C. K., Song, Y., Meng, Q., Zhang, B., Yang, X., et al. (2013). Sherlock: detecting gene-disease associations by matching patterns of expression QTL and GWAS. Am. J. Hum. Genet. 92, 667–680. doi: 10.1016/j.ajhg.2013.03.022
Hernandez, D., and Singleton, A. (2012). Using DNA methylation to understand biological consequences of genetic variability. Neurodegener. Dis. 9, 53–59. doi: 10.1159/000333097
Heyn, H., Moran, S., Hernando-Herraez, I., Sayols, S., Gomez, A., Sandoval, J., et al. (2013). DNA methylation contributes to natural human variation. Genome Res. 23, 1363–1372. doi: 10.1101/gr.154187.112
Hindorff, L. A., Gillanders, E. M., and Manolio, T. A. (2011). Genetic architecture of cancer and other complex diseases: lessons learned and future directions. Carcinogenesis 32, 945–954. doi: 10.1093/carcin/bgr056
Hnisz, D., Abraham, B. J., Lee, T. I., Lau, A., Saint-André, V., Sigova, A. A., et al. (2013). Super-enhancers in the control of cell identity and disease. Cell 155, 934–947. doi: 10.1016/j.cell.2013.09.053
Jones, P. A. (2012). Functions of DNA methylation: islands, start sites, gene bodies and beyond. Nat. Rev. Genet. 13, 484–492. doi: 10.1038/nrg3230
Kaplan, N., Moore, I. K., Fondufe-Mittendorf, Y., Gossett, A. J., Tillo, D., Field, Y., et al. (2009). The DNA-encoded nucleosome organization of a eukaryotic genome. Nature 458, 362–366. doi: 10.1038/nature07667
Kasowski, M., Grubert, F., Heffelfinger, C., Hariharan, M., Asabere, A., Waszak, S. M., et al. (2010). Variation in transcription factor binding among humans. Science 328, 232–235. doi: 10.1126/science.1183621
Kerkel, K., Spadola, A., Yuan, E., Kosek, J., Jiang, L., Hod, E., et al. (2008). Genomic surveys by methylation-sensitive SNP analysis identify sequence-dependent allele-specific DNA methylation. Nat. Genet. 40, 904–908. doi: 10.1038/ng.174
Kilpinen, H., and Dermitzakis, E. T. (2012). Genetic and epigenetic contribution to complex traits. Hum. Mol. Genet. 21, R24–R28. doi: 10.1093/hmg/dds383
Kulis, M., Heath, S., Bibikova, M., Queirós, A. C., Navarro, A., Clot, G., et al. (2012). Epigenomic analysis detects widespread gene-body DNA hypomethylation in chronic lymphocytic leukemia. Nat. Genet. 44, 1236–1242. doi: 10.1038/ng.2443
Lappalainen, T., Sammeth, M., Friedländer, M. R., ’t Hoen, P. A. C., Monlong, J., Rivas, M. A., et al. (2013). Transcriptome and genome sequencing uncovers functional variation in humans. Nature 501, 506–511. doi: 10.1038/nature12531
Li, Q., Seo, J.-H., Stranger, B., McKenna, A., Pe’er, I., Laframboise, T., et al. (2013). Integrative eQTL-based analyses reveal the biology of breast cancer risk loci. Cell 152, 633–641. doi: 10.1016/j.cell.2012.12.034
Li, Y., Sheu, C.-C., Ye, Y., de Andrade, M., Wang, L., Chang, S.-C., et al. (2010). Genetic variants and risk of lung cancer in never smokers: a genome-wide association study. Lancet Oncol. 11, 321–330. doi: 10.1016/S1470-2045(10)70042-5
Lienert, F., Wirbelauer, C., Som, I., Dean, A., Mohn, F., and Schübeler, D. (2011). Identification of genetic elements that autonomously determine DNA methylation states. Nat. Genet. 43, 1091–1097. doi: 10.1038/ng.946
Liu, Y., Aryee, M. J., Padyukov, L., Fallin, M. D., Hesselberg, E., Runarsson, A., et al. (2013). Epigenome-wide association data implicate DNA methylation as an intermediary of genetic risk in rheumatoid arthritis. Nat. Biotechnol. 31, 142–147. doi: 10.1038/nbt.2487
McDaniell, R., Lee, B.-K., Song, L., Liu, Z., Boyle, A. P., Erdos, M. R., et al. (2010). Heritable individual-specific and allele-specific chromatin signatures in humans. Science 328, 235–239. doi: 10.1126/science.1184655
Moen, E. L., Zhang, X., Mu, W., Delaney, S. M., Wing, C., McQuade, J., et al. (2013). Genome-wide variation of cytosine modifications between European and African populations and the implications for complex traits. Genetics 194, 987–996. doi: 10.1534/genetics.113.151381
Paul, D. S., Albers, C. A., Rendon, A., Voss, K., Stephens, J., HaemGen Consortium, et al. (2013). Maps of open chromatin highlight cell type-restricted patterns of regulatory sequence variation at hematological trait loci. Genome Res. 23, 1130–1141. doi: 10.1101/gr.155127.113
Schaub, M. A., Boyle, A. P., Kundaje, A., Batzoglou, S., and Snyder, M. (2012). Linking disease associations with regulatory information in the human genome. Genome Res. 22, 1748–1759. doi: 10.1101/gr.136127.111
Scherf, D. B., Sarkisyan, N., Jacobsson, H., Claus, R., Bermejo, J. L., Peil, B., et al. (2013). Epigenetic screen identifies genotype-specific promoter DNA methylation and oncogenic potential of CHRNB4. Oncogene 32, 3329–3338. doi: 10.1038/onc.2012.344
Schübeler, D. (2012). Molecular biology. Epigenetic islands in a genetic ocean. Science 338, 756–757. doi: 10.1126/science.1227243
Shoemaker, R., Deng, J., Wang, W., and Zhang, K. (2010). Allele-specific methylation is prevalent and is contributed by CpG-SNPs in the human genome. Genome Res. 20, 883–889. doi: 10.1101/gr.104695.109
Stadler, M. B., Murr, R., Burger, L., Ivanek, R., Lienert, F., Schöler, A., et al. (2011). DNA-binding factors shape the mouse methylome at distal regulatory regions. Nature 480, 490–495. doi: 10.1038/nature10716
Weber, M., Davies, J. J., Wittig, D., Oakeley, E. J., Haase, M., Lam, W. L., et al. (2005). Chromosome-wide and promoter-specific analyses identify sites of differential DNA methylation in normal and transformed human cells. Nat. Genet. 37, 853–862. doi: 10.1038/ng1598
Yuan, G.-C. (2012). Linking genome to epigenome. Wiley Interdiscip. Rev. Syst. Biol. Med. 4, 297–309. doi: 10.1002/wsbm.1165
Zhang, D., Cheng, L., Badner, J. A., Chen, C., Chen, Q., Luo, W., et al. (2010). Genetic control of individual differences in gene-specific methylation in human brain. Am. J. Hum. Genet. 86, 411–419. doi: 10.1016/j.ajhg.2010.02.005
Zhang, H., Shi, J., Liang, F., Wheeler, W., Stolzenberg-Solomon, R., and Yu, K. (2014). A fast multilocus test with adaptive SNP selection for large-scale genetic-association studies. Eur. J. Hum. Genet. 22, 696–702. doi: 10.1038/ejhg.2013.201
Keywords: DNA methylation quantitaive trait loci, DNA methylation, GWAS, EWAS, epigenetic regulation
Citation: Heyn H (2014) A symbiotic liaison between the genetic and epigenetic code. Front. Genet. 5:113. doi: 10.3389/fgene.2014.00113
Received: 09 February 2014; Paper pending published: 25 March 2014;
Accepted: 15 April 2014; Published online: 01 May 2014.
Edited by:
Jeff Schwartz, Griffith University, AustraliaReviewed by:
Ian C. G. Weaver, Dalhousie University, CanadaJeff Schwartz, Griffith University, Australia
Guo-Cheng Yuan, Dana-Farber Cancer Institute, USA
Copyright © 2014 Heyn. This is an open-access article distributed under the terms of the Creative Commons Attribution License (CC BY). The use, distribution or reproduction in other forums is permitted, provided the original author(s) or licensor are credited and that the original publication in this journal is cited, in accordance with accepted academic practice. No use, distribution or reproduction is permitted which does not comply with these terms.
*Correspondence: Holger Heyn, Cancer Epigenetics and Biology Program, Bellvitge Biomedical Research Institute, Hospital Duran i Reynals, Avenida Gran Via de l’Hospitalet, 199-203, L’Hospitalet de Llobregat, 08907 Barcelona, Catalonia, Spain e-mail: hheyn@idibell.cat