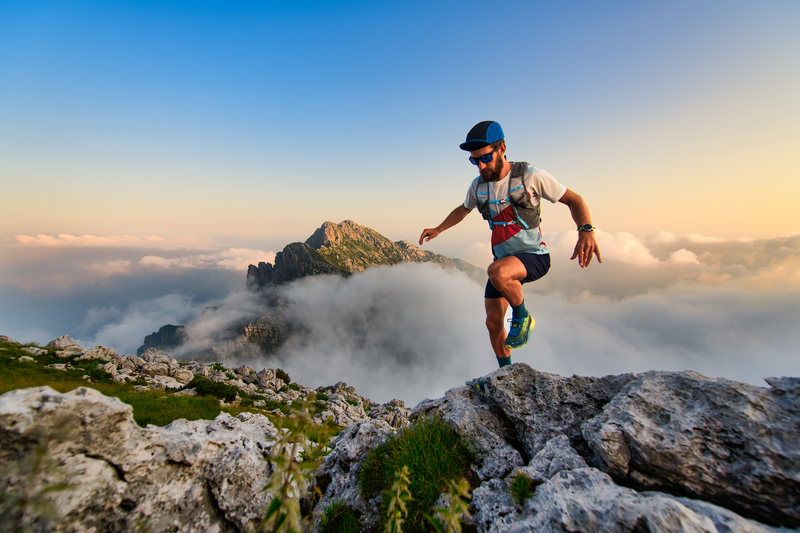
95% of researchers rate our articles as excellent or good
Learn more about the work of our research integrity team to safeguard the quality of each article we publish.
Find out more
HYPOTHESIS AND THEORY article
Front. Genet. , 26 April 2012
Sec. RNA
Volume 3 - 2012 | https://doi.org/10.3389/fgene.2012.00057
This article is part of the Research Topic Genomic “dark matter”: implications for understanding human disease mechanisms, diagnostics, and cures. View all 12 articles
Perhaps no other topic in contemporary genomics has inspired such diverse viewpoints as the 95+% of the genome, previously known as “junk DNA,” that does not code for proteins. Here, we present a theory in which dark matter RNA plays a role in the generation of a landscape of spatial micro-domains coupled to the information signaling matrix of the nuclear landscape. Within and between these micro-domains, dark matter RNAs additionally function to tether RNA interacting proteins and complexes of many different types, and by doing so, allow for a higher performance of the various processes requiring them at ultra-fast rates. This improves signal to noise characteristics of RNA processing, trafficking, and epigenetic signaling, where competition and differential RNA binding among proteins drives the computational decisions inherent in regulatory events.
The emerging picture of the nucleus portrays a multifaceted environment where RNA processing events occur with accuracy, precision, and high resolution. Since diffusion cannot account for the speed and coordination of the molecular events occurring within its matrix, the nucleus must depend on precisely articulated macromolecular architectures and active transport mechanisms to achieve adequate throughput and signal to noise performance (Lanctot et al., 2007; Misteli, 2007). In addition to the execution of baseline processing of RNA, the extensive network of RNA interaction machineries must respond to incoming physiological signaling, such as stress and cues from the physical environment (McKee and Silver, 2007; Sharma and Lou, 2011), by making rapid but precise changes at decision points, while at the same time maintaining robustness of the overall network. In effect, the entire nuclear space is a finely tuned RNA processing machine, designed to maintain accuracy in the dynamic and reversible regulation of myriads of transcriptome processing events simultaneously. Since the expansion of transcriptome processing increases the computational plasticity (Herbert and Rich, 1999) and the information processing capacity of biological networks (Mattick, 2007; St. Laurent and Wahlestedt, 2007), several authors argue that biological complexity itself has RNA complexity at its core (Licatalosi and Darnell, 2010).
Considering only current knowledge of these networks, and without extrapolating to as yet undiscovered regulatory intricacies, their performance already gracefully exceeds that of systems biology models and mechanisms. Its diversity of specific functions, and the finely tuned regulation of those functions in response to physiological signals, suggests the existence undiscovered mechanisms and network design principles at work to maintain robustness of the RNA output of a cell. In fact, recent studies of disease mechanisms suggest that humans can tolerate little loss of signal to noise performance in the nucleus. Healthy physiological function depends on the precision, reliability, and accuracy of the nuclear RNA processing machine, as processing errors in RNA molecules often lead to serious diseases (Garcia-Blanco et al., 2004; Cooper et al., 2009; Venables et al., 2009; Licatalosi and Darnell, 2010; Ward and Cooper, 2010; Jia et al., 2012).
It is in this context that we would like to consider the genomic “dark matter,” one of the major mysteries of the post-genome era. Perhaps no other topic in contemporary genomics has inspired such diverse viewpoints as the 95+% of the genome, previously known as “junk DNA,” that does not code for proteins. Reports of pervasive transcription of these vast “dark matter” regions, combined with frequent identification of families of long or very non-coding RNAs (lncRNAs) originating from them, have opened new chapters of both discovery as well as controversy. The observation that the percentage of “dark matter” genomic sequence correlates monotonically with organismal complexity, for every species sequenced to date (Taft et al., 2007), has inspired theories proposing a central role for these regions in the information processing of complex organisms (Mattick, 2007; St. Laurent and Wahlestedt, 2007). Yet, while an increasing number of specific interactions between lncRNAs and other biological molecules have demonstrated functions for a number of dark matter transcripts (Wang and Chang, 2011), a global concept of function has not yet emerged. In effect, the original reports of pervasive transcription (Kapranov et al., 2002,2007b; Carninci et al., 2005,2008; Katayama et al., 2005) of the mammalian genome have faded somewhat, with focus instead on separately developed lists of lncRNAs detected in specific experiments or filtered by certain properties that hint at functionality (Willingham et al., 2005; Guttman et al., 2009; Khalil et al., 2009; Wai et al., 2010; Askarian-Amiri et al., 2011; Khaitan et al., 2011). These lists of lncRNAs usually only cover a few percent of the genome, representing only a small fraction of the original pervasiveness of dark matter and typically, sample intergenic space as introns of known genes are usually assumed to represent pre-mRNAs. For example, our recent work has shown the presence of numerous very long transcribed regions of intergenic genomic space not currently covered by the lincRNA annotations (Kapranov et al., 2010) and has shown that introns of mouse genes produce stable RNAs regulated separately from the mature protein-coding RNAs (St. Laurent et al., submitted). Partly due to this uncertainty, some authors have cast doubt on the importance of dark matter transcripts, labeling them transcriptional noise (Brosius, 2005; Struhl, 2007; van Bakel and Hughes, 2009; Robinson, 2010; van Bakel et al., 2010), or even arguing that they largely represent “fragments of known pre-mRNAs” (van Bakel et al., 2010). Even the existence of much of the dark matter RNA implied by the early reports of pervasive transcription has stirred recent controversy (van Bakel et al., 2010). On balance, a common view in the field holds that while there is a collection of lncRNAs with specific interactions and functions, they exist among a larger collection of dark matter transcriptional noise.
As the controversy surrounding the function of “dark matter” RNA continues, a number of recent studies provided more comprehensive datasets, through the implementation of improved methodologies to confirm its existence and, more importantly, to measure its relative mass. A recent investigation designed to capture and measure non-exonic signals, revealed surprisingly that dark matter RNAs actually comprise a majority of non-ribosomal non-mitochondrial RNAs in human cells (Kapranov et al., 2010). We also know that the nucleus is rich in dark matter RNA (Cheng et al., 2005). Since the majority of protein-coding RNAs reside in the cytoplasm, the fraction of dark matter RNA is likely to be many folds higher in the nucleus than that of protein-coding RNAs.
Considering the vital importance of maintaining the performance of nuclear processing of all types, the nuclear molecular machineries would not tolerate the accumulation of large amounts of non-functional RNA molecules. Any significant population of such molecules would at best represent a large input of noise into the fine-tuned computational machinery of nuclear processing, not likely to benefit the performance of the nucleus or the cell as a unit. In practical terms, if dark matter had no biological function, the high performance and signal to noise ratios of the nuclear RNA processing machineries would logically conflict with the high levels of dark matter now documented in human cells (Kapranov et al., 2010). In other words, the currently emerging picture of the nucleus contains a paradox: a nuclear micro-environment simultaneously populated by high concentrations of precision RNA processing machineries, and by an astonishing level of noise from dark matter RNA. How can the nucleus precisely regulate such highly accurate processing events in tens of thousands of transcripts simultaneously, while ignoring the massive amount of inherent noise from dark matter RNA existing in the same nuclear space?
To resolve this apparent paradox, and to provide a mechanism for global function of dark matter RNA, in this article we present a theory in which dark matter RNA plays a role in the generation of a landscape of spatial micro-domains coupled to the information signaling matrix of the nuclear landscape. Within and between these micro-domains, dark matter RNAs additionally function to tether RNA interacting proteins and complexes of many different types, and by doing so, allow for a higher performance of the various processes requiring them at ultra-fast rates. This improves signal to noise characteristics of RNA processing, trafficking, and epigenetic signaling, where competition and differential RNA binding among proteins drives the computational decisions inherent in regulatory events.
It is estimated that an average human cells contains 300,000 mRNAs (Hastie and Bishop, 1976), each containing on average 10 exons, a start site, and a poly A+ tail. Thus, every such average molecule had to go through at least 18 splicing reactions (selection of splice donor and acceptor sites) plus selections of the start site and the polyadenylation site. In total, a minimum of 6M processing events had to occur to generate this diversity. This does not take into account (i) all subsequent base modification such as RNA editing, N6-methyladenosine, 5′-cap, (ii) subsequent cleavage events, or (iii) transportation of these RNA molecules to their sites of function or into well demarcated nuclear storage for later use. Nor does it account for the polyA- RNA population that exceeds that of the polyA+ by several folds. Also, if one were to include the ribosomal RNA, that represents ~95% of all cellular RNA (Raz et al., 2011), which is also processed and modified, then the minimal order of the number of cellular processing events needed to accommodate the real complexity of RNAs within a single nucleus is likely to be in the tens of millions.
As a vital step in transcript processing, RNA editing offers further insight into the high level of orchestration of nuclear RNA processing machineries. Adenosine deaminase acting on RNA (ADAR) mediates adenosine to inosine (A-to-I) RNA editing in dsRNA molecules, which often results in distinct downstream physiological outcomes for the edited RNAs. ADAR RNA editing frequently targets coding regions of mRNAs that encode ion channels and other components of the synaptic release machinery (Hoopengardner et al., 2003; Seeburg and Hartner, 2003). Intronic non-coding sequences with extensive complementarity to upstream or downstream exons containing the adenosine destined to be edited can form simple exon–intron hairpin structures (Higuchi et al., 1993; Burns et al., 1997; Hanrahan et al., 2000; Wang et al., 2000) or more complex RNA secondary structures such as a pseudoknot (Reenan, 2005). RNA editing in mRNAs often generates protein products that are not encoded by the literal genomic information, since upon translation the ribosomal machinery interprets inosines as guanosines (Basillo et al., 1962) resulting in amino acid substitutions. Various studies in different genetic model organisms suggest that RNA editing of mRNAs can result in profound changes in protein function (Rosenthal and Bezanilla, 2002; Bhalla et al., 2004; Ingleby et al., 2009).
Execution of this type of modification requires great deal of precision from the RNA processing machinery in terms of identification of RNA molecules to be edited, sites of editing within these molecules and also in the degree of editing at any given site. Editing could be separated into “pinpoint” and “prolific.” The former one results in editing at specific sites in specific RNA molecules. In Drosophila for example, the nervous system editing sites generally demonstrate a high level of conservation across 12 fly genomes, representing 85 million years of evolutionary divergence (Hoopengardner et al., 2003). This high level of conservation includes sites that code for levels of transcript editing in the adult fly as low as a few percent, demonstrating physiological sensitivity for this form of transcript processing. In addition, some RNAs that form extensive dsRNA structures, such as non-coding transcripts, sense–antisense RNAs bound to each other, and exogenous RNAs can serve as ADAR substrates destined for prolific editing (Bass, 2002; Nishikura, 2006), resulting in up to 50% A-to-I conversions (Nishikura et al., 1991; Polson and Bass, 1994). Choice of such substrates is also controlled as not every RNA molecule that can form dsRNA will be edited and not every adenosine in molecules that are substrates for ADAR is edited. The fate of such inosine-rich RNA molecules is different from the ones subject to “pinpoint” editing. They can in fact have at least two fates: retention within the nuclear compartment through dependent localization by p54nrb/Vigilin (Zhang and Carmichael, 2001; Wang et al., 2005) and cytoplasmic degradation by Tudor-SN (Scadden, 2005).
Furthermore, the ADAR information processing pathway is sensitive to environmental stimuli in addition to stress responses. Editing analysis of K+ channel mRNAs between Arctic and tropical octopus species revealed substantial differences in editing levels, which are mediated by temperature variations (Garrett and Rosenthal, 2012). In humans, the three ADAR genes can undergo alternative splicing to produce over a dozen isoforms with heterogeneous RNA target specificities. The inflammatory cascade results in a dramatic induction of many of these ADAR isoforms, resulting in a widespread increase of edited RNAs during mammalian inflammation (Yang et al., 2003a,2003b). Since intronic sequences form dsRNAs with coding regions to serve as ADAR substrates, editing must precede splicing. During these circumstances a regulatory mechanism must exist to ensure an accurate coordination of an extensive network of RNA processing machines to operate with high fidelity to generate dynamic responses upon internal and external stimuli.
In addition to the plethora of transcript variation discussed above, the RNAs produced subsequently traffic into predetermined subcellular localizations. Many transcripts interact with sets of trafficking proteins to migrate to specific nuclear locations such as interchromatin granules (ICGs) or speckles (Spector and Lamond, 2011), for further processing in response to transient physiological signals. Transcripts can also undergo complex cleavage events, followed by 5′ capping, in response to little understood signals and circumstances (Affymetrix/CSHL ENCODE Project, 2009; Mercer et al., 2010). CTN RNA represents an intriguing example where both of these mechanisms are combined. Within minutes of amino acid deprivation or similar cellular stress, signals transduced into the nucleus result in cleavage of the sequestered CTN RNA, and the release and transport to the cytoplasmic translation machinery of the amino acid transporter for which the cleaved RNA product codes (Prasanth et al., 2005). In some cases, cleavage events themselves produce small RNAs, whose activities feedback into splicing decisions, as in the example of the HBII52 snoRNA, which is cleaved from intronic RNA templates in the SNURF-SNRPN locus, and interacts with the serotonin 2C mRNA to regulate its alternative splicing (Kishore and Stamm, 2006).
Considering all of these regulatory layers together, millions of RNA processing events have to happen with accuracy and precision to generate the complexity of RNA present in a cell at any given moment. Many of these events require computation-like decision making as multiple alternative outcomes are available to a cell. In some cases, a single locus can produce hundreds, or even thousands of alternative products of RNA processing. The Drosophila Dscam locus for example, can produce 37,000 distinct isoforms from one “gene” (Wojtowicz et al., 2004). High throughput studies using RNA-seq revealed that 94% of human genes undergo alternative splicing in some tissue (Wang et al., 2008). In light of this output volume, such widespread reliance on alternative splicing points to the magnitude of the regulatory challenge facing nuclear splicing machineries. Since the RNA signals that code for splicing events contain relatively low sequence complexity, and frequently diverge from consensus sequences (Egecioglu and Chanfreau, 2011), they provide only modest energetic and informatic vectors to support the accuracy and reliability of high volume splicing output. As a result, achieving a correct splicing decision at a given site usually depends on a precise sequence of combinatorial events, composed of multiple protein and RNA elements, and even chromatin adaptor systems (Luco et al., 2011), acting both in competition (Witten and Ule, 2011), and in cooperation (Hertel, 2008; Xiao and Lee, 2010).
Performance related challenges would face any system designed to produce such a wide array of molecular outputs. Yet, even with these challenges, the systems performance of nuclear RNA processing appears to be surprisingly high. A recent investigation of cell-to-cell variability of alternative splicing determined that non-transformed cells maintained very low splicing isoform variability between individual cells, and concluded that mammalian cells minimize fluctuations in mRNA isoform ratios by tightly regulating the splicing machinery (Waks et al., 2011). Evidence increasingly supports precise and finely tuned regulation of transcriptome processing events as a rule in the nucleus. For example, a growing number of reports describe links between perturbations in splicing (Cooper et al., 2009; Ward and Cooper, 2010), or transcript localization (Faghihi et al., 2008) and diseases. This trend underlines the importance of high precision and accuracy in RNA based machineries under healthy physiological conditions.
Thus, using as yet little known organizational principles, nuclear RNA processing machineries not only produce processed and modified RNAs with high efficiency and accuracy, but implement a large scale integration of dynamic physiological signals, which then drive precise regulatory control and plasticity in response to a myriad of signaling events. From this perspective, the nuclear RNA processing machineries, and the dynamic structural environment surrounding them, must orchestrate their tasks with high accuracy and precision. They must recognize and distinguish processing motifs in RNA structural signals with high sensitivity and yet reject sub-optimal motifs with a high selectivity. Furthermore, the catalytic processes involved in the processing steps must occur with little or no errors. Once formed, the products must enter downstream trafficking pathways, and RNA whose presence is no longer required must be rapidly degraded to avoid introducing noise into earlier steps in the processing pathways due to RNA-waste accumulation. Finally, the entire multilayer system must maintain sufficient plasticity to quickly respond to thousands of potential information signals from outside the nucleus to generate appropriate alterations in processing steps at determined loci in response to changing physiological signals.
While the mystery of how all of this occurs within the space of the nucleus remains unresolved, it depends on the choreography of combinatorial interactions between transcripts and hundreds of RNA binding proteins (RBPs). RBPs interact with complex combinations of primary and secondary structure signals in RNA, and function in both cooperative and competitive types of architectures, as documented in splicing regulation (Darnell, 2006; Sharma and Black, 2006; Ule and Darnell, 2007; Licatalosi et al., 2008; Hallegger et al., 2010), and more recently in chromatin signaling (Tsai et al., 2010; Zhao et al., 2010). The first RBPs to interact with a given nascent transcript can influence the subsequent folding steps of that RNA, and thereby change the downstream distribution of protein interactions for that RNA. The process of differential recognition of nascent RNA information signals by the correct RBP must occur at a pace complementary to that of transcription as well as subsequent processing or chromatin signaling. To maintain plasticity for the accurate transduction of environmental signals, the RNA–protein interaction landscape must somehow achieve an extraordinary coupling between computational, catalytic, and structural elements.
As investigations continue to reveal the depth and performance of nuclear RNA processing functions, the challenge for systems biologists grows more daunting. Current systems biology modeling cannot account for the precision, accuracy, or signal to noise ratios achieved by RNA processing machineries. Nevertheless, the transcriptome–proteome interface in the nucleus contains a vast store of dynamical information. To consistently make effective use of this information, the nuclear systems network architecture must have a number of key design features, including maintenance of reversibility, temporal coherence (the timing and velocity of information processing between network layers), and the ability to resolve logical conflicts over the spatial extent of the networks that comprise the system. To help explain how nuclear RNA processing networks harness the power of that information, a number of concepts have emerged.
Biological molecules in the nuclear space exist in a constrained environment where diffusion occurs relatively slowly (Albert et al., 2012). Thus, biochemical kinetics can represent hurdle for adequate performance of complex multistep processing pathways, as the entire interdependent system must minimize bottlenecks and flow imbalances. In order to overcome these physical limitations, RNA processing machineries must rely on highly articulated spatial domains, where local environments transduce information efficiently. The concept of global scaffolding can create these performance enhancing interaction topologies. For example, an RNA scaffold can increase the local concentration of an RBP, such as Nova 1, and a corresponding increase in the signal to noise performance of Nova’s influence on splice site selection within that particular spatial domain (Figure 1A). Increasing the local concentration of these factors also permits improved interaction kinetics with less ΔG, making the interactions more reversible (Figure 1B and also below).
FIGURE 1. (A) Information currently available about the regions of dark matter transcription and the actual RNA molecules made from these region comes from various types of experiments and databases. There is relatively little overlap between these different databases suggesting that the actual extend of dark matter transcription is far greater than any one database suggests. (B) A theoretical curve showing expected results of the fraction of the genome that is transcribed as a function of the number of biological sources whose RNA is profiled. The coverage of transcribed genome by protein coding genes including their introns is 42% and lincRNAs bring it up to 58%. However, the full extent of the transcribed genome is expected to be much greater than that.
Structural features in the nucleus that enhance transcriptional control and RNA processing contain a large amount of spatially and temporally coded information content. The nucleolus for example appears to depend on RNA secondary structure signals for its effective formation, as the absence of these RNA secondary structures resulted in complete disarray of the nucleolus (Peng and Karpen, 2007). The reversibility of such events in nuclear architecture means that elements responsible for their formation also encode sufficient information to detect external signals and respond with disassembly and transport of components to other spatial domains or downstream processing pathways (Spector and Lamond, 2011).
With their unique combination of primary, secondary, and tertiary structure, RNA offers a multiplicity of ways to code for biological information. At the core of this system is a language of RNA–protein, and RNA–RNA/DNA recognition implemented by RNA’s unique ability to couple analog and digital signals (St. Laurent and Wahlestedt, 2007). The efficient transduction of that information often depends on its timely recognition by the appropriate RBPs present in the immediate vicinity of an elongating primary transcript. As the transcript emerges from Pol II, it begins to fold. That folding is also influenced by the RBPs that are supposed to interact with it. They influence which of many folding paths that the RNA can take. If the correct RBPs are not right there to quickly associate with the RNA, then the RNA could take another folding path, which would in turn lead to a different set of downstream events, as in the case of Nova splicing proteins and their influence on upstream or downstream splice site choice. So the presence or absence of a given distribution of RBPs in the vicinity of a nascent RNA chain will influence a series of “memory states” that then modulate other processing events downstream. With such a large space of potential RNA–protein interactions, and the requirement for dynamic reversibility of many of their associated signaling events, the system faces a major challenge to achieve an adequate signal to noise ratio for effective function.
Active competition for recognition site on nascent RNA signals directly addresses these problems. Splicing regulation makes abundant use of competing RBPs to enhance the sensitivity, specificity, and regulatory control of splice site decision commitment (Ule and Darnell, 2006; Chen and Manley, 2009). Examples include PTB protein which antagonizes Nova (Polydorides et al., 2000) at overlapping recognition sites, establishing a sensitive switch between two splicing choices. Interesting examples from spliceosome quality control also demonstrate the importance of reversibility, such as involvement of ATPase Prp16p in both forward and discard splicing pathways (Koodathingal et al., 2010).
Competition may also drive accurate computation in the small RNA regulatory pathways, with duplex regions competing for recognition by ADAR vs Drosha/Dicer, with contrasting outcomes depending on which protein prevails (Nishikura, 2006). Similarly, many epigenetic signaling events may be mediated by competitive interactions between lncRNAs and protein components of signaling machineries (Lee, 2011). All of these regulatory mechanisms require effective concentrations of interacting proteins to achieve adequate signal to noise ratios. The Lin28–let7 miRNA interaction provides an interesting example of specificity that would be difficult to achieve with low protein concentrations (Nam et al., 2011; Piskounova et al., 2011).
Erasure of information presents a challenge for any complex system (Lloyd, 2001). In biological systems, thermodynamic constraints make the cost of information innately high, and yet its value can oscillate from vital to worthless or even harmful in seconds once the message or a signal encoded in it is transduced. The dynamics of this “volatile market” reality make erasure of biological information a high priority in any system, but especially in the nucleus where many network pathways converge. While DNA retains the permanent information, a large majority of the dynamical information exists within the transcriptome, as combinatorial accumulations of RNA–protein and RNA–RNA/DNA interactions.
Not surprisingly, reversibility is a key feature of information coding at the transcriptome–proteome interface. The conformational flexibility of RNAs, especially ncRNAs whose secondary structures are not constrained by coding regions, and the dynamic changes in their structure that can occur in response to protein binding and environmental signals provide not only increased symbolic information density, but contribute to the reversibility of RNA–protein interactions. Proteins that bind RNA also tend to contain natively unstructured regions. This could be the basis for structural articulation (i.e., the incorporation of information containing motifs and elements into nuclear scaffolding structures) that improves precise temporal and spatial choreography of RNA processing machineries. For example, interactions between RNA and their cognate proteins often involve natively unstructured regions in the protein, and similarly flexible structures in the RNA (Leulliot and Varani, 2001). These regions of evolutionarily coded local disorder contribute useful properties for information processing. Precisely orienting them within an articulated regional structure increases their sensitivity, specificity, and reversibility, thereby contributing directly to the throughput and precision of nuclear machineries. When these regions form a stepwise interaction with their RNA target, the entropy of the complex is decreased, thereby producing an “entropic spring” effect, which enhances reversibility when the interaction is no longer required (Tompa and Csermely, 2004). Together with reversibility of individual interactions within RNA–protein interaction networks, frequent feedback loops support the reversibility of these networks. These features operate cooperatively to facilitate the timely erasure of information, and the finely tuned response of RNA processing machineries to changes in signaling and various environmental conditions.
Thus, a central part of our argument maintains that the performance and throughput of nuclear RNA processing machineries requires the functional coupling of well-articulated spatial and temporal landscapes in order to maximize the flow of biological information through the components of RNA processing networks.
Several years ago, John Mattick presented the concept of the nucleus as an “RNA machine” (Amaral et al., 2008), arguing that much of the information processing in the nucleus occurs through RNA intermediates, and that ncRNA overcomes the prohibitive regulatory overhead associated with saturated protein–protein regulatory interactions in this environment (Gagen and Mattick, 2005). From the point of view of information theory, this implies that the RNA content of the nucleus functions in a manner roughly equivalent to an information channel, and that the “channel capacity” (information throughput) of this system depends on the available degrees of freedom of the combined population of RNA molecules contained in the system. Consequently, RNA quality control and degradation machinery must actively pursue the elimination of non-functional RNAs that would represent noise to the “RNA machine.” Yet, with the recent discovery that dark matter RNA makes up the majority of cellular RNA by mass (Kapranov et al., 2010; and an even greater majority in the nucleus), it appears that this enigmatic class of RNA does not represent noise in the RNA based information channel of the nucleus, and instead likely comprises an integral part of the information channel itself.
The information containing structural features of dark matter RNAs and their ability to interact with the nuclear proteome appear similar to their coding counterparts. If the primary sequence patterns and secondary structure motifs that determine protein interactions occur with similar densities in both classes of RNAs, then they must both exist in the nucleus in complex with proteins. If dark matter RNAs represented noise or spurious transcription, their predominant mass would compromise signal to noise ratio performance of RNA processing machineries in the entire nucleus, as they depend on the information derived from such interactions. Instead, its high concentration suggests that dark matter RNA functions at the core of the multilayer nuclear “RNA machine” (Amaral et al., 2008).
The large amount of dark matter RNA in the nucleus, establishes the basis for the “intelligent scaffold” concept. Each dark matter RNA acts either in cis or in trans, depending on its own information content (complex combinations of primary sequence motifs and secondary structures), and the proteins with which it interacts. Long dark matter RNAs can form several types of interactions with DNA, and other RNAs, inside spatial domains of chromatin. These can involve direct interactions between RNA and DNA, similar to what occurs between pRNAs transcribed from regions in between rRNA genes, and the T0 element in rRNA promoters (Mayer et al., 2006; Schmitz et al., 2010). Proteins can also mediate the interactions, as recently demonstrated for XIST and transcription factor YY1 (Jeon and Lee, 2011). Alternatively, proteins or RNAs can use co-transcriptional targeting where the transcript is tethered during transcription by RNA polymerase, similar to the mechanism of the TAR RNA targeting by HIV TAT protein (Brady and Kashanchi, 2005). Transcriptional targeting may also occur with the short ncRNAs transcribed from the 5′ ends of many human genes (Wei et al., 1998; Kapranov et al., 2007a; Kanhere and Jenner, 2012). Dark matter RNAs have all three of these mechanisms available to mediate their interactions with DNA and other RNAs, providing a large combinatorial basis for the formation of flexible complexes that drive spatial and computational integration.
As these RNAs accumulate into spatial micro-domains surrounding one or more genomic loci, they establish a region of nuclear space under their influence, which in turn attracts a variety of molecules. The RNAs can interact with many proteins, and other large and small RNAs, often with relatively low affinities, which results in a temporally and spatially distributed macromolecular landscape around that locus (see Figure 2). Since these molecules function primarily in transcriptome processing and epigenetic regulation, the dark matter guided landscapes would facilitate the structural and computational operations of both systems, as well as catalyze crosstalk between them. In this manner dark matter RNAs can effectively establish finely tuned concentration gradients of epigenetic signaling and RNA processing proteins (and small RNAs) for efficient operation of these systems. An intriguing example of this has recently been described as a “molecular cage” for PRC1 complexes. The “molecular cage” apparently uses a combination of methylated H3K27 moieties and low affinity binding sites on nascent lncRNAs to increase the local concentration of PRC1 for chromatin signaling (Beisel and Paro, 2011).
FIGURE 2. The information content of a hypothetical dark matter RNA. Combinations of primary sequence and secondary structure form high affinity interaction sites with high information content (left side). These specific interactions have a large ΔG. At the other end of the spectrum, the same RNA can have a large number of relatively non-specific interaction sites that nevertheless have biological information content and functional significance. Their absence would result in subtle loss of signal to noise characteristics across many affected pathways that could be unrelated to the RNA.
The intelligent scaffold mechanism facilitates the accumulation of higher concentrations of RBPs (and small RNAs) within chromatin regions, as well as the micro-partitioning of these regions at an optional resolution for RNA processing, epigenetic signaling, and transcript expression regulation. Macromolecules within these micro-domains can disassociate from their low affinity binding sites in these dark matter rich micro-regions, as they find higher affinity sites in nascent strands emerging from RNA Pol II transcription. Abundant sites of alternative localization in dark matter equates with more effective differential recognition of RNA motifs by competing RBPs, and increased reversibility of signal transduction in regulatory events. The key here is that signal to noise ratio is not driven only by the size of ΔG, but by the ratio of ΔG “protein A” to ΔG “protein B” or the ratio of ΔG site1 of protein A on the “target” nascent strand RNA molecule to ΔG site2 of the same protein A on the “repository” dark matter RNA molecule. This is shown as “Biological Information Content” on Figure 2. If both ΔGs are large compared to their difference, then the signal is low and the noise is high. A recent experiment that used RNAi knockdown to reduce the expression levels of splicing regulator SRSF1 confirmed the importance of high concentrations of RNA processing proteins to maintain adequate signal to noise ratios. Lowered concentrations of SRSF1 markedly increased the variance of splicing isoform ratios of the target transcript, measured in populations of single cells (Waks et al., 2011).
Adjacent micro-partitions could favor higher concentrations of some proteins over others, due to the heterogeneous distributions of low affinity binding sites along the lengths of dark matter RNA molecules in each micro-partition. The result, depicted in Figure 3, shows varying levels of sequestration of RNA processing components, depending on the systems performance requirements of each component. Overall, higher concentrations of effector components equate with faster kinetics and more finely tunable regulation, which in turn improve signal to noise ratios and system performance.
FIGURE 3. Distribution of different domains with varying ΔG affinities (Y-axis) for different RBPs (balls of different colors) along the length of a hypothetical series of micro-domains each composed of different (very) long non-coding RNAs (X-axis). The heterogeneous landscape of dark matter RNAs creates pockets of enrichment of these different RBPs.
The temporal and spatial dynamics of intelligent scaffolds permit integration of signals from many levels of biological information processing. Changes in the intelligent scaffolding environment of a three-dimensional chromatin micro-region can impact the dynamics of transcriptional folding, processing, localization, and degradation of transcripts as well as chromatin signaling (see Figure 4). For example, dark matter cleavage events can quickly change the structure of the micro-domain by sweeping away large numbers of proteins, RNAs, and scaffold, and at the same time generate small RNAs, or expose regions of RNA complementarity to small RNAs, as described in the recent theory of competing endogenous RNAs (ceRNAs) by the Pandolfi group (Salmena et al., 2012). Cleavage of very long dark matter RNAs, for example those coming from the vlinc regions (Kapranov et al., 2010), could occur even with their RBPs still attached. Cleaved RNAs could then function as lncRNAs. Small RNAs could also interact with sites in tethered vlincs, thereby acting as a sink, or by blocking sites that would otherwise be occupied by other signaling molecules. Under some circumstances, combinations of these other events could serve as the signal to trigger cleavage of the vlincs, which could then form a rapid feed-forward circuit as the cascade of cleavage continues in the entire micro-domain.
FIGURE 4. A nascent RNA strand being synthesized by RNA Pol II interacts with RBPs (balls of various colors), small RNAs and lincRNAs. Interaction between all these molecules is made possible by close proximity of these molecules in the nuclear micro-domains. RBPs and small RNAs are bound to (v)lincRNAs with relatively low specificity and the latter present them in exact architectural and temporal environment to the nascent strand that possess specific motifs for the former. Nuclease action cleaves the non-coding RNA template and thus changes the structure of the scaffold complex. This in turn can change the kinetics of the interaction between the RBPs and the small RNAs bound to it and the sequence of their presentation and interaction with the nascent strand of RNA.
While specific interactions drive the bulk of molecular information processing in biological systems, in the RNA based regulatory networks of the nucleus the performance characteristics of specific interactions are determined by the surrounding micro-environment. The dark matter RNA plays a key role in implementing the dynamic responsiveness of that surrounding micro-environment. Considering its importance, the concept of functions for dark matter RNAs should embrace a continuum, from those that arise from highly specific interactions, to those at the other end of the spectrum that involve lower affinity and less specificity, but nevertheless contribute to the synergistic attributes of the surrounding micro-environment. Those attributes permit the specific interactions, and facilitate their coordination and integration.
Evaluating dark matter RNAs in this fashion provides a context and explanation for the relatively low level of conservation of these RNAs, as many informational elements either do not require conservation, or require only functional conservation. As demonstrated for a growing list of ncRNAs, functionality does not require conservation, at least not in the same way that is known to occur for protein-coding sequences (Pang et al., 2006). The theory predicts increasing concentrations of dark matter complexed with RNA interacting proteins in complex organisms, and helps explain the direct correlation of organismal complexity with the genomic percentage of non-coding regions in all genomes sequenced to date (Taft et al., 2007). It also suggests expansion of regions of RNA interacting regions in proteomes of organisms as evolutionary complexity increases.
The dark matter intelligent scaffold concept focuses on the level of coupling between computation and spatial articulation. The theory holds that large increases in biological complexity required ever increasing levels of coupling between computation and structure, as a key driver of that complexity, and ultimately a measure of organismal fitness. Dark matter RNA was recruited to perform this function, to dynamically bridge these two ostensibly orthogonal dimensions, because its flexible structural and computation features endow it with special qualities to serve as a molecular intermediate in the coding, processing, and distribution of information.
The authors declare that the research was conducted in the absence of any commercial or financial relationships that could be construed as a potential conflict of interest.
We wish to thank Erica Dumais for expert artistic assistance with figure preparation and Juan Pablo Lizarazo for logistical support.
Affymetrix/CSHL ENCODE Project. (2009). Post-transcriptional processing generates a diversity of 5′-modified long and short RNAs. Nature 457, 1028–1032.
Albert, B., Leger-Silvestre, I., Normand, C., and Gadal, O. (2012). Nuclear organization and chromatin dynamics in yeast: biophysical models or biologically driven interactions? Biochim. Biophys. Acta. doi: 10.1016/j.bbagrm.2011.12.010 [Epub ahead of print].
Amaral, P. P., Dinger, M. E., Mercer, T. R., and Mattick, J. S. (2008). The eukaryotic genome as an RNA machine. Science 319, 1787–1789.
Askarian-Amiri, M. E., Crawford, J., French, J. D., Smart, C. E., Smith, M. A., Clark, M. B., Ru, K., Mercer, T. R., Thompson, E. R., Lakhani, S. R., Vargas, A. C., Campbell, I. G., Brown, M. A., Dinger, M. E., and Mattick, J. S. (2011). SNORD-host RNA Zfas1 is a regulator of mammary development and a potential marker for breast cancer. RNA 17, 878–891.
Basillo, C., Wahba, A., Lengyel, P., Speyer, J., and Ochoa, S. (1962). Synthetic polynucleotides and the amino acid code, V. Proc. Natl. Acad. Sci. U.S.A. 48, 613–616.
Bass, B. L. (2002). RNA editing by adenosine deaminases that act on RNA. Annu. Rev. Biochem. 71, 817–846.
Beisel, C., and Paro, R. (2011). Silencing chromatin: comparing modes and mechanisms. Nat. Rev. Genet. 12, 123–135.
Bhalla, T., Rosenthal, J. J., Holmgren, M., and Reenan, R. (2004). Control of human potassium channel inactivation by editing of a small mRNA hairpin. Nat. Struct. Mol. Biol. 11, 950–956.
Brady, J., and Kashanchi, F. (2005). Tat gets the “green” light on transcription initiation. Retrovirology 2, 69.
Brosius, J. (2005). Waste not, want not – transcript excess in multicellular eukaryotes. Trends Genet. 21, 287–288.
Burns, C. M., Chu, H., Rueter, S. M., Hutchinson, L. K., Canton, H., Sanders-Bush, E., and Emeson, R. B. (1997). Regulation of serotonin-2C receptor G-protein coupling by RNA editing. Nature 387, 303–308.
Carninci, P., Kasukawa, T., Katayama, S., Gough, J., Frith, M. C., Maeda, N., Oyama, R., Ravasi, T., Lenhard, B., Wells, C., Kodzius, R., Shimokawa, K., Bajic, V. B., Brenner, S. E., Batalov, S., Forrest, A. R., Zavolan, M., Davis, M. J., Wilming, L. G., Aidinis, V., Allen, J. E., Ambesi-Impiombato, A., Apweiler, R., Aturaliya, R. N., Bailey, T. L., Bansal, M., Baxter, L., Beisel, K. W., Bersano, T., Bono, H., Chalk, A. M., Chiu, K. P., Choudhary, V., Christoffels, A., Clutterbuck, D. R., Crowe, M. L., Dalla, E., Dalrymple, B. P., De Bono, B., Della Gatta, G., Di Bernardo, D., Down, T., Engstrom, P., Fagiolini, M., Faulkner, G., Fletcher, C. F., Fukushima, T., Furuno, M., Futaki, S., Gariboldi, M., Georgii-Hemming, P., Gingeras, T. R., Gojobori, T., Green, R. E., Gustincich, S., Harbers, M., Hayashi, Y., Hensch, T. K., Hirokawa, N., Hill, D., Huminiecki, L., Iacono, M., Ikeo, K., Iwama, A., Ishikawa, T., Jakt, M., Kanapin, A., Katoh, M., Kawasawa, Y., Kelso, J., Kitamura, H., Kitano, H., Kollias, G., Krishnan, S. P., Kruger, A., Kummerfeld, S. K., Kurochkin, I. V., Lareau, L. F., Lazarevic, D., Lipovich, L., Liu, J., Liuni, S., Mcwilliam, S., Madan Babu, M., Madera, M., Marchionni, L., Matsuda, H., Matsuzawa, S., Miki, H., Mignone, F., Miyake, S., Morris, K., Mottagui-Tabar, S., Mulder, N., Nakano, N., Nakauchi, H., Ng, P., Nilsson, R., Nishiguchi, S., Nishikawa, S., Nori, F., Ohara, O., Okazaki, Y., Orlando, V., Pang, K. C., Pavan, W. J., Pavesi, G., Pesole, G., Petrovsky, N., Piazza, S., Reed, J., Reid, J. F., Ring, B. Z., Ringwald, M., Rost, B., Ruan, Y., Salzberg, S. L., Sandelin, A., Schneider, C., Schüonbach, C., Sekiguchi, K., Semple, C. A., Seno, S., Sessa, L., Sheng, Y., Shibata, Y., Shimada, H., Shimada, K., Silva, D., Sinclair, B., Sperling, S., Stupka, E., Sugiura, K., Sultana, R., Takenaka, Y., Taki, K., Tammoja, K., Tan, S. L., Tang, S., Taylor, M. S., Tegner, J., Teichmann, S. A., Ueda, H. R., van Nimwegen, E., Verardo, R., Wei, C. L., Yagi, K., Yamanishi, H., Zabarovsky, E., Zhu, S., Zimmer, A., Hide, W., Bult, C., Grimmond, S. M., Teasdale, R. D., Liu, E. T., Brusic, V., Quackenbush, J., Wahlestedt, C., Mattick, J. S., Hume, D. A., Kai, C., Sasaki, D., Tomaru, Y., Fukuda, S., Kanamori-Katayama, M., Suzuki, M., Aoki, J., Arakawa, T., Iida, J., Imamura, K., Itoh, M., Kato, T., Kawaji, H., Kawagashira, N., Kawashima, T., Kojima, M., Kondo, S., Konno, H., Nakano, K., Ninomiya, N., Nishio, T., Okada, M., Plessy, C., Shibata, K., Shiraki, T., Suzuki, S., Tagami, M., Waki, K., Watahiki, A., Okamura-Oho, Y., Suzuki, H., Kawai, J., Hayashizaki, Y; FANTOM Consortium; RIKEN Genome Exploration Research Group and Genome Science Group (Genome Network Project Core Group). (2005). The transcriptional landscape of the mammalian genome. Science 309, 1559–1563.
Carninci, P., Yasuda, J., and Hayashizaki, Y. (2008). Multifaceted mammalian transcriptome. Curr. Opin. Cell Biol. 20, 274–280.
Chen, M., and Manley, J. L. (2009). Mechanisms of alternative splicing regulation: insights from molecular and genomics approaches. Nat. Rev. Mol. Cell Biol. 10, 741–754.
Cheng, J., Kapranov, P., Drenkow, J., Dike, S., Brubaker, S., Patel, S., Long, J., Stern, D., Tammana, H., Helt, G., Sementchenko, V., Piccolboni, A., Bekiranov, S., Bailey, D. K., Ganesh, M., Ghosh, S., Bell, I., Gerhard, D. S., and Gingeras, T. R. (2005). Transcriptional maps of 10 human chromosomes at 5-nucleotide resolution. Science 308, 1149–1154.
Darnell, R. B. (2006). Developing global insight into RNA regulation. Cold Spring Harb. Symp. Quant. Biol. 71, 321–327.
Egecioglu, D. E., and Chanfreau, G. (2011). Proofreading and spellchecking: a two-tier strategy for pre-mRNA splicing quality control. RNA 17, 383–389.
Faghihi, M. A., Modarresi, F., Khalil, A. M., Wood, D. E., Sahagan, B. G., Morgan, T. E., Finch, C. E., St. Laurent, G. III, Kenny, P. J., and Wahlestedt, C. (2008). Expression of a noncoding RNA is elevated in Alzheimer’s disease and drives rapid feed-forward regulation of beta-secretase. Nat. Med. 14, 723–730.
Gagen, M. J., and Mattick, J. S. (2005). Inherent size constraints on prokaryote gene networks due to “accelerating” growth. Theory Biosci. 123, 381–411.
Garcia-Blanco, M. A., Baraniak, A. P., and Lasda, E. L. (2004). Alternative splicing in disease and therapy. Nat. Biotechnol. 22, 535–546.
Garrett, S., and Rosenthal, J. J. (2012). RNA editing underlies temperature adaptation in K+ channels from polar octopuses. Science 335, 848–851.
Guttman, M., Amit, I., Garber, M., French, C., Lin, M. F., Feldser, D., Huarte, M., Zuk, O., Carey, B. W., Cassady, J. P., Cabili, M. N., Jaenisch, R., Mikkelsen, T. S., Jacks, T., Hacohen, N., Bernstein, B. E., Kellis, M., Regev, A., Rinn, J. L., and Lander, E. S. (2009). Chromatin signature reveals over a thousand highly conserved large non-coding RNAs in mammals. Nature 458, 223–227.
Hallegger, M., Llorian, M., and Smith, C. W. (2010). Alternative splicing: global insights. FEBS J. 277, 856–866.
Hanrahan, C. J., Palladino, M. J., Ganetzky, B., and Reenan, R. A. (2000). RNA editing of the Drosophila para Na(+) channel transcript. Evolutionary conservation and developmental regulation. Genetics 155, 1149–1160.
Hastie, N. D., and Bishop, J. O. (1976). The expression of three abundance classes of messenger RNA in mouse tissues. Cell 9, 761–774.
Herbert, A., and Rich, A. (1999). RNA processing and the evolution of eukaryotes. Nat. Genet. 21, 265–269.
Higuchi, M., Single, F. N., Kohler, M., Sommer, B., Sprengel, R., and Seeburg, P. H. (1993). RNA editing of AMPA receptor subunit GluR-B: a base-paired intron–exon structure determines position and efficiency. Cell 75, 1361–1370.
Hoopengardner, B., Bhalla, T., Staber, C., and Reenan, R. (2003). Nervous system targets of RNA editing identified by comparative genomics. Science 301, 832–836.
Ingleby, L., Maloney, R., Jepson, J., Horn, R., and Reenan, R. (2009). Regulated RNA editing and functional epistasis in Shaker potassium channels. J. Gen. Physiol. 133, 17–27.
Jeon, Y., and Lee, J. T. (2011). YY1 tethers Xist RNA to the inactive X nucleation center. Cell 146, 119–133.
Jia, Y., Mu, J. C., and Ackerman, S. L. (2012). Mutation of a U2 snRNA gene causes global disruption of alternative splicing and neurodegeneration. Cell 148, 296–308.
Kanhere, A., and Jenner, R. G. (2012). Noncoding RNA localisation mechanisms in chromatin regulation. Silence 3, 2.
Kapranov, P., Cawley, S. E., Drenkow, J., Bekiranov, S., Strausberg, R. L., Fodor, S. P., and Gingeras, T. R. (2002). Large-scale transcriptional activity in chromosomes 21 and 22. Science 296, 916–919.
Kapranov, P., Cheng, J., Dike, S., Nix, D. A., Duttagupta, R., Willingham, A. T., Stadler, P. F., Hertel, J., Hackermuller, J., Hofacker, I. L., Bell, I., Cheung, E., Drenkow, J., Dumais, E., Patel, S., Helt, G., Ganesh, M., Ghosh, S., Piccolboni, A., Sementchenko, V., Tammana, H., and Gingeras, T. R. (2007a). RNA maps reveal new RNA classes and a possible function for pervasive transcription. Science 316, 1484–1488.
Kapranov, P., Willingham, A. T., and Gingeras, T. R. (2007b). Genome-wide transcription and the implications for genomic organization. Nat. Rev. Genet. 8, 413–423.
Kapranov, P., St. Laurent, G., Raz, T., Ozsolak, F., Reynolds, C. P., Sorensen, P. H., Reaman, G., Milos, P., Arceci, R. J., Thompson, J. F., and Triche, T. J. (2010). The majority of total nuclear-encoded non-ribosomal RNA in a human cell is ‘dark matter’ un-annotated RNA. BMC Biol. 8, 149. doi: 10.1186/1741-7007-8-149
Katayama, S., Tomaru, Y., Kasukawa, T., Waki, K., Nakanishi, M., Nakamura, M., Nishida, H., Yap, C. C., Suzuki, M., Kawai, J., Suzuki, H., Carninci, P., Hayashizaki, Y., Wells, C., Frith, M., Ravasi, T., Pang, K. C., Hallinan, J., Mattick, J., Hume, D. A., Lipovich, L., Batalov, S., Engstrom, P. G., Mizuno, Y., Faghihi, M. A., Sandelin, A., Chalk, A. M., Mottagui-Tabar, S., Liang, Z., Lenhard, B., and Wahlestedt, C. (2005). Antisense transcription in the mammalian transcriptome. Science 309, 1564–1566.
Khaitan, D., Dinger, M. E., Mazar, J., Crawford, J., Smith, M. A., Mattick, J. S., and Perera, R. J. (2011). The melanoma-upregulated long noncoding RNA SPRY4-IT1 modulates apoptosis and invasion. Cancer Res. 71, 3852–3862.
Khalil, A. M., Guttman, M., Huarte, M., Garber, M., Raj, A., Rivea Morales, D., Thomas, K., Presser, A., Bernstein, B. E., Van Oudenaarden, A., Regev, A., Lander, E. S., and Rinn, J. L. (2009). Many human large intergenic noncoding RNAs associate with chromatin-modifying complexes and affect gene expression. Proc. Natl. Acad. Sci. U.S.A. 106, 11667–11672.
Kishore, S., and Stamm, S. (2006). The snoRNA HBII-52 regulates alternative splicing of the serotonin receptor 2C. Science 311, 230–232.
Koodathingal, P., Novak, T., Piccirilli, J. A., and Staley, J. P. (2010). The DEAH box ATPases Prp16 and Prp43 cooperate to proofread 5′ splice site cleavage during pre-mRNA splicing. Mol. Cell 39, 385–395.
Lanctot, C., Cheutin, T., Cremer, M., Cavalli, G., and Cremer, T. (2007). Dynamic genome architecture in the nuclear space: regulation of gene expression in three dimensions. Nat. Rev. Genet. 8, 104–115.
Lee, J. T. (2011). Gracefully ageing at 50, X-chromosome inactivation becomes a paradigm for RNA and chromatin control. Nat. Rev. Mol. Cell Biol. 12, 815–826.
Leulliot, N., and Varani, G. (2001). Current topics in RNA-protein recognition: control of specificity and biological function through induced fit and conformational capture. Biochemistry 40, 7947–7956.
Licatalosi, D. D., and Darnell, R. B. (2010). RNA processing and its regulation: global insights into biological networks. Nat. Rev. Genet. 11, 75–87.
Licatalosi, D. D., Mele, A., Fak, J. J., Ule, J., Kayikci, M., Chi, S. W., Clark, T. A., Schweitzer, A. C., Blume, J. E., Wang, X., Darnell, J. C., and Darnell, R. B. (2008). HITS-CLIP yields genome-wide insights into brain alternative RNA processing. Nature 456, 464–469.
Luco, R. F., Allo, M., Schor, I. E., Kornblihtt, A. R., and Misteli, T. (2011). Epigenetics in alternative pre-mRNA splicing. Cell 144, 16–26.
Mayer, C., Schmitz, K. M., Li, J., Grummt, I., and Santoro, R. (2006). Intergenic transcripts regulate the epigenetic state of rRNA genes. Mol. Cell 22, 351–361.
McKee, A. E., and Silver, P. A. (2007). Systems perspectives on mRNA processing. Cell Res. 17, 581–590.
Mercer, T. R., Dinger, M. E., Bracken, C. P., Kolle, G., Szubert, J. M., Korbie, D. J., Askarian-Amiri, M. E., Gardiner, B. B., Goodall, G. J., Grimmond, S. M., and Mattick, J. S. (2010). Regulated post-transcriptional RNA cleavage diversifies the eukaryotic transcriptome. Genome Res. 20, 1639–1650.
Misteli, T. (2007). Beyond the sequence: cellular organization of genome function. Cell 128, 787–800.
Nam, Y., Chen, C., Gregory, R. I., Chou, J. J., and Sliz, P. (2011). Molecular basis for interaction of let-7 microRNAs with Lin28. Cell 147, 1080–1091.
Nishikura, K. (2006). Editor meets silencer: crosstalk between RNA editing and RNA interference. Nat. Rev. Mol. Cell Biol. 7, 919–931.
Nishikura, K., Yoo, C., Kim, U., Murray, J. M., Estes, P. A., Cash, F. E., and Liebhaber, S. A. (1991). Substrate specificity of the dsRNA unwinding/modifying activity. EMBO J. 10, 3523–3532.
Pang, K. C., Frith, M. C., and Mattick, J. S. (2006). Rapid evolution of noncoding RNAs: lack of conservation does not mean lack of function. Trends Genet. 22, 1–5.
Peng, J. C., and Karpen, G. H. (2007). H3K9 methylation and RNA interference regulate nucleolar organization and repeated DNA stability. Nat. Cell Biol. 9, 25–35.
Piskounova, E., Polytarchou, C., Thornton, J. E., Lapierre, R. J., Pothoulakis, C., Hagan, J. P., Iliopoulos, D., and Gregory, R. I. (2011). Lin28A and Lin28B inhibit let-7 microRNA biogenesis by distinct mechanisms. Cell 147, 1066–1079.
Polson, A. G., and Bass, B. L. (1994). Preferential selection of adenosines for modification by double-stranded RNA adenosine deaminase. EMBO J. 13, 5701–5711.
Polydorides, A. D., Okano, H. J., Yang, Y. Y., Stefani, G., and Darnell, R. B. (2000). A brain-enriched polypyrimidine tract-binding protein antagonizes the ability of Nova to regulate neuron-specific alternative splicing. Proc. Natl. Acad. Sci. U.S.A. 97, 6350–6355.
Prasanth, K. V., Prasanth, S. G., Xuan, Z., Hearn, S., Freier, S. M., Bennett, C. F., Zhang, M. Q., and Spector, D. L. (2005). Regulating gene expression through RNA nuclear retention. Cell 123, 249–263.
Raz, T., Kapranov, P., Lipson, D., Letovsky, S., Milos, P. M., and Thompson, J. F. (2011). Protocol dependence of sequencing-based gene expression measurements. PLoS ONE 6, e19287. doi: 10.1371/journal. pone.0019287
Reenan, R. A. (2005). Molecular determinants and guided evolution of species-specific RNA editing. Nature 434, 409–413.
Robinson, R. (2010). Dark matter transcripts: sound and fury, signifying nothing? PLoS Biol. 8, e1000370. doi: 10.1371/journal.pbio.1000370
Rosenthal, J. J., and Bezanilla, F. (2002). Extensive editing of mRNAs for the squid delayed rectifier K+ channel regulates subunit tetramerization. Neuron 34, 743–757.
Salmena, L., Poliseno, L., Tay, Y., Kats, L., and Pandolfi, P. P. (2012). A ceRNA hypothesis: the Rosetta Stone of a hidden RNA language? Cell 146, 353–358.
Scadden, A. D. (2005). The RISC subunit Tudor-SN binds to hyper-edited double-stranded RNA and promotes its cleavage. Nat. Struct. Mol. Biol. 12, 489–496.
Schmitz, K. M., Mayer, C., Postepska, A., and Grummt, I. (2010). Interaction of noncoding RNA with the rDNA promoter mediates recruitment of DNMT3b and silencing of rRNA genes. Genes Dev. 24, 2264–2269.
Seeburg, P. H., and Hartner, J. (2003). Regulation of ion channel/neurotransmitter receptor function by RNA editing. Curr. Opin. Neurobiol. 13, 279–283.
Sharma, A., and Lou, H. (2011). Depolarization-mediated regulation of alternative splicing. Front. Neurosci. 5:141. doi: 10.3389/fnins.2011. 00141
Sharma, S., and Black, D. L. (2006). Maps, codes, and sequence elements: can we predict the protein output from an alternatively spliced locus? Neuron 52, 574–576.
Spector, D. L., and Lamond, A. I. (2011). Nuclear speckles. Cold Spring Harb. Perspect. Biol. 3, a000646. doi: 10.1101/cshperspect.a000646.
St. Laurent, G. III, and Wahlestedt, C. (2007). Noncoding RNAs: couplers of analog and digital information in nervous system function? Trends Neurosci. 30, 612–621.
Struhl, K. (2007). Transcriptional noise and the fidelity of initiation by RNA polymerase II. Nat. Struct. Mol. Biol. 14, 103–105.
Taft, R. J., Pheasant, M., and Mattick, J. S. (2007). The relationship between non-protein-coding DNA and eukaryotic complexity. Bioessays 29, 288–299.
Tompa, P., and Csermely, P. (2004). The role of structural disorder in the function of RNA and protein chaperones. FASEB J. 18, 1169–1175.
Tsai, M. C., Manor, O., Wan, Y., Mosammaparast, N., Wang, J. K., Lan, F., Shi, Y., Segal, E., and Chang, H. Y. (2010). Long noncoding RNA as modular scaffold of histone modification complexes. Science 329, 689–693.
Ule, J., and Darnell, R. B. (2006). RNA binding proteins and the regulation of neuronal synaptic plasticity. Curr. Opin. Neurobiol. 16, 102–110.
Ule, J., and Darnell, R. B. (2007). Functional and mechanistic insights from genome-wide studies of splicing regulation in the brain. Adv. Exp. Med. Biol. 623, 148–160.
van Bakel, H., and Hughes, T. R. (2009). Establishing legitimacy and function in the new transcriptome. Brief. Funct. Genomic Proteomic 8, 424–436.
van Bakel, H., Nislow, C., Blencowe, B. J., and Hughes, T. R. (2010). Most “dark matter” transcripts are associated with known genes. PLoS Biol. 8, e1000371. doi: 10.1371/journal.pbio.1000371
Venables, J. P., Klinck, R., Koh, C., Gervais-Bird, J., Bramard, A., Inkel, L., Durand, M., Couture, S., Froehlich, U., Lapointe, E., Lucier, J. F., Thibault, P., Rancourt, C., Tremblay, K., Prinos, P., Chabot, B., and Elela, S. A. (2009). Cancer-associated regulation of alternative splicing. Nat. Struct. Mol. Biol. 16, 670–676.
Wai, D. H., Wu, D. U., Wing, M. R., Arceci, R. J., Reynolds, C. P., Sorensen, P. H., Reaman, G. H., Milos, P. M.,. Lawlor, E. R, Buckley, J. D., Kapranov, P., and Triche, T. J. (2010). Large intergenic noncoding RNAs associated with Ewing sarcoma family of tumors. Proc. Am. Assoc. Cancer Res. #4087.
Waks, Z., Klein, A. M., and Silver, P. A. (2011). Cell-to-cell variability of alternative RNA splicing. Mol. Syst. Biol. 7, 506.
Wang, E. T., Sandberg, R., Luo, S., Khrebtukova, I., Zhang, L., Mayr, C., Kingsmore, S. F., Schroth, G. P., and Burge, C. B. (2008). Alternative isoform regulation in human tissue transcriptomes. Nature 456, 470–476.
Wang, K. C., and Chang, H. Y. (2011). Molecular mechanisms of long noncoding RNAs. Mol. Cell 43, 904–914.
Wang, Q., O’Brien, P. J., Chen, C. X., Cho, D. S., Murray, J. M., and Nishikura, K. (2000). Altered G protein-coupling functions of RNA editing isoform and splicing variant serotonin2C receptors. J. Neurochem. 74, 1290–1300.
Wang, Q., Zhang, Z., Blackwell, K., and Carmichael, G. G. (2005). Vigilins bind to promiscuously A-to-I-edited RNAs and are involved in the formation of heterochromatin. Curr. Biol. 15, 384–391.
Wei, P., Garber, M. E., Fang, S. M., Fischer, W. H., and Jones, K. A. (1998). A novel CDK9-associated C-type cyclin interacts directly with HIV-1 Tat and mediates its high-affinity, loop-specific binding to TAR RNA. Cell 92, 451–462.
Willingham, A. T., Orth, A. P., Batalov, S., Peters, E. C., Wen, B. G., Aza-Blanc, P., Hogenesch, J. B., and Schultz, P. G. (2005). A strategy for probing the function of noncoding RNAs finds a repressor of NFAT. Science 309, 1570–1573.
Witten, J. T., and Ule, J. (2011). Understanding splicing regulation through RNA splicing maps. Trends Genet. 27, 89–97.
Wojtowicz, W. M., Flanagan, J. J., Millard, S. S., Zipursky, S. L., and Clemens, J. C. (2004). Alternative splicing of Drosophila Dscam generates axon guidance receptors that exhibit isoform-specific homophilic binding. Cell 118, 619–633.
Xiao, X., and Lee, J. H. (2010). Systems analysis of alternative splicing and its regulation. Wiley Interdiscip. Rev. Syst. Biol. Med. 2, 550–565.
Yang, J. H., Luo, X., Nie, Y., Su, Y., Zhao, Q., Kabir, K., Zhang, D., and Rabinovici, R. (2003a). Widespread inosine-containing mRNA in lymphocytes regulated by ADAR1 in response to inflammation. Immunology 109, 15–23.
Yang, J. H., Nie, Y., Zhao, Q., Su, Y., Pypaert, M., Su, H., and Rabinovici, R. (2003b). Intracellular localization of differentially regulated RNA-specific adenosine deaminase isoforms in inflammation. J. Biol. Chem. 278, 45833–45842.
Zhang, Z., and Carmichael, G. G. (2001). The fate of dsRNA in the nucleus: a p54(nrb)-containing complex mediates the nuclear retention of promiscuously A-to-I edited RNAs. Cell 106, 465–475.
Keywords: dark matter RNA, non-coding RNA, vlinc RNA, RNA binding protein, biological signaling, RNA editing, RNA processing, molecular scaffold
Citation: St. Laurent G, Savva YA and Kapranov P (2012) Dark matter RNA: an intelligent scaffold for the dynamic regulation of the nuclear information landscape. Front. Gene. 3:57. doi: 10.3389/fgene.2012.00057
Received: 19 February 2012; Accepted: 28 March 2012;
Published online: 26 April 2012.
Edited by:
Claes Wahlestedt, University of Miami Miller School of Medicine, USAReviewed by:
Peng Jin, Emory University School of Medicine, USACopyright: © 2012 St. Laurent, Savva and Kapranov. This is an open-access article distributed under the terms of the Creative Commons Attribution Non Commercial License, which permits non-commercial use, distribution, and reproduction in other forums, provided the original authors and source are credited.
*Correspondence: Georges St. Laurent and Philipp Kapranov, St. Laurent Institute, One Kendall Square, Suite 200LL, Cambridge, MA 02139, USA. e-mail:Z2Vvcmdlc3Q5OEB5YWhvby5jb20=;cGhpbGlwcGswOEBnbWFpbC5jb20=
Disclaimer: All claims expressed in this article are solely those of the authors and do not necessarily represent those of their affiliated organizations, or those of the publisher, the editors and the reviewers. Any product that may be evaluated in this article or claim that may be made by its manufacturer is not guaranteed or endorsed by the publisher.
Research integrity at Frontiers
Learn more about the work of our research integrity team to safeguard the quality of each article we publish.