- 1 Department of Ophthalmology, Keck School of Medicine, University of Southern California, Los Angeles, CA, USA
- 2 Department of Preventive Medicine, Keck School of Medicine, University of Southern California, Los Angeles, CA, USA
- 3 Medical Genetics Institute, Cedars-Sinai Medical Center, Los Angeles, CA, USA
Genotype imputation is a vital tool in genome-wide association studies (GWAS) and meta-analyses of multiple GWAS results. Imputation enables researchers to increase genomic coverage and to pool data generated using different genotyping platforms. HapMap samples are often employed as the reference panel. More recently, the 1000 Genomes Project resource is becoming the primary source for reference panels. Multiple GWAS and meta-analyses are targeting Latinos, the most populous, and fastest growing minority group in the US. However, genotype imputation resources for Latinos are rather limited compared to individuals of European ancestry at present, largely because of the lack of good reference data. One choice of reference panel for Latinos is one derived from the population of Mexican individuals in Los Angeles contained in the HapMap Phase 3 project and the 1000 Genomes Project. However, a detailed evaluation of the quality of the imputed genotypes derived from the public reference panels has not yet been reported. Using simulation studies, the Illumina OmniExpress GWAS data from the Los Angles Latino Eye Study and the MACH software package, we evaluated the accuracy of genotype imputation in Latinos. Our results show that the 1000 Genomes Project AMR + CEU + YRI reference panel provides the highest imputation accuracy for Latinos, and that also including Asian samples in the panel can reduce imputation accuracy. We also provide the imputation accuracy for each autosomal chromosome using the 1000 Genomes Project panel for Latinos. Our results serve as a guide to future imputation based analysis in Latinos.
Introduction
Genotype imputation has become a vital tool in genome-wide association studies (GWAS) and meta-analyses of multiple GWAS results. Imputation enables researchers to increase genomic coverage of each individual GWAS and to meta-analyze data generated using different GWAS chips. Based on a reference panel of samples from identical or similar populations that are genotyped using a dense set of genetic markers, imputation methods infer genotypes at markers that were not directly typed in a study sample. HapMap (Frazer et al., 2007; Altshuler et al., 2010) samples are often employed as the reference panel. More recently, the 1000 Genomes Project (1KGP; Durbin et al., 2010) resource is becoming the primary source for reference panels.
Genome-wide association studies are expanding to minority populations, e.g., Latinos (Gao and Edwards, 2011). While the Latino population is the most rapidly growing and largest minority group in the US, it has historically been understudied. At present, there is no consensus on how to carry out genotype imputation in individuals of Latino ancestry and different studies seem to have adopted different approaches. For example, Fu et al. (2010) used the HapMap Phase 3 (HM3) MEX as the reference panel; Shi et al. (2011) used the CEU, YRI, JPT, and CHB individuals in the HapMap Phase 2 as the reference panel; and Parra et al. (2011) used a combination of the HapMap Phase 2 sample and the HM3 MEX as the reference (see Table 1 for population labels).
1KGP reference panels have clear advantage over HapMap for genotype imputation. First, the 1KGP benefited from the whole-genome sequencing technology, which significantly increased the genomic coverage. This allows more variants, both common and rare, to be imputed in a study sample. Second, the 1KGP has also increased the panel size of each population, which can also improve genotype imputation accuracy. To date, no detailed evaluation of genotype imputation in Latinos has been reported using either the HapMap or 1KGP data; the details of how one should conduct imputation for Latino populations using either panel has not been determined (e.g., what is the best make-up for the reference panel?)
As GWAS and meta-analyses are expanding to Latinos (Fu et al., 2010; Parra et al., 2011), it is particular important to evaluate genotype imputation in Latinos using public imputation panels, particularly those from 1KGP. In this study, we evaluated genotype imputation in Latinos using simulated data and the Illumina OmniExpress GWAS data from the Los Angeles Latino Study (LALES). We elected to use the MACH software (Li and Abecasis, 2006; Li et al., 2010) for imputation because it is highly accurate based on previous reports (Pei et al., 2008; Huang et al., 2009; Li et al., 2010) and is easy to use. We report imputation accuracy as a function of reference panels using the HM3 and 1KGP data and provide the accuracy estimate for each autosomal chromosome. We thus provide guidance for imputation in future studies using Latino data.
Materials and Methods
Study Sample
We conducted this research using the data from LALES, a population-based study of 6,357 Latinos, aged 40 years and older, living in six census tracts in the city of La Puente, Los Angeles County, California. The Los Angeles County, University of Southern California Health Sciences Campus Institutional Review Board Ethics Committee approved this study. Written, informed consent was obtained from all participants. The data obtained from this study have been used to document the prevalence, incidence, and impact of visual impairment, e.g., diabetic retinopathy, age-related macular degeneration, open angle glaucoma, and ocular hypertension in Latinos (Varma et al., 2004).
Genotyping
We genotyped 665 Latinos recruited in LALES using the Illumina OmniExpress BeadChip (∼733 K markers). We also included 18 duplicates to verify reproducibility. The genotyping was performed at the Cedars-Sinai Medical Center. SNPs were called using the Illumina GenomeStudio (v2011.1) software. The average call rate was greater than 99.32%. The reproducibility was greater than 99.99%. We used the software PLINK (Purcell et al., 2007) to perform quality control. Individuals were excluded if genotyping call rates were less than 97%. Markers were excluded if minor allele frequencies were less than 0.01, call rates were less than 95%, or if Hardy–Weinberg equilibrium p-values were less than 10−6. This resulted in there being 647 individuals in the final analysis. SNPs were coded on the forward strand to facilitate the imputation process.
Simulation Study
To evaluate the performance of genotype imputation in Latinos in situations in which the true genotypes are known, we simulated SNP data using the HAPGEN2 software (Su et al., 2011). HAPGEN2 is capable of simulating genotypes conditional on a set of known haplotypes and creating levels of LD structure similar to those in the reference panel (Su et al., 2011). We used the combined recombination rate file for chromosome 22 downloaded from the IMPUTE website (see web resources) and set the effective population size to 11,418 for the HAPGEN2 simulation. Based on phased HM3 MEX haplotypes, we generated 5,000 simulated Latinos, genotyped across 20,085 SNPs on chromosome 22. Principal components analysis by the EIGENSOFT software (Patterson et al., 2006) showed that the simulated individuals overlapped with the HM3 MEX individuals (Figure A1 in Appendix), indicating our simulation mimicked those in real data. We then constructed two reference panels of different sizes: we randomly selected 52 (the current size of the HM3 MEX reference panel) and 200 simulated individuals without replacement and phased their haplotypes using MACH. We then assessed genotype imputation accuracy in an additional 500 simulated individuals. We treated the latter 500 individuals as having known SNPs genotyped using the Illumina OmniExpress, and compared the true (simulated) genotypes with the imputed genotypes for the remaining 11,825 HM3 SNPs on chromosome 22 (those not present on the Illumina OmniExpress chip).
Reference Haplotypes from the HapMap Phase 3 and the 1000 Genomes Project
We downloaded HM3 r2 and 1KGP Phase I (α) phased haplotypes from the MACH website (see Web Resources). In particular, we downloaded the CEU (n = 234), YRI (n = 230), TSI (n = 176), MEX (n = 104), and JPT + CHB (n = 340) population references from the HM3 r2 data and the EUR (n = 762), AFR (n = 492), ASN (n = 572), and AMR (n = 362) population references from the 1KGP Phase I data. The 1KGP reference panels, with the inclusion of whole-genome sequencing data, contain a much larger number of variants than the HM3 reference panels: 38.9 versus 1.4 million. Population labels and the number of haplotypes in each population are shown in Table 1.
Imputations Using MACH
We used MACH (Li and Abecasis, 2006; Li et al., 2010) for carrying out genotype imputation because it is one of the leading programs and has been shown to work well in a variety of settings (Pei et al., 2008; Huang et al., 2009; Li et al., 2009; Nothnagel et al., 2009; Sung et al., 2011). MACH employs a Markov Chain algorithm and imputes missing genotypes taking phased haplotypes as templates (Li et al., 2010). MACH version 1.0.16 was downloaded from the software’s website (see Web Resources). To evaluate genotype imputation accuracy for missing genotypes, we used the “mask” option in MACH to randomly mask 2% of the genotypes in our study sample and then compared the imputed genotypes with the masked genotypes. To evaluate genotypes of untyped markers, we used the standard genotype imputation approach recommended by the authors of MACH. We used 50 iterations of the Markov sampler to ensure reliable results and specified 400 haplotypes when updating the phase for each individual to keep computations tractable.
Results
Results of Simulation Study
We first evaluated genotype imputation in Latinos using simulation. Our first question was: how does the HM3 MEX reference panel perform? The concern here is that it includes just 104 haplotypes. In Figure 1 we show plots of imputation accuracy for data simulated to reflect 500 Latinos. Data was imputed from reference panels constructed from 52 references (104 haplotypes) or 200 references (400 haplotypes). Figures 1A–C show histograms of imputation errors (i.e., cases in which the imputed genotype was incorrect) and MACH Rsq estimates for the imputed genotypes. MACH Rsq is an estimate of the dosage r2, the squared correlation between true genotypes and estimated allelic dosage, so higher Rsq corresponds to better imputation quality (Li et al., 2010). Figure 1A shows the distribution of the number errors per individual, whereas Figure 1B shows the distribution of the number of errors per SNP. In Figure 1C we show boxplots of the MACH Rsq estimates across imputed SNPs. Results for the 200-reference and 52-reference panels are shown in pink and blue, respectively. In Figure 1A, the mean (standard deviation) of the number of errors per individual is 127 (24) versus 418 (48) for imputation based on the 200- and 52-reference panels, respectively, showing a clear improvement when the larger reference panel is used. In Figure 1B, performance is again better for the 200-reference panel than for the 52-reference panel. In Figure 1C, the median (inter quartile range) MACH Rsq is 0.987 (0.998, 0.929) and 0.93 (0.986, 0.656) for the 200-individual and the 52-individual panels, respectively. If we consider Rsq ≥ 0.80 as a criteria for selecting well imputed SNPs, the 200-individual panel gave 25% more well imputed SNPs than the 52-individual panel. Overall, the 200-individual reference panel gave much lower imputation error rate and better imputed SNPs than the 52-individal reference panel. This clearly demonstrates that when using a reference panel drawn from the same population, the size of that panel has a significant impact on imputation accuracy for Latinos, with larger panels performing better. This is consistent with reports from non-Latino studies (Li et al., 2009, 2010; Marchini and Howie, 2010).
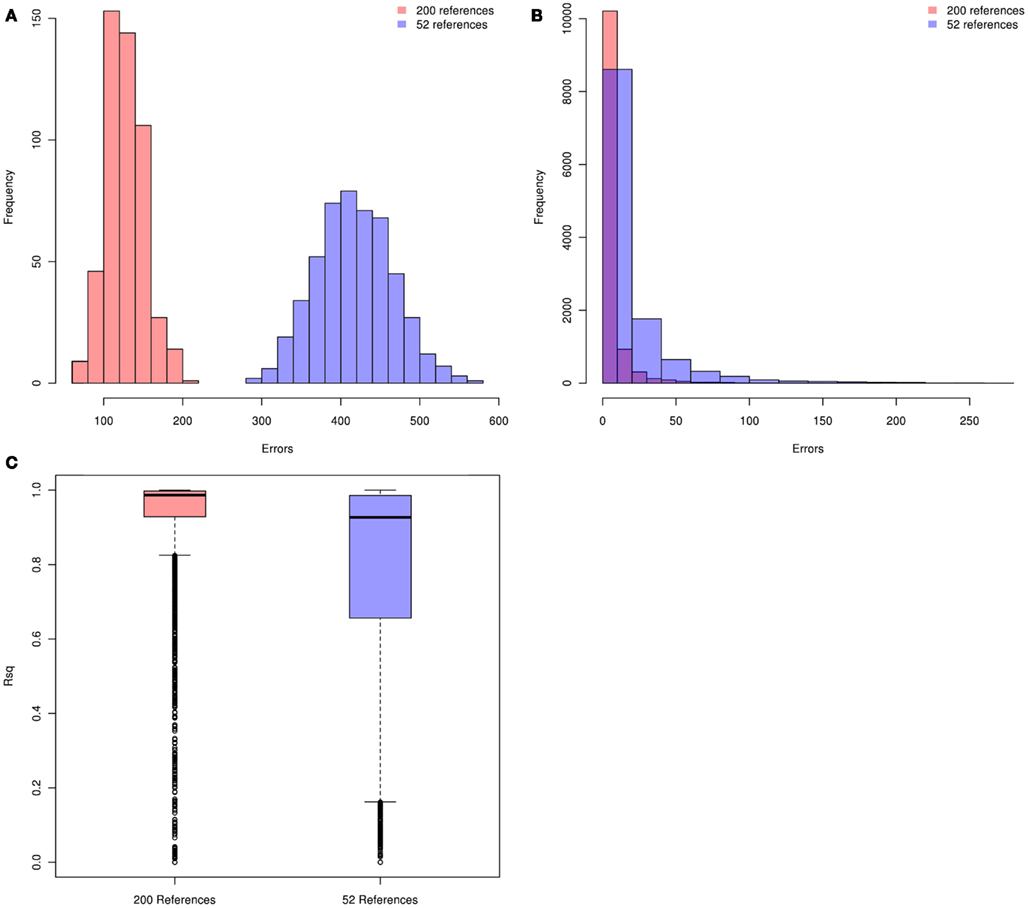
Figure 1. Distributions of per individual and per SNP errors and the imputed MACH Rsq. Pink and blue denote the 200-individual and 52-individual reference panels, respectively. Genotype imputation accuracy is tested in an additional 500 simulated individuals. The true (simulated) genotypes of 11,825 SNPs on chromosome 22 (those HapMap Phase 3 SNPs not present on the Illumina OmniExpree Beadchip) are compared with the imputed genotypes. (A) Distribution of per individual errors. (B) Distribution of per SNP errors. (C) Boxplots of the MACH Rsq for the imputed SNPs.
Results from Empirical Data
We downloaded phased haplotypes from the MACH website (see Table 1). The current imputation resources for Latinos are rather limited compared with resources for European ancestry. In the HM3 data, there are only 104 MEX haplotypes, compared to 410 haplotypes of European ancestry (234 CEU + 176 TSI). In the 1KGP data, there are only 362 Latino haplotypes, compared to 762 haplotypes of European ancestry (174 CEU + 196 TSI + 186 FIN + 178 GBR + 28 IBS). Given the limited imputation panel size for Latinos, how should researchers best carry out genotype imputation? In particular, it is not clear how best to choose the reference panel. Here we compare several possible approaches. One approach is only to include haplotypes from the ancestral populations. Another tested approach is to use a “cosmopolitan” panel, which contains all the haplotypes available, regardless of ancestry (Howie et al., 2011). To shed light on this question, we carried out a series of imputation experiments to seek the optimal imputation panel based on HM3 and 1KGP data.
We randomly selected chromosomes 22 for evaluation purposes. Table 2 shows the imputation error rates for chromosome 22 as a function of the make-up of the reference panel. For imputation using HM3 data, the CEU + YRI + JPT + CHB panel (804 haplotypes) gave lower per genotype error rate (PGER) than the MEX panel (104 haplotypes). This is likely due to the extremely small size of the MEX panel. Adding the CEU and YRI haplotypes to the MEX panel, we see that the PGER is reduced from 6.09 to 5.11% and from 5.11 to 4.19% with the addition of each respective reference panel, which shows the value of adding ancestry related haplotypes to the MEX panel given its small size, and all performed better than the CEU + YRI + JPT + CHB panel. However, when further adding JPT + CHB to the MEX + CEU + YRI panel, PGER increased from 4.19 to 4.24%. We also evaluated the impact of including TSI in the MEX + CEU + YRI panel as a way to increase the European proportion, which resulted in the lowest PGER, 4.00%. The subsequent addition of JPT + CHB to MEX + CEU + YRI + TSI increased the PGER to 4.12%.
For the imputation experiments using 1KGP data, the AMR panel, a combination of MEX, Colombian, and Puerto Rican, gave lower PGER (3.72%) than the MEX panel alone (4.84%), which indicated the value of adding other Latino data to the MEX panel. We then added EUR, AFR, and ASN sequentially to the AMR panel. The PGERs were 3.72, 3.69, and 3.27% for the AMR, AMR + EUR, and AMR + EUR + AFR panels, which showed the decreasing pattern of PGER when ancestry related haplotypes were added to the AMR panel. However, adding ASN to the AMR + EUR + AFR panel increased the PGER from 3.27 to 3.35%. Therefore, adding Asian haplotypes to the AMR + EUR + AFR panel increases imputation errors for Latinos. We also tried including only CEU and YRI haplotypes to the AMR panel, in an attempt to make the imputation panel “cleaner and leaner” by only including haplotypes closely related to Latinos. In Table 2, we see that the AMR + CEU + YRI panel gives the lowest PGER 3.23% among all the reference panels considered, while further adding JPT + CHB increases PGER to 3.35%. Therefore, adding Asian haplotypes to the AMR + CEU + YRI panel can reduce accuracy for the genotype imputation for Latinos. The results in Table 2 show that the AMR + CEU + YRI panel gives the best imputation accuracy for Latinos among all the reference panels considered. We have validated this on another randomly chosen chromosome 9 (Table A1 in Appendix). We also included the per allele error rates, which were approximately half of their corresponding PGERs. The running time was roughly proportional to the memory used, e.g., it took about 4 and 10 days for runs using 4.5 and 11.4 GB memory using our workstation with Xeon 5680 CPUs.
Thus far, we have investigated how genotype imputation performs for Latinos for genotyped SNPs by randomly masking 2% genotypes. In practice, it will also be important to know how genotype imputation performs for SNPs that are untyped for all samples in the study, which is commonly characterized by the MACH Rsq (Li et al., 2010). Using chromosome 22 as an example, we masked all SNPs that are included on the Illumina OmniExpress chip but not included on the Illumina HumanHap610 chip (4,535 SNPs for our GWAS data set). This is analogous to the situation that would arise in a meta-analysis that combined two studies, each of which used one of these two platforms. Then, we compared the dosage r2 with the MACH Rsq to check how they agree with each other for the imputation in Latinos. Figure 2 shows the pairwise plot of the dosage r2 by the MACH Rsq using the AMR + CEU + YRI panel for chromosome 22. The diagonal red line is a perfect fit between r2 and Rsq. We see that Rsq is highly correlated with r2 and gives very good estimate of r2 in general. We saw this pattern on another randomly chosen chromosome 9 (Figure A2 in Appendix). Therefore, it is reasonable to use MACH Rsq to select high-quality imputed SNPs for downstream association analyses in Latinos. Rsq > 0.30 is recommended and often used for Caucasian samples (Scott et al., 2007; Li et al., 2010). However, previous genotype imputation augmented GWAS and meta-analyses using Caucasian samples typically used HapMap phase 2 as the reference panel and filtered imputed SNPs based on both minor allele frequency (MAF) >1% and Rsq > 0.30 (Scott et al., 2007; Kottgen et al., 2010). HapMap is a resource for common SNPs. Moreover, using a MAF > 1% as a filter, it was likely that many of the poorly imputed SNPs would already have been filtered out, therefore an Rsq threshold of 0.30 would not have had much additional effect. Considering the direct relationship between r2 and the effective number of sample size necessary for association tests (Pritchard and Przeworski, 2001), we think a more stringent Rsq threshold, e.g., 0.80, may be more desirable, especially for the impute based on 1KGP data, which include an enormous amount of rare variants.
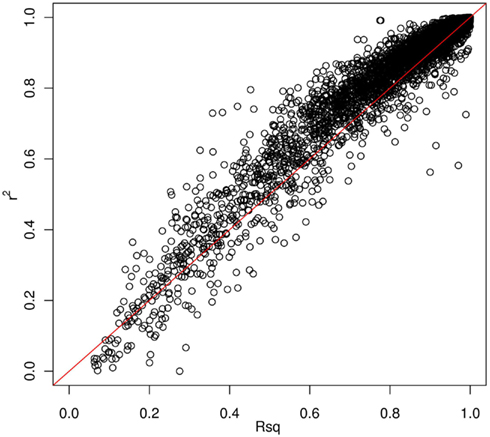
Figure 2. Pairwise plot of the dosage r2 by the MACH Rsq. Diagonal line (red) is a perfect match between the MACH Rsq and the dosage r2. Further off the diagonal line means poorer estimate. The correlation coefficient between Rsq and r2 is 0.96.
We then carried out genotype imputation based on the 1KGP Phase I AMR + CEU + YRI reference panel in our LALES GWAS samples. Figure 3 shows the boxplot of Rsq for 485,313 imputed SNPs on chromosome 22 (all typed SNPs were excluded). The Rsq median is 0.11, 0.03, and 0.87 for no MAF filter, MAF < 0.01, and MAF ≥ 0.01, respectively. It is clear that the majority of common SNPs were imputed very well, with 80% of them (83,990 out of 146,642) having Rsq > 0.8, while most of the rare SNPs were poorly imputed, with only 3.2% of them (11,112 out of 348,333) having Rsq > 0.8. 1KGP aims at capturing rare variants through sequencing technology (Durbin et al., 2010). However, at its current sample size, most of the rare variants were not imputed well for Latinos. But compared with the number of variants on the Illumina OmniExpress chip (10,678 for chromosome 22), the imputation yield (95,102 imputed SNPs had Rsq ≥ 0.80) from it is still very useful with almost ninefold increase in the number of variants that can be interrogated. Therefore, it is a good strategy to include 1KGP imputation in the downstream GWAS analysis. We also carried out imputation using the AMR + EUR + AFR + ASN panel (a cosmopolitan panel) as a comparison. The average Rsq derived from the AMR + EUR + AFR + ASN panel was 0.291, lower than 0.307 from the AMR + CEU + YRI panel. Moreover, the AMR + CEU + YRI panel gave 8.33% more well imputed SNPs (Rsq ≥ 0.80) than the AMR + EUR + AFR + ASN panel. Again, we saw that the AMR + CEU + YRI panel gave better imputation results.
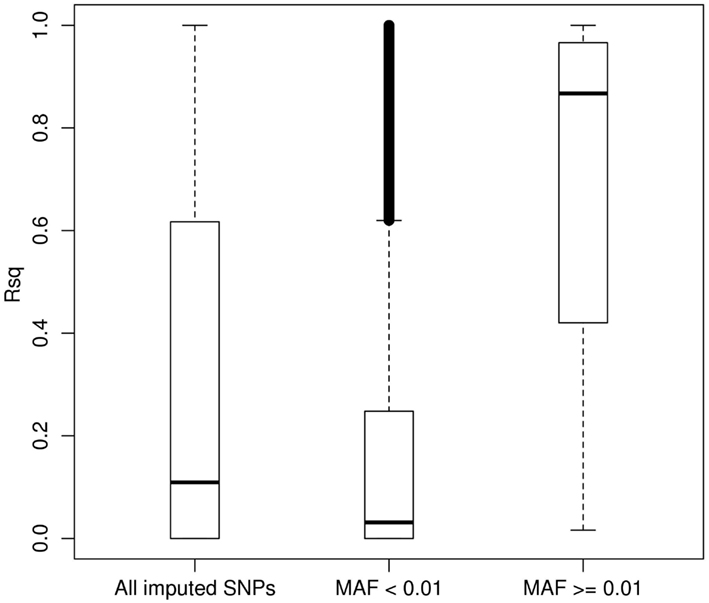
Figure 3. Boxplot of the MACH Rsq for the imputed SNPs stratified by the minor allele frequency. Boxplot of the MACH Rsq for 485,313 imputed SNPs on chromosome 22 (with all typed SNPs by the Illumina OmniExpress excluded) based on the 1000 Genomes Project AMR + CEU + YRI reference panel. Abbreviations: MAF, minor allele frequency.
It is also of interest to assess the performance for each chromosome. For this reason, we randomly masked 2% of the genotypes in the LALES GWAS data and compared them with MACH imputed genotypes. The PGER for each autosomal chromosome is summarized in Figure 4. The PGER is much higher for chromosomes 15–22 than for chromosome 1–14. Larger chromosomes tend to have less recombination events and longer stretch of LD (The International HapMap Consortium, 2005), and hence lower PGER. Chromosome 19 appears to be particularly difficult to impute, while imputation for chromosome 13, 11, and 10 is more successful. It is interesting to note that chromosomes 19 and 13 have the highest and the lowest gene density, respectively (Semple, 2004).
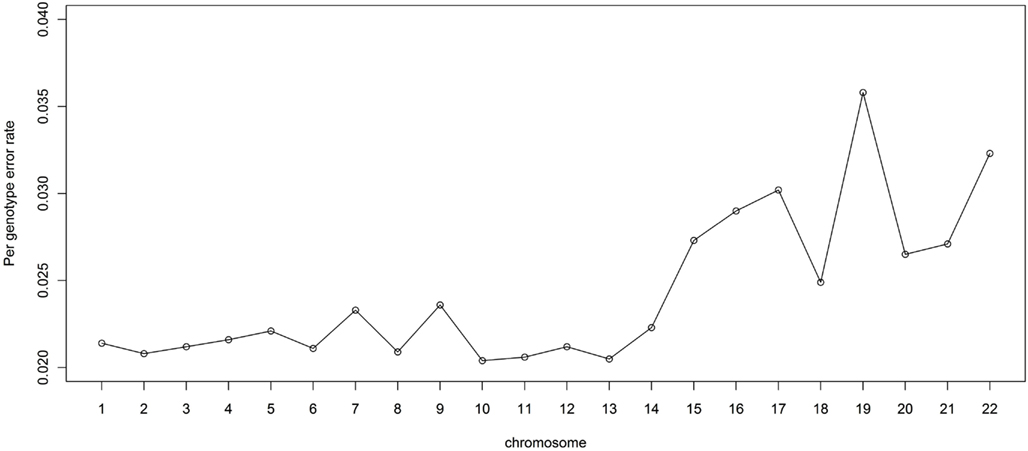
Figure 4. Genotype imputation accuracy by chromosome. Genotype imputation accuracy is measured by per genotype error rate by randomly masking 2% genome-wide SNPs.
Discussion
Historically, Latinos have been understudied in genetic research. Taking chromosomes 22 and 9 as an example, we carried out an evaluation of genotype imputation in Latinos using simulation and the empirical GWAS data from LALES. Among all the reference panels that we considered, the 1KGP AMR + CEU + YRI panel gave the best imputation accuracy. We further picked chromosomes that have the lowest (chromosome 13) and highest (chromosome 19) gene density and validated the same results, i.e., the 1KGP AMR + CEU + YRI panel gave better genotype imputation accuracy than the cosmopolitan panel and the panel with Asian references included (Tables A2 and A3 in Appendix). We also presented imputation accuracy for each autosomal chromosome based on the 1KGP AMR + CEU + YRI panel. From our results, we see that there are several factors that affect the accuracy of genotype imputation for Latinos: the size of the reference panel, the make-up of the reference panel, MAF, and the chromosome concerned.
There are many other genotype imputation software packages, e.g., IMPUTE2 (Howie et al., 2009), BEAGLE (Browning and Browning, 2007, 2009), and fastPHASE (Scheet and Stephens, 2006). Each method may have its advantage over other approaches in some special situations (Li et al., 2009; Marchini and Howie, 2010; Browning and Browning, 2011). When genotype reference panels become larger and larger and cover more populations, it is computationally impractical for one study to be able to cover all available imputation methods using the latest 1KGP reference data. Therefore, we focused on using MACH, one of the leading genotyping imputation software packages, and evaluated genotype imputation for Latinos in this study.
For this study, we aimed to seek high imputation accuracy regardless of the memory used. MACH can be run in two ways. We used the standard genotype imputation approach instead of the alternative approach (see MACH online tutorial) because it was reported that the standard approach gave better accuracy (Pei et al., 2008). But the standard approach requires intensive computing. There are many possible ways to reduce memory usage and to improve computing speed, e.g., (1) break a chromosome into pieces and use a parallel computing cluster; (2) use pre-phasing (Fuchsberger et al., 2011); (3) use minimac, an efficient implementation of MACH, e.g., phase the haplotypes by MACH first and then run the genotype imputation by minimac (Huang et al., 2012); (4) with the advance in graphical processing unit (GPU) devices, it is also attractive to use GPU for accelerating genotype imputation (Kai and Chen, 2011).
There are several limitations of our study. We only included reasonable guesses of the best reference panel for Latinos in our imputation evaluation. There are 14 ethnic groups in 1KGP data (see Table 1) and hence 91, 364, and 1001 for two-way, three-way, and four-way combinations, respectively. It is impossible to enumerate all possible combinations of different ethnic groups. We randomly picked chromosomes 22 and 9 for evaluation and further validated the results on chromosomes 13 (has the lowest gene density) and 19 (has the highest gene density). In practice, genotype imputation for big chromosomes and large sample size can be very computationally intensive using MACH through our brute force approach. Due to the extensive computing resources required, a detailed evaluation of the genotype imputation for Latinos based on 1KGP in other chromosomes is left for future studies. Moreover, our LALES GWAS data were genotyped by the Illumina OmniExpress chip, which has limited coverage for rare variants.
Rare variants have been claimed to be the culprit for the missing heritability for many disease phenotypes. 1KGP is a growing resource to address this issue consisting of an extremely high-density reference panel, which covers an enormous number of rare variants. However, the sample size for each ethnic group is still limited, especially for minority populations. Recent studies have shown that thousands of reference samples may be required to successfully impute rare SNPs (Li et al., 2011). This level of reference panel size requirement can be easily understood through a probability calculation. With 200 references (400 haplotypes), the probability to observe an allele/haplotype of MAF = 0.01 at least once is equal to 1 − (1 − 0.01)400 = 0.98. However, it requires 400 references (800 haplotypes) and 2000 references (4000 haplotypes) to observe an allele/haplotype of MAF = 0.005 and MAF = 0.001, respectively, with a similar level of probability. Therefore, the yield of 1KGP based imputation for rare variants will still be constrained by the limited panel size of each ethnic group. It may help to bring in references from other ethnic backgrounds. But the effectiveness of this strategy will depend upon the genetic distance between the reference and target samples.
Among all the tested reference panels, we found that the 1KGP AMR + CEU + YRI panel gave the lowest error rate for genotype imputation in Latinos. This is not totally unexpected because the Latino population is considered as primarily the result of a three-way admixture of European, Native American, and West African populations (Mao et al., 2007; Price et al., 2007). Admixture analysis by STRUCTURE (Pritchard et al., 2000; Falush et al., 2003) indicated that our Latino subjects had 53, 43, and 4% Caucasian, Native American, and African ancestry, respectively (data not shown). Therefore, when the reference resource is limited in sample number for a particular population, it can help to bring in references of other ancestral populations. Some researchers reported a cosmopolitan panel, which includes all the references, gave better imputation results in some situations (Huang et al., 2009; Li et al., 2010; Howie et al., 2011). We believe use of the cosmopolitan panel is most likely to improve results when the imputation resource of the same population for a target sample is sparse. In HM3, even CEU + YRI + JPT + CHB (which contains no MEX) gave better results than MEX alone. Thus, the relative utility of a reference consisting of a combination of Caucasian, African, and Asian references, and one consisting of just MEX, will depend upon the size of each reference panel.
To date, most of genotype imputation evaluations were done in samples of European, African, and Asian ancestry (Pei et al., 2008; Huang et al., 2009, 2011; Fridley et al., 2010; Shriner et al., 2010; Howie et al., 2011; Li et al., 2011) and only limited reports explored the imputation using 1KGP data (Sung et al., 2011). We present the first extensive evaluation of genotyping imputation for Latinos using the HapMap and 1KGP reference panels. Our results show that (1) The cosmopolitan panel, which includes all the references in 1KGP, is not an optimal solution for the genotype imputation for Latinos; (2) The 1KGP AMR + CEU + YRI reference panel provides the highest imputation accuracy for Latinos, and that also including Asian samples in the panel can reduce imputation accuracy. We also provide the imputation accuracy for each autosomal chromosome using the 1KGP panel for Latinos. Therefore, our results serve as a guide to future imputation based analysis in Latinos.
Web Resources
The URLs for data presented herein are as follows:
HAPGEN, https://mathgen.stats.ox.ac.uk/genetics_software/hapgen/hapgen2.html
IMPUTE, https://mathgen.stats.ox.ac.uk/impute/impute_v1.html#Using_IMPUTE_with_the_HapMap_Data
EIGENSOFT, http://genepath.med.harvard.edu/∼reich/Software.htm
MACH, http://www.sph.umich.edu/csg/abecasis/MACH/download/
HapMap Phase 3 haplotypes, http://www.sph.umich.edu/csg/abecasis/MACH/download/HapMap3.r2.b36.html
1000 Genomes Project Phase I haplotypes, http://www.sph.umich.edu/csg/abecasis/MACH/download/1000G-PhaseI-Interim.html
1000 Genomes Project, http://www.1000genomes.org/
HapMap Project, http://hapmap.ncbi.nlm.nih.gov/
Conflict of Interest Statement
The authors declare that the research was conducted in the absence of any commercial or financial relationships that could be construed as a potential conflict of interest.
Acknowledgments
This work was supported in part by the James H. Zumberge Faculty Research and Innovation Fund at the University of Southern California (to Xiaoyi Gao) and by NIH grant 5U10EY011753-12 (to Rohit Varma). We thank the study participants in LALES, and study staff who helped with the data collection.
References
Altshuler, D. M., Gibbs, R. A., Peltonen, L., Dermitzakis, E., Schaffner, S. F., Yu, F., Bonnen, P. E., De Bakker, P. I., Deloukas, P., Gabriel, S. B., Gwilliam, R., Hunt, S., Inouye, M., Jia, X., Palotie, A., Parkin, M., Whittaker, P., Chang, K., Hawes, A., Lewis, L. R., Ren, Y., Wheeler, D., Muzny, D. M., Barnes, C., Darvishi, K., Hurles, M., Korn, J. M., Kristiansson, K., Lee, C., Mccarrol, S. A., Nemesh, J., Keinan, A., Montgomery, S. B., Pollack, S., Price, A. L., Soranzo, N., Gonzaga-Jauregui, C., Anttila, V., Brodeur, W., Daly, M. J., Leslie, S., Mcvean, G., Moutsianas, L., Nguyen, H., Zhang, Q., Ghori, M. J., Mcginnis, R., Mclaren, W., Takeuchi, F., Grossman, S. R., Shlyakhter, I., Hostetter, E. B., Sabeti, P. C., Adebamowo, C. A., Foster, M. W., Gordon, D. R., Licinio, J., Manca, M. C., Marshall, P. A., Matsuda, I., Ngare, D., Wang, V. O., Reddy, D., Rotimi, C. N., Royal, C. D., Sharp, R. R., Zeng, C., Brooks, L. D., and Mcewen, J. E. (2010). Integrating common and rare genetic variation in diverse human populations. Nature 467, 52–58.
Browning, B. L., and Browning, S. R. (2009). A unified approach to genotype imputation and haplotype-phase inference for large data sets of trios and unrelated individuals. Am. J. Hum. Genet. 84, 210–223.
Browning, S. R., and Browning, B. L. (2007). Rapid and accurate haplotype phasing and missing-data inference for whole-genome association studies by use of localized haplotype clustering. Am. J. Hum. Genet. 81, 1084–1097.
Browning, S. R., and Browning, B. L. (2011). Haplotype phasing: existing methods and new developments. Nat. Rev. Genet. 12, 703–714.
Durbin, R. M., Abecasis, G. R., Altshuler, D. L., Auton, A., Brooks, L. D., Gibbs, R. A., Hurles, M. E., and Mcvean, G. A. (2010). A map of human genome variation from population-scale sequencing. Nature 467, 1061–1073.
Falush, D., Stephens, M., and Pritchard, J. K. (2003). Inference of population structure using multilocus genotype data: linked loci and correlated allele frequencies. Genetics 164, 1567–1587.
Frazer, K. A., Ballinger, D. G., Cox, D. R., Hinds, D. A., Stuve, L. L., Gibbs, R. A., Belmont, J. W., Boudreau, A., Hardenbol, P., Leal, S. M., Pasternak, S., Wheeler, D. A., Willis, T. D., Yu, F., Yang, H., Zeng, C., Gao, Y., Hu, H., Hu, W., Li, C., Lin, W., Liu, S., Pan, H., Tang, X., Wang, J., Wang, W., Yu, J., Zhang, B., Zhang, Q., Zhao, H., Zhou, J., Gabriel, S. B., Barry, R., Blumenstiel, B., Camargo, A., Defelice, M., Faggart, M., Goyette, M., Gupta, S., Moore, J., Nguyen, H., Onofrio, R. C., Parkin, M., Roy, J., Stahl, E., Winchester, E., Ziaugra, L., Altshuler, D., Shen, Y., Yao, Z., Huang, W., Chu, X., He, Y., Jin, L., Liu, Y., Sun, W., Wang, H., Wang, Y., Xiong, X., Xu, L., Waye, M. M., Tsui, S. K., Xue, H., Wong, J. T., Galver, L. M., Fan, J. B., Gunderson, K., Murray, S. S., Oliphant, A. R., Chee, M. S., Montpetit, A., Chagnon, F., Ferretti, V., Leboeuf, M., Olivier, J. F., Phillips, M. S., Roumy, S., Sallee, C., Verner, A., Hudson, T. J., Kwok, P. Y., Cai, D., Koboldt, D. C., Miller, R. D., Pawlikowska, L., Taillon-Miller, P., Xiao, M., Tsui, L. C., Mak, W., Song, Y. Q., Tam, P. K., Nakamura, Y., Kawaguchi, T., Kitamoto, T., Morizono, T., Nagashima, A., Ohnishi, Y., Sekine, A., Tanaka, T., Tsunoda, T., Deloukas, P., Bird, C. P., Delgado, M., Dermitzakis, E. T., Gwilliam, R., Hunt, S., Morrison, J., Powell, D., Stranger, B. E., Whittaker, P., Bentley, D. R., Daly, M. J., de Bakker, P. I., Barrett, J., Chretien, Y. R., Maller, J., McCarroll, S., Patterson, N., Pe’er, I., Price, A., Purcell, S., Richter, D. J., Sabeti, P., Saxena, R., Schaffner, S. F., Sham, P. C., Varilly, P., Altshuler, D., Stein, L. D., Krishnan, L., Smith, A. V., Tello-Ruiz, M. K., Thorisson, G. A., Chakravarti, A., Chen, P. E., Cutler, D. J., Kashuk, C. S., Lin, S., Abecasis, G. R., Guan, W., Li, Y., Munro, H. M., Qin, Z. S., Thomas, D. J., McVean, G., Auton, A., Bottolo, L., Cardin, N., Eyheramendy, S., Freeman, C., Marchini, J., Myers, S., Spencer, C., Stephens, M., Donnelly, P., Cardon, L. R., Clarke, G., Evans, D. M., Morris, A. P., Weir, B. S., Tsunoda, T., Mullikin, J. C., Sherry, S. T., Feolo, M., Skol, A., Zhang, H., Zeng, C., Zhao, H., Matsuda, I., Fukushima, Y., Macer, D. R., Suda, E., Rotimi, C. N., Adebamowo, C. A., Ajayi, I., Aniagwu, T., Marshall, P. A., Nkwodimmah, C., Royal, C. D., Leppert, M. F., Dixon, M., Peiffer, A., Qiu, R., Kent, A., Kato, K., Niikawa, N., Adewole, I. F., Knoppers, B. M., Foster, M. W., Clayton, E. W., Watkin, J., Gibbs, R. A., Belmont, J. W., Muzny, D., Nazareth, L., Sodergren, E., Weinstock, G. M., Wheeler, D. A., Yakub, I., Gabriel, S. B., Onofrio, R. C., Richter, D. J., Ziaugra, L., Birren, B. W., Daly, M. J., Altshuler, D., Wilson, R. K., Fulton, L. L., Rogers, J., Burton, J., Carter, N. P., Clee, C. M., Griffiths, M., Jones, M. C., McLay, K., Plumb, R. W., Ross, M. T., Sims, S. K., Willey, D. L., Chen, Z., Han, H., Kang, L., Godbout, M., Wallenburg, J. C., L’Archevêque, P., Bellemare, G., Saeki, K., Wang, H., An, D., Fu, H., Li, Q., Wang, Z., Wang, R., Holden, A. L., Brooks, L. D., McEwen, J. E., Guyer, M. S., Wang, V. O., Peterson, J. L., Shi, M., Spiegel, J., Sung, L. M., Zacharia, L. F., Collins, F. S., Kennedy, K., Jamieson, R., and Stewart, J. (2007). A second generation human haplotype map of over 3.1 million SNPs. Nature 449, 851–861.
Fridley, B. L., Jenkins, G., Deyo-Svendsen, M. E., Hebbring, S., and Freimuth, R. (2010). Utilizing genotype imputation for the augmentation of sequence data. PLoS ONE 5, e11018. doi:10.1371/journal.pone.0011018
Fu, Y. P., Hallman, D. M., Gonzalez, V. H., Klein, B. E., Klein, R., Hayes, M. G., Cox, N. J., Bell, G. I., and Hanis, C. L. (2010). Identification of diabetic retinopathy genes through a genome-wide association study among Mexican-Americans from Starr County, Texas. J. Ophthalmol. 2010, 861291.
Fuchsberger, C., Howie, B., Stephens, M., Abecasis, G., and Marchini, J. (2011). Pre-phasing: a computationally efficient approach for imputing from new reference panels in genomewide association studies. Am. J. Hum. Genet. 36, S91.
Gao, X., and Edwards, T. L. (2011). Genome-wide association studies: where we are heading? World J. Med. Genet. 1, 23–35.
Howie, B., Marchini, J., and Stephens, M. (2011). Genotype imputation with thousands of genomes. G3 (Bethesda) 1, 457–470.
Howie, B. N., Donnelly, P., and Marchini, J. (2009). A flexible and accurate genotype imputation method for the next generation of genome-wide association studies. PLoS Genet. 5, e1000529. doi:10.1371/journal.pgen.1000529
Huang, J., Ellinghaus, D., Franke, A., Howie, B., and Li, Y. (2012). 1000 Genomes-based imputation identifies novel and refined associations for the Wellcome Trust Case Control Consortium phase 1 Data. Eur. J. Hum. Genet. [Epub ahead of print].doi:10.1038/ejhg.2012.3.
Huang, L., Jakobsson, M., Pemberton, T. J., Ibrahim, M., Nyambo, T., Omar, S., Pritchard, J. K., Tishkoff, S. A., and Rosenberg, N. A. (2011). Haplotype variation and genotype imputation in African populations. Genet. Epidemiol. 35, 766–780.
Huang, L., Li, Y., Singleton, A. B., Hardy, J. A., Abecasis, G., Rosenberg, N. A., and Scheet, P. (2009). Genotype-imputation accuracy across worldwide human populations. Am. J. Hum. Genet. 84, 235–250.
Kai, W., and Chen, G. (2011). GPU accelerated genotype imputation for low-coverage highthroughput whole-genome sequencing data. Am. J. Hum. Genet. 21, S58.
Kottgen, A., Pattaro, C., Boger, C. A., Fuchsberger, C., Olden, M., Glazer, N. L., Parsa, A., Gao, X., Yang, Q., Smith, A. V., O’Connell, J. R., Li, M., Schmidt, H., Tanaka, T., Isaacs, A., Ketkar, S., Hwang, S. J., Johnson, A. D., Dehghan, A., Teumer, A., Pare, G., Atkinson, E. J., Zeller, T., Lohman, K., Cornelis, M. C., Probst-Hensch, N. M., Kronenberg, F., Tonjes, A., Hayward, C., Aspelund, T., Eiriksdottir, G., Launer, L. J., Harris, T. B., Rampersaud, E., Mitchell, B. D., Arking, D. E., Boerwinkle, E., Struchalin, M., Cavalieri, M., Singleton, A., Giallauria, F., Metter, J., De Boer, I. H., Haritunians, T., Lumley, T., Siscovick, D., Psaty, B. M., Zillikens, M. C., Oostra, B. A., Feitosa, M., Province, M., De Andrade, M., Turner, S. T., Schillert, A., Ziegler, A., Wild, P. S., Schnabel, R. B., Wilde, S., Munzel, T. F., Leak, T. S., Illig, T., Klopp, N., Meisinger, C., Wichmann, H. E., Koenig, W., Zgaga, L., Zemunik, T., Kolcic, I., Minelli, C., Hu, F. B., Johansson, A., Igl, W., Zaboli, G., Wild, S. H., Wright, A. F., Campbell, H., Ellinghaus, D., Schreiber, S., Aulchenko, Y. S., Felix, J. F., Rivadeneira, F., Uitterlinden, A. G., Hofman, A., Imboden, M., Nitsch, D., Brandstatter, A., Kollerits, B., Kedenko, L., Magi, R., Stumvoll, M., Kovacs, P., Boban, M., Campbell, S., Endlich, K., Volzke, H., Kroemer, H. K., Nauck, M., Volker, U., Polasek, O., Vitart, V., Badola, S., Parker, A. N., Ridker, P. M., Kardia, S. L., Blankenberg, S., Liu, Y., Curhan, G. C., Franke, A., Rochat, T., Paulweber, B., Prokopenko, I., Wang, W., Gudnason, V., Shuldiner, A. R., Coresh, J., Schmidt, R., Ferrucci, L., Shlipak, M. G., van Duijn, C. M., Borecki, I., Krämer, B. K., Rudan, I., Gyllensten, U., Wilson, J. F., Witteman, J. C., Pramstaller, P. P., Rettig, R., Hastie, N., Chasman, D. I., Kao, W. H., Heid, I. M., and Fox, C. S. (2010). New loci associated with kidney function and chronic kidney disease. Nat. Genet. 42, 376–384.
Li, L., Li, Y., Browning, S. R., Browning, B. L., Slater, A. J., Kong, X., Aponte, J. L., Mooser, V. E., Chissoe, S. L., Whittaker, J. C., Nelson, M. R., and Ehm, M. G. (2011). Performance of genotype imputation for rare variants identified in exons and flanking regions of genes. PLoS ONE 6, e24945. doi:10.1371/journal.pone.0024945
Li, Y., and Abecasis, G. R. (2006). Mach 1.0: rapid haplotype reconstruction and missing genotype inference. Am. J. Hum. Genet. 79, S2290.
Li, Y., Willer, C., Sanna, S., and Abecasis, G. (2009). Genotype imputation. Annu. Rev. Genomics Hum. Genet. 10, 387–406.
Li, Y., Willer, C. J., Ding, J., Scheet, P., and Abecasis, G. R. (2010). MaCH: using sequence and genotype data to estimate haplotypes and unobserved genotypes. Genet. Epidemiol. 34, 816–834.
Mao, X., Bigham, A. W., Mei, R., Gutierrez, G., Weiss, K. M., Brutsaert, T. D., Leon-Velarde, F., Moore, L. G., Vargas, E., Mckeigue, P. M., Shriver, M. D., and Parra, E. J. (2007). A genomewide admixture mapping panel for Hispanic/Latino populations. Am. J. Hum. Genet. 80, 1171–1178.
Marchini, J., and Howie, B. (2010). Genotype imputation for genome-wide association studies. Nat. Rev. Genet. 11, 499–511.
Nothnagel, M., Ellinghaus, D., Schreiber, S., Krawczak, M., and Franke, A. (2009). A comprehensive evaluation of SNP genotype imputation. Hum. Genet. 125, 163–171.
Parra, E. J., Below, J. E., Krithika, S., Valladares, A., Barta, J. L., Cox, N. J., Hanis, C. L., Wacher, N., Garcia-Mena, J., Hu, P., Shriver, M. D., Kumate, J., Mckeigue, P. M., Escobedo, J., and Cruz, M. (2011). Genome-wide association study of type 2 diabetes in a sample from Mexico City and a meta-analysis of a Mexican-American sample from Starr County, Texas. Diabetologia 54, 2038–2046.
Patterson, N., Price, A. L., and Reich, D. (2006). Population structure and eigenanalysis. PLoS Genet. 2, e190. doi:10.1371/journal.pgen.0020190
Pei, Y. F., Li, J., Zhang, L., Papasian, C. J., and Deng, H. W. (2008). Analyses and comparison of accuracy of different genotype imputation methods. PLoS ONE 3, e3551. doi:10.1371/journal.pone.0003551
Price, A. L., Patterson, N., Yu, F., Cox, D. R., Waliszewska, A., Mcdonald, G. J., Tandon, A., Schirmer, C., Neubauer, J., Bedoya, G., Duque, C., Villegas, A., Bortolini, M. C., Salzano, F. M., Gallo, C., Mazzotti, G., Tello-Ruiz, M., Riba, L., Aguilar-Salinas, C. A., Canizales-Quinteros, S., Menjivar, M., Klitz, W., Henderson, B., Haiman, C. A., Winkler, C., Tusie-Luna, T., Ruiz-Linares, A., and Reich, D. (2007). A genomewide admixture map for Latino populations. Am. J. Hum. Genet. 80, 1024–1036.
Pritchard, J. K., and Przeworski, M. (2001). Linkage disequilibrium in humans: models and data. Am. J. Hum. Genet. 69, 1–14.
Pritchard, J. K., Stephens, M., and Donnelly, P. (2000). Inference of population structure using multilocus genotype data. Genetics 155, 945–959.
Purcell, S., Neale, B., Todd-Brown, K., Thomas, L., Ferreira, M. A., Bender, D., Maller, J., Sklar, P., De Bakker, P. I., Daly, M. J., and Sham, P. C. (2007). PLINK: a tool set for whole-genome association and population-based linkage analyses. Am. J. Hum. Genet. 81, 559–575.
Scheet, P., and Stephens, M. (2006). A fast and flexible statistical model for large-scale population genotype data: applications to inferring missing genotypes and haplotypic phase. Am. J. Hum. Genet. 78, 629–644.
Scott, L. J., Mohlke, K. L., Bonnycastle, L. L., Willer, C. J., Li, Y., Duren, W. L., Erdos, M. R., Stringham, H. M., Chines, P. S., Jackson, A. U., Prokunina-Olsson, L., Ding, C. J., Swift, A. J., Narisu, N., Hu, T., Pruim, R., Xiao, R., Li, X. Y., Conneely, K. N., Riebow, N. L., Sprau, A. G., Tong, M., White, P. P., Hetrick, K. N., Barnhart, M. W., Bark, C. W., Goldstein, J. L., Watkins, L., Xiang, F., Saramies, J., Buchanan, T. A., Watanabe, R. M., Valle, T. T., Kinnunen, L., Abecasis, G. R., Pugh, E. W., Doheny, K. F., Bergman, R. N., Tuomilehto, J., Collins, F. S., and Boehnke, M. (2007). A genome-wide association study of type 2 diabetes in Finns detects multiple susceptibility variants. Science 316, 1341–1345.
Semple, C. A. (2004). Deep genomics in shallow times: the finished sequence of human chromosomes 13 and 19. Eur. J. Hum. Genet. 12, 875–876.
Shi, M., London, S. J., Chiu, G. Y., Hancock, D. B., Zaykin, D., and Weinberg, C. R. (2011). Using imputed genotypes for relative risk estimation in case-parent studies. Am. J. Epidemiol. 173, 553–559.
Shriner, D., Adeyemo, A., Chen, G., and Rotimi, C. N. (2010). Practical considerations for imputation of untyped markers in admixed populations. Genet. Epidemiol. 34, 258–265.
Su, Z., Marchini, J., and Donnelly, P. (2011). HAPGEN2: simulation of multiple disease SNPs. Bioinformatics 27, 2304–2305.
Sung, Y. J., Wang, L., Rankinen, T., Bouchard, C., and Rao, D. C. (2011). Performance of genotype imputations using data from the 1000 genomes project. Hum. Hered. 73, 18–25.
The International HapMap Consortium. (2005). A haplotype map of the human genome. Nature 437, 1299–1320.
Varma, R., Paz, S. H., Azen, S. P., Klein, R., Globe, D., Torres, M., Shufelt, C., and Preston-Martin, S. (2004). The Los Angeles Latino Eye Study: design, methods, and baseline data. Ophthalmology 111, 1121–1131.
Appendix
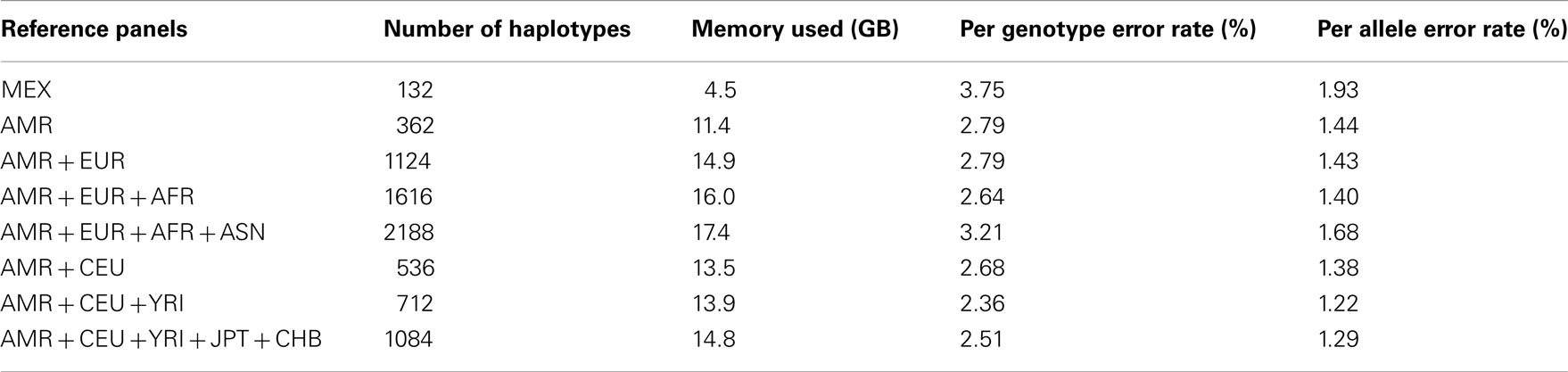
Table A1. Genotype imputation accuracy for chromosome 9 based on the 1000 Genomes Project reference panels.

Table A2. Genotype imputation accuracy for chromosome 13 based on the 1000 Genomes Project reference panels.

Table A3. Genotype imputation accuracy for chromosome 19 based on the 1000 Genomes Project reference panels.
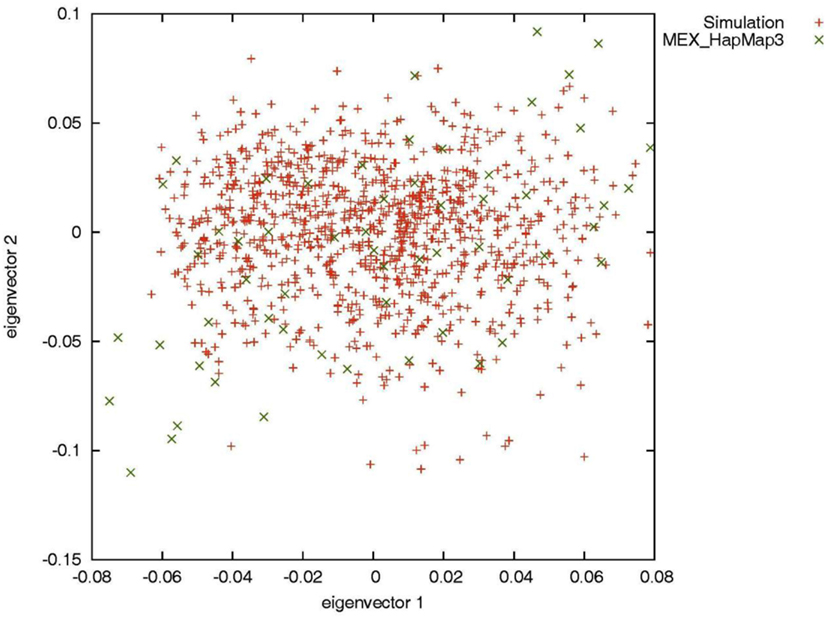
Figure A1. Principal components analysis of the simulated individuals and the HapMap Mexican–American individuals.
Keywords: genotype imputation, Latino, HapMap Project, 1000 Genomes Project
Citation: Gao X, Haritunians T, Marjoram P, Mckean-Cowdin R, Torres M, Taylor KD, Rotter JI, Gauderman WJ and Varma R (2012) Genotype imputation for Latinos using the HapMap and 1000 Genomes Project reference panels. Front. Gene. 3:117. doi: 10.3389/fgene.2012.00117
Received: 20 March 2012; Paper pending published: 12 April 2012;
Accepted: 05 June 2012; Published online: 27 June 2012.
Edited by:
Rongling Wu, Pennsylvania State University, USACopyright: © 2012 Gao, Haritunians, Marjoram, Mckean-Cowdin, Torres, Taylor, Rotter, Gauderman and Varma. This is an open-access article distributed under the terms of the Creative Commons Attribution Non Commercial License, which permits non-commercial use, distribution, and reproduction in other forums, provided the original authors and source are credited.
*Correspondence: Xiaoyi Gao, Departments of Ophthalmology and Preventive Medicine, Keck School of Medicine, University of Southern California, Los Angeles, CA 90033, USA. e-mail:eGlhb3lpZ2FAdXNjLmVkdQ==