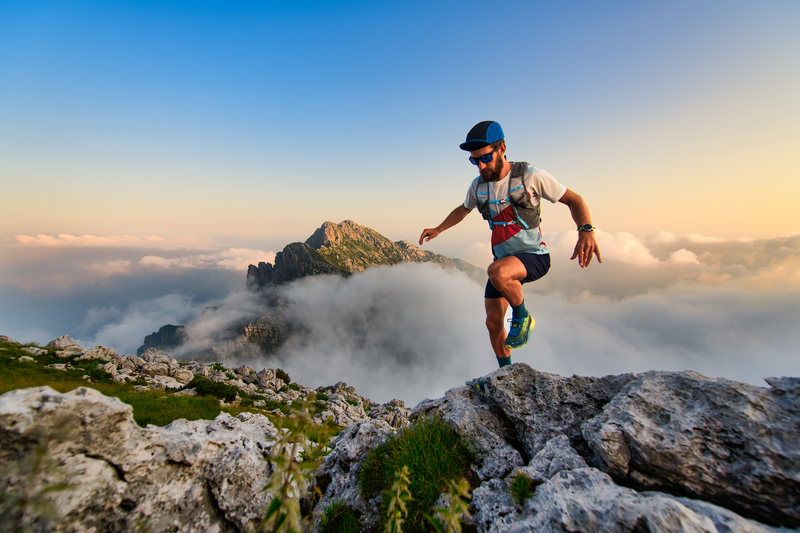
94% of researchers rate our articles as excellent or good
Learn more about the work of our research integrity team to safeguard the quality of each article we publish.
Find out more
ORIGINAL RESEARCH article
Front. Future Transp. , 08 February 2024
Sec. Connected Mobility and Automation
Volume 4 - 2023 | https://doi.org/10.3389/ffutr.2023.1239744
Current quantitative methods to evaluate pavement conditions in the United States are most commonly focused on construction acceptance using the International Roughness Index (IRI). However, from an asset management perspective, qualitative visual inspection techniques are the most prevalent. Modern vehicles with factory-equipped sensors drive these roadways daily and can passively assess the condition of infrastructure at an accuracy level somewhere between qualitative assessment and rigorous construction acceptance techniques. This paper compares crowdsourced ride quality data with an industry standard inertial profiler on a 7-mile bi-directional construction zone. A linear correlation was performed on 14 miles of I-65 that resulted in an R2 of 0.7 and a p-value of <0.001, but with a modest fixed offset bias. The scalability of these techniques is illustrated with graphics characterizing IRI values obtained from 730,000 crowdsourced data segments over 5,800 miles of I-80 in April of 2022 and October 2022. This paper looks at the use of standard original equipment manufacturer (OEM) on-board sensor data from production vehicles to assess approximately 100 miles of roadway pavements before, during, and after construction. The completed construction projects observed IRI improvements of 10 in/mi to 100 in/mi. These results suggest that it is now possible to monitor pavement ride quality at a system level, even with a small proportion of connected vehicles (CV) providing roughness data.
Connected vehicles (CV) are revolutionizing the way we travel on roads and highways, and often generate a wealth of data that can be used to improve the safety and efficiency of our transportation infrastructure (Ye and Yamamoto, 2019; Gueriau and Dusparic, 2020). Current penetration of connected vehicle data for various use cases is 3%–6% depending on the location of analysis (Sakhare et al., 2022). This data can provide a multitude of information to agencies even with low penetration rates. As vehicles become increasingly connected and equipped with advanced sensors/communication technologies, they can generate and transmit real-time data on traffic conditions, weather, road quality, and much more (Hadi et al., 2017; Intelligent Transportation Systems, 2023; Sakhare et al., 2023). Some of the use cases for connected vehicle data include impact of technology (digital alerts and automated speed enforcement), traffic signal analytics (impacts of timing changes), and infrastructure assessment (exit utilization, electric vehicle charging placement, and pavement marking evaluation) (Day et al., 2017; Mahlberg et al., 2021a; Sakhare et al., 2021; Desai et al., 2022; Mathew et al., 2022; Saldivar-Carranza et al., 2022). There are no established best practices for the use of connected vehicle data in transportation asset management (TAM) systems, but substantial opportunities are being created to use CV data for TAM systems as the penetration and technology continues to grow (Dennis et al., 2014a). This paper illustrates how this CV data can be harnessed by transportation agencies to assess the performance of our road network infrastructure and to identify areas where improvements are needed. This study contributes to the transportation field by assessing the accuracy and scalability of connected vehicle data for pavement quality evaluation.
Well maintained roads are essential for economic development, public safety, and quality of life. Smooth pavements provide a reliable and efficient transportation network that allows businesses to move goods and people. Many agencies utilize various software to track and manage pavement condition, design, and maintenance called Pavement Management Systems (PMS). This software often includes modules for data collection, analysis, performance modeling, maintenance and rehabilitation planning, and reporting and visualization at a project and network level (Lamptey et al., 2005; Flora et al., 2010; Wang and Pyle, 2019).
Pavement quality evaluation is typically done using a combination of visual inspections, physical measurements, and non-destructive testing techniques (Okine and Adarkwa, 2013; Pierce et al., 2013; Buttlar and Islam, 2014; Seraj et al., 2016; Kamranfar et al., 2022). A standard practice for evaluating pavements at scale is by capturing the International Roughness Index (IRI) with a pavement roughness profiler or an inertial profiler. This method is typically performed on all pavement types including asphalt and concrete and requires dedicated equipment including calibrated lasers, accelerometers, GPS, cameras, and other sensors (Sayers and Karamihas, 1998; Pawar et al., 2018). The data from all sensors are combined to generate an overall condition of the road surface quality. Values are reported in m/km or in/mi, and low values indicate better pavement quality (Kırbaş, 2021). Recent initiatives use LiDAR for pavement inspection because it can provide additional information on roadway drainage, pavement markings, and lane widths (Mekker et al., 2018; Ravi et al., 2020a; Ravi et al., 2020b; Cheng et al., 2020; Lin et al., 2020; Mahlberg et al., 2021b; Lin et al., 2021; Ravi et al., 2021; Mahlberg et al., 2022a; Feng et al., 2022). The data collected from these evaluations can be used to prioritize maintenance and rehabilitation efforts, optimize pavement design and material selection, and improve the overall performance of transportation infrastructure. A drawback to current techniques for pavement assessment is the requirement of dedicated equipment and personnel to drive the road network, which is time consuming and expensive.
The United States has a roadway network of over eight million lane miles which generates almost 8 billion vehicle miles per day (Bureau of Transportation Statistics, 2023; Estimated, 2023). Indiana has almost 90,000 bi-directional miles made up of almost 66,000 local, 21,000 state and US routes, and 2,500 interstate bi-directional miles (INDOT, 2023b; INDOT, 2023a; Indiana, 2023). For a singular dedicated vehicle to assess all roadways in Indiana, it would take over 1,800 h or 75 days driving non-stop. An alternative to this is utilizing data from vehicles that drive these roadways daily. Other studies have looked at methods to employ the use of production vehicles by attaching additional sensors to the vehicle including GPS, accelerometers, LiDAR and others to simulate laser profiler IRI (Dennis et al., 2014b; Bridgelall et al., 2018; Maryam et al., 2023). These studies provide correlated values but lacked scalability due to after-market sensor additions and a deficiency in penetration (Dennis et al., 2014b; Jang et al., 2017; Maryam et al., 2023). Current production vehicles have extensive built-in instrumentation that can be used to passively assess the condition of pavement. Through the integration of enhanced sensors, accelerometers and cellular connections, production vehicles can provide accurate, reliable, and repeatable pavement roughness values.
In addition, connected vehicle data provides near real-time data, which can help to identify areas of emerging concern and prioritize maintenance and repair efforts through data-driven inventory. This can be especially important for high-traffic areas or areas that are prone to damage as it allows for quick and targeted interventions to prevent further deterioration. The use and validation of this data source are proposed in Mahlberg et al., 2022 (Mahlberg et al., 2022b). Production vehicle data for pavement quality leverages individual wheel speed via rotational sensors in combination with drivetrain data to provide accurate roughness information (Magnusson and Svantesson, 2021). This method improves the reliability and reproducibility of pavement assessments and provides solutions to capture this information at scale, allowing for more informed decision-making.
An example of a road with a high IRI can be observed in Supplementary Figure S1. Connected vehicle data was used to identify this road section as an area of interest. Captured field validation images reveal the pavement visibly distressed including pothole patching, cracking, and rutting.
The objective of this study is to evaluate the feasibility of monitoring and screening pavement quality of construction zones and a transcontinental route using crowdsourced road roughness data. Large-scale IRI sampling is possible because of the ubiquitous coverage provided by crowdsourced production vehicles. Although there are many additional pavement condition performance measures beyond IRI that agencies track, this paper focuses on IRI.
The data collection devices used in this study included:
• GoPro cameras for qualitative pavement roughness validation;
• a single production vehicle allowing controlled testing on prescribed routes;
• a calibrated inertial profiler commonly used to measure IRI to assess contract compliance on paving projects;
• road roughness service provided by anonymized connected vehicles operating in the United States providing aggregated segment level pavement roughness data.
In the first phase, agency representatives provided seven construction locations of interest for collecting data. There were over 95 miles evaluated: once in Spring 2022 before construction and once in Winter 2022, with most of the projects having substantial resurfacing or reconstruction activities between the two periods. The identified construction zones were on US and State Routes. A single production vehicle equipped with an onboard camera was used to collect quantitative sensor and qualitative image data of the zones simultaneously. Six of the construction zones that were evaluated with the single production vehicle can be seen in Supplementary Figure S2 labeled as “La Porte District Construction Zone”. Later in the study, an inertial profiler was used to determine the accuracy and validity of the production vehicle data. Due to the cost of capturing data with the inertial profiler, this data was only captured on one 14-mile route seen in Supplementary Figure S2 labeled “Crawfordsville District Construction Zone”. For purposes of this study the difference between asphalt and concrete pavement were not evaluated as there is an established difference in roughness between the two materials (Smith et al., 2002; Smith and Ram, 2016) but the pavement improvements performed among the projects varied material type (old asphalt to new concrete, old asphalt to new asphalt, etc).
For this phase of the study, a single production vehicle was utilized for data collection to evaluate these techniques in a controlled manner and to provide representative field images before the project began and after it was completed. Images were taken at half-second intervals with timestamps and GPS location information. Both images and vehicle data were linear referenced to the nearest 0.16 km (0.1 mi) reference marker for analysis.
Data for the “before construction” period was collected on all routes from the end of March 2022 until the end of April 2022. Data for the “after construction” period was collected the month of November and December 2022. An overall view of before and after construction on all routes can be seen in Supplementary Figure S3 below. Every construction route observed improvement except for I-65 northbound, which will be discussed in further detail in the Crowdsourced Vehicles Results and Discussion section.
When summarizing the success of the resurfacing projects, agencies want to see improvements in ride quality. Supplementary Table S1 summarizes the results with each construction zone project number, route name, overall average change, and the total length analysed for each construction zone. Every construction zone saw an improvement before and after construction ranging from an IRI value decrease of 0.09–1.67 m/km (6–106 in/mi), with the exception of I-65 northbound. This information can help agencies determine the impact of each construction project and enables them to quantify and assess construction quality performed by contractors.
There are noticeable differences in pavement quality based on the before and after data collection in construction zones. This difference is highly dependent on the road condition before the construction was performed.
US-421 experienced the greatest improvement in IRI, which is highly attributed to the condition the roadway was in before construction. Supplementary Figure S4 shows US-421 by reference marker on the horizontal axis and raw IRI roughness value in the vertical axis. The plot shows the average collected values before construction as orange makers and after construction as black markers. The 15th and 85th percentiles were also plotted for each 0.16 km (0.1 mi) average point. There are two horizontal dotted lines at 1.49 m/km (95 in/mi) and 2.68 m/km (170 in/mi). These lines represent the national standard in the US for IRI thresholds. IRI values less than 1.49 m/km (95 in/mi) are classified as “good” road segments, whereas values below 2.68 m/km (170 in/mi) are “acceptable” road segments (Arhin et al., 2015). Values above 2.68 m/km (170 in/mi) surpass the threshold and require strategic repairs, maintenance, and rehabilitation. These thresholds set by the Federal Highway Administration (FHWA) also align with rider comfort (Kırbaş, 2021). Supplementary Figure S4A shows the northbound direction and Supplementary Figure S4B shows the southbound direction. Before construction shows considerable variation in pavement quality based on the fluctuations in values along the route and the tight grouping present in the after analysis. In Supplementary Figure S4B between reference markers 145 and 147, the before and after roughness values align closely with one another since there were no maintenance activities performed on this segment. This demonstrates the repeatability and consistency of the data since these data collections were almost 9 months apart. Three field validation points were chosen on US-421. Callout i in Supplementary Figure S4A can also be seen in Supplementary Figure S5B for spatial reference and in Supplementary Figure S6A,B as field validation images. Callout ii and iii can be seen spatially in Supplementary Figure S5A and in Supplementary Figure S6C–F as validation images respectively. It can also be noted in Supplementary Figure S6B that there is light precipitation occurring while data is collected. Although rain impacts laser profilers due to the water attenuating and distorting the beams (Guo et al., 2015; Infotechnology, 2023), there is generally little impact for production vehicle data.
The change in pavement quality is evident, but to help provide guidance to agencies as to where the highest change was observed, Supplementary Figure S5A geospatially shows the change in IRI values on each road segment. The coloring of each 0.16 km (0.1 mi) reference marker is based on the difference before construction and after construction. In both the southbound direction (Supplementary Figure S5A) and the northbound direction (Supplementary Figure S5B) much of the road segment saw IRI values decrease by 0.8 m/km to 2.3 m/km (50–150 in/mi). It can be observed that north of the construction zone (reference marker 145, callout iv) in Supplementary Figure S5A, there was no change in the pavement quality which is expected as this area did not receive maintenance activities due to being outside of the construction limits.
Supplementary Figure S5A,C,F show the before construction pavement conditions and Supplementary Figure S6B,D,Fshow the after conditions. The before construction images show visible signs of pavement deterioration including alligator cracking, longitudinal cracking, and rutting. Supplementary Figure S6A,B demonstrate the large gap in IRI values seen as callout i on US-421 northbound (Supplementary Figure S4A) and Supplementary Figure S6C,D demonstrate the large gap seen as callout ii on US-421 northbound (Supplementary Figure S4B). Callout iii on US-421 southbound at reference marker 141.52 on Supplementary Figure S4B shows similar values for before construction and after construction. Using the field validation images, it is apparent this location has a bridge. The observed values at this location are due to no maintenance being performed on this bridge deck and the bridge joints.
A similar analysis was conducted for construction project R43165 Willow Creek Road. This local road in Portage, Indiana is a two to four lane road and saw an improvement of nearly −1.23 m/km (−78 in/mi) in IRI values on approximately 1.5 miles. The road quality roughness diagrams for before and after construction can be seen in Supplementary Figure S7. Supplementary Figure S7A,B show northbound and southbound directions, respectively. Callouts i, ii, iii are field validation points from GoPro images seen in Supplementary Figure S8A–F respectively.
The production vehicle data and field validation images help visualize and showcase the changes before and after construction. The analysis helps validate the use of production vehicle data qualitatively.
Although a single vehicle could be driven by agency personnel around the state to replicate the standard practice with production vehicles, a much more scalable approach would be to passively receive this data from a fleet of vehicles daily. Roughness data derived from production vehicle sensors provide a robust system for agencies to evaluate pavement quality. Such an approach would allow an agency to have a dynamically updated view of their pavement quality over time. The I-65 road widening construction project near Lebanon, Indiana is a multi-year project being completed in phases. Part of the first phase was completed on 20 June 2022, and traffic in the southbound direction was shifted to the newly constructed pavement, while the northbound direction remained on the old pavement. The southbound direction provided an analysis of old pavement versus new pavement, while the northbound direction provided an analysis of the same pavement. Supplementary Figure S9A shows the production vehicle roughness values for the northbound direction (Supplementary Figure S9A) and southbound direction (Supplementary Figure S9B). Northbound reveals the pavement condition deterioration over the course of the 6-month time span, while the southbound direction saw improvement once the new pavement was utilized.
Supplementary Figure S10A shows an INDOT traffic camera in this work zone and shows both directions of vehicles traveling on their respective old pavement. Supplementary Figure S10B shows the southbound direction traveling on the new pavement and the northbound direction traveling on the old pavement. These images help validate what is observed in the graphs in Supplementary Figure S9 with the northbound direction seeing a small amount of deterioration overtime and the southbound direction seeing improvement but not to the extent of the resurface projects due to intermediate travel lanes and construction.
To assess the relationship between production vehicle data and industry standard IRI data collection, an agency calibrated IRI inertial profiler also collected data on this route. The inertial profiler seen in Supplementary Figure S11A computes roughness using the vehicle’s speed, accelerometer, and laser scanners to determine the height to the pavement (callout i and ii) (Sayers and Karamihas, 1998). Data was captured at a speed of 88 kph (55 mph) since the maximum operating speed of the profiler is around 96 kph (60 mph). For both the production vehicle and profiler, the results are dependent on the wheel path. Supplementary Figure S11B shows the interior of the profiler, callout iii shows the dash camera, which takes images every 30 m (100 ft), and callout iv shows the user interface.
The profiler generates IRI values every 3 m (10 ft), and the production vehicles produce IRI values every 0.02 s. Due to the difference in data collection frequency, both the profile data and production vehicle were mapped to the nearest 0.16 km (0.1 mi) reference marker on Interstate 65, which is approximately 16 m (53 ft).
A comparison of the crowdsourced roughness data (red) and the inertial profiler data (black) is shown in Supplementary Figure S12 below. Profiler data is data collected from the inertial profiler providing raw IRI roughness values. Crowdsourced roughness data is collected from crowdsourced production vehicles, and the relatively large number of crowdsourced data points mitigates the impact of anomalies and outliers. Crowdsourced roughness data is linear referenced without lane-level fidelity for current fleet models. Supplementary Figure S12A shows the southbound crowdsourced roughness and profiler roughness before construction (lane change), and Supplementary Figure S12B shows the data after the lane change.
A similar comparison was done in the northbound direction observed in Supplementary Figure S12C, D. Supplementary Figure S12C shows northbound crowdsourced roughness and profiler roughness before the lane change (that was done for the southbound direction only) and Supplementary Figure S12D shows data after that southbound lane change.
The crowdsourced data tracks the profiler data well for both the northbound and southbound directions. To provide a statistical evaluation of this relationship, average profiler IRI and crowdsourced production vehicle IRI values for each 0.16 km (0.1 mile) section is plotted in a scatter plot (Supplementary Figure S13). A linear trendline is fit over the points with an R2 of 0.7. A Pearson test was also performed and a p-value of <0.001 suggests there is statistical significance to reject the null hypothesis of: no linear correlation between the profiler data and the crowdsourced data. The correlation value for the Pearson test is 0.83 indicating a strong positive correlation between the profiler IRI values and the CV crowdsourced IRI values. A systematic offset can be observed in Supplementary Figure S13 and although not sufficient for construction contract acceptance, the strong correlation suggests crowdsourced roughness data is sufficient for asset management applications. The difference can be attributed to how each technology estimates IRI, or that due to multiple passes being performed with crowdsourced data, a holistic value is being obtained. Longer term, a more objective comparison of the methods between these systems are required to determine the reason behind the offset. Even with the offset, this data would allow agencies to view their pavement quality in near real-time at an acceptable fidelity before investing valuable time and resources to collect higher-precision data with the inertial profiler.
Scheduling inertial profiler runs requires considerable planning and coordination to perform data collection on a specific roadway. Similarly, challenges are faced by scheduling a driver to use a specific CV vehicle to drive a route. In contrast, data from crowdsourced production vehicles are available within a day, providing aggregated roughness values for roadway segments estimated by multiple vehicle-passes over the segment in the previous 60 days. Uncertainty values are provided for each segment, as different vehicles may take different wheel paths through the system, e.g., avoidance of potholes, and may produce different results in the short-term. Crowdsourced data helps agencies efficiently prioritize maintenance activities without the need for data collection. The scalability of this data also allows agencies to perform a systemwide assessment before allocating dollars to projects.
An example of scaling this nationally can be seen by using over 350,000 data segments from 1 April 2022, and over 370,000 data segments from 1 October 2022, on interstate I-80. Supplementary Figure S14 shows pavement quality for almost 2,900 miles of I-80 which runs coast to coast in the United States. The roughness data for every mile is colour coded by the FHWA thresholds. The horizontal axis shows the proportion of the 1-mile segment operating under different pavement quality and the vertical axis shows the reference marker and the corresponding state. Supplementary Figure S14A shows pavement quality for I-80 westbound in April 2022 (approximately before construction season) and Supplementary Figure S14B shows I-80 westbound in October 2022 (after construction season). Comparing Supplementary Figure S14A, B, the state border between Nebraska and Wyoming (callout i) shows considerable improvement in pavement quality. Similarly, the sections near reference marker 300 in Nevada (callout ii) also shows substantial improvement in pavement quality. The improvements in pavement quality between Supplementary Figure S14A, B suggests a strong possibility of construction activities between these periods. A similar analysis was performed in the eastbound direction and similar results were found with improvements in Nebraska and Wyoming.
Connected vehicle data can augment traditional methods of collecting pavement roughness data by enabling scalability and repeatability of the data analysis. This paper demonstrated both quantitatively and qualitatively how connected vehicle data provides an opportunity for dynamic and scalable operational assessment of pavement quality. Both a single production vehicle and crowdsourced data were utilized to provide accurate pavement roughness measures.
• Supplementary Figure S13 demonstrates accuracy and reliability of crowdsourced pavement roughness compared to the industry standard of an inertial profiler. The comparison had an R2 of 0.7 and a p-value of <0.001.
• Over 5,800 miles of I-80 in April 2022 and October 2022 were evaluated using the proposed methodology with 730,000 crowdsourced data segments used.
This analysis provides context and suggests that using this data at scale can be utilized for network-wide analysis. Agencies can view their pavement quality in near real-time at an acceptable fidelity before investing valuable time and resources to collect higher-precision data with the inertial profiler. This grants them the ability to monitor the improvement of pavement before and after construction projects and observe pavement deterioration over time. Scaling this to transcontinental I-80 reveals areas in need of attention and enables agencies to realize how effective their improvement projects have been (Supplementary Figure S14). Crowdsourced data enables agencies to justify and reinforce the need for improvement projects on not only state routes and interstates, but for local roads as well. These techniques can also start a nationwide dialogue to revisit current guidelines for pavement quality evaluation and initiate conversations as to how design procedures may be expanded to incorporate new datasets for the next-generation of pavement maintenance assessment.
The data analyzed in this study is subject to the following licenses/restrictions: This data must be purchased from the data provider NIRA Dynamics AB. Requests to access these datasets should be directed to BJÖRN ZACHRISSON Ympvcm4uemFjaHJpc3NvbkBuaXJhZHluYW1pY3Muc2U=.
The authors confirm contribution to the paper as follows: Study conception and design: JAM, HL, and DB. Data collection: JAM, HL, and BZ. Analysis and interpretation of results: JAM, HL, and JKM. Draft manuscript preparation: JAM and HL. All authors reviewed the results and approved the final version of the manuscript.
This work was funded through Agreement A249-18-ON180087 and STIND 75458 between Purdue University and the Indiana Department of Transportation. The crowdsourced road roughness data was provided by NIRA Dynamics, AB. The contents of this paper reflect the views of the authors, who are responsible for the facts and accuracy of the data presented herein, and do not necessarily reflect the official views or policies of the sponsoring organizations or data vendors. These contents do not constitute a standard, specification, or regulation.
HL and JAM are a co-founders of LSM Analytics, a company that aims to further develop and commercialize some of the technologies/methods described in this manuscript. BZ was employed by NIRA Dynamics AB.
The remaining authors declare that the research was conducted in the absence of any commercial or financial relationships that could be construed as a potential conflict of interest.
All claims expressed in this article are solely those of the authors and do not necessarily represent those of their affiliated organizations, or those of the publisher, the editors and the reviewers. Any product that may be evaluated in this article, or claim that may be made by its manufacturer, is not guaranteed or endorsed by the publisher.
The Supplementary Material for this article can be found online at: https://www.frontiersin.org/articles/10.3389/ffutr.2023.1239744/full#supplementary-material
Arhin, S. A., Noel, E. C., and Ribbiso, A. (2015). Acceptable international roughness index thresholds based on present serviceability rating. J. Civ. Eng. Res. 5 (4), 90–96. doi:10.5923/j.jce.20150504.03
Bridgelall, R., Rahman, M., Daleiden, J. F., and Tolliver, D. (2018). Error sensitivity of the connected vehicle approach to pavement performance evaluations. Int. J. Pavement Eng. 19 (1), 82–87. doi:10.1080/10298436.2016.1162307
Bureau of Transportation Statistics, (2023). Vehicle-miles | bureau of transportation Statistics. https://www.bts.gov/content/us-vehicle-miles (Accessed March 1, 2023).
Buttlar, W., and Islam, S. (2014). Integration of smart-phone-based pavement roughness data collection tool with asset management system. Available at: https://www.researchgate.net/publication/272355273_Integration_of_Smart-Phone-Based_Pavement_Roughness_Data_Collection_Tool_with_Asset_Management_System.
Cheng, Y. T., Patel, A., Wen, C., Bullock, D., and Habib, A. (2020). Intensity thresholding and deep learning based lane marking extraction and lane width estimation from mobile light detection and ranging (LiDAR) point clouds. Remote Sens. 12 (9), 1379. doi:10.3390/RS12091379
Day, C. M., Li, H., Richardson, L. M., Howard, J., Platte, T., Sturdevant, J. R., et al. (2017). Detector-free optimization of traffic signal offsets with connected vehicle data. Transp. Res. Rec. 2620, 54–68. doi:10.3141/2620-06
Dennis, E., Hong, Q., Wallace, R., Tansil, W., and Smith, M. (2014a). Pavement condition monitoring with crowdsourced connected vehicle data. Transp. Res. Rec. 2460 (1), 31–38. doi:10.3141/2460-04
Dennis, E. P., Hong, Q., Wallace, R., Tansil, W., and Smith, M. (2014b). Pavement condition monitoring with crowdsourced connected vehicle data. Transp. Res. Rec. 2460 (1), 31–38. doi:10.3141/2460-04
Desai, J., Mathew, J. K., Li, H., and Bullock, D. M. (2022). Leveraging connected vehicle data to assess interstate exit utilization and identify charging infrastructure investment allocation opportunities. World Electr. Veh. J. 13 (9), 167. doi:10.3390/wevj13090167
Estimated, U. S. (2023). Roadway lane-miles by functional system | bureau of transportation Statistics. https://www.bts.gov/content/table-1-6-estimated-us-roadway-lane-miles-functional-systema (Accessed March 1, 2023).
Feng, Z., El Issaoui, A., Lehtomäki, M., Ingman, M., Kaartinen, H., Kukko, A., et al. (2022). Pavement distress detection using terrestrial laser scanning point clouds – accuracy evaluation and algorithm comparison. ISPRS Open J. Photogrammetry Remote Sens. 3, 100010. doi:10.1016/j.ophoto.2021.100010
Flora, W. F., Ong, G., and Sinha, K. C. (2010). Development of a structural index as an integral part of the overall pavement quality in the INDOT PMS, West Lafayette, Indiana: Indiana Department of Transportation and Purdue University. doi:10.5703/1288284314261
Gueriau, M., and Dusparic, I. (2020). “Quantifying the impact of connected and autonomous vehicles on traffic efficiency and safety in mixed traffic,” in 2020 IEEE 23rd International Conference on Intelligent Transportation Systems (ITSC), Rhodes, Greece, September, 2020, 1–8. doi:10.1109/ITSC45102.2020.9294174
Guo, J., Zhang, H., and Zhang, X. J. (2015). Propagating characteristics of pulsed laser in rain. Int. J. Antennas Propag. 2015, 1–7. doi:10.1155/2015/292905
Hadi, M., Xiao, Y., Iqbal, S., and Khazraeian, S. (2017). Utilization of connected vehicle data to support traffic management decisions. Florida: Department of Transportation. Available at: https://rosap.ntl.bts.gov/view/dot/35836/dot_35836_DS1.pdf.
Indiana, (2023). List of state roads in Indiana. https://thisisindiana.angelfire.com/stateroadsindiana.htm (Accessed March 1, 2023).
INDOT (2023a). Indiana road system facts. https://www.in.gov/indot/about-indot/central-office/asset-data-collection/indiana-road-system-facts/ (Accessed March 1, 2023).
INDOT (2023b). Indiana state transportation map facts. https://www.in.gov/indot/resources/maps/indiana-state-transportation-map-facts/ (Accessed March 1, 2023).
Infotechnology, (2023). Pavements - inertial profiler – pavement (IP). Federal highway administration. https://infotechnology.fhwa.dot.gov/inertial-profiler-road-pavement/ (Accessed November 9, 2023).
Intelligent Transportation Systems, (2023). Intelligent transportation systems - road weather connected vehicle applications. https://www.its.dot.gov/research_archives/road_weather/road_weather_progress.htm (Accessed March 2, 2023).
Jang, J., Yang, Y., Smyth, A. W., Cavalcanti, D., and Kumar, R. (2017). Framework of data acquisition and integration for the detection of pavement distress via multiple vehicles. J. Comput. Civ. Eng. 31 (2), 1–15. doi:10.1061/(asce)cp.1943-5487.0000618
Kamranfar, P., Lattanzi, D., Shehu, A., and Stoffels, S. (2022). Pavement distress recognition via wavelet-based clustering of smartphone accelerometer data. J. Comput. Civ. Eng. 36 (4), 1–12. doi:10.1061/(asce)cp.1943-5487.0001022
Kırbaş, U. (2021). Determination of ride comfort thresholds based on international roughness index for asphalt concrete pavements. Int. J. Pavement Eng., 1–13. doi:10.1080/10298436.2021.2011279
Lamptey, G., Ahmad, M., Labi, S., and Sinha, K. (2005). Life cycle cost analysis for INDOT pavement design procedures. Fed. Highw. Adm. 53 (9), 1689–1699. doi:10.5703/1288284313261
Lin, Y., Manish, R., Bullock, D., and Habib, A. (2021). Comparative analysis of different mobile LiDAR mapping systems for ditch line characterization. Remote Sens. 13 (13), 2485. doi:10.3390/rs13132485
Lin, Y. C., Bullock, D., and Habib, A. (2020). Mapping roadway drainage ditches using mobile LiDAR. Int. Archives Photogrammetry, Remote Sens. Spatial Inf. Sci. - ISPRS Archives 43 (B1), 187–192. doi:10.5194/isprs-archives-XLIII-B1-2020-187-2020
Magnusson, P., and Svantesson, T. (2021). Road condition monitoring. 10,953,887. USPTO. Available at: https://patents.google.com/patent/US10953887B2/en.
Mahlberg, J. A., Cheng, Y.-T., Bullock, D. M., and Habib, A. (2021b). Leveraging LiDAR intensity to evaluate roadway pavement markings. Future Transp. 1 (3), 720–736. doi:10.3390/futuretransp1030039
Mahlberg, J. A., Li, H., Cheng, Y.-T., Habib, A., and Bullock, D. M. (2022a). Measuring roadway lane widths using connected vehicle sensor data. Sensor 22 (19), 7187. doi:10.3390/s22197187
Mahlberg, J. A., Li, H., Zachrisson, B., Leslie, D. K., and Bullock, D. M. (2022b). Pavement quality evaluation using connected vehicle data. Sensors 22 (23), 9109–9118. doi:10.3390/s22239109
Mahlberg, J. A., Sakhare, R. S., Li, H., Mathew, J. K., Bullock, D. M., and Surnilla, G. C. (2021a). Prioritizing roadway pavement marking maintenance using lane keep assist sensor data. Sensors 21, 6014–6016. doi:10.3390/s21186014
Maryam, S., Golroo, A., Tavakoli, D., and Fahmani, M. (2023). Potential applications of connected vehicles in pavement condition evaluation: a brief review. Road Mater. Pavement Des., 1–25. doi:10.1080/14680629.2023.2237597
Mathew, J. K., Li, H., Landvater, H., and Bullock, D. M. (2022). Using connected vehicle trajectory data to evaluate the impact of automated work zone speed enforcement. Sensors 22 (8), 2885–2915. doi:10.3390/s22082885
Mekker, M. M., Lin, Y. J., Elbahnasawy, M. K. I., Shamseldin, T. S. A., Li, H., Habib, A. F., et al. (2018). Application of LiDAR and connected vehicle data to evaluate the impact of work zone geometry on freeway traffic operations. Transp. Res. Rec. 2672 (16), 1–13. doi:10.1177/0361198118758050
Okine, A., and Adarkwa, O. (2013). Pavement condition surveys – overview of current practices. Del. Cent. Transp. 19716 (302), 1–71.
Pawar, P. R., Mathew, A. T., and Saraf, M. R. (2018). IRI (international roughness index): an indicator of vehicle response. Mater. Today Proc. 5 (5), 11738–11750. doi:10.1016/j.matpr.2018.02.143
Pierce, L. M., McGovern, G., and Zimmerman, K. A. (2013). Practical guide for quality management of pavement condition data collection. Washington, DC, USA: U.S. Department of Transportation, 170. Available at: https://www.fhwa.dot.gov/pavement/management/qm/data_qm_guide.pdf.
Ravi, R., Bullock, D., and Habib, A. (2020a). Highway and airport runway pavement inspection using mobile LiDAR. Int. Archives Photogrammetry, Remote Sens. Spatial Inf. Sci. - ISPRS Archives 43 (B1), 349–354. doi:10.5194/isprs-archives-XLIII-B1-2020-349-2020
Ravi, R., Bullock, D., and Habib, A. (2021). Pavement distress and debris detection using a mobile mapping system with 2D profiler LiDAR. Transp. Res. Rec. J. Transp. Res. Board 2021, 428–438. doi:10.1177/03611981211002529
Ravi, R., Cheng, Y. T., Lin, Y. C., Lin, Y. J., Hasheminasab, S. M., Zhou, T., et al. (2020b). Lane width estimation in work zones using LiDAR-based mobile mapping systems. IEEE Trans. Intelligent Transp. Syst. 21 (12), 5189–5212. doi:10.1109/TITS.2019.2949762
Sakhare, R. S., Desai, J. C., Mahlberg, J., Mathew, J. K., Kim, W., Li, H., et al. (2021). Evaluation of the impact of queue trucks with navigation alerts using connected vehicle data. J. Transp. Technol. 11 (04), 561–576. doi:10.4236/jtts.2021.114035
Sakhare, R. S., Hunter, M., Mukai, J., Li, H., and Bullock, D. M. (2022). Truck and passenger car connected vehicle penetration on Indiana roadways. J. Transp. Technol. 12 (04), 578–599. doi:10.4236/jtts.2022.124034
Sakhare, R. S., Zhang, Y., Li, H., and Bullock, D. M. (2023). Impact of rain intensity on interstate traffic speeds using connected vehicle data. Vehicles 5 (1), 133–155. doi:10.3390/vehicles5010009
Saldivar-Carranza, E., Li, H., Mathew, J., Fisher, C., and Bullock, D. M. (2022). Signalized corridor timing plan change assessment using connected vehicle data. J. Transp. Technol. 12 (03), 310–322. doi:10.4236/jtts.2022.123019
Sayers, M. W., and Karamihas, S. M. (1998). The little book of profiling. https://deepblue.lib.umich.edu/bitstream/handle/2027.42/21605/90151.pdf.
Seraj, F., van der Zwagg, B. J., Dilo, A., Luarasi, T., and Havinga, P. (2016). “RoADS: a road pavement monitoring systmem for anomaly detection using smart phones,” in Big Data Analytics in the Social and Ubiquitous Context, Nancy, France, September, 2016. doi:10.1007/978-3-319-29009-6_7
Smith, K., and Ram, P. (2016). Measurıng and specıfyıng pavement smoothness. https://www.fhwa.dot.gov/pavement/pubs/hif16032.pdf.
Smith, K. L., Titus-Glover, L., and Evans, L. D. (2002). Pavement smoothness index relationships: final report. http://www.fhwa.dot.gov/pavement/pub_listing.cfm?areas=Smoothness.
Wang, Z., and Pyle, T. (2019). Implementing a pavement management system: the caltrans experience. Int. J. Transp. Sci. Technol. 8 (3), 251–262. doi:10.1016/j.ijtst.2019.02.002
Keywords: connected vehicles (cv), crowdsource data, profiler, international roughness index (IRI), pavement quality
Citation: Mahlberg JA, Li H, Zachrisson B, Mathew JK and Bullock DM (2024) Applications of using connected vehicle data for pavement quality analysis. Front. Future Transp. 4:1239744. doi: 10.3389/ffutr.2023.1239744
Received: 13 June 2023; Accepted: 13 November 2023;
Published: 08 February 2024.
Edited by:
Qixiu Cheng, University of Bristol, United KingdomCopyright © 2024 Mahlberg, Li, Zachrisson, Mathew and Bullock. This is an open-access article distributed under the terms of the Creative Commons Attribution License (CC BY). The use, distribution or reproduction in other forums is permitted, provided the original author(s) and the copyright owner(s) are credited and that the original publication in this journal is cited, in accordance with accepted academic practice. No use, distribution or reproduction is permitted which does not comply with these terms.
*Correspondence: Darcy M. Bullock, ZGFyY3lAcHVyZHVlLmVkdQ==
Disclaimer: All claims expressed in this article are solely those of the authors and do not necessarily represent those of their affiliated organizations, or those of the publisher, the editors and the reviewers. Any product that may be evaluated in this article or claim that may be made by its manufacturer is not guaranteed or endorsed by the publisher.
Research integrity at Frontiers
Learn more about the work of our research integrity team to safeguard the quality of each article we publish.