- 1Institute of Human and Industrial Engineering, Karlsruhe Institute of Technology, Karlsruhe, Germany
- 2Fraunhofer Institute of Optronics, System Technologies und Image Exploitation, Karlsruhe, Germany
Introduction: To avoid the uncertainty present in a cooperation between manual vehicles (MVs) and automated vehicles (AVs), cooperative connected automated mobility (CCAM) is frequently proposed. In this contribution, we consider the situation of a motorway slip road where an AV (SAE level 3 or higher) on the motorway cooperates by opening a suitable gap for an entering AV. To ensure a meeting of the cooperation partners, speed adjustment by the system might often be necessary.
Methods: To investigate the acceptance of an automated cooperative motorway access assistant a driving simulator study (N = 41) was conducted. Participants drove six laps on a virtual test track in a level 3 AV. Three levels of speed adjustment and two levels of an HMI (with or without HMI) were tested. They experienced the automated cooperative motorway access assistant in two situations. Firstly, when they entered the motorway themselves and benefited from the system, and secondly, when the system reduced their vehicle's speed to allow for cooperation with another vehicle on a motorway slip road ahead.
Results: The results show that the acceptance of the assistant depends significantly on the magnitude of speed reduction: the greater the speed adjustment, the worse the user rating, and the more often the cooperation was terminated. Obviously, the acceptance depends on the perspective: If the users themselves benefit from the speed adjustment of others, they rate the system significantly better than if they themselves must accept the restriction. Only in the case of a small speed reduction, the assistant is rated as well as if the user benefits from the system. The availability of a human-machine interface (HMI) providing information about the system status influenced acceptance only marginally but had a significant influence on the trust in the automation.
Discussion: The results suggest that people are willing to accept a personal disadvantage in order to give others an advantage in the context of CCAM. However, acceptance depends on the magnitude of the disadvantage. The overall agreement scores of the system with HMI did not differ from those without HMI, although the HMI was found to slightly reduce the termination of cooperation. Three possible reasons for this result, which differs from previous research, are discussed.
1 Introduction
Motorway access is considered a critical situation for manual vehicles. It is identified as one of the key causes of traffic jams on German motorways (Treiber and Kesting, 2010). For automated vehicles, this situation is even more demanding. This is partly because interaction with human partners proves to be difficult. There is a comprehensive body of research on human behavior at motorway slip roads, for example, on slip road speed (Choudhury et al., 2009; Kondyli and Elefteriadou, 2010; van Beinum et al., 2018) or gap acceptance (Choudhury et al., 2009; Marczak et al., 2013). However, those papers show above all that the safety distance is frequently undercut (Daamen et al., 2010; Kusuma et al., 2015; van Beinum et al., 2018) and ramping drivers force merging to the target lane (Choudhury et al., 2009). While human drivers usually accept this to enable merging, the legal situation does not allow it for automated vehicles (Dolianitis et al., 2019).
A promising approach to solve this problem is connected cooperative driving. It avoids cooperation with human partners. Instead, other automated vehicles open a sufficient gap for safe and legally compliant merging. The effectiveness of such a system was shown by Rios-Torres and Malikopoulos (2017) in a simulation study. They report a reduction in fuel consumption and travel time as well as the prevention of congestion and accidents. However, a coincidental encounter between two automated vehicles in the short time window of an on-ramp to the motorway is unlikely with low penetration rates. The meeting must be arranged. Therefore, a speed adjustment of both cooperating partners is needed, including the non-benefiting partner (the one already on the motorway). Our contribution deals with the question of how to design such a connected cooperative motorway access assistant to ensure that both partners accept the required speed adjustments. The questions investigated by our study are:
• What is the maximum acceptable speed reduction while cooperating?
• Does an HMI have an influence on the maximum accepted speed reduction?
Various contributions demonstrate the benefits of connected cooperative driving in different scenarios. As described in the introduction, Rios-Torres and Malikopoulos (2017) showed in a simulation that by implementing cooperative connected vehicle coordination at motorway slip roads, both fuel consumption and travel time can be reduced significantly, while congestion and collisions are avoided. Zheng et al. (2020) used a cooperative strategy for connected and automated vehicles to optimize traffic safety and traffic flow at lane changes on motorways. Further analyses showed a positive influence of connected vehicles on the stability of the traffic flow as well as on the throughput by preventing the formation of shock waves and their propagation (Talebpour and Mahmassani, 2016). Calvert et al. (2017) argue that mere connectivity will initially have a negative impact on traffic flow, as the required time gaps of automated vehicles are higher. Only an increasing penetration rate of cooperative AVs can reverse this negative effect. As a limitation, these approaches have in common that the results have been obtained exclusively from simulations and disregard human factors such as user acceptance and trust.
Arguably, the most well-known acceptance model is the Technology Acceptance Model (TAM) by Davis (1989) and its further developments. It assumes that the “Attitude Toward Using” is determined by two variables: the “Perceived Usefulness,” i.e., the assessment of whether a technology is useful for the user, and the “Perceived Ease of Use,” i.e., whether the technology is easily useable. A positive assessment of these factors leads to the user’s intention to use the technology. Conversely, a lack of acceptance leads to a system not being used, which in terms of automated driving means that the intended increase in road safety is no longer given. A concept closely related to acceptance is trust (Kaur and Rampersad, 2018; Lee and See, 2004). Trust in the context of human-machine interaction is defined by as “the attitude that an agent will help achieve an individual’s goals in a situation characterized by uncertainty and vulnerability” (Lee and See, 2004). Trust is also correlated with the frequency of use of an automated driving system (Dikmen and Burns, 2017).
Fank et al. (2021) highlighted the importance of an HMI for user acceptance of a cooperative system. They developed a user interface for cooperative truck overtaking and evaluated it in a driving simulator study with thirty truck drivers. They were able to show that drivers were willing to use a cooperative system, particularly, if they are provided with a suitable HMI. They concluded that the success of a cooperative system depends on driver acceptance and trust towards the system. The influence of an HMI on acceptance and trust in the automation was also investigated by Forster et al. (2017). They compared the effect of a non-specific warning tone with a semantic speech output during the execution of different automatic driving maneuvers in a driving simulator. The results showed that describing an automatic function appropriately to the driver increases their trust in automation as well as acceptance of the system. Haar et al. (2022) considered lane changes on motorways in a driving simulation study with N = 52 participants. They investigated how the willingness to cooperate can be supported by means of vehicle-to-vehicle communication. The results showed that a head-up display providing additional information about the cooperation partners’ intentions supplementary to the regular turn signal might be beneficial. Their approach appeared to lead to behavior that is more permissive, makes drivers perceive other drivers as more cooperative and reduces the overall ambiguity in the lane change situation.
The approach we propose in the present contribution demands drivers to selflessly accept a speed reduction to allow others to enter the motorway safely and comfortably and to trust the systems speed adaption decisions. A similar approach was taken by Reinolsmann et al. (2021). They developed an active gap metering signalization system that indicates drivers in the right lane to actively reduce their speed and adjust their headway so that vehicles coming from the driveway can merge safely. In a driving simulator study with 64 participants, they showed that the safety distances were significantly improved with the help of the signalizations. The idea that people in road traffic are not solely motivated by their own advantage, but also show selfless behavior, was already considered by Kesting et al. (2007). Their lane change model presents the decision to change lanes as a trade-off between the expected own advantage and the disadvantage imposed on other drivers when changing lanes. The politeness parameter included in their model allows the motivation for the lane change to vary from purely selfish to selfless. However, to the authors’ knowledge, there are no studies on user acceptance of a system that reduces speed below the speed limit over a longer period for altruistic reasons.
The literature, thought, provides evidence that allows the generation of hypotheses: As shown earlier, according to the TAM, acceptance is determined by, i.a., “Perceived Usefulness” (Davis, 1989). Accordingly, a system that significantly reduces the speed without the user deriving any personal benefit should not be accepted, or acceptance should at least be significantly higher if a personal benefit is present. In further development of the model (TAM2; Venkatesh and Davis, 2000), however, the factor “Subjective Norm” was added, among others. One social norm in road traffic is that of mutual consideration. The Kesting et al. (2007) lane change model also shows that social factors such as “politeness” have an influence on our decisions in road traffic. Thus, there appears to be a trade-off between personal benefit (or the reduction of benefit by the amount of speed reduction) and the benefit of others. One can assume that this trade-off is more likely to be due to the disadvantage of the acceptance of the system with a stronger personal restriction (i.e., stronger speed reduction). In addition, the research literature shows a positive effect of an HMI on acceptance and trust in automated systems. From those findings, the following hypotheses were derived:
H1. The degree of acceptance of the system depends on the speed during the cooperation phase. Acceptance decreases the more the automation decreases the speed during the cooperation phase.
H2. The degree of acceptance depends on the user’s perspective. Acceptance is higher when the user benefits from the cooperation when they are on the slip road than when they are on the highway and not benefitting.
H3. The degree of acceptance depends on the HMI. The availability of an HMI leads to higher user acceptance. It also moderates the effect of speed on acceptance: The HMI can reduce the negative influence of reduced speed on acceptance.
2 Methods
To address these hypotheses, a user study in a driving simulator was conducted. On a simulated test track, the participants experienced the Automated Motorway Access Assistant System from both viewpoints: Entering the motorway as well as driving on the motorway and letting someone else enter. In both cases, the coordination partner was a scripted car. The speed of the ego vehicle changed in a randomized pattern while cooperating on the highway to simulate the required adjustments for the cooperation to succeed. Both HMI and randomized speeds were varied in multiple laps on the circuit. Figure 1 shows an overview of the track.
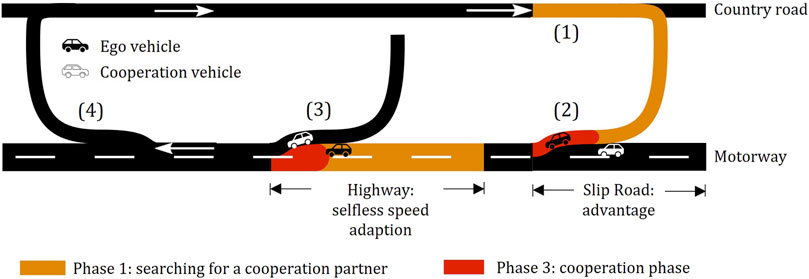
Figure 1. Overview of the study concept and track. Test participants (black) experience the cooperative motorway assistant on a circuit starting on a country road (1), followed by a motorway on-ramp (2), a stretch on the motorway where another on-ramp is passed (3) and an exit (4), after which the turn is made back onto the country road (1). Cooperation phases are marked in orange (phase 1: searching for a cooperation partner) and red (phase 3: cooperation phase). On the motorway, test participants are confronted with varying speed changes to simulate the control loop necessary to meet the cooperation partner (white) at the right time.
2.1 Independent and dependent variables
Three independent variables are examined in this experiment. The study design is a within-subject design (2 × 3 × 2), allowing all subjects to perform with all independent variables and their combinations. The first is “availability of an HMI,” with the two levels “with HMI” and “without HMI,” respectively. The second is “speed during the cooperation phase” with the three levels “70–90 km/h,” “90–110 km/h,” and “110–130 km/h.” Speed fluctuates in the indicated ranges simulating a realistic adaptation to the speed of the cooperating partner. A speed limit of 130 km/h was applied in the relevant section of the motorway. Thirdly, the driver’s perspective is evaluated: The subjects experienced the system both from the perspective of the vehicle on the slip road and as a cooperating partner in the right lane. The condition order of the availability of an HMI was randomized: Every second participant started with a block of three laps (according to the three levels of the speed condition) with HMI, the other half without HMI. The variable “speed during the cooperation phase” was thoroughly randomized within the HMI condition. The perspective is conditioned by the track: subjects experience the two perspectives alternately, starting with their own slip road.
The dependent variables are acceptance, trust, and perceived safety. Acceptance is operationalized by two means: Firstly, the subjects were instructed to terminate the cooperation by taking over control if they did not accept the systems decisions. Standardized questionnaires for measuring technology acceptance like the TAM (Davis, 1989) usually consist of several items. This serves to capture different aspects of acceptance and to increase reliability. In the present study, however, acceptance is surveyed while driving, which makes it impossible to answer several items for reasons of time and distraction. Therefore, acceptance was measured through a one-item questionnaire, which is asked after each cooperation situation. A machine voice asked how satisfied they were with the cooperation. Subjects answered the question on a 5-point scale ranging from “very unsatisfied” to “very satisfied” on the touch display in the center console.
Trust is captured by a “Trust in Automated Systems Questionnaire” (Pöhler et al., 2016). Perceived safety is captured by the Godspeed V (Bartneck et al., 2009). The variables were rated on a scale from 1 (very low) to 6 (very high). Finally, users were asked whether they preferred the version with HMI or without HMI. If they took over control during the experiment, they were also asked to state the reason in an open question.
2.2 Apparatus
The driving simulator consists of an Audi A3 with full functionality of all inputs such as steering wheel, gearshift, and pedals (see Figure 2). The car is surrounded by three screens onto which high-resolution projectors cast projections of the simulated driving environment. The exterior mirrors are equipped with displays, and there is a large screen behind the vehicle for a realistic impression when looking in the rear-view mirror. The driving simulation is realized using the simulation software SILAB® 6.5.
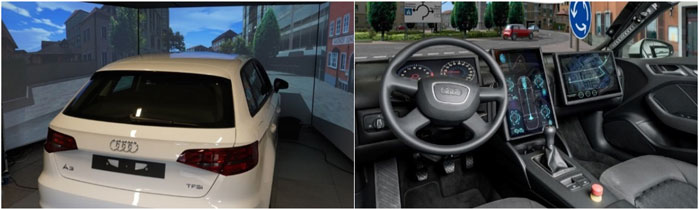
Figure 2. Driving Simulator frontal projection screens (left) and interior of the simulator vehicle (right).
The system allows switching between manual control and autonomous driving mode. A user initiates takeover from the autonomous mode by taking over the steering wheel or using the pedals. To give control back to the autonomous mode, the user pushes a button in the center console. An icon in the instrument cluster indicates the autonomous driving mode to the user.
2.3 Experimental system
The HMI of the Automated Motorway Access Assistant System was designed as follows. It consists of two main elements: An animated icon, and an audio signal. The assistant system covers four phases: 1) Looking for a cooperation partner, 2) finding a cooperation partner, 3) the cooperation period itself and 4) the end of the cooperation. When the cooperation is terminated by the driver the HMI disappears. Table 1 shows the representation of the two elements (icon and signal) in each phase. The turquoise color chosen for the animation of the icons regards previous work recommending this color for the display of automated driving (Faas and Baumann, 2019; Werner, 2019). The HMI was identical for either perspective - both when merging onto the motorway themselves, and while driving on the motorway when cooperation with a simulated cooperation partner was arranged. The HMI was displayed in the digital instrument cluster between the speedometer and rev counter. Figure 2 shows a picture of the basic configuration of the vehicle. For the study, the display of the speedometer and rev counter was reduced in size so that these two displays and the HMI each took up about a third of the screen. The HMI was developed in an iterative process to optimize comprehensibility. In the follow-up survey, the comprehensibility of the system was rated on a scale from 1 (not comprehensible at all) to 7 (fully comprehensible) with M = 6.00 (SD = 0.99).
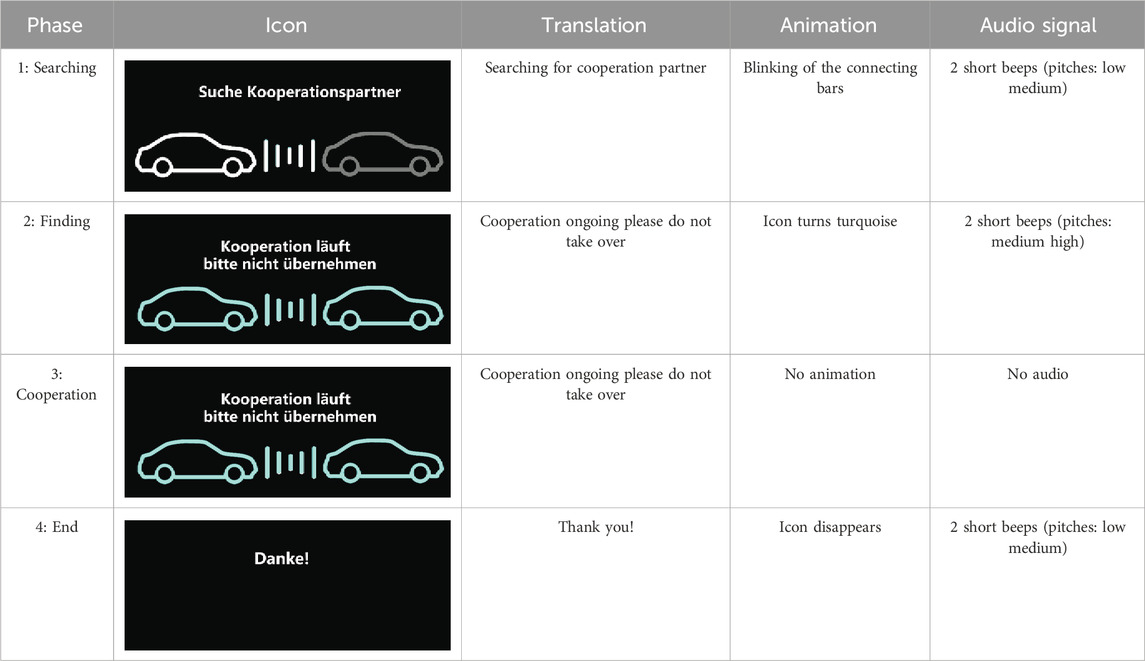
Table 1. The four phases of the automated motorway access assistant system with the respective HMI elements.
2.4 Test task
In order to test the hypotheses, a driving simulation test task was designed and implemented. It consists of a circuit (Figure 1), starting on a country road (1), followed by a motorway on-ramp (2), a stretch on the motorway where another on-ramp is passed (3) and an exit (4), after which the turn is made back onto the country road (1). In each lap, the subjects experience two cooperation phases. The first while entering the motorway themselves; the second while passing the second slip road. The motorway contains three lanes in each direction with a speed limit of 130 km/h indicated by signs. Other vehicles were simulated on all lanes, creating an average traffic density. The distance between the cooperation partners vehicles was identical in all situations.
During cooperation, either a human-machine interface (HMI) is presented to the test persons (see Section 2.3), or they go through the cooperation scenario without an HMI. While driving on the motorway during the second cooperation phase, the automated system reduces the speed of the vehicle to either 110–130, 90–110, or 70–90 km/h, respectively. Each speed level occurs for two laps, one with and one without HMI. This way, the speed reduction simulates the necessary speed adjustment which guarantees that the two cooperating vehicles would meet at the slip road.
2.5 Procedure
41 test persons (Mean age = 28.05, SD = 10.02; mean years of holding a driving license = 9.78, SD = 8.77; 29% female) participated. They were recruited via two distribution lists of our research institutes or via walk-in acquisition and were paid 15 Euro compensation each. The procedure started with a pre-survey in which the subjects filled in demographic data and the Godspeed questionnaire (Bartneck et al., 2009) on the topic of perceived safety. This was used to record the baseline of the current general perception of safety. After that, an introduction to the driving simulator followed.
In a training phase, the subjects drove manually on a country road, which was not part of the simulated test track, to get used to the simulation environment as well as the control of the vehicle. They also trained handover scenarios between manual control and an automated driving mode. After familiarization, the subjects were informed about the aim of the study, and the functionality of the Automated Motorway Access Assistant System was introduced. In order not to confound the condition “no HMI first,” the HMI was not mentioned in the instruction. Rather, it was referred to as “two versions of the assistant” that were to be compared. In addition, subjects were instructed to take control if they felt unsafe or did not like the behavior of their vehicle, but after a short time to return control to the automation so that the experiment could continue.
After the instruction, the subjects performed the test phase where they drove six laps on the circuit. They were assigned randomly to drive the block of three laps with or without HMI first. Within the blocks, the order of the speed levels was also randomized between the test persons. This results in an order randomization of the HMI variable and a complete randomization of the speed level variable. The driver’s perspective is not randomized in this design, as the participants always drive the same lap, starting with their own slip road.
After each cooperation, the subjects were asked to rate their acceptance, as stated in Section 2.1. After three laps, they came to a stop at a stop sign and were asked to rate their perceived control and their trust in the system (c.f. 2.1 Independent and dependent variables). After the last lap, the test persons completed the post questionnaire. It consisted of questions about the subjects’ understanding and preference of HMI components. Also, they again rated their trust in the system as well as their system acceptance and retook the Godspeed questionnaire on perceived safety. Finally, they rated the system with HMI and the system without HMI on a scale from 1 (do not like it at all) to 10 (do like it very much) and named which version of the system they preferred (with or without HMI). Each user session lasted approximately 1.5 h.
3 Results
3.1 H1: speed during the cooperation phase
To answer hypothesis 1, the influence of speed reduction on the two operationalization’s of acceptance was analyzed. Only the cooperation situations while driving on the motorway were included in the analysis, as the change in speed only occurred here. Please note that the inclusion of data from the other perspective still led to significant results in the main effect as well as the contrasts. An ANOVA with repeated measures shows a significant influence of the speed level on the rating of acceptance in the questionnaire (F (2, 80) = 35.08, p < 0.001, ηp2 = 0.47). The contrast analysis between the speed levels “70–90 km/h” and “90–110 km/h” (F (1, 40) = 23.16, p < 0.001, ηp2 = 0.37) as well as “90–110 km/h” and “110–130 km/h” (F (1, 40) = 14.28, p < 0.001, ηp2 = 0.26) are significant and show a higher acceptance the lower the speed reduction (see Figure 3).
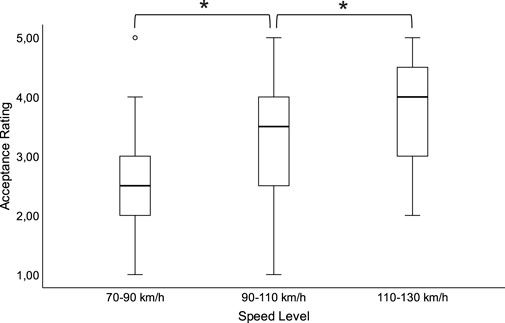
Figure 3. Acceptance rating of the three speed intervals. Error bars represent 95% confidence interval. * Significant difference within speed level, p < 0.001.
The analysis of the number of cooperation terminations was carried out using the McNemar test (McNemar, 1947), with pooled data over both HMI conditions and perspectives. The second operationalization of acceptance also shows a significant influence of the speed level: At speed level “110–130 km/h,” the cooperation is interrupted only 3 times, at speed level “90–110 km/h” significantly more often with 15 times (p = 0.006). At the highest speed reduction (“70–110 km/h”), however, the number of terminations (19) no longer increases significantly (p = 0.687, see Figure 4).
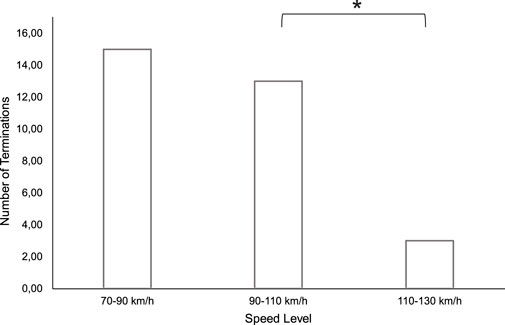
Figure 4. Number of cooperation terminations during the cooperation phase on the motorway perspective. Error bars represent 95% confidence interval. * Significant difference within speed level, p = 0.006.
3.2 H2: perspective
Hypothesis 2 deals with the influence of perspective (slip road vs. motorway) and thus the personal benefit from cooperation. For this purpose, the “perspective” factor was included in the analysis, resulting in a two-way ANOVA with repeated measures. The perspective has a significant influence on the acceptance rating: F (1, 40) = 28.86, p < 0.001, ηp2 = 0.42. The rating is significantly more positive if no personal restrictions in the form of a speed reduction had to be accepted (see Figure 5). The interaction term of speed level and perspective is also significant: F (2, 80) = 25.82, p < 0.001, ηp2 = 0.39. Analysis of within-subject contrasts shows the following: Only in the case of the lowest restriction (speed level “110–130 km/h”), the acceptance rating does not differ significantly between the two perspectives (t (40) = 1.20, p = 0.12). In the other two conditions, the system was rated significantly worse when subjects drove on the motorway, ergo did not benefit themselves: t (40) = 3.94, p < 0.001 for speed level “90–110 km/h” and t (40) = 7.56, p < 0.001 for speed level “70–90 km/h.”
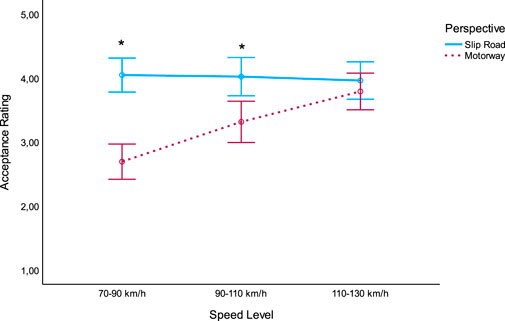
Figure 5. Acceptance rating of the three speed intervals for both perspectives. Error bars represent 95% confidence interval. * Significant difference within speed level, p < 0.001.
Similarly, the number of cooperation terminations depends on the perspective: While driving onto the motorway, the cooperation was terminated 3 times, while driving on the motorway 37 times (p < 0.001).
3.3 H3: HMI
The third hypothesis regards the influence of an HMI on the acceptance of the system. The factor HMI is included in the ANOVA. Contrary to the assumption, the HMI does not lead to a significantly different acceptance rating in the questionnaire: F (1, 40) = 0.002, p = 0.967, ηp2 = 0.00. The interaction terms with the other variables also show no significant interaction effects (“Speed Level”: F (2, 80) = 0.38, p = 0.686, ηp2 = 0.01; “Perspective”: F (1, 40) = 0.43, p = 0.515, ηp2 = 0.01). However, the McNemar test reveals a significant effect of the HMI on the number of terminations at speed level “90–100 km/h” (p = 0.006). For the other two speed levels, no significant effect was found (see Table 2).
To rule out the possibility that the results of the number of terminations were due to a carry-over effect from the previous condition, a further analysis was carried out. In the style of a between-subject-design, the differences in the first block of the experiment between the two HMI conditions were analyzed. Similarly, this analysis only shows a significant influence of the HMI on the number of terminations for the speed level “90–100 km/h” (p = 0.039).
3.4 Further analyses
Due to the lack of hypotheses, the analyses in this chapter are α-error corrected. The interim survey after the first three laps showed the following results. Perceived safety is independent of the availability of the HMI (z (23) = −0.53, p = 0.598). However, the HMI has a significant influence on trust in the automation. Trust is significantly higher with HMI (t (40) = −3.38, p < 0.001), and distrust is significantly lower (t (40) = 5.02, p < 0.001).
19 of the 41 subjects (46.3%) took over vehicle control at least once during the cooperation phases. The most frequently mentioned reasons in an open question format were “ego vehicle too slow” (12 mentions), “too little distance to other vehicles” (5), “behavior of the ego vehicle not comprehensible” (2) and “abrupt braking or acceleration” (2).
The motorway access assistant with HMI is rated significantly better (t (40) = −9.26, p < 0.001) in the post-questionnaire. The visual cooperation symbol is rated as the most helpful component of the HMI (M = 4.39, SD = 0.89), followed by the text (M = 4.17, SD = 1.07) and the sound effect (M = 3.88, SD = 1.27). Moreover, three other suggestions for improving the HMI were rated on a scale from “1 = not at all helpful” to “5 = very helpful.” The option “reason for vehicle speed adjustment” is considered most helpful (M = 4.00, SD = 1.31), followed by “position of cooperation partner” on a map (M = 3.57, SD = 1.33) and “speed of cooperation partner” (M = 3.38, SD = 1.25). In an open question, five people additionally expressed the demand for more information for identifying the cooperation partner, and four people expressed the demand for a countdown to the completion of the cooperation phase.
4 Discussion
The presented study shows that the acceptance of the cooperative connected motorway slip road assistant depends on the extent of the speed restrictions that are required to ensure the function of the assistant. Only at the lowest speed restriction (speed level “110–130 km/h”) the acceptance of the test persons was as high as at their own motorway entrance when they benefit from the system themselves. This is also reflected in the number of interventions in the system: The greater the reduction in speed, the more frequently cooperation is terminated. In contrast, during the driver’s own joining of the motorway, control is only very rarely taken over to override the assistant. Contrary to the hypothesis, the HMI has only a very small influence on the acceptance of the assistant: The rating in the questionnaire does not differ on any speed interval. However, in the intermediate speed interval (“90–110 km/h”), the HMI reduces the number of cancellations. Such a tendency is also evident for the slowest interval (“70–90 km/h”). Both with and without HMI, cooperation is rarely terminated at 110–130 km/h.
Therefore, the first hypothesis can be considered confirmed: Acceptance decreases with the reduction of speed during the cooperation phase. The second hypothesis is also accepted, acceptance is higher when users benefit from the assistant. The assistant is evaluated not worse on the motorway compared to during the slip road only at the lowest speed restriction. The third hypothesis is largely not confirmed: The HMI does not lead to a better acceptance rating and does not moderate the effect of speed on acceptance. Only at one speed level (90–110 km/h), the HMI can reduce the intervention in the system. However, it was shown that the availability of an HMI can increase the trust in an automated system.
Similar to the finding in the research literature (Kesting et al., 2007; Reinolsmann et al., 2021), subjects in the present study show partly selfless behavior to increase the safety and comfort of others. Although the assistant is rated better when the subjects themselves benefit from it, overall cooperation is rarely terminated. Out of 246 laps in which cooperation could have been terminated in favor of faster driving, subjects did so only 40 times.
However, contrary to what is inferred from the literature (Fank et al., 2021; Forster et al., 2017; Haar et al., 2022), an HMI had no significant influence on the systems acceptance. The HMI only led to less frequent interruptions of the cooperation in the medium speed interval (“90–110 km/h”). There may be several reasons for this. Firstly, it is possible that the HMI used in the study provided too little or not the relevant information for the test persons; hence, they might not have paid attention to it. Another potential reason is that the test persons relied so much on the automated vehicle control that they did not need any further information to accept the system. Thirdly, the HMI may have played a much smaller role for the subjects than the speed reduction of the vehicle. The question about the acceptance of the system may, therefore, have been based exclusively on speed. This is also supported by the fact that the HMI had a significant influence on the trust in the system. This result is consistent with (Forster et al., 2017), who found the availability of an informative HMI to be an important factor for trust and acceptance in the automated system. Beyond the existing literature, the study provides data on the acceptance of variable speed reduction to enable a cooperative motorway access.
The study is inherently subject to some limitations. Even though the validity of driving simulators has been repeatedly confirmed in the literature (e.g., Godley et al., 2002; Shechtman et al., 2009), the generalizability of the results is constrained. A naturalistic driving study should be carried out at a later stage of the system development to evaluate the validity of the results. The lack of actual time pressure from the experimental scenario may also have had an impact on the subjects’ patience and thus their willingness to engage in altruistic behavior. In addition, only the initial contact with the system was investigated in the approximately one-hour driving time. Future research should investigate how acceptance develops after a period of greater familiarization and how the relevance of the HMI develops, when the user is familiar with the system. Furthermore, only one draft of an HMI was used. It should be further developed in the future with the help of the user feedback collected in this study.
5 Conclusion
We presented a study on an Automated Motorway Access Assistant System. The focus of the study was the acceptance of speed changes to facilitate the cooperation as well as the influence of an HMI on the acceptance of the assistance system overall. The data provides evidence that users accept a connected cooperative system even if it does not act solely for their own benefit. The acceptance was highest when drivers benefited themselves by joining the motorway unrestrictedly. Similar acceptance could only be achieved for small restrictions when driving on the motorway letting others join cooperatively. Larger speed reductions facilitating the cooperation led to decreased acceptance of the system. While an HMI does not increase the reported acceptance, it can increase tolerance for moderate restrictions, and it also increases trust in the system. The results are promising, and they provide a solid base for further development of such a system that can improve safety and comfort at motorway slip roads.
Data availability statement
The datasets presented in this article are not readily available because participants did not consent to share the data in the publication process. Further inquiries can be directed to the corresponding author.
Ethics statement
The studies involving humans were approved by Ethics Committee of Karlsruhe Institute of Technology. The studies were conducted in accordance with the local legislation and institutional requirements. The participants provided their written informed consent to participate in this study.
Author contributions
SE: Conceptualization, Data curation, Formal Analysis, Investigation, Methodology, Visualization, Writing–original draft. MM: Conceptualization, Software, Writing–review and editing. JH: Conceptualization, Writing–review and editing. BD: Funding acquisition, Resources, Supervision, Writing–review and editing.
Funding
The author(s) declare that financial support was received for the research, authorship, and/or publication of this article. This research was funded by the project “Profilregion Mobilitätssysteme Karlsruhe”, which is funded by the Ministry of Economic Affairs, Labour and Housing in Baden-Württemberg and as a national High Performance Center by the Fraunhofer-Gesellschaft.
Acknowledgments
Special thanks go to Yang Li who contributed to the design of the HMI, as well as to Marco Wetter and Marco Pattke who contributed to the implementation and survey of the study.
Conflict of interest
The authors declare that the research was conducted in the absence of any commercial or financial relationships that could be construed as a potential conflict of interest.
Publisher’s note
All claims expressed in this article are solely those of the authors and do not necessarily represent those of their affiliated organizations, or those of the publisher, the editors and the reviewers. Any product that may be evaluated in this article, or claim that may be made by its manufacturer, is not guaranteed or endorsed by the publisher.
References
Bartneck, C., Kulić, D., Croft, E., and Zoghbi, S. (2009). Measurement instruments for the anthropomorphism, animacy, likeability, perceived intelligence, and perceived safety of robots. Int. J. Soc. Robotics 1 (1), 71–81. doi:10.1007/s12369-008-0001-3
Calvert, S. C., Schakel, W. J., and van Lint, J. W. C. (2017). Will automated vehicles negatively impact traffic flow?. J. Adv. Transp. 2017, 1–17. doi:10.1155/2017/3082781
Choudhury, C. F., Ramanujam, V., and Ben-Akiva, M. E. (2009). Modeling acceleration decisions for freeway merges. Transp. Res. Rec. J. Transp. Res. Board 2124 (1), 45–57. doi:10.3141/2124-05
Daamen, W., Loot, M., and Hoogendoorn, S. P. (2010). Empirical analysis of merging behavior at freeway on-ramp. Transp. Res. Rec. J. Transp. Res. Board 2188 (1), 108–118. doi:10.3141/2188-12
Davis, F. D. (1989). Perceived usefulness, perceived ease of use, and user acceptance of information technology. MIS Q. 13 (3), 319. doi:10.2307/249008
Dikmen, M., and Burns, C. (2017). “Trust in autonomous vehicles: the case of tesla autopilot and summon,” in 2017 IEEE International Conference on Systems, Man, and Cybernetics (SMC), Banff, AB, October 05–08, 2017 (IEEE). doi:10.1109/smc.2017.8122757
Dolianitis, A., Chalkiadakis, C., Mylonas, C., and Tzanis, D. (2019). “How will autonomous vehicles operate in an unlawful environment? - the potential of autonomous vehicles for disregarding the law,” in 6th International Conference on Models and Technologies for Intelligent Transportation Systems (MT-ITS), Cracow, Poland, June 05–07, 2019, 1–10. doi:10.1109/mtits.2019.8883326
Faas, S. M., and Baumann, M. (2019). Light-based external human machine interface: color evaluation for self-driving vehicle and pedestrian interaction. Proc Hum Factors Ergon Soc Annu Meet, 63 (1), 1232–1236. doi:10.1177/1071181319631049
Fank, J., Knies, C., and Diermeyer, F. (2021). “After you! Design and evaluation of a human machine interface for cooperative truck overtaking maneuvers on freeways,” in 13th International Conference on Automotive User Interfaces and Interactive Vehicular Applications, Leeds, United Kingdom, September 9–14, 2021 (New York, NY, USA: ACM), 90–98. doi:10.1145/3409118.3475139
Forster, Y., Naujoks, F., and Neukum, A. (2017). “Increasing anthropomorphism and trust in automated driving functions by adding speech output,” in 2017 IEEE Intelligent Vehicles Symposium (IV), Los Angeles, CA, June 11–14, 2017 (IEEE). doi:10.1109/ivs.2017.7995746
Godley, S. T., Triggs, T. J., and Fildes, B. N. (2002). Driving simulator validation for speed research. Accid. Analysis and Prev. 34 (5), 589–600. doi:10.1016/s0001-4575(01)00056-2
Haar, A., Haeske, A. B., Kleen, A., Schmettow, M., and Verwey, W. B. (2022). Improving clarity, cooperation and driver experience in lane change manoeuvres. Transp. Res. Interdiscip. Perspect. 13, 100553. doi:10.1016/j.trip.2022.100553
Kaur, K., and Rampersad, G. (2018). Trust in driverless cars: investigating key factors influencing the adoption of driverless cars. J. Eng. Technol. Manag. 48, 87–96. doi:10.1016/j.jengtecman.2018.04.006
Kesting, A., Treiber, M., and Helbing, D. (2007). General lane-changing model MOBIL for car-following models. Transp. Res. Rec. J. Transp. Res. Board 1999 (1), 86–94. doi:10.3141/1999-10
Kondyli, A., and Elefteriadou, L. (2010). “Driver behavior at freeway-ramp merging areas based on instrumented vehicle observations,” in Conference: 5th International Congress on Transport Research, Volos, Greece.
Kusuma, A., Liu, R., Choudhury, C., and Montgomery, F. (2015). “Lane-changing characteristics at weaving section,” in Transportation Research Board 94th Annual Meeting, Washington, DC, January 11–15, 2015
Lee, J. D., and See, K. A. (2004). Trust in automation: designing for appropriate reliance. Hum. Factors 46 (1), 50–80. doi:10.1518/hfes.46.1.50_30392
Marczak, F., Daamen, W., and Buisson, C. (2013). Merging behaviour: empirical comparison between two sites and new theory development. Transp. Res. Part C Emerg. Technol. 36, 530–546. doi:10.1016/j.trc.2013.07.007
McNemar, Q. (1947). Note on the sampling error of the difference between correlated proportions or percentages. Psychometrika 12 (2), 153–157. doi:10.1007/bf02295996
Pöhler, G., Heine, T., and Deml, B. (2016). Itemanalyse und Faktorstruktur eines Fragebogens zur Messung von Vertrauen im Umgang mit automatischen Systemen. Z. für Arbeitswiss. 70 (3), 151–160. doi:10.1007/s41449-016-0024-9
Reinolsmann, N., Alhajyaseen, W., Brijs, T., Pirdavani, A., Hussain, Q., and Brijs, K. (2021). Investigating the impact of a novel active gap metering signalization strategy on driver behavior at highway merging sections. Transp. Res. Part F Traffic Psychol. Behav. 78, 42–57. doi:10.1016/j.trf.2021.01.017
Rios-Torres, J., and Malikopoulos, A. A. (2017). Automated and cooperative vehicle merging at highway on-ramps. IEEE Trans. Intelligent Transp. Syst. 18 (4), 780–789. doi:10.1109/TITS.2016.2587582
Shechtman, O., Classen, S., Awadzi, K., and Mann, W. (2009). Comparison of driving errors between on-the-road and simulated driving assessment: a validation study. Traffic Inj. Prev. 10 (4), 379–385. doi:10.1080/15389580902894989
Talebpour, A., and Mahmassani, H. S. (2016). Influence of connected and autonomous vehicles on traffic flow stability and throughput. Transp. Res. Part C Emerg. Technol. 71, 143–163. doi:10.1016/j.trc.2016.07.007
Treiber, M., and Kesting, A. (2010). “Stauentstehung und Stauausbreitung,” in Springer-Lehrbuch. Verkehrsdynamik und -simulation. Editors M. Treiber, and A. Kesting (Berlin, Heidelberg: Springer Berlin Heidelberg), 257–266. doi:10.1007/978-3-642-05228-6_17
Van Beinum, A., Farah, H., Wegman, F., and Hoogendoorn, S. (2018). Driving behaviour at motorway ramps and weaving segments based on empirical trajectory data. Transp. Res. Part C Emerg. Technol. 92, 426–441. doi:10.1016/j.trc.2018.05.018
Venkatesh, V., and Davis, F. D. (2000). A theoretical extension of the technology acceptance model: four longitudinal field studies. Manag. Sci. 46 (2), 186–204. doi:10.1287/mnsc.46.2.186.11926
Werner, A. (2019). New colours for autonomous driving: an evaluation of chromaticities for the external lighting equipment of autonomous vehicles. Colour. Turn. 0 (1). doi:10.25538/tct.v0i1.692
Keywords: cooperative connected driving, motorway slip road, user interface, driving simulator study, user study
Citation: Ehrhardt S, Martin M, Hild J and Deml B (2024) User expectations for an automated motorway access assistant system. Front. Future Transp. 5:1420073. doi: 10.3389/ffutr.2024.1420073
Received: 19 April 2024; Accepted: 11 September 2024;
Published: 24 September 2024.
Edited by:
David Kidd, Insurance Institute for Highway Safety (IIHS), United StatesReviewed by:
Nichole L. Morris, University of Minnesota Twin Cities, United StatesRahul Bhadani, The University of Alabama in Huntsville, United States
Copyright © 2024 Ehrhardt, Martin, Hild and Deml. This is an open-access article distributed under the terms of the Creative Commons Attribution License (CC BY). The use, distribution or reproduction in other forums is permitted, provided the original author(s) and the copyright owner(s) are credited and that the original publication in this journal is cited, in accordance with accepted academic practice. No use, distribution or reproduction is permitted which does not comply with these terms.
*Correspondence: Sofie Ehrhardt, c29maWUuZWhyaGFyZHQyQGtpdC5lZHU=