- 1Plant Molecular & Cellular Biology Graduate Program, University of Florida, Gainesville, FL, United States
- 2Department of Plant Pathology, University of Florida, Gainesville, FL, United States
- 3United States Department of Agriculture, Agricultural Research Service, Tropical Agriculture Research Station, Mayagüez, PR, United States
- 4Department of Microbiology & Cell Science, University of Florida, Gainesville, FL, United States
- 5University of Florida Genetics Institute, Gainesville, FL, United States
In warm and humid regions, the productivity of sorghum is significantly limited by the fungal hemibiotrophic pathogen Colletotrichum sublineola, the causal agent of anthracnose, a problematic disease of sorghum (Sorghum bicolor (L.) Moench) that can result in grain and biomass yield losses of up to 50%. Despite available genomic resources of both the host and fungal pathogen, the molecular basis of sorghum−C. sublineola interactions are poorly understood. By employing a dual-RNA sequencing approach, the molecular crosstalk between sorghum and C. sublineola can be elucidated. In this study, we examined the transcriptomes of four resistant sorghum accessions from the sorghum association panel (SAP) at varying time points post-infection with C. sublineola. Approximately 0.3% and 93% of the reads mapped to the genomes of C. sublineola and Sorghum bicolor, respectively. Expression profiling of in vitro versus in planta C. sublineola at 1-, 3-, and 5-days post-infection (dpi) indicated that genes encoding secreted candidate effectors, carbohydrate-active enzymes (CAZymes), and membrane transporters increased in expression during the transition from the biotrophic to the necrotrophic phase (3 dpi). The hallmark of the pathogen-associated molecular pattern (PAMP)-triggered immunity in sorghum includes the production of reactive oxygen species (ROS) and phytoalexins. The majority of effector candidates secreted by C. sublineola were predicted to be localized in the host apoplast, where they could interfere with the PAMP-triggered immunity response, specifically in the host ROS signaling pathway. The genes encoding critical molecular factors influencing pathogenicity identified in this study are a useful resource for subsequent genetic experiments aimed at validating their contributions to pathogen virulence. This comprehensive study not only provides a better understanding of the biology of C. sublineola but also supports the long-term goal of developing resistant sorghum cultivars.
1 Introduction
Colletotrichum sublineola (Henn.) in Kabat & Bubak, the causative agent of anthracnose in sorghum (Sorghum bicolor (L.) Moench), is a hemibiotrophic pathogen. It is characterized by initially infecting living host tissue (biotrophic phase) and subsequently transitioning to killing and feeding on dead host tissue (necrotrophic phase) (Figure 1). C. sublineola infection of sorghum leaves manifests itself by the appearance of lesions with dark red or tan margins (Pastor-Corrales and Frederiksen, 1978). The necrotic parts of the surface contain acervuli that display dark setae (protruding specialized hyphae with thick melanized walls) (Pastor-Corrales and Frederiksen, 1978). The progression of anthracnose into the stem gives rise to red rot, which is characterized by the appearance of red marbling in the stalk and subsequently weakens the stem’s integrity (Lebeau et al., 1951; Harris et al., 1964; Tesso et al., 2012). In the later stages of development, infection can be observed in the rachis, panicle branches, and seeds, leading to a direct reduction in grain yield (Harris et al., 1964). Efficient development of resistant cultivars and effective disease management strategies against anthracnose benefit from a comprehensive understanding of the interactions between sorghum and C. sublineola, which is presently incomplete (Stutts and Vermerris, 2020).
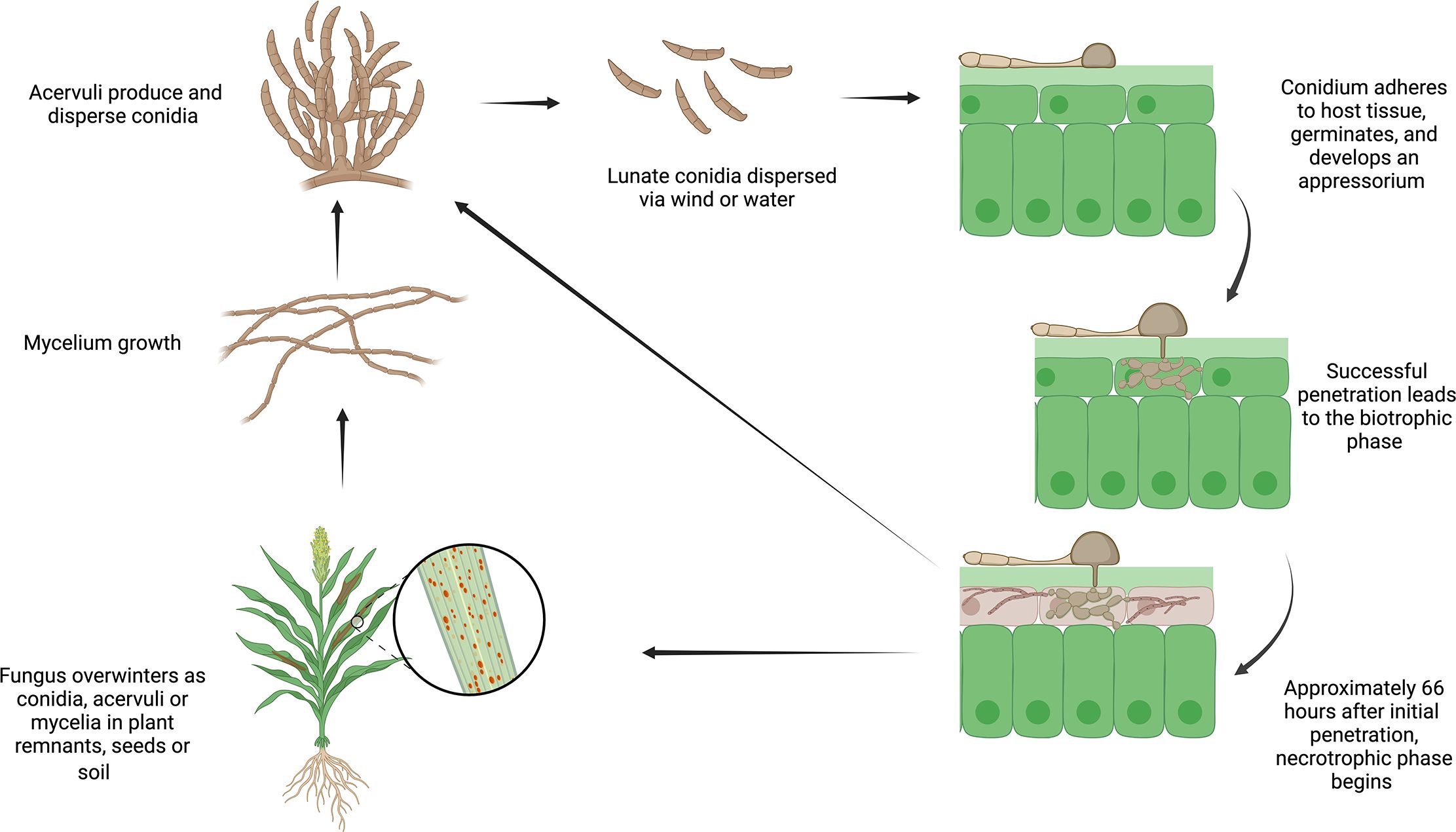
Figure 1 The life cycle of Colletotrichum sublineola, adapted from Crouch and Beirn (2009). Figure created with BioRender.com.
The evolutionary “arms race” between plants and pathogens is characterized by a continual battle for survival and adaptation (Jones and Dangl, 2006). This interaction is characterized by the initiation of a pattern-triggered immune response (PTI) upon the recognition of pathogen/damage-associated molecular patterns (PAMPs/DAMPs) (Jones and Dangl, 2006; Win et al., 2012). Conversely, pathogens can overcome this defense layer by releasing effectors that facilitate the colonization of the host. Effectors are proteins that disrupt the structure or processes of the host cell, suppressing defense responses and increasing access to nutrients (Win et al., 2012). Detection of pathogen effectors by receptors encoded by the host’s resistance (R) genes activates the second defense layer, known as effector-triggered immunity (ETI), which may lead to a hypersensitive reaction (HR) (Win et al., 2012). To date, there have been several studies reporting sorghum’s resistance responses in different genotypes that demonstrated the existence of multiple resistance mechanisms (reviewed by Stutts and Vermerris, 2020 and Abreha et al., 2021). However, the identification and characterization of key molecular determinants of C. sublineola pathogenicity have yet to be elucidated.
Past studies on the diversity of C. sublineola pathotypes uncovered differences in diversity that are dependent on year, location, and/or presence of dominant haplotypes (Rosewich et al., 1998; Valèrio et al., 2005; Thakur et al., 2007; Moore et al., 2008; Prom et al., 2024). The high variability and genetic diversity of C. sublineola make it challenging to breed sorghum cultivars that are reliably resistant across years and locations. To enhance sorghum resistance against the pathogen, continuous improvements in genome annotation, re-sequencing of various host genotypes and pathogen strains, and functional genomics approaches are crucial. The availability of genome sequences for various pathotypes of C. sublineola enables further genome sequencing and transcriptomics studies to identify expressed genes during different infection stages (Baroncelli et al., 2014; Buiate et al., 2017; Baldrich et al., 2021).
In a notable comparative genomic study, gene models from two strains of the closely related species C. graminicola and C. sublineola, pathogens of maize and sorghum, respectively, were analyzed to identify genes that are not conserved, indicating potential candidates for involvement in host specificity (Buiate et al., 2017). The genomes of C. graminicola M1.001 and C. sublineola CgSl1 harbored approximately 1,000 genes encoding predicted proteins that were unique to each species, termed non-conserved proteins (NCPs). A majority (>65%) of the NCPs in both strains did not match any conserved protein family (Pfam) categories. Among the minority of NCPs with Pfam classifications, the largest groups consisted of transporters, cytochrome P450s, secondary metabolite-associated proteins, carbohydrate-active enzymes (CAZymes), and transcription factors. This highlights the importance of adapting to diverse aspects of each host environment and in the secretion or evasion of toxic secondary metabolites (Buiate et al., 2017).
While significant progress has been made in recent years regarding disease resistance loci and defense mechanisms, our understanding of the interactions between sorghum and C. sublineola remains incomplete. Advances in genomics, transcriptomics, proteomics, and metabolomics studies can help elucidate the complex molecular crosstalk during sorghum–C. sublineola interactions (Stutts and Vermerris, 2020). However, it is only recently that transcriptomics (Gan et al., 2013; Buiate et al., 2017; Wang et al., 2020; Wolf et al., 2024) and metabolomics (Tugizimana et al., 2019) approaches have started to be applied to study the interactions between sorghum and C. sublineola.
Dual-RNA-sequencing offers a comprehensive insight into host–pathogen interactions (Westermann et al., 2012). Tierney et al. (2012) reported the first successful application of dual RNA-seq to a eukaryotic interaction model between Candida albicans and dendritic cells in its mouse host. This approach has also been utilized in sorghum–Bipolaris sorghicola interaction via de novo assembly of the pathogen transcriptome to identify key genes in the plant–pathogen interaction (Yazawa et al., 2013). Here, we present a dual RNA-sequencing approach to examine the interaction between different sorghum genotypes and C. sublineola at the molecular level at different stages of infection. Furthermore, the infection process was observed in planta with epi-fluorescence microscopy.
2 Materials and methods
2.1 Colletotrichum sublineola growth conditions and infection in sorghum for RNA-sequencing
C. sublineola isolates were collected from sorghum leaf tissue at the UF North Florida Research and Education Center-Suwannee Valley near Live Oak, FL (30.313277 N, 82.902158 W) according to Felderhoff et al. (2016). Isolates were cultured on potato dextrose agar (PDA) at room temperature for 10 to 14 days. To create a conidial suspension, 1–5 mL of sterile ddH2O was added to the Petri dish, and the agar surface was gently agitated using a sterile L-shaped spreader. The conidial concentration in the suspension was determined using a hemocytometer and adjusted to a final concentration of 106 conidia/mL. A mock solution was prepared using sterile water. Both the conidial and mock solutions were supplemented with three drops of Tween-20 (Sigma-Aldrich, St Louis, MO) for a final volume of 5 mL and stirred at low speed for 10 minutes. Subsequently, these solutions were transferred into spray bottles according to the respective treatments.
The sorghum accessions SC17, SC110, SC1033, and SC1330 were selected for analysis because they have different mechanisms to combat anthracnose. Accessions SC17, SC110, and SC1330 have resistance alleles for one of the three anthracnose-resistance loci on chromosome 5 (Supplementary Table 1), whereas SC1033 is susceptible at all three loci but may have resistance alleles at other loci (Cuevas et al., 2018). Since anthracnose resistance is a multigenic trait, these alleles offer only partial resistance and do not entirely prevent disease (Supplementary Figure 1). Accessions were cultivated in a greenhouse in a split-split plot design for accession and time points (1, 3, 5 dpi) with four biological replicates (n = 4 per time point) as described by Wolf et al. (2024). Mock-inoculated and inoculated treatments were grown in separate plastic tents to prevent contamination of the control treatment. At the fifth-leaf stage, plants were sprayed with 1 mL of the conidial suspension or the mock-inoculation solution.
2.2 Isolation of fungal and plant RNA and library preparation for RNA-sequencing
RNA was extracted from C. sublineola cultured for two weeks on PDA plates with a nylon membrane (2 µm) overlay (Sigma-Aldrich, St. Louis, MO) and fungal samples were subjected to two chloroform extractions prior to RNA extraction as described by Schumann et al. (2013). Mock-inoculated control and inoculated leaf tissue samples were collected from all four sorghum genotypes at 1, 3, and 5 dpi. Tissue was immediately frozen in liquid nitrogen and homogenized with a BeadBlaster24 (Benchmark Scientific, Sayreville, NJ) and subjected to RNA extraction as described by Wolf et al. (2024). Approximately 1–3 μg of total RNA per sample was sent to Novogene, Inc. (Sacramento, CA) for library preparation and paired-end sequencing with the Illumina NovaSeq 6000 PE150 sequencing platform. The transcripts obtained from C. sublineola cultured on PDA plates were referred to as in vitro transcripts and served as a reference for the transcripts obtained from C. sublineola growing in infected sorghum tissue, which were referred to as in planta transcripts.
2.3 Bioinformatics analysis
Raw reads were processed with FASTQC (v0.11.7) to remove low-quality reads (Andrews, 2010). Trimmomatic (v.0.35) was used to eliminate adapter sequences and to improve read quality (Bolger et al., 2014). Reads with a Phred score > 20 and read length > 50 bp were selected for downstream analysis (Corchete et al., 2020). The subsequent trimmed and filtered pair-end reads were aligned to the BTx623 sorghum reference genome v. 3.1 (Paterson et al., 2009; McCormick et al., 2018) and the C. sublineola reference genome (Baroncelli et al., 2014) using HiSat2 (v2.2.1) (Kim et al., 2015). The number of reads aligned was counted using HTSeq (v.0.11.2) in combination with EdgeR’s (v3.28.0) trimmed mean of M values (TMM) to normalize the data for effective library size with TMM normalization, RNA composition, sequencing depth, and corrected for multiple testing (Robinson et al., 2010; Anders et al., 2015). A generalized linear model (GLM) quasi-likelihood F-test with a false discovery rate (FDR) of 0.05 was used to identify differentially expressed genes (DEGs) (Benjamini and Hochberg, 1995).
2.4 KEGG enrichment analysis for differentially expressed sorghum genes
KEGG Orthology Based Annotation System (KOBAS) 2.0 was used for enrichment analysis of the differentially expressed genes (Bu et al., 2021). KOBAS offers pathway enrichment analysis by assigning orthology to input genes and maps them to Kyoto Encyclopedia of Genes and Genomes (KEGG) pathways (Kanehisa et al., 2004). A Fisher’s exact test was used to determine the significantly enriched pathways while controlling the FDR (Benjamini and Hochberg, 1995). Significantly enriched pathways were determined based on adjusted p-values or q-values below a predefined threshold (FDR < 0.05).
2.5 Gene ontology annotation of fungal up-regulated genes
Significantly up-regulated genes at the different sampling time points were assigned Gene Ontology (GO) (Gene Ontology Consortium, 2021) terms using BLAST2GO (Götz et al., 2008) in OmicsBox software (OmicsBox, 2019). BLASTP (Altschul et al., 1990) was utilized to identify amino acid sequences with similarities to the query sequences in the NCBI protein sequence database with an e-value cutoff of 1 × 10−3 (Buchfink et al., 2021), then GO mapping was used to retrieve GO terms associated with the BLAST hits (Ashburner et al., 2000; Götz et al., 2008; OmicsBox, 2019). GO annotations were assigned to the query sequences based on the GO mapping results using default filters (Ashburner et al., 2000; Götz et al., 2008; OmicsBox, 2019). These results were categorized using GO-Slim to summarize GO annotations (Götz et al., 2008; OmicsBox, 2019). For each time point comparison, BLAST2GO was used to calculate the abundance of GO classifications for query sequences of the genes that were statistically significantly up-regulated.
2.6 Fungal effector prediction
Effector proteins were predicted using a streamlined bioinformatics analysis according to the procedure of Lu et al. (2022). First, predicted proteins were analyzed for the presence of signal peptides with SignalP 5.0 (Almagro Armenteros et al., 2019) and extracellular localization with WoLF-PSORT (Horton et al., 2007). Next, DeepTMHMM (Hallgren et al., 2022) and PredGPI (Pierleoni et al., 2008) were used to exclude proteins with transmembrane helices and glycosylphosphatidylinositol (GPI) anchors, respectively. EffectorP 3.0 was used to identify and categorize predicted effector proteins secreted into the apoplast, cytoplasm, or both (Sperschneider and Dodds, 2022).
2.7 Characterization of putative effectors and structural network analysis
The amino acid sequences of annotated and unannotated (no signal peptide, no transmembrane domain, no assigned function) putative effector proteins were analyzed with SignalP v5.0 (Almagro Arementeros et al., 2019) to identify predicted cleavage sites and N-terminal signal peptides. All subsequent analyses were performed on effector sequences lacking their signal peptide. Nuclear localization sequences (NLS) were predicted for all effectors with NLStradamus (Nguyen Ba et al., 2009). Structural modeling of effectors was performed with AlphaFold2 (Jumper et al., 2021; Mirdita et al., 2022). A tolerance of 0.5 was employed for alphafold2_ptm monomer prediction, and models were ranked based on predicted LDDT (pLDDT) scores to determine the best model. The top-ranked AlphaFold model was utilized for protein structural network construction and FoldSeek analyses (van Kempen et al., 2024). Protein structural networks were constructed as described by Teulet et al. (2023), using a template modeling (TM)-score threshold of 0.6. Protein structural networks were visualized with the R package IGRAPH employing the Louvain community detection method (Csárdi et al., 2006; Blondel et al., 2008).
2.8 Identification of fungal proteins predicted to be carbohydrate-active enzymes
Identification of carbohydrate-active enzymes (CAZymes) was performed using dbCAN3 (https://bcb.unl.edu/dbCAN2/) (Zheng et al., 2023). The dbCAN3 web server provides Hidden Markov Models of Emission for Recognition (HMMER) search of the dbCAN HMM database (e-value <1 × 10–15 and coverage >0.35) (Finn et al., 2011), and a DIAMOND (Buchfink et al., 2015) search of the CAZy pre-annotated CAZyme sequence database (e-value <10–102). CAZyme families have been defined and classified by the CAZy database (Lombard et al., 2014), forming six major classes: glycosyltransferases (GTs), glycoside hydrolases (GHs), polysaccharide lyases (PLs), carbohydrate esterases (CEs), carbohydrate-binding modules (CBM) and enzymes for the auxiliary activities (AAs).
2.9 Classification of membrane transporters
Predicted membrane transporters were classified based on the GO annotations (Götz et al., 2008) related to transmembrane transport (GO:0055085) and transporter activity (GO:0005215).
2.10 Pathogen-Host Interaction database
To identify similarities to proteins indicative of known pathogenicity and virulence factors present in the Pathogen-Host Interaction (PHI) database (www.phi-base.org) (Winnenburg et al., 2006), predicted C. sublineola protein sequences were used as queries in PHIB-BLAST (http://phi-blast.phi-base.org/) (Urban et al., 2019) version 4.16 with an e-value cutoff of 1 × 10−5.
2.11 Identification of biosynthetic gene clusters
Biosynthetic gene clusters (BGCs) in C. sublineola were identified using antiSMASH version 7.1.0 for fungi (Blin et al., 2023). Genome sequences of interest were uploaded by NCBI accession number and BGCs were detected using relaxed detection strictness. ClusterBLAST (Blin et al., 2023) was utilized to determine similar BGCs in other fungal pathogens.
2.12 Aniline blue staining of infected sorghum leaf tissue
SC1033 plants were grown in a greenhouse and leaves were collected at the fifth-leaf stage. Leaves were cut into sections (7.5 × 2.5 cm2), placed in a Petri dish, and drop-inoculated using a syringe with 1 mL of a conidial suspension prepared as described previously. Leaf tissue (n = 3) was collected for fixation and staining at 1-, 3-, and 5 days post-inoculation based on the protocol by Bhadauria et al. (2010) and Hood and Shew (1996). At each time point, leaf tissue was placed in a fixative solution consisting of 60% (v/v) methanol, 30% (v/v) chloroform, and 10% (v/v) glacial acetic acid until required for staining. Leaf samples were rehydrated by immersion in an ethanol series with decreasing concentrations (100, 80, 70, and 50 (v/v) % in water). Leaf tissue was then placed in 1M KOH solution in individual 1.5 mL microcentrifuge tubes and incubated in a thermoblock (Benchmark Scientific, Sayreville, NJ) at 100°C for 15 minutes. The leaf tissue was washed three times for 15 minutes with deionized water. Samples were stained with 0.05% (w/v) aniline blue (Sigma-Aldrich, St Louis, MO) in 150 mM K2HPO4 at pH 9.5 overnight. Leaves were then de-stained in 150 mM K2HPO4 at pH 9.5, mounted on a microscope slide, and covered with a cover slip. The infection process was observed using a Leica DMR compound epi-fluorescence microscope (Leica Microsystems, Wetzlar, Germany) with a narrow UV long pass (LP) filter set (355–375 nm excitation, and 400LP nm emission). Images were captured with a Leica DFC450 C CCD camera using Leica Application Suite X software v.4.8.
3 Results
3.1 RNA-Seq mapping statistics of reads mapped to the sorghum and C. sublineola genomes
To elucidate the sorghum responses to C. sublineola in inoculated leaf tissue and to profile the gene expression dynamics of C. sublineola throughout the infection process, we performed a dual RNA-seq approach on tissue samples collected at three time points. Approximately 42 million reads obtained from individual inoculated and mock-inoculated samples were mapped to the Sorghum bicolor BTx623 reference genome v3.1.1 (Paterson et al., 2009; McCormick et al., 2018) (Supplementary Data 1). Additionally, an average of 126,000 reads were mapped to the C. sublineola genome (Baroncelli et al., 2014) from in planta transcripts (Supplementary Data 1). This meant that, on average, 93% of the reads from inoculated and mock-inoculated samples mapped to the sorghum genome, and 0.3% of reads mapped to the C. sublineola genome (Supplementary Data 1), the difference reflective of the fact that the fungal cells were far outnumbered by plant cells in the leaf tissue used for RNA extraction. In parallel to the in planta analysis, transcriptomic data were gathered from the C. sublineola isolate cultivated in vitro. This dataset served as a reference to delineate differences in expression relative to growth in planta (Supplementary Data 1).
To analyze the relatedness of the biological replicates and gene expression patterns between samples from both the host and pathogen, a principal component analysis (PCA) was conducted using OmicsBox. The PC score plot of sorghum samples revealed distinct gene expression patterns based on genotype (Figure 2A). The first two principal components (PC1 and PC2) explained 16% and 14% of the total variance in the dataset, respectively. The PCA of C. sublineola transcripts indicated distinct gene expression patterns in in vitro (control) and in planta (inoculated) samples (Figure 2B). Almost all of the variance (96%) in the dataset was captured in PC1. In planta samples from different time points overlapped in the PC score plot (Figure 2B; Supplementary Figure 2). Notably, the overlap of 3 dpi samples with 1 dpi and 5 dpi samples indicated overall similar expression profiles. The larger library size of one replicate at 3 dpi likely caused it to be an outlier. The expression profile of the in planta samples by time point explained 43% of the variance (PC1) (Supplementary Figure 2).
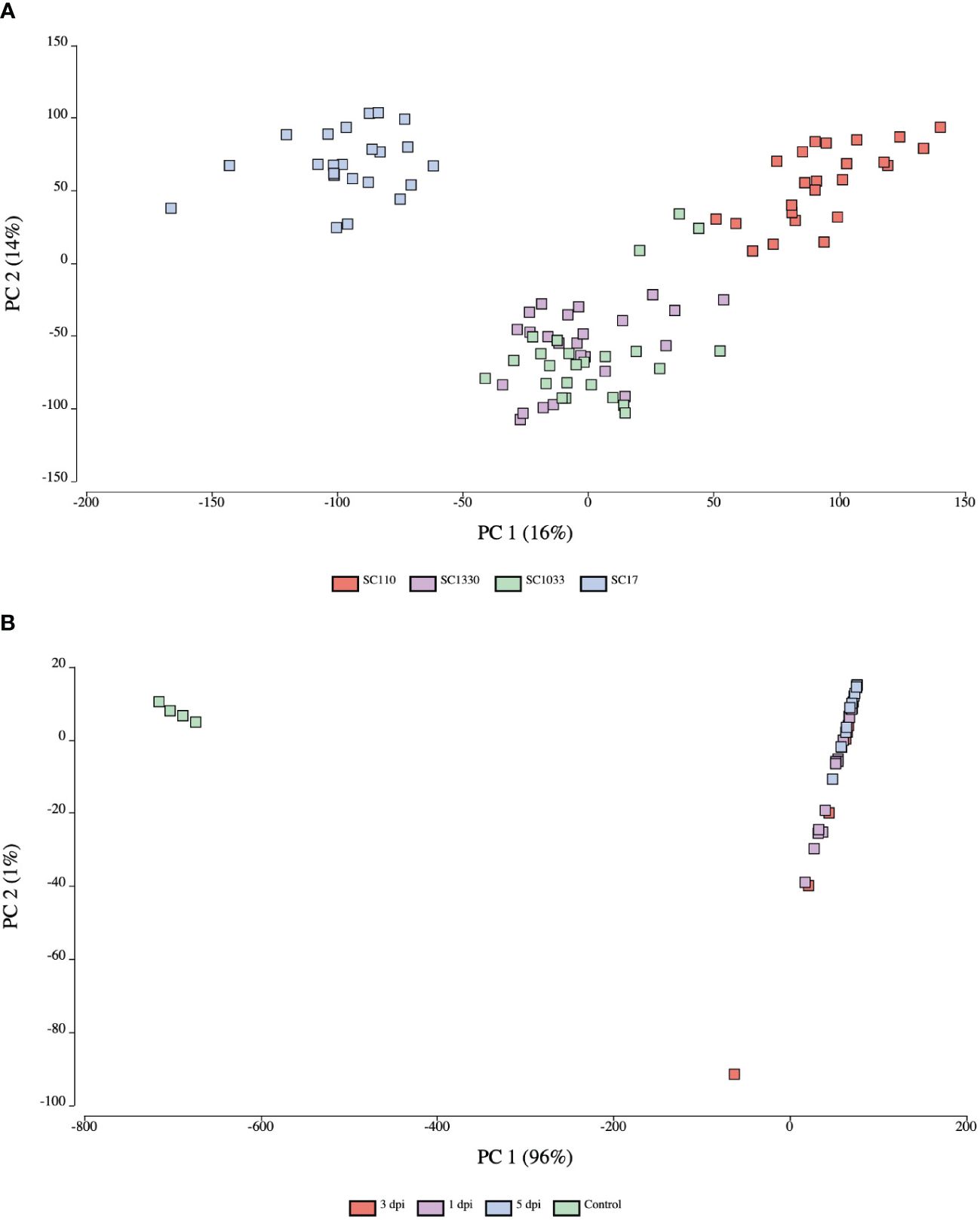
Figure 2 Principal component score plots of expression profiles from host and pathogen samples. (A) Sorghum samples clustered by genotype: SC17 (blue), SC110 (red), SC1033 (green), and SC1330 (purple). (B) C. sublineola samples clustered by in planta (inoculated) and in vitro (control) (green) samples. Inoculated samples were collected at 1 dpi (purple), 3 dpi (red), and 5 dpi (blue). Figures created in OmicsBox.
3.2 Differentially expressed genes in sorghum in response to infection
An analysis of differentially expressed genes in inoculated samples compared to mock-inoculated controls across all genotypes at 1, 3, and 5 dpi revealed that at 1 dpi a total of 93 genes were up-regulated and 102 were down-regulated, and that 162 of the total differentially expressed genes (DEGs) were unique to 1 dpi (Figure 3A). KEGG pathway enrichment of the up-regulated genes at 1 dpi revealed pathways enriched for sphingolipid metabolism, carotenoid biosynthesis, and SNARE interactions in vesicle transport (Figure 4A). At 3 dpi there were 343 up-regulated and 216 down-regulated genes, and 488 of the total DEGs were uniquely expressed at 3 dpi (Figure 3A). Enriched biological pathways represented by up-regulated genes at 3 dpi included flavonoid biosynthesis, specifically the biosynthesis of flavones and flavonols (Figure 4B). At 5 dpi a total of 139 genes were up-regulated, 59 were down-regulated, and 137 genes were uniquely expressed at 5 dpi (Figure 3A). KEGG pathway enrichment of the up-regulated DEGs at 5 dpi identified genes involved in biotin metabolism and the biosynthesis of flavonoids (specifically flavones and flavonols), stilbenoids, diarylheptanoids and various other secondary metabolites (Figure 4C).
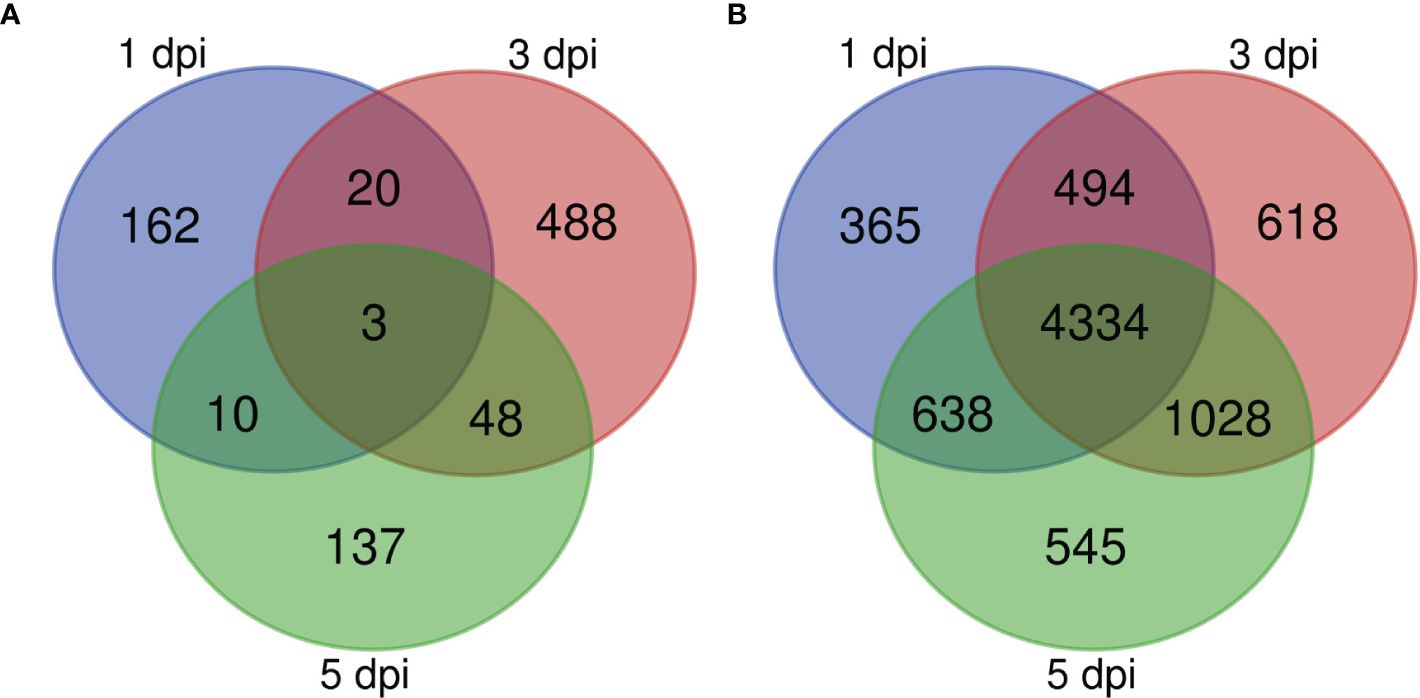
Figure 3 Venn diagram showing the numbers of DEGs identified in (A) sorghum and (B) C. sublineola transcriptomes at 1, 3, and 5 dpi. Figure made with the tool available at: https://bioinformatics.psb.ugent.be/webtools/Venn/.
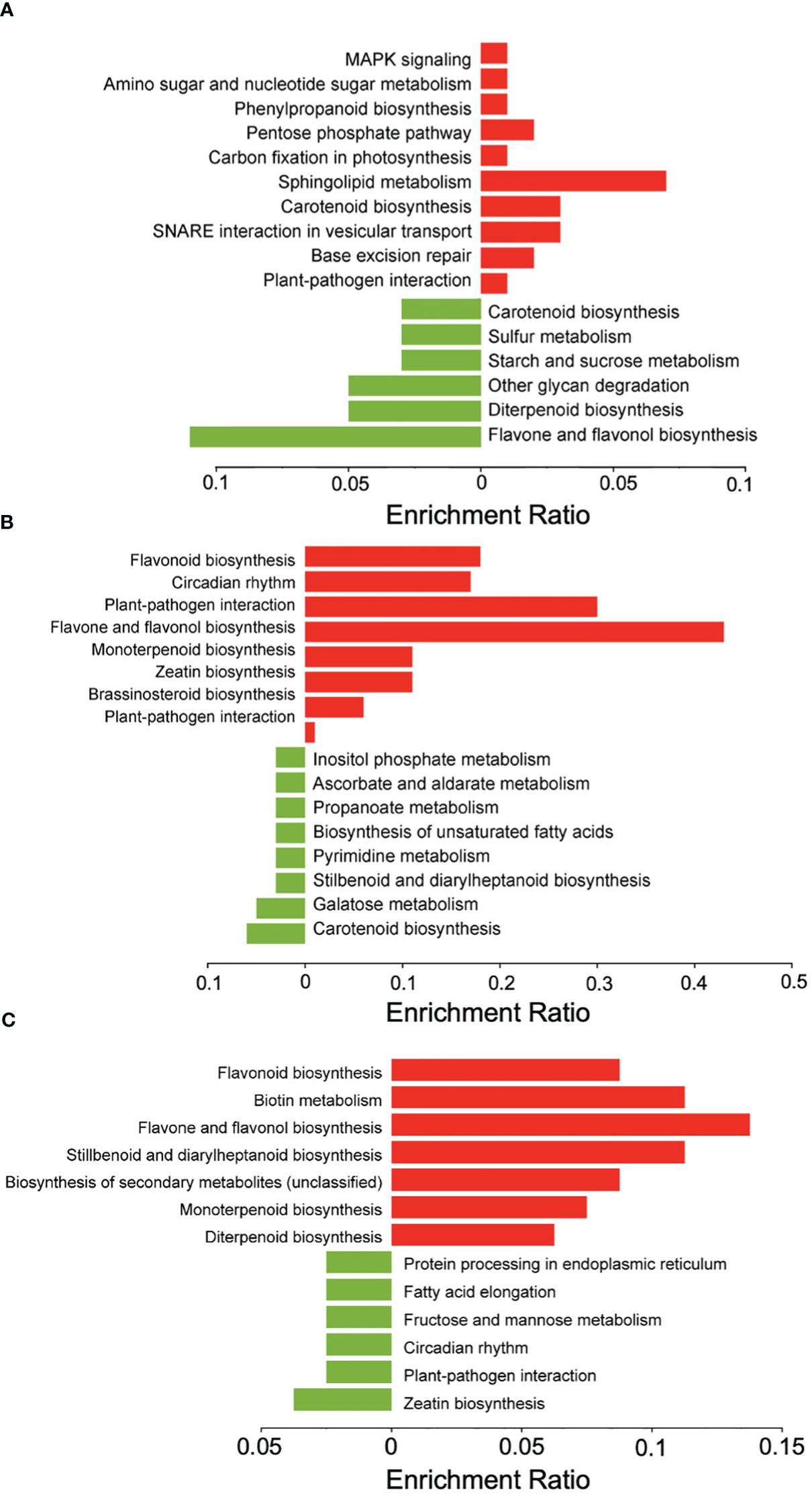
Figure 4 KEGG pathway enrichment analysis of DEGs in sorghum. Down-regulated genes (green) and upregulated genes (red) at (A) 1 dpi, (B) 3 dpi, and (C) 5 dpi in inoculated versus mock-inoculated samples. Figure created with SRPlot (Tang et al., 2023).
3.3 Differentially expressed C. sublineola genes during infection
The comparison between in planta (inoculated) and in vitro (control) C. sublineola samples revealed that the vast majority of DEGs were expressed at lower levels in planta: 7,469 genes versus only 459 genes with higher expression during infection. It is likely that the disproportionally large differences in DEGs are due to the substantial difference between the number of C. sublineola reads in vitro versus in planta samples. Gene Ontology (GO) annotation indicated that most DEGs had molecular functions related to hydrolase, transferase activity, oxidoreductase, protein catalytic and transporter activity, as well as DNA binding (Figure 5). Biological processes represented by these DEGs were transmembrane transport, regulation of transcription, and carbohydrate, lipid, and amino acid metabolism (Figure 5). These results indicated a lack of nutrient availability in planta caused the low expression of genes involved in energy-related metabolism, nutrient acquisition, and growth in an attempt to conserve resources and adapt to the host environment.
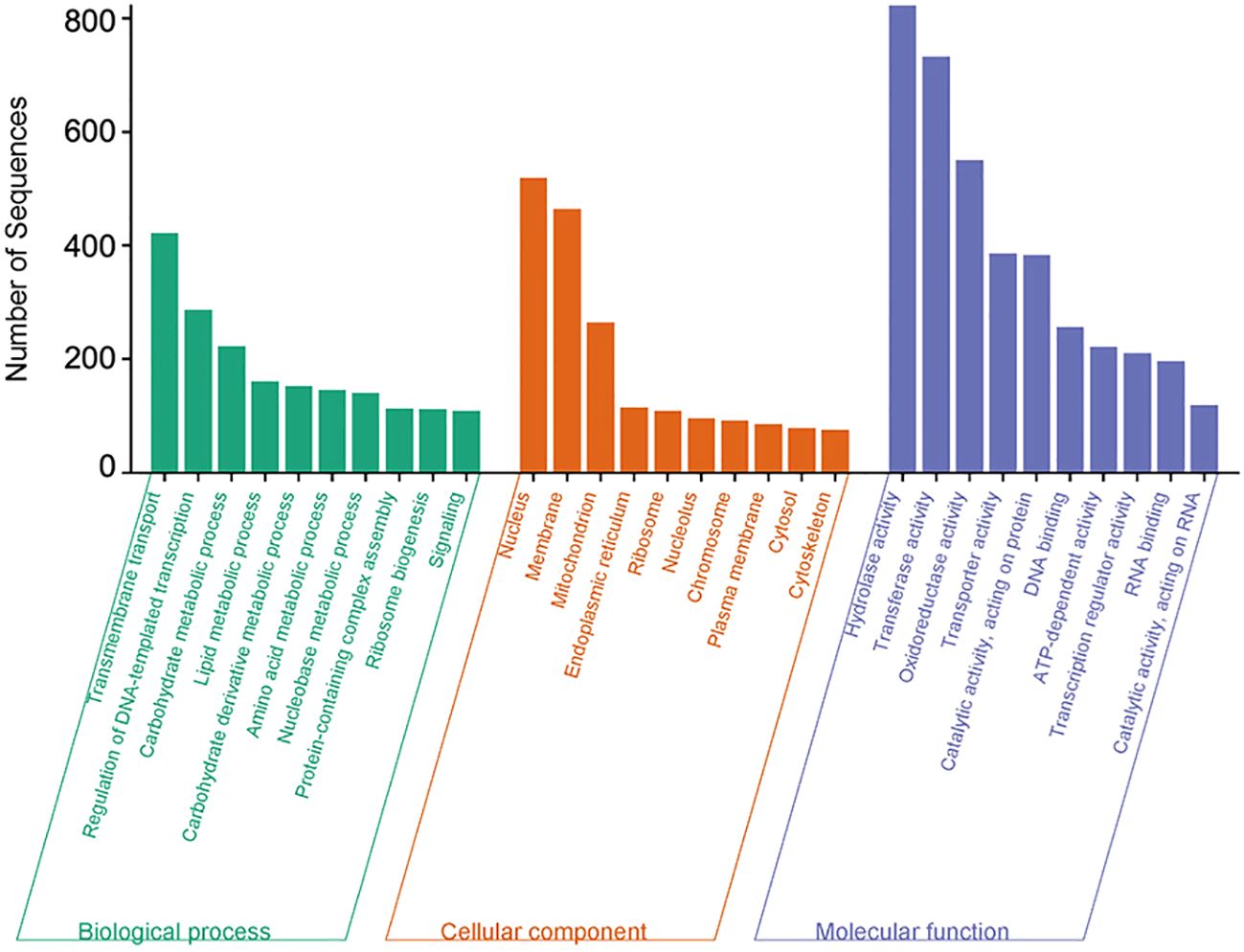
Figure 5 Overrepresented Gene Ontology (GO) terms of C. sublineola DEGs in inoculated versus control samples. Figure created with SRPlot (Tang et al., 2023).
Expressed genes in C. sublineola were compared between time points (1, 3, 5 dpi) to identify genes important for infection during the transition from the biotrophic phase (1 dpi) to the necrotrophic phase (5 dpi). At 1 dpi 238 genes were up-regulated, and 5,593 genes were down-regulated. Among the collective set of fungal DEGs, 365 were unique to 1 dpi (Figure 3B). At 3 dpi a total of 411 up-regulated genes and 6,063 down-regulated genes were identified and 618 of the total DEGs were unique to 3 dpi (Figure 3B). At 5 dpi 158 up-regulated genes and 6,387 down-regulated genes were identified and 545 genes were expressed uniquely at this timepoint (Figure 3B).
GO annotation for up-regulated genes at different time points during infection revealed an overrepresentation of biological processes related to transmembrane transport, carbohydrate metabolism, and lipid metabolism (Figure 6). Subsequently, the proteins encoded by the up-regulated genes were predicted to be primarily located in the membrane (Figure 6). Molecular processes of up-regulated genes were associated with oxidoreductase, hydrolase, transferase, and transport activities (Figure 6). Thus, among the enzymes encoded by up-regulated genes, oxidoreductases and hydrolases were the most abundant classes (Figure 7). Analysis of the up-regulated genes at 3 dpi implicated that distinct processes were occurring at this time point compared to the other two time points (1 and 5 dpi), including cell wall biogenesis, which includes cell wall organization and secretion of proteins into the extracellular space (Figure 6).
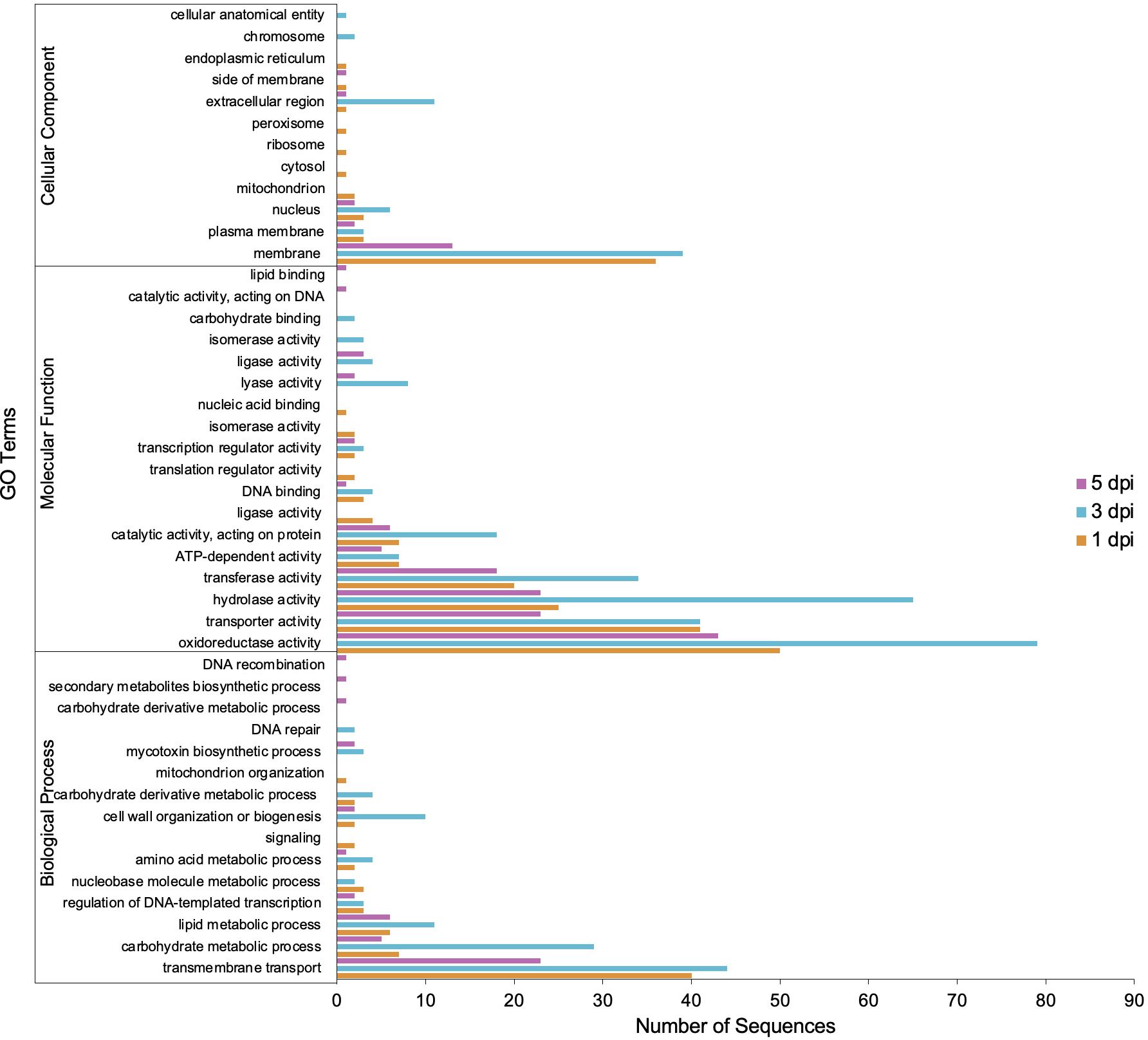
Figure 6 Gene Ontology (GO) terms associated with C. sublineola genes up-regulated in the in planta versus in vitro samples by timepoint.
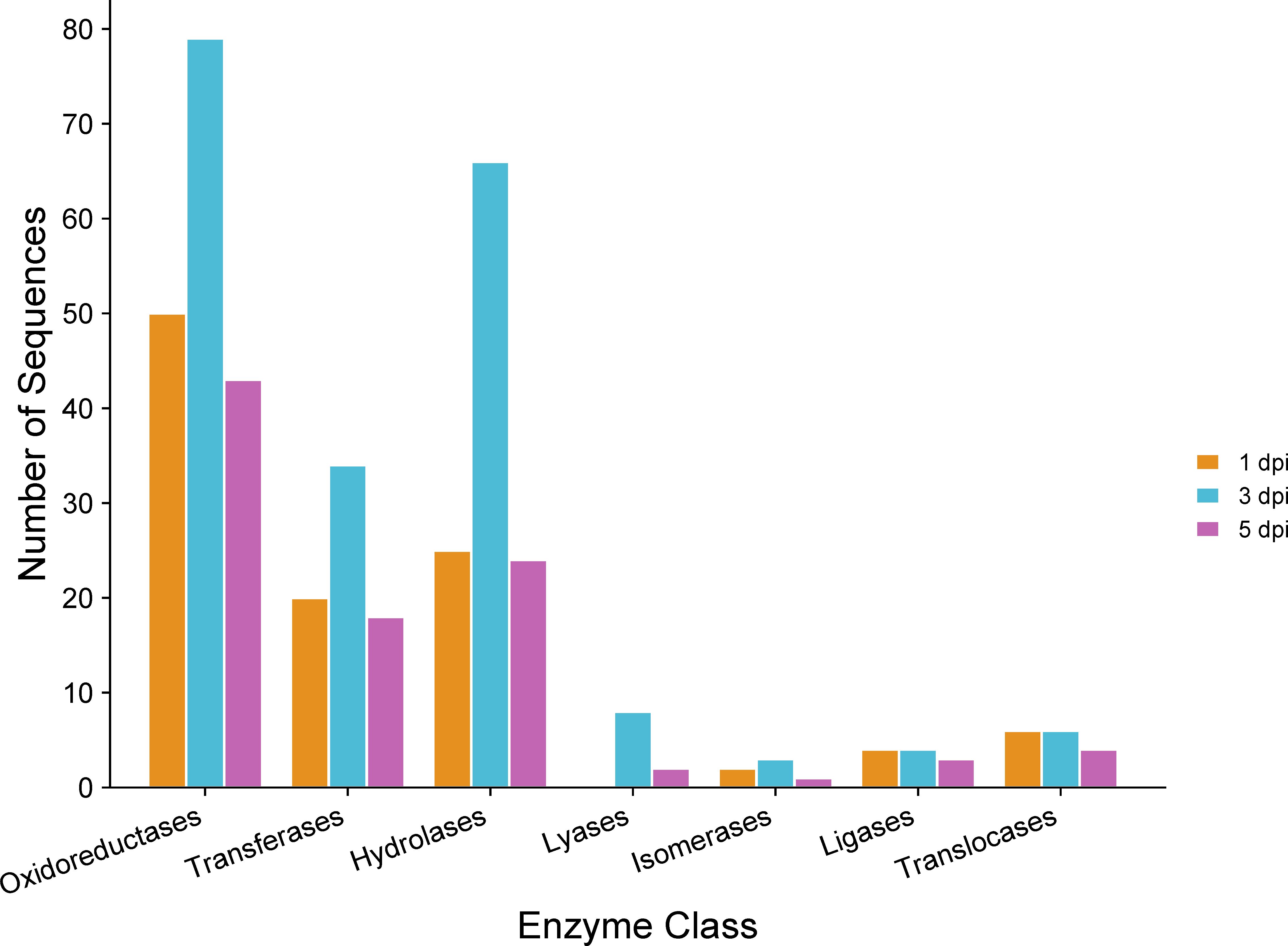
Figure 7 Gene Ontology (GO) of enzymes encoded by C. sublineola genes up-regulated in planta versus in vitro samples at different time points. Figure created with SRPlot (Tang et al., 2023).
In genotype SC17, 90 C. sublineola genes were up-regulated and 5,121 were down-regulated relative to C. sublineola cultured in vitro. In SC110, 216 genes were up-regulated and 5,772 down-regulated, whereas in SC1330 there were 484 up-regulated genes and 3,995 down-regulated genes. In accession SC1033, which lacked resistance alleles at the three candidate resistance loci on chromosome 5, 99 C. sublineola genes were up-regulated and 6,742 down-regulated.
An analysis of the up-regulated C. sublineola genes shared between sorghum accessions revealed that SC1330 displayed the highest number of unique up-regulated genes (Supplementary Figure 3). The different expression patterns imply that C. sublineola deployed different strategies to infect sorghum genotypes with different resistance mechanisms.
3.4 Carbohydrate-active enzymes deployed by C. sublineola to facilitate infection
Carbohydrate-active enzymes (CAZymes) are involved in various crucial biological processes, including cell wall biogenesis, signaling, and energy production (Park et al., 2010). The CAZymes of classes carbohydrate esterases (CE), glycoside hydrolases (GH), and polysaccharide lyases (PL) are often known as cell wall degrading enzymes (CWDEs) because they play pivotal roles in the decomposition of plant cell walls (PCW) (Ospina-Giraldo et al., 2010). Profiling the expression of genes encoding different classes of CAZymes during infection can reveal strategies used to degrade the host cell wall.
Analysis of proteins encoded by significantly up-regulated genes revealed 17 predicted CAZymes expressed at 1 dpi, 50 at 3 dpi, and 17 at 5 dpi (Supplementary Data 2). It is noteworthy that certain genes were predicted to encode enzymes that represent multiple classes of CAZymes (Supplementary Data 2). A detailed classification of these CAZymes at each time point highlighted the prevalence of auxiliary activities (AA) (Figure 8), referring to proteins defined by their role in assisting other CAZymes in the degradation of the plant cell wall (Levasseur et al., 2013). Specifically, AA enzymes facilitate the breakdown of lignocellulosic biomass by catalyzing redox reactions, often involving the generation of reactive oxygen species (ROS) (Levasseur et al., 2013). This oxidative activity can contribute to the depolymerization of lignin so that the polysaccharides in plant cell walls become better accessible to CAZymes with hydrolytic activity (Levasseur et al., 2013). Indeed, the GHs were prominently represented among the CAZymes. GHs hydrolyze the glycosidic bond between two or more carbohydrates, or between a carbohydrate and a non-carbohydrate moiety, such as a protein or a lipid (Cantarel et al., 2009). GHs are involved in the degradation of cellulose, hemicellulosic polysaccharides, and pectins (Rafiei et al., 2021). The next abundant class of CAZymes encoded by C. sublineola genes up-regulated during infection are carbohydrate-binding modules (CBMs; Cantarel et al., 2009). CBMs enhance the activity of enzymes by targeting and promoting a prolonged interaction with the substrate (reviewed by Sidar et al. (2020)) and are most often linked to catalytic modules of other CAZymes present in the same polypeptide. In contrast, PLs were much less abundant. PLs mainly degrade glycosaminoglycans and pectins (Yip and Withers, 2006; Cantarel et al., 2009), which are not major components of the sorghum cell wall.
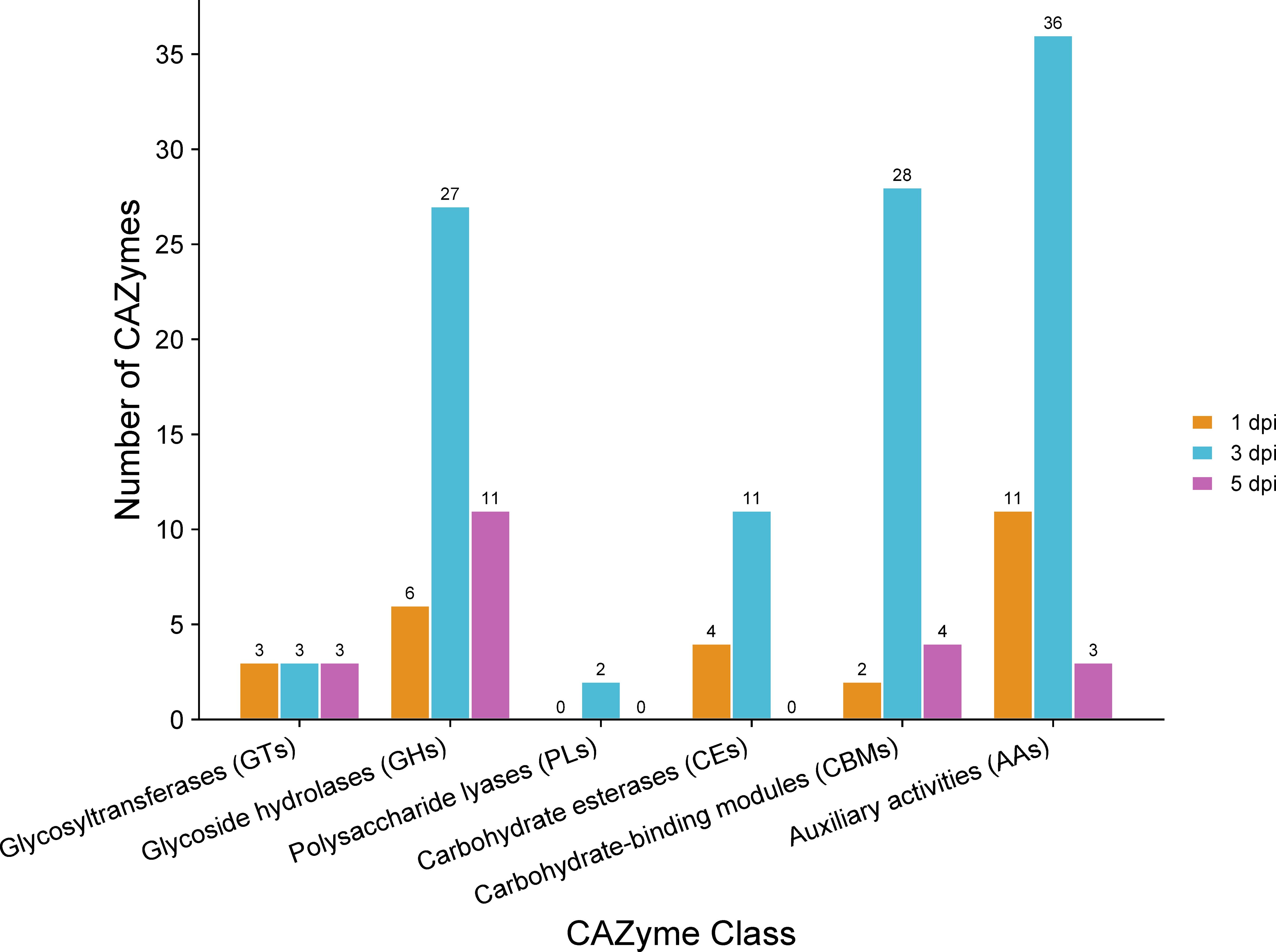
Figure 8 Classes of CAZymes encoded by C. sublineola genes up-regulated in the in planta versus in vitro samples at different time points. Figure created with SRPlot (Tang et al., 2023).
3.5 Predicted fungal effectors secreted by C. sublineola
Fungal effectors are characterized as small secreted proteins (SSPs) that primarily act to modulate host physiology, often by suppressing host defenses or shielding the pathogen from the host’s defense responses intended to impede pathogen growth (de Jonge et al., 2011). Some effectors are translocated and function within the host cell’s cytoplasm, while others operate in the extracellular space outside the plant cell (apoplast) (de Jonge et al., 2011). Significantly up-regulated C. sublineola genes in the in planta versus in vitro samples by time point post-inoculation and by accession were analyzed to determine if they encode putative fungal effectors (Supplementary Data 3). All predicted effectors expressed by time point are summarized in Supplementary Data 3. At 1 dpi, a total of 16 genes encoding predicted effectors were up-regulated. By 3 dpi, this count increased to 62, and at 5 dpi decreased to 15. Several of these genes were expressed at multiple time points (Supplementary Data 3). The increase in DEGs encoding predicted effectors at 3 dpi indicated the deployment of effectors facilitating the pathogen’s transition from the biotrophic to the necrotrophic phase. According to the effector localization predictions, most of these candidate effectors are localized in the plant apoplastic space (Figure 9; Supplementary Table 2). Predicted effectors were compared to proteins in the Pathogen-Host Interaction (PHI) database to infer their roles during different stages of the infection process. A total of 19 predicted C. sublineola effectors were highly similar (threshold e-value of 1 × 10−5) to known pathogenicity and virulence factors of other fungal pathogens (Supplementary Data 3). A number of these were of particular interest because they provide insights into the way C. sublineola interacts with its host, as discussed below.
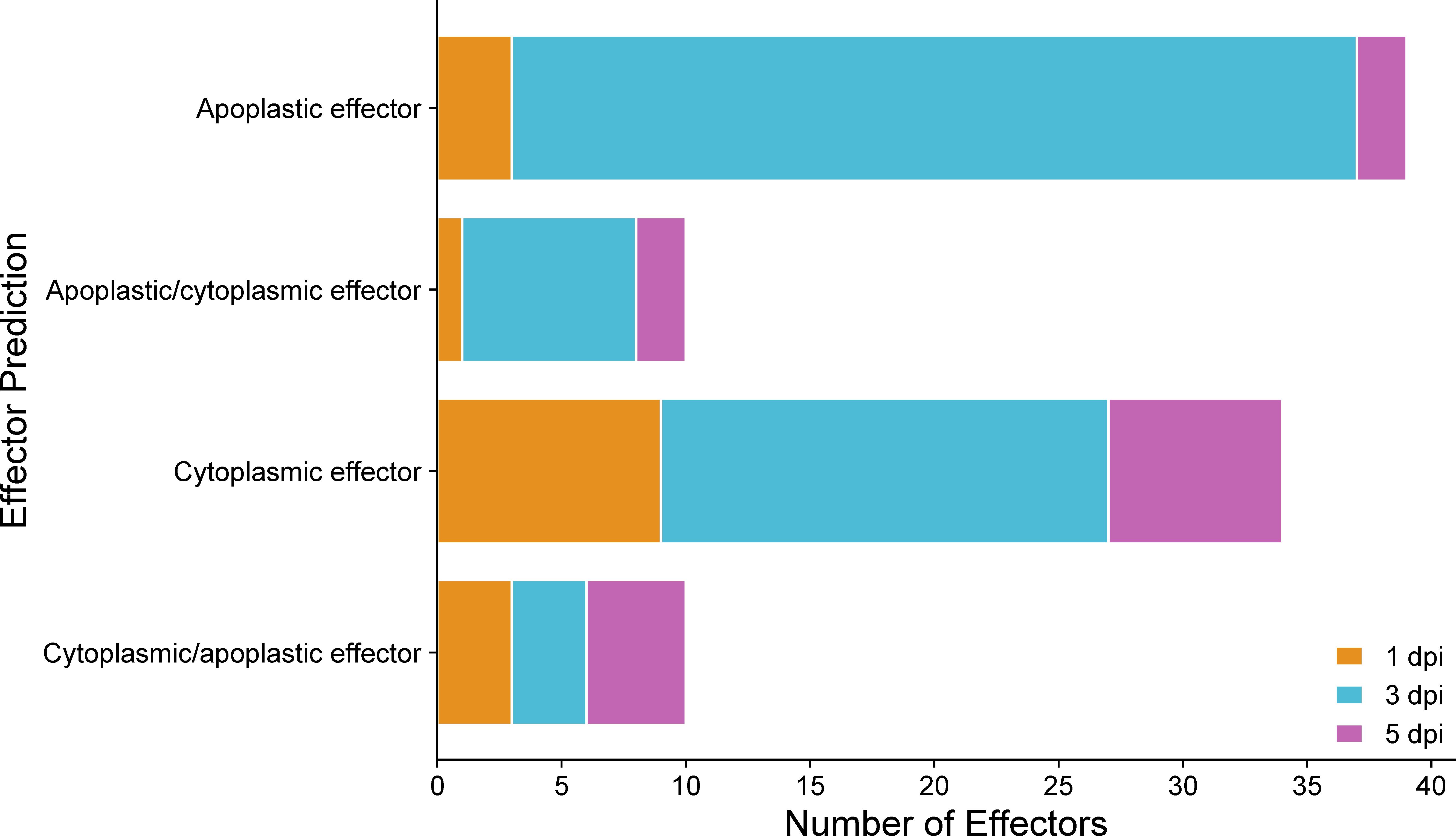
Figure 9 Predicted localization of effectors encoded by C. sublineola genes up-regulated in the in planta versus in vitro samples at different time points. Figure created with SRPlot (Tang et al., 2023). When both the apoplast and cytoplasm are listed as predicted locations, the location listed first has the higher prediction score.
At 1 dpi the C. sublineola gene CSUB01_08309 was up-regulated in the in planta versus in vitro samples. This gene encodes a predicted effector (A0A066XPB8_COLSU) with a sequence similar to Sclerotinia sclerotiorum cutinase 1 (SsCut1; e-value = 8 × 10−91) (Supplementary Data 3). S. sclerotiorum secretes SsCut1 to disrupt the cuticle layer of Arabidopsis thaliana and increased expression of SsCut1 promotes the virulence of S. sclerotiorum (Gong et al., 2022). Additionally, at 1 dpi C. sublineola gene CSUB01_05831 is up-regulated. It is predicted to encode a deuterolysin (metalloprotease) protein (A0A066XJ51_COLSU) that has significant similarity (e-value = 1 × 10−11) to avirulence (Avr) effector Avr-Pita encoded by Magnaporthe oryzae during the infection of rice, causing rice blast disease (Supplementary Data 3). Avr-Pita accumulates in the biotrophic interfacial complex (BIC) and is delivered into host cells by invasive hyphae (Khang et al., 2010). Avr-Pita targets the host mitochondria and interacts with the cytochrome c oxidase (COX) assembly protein OsCOX11, a key regulator of mitochondrial ROS metabolism in rice (Han et al., 2021). Avr-Pita enhances COX activity and decreases ROS accumulation, and therefore suppresses host innate immunity by disrupting ROS metabolism in the mitochondria (Han et al., 2021).
Four C. sublineola genes expressed at 3 dpi (CSUB01_08906, CSUB01_02278, CSUB01_09878, and CSUB01_09688) are predicted to encode effectors (A0A066XNR3_COLSU, A0A066X9Z0_COLSU, A0A066XMA2_COLSU, and A0A066XNJ6_COLSU) that are similar to M. oryzae cell death-inducing protein 4 (MoCDIP4) (Supplementary Data 3). The effector MoCDIP4 is classified as a member of the glycosyl hydrolase family 61 and targets a heat shock-dynamin protein (HSP40-DRP) complex. The targeting leads to the perturbation of mitochondrial dynamics, thereby inhibiting mitochondria-mediated plant immunity such as ROS production and defense-related gene expression (Xu et al., 2020).
The three C. sublineola genes CSUB01_11922, CSUB01_04305, and CSUB01_10464 expressed at 3 dpi and 5 dpi encode proteins A0A066XAG3_COLSU, A0A066X6X5_COLSU, and A0A066XAZ6_COLSU, respectively, that share significant similarity to a Cladosporium fulvum lysin motif effector Ecp6 (Supplementary Data 3). Ecp6 is a chitin‐binding effector and has three lysin motif (LysM) domains that sequester chito‐oligosaccharides released from the cell walls of invading hyphae to prevent their recognition by extracellular chitin‐binding tomato immune receptors (Mesarich et al., 2023). This, in turn, prevents the activation of chitin‐triggered immune responses in tomato (Bolton et al., 2008; de Jonge et al., 2010). These three C. sublineola genes encode proteins that also share significant sequence similarity to the Secreted LysM Protein 1 (Slp1) expressed by M. oryzae in the infection of rice (Mentlak et al., 2012) (Supplementary Data 3). Slp1 accumulates at the interface between the fungal cell wall and the rice plasma membrane and sequesters chitin oligosaccharides to prevent PAMP-triggered immunity, including the production of ROS and plant defense gene expression (Mentlak et al., 2012).
Four genes (CSUB01_02278, CSUB01_10115, CSUB01_03746, and CSUB01_06499) predicted to encode endo-1,4-β-xylanases (A0A066X9Z0_COLSU, A0A066XFB3_COLSU, A0A066XQM7_COLSU, and A0A066XGR6_COLSU) are expressed at 3 and 5 dpi and have high similarity to M. oryzae endo-β-1,4-xylanases MoXYL1A and MoXYL1B (Supplementary Data 3). Endo-β-1,4-xylanases are extracellular enzymes responsible for catalyzing the hydrolysis of xylans (Collins et al., 2005), which include glucuronoarabinoxylans, the predominant hemicellulosic polysaccharide in monocot cell walls (Carpita and Gibeaut, 1993). Thus, endo-1,4-β-xylanases play a significant role in fungal penetration and colonization (Beliën et al., 2006; Dornez et al., 2010) and induce necrosis in host tissues (Walton, 1994). MoXYL1A is proposed to enhance the virulence of M. oryzae by disrupting the function of the host chloroplast (Shabbir et al., 2022). On the other hand, MoXYL1B does not play a significant role in virulence. It is, however, essential for the proper asexual reproduction of the fungus (Shabbir et al., 2022).
3.6 Structural similarity of C. sublineola candidate effectors
Given the subcellular localization predictions by WoLF-PSORT, predicted C. sublineola effector protein sequences were evaluated for the presence of known nuclear localization sequences (NLS). Five predicted C. sublineola effectors with NLSs were identified, suggesting potential nuclear translocation upon secretion into sorghum (Supplementary Data 4). To characterize the ‘effector-ome’ of C. sublineola and to gain insights into additional candidate effectors encoded by unannotated genes, protein structural clustering was performed on protein structures generated in AlphaFold from coding sequences. FoldSeek analyses revealed structural similarities between C. sublineola candidate effectors and those of other Colletotrichum species, as well as significant similarities with effectors of other hemibiothrophic fungi (Supplementary Data 4), including Zymoseptoria tritici and M. oryzae (Pyricularia oryzae) (Sánchez-Vallet et al., 2015; Fernandez and Orth, 2018). These results suggest potential conserved infection strategies across hosts, which may be due to their hemibiotrophic lifestyle on monocot hosts.
Additionally, protein structural similarity clustering employing the Louvain community detection method identified eight protein clusters, suggesting distinct functional groups among candidate effectors (Figure 10). The majority of the C. sublineola candidate effectors did not cluster with other effectors, indicating unique structural features possibly related to specific functions during infection. Notably, three clusters corresponded to cell wall proteins, endo-1,4-β-xylanases, and carboxylic ester hydrolases. These classes of effectors are crucial for the successful infection of M. oryzae in rice (Liang et al., 2022; Liu et al., 2022; Lee et al., 2024). The putative effectors A0A066XNJ6_COLSU, A0A066XNR6_COLSU, and A0A066XMA2_COLSU clustered together. Based on PHIB-BLAST analyses, A0A066XNJ6_COLSU and A0A066XMA2_COLSU were predicted to contain a putative fungal cellulose binding domain (also known as carbohydrate binding module), while A0A066XNR6_COLSU may be a member of glycosyl hydrolase family 61. Despite varied annotations, FoldSeek analyses indicated a structural similarity to known endo-β-1,4-glucanase D proteins, implying potentially shared functionalities (Supplementary Data 4). Furthermore, the analysis revealed five clusters with at least one uncharacterized protein, that could be indicative of functional similarities based on shared structural features with other members of the cluster. For instance, uncharacterized protein A0A066X3X1_COLSU displayed structural similarity to A0A066X8J3_COLSU, a predicted hydrophobin, which is a member of a class of proteins involved in conidial germination and appressorium development in M. oryzae (Kim et al., 2005).
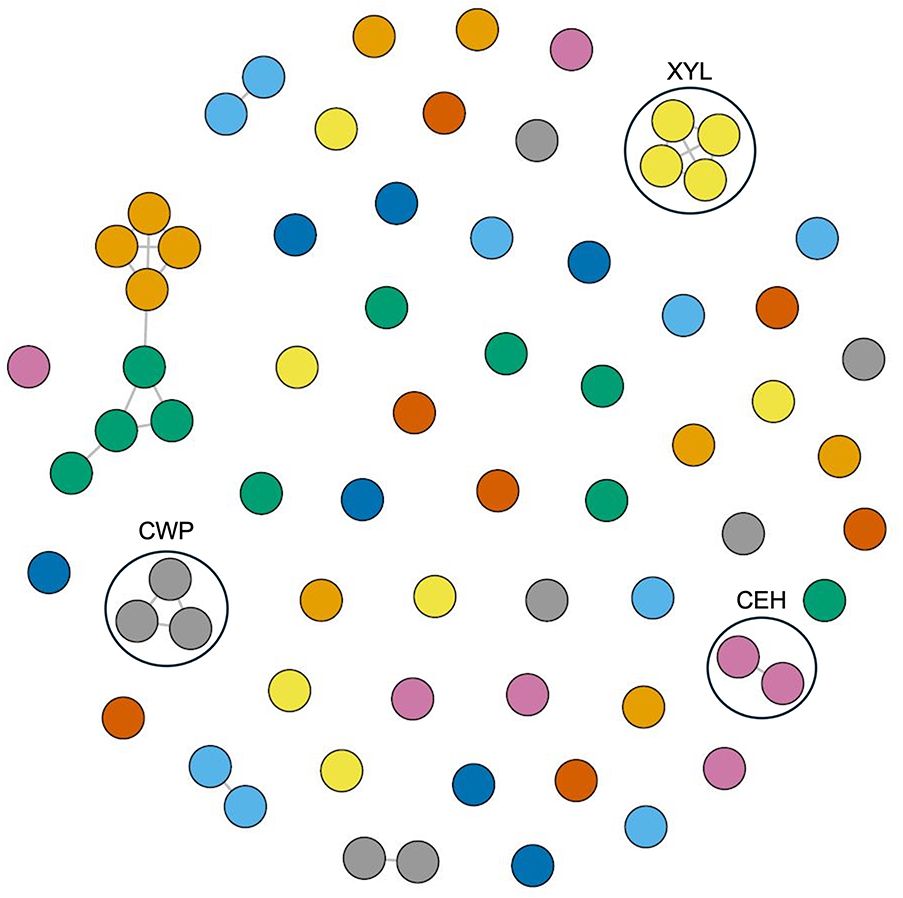
Figure 10 Protein structural network and community analysis of C. sublineola effectors. Effectors with a TM-score of 0.6 are considered structurally similar and are connected by edges (lines). Effectors (circles) lacking edges are considered to have no structural similarity to other effectors. Effectors displayed in the same color with edge connections indicate structural similarity. Black circles indicate clusters of effectors with known functions. Abbreviations: Cell wall proteins (CWP), endo-1,4-β-xylanases (XYL), and carboxylic ester hydrolases (CEH).
3.7 Utilization of membrane transporters by C. sublineola to acquire nutrients and mitigate host defense responses
The prolific expression of genes associated with transmembrane transport (Figure 6) potentially reflects the different strategies to evade host defenses, deliver effectors, and acquire nutrients from the host. Identified membrane transporters encoded by up-regulated genes were compared to the Pathogen-Host Interaction (PHI) database. Several C. sublineola genes expressed at all time points encoded proteins significantly similar to hexose transporters in Colletotrichum higginsianum (ChHxt) (Supplementary Data 5). A total of six ChHxt genes, ChHxt1 to ChHxt6, exhibit specific expression patterns in different infection phases of C. higginsianum in Arabidopsis (Yuan et al., 2021). ChHxt4 is required for fungal infection in both biotrophic and necrotrophic stages, while ChHxt6 is important for the formation of necrotrophic hyphae during infection (Yuan et al., 2021). Thus, the expression of ChHxt genes regulates fungal virulence by modulating the utilization of hexoses.
Additionally, several C. sublineola genes expressed at all time points encoded proteins significantly similar to ATP-binding cassette (ABC) transporters in various fungal pathogens, most notably the hemibiotroph M. oryzae (Supplementary Data 5). ABC transporters play pivotal roles in the tolerance and resistance against toxic substances, either by sequestering hydrophobic compounds into specialized organelles or directing them for secretion (Rees et al., 2009; Kim et al., 2013). Investigations into transporter-encoding genes ABC1 to ABC4 in M. oryzae have revealed their regulatory roles in cytotoxicity, mediation of tolerance against antifungal agents, and resistance to oxidative stress, facilitating the pathogen’s ability for successful infections (Urban, 1999; Lee et al., 2005; Sun et al., 2007; Gupta and Chattoo, 2008). Specifically, M. oryzae ABC1 (Urban, 1999) and ABC4 (Gupta and Chattoo, 2008) are crucial for pathogenicity, aiding the fungus in navigating the cytotoxic environment during infection, whereas ABC2 (Lee et al., 2005) and ABC3 contribute to multidrug resistance, with ABC3 playing a specific role in overcoming cytotoxicity and oxidative stress within appressoria during early infection-related morphogenesis (Sun et al., 2007).
3.8 Comparison of C. sublineola gene expression in host genotypes SC110 and SC1033
We further analyzed genes differentially expressed in the pathogen in sorghum accessions SC110 versus SC1033. The host resistance response in SC110 has been elucidated and involves an increase in the production of reactive oxygen species (Wolf et al., 2024). SC1033 lacks resistance alleles at the three anthracnose resistance loci identified on chromosome 5 (Cuevas et al., 2018). A total of 99 genes were up-regulated and four were down-regulated in C. sublineola during infection of SC110 compared to SC1033. Up-regulated genes were compared to the PHI database to determine the known virulence factors implemented by C. sublineola to combat the defense response in SC110. A total of 33 up-regulated genes had significant hits (threshold e-value of 1 × 10−5) to the PHI database (Supplementary Data 6). Up-regulated pathogen genes in SC110 with a substantial number of hits to the PHI database (>50) included the following:
CSUB01_04556 encodes a calcium-transporting ATPase (A0A066X398_COLSU) with similarity to six calcium pumps (Eca1, Spf1, PmcA/B/C, and Pmcr1) in Beauveria bassiana, a fungal pathogen of insects (Supplementary Data 6). All six calcium pumps play vital roles in sustaining antioxidant activity and cell wall integrity of B. bassiana. Deletion of the genes encoding the B. bassiana calcium pumps resulted in increased sensitivities to the oxidants menadione and H2O2 and cell-wall-perturbing agents, reduced activities of intracellular superoxide dismutases (SODs) and catalases, and altered cell wall components (Wang et al., 2013, 2017).
CSUB01_10385 encodes a putative ketoreductase (KR) domain-containing protein (A0A066XUL6_COLSU) that displays similarity to several polyketide synthases (PKSs) in a variety of different fungal pathogens (Supplementary Data 6). PKSs are enzymes involved in the biosynthesis of polyketides, a diverse class of secondary metabolites contributing to pathogen virulence, host interaction, and environmental adaptation (Fujii, 2010). A0A066XUL6_COLSU is most similar (e-value = 0) to a PKS encoded by fumonisin biosynthetic gene 1 (FUM1) in Gibberella moniliformis (Supplementary Data 6). FUM1 encodes an enzyme that catalyzes the synthesis of a polyketide that forms a key constituent of fumonisin (Procter et al., 2003). Fumonisin is a mycotoxin that is known to be a strong inducer of programmed cell death (PCD) in plants (Lanubile et al., 2022). Since PKS-related genes tend to be organized in biosynthetic gene clusters (BGCs), the genomic region near CSUB01_10385 was analyzed using antiSMASH (Blin et al., 2023) to gain insights into its role during infection. The 20-kb genomic region near CSUB01_10385 has similarities to several BGCs responsible for the production of toxins in different fungi (Supplementary Figure 4). Similarity of BGCs is defined by sequence similarity, gene order/organization, gene composition, regulatory elements, and biological activity. This genomic region in C. sublineola shared the most similarity (21%) to a BGC in Cryphonectria parasitica, which causes chestnut blight disease, and 9% similarity to a BGC in Fusarium fujikuroi. Thus, the BGC identified in C. sublineola could function in the production of a pathogen-specific mycotoxin.
CSUB01_02347 encodes a mitogen-activated protein kinase kinase kinase (MAPKKK) (A0A066X7D3_COLSU) that is most similar (e-value = 0) to MAPKKK Ssk2/Ssk22 in Fusarium graminearum (Supplementary Data 6). Ssk2/Ssk22 is a component of the high osmolarity glycerol (HOG) mitogen-activated protein kinase (MAPK) signaling pathway, a key element that controls adaptation to environmental stress. The HOG pathway is required for fungal growth under hyperosmotic conditions (Ma and Li, 2013). However, since the MAPK pathway is known to be involved in response to oxidative stress in several fungi (Furukawa et al., 2005; Segmüller et al., 2007), Fgssk2 mutants are not only hypersensitive to osmotic stress but also have increased sensitivity to oxidative stresses and to chemicals that mimic cytoplasmic membrane and cell wall stresses (Zheng et al., 2012).
3.9 Assessing pathogenicity of C. sublineola using aniline blue staining and fluorescence microscopy
The infection processes of C. sublineola in sorghum genotype SC1033 were observed with epi-fluorescence and light microscopy. The lunate conidia were not visible with aniline blue staining at any of the selected time points. However, Wharton et al. (2001) reported conidia to produce germ tubes that form globose melanized appressoria. Therefore, conidia are located in close proximity to the appressoria. Appressoria were visible via light microscopy at 3 and 5 dpi (Figure 11A). Once formed, the appressorium punctures the host cell wall visible by a penetration pore in which the infection peg emerges (Figure 12). At 3 dpi, C. sublineola developed bulbous, intracellular, biotrophic hyphae (Figure 12A). After 3 dpi, biotrophic hyphae grew intracellularly, colonizing several host epidermal cells (Figure 12B). The intracellular growth of the biotrophic hyphae was characterized by the constriction of hyphae between cells consistent with observations by Wharton et al. (2001). Eventually, biotrophic hyphae gave rise to thin necrotrophic hyphae (Figure 12B). By 5 dpi, necrotrophic hyphae displayed intracellular, intercellular, and subcuticular growth throughout the mesophyll and vascular tissue (Figure 11B).
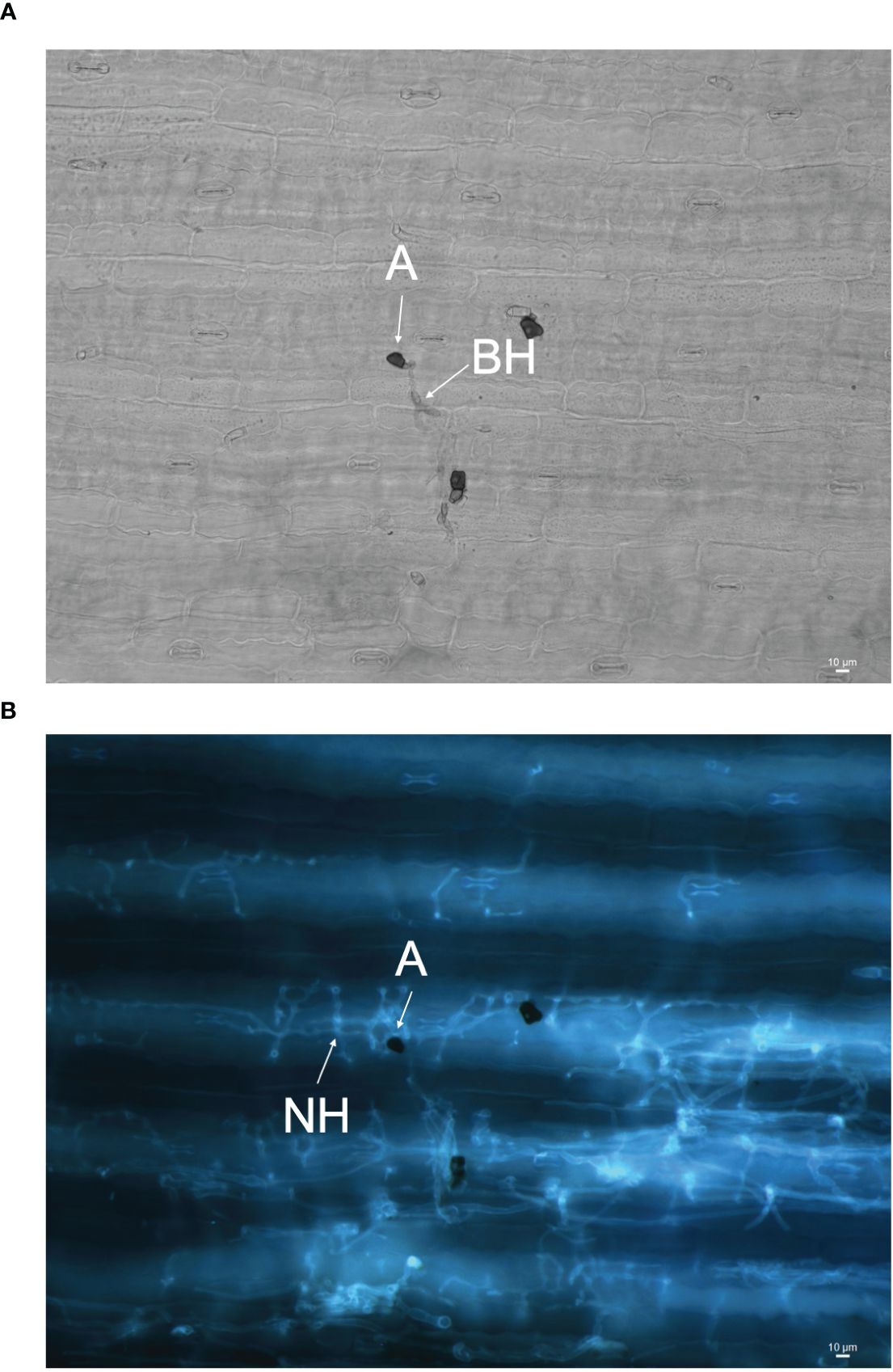
Figure 11 Images of C. sublineola infecting genotype SC1033 at 5 dpi. (A) A melanized appressorium (A) gives rise to biotrophic hyphae (BH) visible with light microscopy in this adaxial view of cleared tissue. (B) Necrotrophic hyphae (NH) associated with the same appressorium are visible using fluorescence microscopy following staining with aniline blue. Images captured at 20× magnification. Scale bars indicate 10 μm.
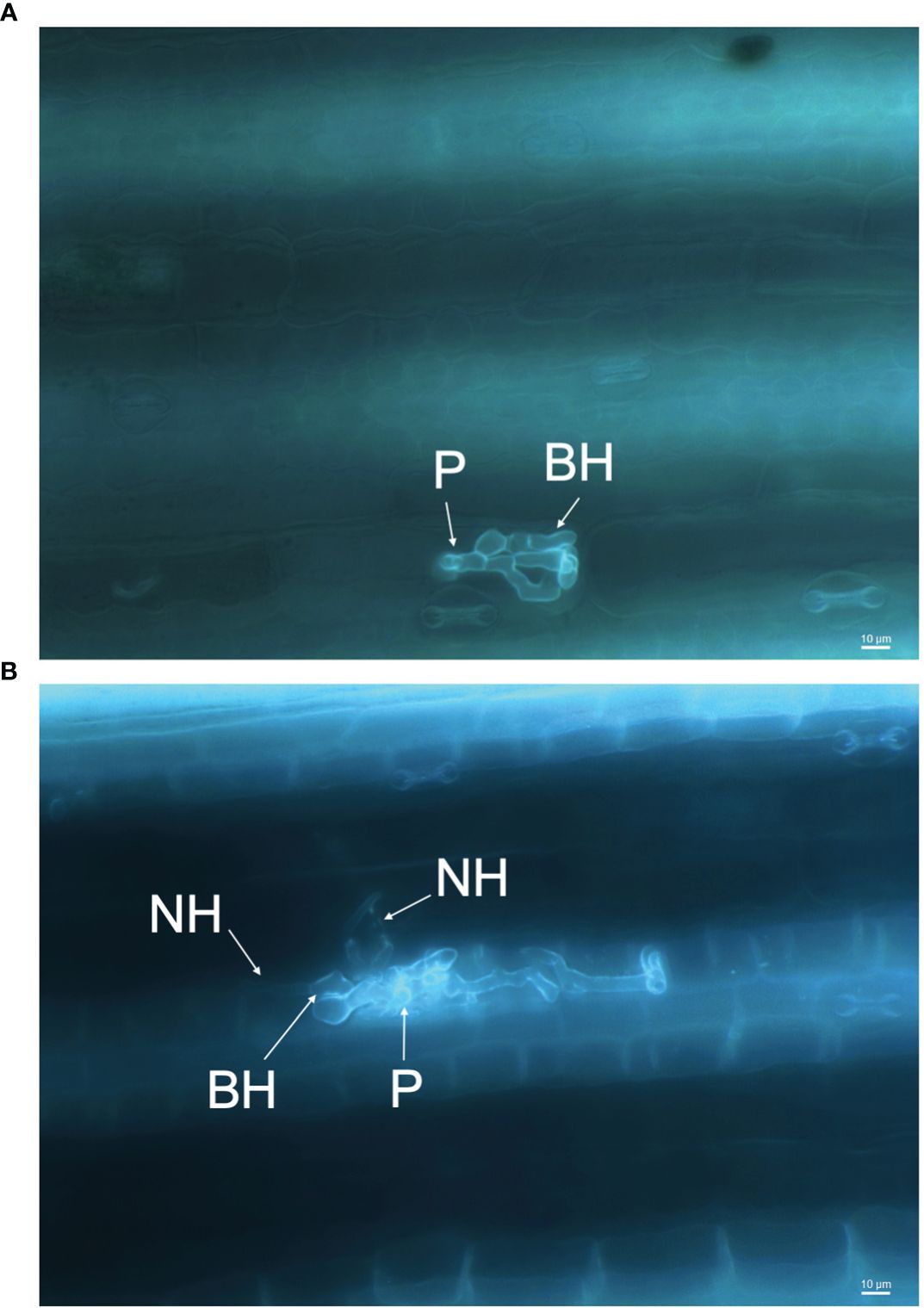
Figure 12 Infection process of C. sublineola on sorghum genotype SC1033. Images captured at 40× magnification of the adaxial side of the leaf following the clearing of pigments and staining with aniline blue. (A) Between 1 and 3 days post-infection (dpi) the appressorium forms an infection peg (P) allowing penetration of the host cuticle and epidermal cell wall. After penetration, an infection vesicle is formed in the host epidermal cell and develops biotrophic hyphae (BH). (B) Between 3 and 5 dpi, BH grow intracellularly, colonizing several host cells, and begin to give rise to distinctly thinner necrotrophic hyphae (NH). Scale bars indicate 10 μm.
4 Discussion
The lifestyle patterns of Colletotrichum species are highly regulated by specific gene families that play pivotal roles in orchestrating the pathogen’s response to host defenses, nutrient acquisition, and environmental cues. For instance, transcriptome analyses of C. higginsianum infecting Arabidopsis thaliana and C. graminicola infecting maize revealed that different categories of genes are transcribed in successive waves that are linked to pathogenic transitions: effectors and secondary metabolism enzymes are induced before penetration and during biotrophy, whereas most hydrolases and transporters are produced later, at the switch to necrotrophy (O’Connell et al., 2012). Conversely, in C. sublineola we observed that all main gene categories increased in expression during the early transition from the biotrophic phase to the necrotrophic phase (3 dpi). At this stage, prominent gene categories relevant to C. sublineola’s pathogenicity were carbohydrate metabolism and transmembrane transport (Figure 6). Therefore, subsequent analysis focused on the identification of virulence factors that could be classified in these categories.
Comparative genome analyses across the genus have revealed that Colletotrichum species exhibit the presence/absence of genes encoding carbohydrate-degrading enzymes tailored to their specific lifestyles and hosts (Gan et al., 2013). C. sublineola deploys a repertoire of CAZymes that reflect the composition of the host cell wall. Sorghum cell walls are comprised mainly of cellulose (45%), hemicellulosic polysaccharides (20–25%), lignin (18–22%), and pectin (3–5%) (Castro et al., 2017). Thus, cellulases, hemicellulases, and enzymes that degrade lignocellulosic structures represented the majority of CWDEs. In contrast, there was a limited upregulation of genes encoding pectinases. This result is consistent with reports in other Colletorichum species. C. higginsianum, which infects Arabidopsis, possesses more than twice the number of pectin-degrading enzymes compared to C. graminicola, a maize pathogen (O’Connell et al., 2012). This difference mirrors their host preferences: the primary cell walls of dicots such as Arabidopsis contain 20–35% pectin versus 5% in the primary cell walls of grasses (Carpita and Gibeaut, 1993; Vogel, 2008; O’Connell et al., 2012).
Apoplastic effectors interfere with PAMP perception mediated by plant membrane-bound pattern recognition receptors (PPR), leading to the inactivation of NADPH oxidases (Jwa and Hwang, 2017). By contrast, cytoplasmic effectors target the MAPK signaling pathway, vesicle trafficking, and metabolic priming, which are essential for apoplastic ROS production (Jwa and Hwang, 2017). The majority of predicted effectors secreted by C. sublineola were localized in the apoplast (Figure 9), indicating that C. sublineola targets the PAMP-immunity pathway likely during the initial colonization of the apoplastic space by necrotrophic hyphae (Figures 12B, 13). During the transition from biotrophy to necrotrophy, C. sublineola expressed chitin‐binding effectors that sequester chito‐oligosaccharides released from the cell walls of invading hyphae to prevent their recognition by extracellular chitin‐binding immune receptors, thus preventing the activation of PAMP-triggered immunity (Figure 13). Previous expression analyses have identified chitinase (PR3) and β-1,3-glucanase to be pathogen-inducible genes in sorghum, indicating that the C. sublineola cell wall serves as a PAMP that is detected by the host receptors (Li et al., 2013; Ahn et al., 2019). Our expression data suggest that C. sublineola secretes specific effectors in the apoplast to prevent recognition via PTI.
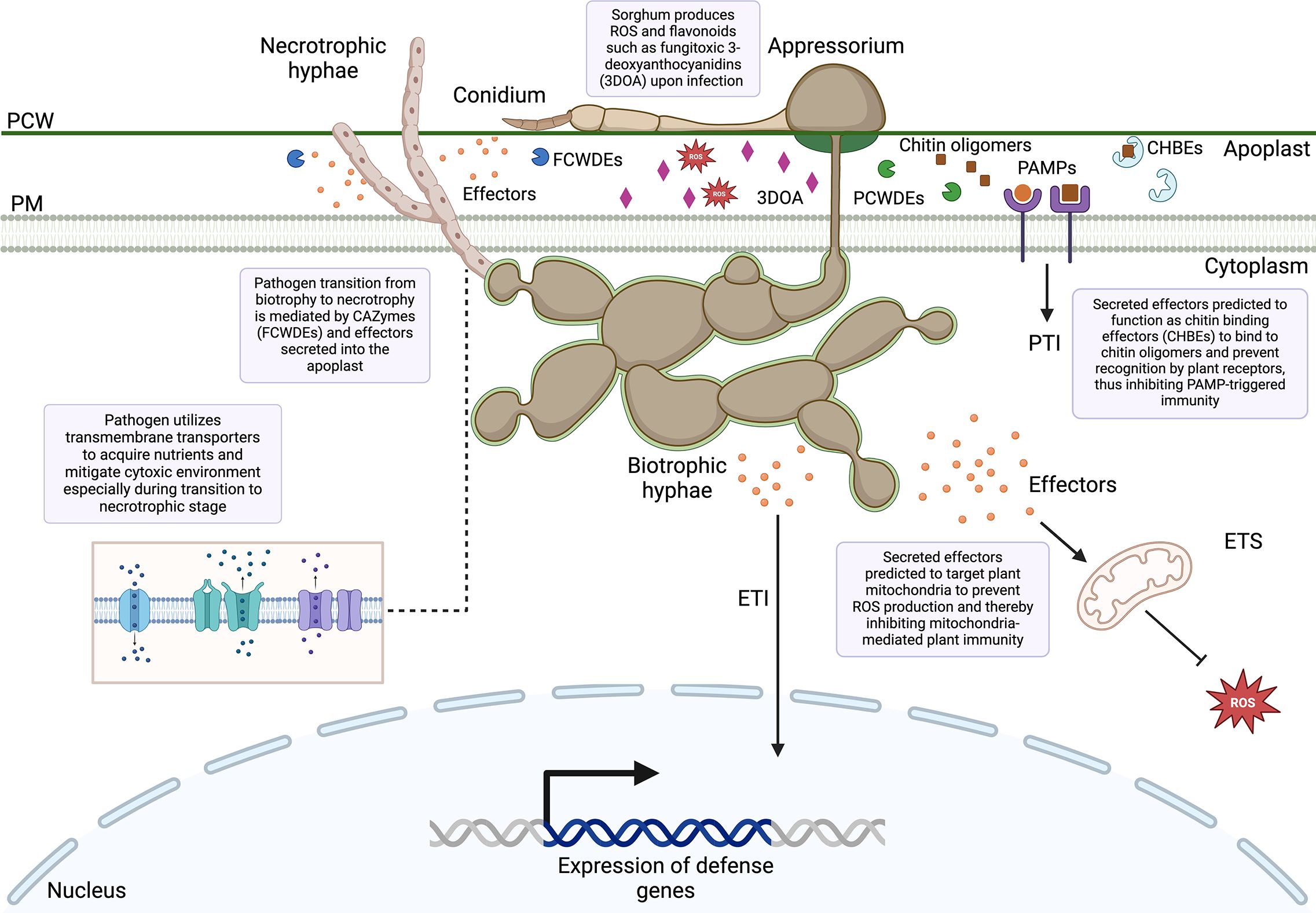
Figure 13 Overview of sorghum–Colletotrichum sublineola interactions uncovered from the dual RNA-seq. PCW, Plant cell wall; PM, plasma membrane; FCWDEs, fungal cell wall degrading enzymes; PCWDEs, plant cell wall degrading enzymes; PAMPs, pathogen-associated molecular patterns; PTI, PAMP-triggered immunity; 3DOA, 3-deoxyanthocyanidins; CHBEs, chitin-binding effectors; ROS, reactive oxygen species; ETI, effector-triggered immunity; ETS, effector-triggered susceptibility.
It has been well documented that inducible defense responses in resistant genotypes of sorghum include early accumulation of ROS, such as hydrogen peroxide (H2O2), and phytoalexins, which decrease the formation of appressoria (Basavaraju et al., 2009). ROS act as cellular signaling molecules to trigger plant immune responses. We uncovered candidate effectors that target multiple steps in the ROS signaling pathway in the host (Figure 13). Several identified candidate effectors are predicted to target mitochondria, where they may alter mitochondrial morphology and metabolism. Mitochondria play an active role in altering intracellular metabolism to defend against pathogen attack, mediating hormone-driven signaling, and facilitating signal transduction. This involvement leads to the generation of ROS and reactive nitrogen species, ultimately triggering programmed cell death (Xu et al., 2020). Moreover, mitochondria function as a signaling platform in mammals, activating downstream immune responses upon the recognition of viral pathogen-associated molecular patterns (PAMPs) (Angajala et al., 2018; Mohanty et al., 2019). Similarly, in plant cells, mitochondria could serve as a signaling organelle, amplifying defense responses through the activation of various signals such as nitric oxide, ROS, or salicylic acid (Colombatti et al., 2014).
The phenylpropanoid and flavonoid pathways are the central hub of the metabolism producing anti-fungal compounds in response to anthracnose infection in sorghum (Li et al., 2013; Tugizimana et al., 2019). Phytoalexins produced uniquely by sorghum include a group of flavonoids known as 3-deoxyanthocyanidins, primarily luteolinidin and apigeninidin (Huang and Backhouse, 2004). Notably, at 1 dpi the host flavonoid biosynthesis-related genes are down-regulated (Figure 4A) while they are highly up-regulated at 3 dpi (Figure 4B) and 5 dpi (Figure 4C). This observation indicates that C. sublineola could evade early (<3 dpi) host defense responses by compromising flavonoid production with the help of secreted effectors. Manipulation of flavonoid metabolism has been reported for the biotrophic fungus Ustilago maydis (now called Mycosarcoma maydis), the causal agent of smut in maize, in which the virulence-promoting secreted effector protein Tin2 is responsible for diverting intermediates used for the defense-related production of lignin by inducing anthocyanin biosynthesis (Tanaka et al., 2014).
Previous studies have demonstrated that early flavonoid phytoalexin accumulation in sorghum is important in preventing the proliferation of C. sublineola fungal hyphae during infection (Wharton et al., 2001; Basavaraju et al., 2009). The vesicles surrounding the infection site accumulate 3-deoxyanthocyanidins, and associate with the plasma membrane. This process results in the host cell collapsing and the release of fungitoxic 3-deoxyanthocyanidins into both the apoplast and the pathogen (Nielsen et al., 2005; Buiate et al., 2017).
Genes encoding transmembrane transporters were expressed across all time points by C. sublineola, similar to what has been observed for M. oryzae, indicating a potential strategy for C. sublineola to overcome sorghum’s early defense responses by mitigating the effects of cytotoxic compounds and oxidative stress (Figure 13) (Urban, 1999; Lee et al., 2005; Sun et al., 2007; Gupta and Chattoo, 2008).
Since many genes similar to ChHxt hexose transporters are expressed throughout infection, it suggests a diversity of transporters is required to allocate nutrients from the changing environment in the host during the switch from the biotrophic phase to the necrotrophic phase. The necrotrophic infection phase occurs when secondary (necrotrophic) hyphae, which are distinctly thinner than primary (biotrophic) hyphae, begin to spread throughout host tissue. Necrotrophy begins at approximately 3 dpi (Figure 12B). Based on our analyses, the transition between biotrophy and necrotrophy is facilitated by CAZymes, transmembrane transporters, and secreted effectors. Notably, we uncovered genes encoding biotrophy-associated secreted protein 3 (BAS3) as a predicted secreted effector at 3 dpi (Supplementary Data 2), which is known to facilitate this transition in M. oryzae, via secretion by invasive hyphae (Wang et al., 2019). During this transition, it is characteristic of Colletotrichum species to produce toxins that kill host tissue, which rapidly becomes necrotic (Münch et al., 2008). Although toxin biosynthetic genes did not represent a main fungal gene category during infection, an up-regulation of genes associated with potential toxin biosynthetic genes (Supplementary Figure 4) is observed at 3 and 5 dpi (Figure 6), further indicating the switch to necrotrophy occurs within this timeframe.
Wolf et al. (2024) proposed that the F-box protein encoded by Sobic.005G172300 targets proteins involved in the biosynthesis of ascorbic acid for polyubiquitination through the SCF E3 ubiquitin ligase, causing their degradation via the proteasome. Hence, the upregulation of Sobic.005G172300 in SC110 results in the transcriptional downregulation of key genes encoding ascorbic acid biosynthetic enzymes. In turn, the decreased concentration of ascorbic acid generates an increase in ROS. Accumulation of ROS by the host damages fungal cells and hinders the pathogen’s ability to establish infection. Here, we uncovered several C. sublineola genes up-regulated during infection of SC110 are associated with mitigating oxidative stress as well as genes necessary for fungal cell wall integrity likely to combat the degradation of the fungal cell wall due to host ROS. Moreover, genes responsible for the biosynthesis of putative toxins were up-regulated. Oxidative stress is often a prerequisite for toxin production (Reverberi et al., 2005, 2010). ROS can stimulate the expression of genes involved in toxin biosynthesis in fungi by acting as signaling molecules. For example, the cereal pathogen F. graminearum produces the mycotoxin deoxynivalenol (DON), which is a virulence factor induced by ROS (Nguyen et al., 2013). Therefore, the accumulation of host ROS in SC110 may trigger the production of toxins by C. sublineola to enhance pathogen defense in response to the oxidative burst.
The dual RNA-sequencing approach presented here improves our current knowledge of the molecular mechanisms between the sorghum and C. sublineola interaction (Figure 13). Notably, the identification of secreted predicted effectors provides valuable insights into the functional diversity and potential roles of these proteins during infection. These data provide a foundation for subsequent experimental validation, such as the transient expression of fluorescent protein-tagged effectors in planta that could confirm their contributions to pathogen virulence. Given the genetic diversity of C. sublineola, experiments conducted with isolates from diverse geographic locations in which the -omics approaches used in this study are combined with high-throughput genomics tools (e.g., genotyping by sequencing) will form the basis for the effective protection of sorghum against this aggressive fungal pathogen.
Data availability statement
The original contributions presented in the study are publicly available. The RNA-sequencing data are deposited at the National Center for Biotechnology Information (NCBI) Sequence Read Archive (SRA). The BioProject ID for the data representing the sorghum samples is PRJNA961726, and for the data from the in vitro cultured C. sublineola is PRJNA1114779.
Author contributions
SV: Conceptualization, Data curation, Formal analysis, Investigation, Methodology, Visualization, Writing – original draft, Writing – review & editing. EW: Investigation, Methodology, Writing – review & editing, Writing – original draft. JR: Conceptualization, Investigation, Methodology, Resources, Supervision, Writing – review & editing. HC: Conceptualization, Funding acquisition, Methodology, Writing – review & editing. WV: Conceptualization, Funding acquisition, Investigation, Project administration, Resources, Supervision, Writing – review & editing.
Funding
The author(s) declare financial support was received for the research, authorship, and/or publication of this article. Funding from the U.S. Department of Energy Office of Science, Biological and Environmental Research (BER) Grant Nos. DE-SC0019097 (WV) and DE-SC0014171 (HC) are gratefully acknowledged. SV and EW received financial support from the University of Florida Graduate School and the College of Agricultural & Life Sciences.
Acknowledgments
The authors thank Ben Broughton and Mike Boyette at the UF North Florida Research and Education Center-Suwannee Valley for preparing and managing the field plots, and Alyssa Davis for technical assistance with lab experiments.
Conflict of interest
The authors declare that the research was conducted in the absence of any commercial or financial relationships that could be construed as a potential conflict of interest.
Publisher’s note
All claims expressed in this article are solely those of the authors and do not necessarily represent those of their affiliated organizations, or those of the publisher, the editors and the reviewers. Any product that may be evaluated in this article, or claim that may be made by its manufacturer, is not guaranteed or endorsed by the publisher.
Supplementary material
The Supplementary Material for this article can be found online at: https://www.frontiersin.org/articles/10.3389/ffunb.2024.1437344/full#supplementary-material
References
Abreha K. B., Ortiz R., Carlsson A. S., Geleta M. (2021). Understanding the sorghum–Colletotrichum sublineola interactions for enhanced host resistance. Front. Plant Sci. 12, 641969. doi: 10.3389/fpls.2021.641969
Ahn E., Prom L. K., Odvody G., Magill C. (2019). Defense responses against the sorghum anthracnose pathogen in leaf blade and midrib tissue of johnsongrass and sorghum. Physiol. Mol. Plant Pathol. 106, 81–86. doi: 10.1016/j.pmpp.2018.12.008
Almagro Armenteros J. J., Tsirigos K. D., Sønderby C. K., Petersen T. N., Winther O., Brunak S., et al. (2019). SignalP 5.0 improves signal peptide predictions using deep neural networks. Nat. Biotechnol. 37, 420–423. doi: 10.1038/s41587-019-0036-z
Altschul S. F., Gish W., Miller W., Myers E. W., Lipman D. J. (1990). Basic local alignment search tool. J. Mol. Biol. 215, 403–410. doi: 10.1016/S0022-2836(05)80360-2
Anders S., Pyl P. T., Huber W. (2015). HTSeq: A Python framework to work with high-throughput sequencing data. Bioinformatics 31, 166–169. doi: 10.1093/bioinformatics/btu638
Andrews S. (2010). FastQC: A quality control tool for high throughput sequence data. Available online at: https://www.bioinformatics.babra ham.ac.uk/projects/fastqc.
Angajala A., Lim S., Phillips J. B., Kim J.-H., Yates C., You Z., et al. (2018). Diverse roles of mitochondria in immune responses: Novel insights into immuno-metabolism. Front. Immunol. 9. doi: 10.3389/fimmu.2018.01605
Ashburner M., Ball C. A., Blake J. A., Botstein D., Butler H., Cherry J. M., et al. (2000). Gene Ontology: tool for the unification of biology. Nat. Genet. 25, 25–29. doi: 10.1038/75556
Baldrich P., Chaya T., Caplan J. L., Meyers B. C. (2021). Genome assembly and transcriptome of Colletotrichum sublineola CsGL1, a new resource to study anthracnose disease in sorghum. Mol. Plant-Microbe Interact. 34, 1209–1211. doi: 10.1094/MPMI-04-21-0094-A
Baroncelli R., Sanz-Martín J. M., Rech G. E., Sukno S. A., Thon M. R. (2014). Draft genome sequence of Colletotrichum sublineola, a destructive pathogen of cultivated sorghum. Genome announcements 2, e00540–e00514. doi: 10.1128/genomeA.00540-14
Basavaraju P., Shetty N. P., Shetty H. S., De Neergaard E., Jørgensen H. J. L. (2009). Infection biology and defence responses in sorghum against Colletotrichum sublineolum. J. Appl. Microbiol. 107, 404–415. doi: 10.1111/jam.2009.107.issue-2
Beliën T., Van Campenhout S., Robben J., Volckaert G. (2006). Microbial endoxylanases: effective weapons to breach the plant cell-wall barrier or, rather, triggers of plant defense systems? Mol. Plant-Microbe Interact. 19, 1072–1081. doi: 10.1094/MPMI-19-1072
Benjamini Y., Hochberg Y. (1995). Controlling the false discovery rate: A practical and powerful approach to multiple testing. J. R. Stat. Society: Ser. B (Methodological) 57, 289–300. doi: 10.1111/j.2517-6161.1995.tb02031.x
Bhadauria V., Miraz P., Kennedy R., Banniza S., Wei Y. (2010). Dual trypan-aniline blue fluorescence staining methods for studying fungus-plant interactions. Biotech. Histochem. 85, 99–105. doi: 10.3109/10520290903132196
Blin K., Shaw S., Augustijn H. E., Reitz Z. L., Biermann F., Alanjary M., et al. (2023). antiSMASH 7.0: new and improved predictions for detection, regulation, chemical structures and visualisation. Nucleic Acids Res. 51, W46–W50. 10.1093/nar/gkad344
Blondel V. D., Guillaume J.-L., Lambiotte R., Lefebvre E. (2008). Fast unfolding of communities in large networks. J. Stat. Mechanics 2008, P10008. doi: 10.1088/1742-5468/2008/10/P10008
Bolger A. M., Lohse M., Usadel B. (2014). Trimmomatic: A flexible trimmer for Illumina sequence data. Bioinformatics 30, 2114–2120. doi: 10.1093/bioinformatics/btu170
Bolton M. D., Van Esse H. P., Vossen J. H., De Jonge R., Stergiopoulos I., Stulemeijer I. J. E., et al. (2008). The novel Cladosporium fulvum lysin motif effector Ecp6 is a virulence factor with orthologues in other fungal species. Mol. Microbiol. 69, 119–136. doi: 10.1111/j.1365-2958.2008.06270.x
Bu D., Luo H., Huo P., Wang Z., Zhang S., He Z., et al. (2021). KOBAS-i: intelligent prioritization and exploratory visualization of biological functions for gene enrichment analysis. Nucleic Acids Res. 49, W317–W325. doi: 10.1093/nar/gkab447
Buchfink B., Reuter K., Drost H.-G. (2021). Sensitive protein alignments at tree-of-life scale using DIAMOND. Nat. Methods 18, 366–368. doi: 10.1038/s41592-021-01101-x
Buchfink B., Xie C., Huson D. H. (2015). Fast and sensitive protein alignment using DIAMOND. Nat. Methods 12, 59–60. doi: 10.1038/nmeth.3176
Buiate E. A. S., Xavier K. V., Moore N., Torres M. F., Farman M. L., Schardl C. L., et al. (2017). A comparative genomic analysis of putative pathogenicity genes in the host-specific sibling species Colletotrichum graminicola and Colletotrichum sublineola. BMC Genomics 18, 67. doi: 10.1186/s12864-016-3457-9
Cantarel B. L., Coutinho P. M., Rancurel C., Bernard T., Lombard V., Henrissat B. (2009). The Carbohydrate-Active EnZymes database (CAZy): an expert resource for Glycogenomics. Nucleic Acids Res. 37, D233–D238. doi: 10.1093/nar/gkn663
Carpita N. C., Gibeaut D. M. (1993). Structural models of primary cell walls in flowering plants: consistency of molecular structure with the physical properties of the walls during growth. Plant J. 3, 1–30. doi: 10.1111/j.1365-313X.1993.tb00007.x
Castro E., Nieves I. U., Rondón V., Sagues W. J., Fernández-Sandoval M. T., Yomano L. P., et al. (2017). Potential for ethanol production from different sorghum cultivars. Ind. Crops Products 109, 367–373. doi: 10.1016/j.indcrop.2017.08.050
Collins T., Gerday C., Feller G. (2005). Xylanases, xylanase families and extremophilic xylanases. Fed. Eur. Microbiological Societies Microbiol. Rev. 29, 3–23. doi: 10.1016/j.femsre.2004.06.005
Colombatti F., Gonzalez D. H., Welchen E. (2014). Plant mitochondria under pathogen attack: A sigh of relief or a last breath? Mitochondrion 19, 238–244. doi: 10.1016/j.mito.2014.03.006
Corchete L. A., Rojas E. A., Alonso-López D., De Las Rivas J., Gutiérrez N. C., Burguillo F. J. (2020). Systematic comparison and assessment of RNA-seq procedures for gene expression quantitative analysis. Sci. Rep. 10, 19737. doi: 10.1038/s41598-020-76881-x
Csárdi G., Nepusz T., Müller K., Horvát S., Traag V., Zanini F., et al. (2006). The igraph software package for complex network research. Int. J. Complex Syst. Sci. 1695.
Cuevas H. E., Prom L. K., Cooper E. A., Knoll J. E., Ni X. (2018). Genome-wide association mapping of anthracnose (Colletotrichum sublineolum) resistance in the US sorghum association panel. Plant Genome 11, 170099. doi: 10.3835/plantgenome2017.11.0099
de Jonge R., Bolton M. D., Thomma B. P. (2011). How filamentous pathogens co-opt plants: the ins and outs of fungal effectors. Curr. Opin. Plant Biol. 14, 400–406. doi: 10.1016/j.pbi.2011.03.005
de Jonge R., Peter Van Esse H., Kombrink A., Shinya T., Desaki Y., Bours R., et al. (2010). Conserved fungal LysM effector Ecp6 prevents chitin-triggered immunity in plants. Science 329, 953–955. doi: 10.1126/science.1190859
Dornez E., Croes E., Gebruers K., De Coninck B., Cammue B. P. A., Delcour J. A., et al. (2010). Accumulated evidence substantiates a role for three classes of wheat xylanase inhibitors in plant defense. Crit. Rev. Plant Sci. 29, 244–264. doi: 10.1080/07352689.2010.487780
Felderhoff T. J., McIntyre L. M., Saballos A., Vermerris W. (2016). Using genotyping by sequencing to map two novel anthracnose resistance loci in Sorghum bicolor. G3 Genes|Genomes|Genetics 6, 1935–1946. doi: 10.1534/g3.116.030510
Fernandez J., Orth K. (2018). Rise of a cereal killer: The biology of Magnaporthe oryzae biotrophic growth. Trends Microbiol. 26, 582–597. doi: 10.1016/j.tim.2017.12.007
Finn R. D., Clements J., Eddy S. R. (2011). HMMER web server: interactive sequence similarity searching. Nucleic Acids Res. 39, W29–W37. doi: 10.1093/nar/gkr367
Fujii I. (2010). Functional analysis of fungal polyketide biosynthesis genes. J. Antibiotics 63, 207–218. doi: 10.1038/ja.2010.17
Furukawa K., Hoshi Y., Maeda T., Nakajima T., Abe K. (2005). Aspergillus nidulans HOG pathway is activated only by two-component signalling pathway in response to osmotic stress. Mol. Microbiol. 56, 1246–1261. doi: 10.1111/j.1365-2958.2005.04605.x
Gan P., Ikeda K., Irieda H., Narusaka M., O’Connell R. J., Narusaka Y., et al. (2013). Comparative genomic and transcriptomic analyses reveal the hemibiotrophic stage shift of Colletotrichum fungi. New Phytol. 197, 1236–1249. doi: 10.1111/nph.12085
Gene Ontology Consortium (2021). The Gene Ontology resource: enriching a GOld mine. Nucleic Acids Res. 49, D325–D334. doi: 10.1093/nar/gkaa1113
Gong Y., Fu Y., Xie J., Li B., Chen T., Lin Y., et al. (2022). Sclerotinia sclerotiorum SsCut1 modulates virulence and cutinase activity. J. Fungi (Basel) 8, 526. doi: 10.3390/jof8050526
Götz S., García-Gómez J. M., Terol J., Williams T. D., Nagaraj S. H., Nueda M. J., et al. (2008). High-throughput functional annotation and data mining with the Blast2GO suite. Nucleic Acids Res. 36, 3420–3435. doi: 10.1093/nar/gkn176
Gupta A., Chattoo B. B. (2008). Functional analysis of a novel ABC transporter ABC4 from Magnaporthe grisea. FEMS Microbiol. Lett. 278, 22–28. doi: 10.1111/fml.2008.278.issue-1
Hallgren J., Tsirigos K. D., Pedersen M. D., Almagro Armenteros J. J., Marcatili P., Nielsen H., et al. (2022). DeepTMHMM predicts alpha and beta transmembrane proteins using deep neural networks. bioRxiv. doi: 10.1101/2022.04.08.487609
Han J., Wang X., Wang F., Zhao Z., Li G., Zhu X., et al. (2021). The fungal effector Avr-Pita suppresses innate immunity by increasing COX activity in rice mitochondria. Rice 14, 12. doi: 10.1186/s12284-021-00453-4
Harris H. B., Johnson B. J., Dobson J. W., Luttrell E. S. (1964). Evaluation of anthracnose on grain sorghum. Crop Sci. 4, 460–462. doi: 10.2135/cropsci1964.0011183X000400050006x
Hood M. E., Shew H. D. (1996). Applications of KOH-aniline blue fluorescence in the study of plant-fungal interactions. Phytopathology 86, 704. doi: 10.1094/Phyto-86-704
Horton P., Park K.-J., Obayashi T., Fujita N., Harada H., Adams-Collier C. J., et al. (2007). WoLF PSORT: protein localization predictor. Nucleic Acids Res. 35, W585–W587. doi: 10.1093/nar/gkm259
Huang L., Backhouse D. (2004). Effects of Fusarium species on defence mechanisms in sorghum seedlings. NZPP 57, 121–124. doi: 10.30843/nzpp.2004.57
Jones J. D. G., Dangl J. L. (2006). The plant immune system. Nature 444, 323–329. doi: 10.1038/nature05286
Jumper J., Evans R., Pritzel A., Green T., Figurnov M., Ronneberger O., et al. (2021). Highly accurate protein structure prediction with AlphaFold. Nature 596, 583–589. doi: 10.1038/s41586-021-03819-2
Jwa N.-S., Hwang B. K. (2017). Convergent evolution of pathogen effectors toward reactive oxygen species signaling networks in plants. Front. Plant Sci. 8, 1687. doi: 10.3389/fpls.2017.01687
Kanehisa M., Goto S., Kawashima S., Okuno Y., Hattori M. (2004). The KEGG resource for deciphering the genome. Nucleic Acids Res. 32, D277–D280. doi: 10.1093/nar/gkh063
Khang C. H., Berruyer R., Giraldo M. C., Kankanala P., Park S.-Y., Czymmek K., et al. (2010). Translocation of Magnaporthe oryzae effectors into rice cells and their subsequent cell-to-cell movement. Plant Cell 22, 1388–1403. doi: 10.1105/tpc.109.069666
Kim S., Ahn I.-P., Rho H.-S., Lee Y.-H. (2005). MHP1, a Magnaporthe grisea hydrophobin gene, is required for fungal development and plant colonization. Mol. Microbiol. 57, 1224–1237. doi: 10.1111/j.1365-2958.2005.04750.x
Kim D., Langmead B., Salzberg S. L. (2015). HISAT: A fast spliced aligner with low memory requirements. Nat. Methods 12, 357–360. doi: 10.1038/nmeth.3317
Kim Y., Park S.-Y., Kim D., Choi J., Lee Y.-H., Lee J.-H., et al. (2013). Genome-scale analysis of ABC transporter genes and characterization of the ABCC type transporter genes in Magnaporthe oryzae. Genomics 101, 354–361. doi: 10.1016/j.ygeno.2013.04.003
Lanubile A., De Michele R., Loi M., Fakhari S., Marocco A., Paciolla C. (2022). Cell death induced by mycotoxin fumonisin B1 is accompanied by oxidative stress and transcriptional modulation in Arabidopsis cell culture. Plant Cell Rep. 41, 1733–1750. doi: 10.1007/s00299-022-02888-5
Lebeau F. J., Stokes L. E., Coleman O. H., Lebeau F. J., Stokes L. E., Coleman O. H. (1951). Anthracnose and red rot of Sorghum. Technical Bulletin No. 1035. United States Department of Agriculture, Economic Research Service. doi: 10.22004/AG.ECON.156409
Lee G. H., Min C. W., Yoo J. S., Jang J. W., Yoon J., Cho L., et al. (2024). Endo-1,4-beta-xylanase 1 (MoXYL1) secreted by Magnaporthe oryzae triggers defense responses and induces cell death in rice. Physiologia Plantarum 176, e14174. doi: 10.1111/ppl.14174
Lee Y.-J., Yamamoto K., Hamamoto H., Nakaune R., Hibi T. (2005). A novel ABC transporter gene ABC2 involved in multidrug susceptibility but not pathogenicity in rice blast fungus, Magnaporthe grisea. Pesticide Biochem. Physiol. 81, 13–23. doi: 10.1016/j.pestbp.2004.07.007
Levasseur A., Drula E., Lombard V., Coutinho P. M., Henrissat B. (2013). Expansion of the enzymatic repertoire of the CAZy database to integrate auxiliary redox enzymes. Biotechnol. Biofuels 6, 41. doi: 10.1186/1754-6834-6-41
Li L., Zhu F., Liu H., Chu A., Lo C. (2013). Isolation and expression analysis of defense-related genes in sorghum–Colletotrichum sublineolum interaction. Physiol. Mol. Plant Pathol. 84, 123–130. doi: 10.1016/j.pmpp.2013.08.005
Liang D., Qi Z., Du Y., Yu J., Yu M., Zhang R., et al. (2022). Identification of differentially expressed genes reveal conserved mechanisms in the rice-Magnaporthe oryzae interaction. Front. Plant Sci. 13. doi: 10.3389/fpls.2022.723356
Liu N., Qi L., Huang M., Chen D., Yin C., Zhang Y., et al. (2022). Comparative secretome analysis of Magnaporthe oryzae identified proteins involved in virulence and cell wall integrity. Genomics Proteomics Bioinf. 20, 728–746. doi: 10.1016/j.gpb.2021.02.007
Lombard V., Golaconda Ramulu H., Drula E., Coutinho P. M., Henrissat B. (2014). The carbohydrate-active enzymes database (CAZy) in 2013. Nucleic Acids Res. 42, D490–D495. doi: 10.1093/nar/gkt1178
Lu X., Miao J., Shen D., Dou D. (2022). Proteinaceous effector discovery and characterization in plant pathogenic Colletotrichum fungi. Front. Microbiol. 13. doi: 10.3389/fmicb.2022.914035
Ma D., Li R. (2013). Current understanding of HOG-MAPK pathway in Aspergillus fumigatus. Mycopathologia 175, 13–23. doi: 10.1007/s11046-012-9600-5
McCormick R. F., Truong S. K., Sreedasyam A., Jenkins J., Shu S., Sims D., et al. (2018). The Sorghum bicolor reference genome: Improved assembly, gene annotations, a transcriptome atlas, and signatures of genome organization. Plant J. 93, 338–354. doi: 10.1111/tpj.13781
Mentlak T. A., Kombrink A., Shinya T., Ryder L. S., Otomo I., Saitoh H., et al. (2012). Effector-mediated suppression of chitin-triggered immunity by Magnaporthe oryzae is necessary for rice blast disease. Plant Cell. 24, 322–335. doi: 10.1105/tpc.111.092957
Mesarich C. H., Barnes I., Bradley E. L., De La Rosa S., De Wit P. J. G. M., Guo Y., et al. (2023). Beyond the genomes of Fulvia fulva (syn. Cladosporium fulvum) and Dothistroma septosporum: New insights into how these fungal pathogens interact with their host plants. Mol. Plant Pathol. 24, 474–494. doi: 10.1111/mpp.13309
Mirdita M., Schütze K., Moriwaki Y., Heo L., Ovchinnikov S., Steinegger M. (2022). ColabFold: making protein folding accessible to all. Nat. Methods 19, 679–682. doi: 10.1038/s41592-022-01488-1
Mohanty A., Tiwari-Pandey R., Pandey N. R. (2019). Mitochondria: the indispensable players in innate immunity and guardians of the inflammatory response. J. Cell Communication Signaling 13, 303–318. doi: 10.1007/s12079-019-00507-9
Moore J. W., Ditmore M., TeBeest D. O. (2008). Pathotypes of colletotrichum sublineolum in Arkansas. Plant Dis. 92, 1415–1420. doi: 10.1094/PDIS-92-10-1415
Münch S., Lingner U., Floss D. S., Ludwig N., Sauer N., Deising H. B. (2008). The hemibiotrophic lifestyle of Colletotrichum species. J. Plant Physiol. 165, 41–51. doi: 10.1016/j.jplph.2007.06.008
Nguyen Ba A. N., Pogoutse A., Provart N., Moses A. M. (2009). NLStradamus: a simple Hidden Markov Model for nuclear localization signal prediction. BMC Bioinf. 10, 202. doi: 10.1186/1471-2105-10-202
Nguyen T.V., Kröger C., Bönnighausen J., Schäfer W., Bormann J. (2013). The ATF/CREB transcription factor Atf1 is essential for full virulence, deoxynivalenol production, and stress tolerance in the cereal pathogen Fusarium graminearum. Mol Plant Microbe Interact. 26, 1378–1394. doi: 10.1094/MPMI-04-13-0125-R
Nielsen K. A., Gotfredsen C. H., Buch-Pedersen M. J., Ammitzbøll H., Mattsson O., Duus J.Ø., et al. (2005). Inclusions of flavonoid 3-deoxyanthocyanidins in Sorghum bicolor self-organize into spherical structures. Physiol. Mol. Plant Pathol. 65, 187–196. doi: 10.1016/j.pmpp.2005.02.001
O’Connell R. J., Thon M. R., Hacquard S., Amyotte S. G., Kleemann J., Torres M. F., et al. (2012). Lifestyle transitions in plant pathogenic Colletotrichum fungi deciphered by genome and transcriptome analyses. Nat. Genet. 44, 1060–1065. doi: 10.1038/ng.2372
OmicsBox (2019). OmicsBox – bioinformatics made easy (Valencia, Spain: BioBam Bioinformatics). Available at: https://www.biobam.com/omicsbox/?cn-reloaded=1.
Ospina-Giraldo M. D., Griffith J. G., Laird E. W., Mingora C. (2010). The CAZyome of Phytophthora spp.: A comprehensive analysis of the gene complement coding for carbohydrate-active enzymes in species of the genus Phytophthora. BMC Genomics 11, 525. doi: 10.1186/1471-2164-11-525
Park B. H., Karpinets T. V., Syed M. H., Leuze M. R., Uberbacher E. C. (2010). CAZymes Analysis Toolkit (CAT): web service for searching and analyzing carbohydrate-active enzymes in a newly sequenced organism using CAZy database. Glycobiology 20 (12), 1574–1584. doi: 10.1093/glycob/cwq106
Pastor-Corrales M., Frederiksen R. (1978). “Sorghum anthracnose,” in sorghum diseases: a world review (Proceedings of International Workshop on Sorghum Diseases), 289–291.
Paterson A. H., Bowers J. E., Bruggmann R., Dubchak I., Grimwood J., Gundlach H., et al. (2009). The Sorghum bicolor genome and the diversification of grasses. Nature 457, 551–556. doi: 10.1038/nature07723
Pierleoni A., Martelli P. L., Casadio R. (2008). PredGPI: a GPI-anchor predictor. BMC Bioinf. 9, 392. doi: 10.1186/1471-2105-9-392
Proctor R. H., Brown D. W., Plattner R. D., Desjardins A. E. (2003). Co-expression of 15 contiguous genes delineates a fumonisin biosynthetic gene cluster in Gibberella moniliformis. Fungal Genet. Biol. 38, 237–249. doi: 10.1016/S1087-1845(02)00525-X
Prom L. K., Ahn E. J. S., Perumal R., Cuevas H. E., Rooney W. L., Isakeit T. S., et al. (2024). Genetic diversity and classification of Colletotrichum sublineola pathotypes using a standard set of sorghum differentials. J. Fungi 10, 3. doi: 10.3390/jof10010003
Rafiei V., Vélëz H., Tzelepis G. (2021). The role of glycoside hydrolases in phytopathogenic fungi and oomycetes virulence. Int. J. Mol. Sci. 22, 9359. doi: 10.3390/ijms22179359
Rees D. C., Johnson E., Lewinson O. (2009). ABC transporters: the power to change. Nat. Rev. Mol. Cell Biol. 10, 218–227. doi: 10.1038/nrm2646
Reverberi M., Fabbri A. A., Zjalic S., Ricelli A., Punelli F., Fanelli C. (2005). Antioxidant enzymes stimulation in Aspergillus parasiticus by Lentinula edodes inhibits aflatoxin production. Appl Microbiol. Biotechnol. 69, 207–215. doi: 10.1007/s00253-005-1979-1
Reverberi M., Ricelli A., Zjalic S., Fabbri A. A., Fanelli C. (2010). Natural functions of mycotoxins and control of their biosynthesis in fungi. Appl. Microbiol. Biotechnol. 87, 899–911. doi: 10.1007/s00253-010-2657-5
Robinson M. D., McCarthy D. J., Smyth G. K. (2010). EdgeR: A bioconductor package for differential expression analysis of digital gene expression data. Bioinformatics 26, 139–140. doi: 10.1093/bioinformatics/btp616
Rosewich U. L., Pettway R. E., McDonald B. A., Duncan R. R., Frederiksen R. A. (1998). Genetic structure and temporal dynamics of a Colletotrichum graminicola population in a sorghum disease nursery. Phytopathology. 88, 1087–1093. doi: 10.1094/PHYTO.1998.88.10.1087
Sánchez-Vallet A., McDonald M. C., Solomon P. S., McDonald B. A. (2015). Is Zymoseptoria tritici a hemibiotroph? Fungal Genet. Biol. 79, 29–32. doi: 10.1016/j.fgb.2015.04.001
Schumann U., Smith N. A., Wang M.-B. (2013). A fast and efficient method for preparation of high-quality RNA from fungal mycelia. BMC Res. Notes 6, 71. doi: 10.1186/1756-0500-6-71
Segmüller N., Ellendorf U., Tudzynski B., Tudzynski P. (2007). BcSAK1, a Stress-Activated Mitogen-Activated Protein Kinase, is involved in vegetative differentiation and pathogenicity in Botrytis cinerea. Eukaryotic Cell 6, 211–221. doi: 10.1128/EC.00153-06
Shabbir A., Batool W., Yu D., Lin L., An Q., Xiaomin C., et al. (2022). Magnaporthe oryzae chloroplast targeting endo-β-1,4-xylanase I MoXYL1A regulates conidiation, appressorium maturation and virulence of the rice blast fungus. Rice 15, 44. doi: 10.1186/s12284-022-00584-2
Sidar A., Albuquerque E. D., Voshol G. P., Ram A. F. J., Vijgenboom E., Punt P. J. (2020). Carbohydrate Binding Modules: diversity of domain architecture in amylases and cellulases from filamentous microorganisms. Front. Bioengineering Biotechnol. 8. doi: 10.3389/fbioe.2020.00871
Sperschneider J., Dodds P. N. (2022). EffectorP 3.0: Prediction of apoplastic and cytoplasmic effectors in fungi and oomycetes. Mol. Plant-Microbe Interact. 35, 146–156. doi: 10.1094/MPMI-08-21-0201-R
Stutts L. R., Vermerris W. (2020). Elucidating anthracnose resistance mechanisms in sorghum—A review. Phytopathology. 110, 1863–1876. doi: 10.1094/PHYTO-04-20-0132-RVW
Sun C. B., Suresh A., Deng Y. Z., Naqvi N. I. (2007). A multidrug resistance transporter in Magnaporthe is required for host penetration and for survival during oxidative stress. Plant Cell. 18, 3686–3705. doi: 10.1105/tpc.105.037861
Tanaka S., Brefort T., Neidig N., Djamei A., Kahnt J., Vermerris W., et al. (2014). A secreted Ustilago maydis effector promotes virulence by targeting anthocyanin biosynthesis in maize. eLife 3, e01355. doi: 10.7554/eLife.01355
Tang D., Chen M., Huang X., Zhang G., Zeng L., Zhang G., et al. (2023). SRplot: A free online platform for data visualization and graphing. PloS One 18, e0294236. doi: 10.1371/journal.pone.0294236
Tesso T. T., Perumal R., Little C. R., Adeyanju A., Radwan G. L., Prom L. K., et al. (2012). Sorghum pathology and biotechnology - a fungal disease perspective: Part II. Anthracnose, stalk rot, and downy mildew. Eur. J. Plant Sci. Biotechnol. 6, 31–44.
Teulet A., Quan C., Evangelisti E., Wanke A., Yang W., Schornack S. (2023). A pathogen effector FOLD diversified in symbiotic fungi. New Phytologist 239, 1127–1139. doi: 10.1111/nph.18996
Thakur R., Rao V., Wu S., Subbara K., Mathur K., Tailor H., et al. (2007). Genetic resistance to foliar anthracnose in sorghum and pathogenic variability in Colletotrichum graminicola. Indian Phytopathol. 60, 13–23.
Tierney L., Linde J., Müller S., Brunke S., Molina J. C., Hube B., et al. (2012). An interspecies regulatory network inferred from simultaneous RNA-seq of Candida albicans invading innate immune cells. Front. Microbiol. 3. doi: 10.3389/fmicb.2012.00085
Tugizimana F., Steenkamp P. A., Piater L. A., Labuschagne N., Dubery I. A. (2019). Unravelling the metabolic reconfiguration of the post-challenge primed state in Sorghum bicolor responding to Colletotrichum sublineolum infection. Metabolites 9, 194. doi: 10.3390/metabo9100194
Urban M. (1999). An ATP-driven efflux pump is a novel pathogenicity factor in rice blast disease. EMBO J. 18, 512–521. doi: 10.1093/emboj/18.3.512
Urban M., Cuzick A., Seager J., Wood V., Rutherford K., Venkatesh S. Y., et al. (2019). PHI-base: the pathogen–host interactions database. Nucleic Acids Res. 48 (D1), D613–D620. doi: 10.1093/nar/gkz904
Valèrio H. M., Rèsende M. A., Weikert-Oliveira R. C. B., Casela C. R. (2005). Virulence and molecular diversity in Colletotrichum graminicola from Brazil. Mycopathologia 159, 449–459. doi: 10.1007/s11046-005-0373-y
van Kempen M., Kim S. S., Tumescheit C., Mirdita M., Lee J., Gilchrist C. L. M., et al. (2024). Fast and accurate protein structure search with Foldseek. Nat. Biotechnol. 42, 243–246. doi: 10.1038/s41587-023-01773-0
Vogel J. (2008). Unique aspects of the grass cell wall. Curr. Opin. Plant Biol. 11, 301–307. doi: 10.1016/j.pbi.2008.03.002
Walton J. D. (1994). Deconstructing the cell wall. Plant Physiol. 104, 1113–1118. doi: 10.1104/pp.104.4.1113
Wang L., Chen M., Zhu F., Fan T., Zhang J., Lo C. (2020). Alternative splicing is a Sorghum bicolor defense response to fungal infection. Planta. 251, 14. doi: 10.1007/s00425-019-03309-w
Wang C., Liu Y., Liu L., Wang Y., Yan J., Wang C., et al. (2019). The biotrophy-associated secreted protein 4 (BAS4) participates in the transition of Magnaporthe oryzae from the biotrophic to the necrotrophic phase. Saudi J. Biol. Sci. 26, 795–807. doi: 10.1016/j.sjbs.2019.01.003
Wang J., Zhou G., Ying S., Feng M. (2013). P-type calcium ATPase functions as a core regulator of Beauveria bassiana growth, conidiation and responses to multiple stressful stimuli through cross-talk with signalling networks. Environ. Microbiol. 15, 967–979. doi: 10.1111/1462-2920.12044
Wang J., Zhu X.-G., Ying S.-H., Feng M.-G. (2017). Differential roles for six P-type calcium ATPases in sustaining intracellular Ca2+ homeostasis, asexual cycle and environmental fitness of Beauveria bassiana. Sci. Rep. 7, 1420. doi: 10.1038/s41598-017-01570-1
Westermann A. J., Gorski S. A., Vogel J. (2012). Dual RNA-seq of pathogen and host. Nat. Rev. Microbiol. 10, 618–630. doi: 10.1038/nrmicro2852
Wharton P. S., Julian A. M., O’Connell R. J. (2001). Ultrastructure of the infection of Sorghum bicolor by Colletotrichum sublineolum. Phytopathology 91, 149–158. doi: 10.1094/PHYTO.2001.91.2.149
Win J., Chaparro-Garcia A., Belhaj K., Saunders D. G. O., Yoshida K., Dong S., et al. (2012). Effector biology of plant-associated organisms: Concepts and perspectives. Cold Spring Harbor Symp. Quantitative Biol. 77, 235–247. doi: 10.1101/sqb.2012.77.015933
Winnenburg R., Baldwin T. K., Urban M., Rawlings C., Köhler J., Hammond-Kosack K. E. (2006). PHI-base: a new database for pathogen host interactions. Nucleic Acids Res. 34, D459–D464. doi: 10.1093/nar/gkj047
Wolf E. S. A., Vela S., Cuevas H. E., Vermerris W. (2024). A sorghum F-box protein induces an oxidative burst in the defense against Colletotrichum sublineola. Phytopathology. 114, 405–417. doi: 10.1094/PHYTO-06-23-0184-R
Xu G., Zhong X., Shi Y., Liu Z., Jiang N., Liu J., et al. (2020). A fungal effector targets a heat shock-dynamin protein complex to modulate mitochondrial dynamics and reduce plant immunity. Sci. Adv. 6, eabb7719. doi: 10.1126/sciadv.abb7719
Yazawa T., Kawahigashi H., Matsumoto T., Mizuno H. (2013). Simultaneous transcriptome analysis of sorghum and Bipolaris sorghicola by using RNA-seq in combination with de novo transcriptome assembly. PLoS ONE. 8, e62460. doi: 10.1371/journal.pone.0062460
Yip V. L., Withers S. G. (2006). Breakdown of oligosaccharides by the process of elimination. Curr. Opin. Chem. Biol. 10, 147–155. doi: 10.1016/j.cbpa.2006.02.005
Yuan Q., Yan Y., Sohail M. A., Liu H., Huang J., Hsiang T., et al. (2021). A novel hexose transporter ChHxt6 is required for hexose uptake and virulence in Colletotrichum higginsianum. Int. J. Mol. Sci. 22, 5963. doi: 10.3390/ijms22115963
Zheng J., Ge Q., Yan Y., Zhang X., Huang L., Yin Y. (2023). dbCAN3: automated carbohydrate-active enzyme and substrate annotation. Nucleic Acids Res. 51, W115–W121. doi: 10.1093/nar/gkad328
Keywords: anthracnose, defense, effector, hemibiotroph, immunity, Sorghum bicolor, transcriptomics
Citation: Vela S, Wolf ESA, Rollins JA, Cuevas HE and Vermerris W (2024) Dual-RNA-sequencing to elucidate the interactions between sorghum and Colletotrichum sublineola. Front. Fungal Biol. 5:1437344. doi: 10.3389/ffunb.2024.1437344
Received: 23 May 2024; Accepted: 19 July 2024;
Published: 16 August 2024.
Edited by:
Lars Matthias Voll, University of Marburg, GermanyReviewed by:
Jie Zhu, University of California, Davis, United StatesBenjamin A. Horwitz, Technion Israel Institute of Technology, Israel
Copyright © 2024 Vela, Wolf, Rollins, Cuevas and Vermerris. This is an open-access article distributed under the terms of the Creative Commons Attribution License (CC BY). The use, distribution or reproduction in other forums is permitted, provided the original author(s) and the copyright owner(s) are credited and that the original publication in this journal is cited, in accordance with accepted academic practice. No use, distribution or reproduction is permitted which does not comply with these terms.
*Correspondence: Wilfred Vermerris, wev@ufl.edu
†ORCID: Saddie Vela, orcid.org/0000-0001-9504-7159
Emily S. A. Wolf, orcid.org/0000-0002-0331-3788
Jeffrey A. Rollins, orcid.org/0000-0002-9897-6292
Hugo E. Cuevas, orcid.org/0000-0001-7272-6933
Wilfred Vermerris, orcid.org/0000-0002-4582-3436