- 1 Laboratório de Química e Função de Proteínas e Peptídeos, Centro de Biociências e Biotecnologia, Universidade Estadual do Norte Fluminense Darcy Ribeiro, Campos dos Goytacazes, Brazil
- 2 National Center for Biotechnology Information, National Library of Medicine, National Institutes of Health, Bethesda, MD, USA
Large-scale chemical genetics screens (chemogenomics) in yeast have been widely used to find drug targets, understand the mechanism-of-action of compounds, and unravel the biochemistry of drug resistance. Chemogenomics is based on the comparison of growth of gene deletants in the presence and absence of a chemical substance. Such studies showed that more than 90% of the yeast genes are required for growth in the presence of at least one chemical. Analysis of these data, using computational approaches, has revealed non-trivial features of the natural chemical tolerance systems. As a result two non-overlapping sets of genes are seen to respectively impart robustness and evolvability in the context of natural chemical resistance. The former is composed of multidrug-resistance genes, whereas the latter comprises genes sharing chemical genetic profiles with many others. Recent publications showing the potential applications chemogenomics in studying the pharmacological basis of various drugs are discussed, as well as the expansion of chemogenomics to other organisms. Finally, integration of chemogenomics with sensitive sequence analysis and ubiquitination/phosphorylation data led to the discovery of a new conserved domain and important post-translational modification pathways involved in stress resistance.
Basic Concepts of Chemical Genetics
The budding yeast Saccharomyces cerevisiae with its unparalleled genetic tractability has undoubtedly become the model of choice for large-scale studies on transcription, protein–protein, and genetic interactions (Suter et al., 2006). Nevertheless, we are far from understanding biochemistry of all yeast gene products and their interactions (Pena-Castillo and Hughes, 2007). One of the major advances brought about by yeast functional genomics was the development of libraries of heterozygous and homozygous deletion strains (Winzeler et al., 1999; Giaever et al., 2002), in which copies (one or both) of a given gene are deleted. A key feature of such libraries is the insertion of unique sequences (bar codes) for the identification of each strain, allowing competitive growth experiments and rapid quantification using hybridization (microarrays; Shoemaker et al., 1996), or sequencing methods (Smith et al., 2009). The genome-wide application of deletant libraries to screen chemical compounds is called chemogenomics (or reverse chemical genetics; Roemer et al., 2011), which is based on growth of homozygous and/or heterozygous deletants in presence versus absence of a chemical compound can uncover functions of the gene in allowing growth in the presence of the compound (e.g., in a detoxification mechanism). When heterozygous libraries are used, there is also an opportunity to find the compound targets through drug-induced haploinsufficiency: with one gene copy deleted and the product of the other targeted by the bioactive molecule, resulting in a measurable growth deficiency (Giaever et al., 2004; Lum et al., 2004; Ho et al., 2011). Similarly, the gene dosage can also be increased by the use of plasmids and screening the transformed strains for resistance against a given chemical (Rine et al., 1983). Integration of different gene dosage techniques further increases the sensitivity and specificity of such chemogenomics approaches to define drug targets (Hoon et al., 2008). Importantly, it has been shown that there is a good correlation between the results obtained by independent groups employing different methodologies used (Hughes et al., 2004; Roemer et al., 2011).
Early studies suggested that 17–20% of the yeast genes are essential under standard laboratory conditions (Winzeler et al., 1999; Giaever et al., 2002). Despite the importance of the “essential gene complement” in defining certain core molecular processes (e.g., translation, transcription, and DNA replication), it fails to capture the gene functions associated with non-standard conditions (e.g., stress response). Several publications on chemogenomics (Winzeler et al., 1999; Birrell et al., 2002; Lum et al., 2004; Parsons et al., 2004, 2006; Hillenmeyer et al., 2008) and data integration (Parsons et al., 2004; Venancio et al., 2010a,b) have been shifting the paradigm of gene essentiality toward a condition-dependent view that is more resonant with the natural lifestyle of yeasts. Such a view finds strong support in a remarkable study by Hillenmeyer et al. (2008) showing that 95% of the yeast mutants had their fitness compromised in at least one environmental/chemical condition. Here we highlight recent discoveries in chemogenomics with special attention given to the evolution of chemical tolerance systems in fungi.
Systems Biology and the Nature of Chemical Genetic Interactions
Results from chemogenomics screens can be computationally represented and analyzed using matrix and graph-based (network) approaches (Sharom et al., 2004; Parsons et al., 2006; Wuster and Madan Babu, 2008). A chemical genetic network can be simply defined as a set of nodes (genes and chemicals) connected by directed edges (chemical genetic interactions; Figure 1). Because a chemical genetic interaction reflects the importance of a gene in the natural resistance against a given compound, highly connected genes in this network are called “multidrug-resistance” genes (MDRs). Using different analytical methods, several groups have shown that MDRs are clearly over-represented in transmembrane transporters and endosomal sorting complexes (ESCRT and retromer), implying that vesicular trafficking, and efflux pumps are major detoxification routes (Parsons et al., 2006; Hillenmeyer et al., 2008; Jo et al., 2008; Ruotolo et al., 2008; Venancio et al., 2010a; Roemer et al., 2011).
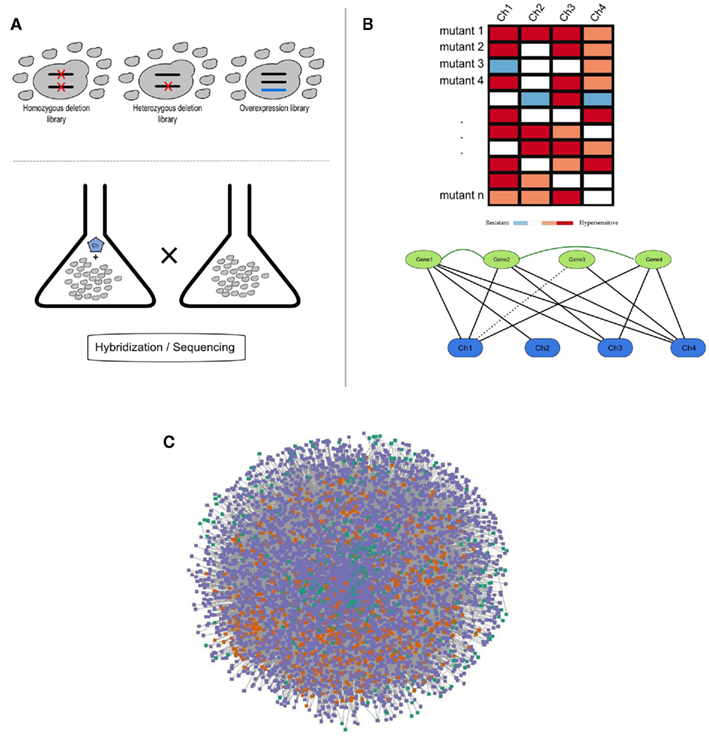
Figure 1. (A) Schematic representation of a chemogenomics screen. Homozygous, heterozygous, and overexpression mutant libraries are grown in the presence and absence of a chemical. The growth of each mutant is then measured using hybridization or sequencing methods; (B) Chemogenomics data can be represented as a heatmap or modeled as a network (black solid and dashed edges for susceptibility and resistance, respectively) and genes with similar chemical genetic profiles are subsequently linked (dark-green edges). This rationale was used to construct the SCPnet. Only interactions reflecting increased susceptibility were used; (C) Network representation of 34 integrated chemogenomic datasets (i.e., CPnet; Venancio et al., 2010b). Color codes: SCP-hubs (orange), chemicals (green), and other genes (purple). See the text for details on the SCP-hubs definition.
Using this framework, Venancio et al. assembled the chemical-phenotype network (CPnet), integrating data from 34 distinct chemogenomic datasets, comprising 5233 ORFs and 425 chemicals connected by 54,769 links. In addition to studying genes individually, it is likely that chemical tolerance involves epistatic (genetic) interactions between different genes. One way this issue can be approached is by using a network linking genes with significantly similar chemogenomic profiles (i.e., the Shared Chemical-Phenotype network, SCPnet; Venancio et al., 2010b). The SCPnet comprises 4631 genes and 40,102 edges, for which the significance was computed by simulating degree-preserving random CPnets and purging non-significant interactions (p > 0.001). In this context it should be noted that the degree distribution of these networks differs from many of the classical biological networks like the transcription network, the protein interaction network, or genetic interaction networks, which show power–law like degree distributions. Instead these networks are rather distinctive among biological networks in showing distinctive degree distributions whose tails might be approximated by exponential functions. Nonetheless, they contain a relatively small number of nodes with large degrees. These most highly connected genes might be termed “hubs” of these networks (Venancio et al., 2010b). Although network reconstructions from correlation data have some potential pitfalls (Gomez et al., 2009), support for the SCPnet was obtained from large-scale protein and genetic interaction networks (Venancio et al., 2010b). By analyzing genetic interactions among yeast genes conferring resistance to the methyl methanesulfonate (MMS; DNA-damaging chemical), St Onge et al. (2007) found support for distinct types of such genetic interactions. In contrast to almost all the biological networks studied so far, the SCPnet is highly assortative (Newman, 2003), showing a strong hub interconnectivity with might play some role in natural tolerance against chemical stress (Venancio et al., 2010b).
MDRs Versus SCPnet Hubs: What Do They Tell Us?
MDRs are strikingly under-represented among SCP-hubs, suggesting that the SCP-hubs counter chemical stress by a distinct mechanism (Venancio et al., 2010b). It emerges that SCP-hubs tend to have upstream TATA boxes, favoring transcription driven by the SAGA complex (Basehoar et al., 2004; Huisinga and Pugh, 2004). In contrast, MDRs tend to be TATA-less, typically dominated by the TFIID transcriptional complex (Basehoar et al., 2004; Huisinga and Pugh, 2004). It has been shown that the presence of upstream TATA boxes increases the variability in gene expression (i.e., noise), which can confer fitness advantages in drastically changing environmental conditions (Blake et al., 2006; Tirosh et al., 2006). SCP-hubs typically show significant divergence in their transcriptional output in long-term laboratory evolution experiments under sub-optimal conditions (Ferea et al., 1999). Further, SCP-hubs are enriched in fast-evolving genes, which might be restricted to the Saccharomycotina clade, and appear to be under positive selection. Rapid divergence and variability both at the level of the protein sequence and transcription regulation suggest the SCP-hubs as the evolvability (i.e., flexibility to change due to low selection pressure) component allowing the system to cope with rapidly changing conditions (Venancio et al., 2010b).
Genes required for growth under stress are generally not the ones induced by it (Birrell et al., 2002; Tai et al., 2007), probably because proteins required for rapid chemical tolerance must be immediately available upon exposure to harsh conditions. Stress-induced genes can be involved in the acquisition of resistance to impending severe stress that otherwise would kill the previously unexposed cells (Gasch et al., 2000; Berry et al., 2011). Further, responses to a primary stress can cross-protect against distinct secondary stress conditions (Berry et al., 2011). This phenomenon can result from similar downstream effects of various stresses or an evolutionary signature of a transcriptional preparation for successive harsh environmental conditions, as demonstrated for yeast (Berry and Gasch, 2008) and bacteria (Tagkopoulos et al., 2008; Mitchell et al., 2009). It was observed that SCP-hubs are over-represented in stress-induced genes, which along with their high evolvability let us to hypothesize a critical role in adaptive processes conferring stress tolerance (Venancio et al., 2010b). By definition, a single MDR gene can protect cells against various chemicals. Interestingly, MDRs are also over-represented among phenotypic capacitors – the genes that buffer phenotypic variation (Levy and Siegal, 2008), indicating that morphological robustness is a key feature of MDR.
Taken these observations together, the gene complement involved in stress response is partitioned in two major components: MDRs, which are more conserved, less-noisy in expression, and providing phenotypic capacitance. These features are likely to provide robustness to the system. SCP-hubs constitute a more variable system with noisy expression that could work by probing the changing environmental conditions with different counter-strategies. Interestingly, among the previously uncharacterized SCP-hubs are several distinct enzymatic domains, such as GCN5-like acetyltransferases (Ygr111w and Yor012w), NTN, and C–N hydrolases (Yil165c), among several others (Venancio et al., 2010b). The detoxification potential of such enzymatic activities has been recently shown by the identification of the SAM-dependent methyltransferase Crg1 as a key player in chemical stress response and lipid homeostasis. Follow-up experiments showed that Crg1 methylates cantharidin in vitro (Lissina et al., 2011). Interestingly, Crg1 has a relevant number of links in the SCPnet, probably being part of the cooperative architecture discussed above. Thus, some SCP-hubs potentially neutralize deleterious compounds and the dense interactions between them indicate a cooperative resistance mechanism composed by serial or parallel biochemical reactions.
Ubiquitination and Phosphorylation Play Critical Roles in Chemical Stress Tolerance
The general lack of transcriptional alterations of MDRs by the chemicals to they confer resistance raises questions regarding the regulation of their protein products (Gasch et al., 2000; Venancio et al., 2010b; Berry et al., 2011). Integration of the chemogenomics with phosphorylation and ubiquitination data showed that MDRs are preferentially ubiquitinated and phosphorylated when compared to other genes in the network (Ptacek et al., 2005; Fiedler et al., 2009; Venancio et al., 2009). This analysis allowed the prediction of unexpected stress-related functions for a poorly characterized E1-enzyme (Ykl027w; Burroughs et al., 2009; Venancio et al., 2009), proteasomal chaperones (Poc1, Poc2, Poc3, Poc4; Le Tallec et al., 2007), the outer-membrane mitochondrial deubiquitinating enzyme Ubp16 (Kinner and Kolling, 2003), and the predicted desumoylating enzyme Wss1 (Iyer et al., 2004; Venancio et al., 2010b). Additional analysis suggest the Ub-system as a regulator of peroxisome recycling (i.e., pexophagy and de novo peroxisomal biogenesis), an organelle with paramount importance in chemical tolerance (Figure 2; Eckert and Johnsson, 2003; Smith and Aitchison, 2009; Venancio et al., 2009, 2010b).
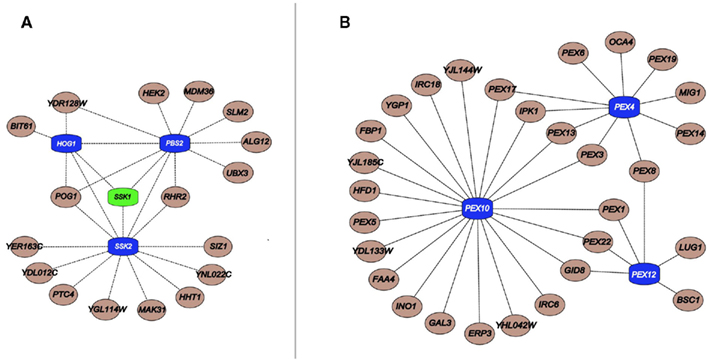
Figure 2. (A) Important components linked to HOG pathway kinases (blue) and its upstream regulator Ssk1 (green); (B) Critical peroxisomal component linked to the Ub pathway genes Pex4, Pex10, and Pex12 (blue). Interactions were obtained from a previous publication integrating chemogenomics data (Venancio et al., 2010b).
In a similar vein, with regards to phosphorylation, the SCPnet revealed that the high osmolarity glycerol (HOG) pathway (Hog1, Ssk2, and Pbs2) is the most important kinase cascade in chemical stress tolerance (Figure 2; Eckert and Johnsson, 2003; Smith and Aitchison, 2009; Venancio et al., 2010b). The HOG pathway is triggered by increased environmental osmolarity and raises glycerol concentration to control the intracellular osmotic pressure (Brewster et al., 1993). Although its association and the toleration of certain harsh conditions has been long demonstrated (e.g., oxidative stress; Pahlman et al., 2001), chemogenomic data vastly expands the role of glycerol as a general chemoprotectant (Venancio et al., 2010b). Chemogenomics data has also revealed the enigmatic haspin-like kinases Alk2 as a key player in chemical resistance. Orthologs of this kinase in plants and animals have been shown to mediate histone H3 phosphorylation (Wang et al., 2010). It would be interesting to investigate if Alk2-mediated phosphorylation of histone H3 or other chromatin proteins have some role in chemical stress tolerance. Finally, if interpreted in the context of recent advances in deciphering and annotating kinase-substrate pairs (Mok et al., 2011; Sharifpoor et al., 2011), chemogenomics datasets might uncover other novel biochemical pathways involved in stress resistance.
Integration of Chemogenomics Data, Protein–Protein Interactions, and Sequence Analysis
One interesting and frequently under-appreciated aspect of chemogenomics is its potential to suggest functions for unknown proteins, biochemical pathways, and protein complexes. This is especially important because many proteins involved in stress response are under positive selection and/or are lineage-specific, hindering homology-based function prediction. In line with this observation, Venancio and Aravind (2010) described a novel tail-anchored cysteine-rich transmembrane (TM) domain (CYSTM), present in some SCP-hubs (YDL012C and YDR210W) and conserved in fast-evolving stress resistance proteins from other eukaryotes. Structural analysis suggest that CYSTM proteins might regulate redox potential or access to metal ions by means of the conserved cysteines found in their TM segments (Venancio and Aravind, 2010).
Eukaryotic genomes are characterized by several closely related paralogs that are typically thought of as being functionally fungible. Just as chemogenomics data allowed an alternative perspective on gene essentiality, it is also promising to affect our understanding of the apparent redundancy among close paralogs. One notable example is provided by the closely related paralogous Rab GTPases Ypt31 and Ypt32, both of which interact with the myosin V motor to mediate polarized secretion (Lipatova et al., 2008). However, they show a striking dissimilarity in their chemogenomics profiles – Ypt31 is connected to 39 chemicals, while the Ypt32 is connected to only a single compound (Venancio et al., 2010b). This suggests that Ypt31 is probably dedicated for the polarized secretion in relation to chemical resistance. Likewise, one of the main MDRs emerging from major chemogenomics studies conducted to date (Hillenmeyer et al., 2008) is the EPS15-homology domain protein Irs4. Its close paralog Tax4 is not a MDR and show little relevance in chemical stress tolerance. Given the involvement of both paralogs in autophagy (Bugnicourt et al., 2008), Irs4 might represent a specialized stress-related component of the autophagic processes. Pairs of paralogous kinases such as Ssk22 and Ssk2 and Alk1 and Alk2 also display strongly discrepant chemogenomic profiles, suggesting a similar differentiation of arguably redundant paralogs for stress-related as opposed to other regulatory contexts (Venancio et al., 2010b).
Further, integration of protein–protein interaction data and curation of subcellular protein complexes (Pu et al., 2009) with chemogenomic profiles may as well provide clues on the roles of major complexes in stress tolerance (Venancio et al., 2010a). Such work shows that the primary subcellular complexes directly targeted by particular substances can be confidently identified in several instances. For example, RNA-degrading exosome emerged as the major target of 5-fluorouracil while the ribosomal subunits emerged as the primary target of neomycin (Lum et al., 2004; Parsons et al., 2004; Tor, 2006; Kammler et al., 2008; Venancio et al., 2010a). Surprisingly, most of the chemical–protein complex linkages appear to be the result of indirect functional interactions, indicating that particular subcellular complexes might have a role as general buffers against chemical stress. Such buffering systems appeared to be enriched in chromatin and vesicular trafficking (particularly vesicle tethering) complexes. Interestingly, some vesicular transport and chromatin complexes have been also shown to be involved in acquisition of both direct and cross-tolerance against stress (Alejandro-Osorio et al., 2009; Zakrzewska et al., 2011). Specifically, the histone deacetylase Rpd3 was found to have a critical role in the induction and repression of environmental stress response genes under multiple stress conditions (Alejandro-Osorio et al., 2009). Hence, the stabilization of particular transcriptional programs at the chromatin-level and consignment of deleterious chemicals to specific routes of the intracellular trafficking apparatus are likely to constitute general strategies to counter chemical stress. Transcriptional changes typically accumulate across the evolution of different species. Nevertheless, when contrasting transcriptional changes in response to stress across four yeast species, it has been show that a great part of the differences in individual genes are either compensated by changes in functionally related genes or reflect transitions between stress-inducible and constitutive activities – maintaining the phenotypic outcome in the different species (Tirosh et al., 2011).
Chemogenomics and the Discovery of Small-Molecules’ Mode-of-Action
In addition to the gene-centered studies presented above, chemogenomics data have also been extensively employed in the study of the mechanism-of-action (MoA) of various bioactive substances (Hughes et al., 2004; Roemer et al., 2011), under the premise that by clustering substances with similar chemical genetics profiles one can understand their MoA (Brown et al., 2006). Conceptually, by looking at the chemogenomics data as a bipartite network of genes and chemicals, it is just a matter of looking at the same data in the opposite direction (Figure 1).
Giaever et al. (2004) found that three therapeutically distinct substances that impair the growth of ERG24 heterozygous deletants share a common structural core, demonstrating the cells may have similar physiological responses against chemically related bioactive molecules. Lum et al. (2004) identified the SIN-1, the first metabolic derivative of the vasodilator molsidomine, as a potent inhibitor of the lanosterol synthase Erg7p, a finding of potential pharmacological interest. Lee et al. found that similar DNA-damaging substances can damage DNA not only in cognate ways but also by clearly distinct mechanisms. In addition, they have found 34 uncharacterized genes as novel DNA damage repair candidates. Hence, new genetic interactions between well-characterized DNA repair genes, indicating that the wiring of this system is still incomplete (Lee et al., 2005). By studying 75 compounds and 7 natural extracts, Parsons et al. found that compounds with similar chemical genetic profiles triggered analogous cellular effects (e.g., staurosporine and caspofungin, affecting cell-wall homeostasis). Through computational analysis and follow-up chemical characterization, they were able to identify the active component of crude extracts, emphasizing the pharmaceutical potential of chemogenomic screenings (Parsons et al., 2006). Taken together, such results clearly demonstrate the pharmacological potential of chemogenomics (Roemer et al., 2011). More recently, chemogenomic approaches were also used to elucidate the MoA of elesclomol and curcumin, showing that chemogenomic screens can be equally useful in studying therapeutics from either modern and traditional medicine (Minear et al., 2011; Blackman et al., 2012).
Conclusion and Future Perspectives
We herein review some recent advances in chemogenomics with a particular emphasis on how the integration of multiple datasets with other sources of biological information can help to discover previously unknown biochemical mechanisms, with potential applications in pharmacology and medicine. The results discussed here suggest that SCPnet interactions might be used to complement protein–protein and genetic interaction networks and could thus foster future analysis of double mutants using yeast synthetic genetic array (Tong et al., 2004) combined with exposure to specific bioactive molecules (Boucher et al., 2009; Roemer et al., 2011). Kapitzky et al. have found that the combination of data from S. cerevisiae and Schizosaccharomyces pombe improves MoA prediction accuracy. Further, they were able to identify a novel DNA-damaging substance with preserved MoA in human cells (Kapitzky et al., 2010). The extension of chemogenomics screens to pathogenic organisms such as Candida albicans (Xu et al., 2007; Oh et al., 2010) suggests that these approaches might come to bear on effective drug development. Taken together with our own observations, this indicates that chemogenomic screens may benefit from an evolutionary-oriented framework. Therefore, yeast chemogenomics serves as a benchmark and model for the development of similar screens for other organisms. Comparison of the major genes emerging from chemogenomics results across several species promises to be fruitful area for future studies.
Conflict of Interest Statement
The authors declare that the research was conducted in the absence of any commercial or financial relationships that could be construed as a potential conflict of interest.
Acknowledgments
The authors acknowledge the Intramural Research Program of the NIH (USA) for funding L. Aravind and FAPERJ (Brazil) for funding Thiago Motta Venancio and Daniel Bellieny-Rabelo.
References
Alejandro-Osorio, A. L., Huebert, D. J., Porcaro, D. T., Sonntag, M. E., Nillasithanukroh, S., Will, J. L., and Gasch, A. P. (2009). The histone deacetylase Rpd3p is required for transient changes in genomic expression in response to stress. Genome Biol. 10, R57.
Basehoar, A. D., Zanton, S. J., and Pugh, B. F. (2004). Identification and distinct regulation of yeast TATA box-containing genes. Cell 116, 699–709.
Berry, D. B., and Gasch, A. P. (2008). Stress-activated genomic expression changes serve a preparative role for impending stress in yeast. Mol. Biol. Cell 19, 4580–4587.
Berry, D. B., Guan, Q., Hose, J., Haroon, S., Gebbia, M., Heisler, L. E., Nislow, C., Giaever, G., and Gasch, A. P. (2011). Multiple means to the same end: the genetic basis of acquired stress resistance in yeast. PLoS Genet. 7, e1002353. doi:10.1371/journal.pgen.1002353
Birrell, G. W., Brown, J. A., Wu, H. I., Giaever, G., Chu, A. M., Davis, R. W., and Brown, J. M. (2002). Transcriptional response of Saccharomyces cerevisiae to DNA-damaging agents does not identify the genes that protect against these agents. Proc. Natl. Acad. Sci. U.S.A. 99, 8778–8783.
Blackman, R. K., Cheung-Ong, K., Gebbia, M., Proia, D. A., He, S., Kepros, J., Jonneaux, A., Marchetti, P., Kluza, J., Rao, P. E., Wada, Y., Giaever, G., and Nislow, C. (2012). Mitochondrial electron transport is the cellular target of the oncology drug elesclomol. PLoS ONE 7, e29798. doi:10.1371/journal.pone.0029798
Blake, W. J., Balazsi, G., Kohanski, M. A., Isaacs, F. J., Murphy, K. F., Kuang, Y., Cantor, C. R., Walt, D. R., and Collins, J. J. (2006). Phenotypic consequences of promoter-mediated transcriptional noise. Mol. Cell 24, 853–865.
Boucher, H. W., Talbot, G. H., Bradley, J. S., Edwards, J. E., Gilbert, D., Rice, L. B., Scheld, M., Spellberg, B., and Bartlett, J. (2009). Bad bugs, no drugs: no ESKAPE! An update from the Infectious Diseases Society of America. Clin. Infect. Dis. 48, 1–12.
Brewster, J. L., De Valoir, T., Dwyer, N. D., Winter, E., and Gustin, M. C. (1993). An osmosensing signal transduction pathway in yeast. Science 259, 1760–1763.
Brown, J. A., Sherlock, G., Myers, C. L., Burrows, N. M., Deng, C., Wu, H. I., Mccann, K. E., Troyanskaya, O. G., and Brown, J. M. (2006). Global analysis of gene function in yeast by quantitative phenotypic profiling. Mol. Syst. Biol. 2, 2006 0001.
Bugnicourt, A., Mari, M., Reggiori, F., Haguenauer-Tsapis, R., and Galan, J. M. (2008). Irs4p and Tax4p: two redundant EH domain proteins involved in autophagy. Traffic 9, 755–769.
Burroughs, A. M., Iyer, L. M., and Aravind, L. (2009). Natural history of the E1-like superfamily: implication for adenylation, sulfur transfer, and ubiquitin conjugation. Proteins 75, 895–910.
Eckert, J. H., and Johnsson, N. (2003). Pex10p links the ubiquitin conjugating enzyme Pex4p to the protein import machinery of the peroxisome. J. Cell Sci. 116, 3623–3634.
Ferea, T. L., Botstein, D., Brown, P. O., and Rosenzweig, R. F. (1999). Systematic changes in gene expression patterns following adaptive evolution in yeast. Proc. Natl. Acad. Sci. U.S.A. 96, 9721–9726.
Fiedler, D., Braberg, H., Mehta, M., Chechik, G., Cagney, G., Mukherjee, P., Silva, A. C., Shales, M., Collins, S. R., Van Wageningen, S., Kemmeren, P., Holstege, F. C., Weissman, J. S., Keogh, M. C., Koller, D., Shokat, K. M., and Krogan, N. J. (2009). Functional organization of the S. cerevisiae phosphorylation network. Cell 136, 952–963.
Gasch, A. P., Spellman, P. T., Kao, C. M., Carmel-Harel, O., Eisen, M. B., Storz, G., Botstein, D., and Brown, P. O. (2000). Genomic expression programs in the response of yeast cells to environmental changes. Mol. Biol. Cell 11, 4241–4257.
Giaever, G., Chu, A. M., Ni, L., Connelly, C., Riles, L., Veronneau, S., Dow, S., Lucau-Danila, A., Anderson, K., Andre, B., Arkin, A. P., Astromoff, A., El-Bakkoury, M., Bangham, R., Benito, R., Brachat, S., Campanaro, S., Curtiss, M., Davis, K., Deutschbauer, A., Entian, K. D., Flaherty, P., Foury, F., Garfinkel, D. J., Gerstein, M., Gotte, D., Guldener, U., Hegemann, J. H., Hempel, S., Herman, Z., Jaramillo, D. F., Kelly, D. E., Kelly, S. L., Kotter, P., Labonte, D., Lamb, D. C., Lan, N., Liang, H., Liao, H., Liu, L., Luo, C., Lussier, M., Mao, R., Menard, P., Ooi, S. L., Revuelta, J. L., Roberts, C. J., Rose, M., Ross-Macdonald, P., Scherens, B., Schimmack, G., Shafer, B., Shoemaker, D. D., Sookhai-Mahadeo, S., Storms, R. K., Strathern, J. N., Valle, G., Voet, M., Volckaert, G., Wang, C. Y., Ward, T. R., Wilhelmy, J., Winzeler, E. A., Yang, Y., Yen, G., Youngman, E., Yu, K., Bussey, H., Boeke, J. D., Snyder, M., Philippsen, P., Davis, R. W., and Johnston, M. (2002). Functional profiling of the Saccharomyces cerevisiae genome. Nature 418, 387–391.
Giaever, G., Flaherty, P., Kumm, J., Proctor, M., Nislow, C., Jaramillo, D. F., Chu, A. M., Jordan, M. I., Arkin, A. P., and Davis, R. W. (2004). Chemogenomic profiling: identifying the functional interactions of small molecules in yeast. Proc. Natl. Acad. Sci. U.S.A. 101, 793–798.
Gomez, S., Jensen, P., and Arenas, A. (2009). Analysis of community structure in networks of correlated data. Phys. Rev. E Stat. Nonlin. Soft Matter Phys. 80, 016114.
Hillenmeyer, M. E., Fung, E., Wildenhain, J., Pierce, S. E., Hoon, S., Lee, W., Proctor, M., St Onge, R. P., Tyers, M., Koller, D., Altman, R. B., Davis, R. W., Nislow, C., and Giaever, G. (2008). The chemical genomic portrait of yeast: uncovering a phenotype for all genes. Science 320, 362–365.
Ho, C. H., Piotrowski, J., Dixon, S. J., Baryshnikova, A., Costanzo, M., and Boone, C. (2011). Combining functional genomics and chemical biology to identify targets of bioactive compounds. Curr. Opin. Chem. Biol. 15, 66–78.
Hoon, S., Smith, A. M., Wallace, I. M., Suresh, S., Miranda, M., Fung, E., Proctor, M., Shokat, K. M., Zhang, C., Davis, R. W., Giaever, G., St Onge, R. P., and Nislow, C. (2008). An integrated platform of genomic assays reveals small-molecule bioactivities. Nat. Chem. Biol. 4, 498–506.
Hughes, T., Andrews, B., and Boone, C. (2004). Old drugs, new tricks: using genetically sensitized yeast to reveal drug targets. Cell 116, 5–7.
Huisinga, K. L., and Pugh, B. F. (2004). A genome-wide housekeeping role for TFIID and a highly regulated stress-related role for SAGA in Saccharomyces cerevisiae. Mol. Cell 13, 573–585.
Iyer, L. M., Koonin, E. V., and Aravind, L. (2004). Novel predicted peptidases with a potential role in the ubiquitin signaling pathway. Cell Cycle 3, 1440–1450.
Jo, W. J., Loguinov, A., Chang, M., Wintz, H., Nislow, C., Arkin, A. P., Giaever, G., and Vulpe, C. D. (2008). Identification of genes involved in the toxic response of Saccharomyces cerevisiae against iron and copper overload by parallel analysis of deletion mutants. Toxicol. Sci. 101, 140–151.
Kammler, S., Lykke-Andersen, S., and Jensen, T. H. (2008). The RNA exosome component hRrp6 is a target for 5-fluorouracil in human cells. Mol. Cancer Res. 6, 990–995.
Kapitzky, L., Beltrao, P., Berens, T. J., Gassner, N., Zhou, C., Wuster, A., Wu, J., Babu, M. M., Elledge, S. J., Toczyski, D., Lokey, R. S., and Krogan, N. J. (2010). Cross-species chemogenomic profiling reveals evolutionarily conserved drug mode of action. Mol. Syst. Biol. 6, 451.
Kinner, A., and Kolling, R. (2003). The yeast deubiquitinating enzyme Ubp16 is anchored to the outer mitochondrial membrane. FEBS Lett. 549, 135–140.
Le Tallec, B., Barrault, M. B., Courbeyrette, R., Guerois, R., Marsolier-Kergoat, M. C., and Peyroche, A. (2007). 20S proteasome assembly is orchestrated by two distinct pairs of chaperones in yeast and in mammals. Mol. Cell 27, 660–674.
Lee, W., St Onge, R. P., Proctor, M., Flaherty, P., Jordan, M. I., Arkin, A. P., Davis, R. W., Nislow, C., and Giaever, G. (2005). Genome-wide requirements for resistance to functionally distinct DNA-damaging agents. PLoS Genet. 1, e24. doi:10.1371/journal.pgen.0010024
Levy, S. F., and Siegal, M. L. (2008). Network hubs buffer environmental variation in Saccharomyces cerevisiae. PLoS Biol. 6, e264. doi:10.1371/journal.pbio.0060264
Lipatova, Z., Tokarev, A. A., Jin, Y., Mulholland, J., Weisman, L. S., and Segev, N. (2008). Direct interaction between a myosin V motor and the Rab GTPases Ypt31/32 is required for polarized secretion. Mol. Biol. Cell 19, 4177–4187.
Lissina, E., Young, B., Urbanus, M. L., Guan, X. L., Lowenson, J., Hoon, S., Baryshnikova, A., Riezman, I., Michaut, M., Riezman, H., Cowen, L. E., Wenk, M. R., Clarke, S. G., Giaever, G., and Nislow, C. (2011). A systems biology approach reveals the role of a novel methyltransferase in response to chemical stress and lipid homeostasis. PLoS Genet. 7, e1002332. doi:10.1371/journal.pgen.1002332
Lum, P. Y., Armour, C. D., Stepaniants, S. B., Cavet, G., Wolf, M. K., Butler, J. S., Hinshaw, J. C., Garnier, P., Prestwich, G. D., Leonardson, A., Garrett-Engele, P., Rush, C. M., Bard, M., Schimmack, G., Phillips, J. W., Roberts, C. J., and Shoemaker, D. D. (2004). Discovering modes of action for therapeutic compounds using a genome-wide screen of yeast heterozygotes. Cell 116, 121–137.
Minear, S., O’Donnell, A. F., Ballew, A., Giaever, G., Nislow, C., Stearns, T., and Cyert, M. S. (2011). Curcumin inhibits growth of Saccharomyces cerevisiae through iron chelation. Eukaryotic Cell 10, 1574–1581.
Mitchell, A., Romano, G. H., Groisman, B., Yona, A., Dekel, E., Kupiec, M., Dahan, O., and Pilpel, Y. (2009). Adaptive prediction of environmental changes by microorganisms. Nature 460, 220–224.
Mok, J., Zhu, X., and Snyder, M. (2011). Dissecting phosphorylation networks: lessons learned from yeast. Expert Rev. Proteomics 8, 775–786.
Newman, M. E. (2003). Mixing patterns in networks. Phys. Rev. E Stat. Nonlin. Soft Matter Phys. 67, 026126.
Oh, J., Fung, E., Schlecht, U., Davis, R. W., Giaever, G., St Onge, R. P., Deutschbauer, A., and Nislow, C. (2010). Gene annotation and drug target discovery in Candida albicans with a tagged transposon mutant collection. PLoS Pathog. 6, e1001140. doi:10.1371/journal.ppat.1001140
Pahlman, A. K., Granath, K., Ansell, R., Hohmann, S., and Adler, L. (2001). The yeast glycerol 3-phosphatases Gpp1p and Gpp2p are required for glycerol biosynthesis and differentially involved in the cellular responses to osmotic, anaerobic, and oxidative stress. J. Biol. Chem. 276, 3555–3563.
Parsons, A. B., Brost, R. L., Ding, H., Li, Z., Zhang, C., Sheikh, B., Brown, G. W., Kane, P. M., Hughes, T. R., and Boone, C. (2004). Integration of chemical-genetic and genetic interaction data links bioactive compounds to cellular target pathways. Nat. Biotechnol. 22, 62–69.
Parsons, A. B., Lopez, A., Givoni, I. E., Williams, D. E., Gray, C. A., Porter, J., Chua, G., Sopko, R., Brost, R. L., Ho, C. H., Wang, J., Ketela, T., Brenner, C., Brill, J. A., Fernandez, G. E., Lorenz, T. C., Payne, G. S., Ishihara, S., Ohya, Y., Andrews, B., Hughes, T. R., Frey, B. J., Graham, T. R., Andersen, R. J., and Boone, C. (2006). Exploring the mode-of-action of bioactive compounds by chemical-genetic profiling in yeast. Cell 126, 611–625.
Pena-Castillo, L., and Hughes, T. R. (2007). Why are there still over 1000 uncharacterized yeast genes? Genetics 176, 7–14.
Ptacek, J., Devgan, G., Michaud, G., Zhu, H., Zhu, X., Fasolo, J., Guo, H., Jona, G., Breitkreutz, A., Sopko, R., Mccartney, R. R., Schmidt, M. C., Rachidi, N., Lee, S. J., Mah, A. S., Meng, L., Stark, M. J., Stern, D. F., De Virgilio, C., Tyers, M., Andrews, B., Gerstein, M., Schweitzer, B., Predki, P. F., and Snyder, M. (2005). Global analysis of protein phosphorylation in yeast. Nature 438, 679–684.
Pu, S., Wong, J., Turner, B., Cho, E., and Wodak, S. J. (2009). Up-to-date catalogues of yeast protein complexes. Nucleic Acids Res. 37, 825–831.
Rine, J., Hansen, W., Hardeman, E., and Davis, R. W. (1983). Targeted selection of recombinant clones through gene dosage effects. Proc. Natl. Acad. Sci. U.S.A. 80, 6750–6754.
Roemer, T., Davies, J., Giaever, G., and Nislow, C. (2011). Bugs, drugs and chemical genomics. Nat. Chem. Biol. 8, 46–56.
Ruotolo, R., Marchini, G., and Ottonello, S. (2008). Membrane transporters and protein traffic networks differentially affecting metal tolerance: a genomic phenotyping study in yeast. Genome Biol. 9, R67.
Sharifpoor, S., Nguyen Ba, A. N., Young, J. Y., Van Dyk, D., Friesen, H., Douglas, A. C., Kurat, C. F., Chong, Y. T., Founk, K., Moses, A. M., and Andrews, B. J. (2011). A quantitative literature-curated gold standard for kinase-substrate pairs. Genome Biol. 12, R39.
Sharom, J. R., Bellows, D. S., and Tyers, M. (2004). From large networks to small molecules. Curr. Opin. Chem. Biol. 8, 81–90.
Shoemaker, D. D., Lashkari, D. A., Morris, D., Mittmann, M., and Davis, R. W. (1996). Quantitative phenotypic analysis of yeast deletion mutants using a highly parallel molecular bar-coding strategy. Nat. Genet. 14, 450–456.
Smith, A. M., Heisler, L. E., Mellor, J., Kaper, F., Thompson, M. J., Chee, M., Roth, F. P., Giaever, G., and Nislow, C. (2009). Quantitative phenotyping via deep barcode sequencing. Genome Res. 19, 1836–1842.
Smith, J. J., and Aitchison, J. D. (2009). Regulation of peroxisome dynamics. Curr. Opin. Cell Biol. 21, 119–126.
St Onge, R. P., Mani, R., Oh, J., Proctor, M., Fung, E., Davis, R. W., Nislow, C., Roth, F. P., and Giaever, G. (2007). Systematic pathway analysis using high-resolution fitness profiling of combinatorial gene deletions. Nat. Genet. 39, 199–206.
Suter, B., Auerbach, D., and Stagljar, I. (2006). Yeast-based functional genomics and proteomics technologies: the first 15 years and beyond. BioTechniques 40, 625–644.
Tagkopoulos, I., Liu, Y. C., and Tavazoie, S. (2008). Predictive behavior within microbial genetic networks. Science 320, 1313–1317.
Tai, S. L., Snoek, I., Luttik, M. A., Almering, M. J., Walsh, M. C., Pronk, J. T., and Daran, J. M. (2007). Correlation between transcript profiles and fitness of deletion mutants in anaerobic chemostat cultures of Saccharomyces cerevisiae. Microbiology 153, 877–886.
Tirosh, I., Weinberger, A., Carmi, M., and Barkai, N. (2006). A genetic signature of interspecies variations in gene expression. Nat. Genet. 38, 830–834.
Tirosh, I., Wong, K. H., Barkai, N., and Struhl, K. (2011). Extensive divergence of yeast stress responses through transitions between induced and constitutive activation. Proc. Natl. Acad. Sci. U.S.A. 108, 16693–16698.
Tong, A. H., Lesage, G., Bader, G. D., Ding, H., Xu, H., Xin, X., Young, J., Berriz, G. F., Brost, R. L., Chang, M., Chen, Y., Cheng, X., Chua, G., Friesen, H., Goldberg, D. S., Haynes, J., Humphries, C., He, G., Hussein, S., Ke, L., Krogan, N., Li, Z., Levinson, J. N., Lu, H., Menard, P., Munyana, C., Parsons, A. B., Ryan, O., Tonikian, R., Roberts, T., Sdicu, A. M., Shapiro, J., Sheikh, B., Suter, B., Wong, S. L., Zhang, L. V., Zhu, H., Burd, C. G., Munro, S., Sander, C., Rine, J., Greenblatt, J., Peter, M., Bretscher, A., Bell, G., Roth, F. P., Brown, G. W., Andrews, B., Bussey, H., and Boone, C. (2004). Global mapping of the yeast genetic interaction network. Science 303, 808–813.
Tor, Y. (2006). The ribosomal A-site as an inspiration for the design of RNA binders. Biochimie 88, 1045–1051.
Venancio, T. M., and Aravind, L. (2010). CYSTM, a novel cysteine-rich transmembrane module with a role in stress tolerance across eukaryotes. Bioinformatics 26, 149–152.
Venancio, T. M., Balaji, S., and Aravind, L. (2010a). High-confidence mapping of chemical compounds and protein complexes reveals novel aspects of chemical stress response in yeast. Mol. Biosyst. 6, 175–181.
Venancio, T. M., Balaji, S., Geetha, S., and Aravind, L. (2010b). Robustness and evolvability in natural chemical resistance: identification of novel systems properties, biochemical mechanisms and regulatory interactions. Mol. Biosyst. 6, 1475–1491.
Venancio, T. M., Balaji, S., Iyer, L. M., and Aravind, L. (2009). Reconstructing the ubiquitin network: cross-talk with other systems and identification of novel functions. Genome Biol. 10, R33.
Wang, F., Dai, J., Daum, J. R., Niedzialkowska, E., Banerjee, B., Stukenberg, P. T., Gorbsky, G. J., and Higgins, J. M. (2010). Histone H3 Thr-3 phosphorylation by Haspin positions Aurora B at centromeres in mitosis. Science 330, 231–235.
Winzeler, E. A., Shoemaker, D. D., Astromoff, A., Liang, H., Anderson, K., Andre, B., Bangham, R., Benito, R., Boeke, J. D., Bussey, H., Chu, A. M., Connelly, C., Davis, K., Dietrich, F., Dow, S. W., El Bakkoury, M., Foury, F., Friend, S. H., Gentalen, E., Giaever, G., Hegemann, J. H., Jones, T., Laub, M., Liao, H., Liebundguth, N., Lockhart, D. J., Lucau-Danila, A., Lussier, M., M’Rabet, N., Menard, P., Mittmann, M., Pai, C., Rebischung, C., Revuelta, J. L., Riles, L., Roberts, C. J., Ross-Macdonald, P., Scherens, B., Snyder, M., Sookhai-Mahadeo, S., Storms, R. K., Veronneau, S., Voet, M., Volckaert, G., Ward, T. R., Wysocki, R., Yen, G. S., Yu, K., Zimmermann, K., Philippsen, P., Johnston, M., and Davis, R. W. (1999). Functional characterization of the S. cerevisiae genome by gene deletion and parallel analysis. Science 285, 901–906.
Wuster, A., and Madan Babu, M. (2008). Chemogenomics and biotechnology. Trends Biotechnol. 26, 252–258.
Xu, D., Jiang, B., Ketela, T., Lemieux, S., Veillette, K., Martel, N., Davison, J., Sillaots, S., Trosok, S., Bachewich, C., Bussey, H., Youngman, P., and Roemer, T. (2007). Genome-wide fitness test and mechanism-of-action studies of inhibitory compounds in Candida albicans. PLoS Pathog. 3, e92. doi:10.1371/journal.ppat.0030092
Zakrzewska, A., Van Eikenhorst, G., Burggraaff, J. E., Vis, D. J., Hoefsloot, H., Delneri, D., Oliver, S. G., Brul, S., and Smits, G. J. (2011). Genome-wide analysis of yeast stress survival and tolerance acquisition to analyze the central trade-off between growth rate and cellular robustness. Mol. Biol. Cell 22, 4435–4446.
Keywords: chemogenomics, yeast, chemical genetics, evolution, multi drug resistance, biochemistry, ubiquitin, phosphorylation
Citation: Venancio TM, Bellieny-Rabelo D and Aravind L (2012) Evolutionary and biochemical aspects of chemical stress resistance in Saccharomyces cerevisiae. Front. Gene. 3:47. doi: 10.3389/fgene.2012.00047
Received: 12 January 2012; Accepted: 15 March 2012;
Published online: 30 March 2012.
Edited by:
Norman Pavelka, Agency for Science, Technology and Research (A*STAR), SingaporeReviewed by:
Roger Guimera, Institució Catalana de Recerca i Estudis Avançats–Universitat Rovira i Virgili, SpainHyungwon Choi, National University of Singapore, Singapore
Copyright: © 2012 Venancio, Bellieny-Rabelo and Aravind. This is an open-access article distributed under the terms of the Creative Commons Attribution Non Commercial License, which permits non-commercial use, distribution, and reproduction in other forums, provided the original authors and source are credited.
*Correspondence: Thiago Motta Venancio, Laboratório de Química e Função de Proteínas e Peptídeos, Centro de Biocigências e Biotecnologia, Universidade Estadual do Norte Fluminense Darcy Ribeiro, Av. Alberto Lamego 2000, Campos dos Goytacazes, Rio de Janeiro, Brazil. e-mail: thiago.venancio@gmail.com