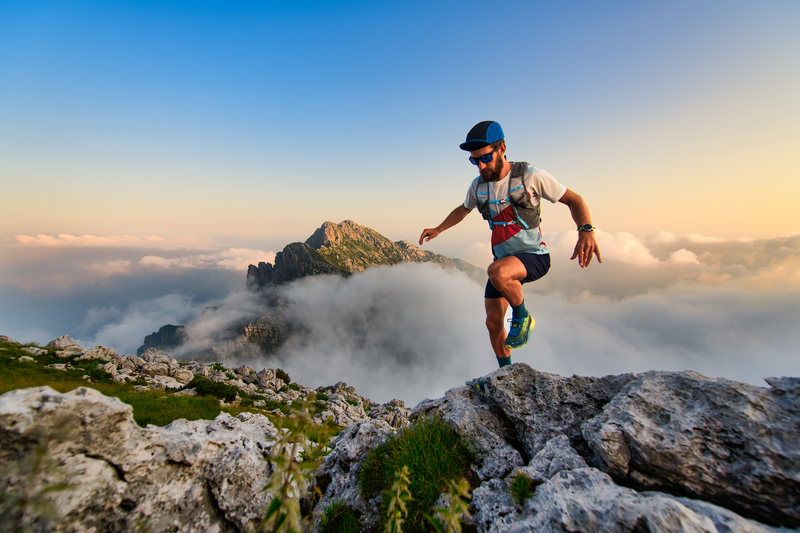
94% of researchers rate our articles as excellent or good
Learn more about the work of our research integrity team to safeguard the quality of each article we publish.
Find out more
ORIGINAL RESEARCH article
Front. For. Glob. Change
Sec. Forest Growth
Volume 8 - 2025 | doi: 10.3389/ffgc.2025.1566459
The final, formatted version of the article will be published soon.
You have multiple emails registered with Frontiers:
Please enter your email address:
If you already have an account, please login
You don't have a Frontiers account ? You can register here
Forest stocking guidelines traditionally reference self-thinning lines representing the tradeoff between maximum trees per unit area vs. maximum mean tree size for even-aged stands. While selfthinning lines are roughly linear on logarithmic scales, certain forest types display a curvilinear "mature stand boundary" (MSB). The existence of the MSB suggests that beyond self-thinning, processes such as recruitment limitation, density-independent mortality, and their interactions with site quality may also contribute to a more universal maximum size-density boundary (MSDB). To advance forest modeling and the management of mature stands under global change, we investigated: 1) how the MSDB may differ as stands biologically mature in response to climate and N deposition, 2) whether mortality and recruitment contribute to the curvilinearity of the MSDB. To accomplish this, we compiled forest inventory, climate, and total N deposition data for four western U.S. forest types (California mixed-conifer, ponderosa pine, Douglas-fir, and pinyon-juniper). We examined three aspects of climate: thermal loading, aridity, and seasonality of precipitation. We used 0.95 quantile regression to model the MSDB and generalized linear modeling for mortality and recruitment. Unlike studies of even-aged stands that found abrupt MSBs, we found evidence for curvilinear MSDBs in all four forest types, with climate and/or N deposition modulating the degree of curvilinearity. Aridity constrained maximum stocking in medium-large diameter stands of California mixed-conifer and Douglas-fir, while higher growing-season precipitation constrained maximum stocking in large-diameter ponderosa pine. Heavier N deposition lowered maximum stocking in large-diameter stands of California mixed-conifer and pinyon-juniper. In California mixed-conifer and Douglas-fir, N deposition steepened the slope of the MSDB in small-diameter stands. Mortality was consistent along the MSDB for ponderosa pine, concentrated in large-diameter California mixed-conifer and Douglas-fir stands, and small-diameter pinyon-juniper stands. Recruitment was elevated in small-diameter stands of all four forest types. Our results support roles for both mortality and recruitment in driving curvilinear MSDBs. Our findings caution against assuming that self-thinning consistently defines the MSDB throughout stand development, while having important implications for the management of mature and old-growth stands under global change, especially at extremes of resource availability where the limitations of traditional tools may be most acute.
Keywords: Self-thinning boundary line, Stand density index, mature and old-growth forest, Mortality and recruitment, nitrogen deposition, quantile regression, ponderosa pine, Douglasfir
Received: 24 Jan 2025; Accepted: 12 Mar 2025.
Copyright: © 2025 Looney and Shaw. This is an open-access article distributed under the terms of the Creative Commons Attribution License (CC BY). The use, distribution or reproduction in other forums is permitted, provided the original author(s) or licensor are credited and that the original publication in this journal is cited, in accordance with accepted academic practice. No use, distribution or reproduction is permitted which does not comply with these terms.
* Correspondence:
Christopher E Looney, Pacific Southwest Research Station, Forest Service (USDA), Albany, United States
Disclaimer: All claims expressed in this article are solely those of the authors and do not necessarily represent those of their affiliated organizations, or those of the publisher, the editors and the reviewers. Any product that may be evaluated in this article or claim that may be made by its manufacturer is not guaranteed or endorsed by the publisher.
Research integrity at Frontiers
Learn more about the work of our research integrity team to safeguard the quality of each article we publish.