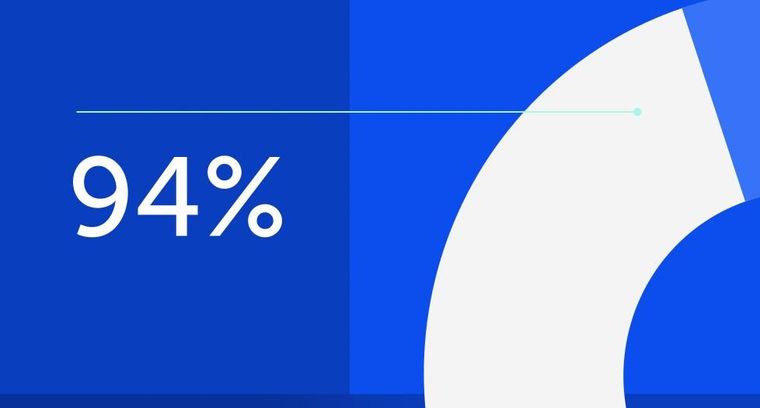
94% of researchers rate our articles as excellent or good
Learn more about the work of our research integrity team to safeguard the quality of each article we publish.
Find out more
ORIGINAL RESEARCH article
Front. Future Transp., 14 December 2022
Sec. Transportation Systems Modeling
Volume 3 - 2022 | https://doi.org/10.3389/ffutr.2022.1031021
This article is part of the Research TopicWomen in Transportation Research 2022View all 4 articles
The use of on-demand delivery services increased in Brazil during the COVID-19 pandemic, mainly by requests for ready meals. While consumers appreciate convenience, the delivery fee is a decisive factor in the purchase process. However, the delivery fee pricing strategy of on-demand delivery service platforms has not been discussed in the literature. Thus, this study aims to analyze the factors that influence the delivery fee pricing of on-demand delivery services and explores the impact of delivery fee strategies on the remuneration of couriers. We collected data from three leading on-demand delivery service platforms regarding product type, order price, service fee, delivery fee, order day, order time, waiting time, and distance. As a result, our database comprises 1,440 orders in 12 Brazilian municipalities. A linear regression model was estimated to identify the factors influencing the delivery fee pricing considering different product types. Findings showed that product type has a diverse effect on delivery fee pricing. Moreover, distance, regardless of the product type, positively influences the delivery fee. The delivery fee of the ready meals is affected by the service fee, waiting time, order day, and order time. Furthermore, the waiting time and order day affect the delivery fee of supermarket and bakery products and beverages. Finally, the delivery fee of medical products is influenced by order day and time. Findings can be helpful for the pricing strategy of on-demand delivery services.
The use of on-demand delivery services began in the 1960s (Nordzi et al., 2018), where goods such as pizza and documents were delivered. These delivery services have increased with the diffusion of platforms through application services, which act as intermediaries in the supply and demand of labor to provide services (Tassinari and Maccarrone, 2020; Allen et al., 2021). On-demand delivery services are part of the sharing economy (Choi et al., 2020).
The on-demand delivery service is based on attending immediately to the customers’ wishes for goods or services (Taylor, 2018). It is part of the on-demand and gig economies because they use the sharing economy concept. The delivery person must own a car, motorcycle, or bicycle and, when not in use, provide use of their vehicle to someone else (Chen H et al., 2019). The remuneration of delivery person is by cash or other temporary service made available by the service providers. Access to goods and services is provided by establishing connections between small service providers and consumers. In a gig economy, apps allow one to measure, observe, and control the work process (Gregory, 2021). Moreover, the apps provide flexibility, instability, and legal and financial uncertainties for workers (Allen et al., 2021).
The advance of e-commerce and the popularization of applications for smartphones have made the demands of customers more sophisticated, contributing to a better balance between supply and demand (Dablanc et al., 2017; Xu, 2021) and imposing short delivery times (Dablanc et al., 2017; Zhang et al., 2019). In developing countries, on-demand delivery services have caused the replacement of active travel—walking or cycling—for vehicle-based delivery services, with negative impacts on road safety, public health, and the environment (Wang and He, 2021).
On-demand delivery services require, in general, a short delivery time, also referred to as instant delivery services. This phenomenon was studied in countries such as France (Dablanc et al., 2017; Aguilera et al., 2018), England (Allen et al., 2021), China (Nordzi et al., 2018; Tong et al., 2020), and Brazil (Abilio et al., 2021; Abilio et al., 2021; Mello et al., 2022). However, most of the literature focuses on the operational processes of on-demand delivery services, analyzing route optimization based on customer satisfaction (Zhang et al., 2020), simulations for scheduling instant deliveries (Huang et al., 2020), evaluating the operational performance of meal deliveries and their impacts on transport and the environment (Allen et al., 2021), proposing an application of on-demand runner booking services (Nordzi et al., 2018), evaluating the dynamics of the pricing of on-demand food delivery apps (Tong et al., 2020), the commission fee of on-demand services (Li and Wang, 2021), and analyzing the optimal pricing decision and the impacts brought by customers’ risk attitudes on the platform’s profit (Choi et al., 2020). Moreover, some scholars have analyzed the working conditions of couriers (Abilio et al., 2021; Abilio et al., 2021) and the relationship between the delivery fee and the remuneration of couriers (Mello et al., 2022).
With the COVID-19 pandemic, as many consumers stayed at home to avoid the contagion, there was a change in consumer behavior which generated high demand for online shopping, supermarket deliveries, and ready meals (Budd and Ison, 2020). In Brazil, the COVID-19 pandemic has increased the use of on-demand delivery services, especially ready meals. These deliveries are time-sensitive, as the customer wants to be served immediately (Taylor, 2018). Instant deliveries, therefore, impose a strict deadline for delivery that, in turn, should occur within up to 2 h (Dablanc et al., 2017; Zhang et al., 2020). Instant delivery services are part of a diversity of urban freight delivery modalities that modify personal mobility patterns, challenging freight transport operations and practices and urban planning (Bjørgen et al., 2021).
On-demand delivery pressures transportation infrastructure, negatively contributing to environmental impacts and imposing challenges for cities (Allen et al., 2021). In low-income countries, most of these deliveries are made using motorcycles, increasing the probability of crashes (Chung et al., 2014). Moreover, instant delivery services expose couriers to traffic vulnerability, especially in large urban centers (Dablanc et al., 2017). Another negative aspect of the on-demand economy is related to the working conditions, particularly the perceived exploitation of couriers and other workers in the so-called gig economy (Dablanc et al., 2017; Allen et al., 2021; Mello et al., 2022).
The market for on-demand delivery services has grown in recent years. Brazil has one of the fastest growing on-demand delivery markets in the world. At the same time, China, the United States, South Korea, the United Kingdom, and India are the top revenue markets. In 2021, 61.3 million users generated a revenue of 2.65 billion dollars for Brazilian companies (Statista, 2022). Furthermore, although restaurant-to-consumer delivery represents 70% of users and 80% of revenue of on-demand delivery services in Brazil, other products have increased from 3.3% in 2019 to 6.3% in 2022 (Statista, 2022).
The delivery fee attracts consumers and influences their purchase patterns (Nguyen et al., 2019). In addition, the delivery fee affects the remuneration of couriers. However, the pricing strategy is infrequently explored by scholars. Because the delivery fees on on-demand platforms vary, it is difficult to analyze the couriers’ earnings (Goods et al., 2019) based only on consumer demand, as the customer pays for a delivery service without knowing how it contributes to the remuneration of couriers (Tong et al., 2020). Xu (2021) emphasized that customers of on-demand food services, when placing their orders, believe that their payment is received by the restaurant even if they pay through the platform and that the platform charges a certain proportion of the order cost from the restaurant. However, this does not occur in practice. The apps adjust the delivery fee according to dynamic pricing based on factors such as demand, market supply, extreme weather, promotions, holidays, and weekends (Tong et al., 2020). Moreover, the apps retain part of the profit from restaurant sales, and nothing is known about the remuneration of couriers (Chen M et al., 2019; Tong et al., 2020). Tong et al. (2020) stated that whether delivery fee fluctuations are based on real-time supply-and-demand conditions or distance or product weight/size is unclear.
The delivery fee supports the logistics cost of online retailers (Lewis, 2006) and influences consumer purchase patterns (Nguyen et al., 2019). Literature concerning delivery fees has investigated the effect of delivery fees on e-consumer behavior patterns (Lewis, 2006; Lewis et al., 2006; Becerril-Arreola et al., 2013; Nguyen et al., 2019). The delivery fee guides consumer preferences for delivery options in e-shops (Nguyen et al., 2019) and influences the order incident rate (Lewis, 2006). However, few scholars have addressed the factors influencing the delivery fee of on-demand platforms. The pioneering study of Tong et al. (2020) investigated the pricing strategy of on-demand food service platforms in China. The authors found the importance of delivery time and rating for promoting demand. However, demand for short delivery times encourages high driving speed from couriers. To avoid high speeds in urban areas and consequently the increase in violations and the risk of traffic accidents, Federal Law No. 12,436/11 was created in Brazil, aiming at prohibiting the use of practices that encourage increased speed of motorcyclists during the delivery service. Understanding the different factors influencing the delivery rate is necessary to create public policies that mitigate the externalities that involve the delivery service. It is also essential to know how the delivery fee can encourage the mode of consumption, causing a change in consumer habits and promoting significant changes in urban freight transport and, consequently, in urban mobility. Moreover, the delivery fee could increase the balance of the delivery demand market and the supply of couriers (Tong et al., 2020). Moreover, according to Tong et al. (2020), there is no clear consensus in the literature regarding the pricing strategies of on-demand delivery services.
Although many scholars have addressed on-demand delivery services (Dablanc et al., 2017; Choi et al., 2020; Tong et al., 2020; Allen et al., 2021; Mello et al., 2022), little can be said about the effects of these variables on delivery fee pricing. Thus, the problem addressed in this study is how the factors related to on-demand delivery requests affect the delivery fee. Delivery fee pricing was explored only by Tong et al. (2020), who analyzed China’s on-demand food service market. Understanding these factors allows for better pricing of the delivery fee by establishments to promote fair remuneration for couriers.
In order to reduce this research gap, this study aims to analyze the factors that influence the delivery fee pricing of on-demand delivery services and explores the impact of delivery fee strategies on the remuneration of couriers. Based on extensive data collection in the three leading Brazilian on-demand delivery services applications, we obtained information about the delivery fee, service fee, order price, product type, order day, order time, and distance. Moreover, this study explored other products, such as beverages and medicine, from those studied by Tong et al. (2020), who focused only on the food service sector.
The contribution of this study refers to the identification of the factors that influence the pricing of the delivery fee by different products type offered by the primary on-demand delivery services in Brazil. Furthermore, we explored the impact of delivery fee strategies on the remuneration of couriers. For Goods et al. (2019), the income varies according to courier working hours, pricing strategies, and customer demand (Goods et al., 2019). Mello et al. (2022) showed that the distance traveled to perform the delivery influences its delivery fee. In contrast, the productivity of couriers is negatively impacted by delivery fees (Oliveira et al., 2021). Findings provided evidence about the factors that influence delivery fee pricing of the on-demand delivery service market in the country. By estimating a linear regression model, we hope the findings can be helpful for the pricing strategy of on-demand services and the remuneration of couriers, as well as supporting public policies to mitigate the externalities of this service.
This study is structured into five sections. After this introductory section, Section 2 presents the research approach. The results are provided in Section 3. Finally, Section 4 concludes this study with a discussion and conclusion.
Data were collected from the three main on-demand delivery service applications with coverage services in Brazilian cities. Coverage cities were identified on the website of each application. Thus, the cities with coverage from the three applications were the first selection used. From this selection, 12 cities were randomly selected in order to proceed with data collection. The selected municipalities have more than 5,00,000 inhabitants in seven state capitals (Belém-PA, Belo Horizonte-MG, Curitiba-PR, Fortaleza-CE, Recife-PE, São Paulo-SP, and Brasília-DF) and five cities in the countryside (Campinas-SP, Ribeirão Preto-SP, São José dos Campos-SP, Sorocaba-SP, and Uberlândia-MG). In addition, a random place located in the city center was chosen as a delivery destination.
Data collection consisted of initiating an order without completing the purchase. We did it for different products, classified as ready meals, snacks, desserts, supermarket or bakery products, medicines, and beverages. Previously, the types of products had been categorized (e.g., Advil ibuprofen pills as medicines). Then, the products were randomly selected, depending on their supply at the time of data collection. Once the product was established, the following data were recorded:
1) Delivery fee: value paid by the customer for the delivery service.
2) Service fee: fee paid by the customer to cover operational costs with the platform.
3) Delivery distance: distance between the establishment and the delivery destination.
4) Product price: the price of the intended product.
5) Waiting time: the time required for delivery to take place and including the preparation time of the order and the delivery time.
6) Order time: the time that the order would take place.
7) Order day: the day of the week the order would take place.
Using this procedure, we collected data from 1,440 initiated orders. Data were collected from November to December 2020. Concerning the days, Figure 1A shows that data were collected mainly on Wednesday and Tuesday, between 11 a.m. and 3 p.m. and from 6 p.m. onward (Figure 1B). Data collection was performed on different days and times to identify variations in the delivery fee. Concerning the products, we initiate orders from ready meals and snacks, such as pizza or hamburgers, desserts (ice cream or cake), and beverages (alcoholic beverages or soft drinks) (Figure 1C). Most orders had an expected waiting time of 31–40 min (Figure 1D). It is important to note that the day of the week of orders does not imply a peak day of consumers, as the time of simulated orders corresponds only to the time that the data were collected.
FIGURE 1. Data collection information: (A) week of the day of the order, (B) order time, (C) product type, (D) expected waiting time.
A linear model was estimated with the data, considering the delivery fee as the response variable (Y). Additionally, the explanatory variables are the distance, service fee, product price (continuous variables), expected waiting time, product type, weekday, and order time (categorical variables). We estimated the following models: 1) all products (M1), 2) ready meals (M2), 3) supermarket and bakery products (M3), 4) beverages (M4), and 5) medical products (M5). Since we mixed continuous and categorical variables, we standardized the regression coefficients using the l m beta package (Behrendt, 2015).
We calculated the Variance Inflation Factor (VIF) to identify multicollinearity among the explanatory variables. Finally, we verified the significance of the variables by estimating a model without the variable and comparing the estimated models using the likelihood-ratio test. We estimated the linear model in the R environment.
Table 1 shows descriptive statistics for delivery and service fees, product price, distance, and delivery time. We identified orders with free delivery and service fees in all cities considering the minimum value. The highest delivery fee (represented by the maximum value) ranged from BRL 11.70 (€1 ≈ BRL 5.3 in August 2022) in São José dos Campos to BRL 23.00 in Belém, whereas São Paulo had the highest service fee (BRL 12.30). The average delivery fee among the cities was BRL 6.34, whereas the average service fee was BRL 0.62. Concerning the product price, we observed inexpensive products available for on-demand delivery, such as in Belém (BRL 0.85) and Campinas (BRL 0.70). The minimum price in all cities is BRL 0.70, the average cost is BRL 28.20, and the maximum price is BRL 175.90. The longest distance ranged from 8.10 km in Sorocaba and São José dos Campos to 21.10 km in São Paulo. The average distance in all cities is 3.61 km. We observed different product prices, delivery, and service fees based on descriptive statistics. Moreover, delivery distances vary among the cities. Therefore, we can say that the characteristics of these continuous variables are different for the cities considered in the analysis.
Table 2 shows the estimated coefficients of the models. All models have statistical significance (F-statistics). Moreover, VIF was lower than 8 in all cases, indicating no multicollinearity between the variables. The small values of the residual standard error suggest that the models fit the data. We analyzed the results considering the intercept, the operational variables of the on-demand delivery service (distance and delivery service), and the variables concerning the order (product price, waiting time, order time, and order day).
The intercept can be interpreted as the fixed delivery fee. Except for M3, other estimated intercepts have statistical significance. In this case, the lack of statistical significance of the intercept indicates that other variables are necessary to evaluate the delivery fee of supermarkets and bakery products, which were not considered in this study. Moreover, the intercept values are positive, as expected for analysis. The fixed delivery fee varies from BRL 3.25 (all products) to BRL 14.07 (medicines products). Thus, the type of product influences the fixed delivery fee.
Concerning the operational variables of on-demand delivery service, the distance also positively affects the delivery fee, as expected. The estimated coefficients vary from BRL 0.44/km (M1 and M2) to BRL 0.83/km (M5), all statistically significant. The service fee is statistically significant only for ready meals, negatively affecting delivery fees. Thus, although some apps apply a service fee in some cities, their effect seems to be isolated when the city variable is not considered. The negative effect of the service fee on delivery fee may stimulate consumption, as you pay less to receive your products if you buy more products or pay for more expensive items.
Concerning the variables related to the order, product price positively influences the delivery fee of ready meal products. However, its influence is small, as increasing the product price by one currency unit increases the delivery fee by 0.02 for a ready meal. However, the product price negatively influenced supermarkets and bakeries, beverages, and medicines products, without statistical significance.
The waiting time did not influence the delivery fee, except for ready meals. However, the effect is negative for waiting times longer than 60 min and positive for 41–50 min waiting time, considering the waiting time of 20 min as a reference. Similarly, considering Sunday as a reference day, the order day does not affect the delivery fee, except for orders for ready meals, supermarket, and bakery products on Friday. Moreover, the order day influences the delivery fee for supermarket and bakery products on all days except for Monday. Finally, considering 11 a.m.–3 p.m. as a reference time, medical products are negatively influenced by time. In contrast, this influence is negative only for ready-meal orders requested from 6 p.m.
Based on these results, we concluded that delivery fees vary according to the product type. Additionally, concerning ready meals, the following variables have a positive effect on delivery fee: distance, product price, 41–50 min waiting time, and orders on Friday. In contrast, the service fee, 60 min waiting time, orders on Wednesday, and orders from 6 p.m. have a negative effect on the delivery fee. Concerning supermarket and bakery products, distance and orders on Wednesday, Tuesday, Thursday, Friday, and Saturday have a positive effect. Regarding beverages, distance had a positive effect on delivery fees. Finally, concerning medicinal products, distance has a positive effect, while orders on Tuesday, from 3 p.m., have a negative effect.
Table 3 shows the p-value of comparing the full model and removing each variable considered in the modeling. As the p-value is below 0.05, the full model is better than removing the specific variable. Thus, we observe that the distance improves the fit of the model, regardless of product type. Additionally, service fees, product prices, waiting times, order days, and order times improve the fit of the ready-meal model (M2). Moreover, the waiting time and order day improve the fit of the supermarket and bakery product model (M3) and beverage model (M4). Finally, order day and order time improve the fit of the medicine products model (M5). Thus, we observed that some variables are essential for delivery fee pricing to attract consumers to on-demand delivery services, such as service fees and product prices for ready meals; waiting time for supermarket, bakery, and beverage products; order day for supermarket, bakery, beverage, and medical products; order time for ready meals and medical products.
The positive influence of distance on delivery fees identified in this study converges with other results by Tong et al. (2020) and Mello et al. (2022). In addition, the product price of ready meals positively influenced the delivery fees, confirming the findings of Tong et al. (2020). However, most studies dealt with on-demand ready-meal delivery services. Thus, this study advances the literature, showing that the product type has a positive and diverse effect on delivery fees, a fact not explored by other researchers.
Considering the order day, the estimated coefficient for ready meals is positive on Fridays and negative for Wednesdays and Thursdays. Furthermore, Tong et al. (2020) found lower delivery fees on weekends, as also observed in this study. Finally, the service fee negatively influences the delivery fee of ready meals, indicating that pricing strategies reduce the delivery fee, stimulating consumption. As mentioned, the service fee is one pricing strategy observed in some cities, yet there are no clear rules on its structure.
Based on data from the three leading on-demand delivery service platforms in Brazil, the problem addressed in this study concerns how the factors related to on-demand delivery requests affect the delivery fee. Thus, we analyzed the factors that influence the delivery fee pricing of on-demand delivery services and explored the impact of delivery fee strategies on the remuneration of couriers.
The coefficients of the estimated models showed that delivery fee pricing is diverse across product types. Moreover, the distance positively influences the delivery fee, regardless of the product type. Furthermore, findings indicated that waiting time and order day affect the delivery fee for some products type. Thus, if you buy more, you may pay less for the delivery fee, regardless of the product prices. Tong et al. (2020) stated that dynamic pricing strategies are part of the market strategy for increasing consumption. However, analyzing the delivery fee considering the amount paid by the consumer did not provide an accurate understanding of the delivery pricing strategies, as the delivery fee paid by consumers is not the delivery fee received by couriers.
Based on the results, we explored the impact of delivery fee strategies on the remuneration of couriers. As the revenue of couriers is directly related to the number of deliveries and the distance traveled to perform the delivery, it is essential to explore the consequences of pricing the delivery fee for couriers. In Brazil, the delivery services of online applications were recently regulated by law. Despite this, the workers have not achieved fair remuneration. Moreover, studies have pointed out the long working hours required to obtain a minimum wage (Mello et al., 2022).
In contrast, when the remuneration of couriers is fair, their productivity, measured by the number of deliveries made per day, is low (Oliveira et al., 2021). For many people who work as couriers for on-demand delivery services, this is a second job or a way to leave unemployment, where the rates are high in Brazil. As the pricing of remuneration is entirely unknown for couriers, the need for cash leads many of them to accept deliveries with minimal profit.
In this way, as the delivery fee is a factor that increases the use of on-demand delivery services, it can also be a factor that regulates the number of couriers available to carry out the delivery. However, the delivery time may increase with high demand and the low number of couriers available to perform deliveries. Therefore, finding the break-even point in the delivery fee pricing is essential to meet the growing demand while providing fair remuneration to couriers. However, finding the balance point is not easy when the delivery fee is a factor in attracting users to on-demand delivery services. For example, it is usual for on-demand delivery service apps in Brazil to offer free delivery. As a result, there are some users who only use the service when the delivery cost is free. In this way, there is a vicious circle fueled by the stimulus of free shipping to increase on-demand delivery services and the low remuneration of couriers because it is an activity often developed as a second job or when the individual is unemployed.
The results indicated that distance is a factor that influences the delivery fee pricing of any product type. Thus, knowing the operational costs of delivery per kilometer allows the couriers to understand the profits better and, consequently, improve their remuneration. In this way, the working day becomes a strategic instrument to obtain a fair income instead of a minimum payment.
For future studies, we suggest developing a tool for couriers to analyze delivery profit considering operational delivery costs. Moreover, as data were collected during the COVID-19 pandemic, where social distance measures may have influenced the increase in the demand for on-demand delivery services, further analysis is needed to verify if the variables analyzed remain significant after the COVID-19 pandemic. In addition, the modeling can incorporate other factors and use different strategies to investigate the influence of dummy variables related to the product type. Finally, further studies could investigate consumer rates and delivery fees.
The raw data supporting the conclusions of this article will be made available by the author without undue reservation.
All authors listed have made a substantial, direct, and intellectual contribution to the work and approved it for publication.
The authors thank CNPq and FACEPE for supporting this research. Moreover, the authors thank Francisco Gildemir Ferreira da Silva for the modeling support.
The authors declare that the research was conducted in the absence of any commercial or financial relationships that could be construed as a potential conflict of interest.
All claims expressed in this article are solely those of the authors and do not necessarily represent those of their affiliated organizations or those of the publisher, the editors, and the reviewers. Any product that may be evaluated in this article, or claim that may be made by its manufacturer, is not guaranteed or endorsed by the publisher.
Abilio, L. C., Grohmann, R., and Weiss, H. C. (2021). Struggles of delivery workers in Brazil: Working conditions and collective organization during the pandemic. J. Labor Soc. 24 (4), 598–616. doi:10.1163/24714607-bja10012
Aguilera, A., Dablanc, L., and Rallet, A. (2018). L’envers et l’endroit des plateformes de livraison instantanée. Réseaux 212 (6), 23–49. doi:10.3917/res.212.0023
Allen, J., Piecyk, M., Cherrett, T., Juhari, M. N., McLeod, F., Piotrowska, M., et al. (2021). Understanding the transport and CO2 impacts of on-demand meal deliveries: A london case study. Cities 108, 102973. doi:10.1016/j.cities.2020.102973
Becerril-Arreola, R., Leng, M., and Parlar, M. (2013). Online retailers’ promotional pricing, free-shipping threshold, and inventory decisions: A simulation-based analysis. Eur. J. Operational Res. 230 (2), 272–283. doi:10.1016/j.ejor.2013.04.006
Behrendt, S. (2015). Package “lm.beta”. Available at: https://cran.r-project.org/web/packages/lm.beta/lm.beta.pdf (Accessed October 28, 2022).
Bjørgen, A., Bjerkan, K. Y., and Hjelkrem, O. A. (2021). E-groceries: Sustainable last mile distribution in city planning. Res. Transp. Econ. 87, 100805. doi:10.1016/j.retrec.2019.100805
Budd, L., and Ison, S. (2020). Responsible transport: A post-COVID agenda for transport policy and practice. Transp. Res. Interdiscip. Perspect. 6, 100151. doi:10.1016/j.trip.2020.100151
Chen H, H.-K., Chou, H.-W., and Hung, S.-C. (2019). Interrelationships between behaviour intention and its influential factors for consumers of motorcycle express cargo delivery service. Transp. A Transp. Sci. 15 (2), 526–555. doi:10.1080/23249935.2018.1509401
Chen, M., Hu, M., and Wang, J. (2019). Food delivery service and restaurant: Friend or foe? Manag. Sci.68 (9), 6355–7064. doi:10.1287/mnsc.2021.4245
Choi, T.-M., Guo, S., Liu, N., and Shi, X. (2020). Optimal pricing in on-demand-service-platform-operations with hired agents and risk-sensitive customers in the blockchain era. Eur. J. Operational Res. 284 (3), 1031–1042. doi:10.1016/j.ejor.2020.01.049
Chung, Y., Song, T.-J., and Yoon, B.-J. (2014). Injury severity in delivery-motorcycle to vehicle crashes in the Seoul metropolitan area. Accid. Analysis Prev. 62, 79–86. doi:10.1016/j.aap.2013.08.024
Dablanc, L., Morganti, E., Arvidsson, N., Woxenius, J., Browne, M., and Saidi, N. (2017). The rise of on-demand “Instant Deliveries” in European cities. Supply Chain Forum Int. J. 18 (4), 203–217. doi:10.1080/16258312.2017.1375375
Goods, C., Veen, A., and Barratt, T. (2019). Is your gig any good?” Analyzing job quality in the Australian platform-based food-delivery sector. J. Industrial Relat. 61 (4), 502–527. doi:10.1177/0022185618817069
Gregory, K. (2021). My life is more valuable than this”: Understanding risk among on-demand food couriers in edinburgh. Work, Employ. Soc. 35 (2), 316–331. doi:10.1177/0950017020969593
Huang, W., Zhao, Z., An, X., and Min, G., (2020). “Dynamic scheduling for urban instant delivery with strict deadlines,” in Proceedings of the ICC 2020 - 2020 IEEE International Conference on Communications (ICC). ICC 2020 - 2020 IEEE International Conference on Communications (ICC), Duplin, Ireland, 07-11 June 2020, 1–6. doi:10.1109/ICC40277.2020.9148877
Lewis, M., Singh, V., and Fay, S. (2006). An empirical study of the impact of nonlinear shipping and handling fees on purchase incidence and expenditure decisions. Mark. Sci. 25 (1), 51–64. doi:10.1287/mksc.1050.0150
Lewis, M. (2006). The effect of shipping fees on customer acquisition, customer retention, and purchase quantities. J. Retail. 82 (1), 13–23. doi:10.1016/j.jretai.2005.11.005
Li, Z., and Wang, G. (2021). Regulating powerful platforms: Evidence from commission fee caps in on-demand services. SSRN J. doi:10.2139/ssrn.3871514
Mello, C. A., De Oliveira Carneiro, C. M., Alves Maia, M. L., De Oliveira, L. K., and Goncalves Ferreira de Araujo, G. (2022). Análise da taxa de entrega de aplicativos de entrega instantânea e a remuneração dos entregadores em cidades brasileiras. Transportes 30 (2), 1–12. doi:10.14295/transportes.v30i2.2641
Nguyen, D. N., de Leeuw, S., Dullaert, W., and Foubert, B. P. J. (2019). What is the right delivery option for you? Consumer preferences for delivery attributes in online retailing. J. Bus. Logist. 40 (4), 299–321. doi:10.1111/jbl.12210
Nordzi, M. A. W. B., Hatta, M. F. M., and Dahlan, A. R. A. (2018). “GoBeli: Providing runner platform for personal shopper and delivery services for urban community,” in Proceedings of the 2018 International Conference on Information and Communication Technology for the Muslim World (ICT4M). 2018 International Conference on Information and Communication Technology for the Muslim World (ICT4M), Kuala Lumpur, Malaysia, 23-25 July 2018, 202–207. doi:10.1109/ICT4M.2018.00045
Oliveira, L. K., de Oliveira, I. K., de Oliveira Leite Nascimento, C., de Oliveira Lobo Cordeiro, C. H., Meira, L. H., Rabay, L., et al. (2021). Identification of factors to improve the productivity and working conditions of motorcycle couriers in Belo Horizonte, Brazil. Case Stud. Transp. Policy 9 (4), 1737–1745. doi:10.1016/j.cstp.2021.09.003
Statista (2022). Online food delivery - Brazil. Available at: https://www.statista.com/outlook/dmo/eservices/online-food-delivery/brazil#revenue (Accessed October 28, 2022).
Tassinari, A., and Maccarrone, V. (2020). Riders on the storm: Workplace solidarity among gig economy couriers in Italy and the UK. Work, Employ. Soc. 34 (1), 35–54. doi:10.1177/0950017019862954
Taylor, T. A. (2018). On-demand service platforms. Manuf. Serv. Oper. Manag. 20 (4), 704–720. doi:10.1287/msom.2017.0678
Tong, T., Dai, H., Xiao, Q., and Yan, N. (2020). Will dynamic pricing outperform? Theoretical analysis and empirical evidence from O2O on-demand food service market. Int. J. Prod. Econ. 219, 375–385. doi:10.1016/j.ijpe.2019.07.010
Wang, Z., and He, S. Y. (2021). Impacts of food accessibility and built environment on on-demand food delivery usage. Transp. Res. Part D Transp. Environ. 100, 103017. doi:10.1016/j.trd.2021.103017
Xu, X. (2021). What are customers commenting on, and how is their satisfaction affected? Decis. Support Syst. 142, 113467. doi:10.1016/j.dss.2020.113467
Zhang, Y., Liu, Y., Li, G., Ding, Y., Chen, N., Zhang, H., et al. (2019). Route prediction for instant delivery. Proc. ACM Interact. Mob. Wearable Ubiquitous Technol. 3 (3), 1–25. doi:10.1145/3351282
Keywords: on-demand delivery service, instant delivery, delivery fee, influential factors, Brazil
Citation: De Oliveira LK, Mello CAd, Carneiro CMdO, Costa TERd, Araújo GGFd and Maia MLA (2022) Identification of factors that influence the delivery fee pricing of on-demand delivery services. Front. Future Transp. 3:1031021. doi: 10.3389/ffutr.2022.1031021
Received: 29 August 2022; Accepted: 29 November 2022;
Published: 14 December 2022.
Edited by:
Russell George Thompson, The University of Melbourne, AustraliaReviewed by:
Nilesh Anand, Rotterdam University of Applied Sciences, NetherlandsCopyright © 2022 De Oliveira, Mello, Carneiro, Costa, Araújo and Maia. This is an open-access article distributed under the terms of the Creative Commons Attribution License (CC BY). The use, distribution or reproduction in other forums is permitted, provided the original author(s) and the copyright owner(s) are credited and that the original publication in this journal is cited, in accordance with accepted academic practice. No use, distribution or reproduction is permitted which does not comply with these terms.
*Correspondence: Leise Kelli De Oliveira, bGVpc2VAZXRnLnVmbWcuYnI=
Disclaimer: All claims expressed in this article are solely those of the authors and do not necessarily represent those of their affiliated organizations, or those of the publisher, the editors and the reviewers. Any product that may be evaluated in this article or claim that may be made by its manufacturer is not guaranteed or endorsed by the publisher.
Research integrity at Frontiers
Learn more about the work of our research integrity team to safeguard the quality of each article we publish.