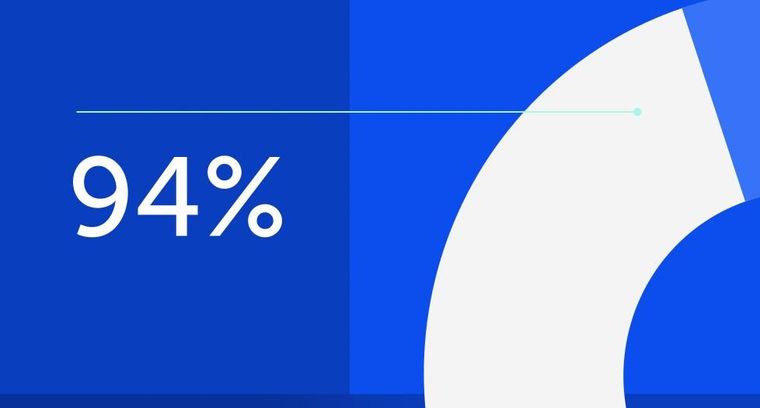
94% of researchers rate our articles as excellent or good
Learn more about the work of our research integrity team to safeguard the quality of each article we publish.
Find out more
ORIGINAL RESEARCH article
Front. Future Transp., 12 March 2025
Sec. Transport Safety
Volume 6 - 2025 | https://doi.org/10.3389/ffutr.2025.1545411
When we travel from one place to another, the first priority during our journey is that, we all wish to reach safely at our destination. Ensuring driver wakefulness is crucial for road safety, as drowsiness is a leading cause of fatal accidents, resulting in physical injuries, financial losses, and loss of life. This paper proposes an anti-sleep driver detection algorithm designed specifically for four-wheelers and larger vehicles to mitigate accidents caused by driver drowsiness. The proposed algorithm leverages deep learning (DL) models, including InceptionV3, VGG16, and MobileNetV2, for real-time detection and classification of driver drowsiness. The models were trained and evaluated using comprehensive performance metrics, such as accuracy, precision, recall, F1 score, and confusion matrix. The proposed method outperforms the traditional approaches such as Support Vector Machines (SVM), K-Nearest Neighbors (KNN), Haar Cascade Classifiers, and other DL architectures like Xception and VGG16, in terms of accuracy and efficiency. Among the tested models, InceptionV3 demonstrated superior performance, achieving an accuracy of 99.18%, a validation loss of 0.85%, and execution time of 0.2 s on Raspberry Pi platform. The results suggest that the proposed algorithm provides a robust and effective solution for real-time driver drowsiness detection thereby contributing towards enhanced safety.
Drowsiness while driving is a condition in which the driver fells sleepy that can lead to serious accidents (Ministry of Road Transport and Highways, Government of India, 2022) and loss of life. Inattentiveness caused by drowsiness has been recognized as a major contributor to traffic collisions and highway fatalities. According to a study by the Central Road Research Institute (CRRI), a premier research institute in India focused on road and transportation engineering, conducting studies to improve road safety, traffic management, and infrastructure development, published in The Financial Express (Dated 15 July 2022), “40% of highway accidents occur due to drivers dozing off. Exhausted drivers who doze off at the wheel are responsible for road accidents” (Suresh et al., 2023). Despite safety campaigns and regulations, drowsy driving continues to be a threat to road safety. However, technology-based solutions like drowsiness detection systems can help mitigate these risks by providing real-time monitoring of a driver’s alertness and issuing timely warnings when signs of fatigue are detected. This can be particularly useful in preventing accidents caused by fatigue-related lapses in concentration (Mahajan and Velaga, 2023; Peng et al., 2024). Optimization techniques, such as integrating invasive weed optimization with differential evolutionary models, have demonstrated significant improvements in training neural networks, which can further enhance system performance and robustness (Movassagh et al., 2023).
Traditional methods for detecting drowsiness, such as electroencephalogram (EEG) monitoring (Venkata Phanikrishna et al., 2023; LaRocco et al., 2020), which measure electrical activity in the brain and provide accurate insights into drowsiness detection, are known for their accuracy however, they are invasive and impractical for everyday and continuous use while driving. “Prior approaches for detecting drowsiness utilized various machine learning (ML) algorithms like Support Vector Machines (SVM), K-Nearest Neighbors (KNN), and Haar Cascade classifiers (Ramzan et al., 2019; Abtahi et al., 2014). Recent advancements in collaborative adversarial networks have shown their potential in improving the robustness of machine learning models, which could be further explored to address the shortcomings of traditional approaches (Alzubi et al., 2020). Additionally, deep learning (DL) methods such as InceptionV3, Xception, VGG16, and a custom EfficientNet model, which shows validation accuracy of 91.81%, 93.6%, 78.6%, and 95%, respectively, in Suresh et al. (2023) and Yashaswini et al. (2024), among others.” Also in drowsiness detection, InceptionV3 and VGG16 models show validation accuracy of 92.3% and 81.7% respectively in Sun et al. (2023). These systems had their limitations, however DL algorithms generally performed better than the traditional ML techniques in image classification tasks and were more capable of handling complex problems. DL algorithms excel in driver drowsiness classification due to their ability to automatically learn relevant features and hierarchical representations from raw data, such as facial expressions and eye movements, without needing handcrafted features. These models, particularly Convolutional Neural Network (CNN) can capture complex patterns in the data, leading to better performance in handling variability and noise compared to traditional ML algorithms. However, the mentioned DL models were less accurate compared to the newly proposed DL model (Suresh et al., 2023). The aim of this proposed work is to develop effective DL algorithms to address the shortcomings of earlier methods and offer a simple, user-friendly solution for early-stage drowsiness detection that can be used on desktops or mobile devices.
The proposed system relies on image processing (Yan et al., 2016) techniques, offering a non-invasive approach to drowsiness detection. The system uses a Universal Serial Bus (USB) camera, a widely-used interface for connecting peripherals, to capture images of the driver’s face, focusing on eye detection to determine whether the eyes are open or closed. By analyzing these images, the system can assess the driver’s state of alertness and generate appropriate alerts to prevent accidents. We have used the MRL (Media Research Lab) Eye Dataset, which contains data of 37 different persons (33 men and 4 women) and consists of 84,898 images of open and closed eyes, publicly available at (Fusek, 2018).
The detection process involves several key steps (Fu et al., 2024), starting with face detection to locate the driver’s head in the image (Sathya and Sudha, 2024). Once the face is identified, the system zeroes in on the eyes to evaluate their status. Closed eyes over a certain duration indicate drowsiness (Kamran et al., 2019), triggering an alert in the form of an audible alarm or even shutting down the vehicle’s engine in extreme cases. This approach is designed to work in various lighting conditions and with different types of drivers, offering a practical solution to reduce drowsy driving incidents.
The proposed system is suitable for four-wheel vehicles and can be installed in a variety of transportation settings, making it a versatile tool for improving road safety. Moreover, the DL based architecture used in this system ensures a high level of accuracy in detecting drowsiness, with models such as InceptionV3, VGG16, and MobileNetV2 being assessed for their performance. The InceptionV3 model achieved an overall accuracy of 99.18% and a validation loss of 0.85% in classifying drowsiness, making it a reliable choice for this application.
Overall, the system not only enhances safety but also has the potential to save lives by reducing the number of accidents caused by drowsiness. With its non-invasive design and high accuracy, it provides a promising approach to addressing one of the leading causes of road accidents.
The paper is organized as follows. Section 2 describes the applied methodology for the proposed real-time anti-sleep alert algorithm, detailing the CNN architectures and computer vision techniques employed. Section 3 elaborates on the dataset selection and model training process, emphasizing the integration of transfer learning with pre-trained models like InceptionV3, VGG16, and MobileNetV2. Section 4 presents a comprehensive performance evaluation, comparing the proposed system against existing methods in terms of accuracy, precision, recall, and F1-score. Section 5 introduces the prototype implementation, including the hardware and software components, followed by testing scenarios in real-world conditions. Lastly, Section 6 concludes the paper by emphasizing the proposed system’s potential in enhancing road safety through efficient and reliable drowsiness detection.
The proposed algorithm leverages CNN (Taye, 2023) and computer vision techniques to detect drowsiness. CNN architectures usually comprise of four main types of layers: convolutional, pooling, activation, and fully connected. Figure 1 depicts a CNN architecture used for classifying eye states (open or closed) to determine drowsiness, illustrating the stages of feature learning through convolution and pooling layers, followed by classification using fully connected and softmax layers. CNN-based image classification algorithms (Chen et al., 2021) have gained immense popularity due to their ability to learn and extract intricate features (Liu et al., 2022) from raw image data automatically. Recent advancements, such as compressed energy-efficient CNNs, have proven effective in distracted driver detection, highlighting the need for lightweight and efficient models for real-time applications (Alzubi et al., 2022). Building upon this, we have utilized different CNN models in this work, including InceptionV3, VGG16, and MobileNetV2. OpenCV offers various face detection methods (Kumar et al., 2019), each differing in terms of accuracy and speed. To implement this in real-time, we need to use the most accurate method for detecting the driver’s drowsiness state. In addition to detecting drowsiness, computer vision has various other applications, including facial recognition, vehicle detection (Deshmukh et al., 2024) and aiding law enforcement agencies in tracking and identifying criminals. The Algorithm 1 describes detection of eye status using DL model.
The InceptionV3 model has 23.8M parameters and 159 layers. This model employs the Inception module (Szegedy et al., 2016), a concept developed by researchers at Google, which uses a combination of convolutions with varying filter sizes, allowing the network to simultaneously consider different levels of detail in the same layer. InceptionV3 features mixed layers where these parallel convolutions occur, and it includes multiple blocks, each with its own sequence of convolutions, batch normalization, and pooling operations.
The data in InceptionV3 first undergoes a few initial convolutional layers before entering the main “mixed” layers containing the distinctive Inception modules. These mixed layers help the network efficiently manage parameters while maintaining a high degree of versatility in feature extraction. Batch normalization is used extensively throughout the model to improve training stability.
For drowsiness detection, the final few layers of the pre-trained InceptionV3 model are replaced with custom-designed layers specific to this task. The adjusted final layer produces two categories: sleepy and not sleepy. This customization allows the pre-trained network to be fine-tuned for the specific problem of drowsiness detection. Figure 2 depicts the architectural diagram of InceptionV3.
The VGG16 (Tao et al., 2021) model has 138 million parameters and 16 layers which is developed by Simonyan and Zisserman. It is known for its straightforward deep CNN design, where the emphasis is on using multiple stacked layers of small 3 × 3 convolutions. This architectural approach was developed by researchers at the Visual Geometry Group (VGG) (Visual Geometry Group, 2024) at the University of Oxford.
Unlike other convolutional architectures that use complex operations or various convolution sizes within a single module, VGG16 exclusively employs uniform 3 × 3 convolutions followed by 2 × 2 max-pooling layers. This consistent structure leads to a deep network with a relatively simple design. The layers are organized into distinct blocks with increasing depth, starting with smaller numbers of filters and gradually increasing as the data moves through the network.
Batch normalization is not part of the original VGG16 architecture, unlike other models like Xception. Each convolutional block ends with a max-pooling layer, and the final fully connected layers are trained for specific tasks like image classification. The model typically ends with a softmax output layer that indicates class probabilities.
When adapting the VGG16 model for a specific task like drowsiness detection, the final layers can be replaced with task-specific ones. For instance, replacing the fully connected layers with a custom-designed structure, like a smaller dense layer and an output layer with two classes, sleepy and not sleepy. This restructured final section allows the model to specialize in detecting drowsiness while leveraging the robust feature extraction from the earlier layers. Figure 3 depicts the architectural diagram of VGG16.
The MobileNetV2 model has 3.4M parameters and 53 layers. Inverted Residuals and Linear Bottlenecks are used in the deep CNN design of MobileNetV2 (Dong et al., 2020) developed by researchers from Google Inc. According to Google Inc., the Inverted Residual architecture (Sandler et al., 2018) allows the network to maintain high efficiency and performance with low computational complexity. It achieves this by utilizing residual blocks with depthwise separable convolutions (Lu et al., 2021) and linear bottlenecks, providing a balance between compactness and effectiveness. The MobileNetV2 model consists of an initial convolution layer followed by a bottleneck layer, then several inverted residual blocks with expansion and depthwise separable convolution, ultimately leading to a fully connected output layer. Batch normalization (Thakkar et al., 2018) is applied after each convolution and inverted residual block to maintain stable training. The final layers of the pre-trained MobileNetV2 model are replaced by a custom-designed set of layers for various specific tasks. For detecting drowsiness, the output of the final layer is defined with two classes: 0 indicates “sleepy” and 1 indicates “not sleepy.” Figure 4 depicts the architectural diagram of MobileNetV2.
Here, facial landmarks are used as a method to detect the specific facial features such as the eyes, nose, mouth, and jawline. It involves identifying a set of key points on the face, such as the corners of the eyes, the tip of the nose, and the edges of the mouth. These key points are then used to determine the position of the facial features with respect to each other. This can be done by using a ML algorithm to detect the facial landmarks (Dewi et al., 2022) in an image. Once the landmarks are detected, they can be used to perform further calculations and analysis on the image. The output of this process is a set of coordinates that represent the position of each facial landmark on the face, which can be used for further processing, as shown in Figure 5.
First, the dataset (Fusek, 2018) has been prepared and categorized for training the CNN model. The CNN models for image classification, using pre-trained bases like MobileNetV2, InceptionV3 and VGG16, all configured for max pooling. These models include Batch Normalization, and a dense layer with L1/L2 (Least Absolute Deviations/Least Squares) regularization, and ReLU (Rectified Linear Unit) activation. Dropout is applied at 45%, followed by a dense output layer with Softmax for multi-class classification. Adamax serves as the optimizer, while categorical cross-entropy is used as the loss function, with accuracy as a key metric.
For the training process, the dataset is prepared and categorized for the CNN models’ training, using transfer learning to adapt MobileNetV2, InceptionV3 and VGG16 to specific needs. This entails modifying the pre-trained models and using targeted datasets to train them for the desired output. The training of the models evaluates precision, recall, F1-Score, loss, Confusion matrix, and accuracy. The same MRL Eye Dataset is utilized across all models for consistency. To compare efficiency and performance, these models undergo training and evaluation. The training is conducted over 50 epochs on the same hardware configuration, ensuring consistency in computational resources.
The MRL Eye Dataset (Fusek, 2018) is an extensive collection of human eye images designed to support various DL and computer vision tasks, which consisted of 84,898 images of open and closed eye. At this moment, the dataset contains the images captured by three different sensors (Intel RealSense RS 300 sensor with 640 × 480 resolution, Imaging Development Systems (IDS) imaging sensor with 1,280 × 1,024 resolution, and Aptina sensor with 752 × 480 resolution. Thirty seven individuals, some wearing glasses for the left or right eye and some without, contributed samples for this study. This dataset includes infrared images in both low and high resolutions, gathered under diverse lighting conditions and with a range of capturing devices. It is a valuable resource for testing multiple features or training classifiers, providing a comprehensive set of data for experimentation and analysis. The proportion of the training dataset to the test dataset was 80 to 20. This allocation provides a substantial amount of data for model training while retaining a sufficient portion for performance evaluation. A few sample photos from the MRL Eye dataset are displayed in Figure 6.
The model underwent training for 50 epochs on the dataset, which required approximately 3.5 h using a batch size of 16.
The model underwent training for 50 epochs on the dataset, which required approximately 6 h using a batch size of 16.
The model underwent training for 50 epochs on the dataset, which required approximately 7 h using a batch size of 16.
The evaluation of the model’s performance involves common metrics (Van Thieu, 2024) such as classification accuracy, precision, recall, and F1-score, which are derived from a confusion matrix. This matrix contains data on true positives (TP), true negatives (TN), false positives (FP), and false negatives (FN). Table 1 presents the confusion matrix for binary class classification. The performance metrics for each class within this matrix are calculated using Equations 1–4.
Accuracy represents the proportion of correct predictions, indicating the classifier’s overall accuracy. Precision measures the accuracy of the model when it predicts a positive class, giving an indication of false positives. Recall reflects the model’s ability to detect true positive cases, providing information on false negatives.
Validation accuracy quantifies a ML model’s ability to accurately predict labels on a dataset it hasn’t been trained with. This metric is commonly used during model training to gauge performance and to avoid overfitting. To avoid this we have used L1/L2 regularization techniques. Validation loss measures the disparity between the forecasted output of a ML model and the real output, considering a distinct dataset that wasn’t part of the training process. It serves as an indicator of the model’s ability to generalize to fresh, unfamiliar data.
Training accuracy refers to the extent to which a ML model accurately categorizes the data it was trained with. It represents the proportion of samples in the training dataset that are accurately categorized by the model during its learning phase. Training loss quantifies how effectively a ML model reduces the gap between the forecasted result versus the real outcome from the training dataset. It represents the error or the difference between the predicted and true results for a given set of training data. The numpy and matplotlib library in Python are involved in generating, evaluating, and visualizing the outcomes achieved during the training and validation phases of the model. Graphs illustrate the accuracy and loss of different models across varying epochs of training and validation. The classification report, which includes metrics like precision, recall, and F1-score, is provided for each model, along with their confusion matrix.
The loss and accuracy curves for VGG16 over epochs are shown in Figure 7. The training and validation loss of the VGG16 model is displayed on the left side of Figure 8, while its accuracy is displayed on the right side. Furthermore, the VGG16 model’s confusion matrix is shown in Figure 8. This model has a validation loss of 11.12% and a peak validation accuracy of 98.80%.
The loss and accuracy curves for InceptionV3 over epochs are shown in Figure 10. The training and validation loss of the InceptionV3 model is displayed on the left side of Figure 9, while its accuracy is displayed on the right side. Furthermore, the InceptionV3 model’s confusion matrix is shown in Figure 10. This model has a validation loss of 5.85% and a peak validation accuracy of 99.18%.
The loss and accuracy curves for MobileNetV2 over epochs are shown in Figure 12. The training and validation loss of the MobileNetV2 model is displayed on the left side of Figure 11, while its accuracy is displayed on the right side. Furthermore, the MobileNetV2 model’s confusion matrix is shown in Figure 12. This model has a validation loss of 8.21% and a peak validation accuracy of 99.01%.
Table 2 displays the training accuracy and loss, as well as validation accuracy and loss, for three models: InceptionV3, VGG16, and MobileNetV2. Likewise, Table 3 provides a comparison of precision, recall, and F1-Score among the various models. The comparison reveals that the InceptionV3 model demonstrates the most superior performance, boasting a validation accuracy of 99.18% and a validation loss of 0.85%. Consequently, the InceptionV3 model is chosen for the deployment of the Real-Time Anti-Sleep Alert Algorithm in practical scenarios.
Table 3. Comparision of precision, recall and f1-score for closed and open eyes of different models on dataset.
To detect the sleepiness of the driver, an model was trained using multiple faces in different conditions. If the Eye Aspect Ratio (EAR) (Dewi et al., 2022) was found to be less than 0.3, the driver can be classified as drowsy. In total, 20 consecutive frames should be considered for calculation, which is crucial in the real-time application of the system. Illustrations of real-time detection of eyes in normal and drowsy situations are depicted in Figures 13, 14 respectively.
Figure 13. Real time detection of eyes in normal situations, where the EAR remains above the threshold value.
Figure 14. Real time detection of eyes in drowsy situations, where the EAR is below the threshold value.
The system further enhances its robustness by integrating a CNN. If the EAR falls below the threshold for 20 consecutive frames, the cropped face region is passed through the CNN for confirmation. This hybrid approach ensures higher accuracy and reduces false positives and negatives. At 30 FPS, this corresponds to approximately 0.67 s of continuous eye closure, aligning more closely with the durations used in existing studies (Bhope, 2019; Thulasimani et al., 2021) and better distinguishing between normal blinks and drowsiness indicators.
The values obtained during the testing of the system, where the threshold value for the number of frames was set to “20”. This implies that the driver will be determined as drowsy if the EAR is less than 0.3 for 20 consecutive frames. If the EAR goes below 0.3 for less than 20 frames, a drowsiness alert will not be generated. Equation 5 shows the formula for the eye aspect ratio, where points
The pre-trained models obtained previously are implemented for real-time drowsiness detection on a Raspberry Pi board, to creaate a real-time anti-sleep alert system. The hardware components required to build the real-time anti-sleep alert system are shown in Figure 16. The specific roles of those components in the functioning of the prototype, which is presented in Figure 17, are described as follows.
The Raspberry Pi 4B serves as the main processing unit for the prototype, a versatile, low-cost single-board computer equipped with a quad-core Cortex-A72 CPU, 2GB RAM, and VideoCore VI GPU, providing sufficient processing power to handle the real-time video processing required for drowsiness detection (Kumar et al., 2020; Biswal et al., 2021). It runs the necessary algorithms for detecting driver fatigue, processes the input from the web camera, and triggers alerts when drowsiness is detected. The choice of Raspberry Pi makes the system compact, cost-effective, and energy-efficient, which is essential for an in-vehicle environment (Daengsi et al., 2021).
A high-definition web camera is mounted in front of the driver to continuously capture video footage of their face. The Logitech HD Webcam C270, with its 720p resolution and RightLight™ technology, ensures clear image capture even in varying lighting conditions. The camera is used to track facial features such as eye movement and head position, which are crucial indicators of drowsiness. The camera must provide clear images in varying light conditions, such as during day and night driving, making infrared capabilities a beneficial addition for night-time detection.
The buzzer acts as the primary alert mechanism in the prototype. When the system detects signs of drowsiness or prolonged eye closure, the Raspberry Pi triggers the buzzer, emitting a loud sound to alert the driver. The CentIoT Speaker Buzzer Module, with its 5V operating voltage and compact printed circuit board (PCB) design, ensures reliable and efficient auditory alerts. This immediate auditory feedback ensures that the driver becomes aware of their drowsiness and can take appropriate action to prevent an accident.
The card reader serves as an additional component that can be used to log driver activity. For example, drivers may use a card to log in before starting a journey, allowing the system to track individual driving sessions. This can be particularly useful for fleet management, where multiple drivers use the same vehicle. The SanDisk ULTRA 64 GB MicroSDXC card, with its high speed and durability, ensures reliable storage of driver-specific data and drowsiness patterns. The system can store data related to each driver’s drowsiness patterns and provide insights into long-term fatigue management.
A reliable power source is critical for the continuous operation of the system. The prototype can be powered either through the vehicle’s battery or through a portable power bank, depending on the vehicle’s configuration. For vehicles with integrated USB power outlets, the Raspberry Pi and connected devices can easily draw power directly, ensuring uninterrupted monitoring throughout the drive. The Raspberry Pi 15.3W USB-C power supply ensures stable and efficient power delivery, critical for maintaining system reliability during operation.
After a drowsiness warning is triggered, the system issues an initial alert using a buzzer. This immediate auditory alert is intended to capture the driver’s attention and prompt corrective action, such as pulling over or focusing on the road. The system continues monitoring the driver’s condition in real time to ensure sustained alertness.
The system tracks real-time EAR values and monitors if the driver’s response (e.g., eyes reopening) indicates regained alertness. If drowsiness indicators persist despite the initial alert, the system escalates the warning to more prominent alerts.
The system is designed to monitor the driver’s face using a web camera that continuously captures live images or video feed. The web camera is strategically placed inside the vehicle to ensure a clear view of the driver’s face, focusing on key facial features like the eyes and head position. This real-time data acquisition is essential for detecting early signs of drowsiness, such as slow eye blinking or head nodding, which are critical indicators of fatigue.
Unlike cloud-based systems, where data is sent to remote servers for processing, this system operates entirely locally, whose work sequence is shown in Figure 18. All image and video data captured by the web camera is processed directly on the Raspberry Pi 4B. The decision to use local data storage and processing instead of relying on a cloud platform is crucial for several reasons which are discussed in the following points.
The primary goal of this system is to provide instantaneous alerts when drowsiness is detected. Sending data to the cloud for processing introduces unavoidable latency due to network transmission delays, which could result in critical time loss. A real-time system requires the ability to analyze data within milliseconds to effectively warn the driver before an accident occurs. The Raspberry Pi 4B, with its sufficient computational power, ensures that data processing happens immediately upon capture, allowing the system to maintain real-time performance with an execution time of just 0.2 s.
The local processing approach also makes the system self-sufficient and operational in environments with poor or no internet connectivity. This is especially important for vehicles traveling through rural areas or regions with unreliable network coverage, where cloud-based systems would struggle to operate. By storing and analyzing data on the Raspberry Pi, the system can function independently of network availability, ensuring continuous operation regardless of location.
Another key advantage of local processing is enhanced privacy and security. Since all data, including potentially sensitive facial images, is processed locally, there is no need to transmit it to external servers, reducing the risk of data breaches or unauthorized access. This makes the system particularly attractive for privacy-conscious users or industries where data security is a top priority, such as in fleet management for commercial vehicles.
Operating without reliance on cloud services also makes the system more cost-effective. Cloud platforms often charge for data storage, bandwidth usage, and computational resources. By keeping all processing local, the system avoids these recurring costs, making it more affordable for individual users or small businesses who wish to implement drowsiness detection without incurring high operational expenses.
After the data is processed locally on the Raspberry Pi, the system assesses the driver’s level of alertness by analyzing the facial features. If the system detects drowsiness, it will trigger an immediate alert, such as a loud buzzer or vibration in the seat. The system continuously monitors the driver’s condition, ensuring they remain alert throughout the drive.
The study introduced an algorithm that uses DL to detect drowsiness by classifying different eye states, incorporating InceptionV3, VGG16, and MobileNetV2 architectures. Performance was evaluated using metrics such as accuracy, precision, recall, F1 score, and the confusion matrix, with impressive validation accuracy achieved across all models: 99.18% for InceptionV3, 98.80% for VGG16, and 99.01% for MobileNetV2. InceptionV3 demonstrated superior performance with rapid execution time on Raspberry Pi, making it highly suitable for real-time applications. The system utilizes non-invasive image processing techniques to classify eye states, offering a practical and scalable solution for fatigue detection and real-time alerts to enhance road safety.
While the results indicate high accuracy and efficiency, the absence of extreme weather conditions in the dataset emphasizes an opportunity for improvement through the inclusion of diverse scenarios, further optimizing the system’s real-world applicability and potential to reduce accidents. Future iterations of the system could incorporate escalating alerts, such as adaptive buzzer volumes, seat vibrations, visual cues, or integration with vehicle safety features like automated deceleration and hazard light activation, to enhance robustness and real-world effectiveness.
The original contributions presented in the study are included in the article/supplementary material, further inquiries can be directed to the corresponding author.
AP: Conceptualization, Data curation, Investigation, Methodology, Software, Writing–original draft. AS: Conceptualization, Investigation, Writing–original draft. PK: Formal Analysis, Methodology, Project administration, Supervision, Validation, Writing–review and editing. VB: Formal Analysis, Funding acquisition, Project administration, Resources, Supervision, Validation, Visualization, Writing–review and editing. OK: Funding acquisition, Project administration, Supervision, Validation, Visualization, Writing–review and editing.
The author(s) declare that financial support was received for the research, authorship, and/or publication of this article. This research was supported by the Science and Engineering Research Board (SERB), Department of Science and Technology, Government of India, under project file number CRG/2021/001215. Additionally, this work received partial support from the Long-Term Conceptual Development of the Research Organization at Škoda Auto University. Additionally, this work was partially supported by the DRISHTI CPS Chair Professorship.
The authors declare that the research was conducted in the absence of any commercial or financial relationships that could be construed as a potential conflict of interest.
The author(s) declare that no Generative AI was used in the creation of this manuscript.
All claims expressed in this article are solely those of the authors and do not necessarily represent those of their affiliated organizations, or those of the publisher, the editors and the reviewers. Any product that may be evaluated in this article, or claim that may be made by its manufacturer, is not guaranteed or endorsed by the publisher.
Abtahi, S., Omidyeganeh, M., Shirmohammadi, S., and Hariri, B. (2014). “Yawdd: a yawning detection dataset,” in Proc. of the 5th ACM multimedia systems conf, 24–28. doi:10.1145/2557642.2563678
Alzubi, J. A., Jain, R., Alzubi, O., Thareja, A., and Upadhyay, Y. (2022). Distracted driver detection using compressed energy efficient convolutional neural network. J. Intelligent & Fuzzy Syst. 42, 1253–1265. doi:10.3233/JIFS–189786
Alzubi, J. A., Jain, R., Kathuria, A., Khandelwal, A., Saxena, A., and Singh, A. (2020). Paraphrase identification using collaborative adversarial networks. J. Intelligent & Fuzzy Syst. 39, 1021–1032. doi:10.3233/jifs–191933
Bhope, R. A. (2019). “Computer vision based drowsiness detection for motorized vehicles with web push notifications,” in 2019 4th Int. conf. on internet of things: smart innovation and usages (IoT-SIU) (IEEE), 1–4. doi:10.1109/IoT–SIU.2019.8777652
Biswal, A. K., Singh, D., Pattanayak, B. K., Samanta, D., and Yang, M. H. (2021). Iot-based smart alert system for drowsy driver detection. Wirel. comm. Mob. Comput. 2021 2021, 6627217. doi:10.1155/2021/6627217
Chen, L., Li, S., Bai, Q., Yang, J., Jiang, S., and Miao, Y. (2021). Review of image classification algorithms based on convolutional neural networks. Remote Sens. 13, 4712. doi:10.3390/rs13224712
Daengsi, T., Poonwichein, T., and Wuttidittachotti, P. (2021). “Drowsiness or sleep detection and alert system: development of a prototype using Raspberry PI,” in 2021 2nd int. Conf. For emerging tech. (INCET) (IEEE), 1–4. doi:10.1109/INCET51464.2021.9456162
Deshmukh, P., Majhi, S., Sahoo, U. K., and Das, S. K. (2024). Vehicle detection in diverse traffic using an ensemble convolutional neural backbone via feature concatenation. Transp. Lett. 16, 838–856. doi:10.1080/19427867.2023.2250622
Dewi, C., Chen, R. C., Jiang, X., and Yu, H. (2022). Adjusting eye aspect ratio for strong eye blink detection based on facial landmarks. PeerJ Comput. Sci. 8, e943. doi:10.7717/peerj-cs.943
Dong, K., Zhou, C., Ruan, Y., and Li, Y. (2020). “Mobilenetv2 model for image classification,” in 2020 2nd int. Conf. On info. Tech. And computer application (ITCA) (IEEE), 476–480. doi:10.1109/ITCA52113.2020.00106
Fu, B., Boutros, F., Lin, C. T., and Damer, N. (2024). A survey on drowsiness detection - modern applications and methods. IEEE Trans. Intell. Veh., 1–23. doi:10.1109/TIV.2024.3395889
Fusek, R. (2018). “Pupil localization using geodesic distance,” in Advances in visual computing (Las Vegas, NV: Springer), 13, 433–444. doi:10.1007/978-3-030-03801-4_38
Kamran, M. A., Mannan, M. M. N., and Jeong, M. Y. (2019). Drowsiness, fatigue and poor sleep’s causes and detection: a comprehensive study. Ieee Access 7, 167172–167186. doi:10.1109/ACCESS.2019.2951028
Kumar, A., Kaur, A., and Kumar, M. (2019). Face detection techniques: a review. AI Rev. 52, 927–948. doi:10.1007/s10462-018-9650-2
Kumar, V. S., Ashish, S. N., Gowtham, I., Balaji, S. A., and Prabhu, E. (2020). Smart driver assistance system using raspberry pi and sensor networks. Microprocess. Microsystems 79, 103275. doi:10.1016/j.micpro.2020.103275
LaRocco, J., Le, M. D., and Paeng, D. G. (2020). A systemic review of available low-cost EEG headsets used for drowsiness detection. Front. Neuroinformatics 14, 553352. doi:10.3389/fninf.2020.553352
Liu, M. Z., Xu, X., Hu, J., and Jiang, Q. N. (2022). Real time detection of driver fatigue based on CNN-LSTM. IET Image Process. 16, 576–595. doi:10.1049/ipr2.12373
Lu, G., Zhang, W., and Wang, Z. (2021). Optimizing depthwise separable convolution operations on gpus. IEEE Trans. Parallel Distributed Syst. 33, 70–87. doi:10.1109/TPDS.2021.3084813
Mahajan, K., and Velaga, N. R. (2023). Effects of partial sleep deprivation: speed management ability and associated crash risk. Transp. Lett. 15, 527–536. doi:10.1080/19427867.2022.2071533
Movassagh, A. A., Alzubi, J. A., Gheisari, M., Rahimi, M., Mohan, S., Abbasi, A. A., et al. (2023). Artificial neural networks training algorithm integrating invasive weed optimization with differential evolutionary model. J. Ambient Intell. Humaniz. Comput. 14, 6017–6025. doi:10.1007/s12652–020–02623–6
Peng, Z., Pan, H., Yuan, R., and Wang, Y. (2024). A comparative analysis of risk factors influencing crash severity between full-time and part-time riding-hailing drivers in China. Transp. Lett., 1–16. doi:10.1080/19427867.2024.2369827
Ramzan, M., Khan, H. U., Awan, S. M., Ismail, A., Ilyas, M., and Mahmood, A. (2019). A survey on state-of-the-art drowsiness detection techniques. IEEE Access 7, 61904–61919. doi:10.1109/ACCESS.2019.2914373
Sandler, M., Howard, A., Zhu, M., Zhmoginov, A., and Chen, L. C. (2018). “Mobilenetv2: inverted residuals and linear bottlenecks,” in Proc. Of the IEEE conf. On computer vision and pattern recognition, 4510–4520. doi:10.1109/CVPR.2018.00474
Sathya, T., and Sudha, S. (2024). An adaptive fuzzy ensemble model for facial expression recognition using poplar optimization and CRNN. IETE J. Res. 70, 4758–4769. doi:10.1080/03772063.2023.2220691
Sun, Z., Chuah, J. H., and Chai, G. M. T. (2023). “Eye state recognition using InceptionV3 and VGG16,” in 2023 Int. Conf. on AI, Blockchain, Cloud Computing, and Data Analytics (ICoABCD) (IEEE), 94–99. doi:10.1109/ICoABCD59879.2023.10390904
Suresh, A., Naik, A. S., Pramod, A., Kumar, N. A., and Mayadevi, N. (2023). “Analysis and implementation of deep convolutional neural network models for intelligent driver drowsiness detection system,” in 2023 7th int. Conf. On intelligent computing and control systems (ICICCS) (IEEE), 553–559. doi:10.1109/ICICCS56967.2023.10142299
Szegedy, C., Vanhoucke, V., Ioffe, S., Shlens, J., and Wojna, Z. (2016). “Rethinking the inception architecture for computer vision,” in Proc. Of the IEEE conf. On computer vision and pattern recognition, 2818–2826. doi:10.1109/CVPR.2016.308
Tao, J., Gu, Y., Sun, J., Bie, Y., and Wang, H. (2021). “Research on VGG16 CNN feature classification algorithm based on transfer learning,” in 2021 2nd China int. SAR symposium (CISS) (IEEE), 1–3. doi:10.23919/CISS51089.2021.9652277
Taye, M. M. (2023). Theoretical understanding of convolutional neural network: concepts, architectures, applications, future directions. Computation 11, 52. doi:10.3390/computation11030052
Thakkar, V., Tewary, S., and Chakraborty, C. (2018). “Batch normalization in CNN — a comparative study with CIFAR-10 data,” in 2018 fifth int. Conf. On emerging Applications of info. Tech. (EAIT) (IEEE), 1–5. doi:10.1109/EAIT.2018.8470438
Thulasimani, L., Poojeevan, P., and Prithashasni, S. P. (2021). Real time driver drowsiness detection using opencv and facial landmarks. Int. J. Aquatic Sci. 12, 4297–4314.
Van Thieu, N. (2024). PerMetrics: a framework of performance metrics forMachine learning models. J. Open Source Softw. 9, 6143. doi:10.21105/joss.06143
Venkata Phanikrishna, B., Jaya Prakash, A., and Suchismitha, C. (2023). Deep review of machine learning techniques on detection of drowsiness using EEG signal. IETE J. Res. 69, 3104–3119. doi:10.1080/03772063.2021.1913070
Visual Geometry Group (2024). “Visual geometry group department of engg. science,”. University of Oxford Available here.
Yan, J. J., Kuo, H. H., Lin, Y. F., and Liao, T. L. (2016). “Real-time driver drowsiness detection system based on perclos and grayscale image processing,” in 2016 int. Symposium on computer, consumer and control (IS3C) (IEEE), 243–246. doi:10.1109/IS3C.2016.72
Keywords: driver drowsiness detection, deep learning models, real-time monitoring, InceptionV3, transfer learning, eye aspect ratio (EAR), Raspberry Pi, driver alert systems
Citation: Pathak AK, Singh AK, Kumar P, Bhatia V and Krejcar O (2025) Real-time anti-sleep alert algorithm to prevent road accidents to ensure road safety. Front. Future Transp. 6:1545411. doi: 10.3389/ffutr.2025.1545411
Received: 14 December 2024; Accepted: 12 February 2025;
Published: 12 March 2025.
Edited by:
Qinaat Hussain, Qatar University, QatarReviewed by:
Jafar A. Alzubi, Al-Balqa Applied University, JordanCopyright © 2025 Pathak, Singh, Kumar, Bhatia and Krejcar. This is an open-access article distributed under the terms of the Creative Commons Attribution License (CC BY). The use, distribution or reproduction in other forums is permitted, provided the original author(s) and the copyright owner(s) are credited and that the original publication in this journal is cited, in accordance with accepted academic practice. No use, distribution or reproduction is permitted which does not comply with these terms.
*Correspondence: Vimal Bhatia, dmJoYXRpYUBpaXRpLmFjLmlu
Disclaimer: All claims expressed in this article are solely those of the authors and do not necessarily represent those of their affiliated organizations, or those of the publisher, the editors and the reviewers. Any product that may be evaluated in this article or claim that may be made by its manufacturer is not guaranteed or endorsed by the publisher.
Research integrity at Frontiers
Learn more about the work of our research integrity team to safeguard the quality of each article we publish.