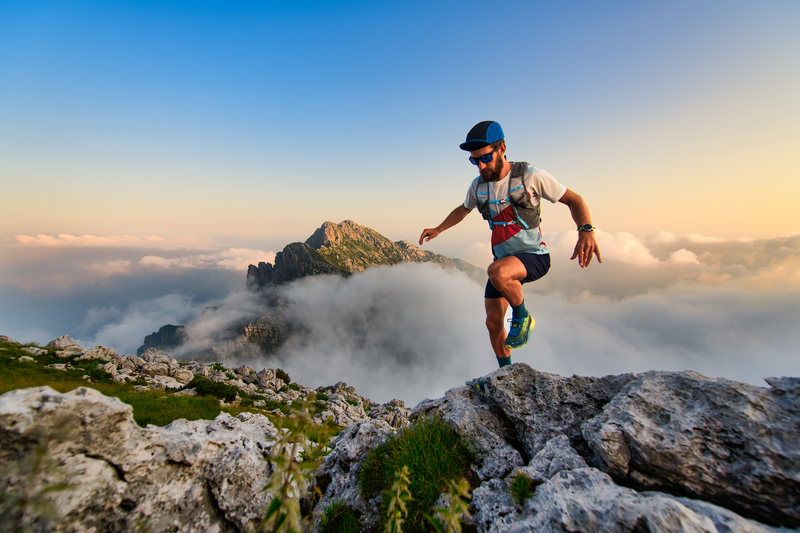
95% of researchers rate our articles as excellent or good
Learn more about the work of our research integrity team to safeguard the quality of each article we publish.
Find out more
MINI REVIEW article
Front. Future Transp. , 28 October 2024
Sec. Transport Safety
Volume 5 - 2024 | https://doi.org/10.3389/ffutr.2024.1458828
Like inattention, dysregulated emotions can seriously distract motorists and disrupt their driving performance. The current review examined the literature on emotion regulation to find relevant strategies for managing intense emotions while driving a motor vehicle. Next, we considered whether those relevant strategies could be used to design future affective automotive interfaces. Results suggested that problem-solving, task-focused coping, mindfulness, and relaxation effectively regulate emotions while driving. These findings highlight that some strategies may be effective but costly to implement while driving (cognitive reappraisal and distraction), which need to be better understood before implementing them into automotive interfaces. Together, these findings suggest the potential benefits of embedding self-regulatory interventions in future automotive interfaces that can help maintain an optimal affective state to reduce distraction and improve safe driving.
Distracted driving is a significant cause of on-road accidents, and automotive interfaces1 can play an active role in helping motorists drive safely. While most past research has focused on countering inattention (for review, see Regan et al., 2017), limited work has evaluated effective ways to regulate intense emotions while driving. However, the transactional model of driver stress (Matthews, 2001) suggests an inherent connection between the motorist operating the vehicle and their environment, which has meaningful impacts on cognition, affect, and performance. In other words, the internal intra-individual factors of the driver (such as emotions and stress) and inter-personal factors (such as socializing in-person or virtually) can have a pervasive association with cognitive resources, thereby impacting optimal driving behavior (Braun et al., 2021; Matthews, 2001).
Regarding internal factors, an important consideration is the emotional state of the motorist. Past research has recognized several specific emotions of particular concern in the driving context (for a targeted review, see Braun et al., 2021; Eyben et al., 2010; Bernstein and Calamia, 2019). One of the most researched emotions is anger while operating vehicles. A 20-year meta-analysis found that angry driving is linked to poor driving outcomes such as risky and aggressive driving with higher driving errors (Zhang and Chan, 2016). Anger was found to cause more errors (Jeon et al., 2011), speeding (Mesken et al., 2007), and unsafe driving (Roidl et al., 2014). When left dysregulated, impulsive behavior is an important contributor to aggressive road rage (Kovácsová et al., 2016). Frustration while driving is also a closely related affective state that’s known to increase negative emotional experiences, often causing anger and road rage (Harris and Nass, 2011). Similarly, stress can be detrimental to attention and engagement, lowering driving performance (Matthews et al., 1998; Tavakoli et al., 2023). A common stress-inducing situation is time pressure, which is harmful to human performance (Szalma et al., 2008) and can lead to risky driving (Shukri et al., 2022). While anger and stress can be higher-intensity states, even sadness, a lower-intensity state, can worsen and increase unsafe driving conditions (Dula and Geller, 2003). These findings are in line with theoretical perspectives (Yerkes and Dodson, 1908; Cai and Lin, 2011) that suggest motorists should be in an optimal driving state (i.e., not too much or too little physiologically aroused) that allows them to have adequate resources for attentive and safe driving. Thus, effective emotion regulation is essential for optimal driving performance (Matthews, 2001; Wickens et al., 2022).
In terms of external factors, inter-personal social conditions are critical. Past work on dual-task interference models in automation research suggests that conflicting tasks compete for limited resources (e.g., Engström et al., 2017; Pashler and Johnston, 1998; Wickens et al., 2004; Wickens, 2021a; Wickens et al., 2021b). For instance, road crashes were associated with responding to calls, using the web browser while driving, or even when reaching for the phone (Charlton, 2009; Dingus et al., 2016; Rakauskas et al., 2004; Venkatraman et al., 2021). This is because talking and texting are cognitively demanding (e.g., need working memory and executive control), leading to increased reaction times in vehicle operation and interfering with driving performance (e.g., Charlton, 2009; Hancock et al., 2012). With more demanding cognitive tasks, self-control becomes more difficult, and people start to have selective attention, change blindness, and compromise safe driving conditions (e.g., Wickens et al., 2021b). Furthermore, the availability of infotainment systems and touchscreen displays with social media has further aggravated the sheer number of social distractors available to motorists, thereby compromising safe driving (e.g., Regan et al., 2017). Thus, the secondary task of engaging in social activities may become intrusive toward driving, leading to socially distracted performance on the road.
Even though drivers should give a higher priority to maintain the appropriate allocation of resources to driving, ignoring any socioemotional interference (Wickens, 2021a; Wickens et al., 2021b), often, this is quite challenging to do (e.g., Engström et al., 2017; Ma et al., 2024), especially when motorists experience intense emotions. Taking the example of road rage (Hennessy and Wiesenthal, 2005; Takaku, 2006; Zhang and Chan, 2016), dysregulated emotions can lead to unsafe driving practices (such as impulsive driving) with tragic consequences, as revealed by 67% of crashes attributed to aggressive driving (Goodwin et al., 2015). While emotional dysregulation can lead to risky, angry, or anxious driving, successful self-regulation can support safe driving behavior (Bjureberg and Gross, 2021). A similar pattern is predicted by the transactional model of driver stress, which suggests that driving performance is impacted by dysregulated socioemotional cognitions (Matthews et al., 1998). Therefore, despite these well-documented adverse effects on driving performance, motorists often experience disruptive internal and interpersonal conditions, and a better understanding of ways to counter emotionally distracted driving is critical.
Yet, a limited understanding exists of efficient approaches motorists can implement to regulate emotions and behavior to maximize safety. How can drivers manage their emotional states to ensure safe driving? Furthermore, given the growth in artificial intelligence, what can automotive user interfaces do to mitigate sub-optimal driving conditions? These questions informed the current literature review. The overarching goal of this work was to identify adaptive approaches to counter the adverse effects of driving under socioemotional distraction with the long-term goal of designing effective strategies for automotive interfaces. We looked for possible self-regulation strategies that effectively manage the dysregulated states of motorists and can potentially be embedded in automotive interfaces. In this paper, we first provide background literature, summarize the key findings from our literature review, and then recognize the current challenges and future implications.
Emotion regulation includes all attempts to modify emotions people are experiencing, when they experience them, and how they express them (Gross, 1998; Koole, 2009). Emotion regulation is generally implemented to meet an underlying goal (Inzlicht et al., 2021), such as the goal to get less angry because it will otherwise hurt driving performance. Given that the current review focused on identifying strategies that lead to positive outcomes (e.g., better health or good driving performance), we adopted the strategy-based effectiveness framework as quantitatively examined by Aldao et al. (2010). Past meta-analysis in the psychopathology context has empirically examined the effectiveness of different strategies by connecting them to mental health outcomes and categorizing them as effective versus ineffective (Aldao et al., 2010). In line with this framework, emotion regulation strategies can be classified as effective if they are linked to adaptive outcomes (e.g., better health or performance; Aldao et al., 2010). In contrast, ineffective strategies are maladaptive in achieving the desired outcomes (e.g., failing to control anger or improve driving performance).
While there are hundreds of emotion regulation strategies, only a few have thus far been examined in the driving context. Following the effectiveness framework (Aldao et al., 2010), we examined the adaptiveness of existing approaches that motorists commonly adopt to manage their socioemotional responses while operating a motor vehicle. Specifically, we examined existing research to establish which emotion regulation strategies effectively regulated motorists’ socioemotional states to improve/maintain optimal driving performance. After reviewing the literature, the following emotion regulation strategies were found to be relevant to socially distracted driving and were associated with potential impact on driving performance, health, or affective states: cognitive reappraisal, instrumental problem-focused coping, task-focused coping, mindfulness, relaxation, forgiveness, hostile emotional coping and confrontation, self-criticism, rumination, and suppression. Below, they have been categorized by the effective (or adaptive) versus ineffective (maladaptive) framework (Aldao et al., 2010).
For each emotion regulation strategy, we describe what effects on driving performance were found and whether they were effective vs ineffective. In order to determine which strategies are effective vs ineffective in regulating driving behavior in emotional contexts, their effect on performance and wellbeing was evaluated. Accordingly, we assessed which adaptive approaches can be built as features into automotive user interfaces to modify motorists’ states and support safe driving. Finally, we recognize gaps in the current literature and highlight steps moving forward to adopt approaches that can mitigate the negative impacts of social distraction while driving. See Table 1 for study summaries.
Following the effectiveness framework for emotion regulation strategies, the adaptiveness of each strategy was examined. If an emotion regulation strategy was found to maintain or improve performance and/or reduce stress or negative emotions, it was deemed effective. Based on these criteria, the following families of strategies were categorized as effective.
Mindfulness is an effective strategy focused on living in the moment when dealing with driving-related stress. It is found to have a calming effect that can reduce anger and make motorists less likely to express aggressive driving behavior (Deffenbacher et al., 2000; Deffenbacher et al., 2003; Deffenbacher et al., 2016; Galovski et al., 2003; Kazemeini et al., 2013). Trait-level mindfulness is generally linked to less anger and aggressive driving (Stephens et al., 2018). Those who are more mindful at trait levels are less aggressive drivers (Duany and Mouloua, 2022). Moreover, mindfulness-based cognitive therapy was found to reduce driving anger and aggression relative to a control group that received typical cognitive-behavioral therapy (Kazemeini et al., 2013). The evidence suggests that tailored mindfulness interventions would help reduce driving stress and aggression.
However, several kinds of mindfulness interventions exist (Lohani et al., 2020), and certain specific elements of it can be adapted to driving context. In terms of adapting mindfulness to vehicular interfaces, mindfulness-based interventions could be introduced to help regulate emotions, but this work is in its infancy. Much needs to be empirically determined regarding components of mindfulness interventions that can be successfully employed while operating a motor vehicle safely. For instance, past research has adopted acceptance to regulate emotions, which includes nonjudgemental processing of one’s experiences (Lohani et al., 2018). In future work, forms of acceptance as an emotion regulation strategy can be taught to drivers to help them accept negative cognitions before acting on them (Kazemeini et al., 2013).
Relaxation is a generic term that includes all efforts to self-soothe, such as calming one’s body (e.g., breath) or manipulating the environment (e.g., aroma). A motorist can initiate relaxation internally, such as efforts to regulate one’s breathing. In fact, a recent study (Balters et al., 2019) tested an intervention to reduce the breathing rate, which can relax the motorist while driving in real-time. At the same time, other forms of relaxation can be built within the environment of automotive interfaces. A good example is from recent literature that supports the use of aroma (Demitrenko et al., 2020) to regulate negative states link anger while operating vehicles (Dmitrenko et al., 2018). These findings suggest that relaxation techniques initiated by automotive interfaces are a promising way to manage intense emotional states that may arise while driving.
Instrumental problem-focused coping involves finding an alternative solution to resolve the issue, such as acknowledging error when at fault. It has been shown to effectively resolve situations intended to engage the other driver without aggression (e.g., short honks and light flashing) without escalating them (Shamoa-Nir, 2023). A particular form of problem-solving strategy is task-focused coping, which focuses on engaging with the driving task and helps motorists not get distracted. While problem-focused coping is a more generic umbrella term, task-focused coping addresses the problem of distraction that may worsen performance. Past research has found that being task-focused helps motorists perform safe driving (Hancock et al., 2012; Shamoa-Nir, 2023). For instance, task-focused drivers drove more carefully when considering speed limits (Hancock et al., 2012). Given the wide range of problems that may arise while driving, this is a challenging strategy to build in automotive interfaces. However, more manageable and frequently occurring problems could be examined and built into future vehicular systems.
Forgiveness is pardoning someone and overcoming resentment towards them. It was associated with more patience and careful driving (Feng et al., 2018; Navon and Taubman-Ben-Ari, 2019). Previous studies have found a significant negative relationship between trait forgiveness and driving anger and aggressive driving behaviors (Bumgarner et al., 2016; Moore and Dahlen, 2008). A recent study (Lakshita and Padmakumari, 2023) found a negative correlation between forgiveness and anger experienced while driving. Individuals who could forgive during negative situations displayed less verbal aggression and tended not to use the vehicle to express anger toward other drivers (Lakshita and Padmakumari, 2023). Even though Bumgarner et al. (2016) found a negative association between forgiveness at trait levels and driving anger, they note that they did not find a direct relationship between forgiveness and reckless driving behavior. However, it still suggests that individuals who tend to forgive others (at the trait level) are likely to have less driving anger, even in situations that would provoke negative emotions (Bumgarner et al., 2016). Overall, current literature suggests that individuals who are more likely to forgive are likely to experience less driving anger. However, forgiveness depends on the situation at hand that needs a resolution, so it may not always be feasible. Also, it is a difficult strategy to embed in an automotive system and may even come at the cost of annoying the motorist.
Cognitive reappraisal includes a cognitive reinterpretation of the driving situation (Gross, 1998). It mitigated intense situations by generating alternative explanations for others’ (aggressive) behaviors, leading to safer driving behaviors (careful driving, better driving scores, optimal speed, and less road rage; Harris and Nass, 2011; Roberton et al., 2012; Takaku, 2006). Indeed, cognitive interventions designed to adopt cognitive reappraisal effectively reduced anger and aggression (Feng et al., 2018; Kazemeini et al., 2013). Cognitive reappraisal has also been adopted in automotive contexts with some success. When regulatory instructions were played in the vehicle while motorists drove, this intervention helped reduce anger, hostility, and frustration levels (Corzo et al., 2024). This suggests that cognitive reappraisal can be adopted in automotive interfaces.
However, it is noteworthy that cognitive reappraisal is considered a cognitively demanding strategy (Sheppes, 2020), and it may not necessarily be feasible in low cognitive resources, high-stress, and time-sensitive situations. Further work is needed to test this specifically in driving contexts. Past research has found that cognitive intervention efficiently reduced total scores of driving anger and physical aggression expression and promoted positive cognitive strategies to improve negative emotions better than the control group (Feng et al., 2018). Cognitive intervention groups experienced greater improvements than the forgiveness intervention regarding obstruction in traffic and using positive cognitive strategies (Feng et al., 2018), suggesting the effectiveness of training cognitive reappraisal via cognitive interventions.
In line with the effectiveness framework (Aldao et al., 2010), strategies were investigated for being maladaptive. If an emotion regulation strategy was found to hurt performance and/or worsen stress or emotional state, it was deemed ineffective. Based on our examination, the following families of strategies were categorized as ineffective.
Hostile and confrontive emotional coping includes negative, reactive, and often aggressive behaviors toward other motorists during frustrating driving scenarios (Matthews, 2002), which can frequently lead to angry and risky driving performance (Emo et al., 2004). It was associated with higher anger and maladaptive behaviors (Lennon and Watson, 2012; Emo et al., 2004; Emo et al., 2016; Shamoa-Nir, 2023) and predicted unsafe driving behaviors such as tailgating, frequent passing, and shorter delays before passing other vehicles, which reduced safety (Emo et al., 2016). Another study found that men with above-average anger engaged in more physical and verbal aggression with other drivers than controls. For example, they reported more anger and intentional harm toward other motorists who were not driving fast enough (Deffenbacher et al., 2000), highlighting its ineffectiveness.
Self-criticism (tendency to negatively evaluate oneself) resulting in feelings of worthlessness, guilt, and failure) increased worrying in traffic environments and lowered self-esteem, leading to counterproductive behaviors such as risky driving behavior (Matthews et al., 1998). Kontogiannis (2006) found a positive association between aggression and self-criticism. They also suggested that aggressive drivers may be aware of their tendency to display aggressive behavior, but they are unable to take actions that could help them prevent their behaviors (Kontogiannis, 2006). Self-criticism is a sign of dysregulation and is associated with ruminative thoughts and negative emotions (Kalokerinos et al., 2017), which may lead to ineffective driving performance, but this remains to be tested in the driving context. On a related note, rumination (i.e., engaging repetitive thought processes) is also considered ineffective based on some trait-level assessments. Motorists who are higher ruminators have higher anger driving scores and a history of aggressive driving (Suhr and Nesbit, 2013).
Suppression (inhibiting ongoing emotional response) was also ineffective and associated with aggressive driving (Popuşoi and Holman, 2016). Relative to controls, drivers with trait anger issues were observed to be angry and anxious while driving (Deffenbacher et al., 2000). Perhaps anger suppression may displace those feelings to unrelated situations like driving in traffic (Deffenbacher et al., 2000). It is possible that those who frequently adopt anger suppression may unknowingly use aggressive driving to release pent-up emotions.
While much work has focused on maintaining optimal levels of attention, a limited understanding exists of effective ways to regulate one’s behavior during emotionally distracted driving. Following the approach of an effectiveness framework (Aldao et al., 2010), we reviewed existing work exploring emotion regulation strategies motorists may adapt to emotional challenges experienced in the driving context. This review found several promising approaches to counter dysregulated socioemotional states, such as by engaging in instrumental problem-solving, task-focused coping, mindfulness, relaxation, forgiveness, and cognitive reappraisal to maintain safer driving behaviors and performance effectively. We highlight three implications of these findings.
First, it is important to communicate these empirical findings to users (i.e., on-road motorists). At the same time, it is challenging to implement the known effective approaches to regulating driving behavior in real-world conditions. Thus, motorists need training to implement effective solutions in distracting or stressful environments. Past work on training cognitive behavioral therapy for anger treatment (Galovski et al., 2003; Galovski et al., 2006; Kazemeini et al., 2013) and music therapy (Wiesenthal et al., 2003) had reasonable success in reducing aggressive driving. Evidence-based training platforms need to be developed to help motorists successfully implement effective emotion regulation strategies.
Second, automotive interfaces can be of tremendous support in helping motorists generate relevant (and personalized) strategies to manage emotional dysregulation (Braun et al., 2019). To design such supportive automotive interfaces (also called future in-vehicle assistants), one approach is that the system senses (i.e., record) and assesses (i.e., determine) intense emotional states (e.g., anger) and suggests possible effective ways to regulate (or augment) the drivers’ emotional state (Lohani et al., 2020; Stokes et al., 2023; Oehl et al., 2019). However, a major challenge is having reliable systems that can sense emotions in real-world settings (Lohani et al., 2019; Lohani et al., 2024). At the same time, recent work has made progress in utilizing reliable behavioral and psychophysiological measures to assess cognitive states when driving on real roads (e.g., Lohani et al., 2020; Lohani et al., 2021). With an improved understanding of effective regulation and success in detecting dysregulated behavioral and physiological responses (Galovski et al., 2003; Yan et al., 2018), better automated technology could be developed (Wan et al., 2017) to trigger warning signs and intervene and promote driving safety (Lohani et al., 2019; Kim et al., 2024).
Finally, it is also important to recognize that motorists may not always be aware or able to de-escalate the situation themselves, which would be a critical opportunity for the automotive interface to intervene and mitigate the problem. Future work should also utilize automation as an in-vehicle intervention to regulate driver states, such as anger (Li et al., 2021; Wu et al., 2022). Interventions can be developed and implemented into intelligent driving systems to train motorists with the skills needed for effective regulation under stressful driving conditions (Hernandez et al., 2014). For instance, personalized speech-based interventions for aggressive drivers (Feng et al., 2018; Harris and Nass, 2011) have shown promising results and, with further work, can be built for everyday consumers. A related challenge is the high-risk and time-sensitive nature of driving on real roads. Having enough time to consult with the motorist may not always be possible (Yang et al., 2023; Garikapati et al., 2024). Thus, much work is needed to find reliable ways of detecting and regulating emotional states under high-risk scenarios via automotive interfaces.
Considering the lessons learned from the current literature review, future automotive interfaces could be embedded with options to initiate and support effective emotion regulation. The emotion regulation literature while operating vehicles thus far has several suggestions for designing interfaces to modify the vehicle environment, which are quite feasible. The interface could be designed to provide external and internal regulation settings that are preferred by the motorist. Such settings could be designed to offer options to regulate the vehicle’s environment based on presets set by the motorist. For instance, when the motorist feels frustrated, their preset favorite aroma could be introduced to mitigate the negativity in the environment (Dmitrenko et al., 2020). Compared to external and environmental management, internal regulation, as needed to be facilitated by the motorists themselves, is harder to do. However, the automotive interfaces could still initiate the process. For example, an interface could utilize self-soothing emotion regulation strategies to manage stress (as tested in past work; Balters et al., 2019; Hernandez et al., 2014). If the interface was already preprogrammed by the motorist even to initiate task-focused coping (e.g., Shamoa-Nir, 2023; Zhao et al., 2024), it could provide what a motorist may need to break the cycle of negativity and rather choose an efficient emotion regulation approach (self-selected by them) to manage their intense emotions. Importantly, motorists should be given the autonomy to be able to control the automotive technology to their liking (Lohani et al., 2020), without which even the best-designed interfaces will remain under-utilized. With rigorous design and testing, automotive interfaces can help motorists untangle intense emotional states, thereby creating safe driving conditions.
At the same time, some limitations need consideration. First, user-friendly design challenges are anticipated as the same set of strategies do not work for all and across situations (Lohani et al., 2022). Thus, a person-centered approach (e.g., Gu et al., 2024) that accounts for individual preferences and dispositions would be more compatible with effectively managing socioemotionally distracted driving. Notably, many other emotion regulation strategies are utilized by people in everyday life (Lohani et al., 2022). However, very few relevant ways to regulate emotions in driving contexts have been studied in the literature thus far, which needs further consideration and exploration. Individual difference factors (such as driving style, stress susceptibility, cultural, and demographic characteristics) of a motorist play a key role in the effectiveness of adaptive regulatory approaches (Ellison-Potter et al., 2001; Shamoa-Nir and Koslowsky, 2010; Suhr and Nesbit, 2013; Tillmann and Hobbs, 1949; Wickens and Wiesenthal, 2005). However, only a few studies exist for a handful of strategies, but replicable studies with adequate power are required in order to gain an individual differences perspective. Given the limited number of studies on emotion regulation in a driving context, enough literature does not exist to examine such individual differences, and this was not the focus of the current literature. This will enable a person-centered design approach and identify what kind of regulation attempts work for whom and when.
Second, due to frequent interruptions by the automotive interface, motorists may often get frustrated or annoyed by the automotive interface trying to change their experiences. Furthermore, in-vehicle automation will need to be successful in gaining acceptance and calibrated trust from its users for optimal success (Winterberger et al., 2016; Lohani et al., 2020; Lohani et al., 2016a; Lohani et al., 2016b; Lohani et al., 2017). Third, some emotion regulation strategies had mixed results and could be resource-demanding. For instance, distraction involves redirecting attention away from negativity (e.g., aggression and stress) and focusing on something else, generally more positive, such as music (Wiesenthal et al., 2003). Using music as a distraction could help lower intense emotions, but it can also add to motorists’ distracted states, further compromising driving performance. Listening to music is a good example of distraction while driving. Motorists, driving while listening to music, were less aggressive, precisely when there was low time pressure (Weisenthal et al., 2003). Distraction, and even reappraisal, may be helpful, but not necessarily under all conditions. For instance, high-traffic conditions may require more cognitive resources, which could compromise driving performance. Costs (cognitive resources) that come with employing emotion regulation strategies (Sheppes, 2020) need consideration to ensure safe driving conditions. Furthermore, some individuals habitually utilize some emotion regulation strategies, which can make them less effortful in implementing them. This emphasizes the importance of designing a person-centered approach to augmenting emotional states.
In conclusion, this review features promising emotion regulation approaches that can be designed and tested in automotive interfaces to maintain motorists’ optimal affective state, thereby reducing distraction and facilitating driving performance. Building support interfaces for regulating drivers’ affective states can benefit fast-growing intelligent driving systems and remarkably improve traffic safety.
ML: Conceptualization, Data curation, Formal Analysis, Investigation, Methodology, Project administration, Supervision, Validation, Writing–original draft, Writing–review and editing. AD: Data curation, Formal Analysis, Investigation, Methodology, Project administration, Validation, Writing–original draft, Writing–review and editing. AA: Data curation, Formal Analysis, Investigation, Methodology, Project administration, Writing–review and editing.
The author(s) declare that no financial support was received for the research, authorship, and/or publication of this article.
The authors declare that the research was conducted in the absence of any commercial or financial relationships that could be construed as a potential conflict of interest.
All claims expressed in this article are solely those of the authors and do not necessarily represent those of their affiliated organizations, or those of the publisher, the editors and the reviewers. Any product that may be evaluated in this article, or claim that may be made by its manufacturer, is not guaranteed or endorsed by the publisher.
1Automotive interfaces are advanced technological systems installed in vehicles to support.
Aldao, A., Nolen-Hoeksema, S., and Schweizer, S. (2010). Emotion-regulation strategies across psychopathology: a meta-analytic review. Clin. Psychol. Rev. 30 (2), 217–237. doi:10.1016/j.cpr.2009.11.004
Balters, S., Landay, J. A., Pablo, E., and Paredes, P. E. (2019). “On-road guided slow breathing interventions for car commuters,” in Extended abstracts of the 2019 CHI conference on human factors in computing systems (CHI EA’19) (Glasgow, Scotland, UK: ACM Press), 1–5. doi:10.1145/3290607.3312785
Bernstein, J. P., and Calamia, M. (2019). Dimensions of driving-related emotions and behaviors: an exploratory factor analysis of common self-report measures. Accid. Analysis and Prev. 124, 85–91. doi:10.1016/j.aap.2019.01.004
Bjureberg, J., and Gross, J. J. (2021). Regulating road rage. Soc. personality Psychol. Compass 15 (3), e12586. doi:10.1111/spc3.12586
Braun, M., Schubert, J., Pfleging, B., and Alt, F. (2019). Improving driver emotions with affective strategies. Multimodal Technol. Interact. 3 (1), 21. doi:10.3390/mti3010021
Braun, M., Weber, F., and Alt, F. (2021). Affective automotive user interfaces–reviewing the state of driver affect research and emotion regulation in the car. ACM Comput. Surv. (CSUR) 54 (7), 1–26. doi:10.1145/3460938
Bumgarner, D. J., Webb, J. R., and Dula, C. S. (2016). Forgiveness and adverse driving outcomes within the past five years: driving anger, driving anger expression, and aggressive driving behaviors as mediators. Transp. Res. Part F Traffic Psychol. Behav. 42 (2), 317–331. doi:10.1016/j.trf.2016.07.017
Cai, H., and Lin, Y. (2011). Modeling of operators' emotion and task performance in a virtual driving environment. Int. J. Human-Computer Stud. 69 (9), 571–586. doi:10.1016/j.ijhcs.2011.05.003
Charlton, S. G. (2009). Driving while conversing: cell phones that distract and passengers who react. Accid. Analysis and Prev. 41 (1), 160–173. doi:10.1016/j.aap.2008.10.006
Corzo, V., Rivera, M. A., Galvis, I., and Cespedes-Guevara, J. (2024). Regulating road rage: effects of cognitive reappraisal and distraction strategies. doi:10.2139/ssrn.4798036
Deffenbacher, J. L., Deffenbacher, D. M., Lynch, R. S., and Richards, T. L. (2003). Anger, aggression, and risky behavior: a comparison of high and low anger drivers. Behav. Res. Ther. 41 (6), 701–718. doi:10.1016/S0005-7967(02)00046-3
Deffenbacher, J. L., Huff, M. E., Lynch, R. S., Oetting, E. R., and Salvatore, N. F. (2000). Characteristics and treatment of high-anger drivers. J. Couns. Psychol. 47 (1), 5–17. doi:10.1037/0022-0167.47.1.5
Deffenbacher, J. L., Stephens, A. N., and Sullman, M. J. M. (2016). Driving anger as a psychological construct: twenty years of research using the Driving Anger Scale. Transp. Res. F Psychol. Behav. 42 (Part 2), 236–247. doi:10.1016/j.trf.2015.10.021
Dingus, T. A., Guo, F., Lee, S., Antin, J. F., Perez, M., Buchanan-King, M., et al. (2016). Driver crash risk factors and prevalence evaluation using naturalistic driving data. Proc. Natl. Acad. Sci. 113 (10), 2636–2641. doi:10.1073/pnas.1513271113
Dmitrenko, D., Maggioni, E., Brianza, G., Holthausen, B. E., Walker, B. N., and Obrist, M. (2020). “CARoma therapy: pleasant scents promote safer driving, better mood, and improved well-being in angry drivers,” in Proceedings of the 2020 CHI conference on human factors in computing systems (CHI’ 20) (New York, NY, USA: Association for Computing Machinery), 1–13. doi:10.1145/3313831.3376176
Dmitrenko, D., Maggioni, E., and Obrist, M. (2018). I smell trouble: using multiple scents to convey driving-relevant information, 234–238. doi:10.1145/3242969.3243015
Duany, J. M., and Mouloua, M. (2022). The role of trait mindfulness in aggressive driving behavior. Proc. Hum. Factors Ergonomics Soc. Annu. Meet. 66 (1), 968–971. doi:10.1177/1071181322661441
Dula, C. S., and Geller, E. S. (2003). Risky, aggressive, or emotional driving: addressing the need for consistent communication in research. J. Saf. Res. 34 (5), 559–566. doi:10.1016/j.jsr.2003.03.004
Ellison-Potter, P., Bell, P., and Deffenbacher, J. (2001). The effects of trait driving anger, anonymity, and aggressive stimuli on aggressive driving behavior. J. Appl. Soc. Psychol. 31 (2), 431–443. doi:10.1111/j.1559-1816.2001.tb00204.x
Emo, A. K., Matthews, G., Funke, G., and Warm, J. S. (2004). Stress vulnerability, coping and risk-taking behaviors during simulated driving. Proc. Hum. Factors Ergonomics Soc. Annu. Meet. 48 (11), 1228–1232. doi:10.1177/154193120404801105
Emo, A. K., Matthews, G., and Funke, G. J. (2016). The slow and the furious: anger, stress and risky passing in simulated traffic congestion. Transp. Res. Part F Traffic Psychol. Behav. 42, 1–14. doi:10.1016/j.trf.2016.05.002
Engstrom, J., Markkula, G., Victor, T., and Merat, N. (2017). Effects of cognitive load on driving performance: the cognitive control hypothesis. Hum. Factors 59, 734–764. doi:10.1177/0018720817690639
Eyben, F., Wöllmer, M., Poitschke, T., Schuller, B., Blaschke, C., Färber, B., et al. (2010). Emotion on the road—necessity, acceptance, and feasibility of affective computing in the car. Adv. Human-Computer Interact. 2010 (1), 1–17. doi:10.1155/2010/263593
Feng, Z., Zhan, J., Ma, C., Lei, Y., Liu, J., Zhang, W., et al. (2018). Is cognitive intervention or forgiveness intervention more effective for the reduction of driving anger in Chinese bus drivers? Transp. Res. Part F Psychol. Behav. 55, 101–113. doi:10.1016/j.trf.2018.02.039
Galovski, T. E., Blanchard, E. B., Malta, L. S., and Freidenberg, B. M. (2003). The psychophsiology of aggressive drivers: comparison to non-aggressive drivers and pre-to post-treatment change following a cognitive-behavioural treatment. Behav. Res. Ther. 41 (9), 1055–1067. doi:10.1016/S0005-7967(02)00242-5
Galovski, T. E., Malta, L. S., and Blanchard, E. B. (2006). Road Rage: assessment and treatment of the angry, aggressive driver. Washington, DC: American Psychological Association. doi:10.1037/11297-000
Garikapati, D., Poovalingam, S., Hau, W., De Castro, R., and Shinde, C. (2024). A comprehensive review of parallel autonomy systems within vehicles: applications, architectures, safety considerations and standards. IEEE Access, 1. doi:10.1109/access.2024.3430933
Goodwin, A., Thomas, L., Kirley, B., Hall, W., O’Brien, N., and Hill, K. (2015). Countermeasures that work: a highway safety countermeasure guide for state.
Gross, J. J. (1998). Sharpening the focus: emotion regulation, arousal, and social competence. Psychol. Inq. 9 (4), 287–290. doi:10.1207/s15327965pli0904_8
Gu, H., Liang, B., and Cao, H. (2024). User-centered framework for assessing the performance of smart car cockpits. Int. J. Adv. Manuf. Technol., 1–14. doi:10.1007/s00170-024-12994-1
Hancock, G. M., Hancock, P. A., and Janelle, C. M. (2012). The impact of emotions and predominant emotion regulation technique on driving performance. Work 41 (Suppl. 1), 3608–3611. doi:10.3233/wor-2012-0666-3608
Harris, H., and Nass, C. (2011). Emotion regulation for frustrating driving contexts. Proc. SIGCHI Conf. Hum. Factors Comput. Syst. doi:10.1145/1978942.1979050
Hennessy, D. A., and Wiesenthal, D. L. (2005). Driving vengeance and willful violations: clustering of problem driving attitudes. J. Appl. Soc. Psychol. 35, 61–79. doi:10.1111/j.1559-1816.2005.tb02093.x
Hernandez, J., McDuff, D., Benavides, X., Amores, J., Maes, P., and Picard, R. (2014). “AutoEmotive: bringing empathy to the driving experience to manage stress,” in Proceedings of the 2014 companion publication on Designing interactive systems, 53–56.
Inzlicht, M., Werner, K. M., Briskin, J. L., and Roberts, B. W. (2021). Integrating models of self-regulation. Annu. Rev. Psychol. 72 (1), 319–345. doi:10.1146/annurev-psych-061020-105721
Jeon, M., Yim, J. B., and Walker, B. N. (2011). “An angry driver is not the same as a fearful driver: effects of specific negative emotions on risk perception, driving performance, and workload,” in Proceedings of the 3rd international conference on automotive user interfaces and interactive vehicular applications, 137–142.
Kalokerinos, E. K., Résibois, M., Verduyn, P., and Kuppens, P. (2017). The temporal deployment of emotion regulation strategies during negative emotional episodes. Emotion 17 (3), 450–458. doi:10.1037/emo0000248
Kazemeini, T., Ghanbari-e-Hashem-Abadi, B., and Safarzadeh, A. (2013). Mindfulness based cognitive group therapy vs cognitive behavioral group therapy as a treatment for driving anger and aggression in Iranian taxi drivers. Psychology 04 (08), 638–644. doi:10.4236/psych.2013.48091
Kim, G., Hwang, S., Seong, M., Yeo, D., Rus, D., and Kim, S. (2024). TimelyTale: a multimodal dataset approach to assessing passengers' explanation demands in highly automated vehicles. Proc. ACM Interact. Mob. Wearable Ubiquitous Technol. 8 (3), 1–60. doi:10.1145/3678544
Kontogiannis, T. (2006). Patterns of driver stress and coping strategies in a Greek sample and their relationship to aberrant behaviors and traffic accidents. Accid. Analysis and Prev. 38 (5), 913–924. doi:10.1016/j.aap.2006.03.002
Koole, S. L. (2009). The psychology of emotion regulation: an integrative review. Cognition Emot. 23 (1), 4–41. doi:10.1080/02699930802619031
Kovácsová, N., Lajunen, T., and Rošková, E. (2016). Aggression on the road: relationships between dysfunctional impulsivity, forgiveness, negative emotions, and aggressive driving. Transp. Res. Part F Traffic Psychol. Behav. 42, 286–298. doi:10.1016/j.trf.2016.02.010
Kwak, S. H., Seo, S. H., Kim, H. S., Chung, S. C., and Min, B. C. (2015). 2C4-1 effects of sleep deprivation and the odor of 100% lavender oiland 100% peppermint oil on unexpected situation while driving. Jpn. J. ergonomics 51 (Suppl. ment), S500–S505. doi:10.5100/jje.51.s500
Lakshitha, M. S., and Padmakumari, P. (2023). Driving anger expression: the predictive role of forgiveness and empathy. Indian J. Posit. Psychol. 14 (3), 280–290.
Lennon, A., and Watson, B. (2012). Coping with aggressive driving: drivers account for how they manage themselves and others to reduce conflict on the road.
Li, W., Zhang, B., Wang, P., Sun, C., Zeng, G., Tang, Q., et al. (2021). Visual-attribute-based emotion regulation of angry driving behaviors. IEEE Intell. Transp. Syst. Mag. 14 (3), 10–28. doi:10.1109/mits.2021.3050890
Lohani, M., Cooper, J. M., Erickson, G., Simmons, T., McDonnell, A., Crabtree, K., et al. (2020). “Driver arousal and workload under partial vehicle automation: a pilot study,” in Proceedings of the human factors ergonomic society. IGI Global. doi:10.1177/1071181320641471
Lohani, M., Cooper, J. M., Erickson, G. G., Simmons, T. G., McDonnell, A. S., Carriero, A. E., et al. (2021). No difference in arousal or cognitive demands between manual and partially automated driving: a multi-method on-road study. Front. Neurosci. 15, 577418. doi:10.3389/fnins.2021.577418
Lohani, M., Cooper, J. M., McDonnell, A. S., Carriero, A. E., Simmons, T. G., Strayer, D. L., et al. (2024). Reliable but multi-dimensional cognitive demand in operating partially automated vehicles: implications for real-world automation research. Cogn. Res. 9, 60. doi:10.1186/s41235-024-00591-5
Lohani, M., Dutton, S., and Elsey, J. S. (2022). A day in the life of a college student during the COVID-19 pandemic: an experience sampling approach to emotion regulation. Appl. Psychol. Health Well-Being 14 (4), 1333–1352. doi:10.1111/aphw.12337
Lohani, M., Payne, B. R., and Isaacowitz, D. M. (2018). Emotional coherence in early and later adulthood during sadness reactivity and regulation. Emotion 18 (6), 789–804. doi:10.1037/emo0000345
Lohani, M., Payne, B. R., and Strayer, D. L. (2019). A review of psychophysiological measures to assess cognitive states in real-world driving. Front. Hum. Neurosci. 13, 57. doi:10.3389/fnhum.2019.00057
Lohani, M., Poitras, E. G., and Stokes, C. (2020). “A framework for human-technology social systems: the role of inter-personal interactions,” in Examining multiple intelligences and digital technologies for enhanced learning opportunities (IGI Global), 281–309. doi:10.4018/978-1-7998-0249-5.ch014
Lohani, M., Stokes, C., McCoy, M., Bailey, C. A., Joshi, A., and Rivers, S. E. (2016b). “Perceived role of physiological sensors impacts trust and reliance on robots,” in 2016 25th IEEE international symposium on robot and human interactive communication (RO-MAN) (IEEE), 513–518.
Lohani, M., Stokes, C., McCoy, M., Bailey, C. A., and Rivers, S. E. (2016a). “Social interaction moderates human-robot trust-reliance relationship and improves stress coping,” in 2016 11th ACM/IEEE international conference on human-robot interaction (HRI) (IEEE), 471–472.
Lohani, M., Stokes, C. K., Oden, K. B., Frazier, S. J., Landers, K. J., Craven, P. L., et al. (2017). “The impact of non-technical skills on trust and stress,” in Proceedings of the companion of the 2017 ACM/IEEE international conference on human-robot interaction, 191–192.
Ma, Y., Xing, Y., Chen, S., and Wu, Y. (2024). Investigating emotion fluctuations in driving behaviors of online car-hailing drivers using naturalistic driving data. Travel Behav. Soc. 36, 100819. doi:10.1016/j.tbs.2024.100819
Matthews, G. (2001). “A transactional model of driver stress,” in Stress, workload, and fatigue, 133–163.
Matthews, G. (2002). Towards a transactional ergonomics for driver stress and fatigue. Theor. Issues Ergonomics Sci. 3 (2), 195–211. doi:10.1080/14639220210124120
Matthews, G., Dorn, L., Hoyes, T. W., Davies, D. R., Glendon, A. I., and Taylor, R. G. (1998). Driver stress and performance on a driving simulator. J. Hum. Factors Ergonomics Soc. 40 (1), 136–149. doi:10.1518/001872098779480569
Mesken, J., Hagenzieker, M. P., Rothengatter, T., and De Waard, D. (2007). Frequency, determinants, and consequences of different drivers’ emotions: an on-the-road study using self-reports, (observed) behaviour, and physiology. Transp. Res. part F traffic Psychol. Behav. 10 (6), 458–475. doi:10.1016/j.trf.2007.05.001
Moore, M., and Dahlen, E. R. (2008). Forgiveness and consideration of future consequences in aggressive driving. Accid. Anal. Prev. 40 (5), 1661–1666. doi:10.1016/j.aap.2008.05.007
Moss, M., Ho, J., Swinburne, S., and Turner, A. (2023). Aroma of the essential oil of peppermint reduces aggressive driving behaviour in healthy adults. Hum. Psychopharmacol. Clin. Exp. 38 (2), e2865. doi:10.1002/hup.2865
Navon, M., and Taubman – Ben-Ari, O. (2019). Driven by emotions: the association between Emotion Regulation, forgivingness, and driving styles. Transp. Res. Part F Traffic Psychol. Behav. 65, 1–9. doi:10.1016/j.trf.2019.07.005
Oehl, M., Ihme, K., Bosch, E., Pape, A., Vukelić, M., and Braun, M. (2019). “Emotions in the age of automated driving - developing use cases for empathic cars,” in Message understanding conference.
Popuşoi, S., and &Holman, A. (2016). Driving anger and aggressive tendency: the moderating role of emotion regulation strategy. Bull. Transilvania Univ. Brasov. Ser. VII Soc. Sci. Law 9 (59), 2.
Rakauskas, M. E., Gugerty, L. J., and Ward, N. J. (2004). Effects of naturalistic cell phone conversations on driving performance. J. Saf. Res. 35 (4), 453–464. 2- s2.0-4944240999. doi:10.1016/j.jsr.2004.06.003
Regan, M. A., Lee, J. D., and Victor, T. (2017). Driver distraction and inattention: advances in research and countermeasures 1.
Roberton, T., Daffern, M., and Bucks, R. S. (2012). Emotion regulation and aggression. Aggress. Violent Behav. 17 (1), 72–82. doi:10.1016/j.avb.2011.09.006
Roidl, E., Frehse, B., and Höger, R. (2014). Emotional states of drivers and the impact on speed, acceleration and traffic violations—a simulator study. Accid. Analysis and Prev. 70, 282–292. doi:10.1016/j.aap.2014.04.010
Shamoa-Nir, L. (2023). Road rage and aggressive driving behaviors: the role of state-trait anxiety and coping strategies. Transp. Res. Interdiscip. Perspect. 18, 100780. doi:10.1016/j.trip.2023.100780
Shamoa-Nir, L., and Koslowsky, M. (2010). Aggression on the road as a function of stress, coping strategies and Driver Style. SSRN Electron. J. doi:10.2139/ssrn.4083010
Sheppes, G. (2020). “Transcending the “good and bad” and “here and now” in emotion regulation: costs and benefits of strategies across regulatory stages,” in Advances in experimental social psychology. Editor B. Gawronski, 185–236. doi:10.1016/bs.aesp.2019.09.003
Shukri, M., Jones, F., and Conner, M. (2022). Theory of planned behaviour, psychological stressors and intention to avoid violating traffic rules: a Multi-Level modelling analysis. Accid. Analysis and Prev. 169, 106624. doi:10.1016/j.aap.2022.106624
Stephens, A. N., Koppel, S., Young, K. L., Chambers, R., and Hassed, C. (2018). Associations between self-reported mindfulness, driving anger and aggressive driving. Transp. Res. Part F Traffic Psychol. Behav. 56, 149–155. doi:10.1016/j.trf.2018.04.011
Stokes, C. K., Lohani, M., DeCostanza, A. H., and Loh, E. (2023). Human–machine social systems: test and validation via military use cases. Handb. Human-Machine Syst., 71–82. doi:10.1002/9781119863663.ch7
Suhr, K. A., and Nesbit, S. M. (2013). Dwelling on “Road Rage”: the effects of trait rumination on aggressive driving. Transp. Res. Part F Psychol. Behav. 21, 207–218. doi:10.1016/J.TRF.2013.10.001
Suzuki, K., Yasuda, M., Sassa, Y., and Harada, S. (2006). Effects of aroma of trees on active safety during car driving. Trans. Jpn. Soc. Mech. Eng. Part C 72 (11), 3584–3592. doi:10.1299/kikaic.72.3584
Szalma, J. A., Hancock, P. A., and Quinn, S. (2008) “A meta-analysis of the effect of time pressure on human performance,”in Proceedings of the human factors and ergonomics society annual meeting, 52. Sage CA: Los Angeles, CA: SAGE Publications, 1513–1516. doi:10.1177/154193120805201944
Takaku, S. (2006). Reducing road rage: an application of the dissonance-attribution model of interpersonal forgiveness1. J. Appl. Soc. Psychol. 36 (10), 2362–2378. doi:10.1111/j.0021-9029.2006.00107.x
Tavakoli, A., Lai, N., Balali, V., and Heydarian, A. (2023). How are drivers’ stress levels and emotions associated with the driving context? A naturalistic study. J. Transp. and Health 31, 101649. doi:10.1016/j.jth.2023.101649
Tillmann, W. A., and Hobbs, G. E. (1949). The accident-prone automobile driver; a study of the psychiatric and social background. Am. J. Psychiatry 106 (5), 321–331. doi:10.1176/ajp.106.5.321
Venkatraman, V., Richard, C. M., Magee, K., and Johnson, K. (2021). Countermeasures that work: a highway safety countermeasures guide for State Highway Safety Offices. 10th edition. National Highway Traffic Safety Administration. doi:10.21949/1526021
Wan, P., Wu, C., Lin, Y., and Ma, X. (2017). On-road experimental study on driving anger identification model based on physiological features by ROC curve analysis. IET Intell. Transp. Syst. 11 (5), 290–298. doi:10.1049/iet-its.2016.0127
Wickens, C. (2021a). Attention: theory, principles, models and applications. Int. J. Human–Computer Interact. 37 (5), 403–417. doi:10.1080/10447318.2021.1874741
Wickens, C. D., Gordon, S. E., Liu, Y., and Lee, J. (2004). An introduction to human factors engineering, 2. Upper Saddle River, NJ: Pearson Prentice Hall.
Wickens, C. D., Helton, W. S., Hollands, J. G., and Banbury, S. (2021b). Engineering psychology and human performance. Routledge.
Wickens, C. M., and Wiesenthal, D. L. (2005). State driver stress as a function of occupational stress, traffic congestion, and trait stress susceptibility. J. Appl. Biobehav. Res. 10 (2), 83–97. doi:10.1111/j.1751-9861.2005.tb00005.x
Wiesenthal, D. L., Hennessy, D. A., and Totten, B. (2003). The influence of music on mild driver aggression. Transp. Res. Part F Psychol. Behav. 6 (2), 125–134. doi:10.1016/S1369-8478(03)00020-2
Wintersberger, P., Riener, A., and Frison, A. K. (2016). “Automated driving system, male, or female driver: who’d you prefer? Comparative analysis of passengers’ mental conditions, emotional states and qualitative feedback,” in Proceedings of the 8th international conference on automotive user interfaces and interactive vehicular applications (automotive’UI 16) (New York, NY, USA: Association for Computing Machinery), 51–58. doi:10.1145/3003715.3005410
Wu, L., Xue, J., Li, W., Wang, K., Zhang, X., and Guo, G. (2022). Toward decreasing the driving risk: speech-based driver’s anger regulation in smart cockpit. IEEE J. Radio Freq. Identif. 6, 764–768. doi:10.1109/jrfid.2022.3208199
Yan, L., Wan, P., Qin, L., and Zhu, D. (2018). The induction and detection method of angry driving: evidences from EEG and physiological signals. Discrete Dyn. Nat. Soc. 2018 (3), 1–16. doi:10.1155/2018/3702795
Yang, S., McKerral, A., Mulhall, M. D., Lenné, M. G., Reimer, B., and Gershon, P. (2023). “Takeover context matters: characterising context of takeovers in naturalistic driving using super cruise and autopilot,” in Proceedings of the 15th international conference on automotive user interfaces and interactive vehicular applications, 112–122.
Yerkes, R. M., and Dodson, J. D. (1908). The relation of strength of stimulus to rapidity of habit-formation. J. Comp. Neurol. Psychol. 18 (5), 459–482. doi:10.1002/cne.920180503
Zhang, T., and Chan, A. H. S. (2016). The association between driving anger and driving outcomes: a meta-analysis of evidence from the past twenty years. Accid. Analysis and Prev. 90, 50–62. doi:10.1016/j.aap.2016.02.009
Keywords: emotion regulation, affective automotive user interfaces, evidence-based interventions, future of automation, driving performance, health
Citation: Lohani M, Do AS and Aisa A (2024) Design considerations for future affective automotive interfaces: a review of self-regulation strategies to manage affect behind the wheel. Front. Future Transp. 5:1458828. doi: 10.3389/ffutr.2024.1458828
Received: 03 July 2024; Accepted: 27 September 2024;
Published: 28 October 2024.
Edited by:
Mariaelena Tagliabue, University of Padua, ItalyReviewed by:
Elisabetta Patron, University of Padua, ItalyCopyright © 2024 Lohani, Do and Aisa. This is an open-access article distributed under the terms of the Creative Commons Attribution License (CC BY). The use, distribution or reproduction in other forums is permitted, provided the original author(s) and the copyright owner(s) are credited and that the original publication in this journal is cited, in accordance with accepted academic practice. No use, distribution or reproduction is permitted which does not comply with these terms.
*Correspondence: Monika Lohani, bW9uaWthLmxvaGFuaUB1dGFoLmVkdQ==
Disclaimer: All claims expressed in this article are solely those of the authors and do not necessarily represent those of their affiliated organizations, or those of the publisher, the editors and the reviewers. Any product that may be evaluated in this article or claim that may be made by its manufacturer is not guaranteed or endorsed by the publisher.
Research integrity at Frontiers
Learn more about the work of our research integrity team to safeguard the quality of each article we publish.