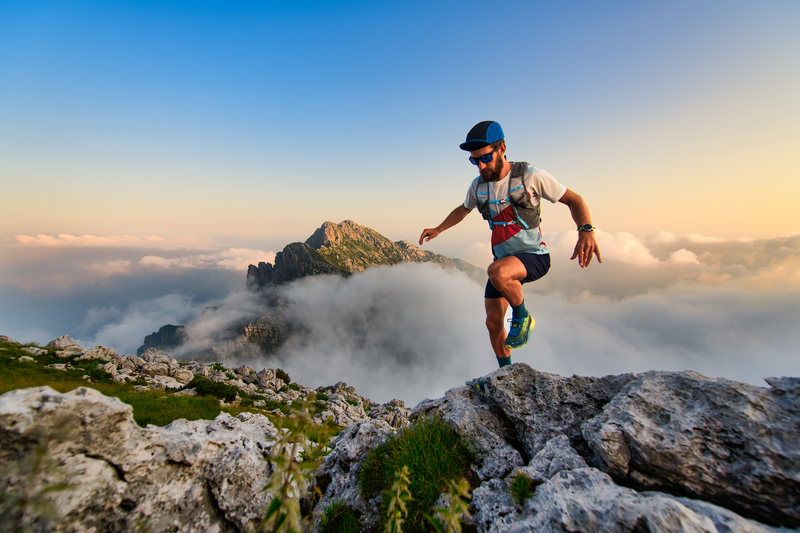
95% of researchers rate our articles as excellent or good
Learn more about the work of our research integrity team to safeguard the quality of each article we publish.
Find out more
ORIGINAL RESEARCH article
Front. Genet. , 18 December 2012
Sec. RNA
Volume 3 - 2012 | https://doi.org/10.3389/fgene.2012.00293
This article is part of the Research Topic Non-coding RNAs: Crossing the Aisle from Cancer to Neurodegeneration View all 39 articles
MicroRNAs (miRNAs) are known to be important post-transcriptional regulators that are involved in the etiology of complex psychiatric traits. The present study aimed to incorporate miRNAs information into pathway analysis using a genome-wide association dataset to identify relevant biological pathways for bipolar disorder (BPD). We selected psychiatric- and neurological-associated miRNAs (N = 157) from PhenomiR database. The miRNA target genes (miTG) predictions were obtained from microRNA.org. Canonical pathways (N = 4,051) were downloaded from the Molecule Signature Database. We employed a novel weighting scheme for miTGs in pathway analysis using methods of gene set enrichment analysis and sum-statistic. Under four statistical scenarios, 38 significantly enriched pathways (P-value < 0.01 after multiple testing correction) were identified for the risk of developing BPD, including pathways of ion channels associated (e.g., gated channel activity, ion transmembrane transporter activity, and ion channel activity) and nervous related biological processes (e.g., nervous system development, cytoskeleton, and neuroactive ligand receptor interaction). Among them, 19 were identified only when the weighting scheme was applied. Many miRNA-targeted genes were functionally related to ion channels, collagen, and axonal growth and guidance that have been suggested to be associated with BPD previously. Some of these genes are linked to the regulation of miRNA machinery in the literature. Our findings provide support for the potential involvement of miRNAs in the psychopathology of BPD. Further investigations to elucidate the functions and mechanisms of identified candidate pathways are needed.
Bipolar disorder (BPD) is a highly heritable psychiatric illness. The genetic components were estimated to account for as high as ∼80% of phenotypic variability (McGuffin et al., 2003). Although many candidate and genome-wide association (GWA) studies have conducted to investigate the complex nature of pathogenetics in BPD, previously reported genetic findings only account for a small proportion of its heritability (Gershon et al., 2011). The missing heritability may be partially explained by the limited numbers, types, and frequency of susceptible variants that currently genotyped in high-throughput array, and other mechanisms such as gene × gene or gene × environment interactions, as well as the heterogeneity in phenotype definitions across studies (Manolio et al., 2009). Nevertheless, large-scale GWA studies remain to be an efficient and promising study design to uncover the underlying etiology of complex psychiatric disorders (Sullivan and Investigators, 2012), while new theoretical framework and statistical approaches must be taken into consideration.
Recently, pathway-based analysis, which simultaneously tests a group of functionally related genes, has been widely used as an alternative and complementary strategy to bring more insights into the biological mechanisms of disease of interest (Wang et al., 2010). In addition, inclusion of prior information from other aspects, such as gene expression or gene regulation in GWAS analysis offers great opportunities to identify new association findings and to generate novel hypotheses (Tintle et al., 2009a). For instance, two prior GWA studies in type 2 diabetes and osteoporosis applied integrative approaches that used gene expression data and pathway-based analysis to identify novel associated pathways and loci (Hsu et al., 2010; Zhong et al., 2010). In addition to gene expression information, other types of data could also be incorporated into pathway-based analysis using GWA data, such as methylation (Chuang et al., 2012) and microRNAs (miRNAs) patterns, especially disease-associated miRNAs.
The miRNAs are one kind of functional non-coding RNAs acting as post-transcriptional regulators for translation and the stability of mRNAs, which involved in a wide range of biological processes, including regulation of brain and neuronal development (Fiore et al., 2008). The miRNA dysregulation has been reported to play important roles in the etiology of many diseases, including complex psychiatric traits (Xu et al., 2010). Previously, many psychiatric- and neurological-associated miRNAs were identified from expression studies of postmortem brain and animal models (Forero et al., 2010), and from genetic association studies of variants in genes encoding miRNAs and binding site of miRNAs target genes (Muinos-Gimeno et al., 2009; The Schizophrenia Psychiatric Genome-Wide Association Study (GWAS) Consortium, 2011).
We have known that an individual miRNA could target hundreds of mRNA molecules (Lim et al., 2005), therefore the target genes of a phenotype-related miRNA may potentially associate with the trait. For example, abnormal expression level of brain-expressed miR-132 has been reported to be associated with psychiatric disorders via affecting the expression of brain-derived neurotrophic factor that is involved in dendritic plasticity (Klein et al., 2007; Wayman et al., 2008; Askland et al., 2009; Forero et al., 2010). In addition, different psychiatric disorders (e.g., schizophrenia and BPD) may share some degree of the genetic factors through the involvement of similar biological pathways. Thus, a single miRNA may play roles in the etiology of more than one psychiatric disorder. Given these features of miRNAs, it is our interest to incorporate information of phenotype-related miRNAs and their predicted targets into the GWA analysis, which provide a new avenue for researchers to investigate the underlying genetic components that are associated with BPD.
In the current study, we performed pathway-based analysis using large-scale GWA dataset of BPD in combination with the data source of miRNAs. The psychiatric- and neurological-associated miRNAs were identified from PhenomiR database and miRNA target predictions were obtained from microRNA.org database. Our main goal is to better identify genes and important biological pathways to be associated with BPD while incorporating the regulatory information of miRNAs.
Figure 1 shows the data integration flowchart in our study to identify the psychiatric- and neurological-associated miRNAs and their target genes, and the annotated pathways for pathway-based analysis. Details are mentioned below.
Figure 1. The flowchart of identification of psychiatric- and neurological-associated miRNAs and their target genes and information for pathway analysis.
The Genetic Association Information Network (GAIN) GWA dataset was downloaded from dbGaP1. We extracted BPD dataset from the GAIN: full details of subject enrollment and genotyping can be obtained in the original article (The GAIN Collaborative Research Group, 2007). The GWA dataset of BPD comprised 1,001 BPD cases and 1,034 controls, which used Affymetrix Genome-Wide Human SNP Array 6.0 platform for SNP (single nucleotide polymorphism) genotyping. After applying quality control filters and excluding SNPs in sexual chromosomes, there were 698,227 autosomal SNPs in the GWA dataset in our analyses.
Information of disease-associated miRNAs was downloaded from the PhenomiR database (Ruepp et al., 2010). The PhenomiR collected published miRNA-disease associations via manual curation. It annotates diseases into 22 classes according to the Online Mendelian Inheritance in Man (OMIM) Morbid Map. In our analysis, we selected all miRNAs that are associated with neurological and psychiatric classes as the candidates of disease-associated miRNAs. In total, there were 157 unique miRNAs to form 293 miRNA-disease association pairs.
The miRNA target predictions were obtained from micorRNA.org database2 (Betel et al., 2008). The micorRNA.org performed miRNA target prediction using miRanda algorithm (John et al., 2004) and scored the likelihood of mRNA downregulation of predicted target sites by using mirSVR algorithm (Betel et al., 2010). The combination of miRanda-mirSVR approach has been shown to effectively identify target predictions to cover a significant number of non-canonical sites, and has competitive ability in predicting expression changes of mRNA or proteins when comparing with other target prediction methods (Betel et al., 2010). In total, 1,097,064 “good mirSVR score-conserved miRNA” target predictions were used in this procedure. Combining these two datasets while follows the criteria of alignment score ≥140, seed site ≥6, free energy ≤−17, and conservation score ≥0.57 (Figure 1), we identified 8,921 genes which were predicted to be the targets of psychiatric- and neurological-associated miRNAs.
We also used another miRNA target prediction algorithm, DIANA-microT, which considers not only strong binding (at least seven consecutive Watson-Crick base pairing nucleotides) but also weak binding ability (only six paired nucleotides or G:U wobble pairs) to predict the miRNA target genes (miTG; Maragkakis et al., 2009a,b). DIANA-microT provides scores for miTG as an indicator for the probability of being a real target site. The calculation of an overall miTG score mainly based on scoring all binding types and conservation profile of all putative miRNA recognition element (MRE) within the 3′UTR using the weighted sum method. Therefore, target genes of psychiatric- and neurological-associated miRNAs with high predictive probability in significant pathways were filtered by the DIANA-microT algorithm. We used a miTG score greater than 19 (a strict threshold) as the selection criterion, which implicates the predicted target was highly reliable being a true miRNA target.
We used PLINK (version 1.07) to conduct single marker association analyses with additive model (Purcell et al., 2007). We first mapped SNPs to genes to obtain gene-level statistic for BPD using the GWA dataset in GAIN. SNPs were mapped to genes if they located within 5 kb of the 5′ upstream and 3′ downstream of a gene using NCBI human genome build 36. For each gene, the smallest P-value (Pmin) among all SNPs within the gene region was used to represent the gene-level statistic. In total, there were 304,343 SNPs assigned into 16,385 genes in the GWA dataset of BPD.
Annotated pathways were obtained from the Molecule Signature Database, MsigDB (Subramanian et al., 2005). MsigDB consists of several online pathway databases, including Kyoto Encyclopedia of Genes and Genomes (KEGG), BioCarta, Reactome, Gene Ontology (GO) terms, and gene sets collected from published literature. From MsigDB, we obtained 4,726 pathways that cover 22,429 genes. Pathways containing less than 10 genes or more than 380 genes were excluded to avoid bias due to extreme small or large pathway size. Thus, there were 4,051 canonical pathways in the pathway-based analyses using the GWA dataset of BPD in the present study.
Pathway-based analyses were conducted using both competitive and self-contained approaches (Wang et al., 2010) to capture a broader range of important pathways. The gene set enrichment analysis (GSEA), as a competitive method, first ranks Pmin values of all genes from the smallest to the largest. Then, for a given pathway, an enrichment score (ES) was calculated based on gene-wise statistic values (tj), which were defined as the χ2 statistic of the corresponding most significant SNP to evaluate association signals (Wang et al., 2007). The sum-statistic (SUM) approach, as a self-contained test, sums up gene-wise statistic values (ti) over the set of genes in a specific pathway (Tintle et al., 2009b). The details of calculation procedures were provided in our previous study (Kao et al., 2012).
We employed a weighting scheme for genes that were predicted to be psychiatric- and neurological-associated miRNA targets in every annotated pathway. First, we calculated the overall proportion of SNPs with P-value <0.05 in the whole GAIN dataset. Second, in a given gene, the proportion of significant SNPs was calculated and then compared with the proportion of significant SNPs in the whole GWA dataset to evaluate whether this gene was informative. The detail of weighting procedures was described as below. For each pathway, n and m represent the number of miTGs and non- miTGs, respectively. Kn and Km were the number of informative genes in the n miTGs and m non-miTGs, respectively. Therefore, the proportion of informative genes in the miTGs and non-miTGs were Kn/n and Km/m. The Harmonic average (H), defined as 1/[(1/mKn) + (1/nKm)], was used as the basis of our weighting scheme for calculating the gene-wise weights of the miRNA and non-miTGs in a pathway and to minimize the potential bias in the pathway analysis due to the variation of pathway size. If Kn/n was greater than Km/m, the weights for miTGs and non-miTGs were assigned mkn/H and nkm/H, respectively. If no informative genes exist in non-miRNA genes, a weight one was assigned to non-miRNA genes; while a weight, ranging from one to six, according to the proportion of informative genes (using 0.1, 0.3, 0.5, 0.7, and 0.9 as cut-off values), was assigned to miRNA genes. Otherwise, equal weights were used for the two sets of genes.
For each pathway, weights were assigned to miTGs and non-miTGs. Then all genes were classified into S set and NS set according to their involvement in a pathway or not. When using competitive methods, genes within a pathway were compared with genes not within the pathway. Regarding to self-contained methods, only genes within the pathway were considered. A total of 5,000 permutations were performed to evaluate the empirical significance level of each pathway. To account for multiple testing issues in the analyses, algorithm proposed by Benjamini and Hochberg (1995) was used to control for false discovery rate (FDR).
A total of 4,051 pathways were constructed and tested for associations with the risk of BPD using the GWA dataset of BPD. With the inclusion of 157 psychiatric- and neurological-associated miRNAs as the prior information into pathway-based analyses, we identified many enriched pathways for BPD. Under four testing scenarios, including weighted and non-weighted GSEA and SUM statistics, there were more than 100 significant pathways associated with BPD at the level of empirical P-value < 0.01, and the number was reduced to more than 40 after FDR correction (Table A1 in Appendix). Comparing with non-weighting scenario, pathway analysis under the weighting scenario identified additional 20 and 223 significant pathways (FDR < 0.01) by using GSEA and SUM methods, respectively (Table A2 in Appendix). Under the non-weighted scheme, the number of enriched pathways identified by both the GSEA and SUM methods with FDR < 0.01 was 43, while the number was 38 under the weighted scheme. The union set of these enriched pathways were in total 62 pathways (Table A3 in Appendix), including 18 annotated GO, 7 KEGG, and 37 curated gene sets. Among these pathways, 19 significant pathways were identified by both the GSEA and SUM methods using both non-weighted and weighted scheme.
Table 1 showed 19 enriched pathways with stringent criterion of FDR < 0.01, including four GO gene sets and 15 curated gene sets, which exhibited strong associations with BPD under all four statistical scenarios. The three significant GO gene sets, cation transmembrane transporter activity, gated channel activity, and ion transmembrane transporter activity, were ion channel/transporter related. The fourth GO gene set was nervous system development, which was reported to be associated with BPD previously. After performing our weighting scheme, 19 additional pathways were identified at the significance level of FDR < 0.01 (Table 2), including six annotated GO, 3 KEGG, and 10 curated gene sets. Many of them are novel findings for BPD, such as cytoskeleton, retinol metabolism, drug metabolism other enzymes, etc. In total, we found 38 significant enriched pathways for BPD.
Table 1. Enriched pathways with FDRBH < 0.01 under weighted and non-weighted scenarios using GSEA and SUM methods.
Table 2. Enriched pathways with FDRBH<0.01 level under weighting scheme using both GSEA and SUM methods.
The results of miRNA target predictions in 38 enriched pathways were further examined. Initially, miRNA target prediction was performed by using miRanda-mirSVR approach with previously described filtering criteria (Figure 1). There were 2,438 unique genes in 38 significantly enriched pathways. Among them, 546 had Pmin-value less than 0.01. Table 3 summarized the results of miRNA target predictions for these 546 genes. On average, 34.4% of the predicted miTGs had Pmin-value less than 0.01 in enriched pathways, indicating a higher probability of showing associations with BPD. As expected, the larger the numbers of genes or the numbers of miTGs in a pathway, the higher number of miRanda-mirSVR prediction was observed (correlation coefficient = 0.74 and 0.82, respectively) in the 38 enriched pathways. We then applied the second prediction algorithm, DIANA-microT, to increase the stringency of target genes prediction. These results were also shown in Table 3. By using the strict threshold at miTG score 19, we filtered out the predictions with less probability of correct prediction. Among the 38 pathways, as high as 88.9% of the miRanda-mirSVR predictions could be also predicted by DIANA-microT (ranged from 36.8 to 88.9%). The numbers of miRNA target predictions were also reduced (from 0 to 28). In total, there were 469 miRNA target predictions with miTG score >19 for genes with Pmin-value of target gene <0.01, which consisted of 113 unique genes and 45 miRNAs. Among these predictions, 22 miTGs were involved in more than three enriched pathways. The 22 miTGs and their corresponding associated miRNAs are displayed in Table 4. The functions of these genes are mainly related to potassium and calcium ion channels (e.g., KCNMA1, KCNQ5, KCNK2, PKD2, and RYR3), collagen (e.g., COL1A2, COL27A1, and COL5A1), and axon guidance (e.g., NF1B, NAV3, and PTPRD).
Table 3. Predictions of psychiatric- and neurological-associated miRNA target genes with Pmin < 0.01 in 38 significantly enriched pathways.
Analyzing GWA dataset with pathway-based approach utilizes information of multiple loci with similar physiological functions to bring biological insights into the mechanisms of BPD (Torkamani et al., 2008; Askland et al., 2009; Holmans et al., 2009; Peng et al., 2010). Integrating other data sources into the analysis framework further offers more opportunities in identifying disease-associated loci (Wang et al., 2010). The current study especially focuses on information obtained from miRNAs, which are essential in the regulation processes of brain and neuronal development. We performed pathway-based analyses using a GWA dataset of BPD while incorporating the disease-associated miRNA information into analysis. Many important pathways were identified through our analysis framework.
First, four enriched GO terms were identified for BPD, including cation transmembrane transporter activity, gated channel activity, ion transmembrane transporter activity, and nervous system development. Three of them are ion channel/transporter related. Adding the weighting scheme by miRNA information, we further identified two channel-related pathways (Table 2), ion channel activity and substrate specific channel activity. The involvement of ion channels in the etiology of BPD was also implicated in other studies for BPD (Askland et al., 2009). We have known that ion channels and transporters are essential components in regulating neuronal excitability. Abnormality of ion channels has been suggested to be a plausible mechanism underlying BPD. To explain the recurrence and cycling nature of mood episodes in BPD, a kindling model was proposed as these clinical conditions are the consequences of neuronal hyperexcitability, which is linked to abnormal functions of ion channels (Mazza et al., 2007; Blumenfeld et al., 2009). Similarly, results in recent GWA and gene expression studies also support the involvement of ion channel genes in the etiology of BPD (Sklar et al., 2008, 2011; Smolin et al., 2012). Thus, genes in the ion channels or their regulatory loci have been attractive candidates in studying the underlying mechanism for BPD. Our miTGs prediction grants further support for this line of evidence.
In our identified 38 significantly enriched pathways, many miTGs were functionally related to ion channels, especially for potassium (e.g., KCNMA1, KCNQ5, and KCNK2) and calcium (e.g., RYR3 and PKD2) channels. All these genes involved in multiple significant pathways. For instance, KCNMA1 is a calcium-activated potassium channel and KCNQ5 belongs to the voltage-gated delayed rectifier potassium channel gene family. Both of them play important roles in the regulation of neuronal excitability (Laumonnier et al., 2006; Brown and Passmore, 2009). Molecular and functional studies found that defects of KCNMA1 contribute to autism and mental retardation (Laumonnier et al., 2006). For KCNK2 gene that encodes for a two-pore-domain background potassium channel, a recent genetic study revealed its association with susceptibility of major depressive disorder and response to antidepressant treatment (Liou et al., 2009). Additionally, several susceptible genes that cause abnormality in calcium signaling, such as CACNA1C and Bcl-2, were reported to be associated with BPD (Sklar et al., 2008, 2011; Distelhorst and Bootman, 2011). RYR3, a brain-specific ryanodine receptor for controlling intracellular calcium concentration, was found to be a susceptible gene for schizophrenia (Leonard and Freedman, 2006). In addition, the RYR3 knockout mice exhibited some abnormal behaviors, including hyperlocomoter activity and decreased social interaction (Matsuo et al., 2009). Although how these behaviors defects and functional defects of ion channels link with the pathology of BPD are still unclear, it warrants to conduct further basic and functional research to investigate the roles of ion channels in BPD.
Second, applying the weighting scheme for psychiatric- and neurological-associated miRNAs in the analysis, we identified several significant pathways for BPD that were involved many nervous related biological processes in Table 2 (Li et al., 2005; Nakatani et al., 2006; Ryan et al., 2006; Bremner and McCaffery, 2008; Ramocki and Zoghbi, 2008; Torkamani et al., 2008; Askland et al., 2009). Some pathways are novel findings for BPD but show their biological plausibility to neurological disorders in general, such as retinol metabolism (Maden, 2002), while other pathways are novel findings specific to BPD (not reported in other neurological disorders, such as drug metabolism other enzymes). Of note, it is known that necessity of cytoskeletal modulation play a role in the processes of nervous system development (Ramocki and Zoghbi, 2008). Additionally, neuroactive ligand receptor interaction pathway was reported to be associated with substance addiction, which is commonly observed comorbid condition in BPD patients (Li et al., 2005).
The miRNA regulation potentially contributes to the functions of these associated pathways in BPD. Recent findings exhibited the essential roles of miRNA machinery in many aspects of nervous system, including Dicer and miR-124 in neuronal development and miR-134 in synaptic development (Gao, 2008; Saba and Schratt, 2010). Regulation of calcium channel gene expression by miR-103 was also reported (Favereaux et al., 2011). In addition to enriched GO and KEGG pathways, we also identified many significant pathways that were obtained from curated data in the literature in MsigDB, which was mainly based on gene expression studies related with multiple cancers. Examining the functions of miRNA-associated genes in these enriched pathways suggested the involvement of different miRNA regulation in the etiology of BPD, including Notch signaling (e.g., JAG1), axonal growth, and guidance (e.g., CNTN4, NFIB, NAV3, and PTPRD), and cholesterol homeostasis (e.g., ABCA1) (Hekimi and Kershaw, 1993; Bixby, 2000; Karasinska et al., 2009; Mason et al., 2009; Shimoda and Watanbbe, 2009; Pedroso et al., 2012). In addition, our identified BPD-associated pathways consisted of many collagen related genes, such as COL1A2, COL27A1, and COL5A1, implicating that these genes may augment their impacts on BPD through miRNA regulation. Although the connection of collagen and BPD was rarely reported in the literature, the emerging data and evidence, came from in vivo studies suggested that collagen joined the processes of axonal growth and guidance, synaptogenesis, and Schwann cell myelination during the development of nervous system (Hubert et al., 2009).
Among these identified miTGs (in Table 4), some of them have been previously linked to the regulation of miRNA machinery. A recent study showed the mediation of miR-34a and miR-21 on expression of JAG1, to regulate the differentiation of human monocyte-derived dendritic cell, which is involved in five of our identified curated gene sets (Hashimi et al., 2009). In addition, increased expression of NAV3 mRNA was observed in brain tissue of Alzheimer’s disease and was suggested to be regulated by miR-29a (Shioya et al., 2010). On the contrary, the links between miRNAs and BPD were rarely constructed. Most of psychiatric- and neurological-associated miRNAs used in this study were reported to be related to schizophrenia, Alzheimer disease, autism, and Parkinson disease (according to PhenomiR database). Future studies are also needed to uncover the impacts of these miRNAs on the etiology of BPD.
There are some limitations in the present study. First, Pmin-value was used to represent the significance level of a gene. The information of other SNPs in a gene region may be missed. Nevertheless, previous studies showed that using Pmin-value in pathway analysis provides consistent results with other measures of gene-level statistic (Torkamani et al., 2008; Baranzini et al., 2009). Second, despite using more comprehensive pathway (e.g., MsigDB) and miRNA-disease/phenotype (e.g., PhenomiR) databases, the incompleteness of annotated pathways and miRNA-disease/phenotype information could have impacts on the correctness of the identified BPD-associated pathways. Third, the target genes of psychiatric- and neurological-associated miRNAs were predicted by computational methods. Although we used two miRNA target prediction algorithms to increase the correctness of prediction, further experimental validation by using functional studies are still needed in the future.
In conclusion, with integrating currently known psychiatric- and neurological-associated miRNAs as prior information, our pathway-based analyses using the GWA dataset of BPD identified not only previously reported pathways, but also new pathways that showed intriguing biological plausibility for BPD. So far, miRNAs studies in BPD are still in the infant stage. Our findings provided further evidence and support for exploring the roles of miRNA regulation in relation to nervous system for the risk of developing bipolar illness, especially ion channel regulation and axonal development. More, investigations remain to be done to elucidate the functions of these candidate pathways and genes, and the potential mechanisms involved with miRNA-mediated regulation.
The authors declare that the research was conducted in the absence of any commercial or financial relationships that could be construed as a potential conflict of interest.
This research was supported by National Science Council (NSC 99-2314-B-002-140-MY3) and National Health Research Institute (NHRI-EX101-9918NC) grants to Dr. P-H Kuo (PI). The GWA datasets were accessed through the Genetic Association Information Network (GAIN), database of Genotypes and Phenotypes (dbGaP) for bipolar disorder (http://www.ncbi.nlm.nih.gov/). We also thank P. C. Hsiao for his IT support.
Askland, K., Read, C., and Moore, J. (2009). Pathways-based analyses of whole-genome association study data in bipolar disorder reveal genes mediating ion channel activity and synaptic neurotransmission. Hum. Genet. 125, 63–79.
Baranzini, S. E., Galwey, N. W., Wang, J., Khankhanian, P., Lindberg, R., Pelletier, D., et al. (2009). Pathway and network-based analysis of genome-wide association studies in multiple sclerosis. Hum. Mol. Genet. 18, 2078–2090.
Benjamini, Y., and Hochberg, Y. (1995). Controlling the false discovery rate: a practical and powerful approach to multiple testing. J. R. Statist. Soc. B 57, 289–300.
Betel, D., Koppal, A., Agius, P., Sander, C., and Leslie, C. (2010). Comprehensive modeling of microRNA targets predicts functional non-conserved and non-canonical sites. Genome Biol. 11, R90.
Betel, D., Wilson, M., Gabow, A., Marks, D. S., and Sander, C. (2008). The microRNA org resource: targets and expression. Nucleic Acids Res. 36, D149–D153.
Bixby, J. L. (2000). Receptor tyrosine phosphatases in axon growth and guidance. Neuroreport 11, R5–R10.
Blumenfeld, H., Lampert, A., Klein, J. P., Mission, J., Chen, M. C., Rivera, M., et al. (2009). Role of hippocampal sodium channel Nav1.6 in kindling epileptogenesis. Epilepsia 50, 44–55.
Bremner, J. D., and McCaffery, P. (2008). The neurobiology of retinoic acid in affective disorders. Prog. Neuropsychopharmacol. Biol. Psychiatry 32, 315–331.
Brown, D. A., and Passmore, G. M. (2009). Neural KCNQ (Kv7) channels. Br. J. Pharmacol. 156, 1185–1195.
Chuang, L. C., Kao, C. F., Shih, W. L., and Kuo, P. H. (2012). Pathway analysis using information from allele-specific gene methylation in genome-wide association studies for bipolar disorder. PLoS ONE. (in press).
Distelhorst, C. W., and Bootman, M. D. (2011). Bcl-2 interaction with the inositol 1,4,5-trisphosphate receptor: role in Ca2+ signaling and disease. Cell Calcium 50, 234–241.
Favereaux, A., Thoumine, O., Bouali-Benazzouz, R., Roques, V., Papon, M.-A., Salam, S. A., et al. (2011). Bidirectional integrative regulation of Cav1.2 calcium channel by microRNA miR-103: role in pain. EMBO J. 30, 3830–3841.
Fiore, R., Siegel, G., and Schratt, G. (2008). MicroRNA function in neuronal development, plasticity and disease. Biochim. Biophys. Acta 1779, 471–478.
Forero, D. A., Van Der Ven, K., Callaerts, P., and Del-Favero, J. (2010). miRNA genes and the brain: implications for psychiatric disorders. Hum. Mutat. 31, 1195–1204.
Gao, F. B. (2008). Posttranscriptional control of neuronal development by microRNA networks. Trends Neurosci. 31, 20–26.
Gershon, E. S., Alliey-Rodriguez, N., and Liu, C. (2011). After GWAS: searching for genetic risk for schizophrenia and bipolar disorder. Am. J. Psychiatry 168, 253–256.
Hashimi, S. T., Fulcher, J. A., Chang, M. H., Gov, L., Wang, S., and Lee, B. (2009). MicroRNA profiling identifies miR-34a and miR-21 and their target genes JAG1 and WNT1 in the coordinate regulation of dendritic cell differentiation. Blood 114, 404–414.
Hekimi, S., and Kershaw, D. (1993). Axonal guidance defects in a Caenorhabditis elegans mutant reveal cell-extrinsic determinants of neuronal morphology. J. Neurosci. 13, 4254–4271.
Holmans, P., Green, E. K., Pahwa, J. S., Ferreira, M. A., Purcell, S. M., Sklar, P., et al. (2009). Gene ontology analysis of GWA study data sets provides insights into the biology of bipolar disorder. Am. J. Hum. Genet. 85, 13–24.
Hsu, Y. H., Zillikens, M. C., Wilson, S. G., Farber, C. R., Demissie, S., Soranzo, N., et al. (2010). An integration of genome-wide association study and gene expression profiling to prioritize the discovery of novel susceptibility Loci for osteoporosis-related traits. PLoS Genet. 6:e1000977. doi:10.1371/journal.pgen.1000977
Hubert, T., Grimal, S., Carroll, P., and Fichard-Carroll, A. (2009). Collagens in the developing and diseased nervous system. Cell. Mol. Life Sci. 66, 1223–1238.
John, B., Enright, A. J., Aravin, A., Tuschl, T., Sander, C., and Marks, D. S. (2004). Human MicroRNA targets. PLoS Biol. 2:e363. doi:10.1371/journal.pbio.0020363
Kao, C. F., Jia, P., Zhao, Z., and Kuo, P. H. (2012). Enriched pathways for major depressive disorder identified from a genome-wide association study. Int. J. Neuropsychopharmacol. 15, 1401–1411.
Karasinska, J. M., Rinninger, F., Lutjohann, D., Ruddle, P., Franciosi, S., Kruit, J. K., et al. (2009). Specific loss of brain ABCA1 increases brain cholesterol uptake and influences neuronal structure and function. J. Neurosci. 29, 3579–3589.
Klein, M. E., Lioy, D. T., Ma, L., Impey, S., Mandel, G., and Goodman, R. H. (2007). Homeostatic regulation of MeCP2 expression by a CREB-induced microRNA. Nat. Neurosci. 10, 1513–1514.
Laumonnier, F., Roger, S., Guérin, P., Molinari, F., M’Rad, R., Cahard, D., et al. (2006). Association of a functional deficit of the BKCa channel, a synaptic regulator of neuronal excitability, with autism and mental retardation. Am. J. Psychiatry 163, 1622–1629.
Leonard, S., and Freedman, R. (2006). Genetics of chromosome 15q13-q14 in schizophrenia. Biol. Psychiatry 60, 115–122.
Li, C. Y., Mao, X., and Wei, L. (2005). Genes and (Common) Pathways underlying addiction revealed by combining and analyzing candidate gene lists from multiple technology platforms. PLoS Comput. Biol. 4:e2. doi:10.1371/journal.pcbi.0040002
Lim, L. P., Lau, N. C., Garrett-Engele, P., Grimson, A., Schelter, J. M., Castle, J., et al. (2005). Microarray analysis shows that some microRNAs downregulate large numbers of target mRNAs. Nature 433, 769–773.
Liou, Y. J., Chen, T. J., Tsai, S. J., Yu, Y. W., Cheng, C. Y., and Hong, C. J. (2009). Support for the involvement of the KCNK2 gene in major depressive disorder and response to antidepressant treatment. Pharmacogenet. Genomics 19, 735–741.
Maden, M. (2002). Retinoid signalling in the development of the central nervous system. Nat. Rev. Neurosci. 3, 843–853.
Manolio, T. A., Collins, F. S., Cox, N. J., Goldstein, D. B., Hindorff, L. A., Hunter, D. J., et al. (2009). Finding the missing heritability of complex diseases. Nature 461, 747–753.
Maragkakis, M., Alexiou, P., Papadopoulos, G. L., Reczko, M., Dalamagas, T., Giannopoulos, G., et al. (2009a). Accurate microRNA target prediction correlates with protein repression levels. BMC Bioinformatics 10:295. doi:10.1186/1471-2105-10-295
Maragkakis, M., Reczko, M., Simossis, V. A., Alexiou, P., Papadopoulos, G. L., Dalamagas, T., et al. (2009b). DIANA-microT web server: elucidating microRNA functions through target prediction. Nucleic Acids Res. 37, W273–W276.
Mason, S., Piper, M., Gronostajski, R. M., and Richards, L. J. (2009). Nuclear factor one transcription factors in CNS development. Mol. Neurobiol. 39, 10–23.
Matsuo, N., Tanda, K., Nakanishi, K., Yamasaki, N., Toyama, K., Takao, K., et al. (2009). Comprehensive behavioral phenotyping of ryanodine receptor type 3 (RyR3) knockout mice: decreased social contact duration in two social interaction tests. Front. Behav. Neurosci. 3:3. doi:10.3389/neuro.08.003.2009
Mazza, M., Di Nicola, M., Della Marca, G., Janiri, L., Bria, P., and Mazza, S. (2007). Bipolar disorder and epilepsy: a bidirectional relation? Neurobiological underpinnings, current hypotheses, and future research directions. Neuroscientist 13, 392–404.
McGuffin, P., Rijsdijk, F., Andrew, M., Sham, P., Katz, R., and Cardno, A. (2003). The heritability of bipolar affective disorder and the genetic relationship to unipolar depression. Arch. Gen. Psychiatry 60, 497–502.
Muinos-Gimeno, M., Guidi, M., Kagerbauer, B., Martin-Santos, R., Navines, R., Alonso, P., et al. (2009). Allele variants in functional MicroRNA target sites of the neurotrophin-3 receptor gene (NTRK3) as susceptibility factors for anxiety disorders. Hum. Mutat. 30, 1062–1071.
Nakatani, N., Hattori, E., Ohnishi, T., Dean, B., Iwayama, Y., Matsumoto, I., et al. (2006). Genome-wide expression analysis detects eight genes with robust alterations specific to bipolar I disorder: relevance to neuronal network perturbation. Hum. Mol. Genet. 15, 1949–1962.
Pedroso, I., Lourdusamy, A., Rietschel, M., Nothen, M. M., Cichon, S., Mcguffin, P., et al. (2012). Common genetic variants and gene-expression changes associated with bipolar disorder are over-represented in brain signaling pathway genes. Biol. Psychiatry 72, 311–317.
Peng, G., Luo, L., Siu, H., Zhu, Y., Hu, P., Hong, S., et al. (2010). Gene and pathway-based second-wave analysis of genome-wide association studies. Eur. J. Hum. Genet. 18, 111–117.
Purcell, S., Neale, B., Todd-Brown, K., Thomas, L., Ferreira, M. A., Bender, D., et al. (2007). PLINK: a tool set for whole-genome association and population-based linkage analyses. Am. J. Hum. Genet. 81, 559–575.
Ramocki, M. B., and Zoghbi, H. Y. (2008). Failure of neuronal homeostasis results in common neuropsychiatric phenotypes. Nature 455, 912–918.
Ruepp, A., Kowarsch, A., Schmidl, D., Buggenthin, F., Brauner, B., Dunger, I., et al. (2010). PhenomiR: a knowledgebase for microRNA expression in diseases and biological processes. Genome Biol. 11, R6.
Ryan, M. M., Lockstone, H. E., Huffaker, S. J., Wayland, M. T., Webster, M. J., and Bahn, S. (2006). Gene expression analysis of bipolar disorder reveals downregulation of the ubiquitin cycle and alterations in synaptic genes. Mol. Psychiatry 11, 965–978.
Saba, R., and Schratt, G. M. (2010). MicroRNAs in neuronal development, function and dysfunction. Brain Res. 1338, 3–13.
Shimoda, Y., and Watanbbe, K. (2009). Contactins: emerging key roles in the development and function of the nervous system. Cell Adh. Migr. 3, 64–70.
Shioya, M., Obayashi, S., Tabunoki, H., Arima, K., Saito, Y., Ishida, T., et al. (2010). Aberrant microRNA expression in the brains of neurodegenerative diseases: miR-29a decreased in Alzheimer disease brains targets neurone navigator 3. Neuropathol. Appl. Neurobiol. 36, 320–330.
Sklar, P., Ripke, S., Scott, L. J., Andreassen, O. A., Cichon, S., Craddock, N., et al. (2011). Large-scale genome-wide association analysis of bipolar disorder identifies a new susceptibility locus near ODZ4. Nat. Genet. 43, 977–983.
Sklar, P., Smoller, J. W., Fan, J., Ferreira, M. A., Perlis, R. H., Chambert, K., et al. (2008). Whole-genome association study of bipolar disorder. Mol. Psychiatry 13, 558–569.
Smolin, B., Karry, R., Gal-Ben-Ari, S., and Ben-Shachar, D. (2012). Differential expression of genes encoding neuronal ion-channel subunits in major depression, bipolar disorder and schizophrenia: implications for pathophysiology. Int. J. Neuropsychopharmacol. 15, 869–882.
Subramanian, A., Tamayo, P., Mootha, V. K., Mukherjee, S., Ebert, B. L., Gillette, M. A., et al. (2005). Gene set enrichment analysis: a knowledge-based approach for interpreting genome-wide expression profiles. Proc. Natl. Acad. Sci. U.S.A. 102, 15545–15550.
The GAIN Collaborative Research Group. (2007). New models of collaboration in genome-wide association studies: the Genetic Association Information Network. Nat. Genet. 39, 1045–1051.
The Schizophrenia Psychiatric Genome-Wide Association Study (GWAS) Consortium. (2011). Genome-wide association study identifies five new schizophrenia loci. Nat. Genet. 43, 969–976.
Tintle, N., Lantieri, F., Lebrec, J., Sohns, M., Ballard, D., and Bickeboller, H. (2009a). Inclusion of a priori information in genome-wide association analysis. Genet. Epidemiol. 33(Suppl. 1), S74–S80.
Tintle, N. L., Borchers, B., Brown, M., and Bekmetjev, A. (2009b). Comparing gene set analysis methods on single-nucleotide polymorphism data from genetic analysis workshop 16. BMC Proc. 3:S96. doi:10.1186/1753-6561-3-S7-S96.
Torkamani, A., Topol, E. J., and Schork, N. J. (2008). Pathway analysis of seven common diseases assessed by genome-wide association. Genomics 92, 265–272.
Wang, K., Li, M., and Bucan, M. (2007). Pathway-based approaches for analysis of genome wide association studies. Am. J. Hum. Genet. 81, 1278–1283.
Wang, K., Li, M., and Hakonarson, H. (2010). Analysing biological pathways in genome-wide association studies. Nat. Rev. Genet. 11, 843–854.
Wayman, G. A., Davare, M., Ando, H., Fortin, D., Varlamova, O., Cheng, H. Y., et al. (2008). An activity-regulated microRNA controls dendritic plasticity by down-regulating p250GAP. Proc. Natl. Acad. Sci. U.S.A. 105, 9093–9098.
Xu, B., Karayiorgou, M., and Gogos, J. A. (2010). MicroRNAs in psychiatric and neurodevelopmental disorders. Brain Res. 1338, 78–88.
Zhong, H., Yang, X., Kaplan, L. M., Molony, C., and Schadt, E. E. (2010). Integrating pathway analysis and genetics of gene expression for genome-wide association studies. Am. J. Hum. Genet. 86, 581–591.
Table A1. Number of pathways at different cut-off in four statistical scenarios (total number of pathways = 4051).
Table A2. Number of pathways identified by GSEA or SUM methods at significant level of 0.01 under weighted and non-weighted schemes (total number of pathways = 4051).
Keywords: microRNA, bipolar disorder, pathway analysis, genome-wide association, ion channel
Citation: Shih W-L, Kao C-F, Chuang L-C and Kuo P-H (2012) Incorporating information of microRNAs into pathway analysis in a genome-wide association study of bipolar disorder. Front. Gene. 3:293. doi: 10.3389/fgene.2012.00293
Received: 31 August 2012; Paper pending published: 03 October 2012;
Accepted: 27 November 2012; Published online: 18 December 2012.
Edited by:
Peng Jin, Emory University School of Medicine, USACopyright: © 2012 Shih, Kao, Chuang and Kuo. This is an open-access article distributed under the terms of the Creative Commons Attribution License, which permits use, distribution and reproduction in other forums, provided the original authors and source are credited and subject to any copyright notices concerning any third-party graphics etc.
*Correspondence: Po-Hsiu Kuo, Department of Public Health and Institute of Epidemiology and Preventive Medicine, College of Public Health, National Taiwan University, Room 521, No. 17, Xuzhou Road, Taipei 10055, Taiwan. e-mail:cGhrdW9AbnR1LmVkdS50dw==
Disclaimer: All claims expressed in this article are solely those of the authors and do not necessarily represent those of their affiliated organizations, or those of the publisher, the editors and the reviewers. Any product that may be evaluated in this article or claim that may be made by its manufacturer is not guaranteed or endorsed by the publisher.
Research integrity at Frontiers
Learn more about the work of our research integrity team to safeguard the quality of each article we publish.