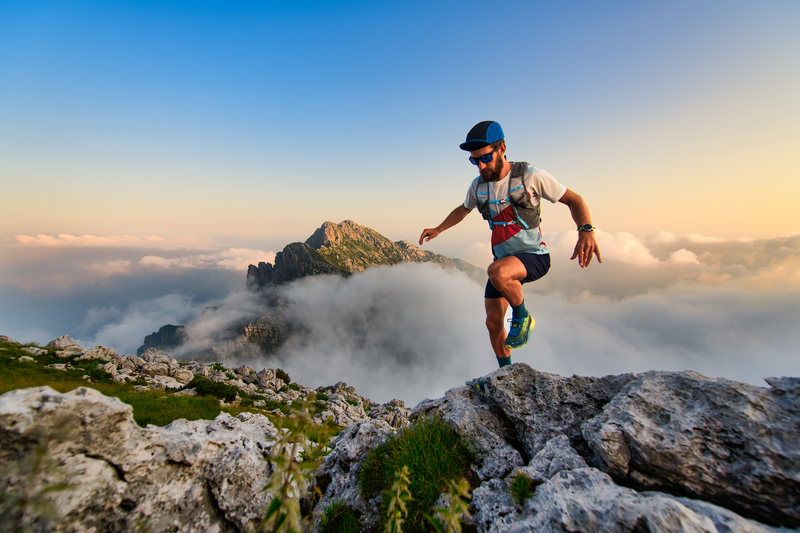
94% of researchers rate our articles as excellent or good
Learn more about the work of our research integrity team to safeguard the quality of each article we publish.
Find out more
ORIGINAL RESEARCH article
Front. Freshw. Sci.
Sec. Freshwater Species Evolution and Ecology
Volume 3 - 2025 | doi: 10.3389/ffwsc.2025.1528481
This article is part of the Research Topic Editors' Showcase: Freshwater Species Evolution and Ecology View all articles
The final, formatted version of the article will be published soon.
You have multiple emails registered with Frontiers:
Please enter your email address:
If you already have an account, please login
You don't have a Frontiers account ? You can register here
Identifying commonalities in how fish navigate rivers near infrastructure will enhance water operations and design by improving our ability to predict engineering outcomes (e.g. barrier construction/removal, fish passage installation) in novel settings before the cost of real-world implementation. Evidence from intermediate-scale computer models (time scales of minutes to days and spatial scales less than 2 km) suggests that fish movement behaviour in rivers is frequently governed by responses to one or more of the following hydrodynamic features: (1) flow direction (i.e., rheotaxis), (2) flow velocity magnitude, (3) turbulence, and (4) depth, plus (5) the integration of information over recent time periods (i.e., memory/experience).However, the lack of consistent modelling approaches, infrequent assessment of each response in isolation and combination, and a focus on a limited number of species means the generality of these responses is uncertain. We use a computer model, specifically a patternoriented modelling approach incorporating individual based models (IBMs), to apply responses to the four hydrodynamic features plus memory/experience in different combinations to study their value for reproducing the movement of an infrequently modelled species and lifestage, upriver migrating adult sea lamprey, Petromyzon marinus. Our analysis indicates that rheotaxis and a response to velocity magnitude as well as recent past experience improve sea lamprey spatio-temporal movement prediction compared to other, simpler forms of modelled behaviour. Sea lamprey movement is also biased toward lower levels of turbulence (e.g., turbulent kinetic energy) or its precursor (i.e., the spatial gradient in water speed). A response to water depth was not found to be important, but the modelled domain was two-dimensional which limited our assessment. As similar responses to hydrodynamic features are found in very different fish, commonalities appear to underlie river navigation across a range of species and life stages that share the goal-oriented behaviour of upriver and downriver movement. The systematic approach of our analysis highlights the accuracy trade-offs of each response, individually and in combination, that often accompany alternative behavioural formulations in a computer model of fish movement. The model structure provides a framework to which future findings from the analyses of additional species in different contexts can be added.
Keywords: modelling, Telemetry, Fish movement behaviour, Flow speed, rheotaxis, Turbulence, Memory, Lamprey
Received: 14 Nov 2024; Accepted: 17 Feb 2025.
Copyright: © 2025 Kerr, Zielinski, Holbrook, Goodwin and McLaughlin. This is an open-access article distributed under the terms of the Creative Commons Attribution License (CC BY). The use, distribution or reproduction in other forums is permitted, provided the original author(s) or licensor are credited and that the original publication in this journal is cited, in accordance with accepted academic practice. No use, distribution or reproduction is permitted which does not comply with these terms.
* Correspondence:
James R Kerr, University of Guelph, Guelph, Canada
Disclaimer: All claims expressed in this article are solely those of the authors and do not necessarily represent those of their affiliated organizations, or those of the publisher, the editors and the reviewers. Any product that may be evaluated in this article or claim that may be made by its manufacturer is not guaranteed or endorsed by the publisher.
Research integrity at Frontiers
Learn more about the work of our research integrity team to safeguard the quality of each article we publish.