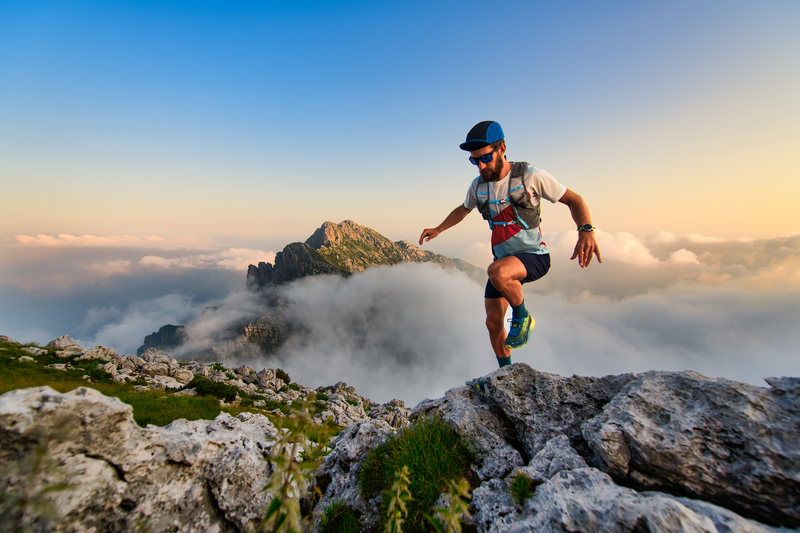
95% of researchers rate our articles as excellent or good
Learn more about the work of our research integrity team to safeguard the quality of each article we publish.
Find out more
ORIGINAL RESEARCH article
Front. For. Glob. Change
Sec. Forest Management
Volume 8 - 2025 | doi: 10.3389/ffgc.2025.1431603
This article is part of the Research Topic Advancing Biodiversity Assessment in Protected Areas through Remote Sensing Techniques View all articles
The final, formatted version of the article will be published soon.
You have multiple emails registered with Frontiers:
Please enter your email address:
If you already have an account, please login
You don't have a Frontiers account ? You can register here
The unmanned aerial vehicle -based light detection and ranging (UAV-LiDAR) can quickly acquire the three-dimensional information of large areas of vegetation, and has been widely used in tree species classification. UAV-LiDAR point clouds of Populus alba, Populus simonii, Pinus sylvestris, and Pinus tabuliformis from 12 sample plots, 2622 tree in total, were obtained in North China, training and testing sets were constructed through data pre-processing, individual tree segmentation, feature extraction, Non-uniform Grid and Farther Point Sampling (NGFPS), and then four tree species were classified efficiently by two machine learning algorithms and two deep learning algorithms. Results showed that PointMLP achieved the best accuracy for identification of the tree species (overall accuracy = 96.94%), followed by RF (overall accuracy = 95.62%), SVM (overall accuracy = 94.89%) and PointNet++(overall accuracy = 85.65%). In addition, the most suitable number of point cloud sampling of single tree is between 1024 and 2048 when using the NGFPS method in the two deep learning models. Furthermore, feature value of elev_percentile_99th has an important influence on tree species classification and tree species with similar crown structures may lead to a higher misidentification rate. The study underscores the efficiency of PointMLP as a robust and streamlined solution, which offers a novel technological support for tree species classification in forestry resource management.
Keywords: deep learning, Tree species, Classification, UAV-LiDAR, point cloud
Received: 12 May 2024; Accepted: 11 Mar 2025.
Copyright: © 2025 Zhang, Liu, Yang, Guo, Hu, Zhang, Yang and Zhang. This is an open-access article distributed under the terms of the Creative Commons Attribution License (CC BY). The use, distribution or reproduction in other forums is permitted, provided the original author(s) or licensor are credited and that the original publication in this journal is cited, in accordance with accepted academic practice. No use, distribution or reproduction is permitted which does not comply with these terms.
* Correspondence:
Zhenhua Hu, College of Forestry, Shanxi Agricultural University, Taigu, China
Disclaimer: All claims expressed in this article are solely those of the authors and do not necessarily represent those of their affiliated organizations, or those of the publisher, the editors and the reviewers. Any product that may be evaluated in this article or claim that may be made by its manufacturer is not guaranteed or endorsed by the publisher.
Research integrity at Frontiers
Learn more about the work of our research integrity team to safeguard the quality of each article we publish.