- 1Ayancik Vocational School, Department of Forestry, Program of Forestry and Forest Products, Sinop University, Sinop, Türkiye
- 2Faculty of Bartin Forestry, Department of Forest Engineering, Bartin University, Bartin, Türkiye
- 3Faculty of Engineering, Architecture and Design, Department of Landscape Architecture, Bartin University, Bartin, Türkiye
- 4General Directorate of Forestry, Directorate of Forestry Administration and Planning Department, Ankara, Türkiye
Introduction: Monitoring LULC changes is crucial for developing strategies for natural resource management, assessing the current potential of a region, and addressing global environmental issues. In this context, this study examines land use and land cover (LULC) changes in forest and non-forest areas of Anamur district, located in the Mediterranean Region of Türkiye, between 2000 and 2020.
Methods: Using the intensity analysis method, which offers a detailed and efficient approach to understanding LULC changes, the study analyzes transitions at interval, category, and transition levels. LULC maps were generated through supervised classification of Landsat satellite images, focusing on seven classes: Coniferous, Broad-Leaved, Mixed, Treeless Gap, Settlement, Agriculture, and Water. The analysis evaluated changes within and between these categories, interpreting the results through graphical outputs. The driving forces behind these changes were also explored, and their underlying causes were discussed.
Results and Discussion: Results at the interval level revealed that the most significant changes occurred during the 2000-2010 period. At the category level, the Coniferous category exhibited the highest degree of change in both intervals. During 2000-2010, Coniferous gains predominantly replaced non-forest areas (Agriculture, Settlement, and Water), while this pattern was less evident in 2010-2020. In contrast, Treeless Gap gains primarily replaced Coniferous areas during 2010-2020, while no significant losses in Treeless Gap were targeted by other categories. Broad-Leaved species were found to heavily target Water losses, likely due to their higher water demands compared to Coniferous species, as supported by prior studies. This research highlights the advantages of intensity analysis in LULC studies, offering insights into spatial changes and their intensity across categories. It aims to promote its adoption and underscores the importance of targeted conservation and land management strategies to mitigate the impacts of forest loss, land use changes, and water resource pressures.
1 Introduction
Environmental problems, especially global warming, have reached a level that threatens all living things. Their effects are now felt intensely all over the world. Land use and land cover (LULC) changes, which are caused by the mutual interaction of natural processes and human impact (Dewan and Yamaguchi, 2009; Halmy et al., 2015; Zheng et al., 2015; Karimi et al., 2018; Jasim, 2025), represent one of the most current and threatening problems (Agarwal et al., 2002; Zadbagher et al., 2018). LULC changes have proven to be the main force for driving environmental changes (Verburg et al., 2015; Ren et al., 2019; Kaptan, 2021). The studies carried out by many researchers from different disciplines show that LULC changes are associated with environment and ecology (Weng, 2001; Hassan et al., 2016; Twisa and Buchroithner, 2019), climate (Tasser et al., 2017; Garg et al., 2019; Gogoi et al., 2019; Bozdogan Sert et al., 2021; Aksoy et al., 2022), biodiversity (Bailey et al., 2016; Marshall et al., 2018; Verstegen et al., 2019; Jung et al., 2020), and they directly or indirectly affect water supply and quality (Patra et al., 2018; Wu et al., 2018; de Mello et al., 2020; Ayek and Zerouali, 2025; Ameen et al., 2025), therefore, revealed that it is key in terms of various applications such as agriculture, forestry, hydrology, geology and ecology (Weng, 2001; Hassan et al., 2016; Twisa and Buchroithner, 2019).
In recent years, LULC changes have become one of the most important research topics, especially in the fields of forestry, landscape planning and climate change adaptation and mitigation (Turner et al., 1994; Watson et al., 2000; Doaemo et al., 2020; Bozdogan Sert et al., 2021; Aksoy et al., 2022; Siddika and Sresto, 2025). Monitoring LULC changes and processes is very significant in terms of determining strategies and policies for natural resource management [Sinha et al., 2015; Organisation for Economic Co-operation and Development (OECD), 2018], providing various information about the current potential of a particular region and global environmental problems (Mialhe et al., 2015; Martins et al., 2016; Nasiri et al., 2019; Keçecioğlu Dağlı and Cengiz, 2022). Especially considering the climate changes in recent years, more consistent data are required about the intensity and causes of LULC changes to develop policies to be obeyed within the scope of combating climate change (Kale et al., 2016; Aksoy and Kaptan, 2021; Bozdogan Sert et al., 2021; Aksoy et al., 2022). For this reason, many researchers from different disciplines have focused on revealing the causes and consequences of land use changes, and investigating their possible location and extension in the coming years (Verburg et al., 2004; Aksoy and Kaptan, 2020; Bozdogan Sert et al., 2021; Keçecioğlu Dağlı, 2021; Aksoy et al., 2022).
Today, it is known that 50% of the earth’s surface has changed due to direct human use such as agriculture, grazing, forestry, industrialization, urban development and transportation (Zadbagher, 2017; Keçecioğlu Dağlı and Cengiz, 2022). Forest areas are an issue that should be considered and evaluated separately among land cover types, as they have the feature of naturally offering various products and ecosystem services such as carbon storage, regulation of air, water, and climate regime. Changes in forest areas with multiple functions have economic, ecological, and socio-cultural effects on local, regional and global scales. The effects of the changes in forest areas have been experienced for many years, and hence considering the forest dynamics, it is necessary to spend many years and intense effort for its compensation and mitigation. Therefore, there is a constant need to understand the driving forces that cause the long-term development and progress of changes in forest areas (Käyhkö et al., 2011; Girma and Hassan, 2014).
Remote sensing (RS) and geographic information systems (GIS) are the two most effective tools that are widely used (Chowdhury et al., 2020) in the detection and monitoring of continuous and dynamic LULC changes (Vivekananda et al., 2021). RS and GIS procedures provide significant advantages in terms of labor, time, and economy compared to traditional methods. Satellite images are used as a base for data generation in general research on LULC change. Many studies use Landsat satellite images to define and report LULC due to their advantages, such as a large data archive, free access, and ease of use. Kolios and Stylios (2013) in Preveza peninsula, Greece, Islam et al. (2016) in coastal areas of Bangladesh, Halmy et al. (2015) in the northwestern coastal desert of Egypt, Zoungrana et al. (2015) in the Black Volta basin of Burkina Faso, Huang et al. (2017) in the Beijing region of China, Aslami and Ghorbani (2018) in the province of Erbil in northwest of Iran, Obodai et al. (2019) in the Ankobra River basin of Ghana, Alam et al. (2020) in the Kashmir Valley of India, Doyle et al. (2021) in Belize, located in the northeast of Central America, Piao et al. (2023) in the Pyongyang Region of North Korea used Landsat satellite images in their studies.
To evaluate and report the LULC changes of an area, it is necessary to superimpose and overlay maps of different time points and create land change matrix tables. However, the direct interpretation of the data given by these tables is insufficient to explain the change processes and their causes (Huang et al., 2012; Kaptan, 2021). Aldwaik and Pontius (2012) developed intensity analysis consisting of three levels; the “Interval Level,” the “Category Level,” and the “Transition Level,” to interpret the processes and causes of changes between existing land categories. Intensity analysis has attracted the attention of many researchers working on LULC changes like John et al. (2014), Zhou et al. (2014), Yang et al. (2017), Huang et al. (2018), Anteneh et al. (2018), Shoyama et al. (2018), Kourosh Niya et al. (2019), Varga et al. (2019), Ekumah et al. (2020), Feng et al. (2020), Nyamekye et al. (2020).
This study explores the changes in forest and non-forest areas in the Anamur district, located along Türkiye’s Mediterranean coast, over two time periods: 2000–2010 and 2010–2020. Using the intensity analysis method, the research examines land use and land cover (LULC) changes across seven main categories: Coniferous, Broad-Leaved, Mixed, Treeless Gap, Settlement, Agriculture, and Water. The main goal is to understand how these categories have changed over time and the extent of transitions between them. The results are presented through visual graphs, offering insights into key processes such as forest loss, land use changes, and pressures on resources. By identifying these patterns and their driving forces, the study aims to contribute to more effective conservation efforts and land management strategies that can help protect forests and ecosystems.
2 Study area
The city of Anamur, within the borders of Mersin Province, located in the Mediterranean Region, at the southern tip of Türkiye, was chosen as the study area. According to the Regional Growth National Strategies, Mersin is defined as a potential metropolitan city that can be an alternative to İstanbul, Ankara, and İzmir in Türkiye (Göksel and Bektaş Balçık, 2019). According to the EU’s regional growth systematic NUTS (The Nomenclature of Territorial Units for Statistics), the study area in the TR62 sub-region was preferred since it has been in a rapidly developing location along the last thirty years due to the industries, urbanization, and tourism. The study area is geographically located between 36° 00′ 56″–36° 30′ 00″ northern latitudes and 32° 32′ 06″–32° 56′ 50″ eastern longitudes (Figure 1). Anamur District within the borders of the metropolitan municipality, is located in the westernmost part of Mersin (Mersin Governorship, 2024a). Since the branches of the Middle Taurus Mountains spreading toward the Mediterranean Sea pass through the territory of the district, the land is rugged and mountainous. At 5–10 km inland from the coast, mountains with heights of 500–1,000–1,500 meters are encountered. Forests, which contribute greatly to the economy of the district, constitute 60% of the district’s surface area. The climate of the district is characteristically Mediterranean, with hot and dry summers and, mild and rainy winters. The total annual precipitation is 1,032 m3 and the average number of rainy days is 75 days (Anamur District Governorship, 2024). It also has a microclimate where subtropical plants and fruits can be grown (Mersin Governorship, 2024a). When the soil groups in the Anamur Stream Basin are analyzed, it is seen that the most prevalent soils under forest cover are non-calcareous brown soils. Under the influence of coniferous forests, brown soils rich in organic matter are common within these regions. Anamur Plain is completely covered with alluvial soils and reddish brown soil types, which are prominent in the areas where vegetation cover is damaged around the plain (Sunkar and Uysal, 2014).
Approximately 63% of the area is forested and 37% is a bare area. While a single tree species is dominant in about 56% of the forest area, coniferous and broad-leaved species form a mixed forest at the 7%. Although Pinus brutia Ten. generally forms pure forests at 100–1000 m intervals from the Mediterranean coast, it is found together with maquis species in damaged areas. Almost all of the areas where the destruction is very high have turned into maquis formations. Species such as Pinus nigra Arn., Abies cilicia Carr., Cedrus libani A. Rich. spread after 1,000 m. After 1,600 m, Cedrus libani A. Rich. and Juniperus excelsa M. Bieb., and Abies cilicia Carr. communities are seen in places. Among the maquis community, which is referred to as the Mediterranean vegetation, Quercus coccifera L., Olea europaea L., Laurus nobilis L., Arbutus andrachne L., Cistus salviifolius L., Nerium oleander L., Cercis siliquastrum L., Ceratonia siliqua L., Styrax officinalis L., Myrtus communis L., and Pistacia terebinthus L. are the major tree and shrub species (Şen, 2019).
According to the population census of 2023, a total of 66,940 people live in the area (TUIK, 2023). While the people living in the highland and mountain villages in the rural areas generally earn their living from forestry practices, those living in flat and near-flat areas earn their living from greenhouse cultivation, primarily through banana and strawberry cultivation.
3 Materials and methods
In the study, Landsat satellite images of the years 2000, 2010, and 2020 were downloaded to produce the land change matrix tables from which the data required for the intensity analysis were taken. It was obtained from the United States Geological Survey [United States Geological Survey (USGS), 2021]. Satellite images were classified using the supervised classification method in ENVI 5.3 by referring to the forest cover type maps of the area. Images of each classified year were superimposed with the ArcGIS 10.8 “Intersect” command to obtain LULC change maps for the years 2000–2010 and 2010–2020. Referring to the stand types information in the attribute tables of these maps, the area values given in m2 for each LULC category were converted to hectares (ha) and area calculations were made and area calculations for each LULC category were carried out in hectares. With the data in the attribute tables, land change matrix tables were produced showing the changes experienced for each category. Intensity analysis was performed and interpreted using the values obtained from the change matrix tables (Figure 2).
3.1 Satellite images and supervised classification
In this study, Landsat-7 ETM+ (Enhanced Thematic Mapper Plus) and Landsat-8 OLI/TIRS (Operational Land Imager/Thermal Infrared Sensor) satellite images downloaded from the USGS web address were referred for the production of land cover and use maps for the years 2000, 2010 and 2020 (Table 1).
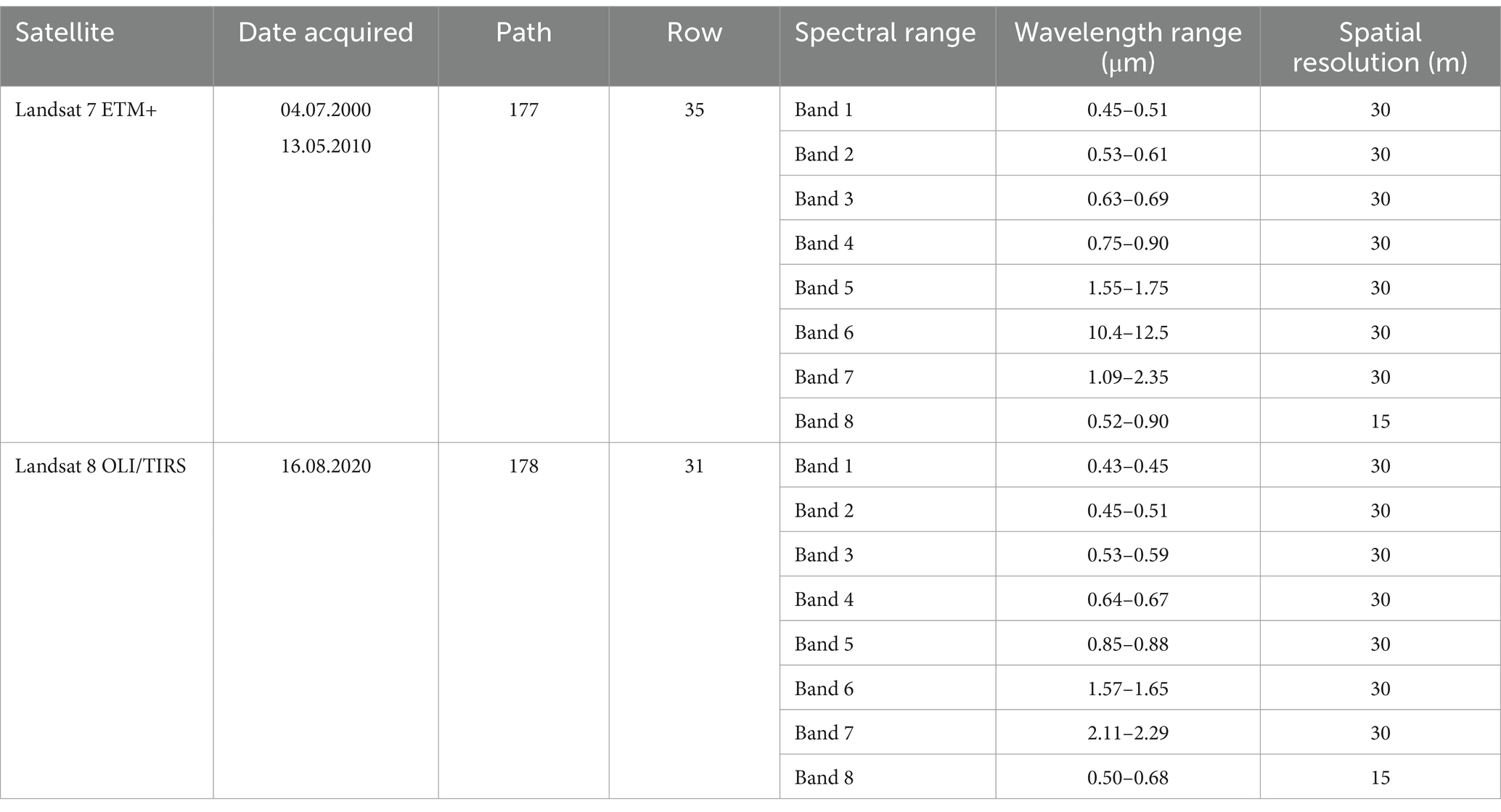
Table 1. Landsat satellite data and band (image) features [United States Geological Survey (USGS), 2021].
The satellite images were classified using supervised classification with Maximum Likelihood Classifier (MLC) in ENVI 5.3, Post-classification accuracy control was performed with the error matrix method based on reference pixels. In creating the error matrix, user accuracy, producer accuracy and overall accuracy are determined. On the other hand, to increase the accuracy of the classification process, satellite images were taken for the same season, and when the low cloudiness (<10%) were preferred.
Traditional methods for accuracy assessment encompass the standard kappa coefficient (Equation 1), overall accuracy (Equation 2), producer’s accuracy (Equation 3), and user’s accuracy (Equation 4). Overall accuracy quantifies the proportion of correctly classified pixels within the analyzed image. User’s accuracy evaluates the proportion of pixels assigned to a specific class but actually belonging to another, whereas producer’s accuracy assesses the proportion of pixels correctly classified as belonging to their actual class. These metrics address commission and omission errors in image classification. The kappa coefficient was derived using the Equation 1 (Petropoulos et al., 2015; Verma et al., 2020):
Kappa coefficient:
Here, r represents the number of rows in the matrix, X denotes the number of observations in the diagonal elements (row i and column i), while xi+ and xii refer to the marginal totals of row r and column i, respectively. N represents the total number of observations.
Overall accuracy:
Producer’s accuracy:
User’s accuracy:
Here, nii indicates the number of pixels that are correctly classified, N is the total pixel count, r denotes the number of rows, and nicol and nirow refer to the totals for the columns and rows, respectively.
For the supervised classification, stand types maps of 2000, 2010, and 2020 obtained from the General Directorate of Forestry (GDF) were used as reference. Land use and cover maps were arranged to include a total of 7 categories: Coniferous, Broad-Leaved, Mixed, Treeless Gap, Settlement, Agriculture, and Water.
To best distinguish and classify land categories from each other, Landsat 7 ETM+ images are red (band 5), green (band 4), and blue (band 3), respectively; in the 2020 Landsat 8 OLI image, red (band 6), green (band 5) and blue (band 4) bands were used. After the composite band raster images were clipped dependent upon the study area, Pan Sharpening and atmospheric correction processes were applied with 1% standard deviation correction to increase the image quality of the images belonging to each year.
3.2 Mapping and detection of LULC changes
To examine LULC, the areas were first divided into two main categories: forest areas and non-forest areas. Forest areas were further classified into Coniferous, Broad-Leaved, Mixed, and Treeless Gap. Non-forest areas were divided into Settlement, Agriculture, and Water. In total, 7 different land categories were created (Table 2). The LULC maps required to examine the changes in time intervals (2000–2010 and 2010–2020), were produced and recorded using the “Intersect” command in ArcGIS 10.8 of two maps of the start time point and the last time point. With the data obtained from these maps, land change matrix tables were created showing the changes between the categories in the Microsoft Excel.
3.3 Intensity analysis
Intensity analysis helps to interpret the calculation results using the data in the land change matrix tables, graphically and mathematically. Intensity analysis gives the results of the size and intensity of the transitions between the categories in a hierarchical approach at three different levels: time interval, category, and transition level (Figure 2). The analysis works with the logic of comparing the intensity of changes between categories with the uniform intensity, which refers to the situation when the intensity of the changes occurred across the categories, is evenly distributed across the spatial and temporal extents (Aldwaik and Pontius, 2012; Anteneh et al., 2018).
Intensity analysis was performed using Microsoft Excel 2007. The workbook, created by Safaa Zakaria Aldwaik in 2009, was updated several times, including modifications in 2011 and 2013. In 2014, Zhilan Deng and Dansheng Liang corrected errors related to the division by zero errors. For a better comprehension and replication of this method for future researchers, it is important to note that the software used for these computations is Microsoft Excel (Pontius, 2024).
The time interval shows in which time intervals the overall annual rate of change is faster or slower by comparing the overall annual rate of change (the size and rate of change (St) to the uniform rate of change (U) for each period) (Tang et al., 2020) For the interval level analysis, the observed annual variation intensity (St) is calculated according to Equation 5, and the uniform annual variation rate (U) is calculated according to Equation 6 (Aldwaik and Pontius, 2012; Aldwaik and Pontius, 2013). If the rate of change (St) is below the uniform rate of change (U), the change is slow, and above it, the change is fast.
where J = a number of categories; i = index for a category at an initial time; j = index for a category at a subsequent time; T = number of time points; t = index for a time point, which ranges from 1 to T − 1; Yt = year at time point t; Ctij = number of pixels that transition from category i at time Yt to category j at time Yt + 1.
Category level examines the variation in the size and intensity of the gross gains (Equation 7) and gross losses (Equation 8) of each category in each time interval (Huang et al., 2012). The analysis compares the annual gain intensity (Gtj) and loss intensity (Lti) of each category with the annual variation intensity (St) calculated according to Equation 1 for the relevant period. If Gtj > S, category j is active in terms of gain, while category j is dormant in terms of gain if Gtj < S. The same interpretation applies to the loss intensity. If Ltj > S, category i is interpreted as an active loser, and if Lti < S, category i is interpreted as a dormant loser.
The transition level examines how the loss and gain intensities of each category change between the relevant categories for each time interval (Aldwaik and Pontius, 2013). Considering the gross gain of category “n,” it is determined according to Equations 9, 10 whether the gain of category n targets the losses of each other category subject to the transition during the time interval. Equation 9 gives the annual transition intensity (Rtin) observed from category “” to the category “n” during the time interval (Yt, Yt + 1) according to the dimension (Yt) at the beginning of the time interval of category i. Equation 10 gives the annual uniform transition intensity (Wtn), which represents the situation when category “n” is assumed to gain evenly across the entire landscape in the time interval (Yt, Yt + 1). If Rtin > Wtn, category i’s losses in time interval t are targeted by category “n” gains, while if Rtin < Wtn, category “n” gains avoid targeting category i’s losses (Kaptan, 2021).
4 Results
4.1 Supervised classification and accuracy assessment
The land use maps were created using 7 basic categories: Coniferous, Broad-Leaved, Mixed, Treeless Gap, Settlement, Agriculture, and Water. These categories were classified based on the stand type maps of the study years under a supervised classification process. The resulting maps are shown in Figure 3, while the error matrix and accuracy index values for the classification process are presented in Table 3. In addition, the areal values of the LULC categories according to the 2020 forest cover type map, which was used as a reference for the classification, and the map of the year 2020 produced after the classification, were compared as in Figure 4. When Table 3 is examined, it is seen that the control pixel numbers of each class and the area (ha) values are almost directly proportional. Among the classes, it is seen that in all three periods, the water class was classified with the least number of reference pixels, with 6,286 pixels in 2000.
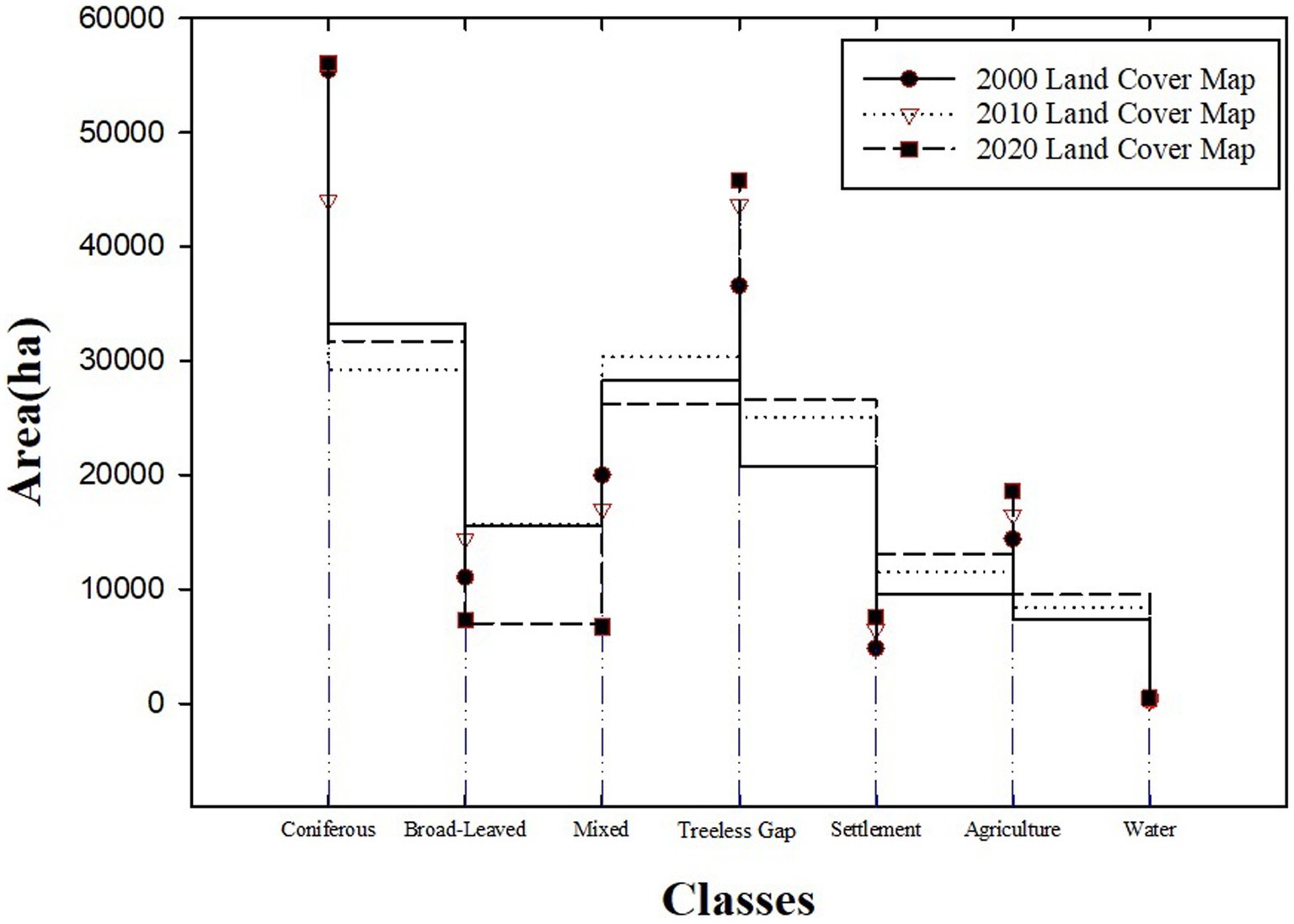
Figure 4. Spatial values of the categories according to the LULC maps of 2000, 2010, and 2020 produced after supervised classification.
Overall accuracy for the years 2000-2010-2020 are 80.558, 91.707 and 92.068%, respectively, while, Kappa coefficients for the years 2000-2010-2020 are 0.73, 0.87 and 0.88, respectively, (Table 3). Producer’s accuracy is over 72% in all classes for all three periods. Statistically, it shows that the accuracy of the supervised classification and the ability to represent the study area is high.
When the classification results of 2010 are examined; it was determined that the overall accuracy rate was 91.707% and the Kappa coefficient was 0.87. Producer’s accuracy is over 85% in all classes, and the “Agriculture” class has the highest producer accuracy at 94.92%. When the classification accuracy values of 2020 were examined, the general accuracy rate was calculated as 92.068% and the Kappa coefficient was calculated as 0.88 (Table 3).
4.2 Changes in LULC
The land transition matrix of the 2000–2010 and 2010–2020 time periods, which was produced by the overlaying the maps produced after supervised classification and forming the input data of the intensity analysis, is given in Table 4. The table shows the changes between categories and the amount of loss, gain, and absolute net change for each period. According to the table, the categories with the widest distribution area in 2000, 2010, and 2020 are Coniferous and Treeless Gap in the forest areas, while the narrowest distribution area is the Water category in the non-forest areas.
The 40% (19,130 ha) decrease during the 20 years from the start time point to the last time point of the study, is associated with the Coniferous (31%) and Mixed (66%) areas. In both time intervals, there was an increase in the Broad-Leaved and Treeless Gap categories, while a net decrease in the Settlement and Agriculture categories in the first time interval and a net increase in the second time interval. Water, on the other hand, experienced a net decrease in both time intervals (Figure 5).
4.3 Intensity analysis
4.3.1 Interval level
The rate and intensity of land change in the study area in the 2000–2010 and 2010–2020 time intervals, the total amount of land use change in each period, annual change intensity, and the intensity of change in all periods are shown in Figure 6.
Each of the bars on the right of the central axis of the graph indicates the intensity of the changes observed for the relevant time interval, and those on the left indicate the percentage of change level. The fact that the bar for the 2010–2020 period on the right of the graph (annual change rate 4.37%) ends before the uniform intensity line (4.70%) indicates that the land use change is slow, and the bar for the 2000–2010 period on the right of the graph extends beyond the uniform intensity line (5.03%), indicates that land use change was rapid for this period. If annual changes in categories were constant over the entire temporal extent, both bars extending to the right would be above the uniform line.
4.3.2 Category level
The category level of the intensity analysis gives the area changes and change intensities of the annual gross gains and gross losses experienced in each category for each period. The results of the category level experienced in the study area at time intervals are shown in Figure 7. The left side of the graph only shows the annual spatial variation of observed gains and losses, while the right side contains detailed information on the intensity of change of gains and losses for each category.
The gain and loss intensity of each category is compared to the uniform line, which represents the situation when the overall intensity of change is evenly distributed over the entire study area. According to this; if the bar for a category extends to the right of the uniform line, it means that the intensity of gain or loss for that category is active (more intense than the overall study area), if it ends before the uniform line, it is dormant (less intense than the overall study area).
According to the results of the analysis, Coniferous in the forest class was the category that experienced the most change in both periods. While it is dormant in terms of gain in the first time interval, the loss of the Coniferous category, which is active in terms of loss and gain in the second time interval, is more intense than the gain. Broad-Leaved and Mixed are active in both time intervals in terms of losses and gains. While the Broad-Leaved category is experienced a heavy gainer in both time intervals, the Mixed category was a heavy loser. The Treeless Gap in the forest is dormant in both time intervals.
The loss intensities of the categories in the non-forest area class are greater than the gain intensities in both time intervals. The settlement, which is active in the first time interval in terms of loss and gain, is completely dormant in the second time interval (Li et al., 2016). Agriculture, which is active in terms of loss and gain, is only active in terms of gain intensity in the second time interval. Although Water is active in terms of gain in both time periods, the loss intensity is higher than the gain.
4.3.3 Transition level intensity analysis
The results of the transition level analysis showing which categories’ gains target the losses of each category are given in Figure 8 as graphics. The left side of each graph shows the size of the annual transitions, while the right side shows the intensity of the annual transitions.
It was observed that the gain of Coniferous, Broad-Leaved, Mixed, Agriculture, Settlement, and Water categories targeted losses in the 2000–2010 period, while Treeless Gap avoided targeting losses. In the 2010–2020 time period, it intensively targeted the losses of only the Broad-Leaved and Mixed categories, while avoiding targeting the area losses of all categories in the non-forest class. While the gain of the Broad-Leaved category targeted Coniferous, Mixed, and Water category losses in the first time interval, it also targeted Agriculture losses along with these categories as gains in the second time interval. The Mixed category intensively targeted Coniferous losses in the first time period and Broad-Leaved losses in the second time period as gains. Treeless Gap targeted Agriculture and Settlement losses in the first time period, and only Coniferous losses in the second time period.
When the gain of the Agriculture, Settlement and Water category in the non-forest area class are examined; while Agriculture category targeted Coniferous, Settlement and Water losses in the first time interval as gain, Broad-Leaved, Settlement and Water categories targeted losses in the second time interval. Settlement continued to target Broad-Leaved, Agriculture and Water losses in the first time interval and only Agriculture and Water losses in the second time interval. Water targeted Coniferous, Broad-Leaved, Mixed and Agriculture losses in the first time interval, and Agriculture and Settlement losses in the second time interval as gains.
5 Discussion
Understanding the changes and transitions in LULC, plays an important role in achieving Türkiye’s Strategic Spatial Planning goals and developing strategies, targets, actions and policies compatible with the United Nations’ Sustainable Development Goals (UN SDGs) (e.g., 6.6, 13.2, 13b, 15.2, 15.3, 15.5, 15.9, 15b) on land degradation neutrality around the scope of sustainable development. Moreover, evaluating and monitoring LULC dynamics in the Mediterranean Region is essential not only for sustainable development but also for enhancing climate change resilience and conserving biodiversity, which are key to ensuring ecological stability and supporting long-term human well-being. Therefore, it is important to evaluate and monitor the dynamics of LULC changes that have occurred in the Mediterranean Region in recent years.
The intensity analysis method offers a more efficient and effective approach than other methods in analyzing changes occurring in LULC categories. The feature of analyzing land changes according to category size and classifying transitions over time brings intensity analysis to the fore (Manzoor et al., 2022; Sakizadeh and Milewski, 2024). In the literature, classical land change matrix is used in LULC change studies and the results of the temporal changes of the categories are given directly. Density analysis, on the other hand, uses the data obtained from the classical change matrix for each time interval as input. Because the analysis subjects these data to a single calculation in a single module and gives three separate results at interval, transition and category level. In this way, not only the spatial changes but also the categories and intensity of the changes are revealed. In this context, the intensity analysis method was used in the study to examine and evaluate the changes in forest areas and land cover in the Anamur region of Türkiye. The study covers a 20-year period in two-time intervals, 2000–2010 and 2010–2020. The changes in LULC categories were examined among the categories grouped under two separate classes as forest and non-forest. Analysis was carried out for a total of seven (7) basic categories: Coniferous, Broad-Leaved, Mixed and Treeless Gap for the forest area class; Settlement, Agriculture and Water for the non-forest area class.
Changes in LULC categories occur due to the combined effect of natural processes and anthropogenic effects. While changes due to natural conditions occur over many years, changes due to human factors occur in a much shorter period of time (Li et al., 2016). When the results obtained from the study are evaluated in this context, it shows that the land cover in Anamur changed under the influence of human activities rather than natural factors, depending on the speed and intensity of land change in the first 10-year period. As in other mountainous parts of Turkey, local people living in the mountainous regions of Anamur have migrated toward the city center for educational and economic reasons. It is thought that this migration movement is the most influential human activity in Anamur and that the use of the area has been most affected and changed for this reason. In the second 10-year period, it is seen that the rate and intensity of land change is slow. This slowdown in the second period can be attributed to the implementation of specific policies and interventions aimed at regulating land use and preserving natural resources. For instance, during the 2010–2020 period, agricultural incentives and forest protection policies played a significant role in reducing the intensity of land cover changes. Policies such as subsidies for sustainable farming practices, restrictions on the conversion of forested lands into settlements, and afforestation programs likely contributed to this trend. Additionally, stricter regulations on urban expansion and zoning policies helped to control the growth of settlement areas, thereby limiting the conversion of arable land and forest areas into construction sites. Similarly, the study by Gong et al. (2020), it was stated that human activities had triggered LULC change, as most of the arable land in the Beijing-Tianjin-Hebei region had been gradually converted into constructed land due to urban expansion. Likewise, in the study by Xie et al. (2023), it was stated that the main driving force of the LULC change seen in Wuhan, China was human activities. However, the rapid changes and intensities experienced in the first period slowed down in the second period, strengthening the idea that natural factors were more effective than human factors in the second period. Policies and regulations regarding Agricultural and Forest Areas can lead to significant changes in land use. In the 2010–2020 period, efforts such as encouraging Agriculture and developing policies for the protection of forest areas slowed down the growth of Agricultural and Settlement areas. The decrease seen in the Settlement and Water categories within forest areas and non-forest areas supports this situation. Supporting the result obtained from the study, Manzoor et al. (2022) also showed that the rate and intensity of land change in Ghana was fast in the first period and slow in the second period, and that cultivated lands continued to expand toward shrub/vegetation for both time periods, which shows that the dynamics of arable land change were consistent between the two time periods. In the study by Rachman et al. (2024) in Jakarta, Indonesia, where they examined the 30-year changes in land use between 1990 and 2020, it is found that the intensity analysis at the transition level provided an active gain in the settled category in all intervals except 1995–2000. Likewise, in this study conducted for Anamur, losses in forest areas continued in both periods and expanded toward Agriculture. Although the intensity of the loss of forest areas was lower at the beginning, it was experienced more in the second period and continued to be the weakest category against the expansion of Agricultural areas and already continues. Forest and non-forest areas are experiencing change due to social and economic development, which is closely related to the land use policies. The expansion of Agricultural and Settlement areas in the second 10 years (2010–2020) was made possible by the increase in population and economic activities in this period and the conversion of forest areas, especially Coniferous areas, into agricultural lands to a large extent. Unfortunately, the loss of forest areas makes it increasingly possible to experience ecologically important consequences such as habitat fragmentation, biodiversity loss and climate change.
According to the land change matrix of the study, forest areas generally decreased by 40% between 2000 and 2020. However, while the Broad-Leaved area and Treeless Gap area increased, which are in the Forest areas class, the Coniferous and Mixed area decreased (Table 4). According to the category level results, which reveal the changes in each category in terms of loss and gain intensity, it was seen that Coniferous was the category that experienced the most intense change for both time periods. Coniferous became active in the second time period and the loss intensity was greater. Broad-Leaved and Mixed are active in both time frames in terms of loss and gain. However, while Broad-Leaved was the intense gaining category for both time periods, Mixed turned out to be the intense losing category (Figure 7). According to the transmission level results of intensity analysis; in both periods, Coniferous, Broad-Leaved and Mixed category gains targeted each other’s losses most intensely. While Coniferous intensively targeted non-forest (Agriculture, Settlement and Water) area losses in the first time period, it was observed that it avoided this in the second time period. On the other hand, Broad-Leaved heavily targeted Water losses in both time periods. It is thought that Broad-Leaved species target water losses due to their higher water and moisture needs compared to Coniferous species. Özçelik’s (2018) study in Turkey supports this idea, finding that Quercus patreae stands have approximately 2.5 times higher water consumption than Pinus nigra Arnold. stands under the same conditions. While Treeless Gap losses were not targeted by any category’s gains in any time period, it was observed that Treeless Gap gains targeted Coniferous losses in the second time period. (Figure 8). According to the official forestry statistics of Türkiye, Coniferous, which was 13,158,774 ha in 2010, decreased by 17% in 2020 to 10,969,246 ha. While Broad-Leaved was 8,378,317 ha in 2010, it decreased by 12% to 7,405,972 ha in 2020. Mixed, which was 4,367,251 ha in 2015, increased by 4% to 4,557,782 ha in 2020 (Forestry Statistics, 2024). While the results of the study for Coniferous were in parallel with the forestry statistics of Türkiye, they were opposite for Broad-Leaved and Mixed. Kaptan et al. (2019), in their study in the Black Sea Region of Türkiye, found that Coniferous and Broad-Leaved areas decreased, while Mixed areas increased. In the study they carried out in Artvin province in the Eastern Black Sea Region of Türkiye, Çavdar and Yolasığmaz (2015), on the other hand, determined that Broad-Leaved and Mixed areas increased, while Coniferous areas decreased between 1985–2006. Contrary to these results, in the study conducted by Manzoor et al. (2022), intensity analysis shows a general increase in the forest category in both periods (1993–2003 and 2003–2015). The Ghanaian government also carried out a tree planting program in Northern Ghana in the 1980s. It is believed that as a result of the Ghanaian government’s policies in the 1980s aimed at reducing pressure on natural forest areas, including a tree planting program in Northern Ghana, the number of trees has increased, mostly on lands not suitable for agriculture. Apart from the afforestation, different reasons have also been cited for the increase in tree cover in certain parts of Ghana despite ongoing deforestation across the country.
The lack of agricultural area in the center where the population is dense, has caused the forest areas in the district to be greatly damaged for economic activities. The increase in altitude from the coast in Anamur has forced the inhabitants to engage in agricultural activities in the city center. This situation has led to the forest fires being started to create agricultural area. However, since fire can cause widespread undesirable damages such as human and economic losses, changes in vegetation cover, runoff, soil degradation, water pollution, etc. (Terêncio et al., 2020; Gültekin and Gültekin, 2024), a systematic policy is needed to identify forest areas suitable for agriculture and minimize the damage (Bayar, 2003). The frequency and severity of disasters such as forest fires can cause losses in forest areas. Zdruli (2014), in his study conducted for 22 countries surrounding the Mediterranean, including Türkiye, emphasized that the main reasons for the decrease in forest areas are forest fires, fuelwood supply, overgrazing, conversion to cultivated land or urbanization. It is known that fires, especially in the Mediterranean basin, have shaped the vegetation of the region since the middle Pliocene period, when dry climate occurred in summers (Rundel et al., 2016; Bahar, 2018). For this reason, it is thought that the impact of forest fires was the main reason for the decrease in fire-sensitive Coniferous areas in the study area. According to the calculations made by TGDF (Turkish General Directorate of Forestry), taking into account the annual number of forest fires that broke out in the last 20 years, the amount of burned area, the total area of administrative borders, and the forest area of the forest fires in the last 20 years, the region, including the study area, is in the class of the most dangerous forest zones (Duran, 2014). According to the Türkiye forestry statistics in 2022, Mersin ranks 7th out of 81 provinces with 99 forest fires (Forestry Statistics, 2024). As suggested by Çelik et al. (2023), the Anamur region, which is under the threat of global warming and drought, is highly vulnerable to forest fires. Therefore, comprehensive measures must be taken to protect Anamur from potential forest fires and to minimize the damage caused by these fires. Global climate change can increase the frequency and intensity of forest fires, leading to a reduction in forest areas. It is especially crucial to prevent deforestation in the northern parts (Treeless Gap) of Anamur and to manage the existing forest areas effectively and sustainably. In this context, it is essential to manage forests in a holistic manner that is compatible with the environment and climate to achieve sustainability goals.
According to the results of the 20-year change obtained for the non-forest area class; Agriculture increased by 65%, while the Settlement and Water categories decreased by 32 and 93%, respectively. According to the land change matrix data, Agriculture achieved the most area gain from the Coniferous and Treeless Gap categories (Table 4). Especially with the increase in agriculture, the need for irrigation water required to grow crops has naturally increased. In addition, sudden and heavy rainfall due to irregularities in the precipitation regime, which is one of the consequences of global climate change, caused surface runoff and drought and could not adequately feed the ground water. Therefore, the irrigation water needed by agricultural crop growers has been supplied in different ways, increasing irregular water use. The results obtained from the study support this situation. According to the category-level results, which reveal the changes in the loss and gain intensities of each category, Settlement was active in terms of both losses and gains during the first time interval but completely dormant during the second time interval. Agriculture was both an active loser and gainer in the first time interval, while it was only active in terms of gain intensity during the second time interval. Water was an active gainer in both time intervals, but its loss intensity was higher than its gain intensity (Figure 7). According to the intensity analysis results at the transition level, Agriculture consistently targeted the area losses of Settlement and Water in both time intervals. Settlement gains most intensely targeted the Agriculture and Water categories in both time intervals. Water consistently targeted the area losses of Agriculture in both time intervals (Figure 8).
The intensity of forest areas has limited agricultural land and caused the population to concentrate in areas suitable for farming due to the economic activities associated with these areas. However, the increasing population has created a need for new agricultural areas, leading to the destruction of forests and their conversion into agricultural land. In their study of the Seyhan Basin in Türkiye’s Eastern Mediterranean Region, Zadbagher et al. (2018) found that agricultural areas continuously increased from 1995 to 2016. Göksel and Bektaş Balçık (2019) also identified an increase in Agriculture areas between 2006 and 2014 in their study on land use and change in Mersin province. Additionally, their study noted that the most significant change in the region was from bare lands to artificial surfaces. Urgilez-Clavijo et al. (2024) stated that the reduction in forest densities was due to their conversion into agricultural areas. However, they noted that this should not be evaluated simply as a reduction in forest areas, as the expansion of cocoa production through traditional agroforestry systems represents a step toward sustainable transition. Parallel to the finding that Settlement gains most intensely targeted the Agriculture and Water categories, Özcan et al. (2018) found a tendency for agricultural lands to be converted into Settlement areas between 1997 and 2012 in their study conducted in Antalya, located in southern Türkiye. It was determined that each category within the forest and non-forest classes experienced the most significant losses and gains among other categories within their respective classes.
The agricultural sector not only meets the food needs of the population but also provides employment opportunities for a large segment of the population and supplies essential inputs to the industrial sector, thereby contributing significantly to the national economy. In this context, the district of Anamur plays an important role in the country’s economy. Anamur specializes in producing high-quality agricultural products, including subtropical fruits (banana, orange, lemon, pomegranate, avocado, kiwi), greenhouse crops (tomato, pepper, eggplant, cucumber, strawberry), and crops grown in sandy soils along the coast (pistachio, broad beans, sesame). Its geographical location, soil structure, and microclimatic conditions enable Anamur to cultivate these special agricultural products, providing an economic advantage to the region. Furthermore, local agricultural products and the food and beverages derived from them enhance Anamur’s tourism potential (Kart Gölgeli, 2016). The agricultural sector in Anamur is a key contributor to the economy, both in Mersin and throughout Türkiye.
Additionally, the province of Mersin has received significant grants under IPARD, providing support for rural development and the agri-food sectors. IPARD, part of the Instrument for Pre-Accession Assistance (IPA), offers special support to rural areas and agri-food sectors in countries in the process of joining the European Union (EU) (European Comission, 2024). Türkiye prepared the IPARD Program, which was approved by the European Commission in 2008 and by Türkiye’s Higher Planning Council in 2009. The implementation of the program began in 2011 (General Directorate of Agricultural Reform, 2024). The program prioritizes the sustainable adaptation of the agricultural sector, the implementation of agri-environmental measures, and the development of the rural economy (Agriculture and Rural Development Support Institution, 2024). Mersin is one of the provinces with the highest number of applications to IPARD, having received 76.7 million TL in grants for 74 projects in 2020, making it the top recipient of grants in Türkiye (Mersin Governorship, 2024b). This highlights the significant role of the agricultural sector in Anamur and explains the observed increase in agricultural areas in the study.
Another significant sector in Anamur’s economic structure is tourism. Although Anamur’s economy is primarily based on agriculture, livestock, and forestry, its location on the Mediterranean coast and favorable climate also make it an important center for marine tourism. The intensive use of marine tourism resources, oversupply in coastal use, a culture of extensive and intensive consumption-based marine tourism, and a move away from conservation practices etc., increase the negative impacts of marine tourism on environmental values. Particularly during the summer months, the population increase and the consequent rise in demand for resources put pressure on urban and district centers, leading to an increase in accommodations such as hotels and pensions. Even a small rise in the consumption of food, water, and energy in tourist activities can affect the ecological balance and lead to significant, unpredictable outcomes at the destination (Sevinç and Duran, 2018). As noted by Rachman et al. (2024) in their study, economic growth and population increase are directly proportional, meaning that economic growth drives population growth, and vice versa.
Economically, the benefits derived from agriculture and tourism can lead to the conversion of forested areas into non-forest areas. The fact that agricultural lands have predominantly targeted Water losses as gains in both periods indicates the process of converting Water areas into agricultural lands. A similar result was observed in the study conducted by Xie et al. (2020). Therefore, the intensity of agricultural land expansion, especially during the 2010–2020 period, may have caused an increase in forest area losses and agricultural land gains. To prevent this, numerous environmental projects are being developed at international, national, and regional levels. Sustainable tourism policies, programs, and practices must continue to be implemented.
According to TUIK data, the population of Anamur was 49,948 in the year 2000 (TUIK, 2000), and it reached 66,994 in 2020 (TUIK, 2020). As a result of urban development and especially tourism development, Anamur has become a major attraction center for surrounding settlements (Bayar, 2003). Urbanization driven by population growth has expanded the city center outward over the 20-year study period, leading to the conversion of some Agricultural areas into Settlement areas in the center (Figure 3). Additionally, the overall expansion of Agricultural areas in the city has led to changes in the urban structure due to tourism-related developments. The increase in Settlement category areas, especially during the second 10-year period, is thought to be attributed to the increasing demand for land alongside economic development. Developments in the agricultural sector have led to the emergence of both local and tourism-oriented rural constructions around agricultural areas, resulting in a more fragmented landscape pattern in the study area. Therefore, it is believed that Settlement areas show a heterogeneous increasing trend throughout the district. Similar results were obtained in the study conducted by Piao et al. (2023) in Pyongyang. Over the 20-year study period, the main urban area in Pyongyang expanded, leading to the conversion of Agricultural areas into Residential areas. The total population of Pyongyang increased from 2,777,000 in 2000 to 3,084,000 in 2020, while the urbanization rate increased from 59,412 in 2000 to 62,381 in 2020, consistent with North Korea’s growth trend. Similarly, in the study conducted by Berdimbetov et al. (2024), it was found that there was a decrease in Forest and Water categories, while there was an increase in Agriculture and Settlement categories between 1995 and 2015. It was determined that the loss of forests contributed to the gain of Agricultural and Urban areas, while water bodies transformed into Treeless Gap and Shrub areas.
Climate change causes a decrease in water supply and a deterioration in water quality in Türkiye. In the Mediterranean region, a decrease in annual rainfall and an increase in extreme precipitation events are observed due to climate change. Urbanization, on the other hand, has led to an increase in temperatures ranging from 0.3 degrees to 0.9°Cin Mediterranean cities between 2001 and 2017. It is anticipated that this trend will continue and affect soil quality and erosion (Climate News, 2019). The rainfall in the Mediterranean Region in April 2024 was 23.0 mm, compared to the normal of 53.5 mm, and the rainfall in April 2023 was 76.9 mm. There has been a 57% decrease in rainfall compared to the normal and a 70% decrease compared to April 2023 (Meteorological Service, 2024). The past-to-present low rainfall and water losses caused by climate change have become a predominant issue for the Water category in Anamur, in parallel with the study by Berdimbetov et al. (2024). Although Water is active in terms of gain in both time intervals, the loss intensity is higher than the gain. The SPEI (Standardized Precipitation-Evapotranspiration Index) value of −1.19 indicates moderate drought in the 2000–2010 period and the SPEI values of −1.44 indicating severe drought in the 2010–2020 period are in parallel with the mentioned losses. There has been a 93% decrease in the Water category over the 20-year period. When comparing the changes in water density between 2000–2010 and 2010–2020, it is observed that the loss is higher in the first time interval. The decrease in water resources reduces the productivity of agricultural areas. Therefore, it is believed that the decrease in the density of agricultural areas during the 2010–2020 period is related to the decrease in water resources and climate change. It can be said that the intensive pressure created by climate change and urbanization, along with the changes in density between areas, also play a significant role.
The areas within the Treeless Gap category in the study area consist almost entirely of highly rugged and rocky areas in mountainous terrain. Therefore, it is expected that Treeless Gap areas, which do not have suitable conditions for agriculture or forestry, will not experience significant losses. Areas targeted for gain by Treeless Gap are likely locations where trees with root development and living opportunities among karstic rocks have thrived. Due to the geological structure consisting of karstic rocks, the disappearance of trees thriving on rocky and stony terrain would have severe consequences and restoring the area to its former state would be quite challenging. The transition from areas with vegetation to Treeless Gap areas is observed to be more pronounced in the second period. If this situation is not controlled, it may increase surface runoff in the future, deteriorate water quality, and have a profound impact on the hydrological cycle (Sakizadeh and Milewski, 2024).
As emphasized by Rachman et al. (2024), development affects environmental quality, and therefore, it is important to control all impacts, whether positive or negative. Deterioration in urban environmental quality is associated with various environmental problems. To create a sustainable and positive environment, governments need to collaborate with communities that directly influence and target progress in the development process. In this context, Gong et al. (2020) also propose recommendations for rural sustainable development and preservation of arable lands in the Beijing-Tianjin-Hebei Region, including providing scientific support institutions, land consolidation projects, and suggestions for the development of sustainable agriculture and industrial improvements.
It is believed that the targeted gain categories in urban development are associated with the surrounding urban growth of the study area. This situation will impose significant pressure on important ecosystem services, including landscape ecology, biodiversity, and natural resources. If not intervened in the near future, closely linked to the changing socio-economic conditions with population growth, the landscape pattern will continue to change, leading to excessive use of natural resources and expansion of built-up areas. Given the threat posed by water scarcity, it is important to develop appropriate objectives and policies within the scope of strategic spatial planning.
Establishing a balance between conservation and utilization is essential to reduce the destruction of agricultural, water, and forest areas. There is a need to strike a balance between forest conservation and urbanization. Changes in policies and regulations related to agriculture, forests, and settlements will affect land use changes. Therefore, it is important for all stakeholders involved in LULC to consider and implement all plans and decisions made in this regard (Hersperger et al., 2018).
6 Conclusion
In this study, LULC changes in forest and non-forest areas were examined by intensity analysis, changes were evaluated at category, time interval and transition level. The method was found effective in detailed analysis. The results are based on satellite image quality and classification accuracy.
It is anticipated that forest fires are the most important factor in the rapid decrease and change in Coniferous areas. The determination that the Treeless Gap category targeted Coniferous losses, especially in the recent time interval, is one of the significant findings of intensity the study. This is because the Treeless Gap category, which refers to state forest areas without trees, includes almost all of the karstic mountainous areas in the region’s highlands. Therefore, forest planning and activities in these areas should prioritize actions for their conservation and improvement. For the study area, which is classified as one of the most dangerous areas in terms of fire sensitivity due to its climate, vegetation, and geographical location, determining policies and implementing them rapidly and effectively to combat forest fires is crucial. The intensive targeting of Water losses as gains by the Agriculture category is another issue that needs attention in terms of water scarcity. Due to global climate change, water levels in many dams in Türkiye have significantly decreased, leading to agricultural production in areas where water has receded. Considering that this situation necessitates the use of water at a high rate in the agricultural areas and rapidly increasing settlement areas in the research area, it is considered as an important indication that a severe drought will be experienced in the region. If this process is not managed properly by applying appropriate landscape planning, forestry, urbanization, and agricultural policies and strategies, it also reveals the fact that very serious migrations to other parts of the country will begin. This migration wave, which is thought to affect the people living in all living conditions, regardless of urban and rural areas, may cause significant bottlenecks in terms of employment and demographic structure in other geographical regions. For this reason, due to the global environmental disasters that have increased rapidly in recent years and whose effects are felt all over the world, there is a need more than ever to monitor, evaluate and report LULC changes, as in this research study.
As global environmental disasters rapidly increase and their effects are experienced worldwide, there is a greater need than ever to monitor, evaluate, and report LULC changes. Particularly in sectors that meet basic human needs such as forestry, landscape planning and design, agriculture, and the food industry, effective policies need to be determined, and proper plans need to be made, making this issue highly important. Changes in forest areas have serious economic and ecological implications, primarily related to global climate change. Therefore, increasing the number and quality of such studies at the local and regional levels is crucial for the development and improvement of sustainable development, sustainable forest management, and sustainable landscape planning efforts and policies.
Data availability statement
The original contributions presented in the study are included in the article/supplementary material, further inquiries can be directed to the corresponding author.
Author contributions
HA: Formal analysis, Methodology, Writing – review & editing, Data curation, Software, Supervision. SK: Formal analysis, Methodology, Writing – review & editing, Writing – original draft. PK: Formal analysis, Visualization, Writing – review & editing. DA: Data curation, Methodology, Resources, Writing – review & editing.
Funding
The author(s) declare that no financial support was received for the research, authorship, and/or publication of this article.
Acknowledgments
We would like to thank the General Directorate of Forestry, Department of Forestry Administration and Planning their staff for supplying and delivering the forest cover-type maps and forest management plans.
Conflict of interest
The authors declare that the research was conducted in the absence of any commercial or financial relationships that could be construed as a potential conflict of interest.
Publisher’s note
All claims expressed in this article are solely those of the authors and do not necessarily represent those of their affiliated organizations, or those of the publisher, the editors and the reviewers. Any product that may be evaluated in this article, or claim that may be made by its manufacturer, is not guaranteed or endorsed by the publisher.
References
Agarwal, C., Green, G. M., Grove, J. M., Evans, T. P., and Schweik, C. M. (2002). A review and assessment of land-use change models: Dynamics of space, time, and human choice. Gen. Tech. Rep. NE-297. Newton Square, PA: U.S. Department of Agriculture, Forest Service, Northeastern Research Station.
Agriculture and Rural Development Support Institution (2024). Rural development program twelfth application call announcement. Available online at: http://www.anamurtso.org.tr/tr/!!2300/bilgi-bankasi-tarim-ve-kirsal-kalkinmayi-destekleme-kurumu (Accessed April 23, 2024)
Aksoy, H., and Kaptan, S. (2020). Simulation of future forest and land use/cover changes (2019–2039) using the cellular automata-Markov model. Geocarto Int. 37, 1183–1202. doi: 10.1080/10106049.2020.1778102
Aksoy, H., and Kaptan, S. (2021). Monitoring of land use/land cover changes using GIS and CA-Markov modeling techniques: a study in northern Turkey. Environ. Monit. Assess. 193, 507–521. doi: 10.1007/s10661-021-09281-x
Aksoy, H., Kaptan, S., Varol, T., Cetin, M., and Ozel, H. B. (2022). Exploring land use/land cover change by using intensity analysis method in Yenice. Int. J. Environ. Sci. Technol. 19, 10257–10274. doi: 10.1007/s13762-021-03847-5
Alam, A., Bhat, M. S., and Maheen, M. (2020). Using Landsat satellite data for assessing the land use and land cover change in Kashmir valley. GeoJournal 85, 1529–1543. doi: 10.1007/s10708-019-10037-x
Aldwaik, S. Z., and Pontius, R. G. Jr. (2012). Intensity analysis to unify measurements of size and stationarity of land changes by interval, category, and transition. Landsc. Urban Plan. 106, 103–114. doi: 10.1016/j.landurbplan.2012.02.010
Aldwaik, S. Z., and Pontius, R. G. Jr. (2013). Map errors that could account for deviations from a uniform intensity of land change. Int. J. Geogr. Inf. Sci. 27, 1717–1739. doi: 10.1080/13658816.2013.787618
Ameen, M. H., Jumaah, H. J., and Khursheed, N. K. (2025). An analysis of hydrologic dynamics in Hamrin Lake, Iraq using remote sensing and GIS techniques. DYSONA Appl. Sci. 6, 96–103. doi: 10.30493/DAS.2024.478002
Anamur District Governorship. (2024). Anamurun Coğrafyasi. T.C. Anamur Kaymakamlığı. Available online at: http://www.anamur.gov.tr/anamurun-cografyasi (Accessed November 20, 2024)
Anteneh, Y., Stellmacher, T., Zeleke, G., Mekuria, W., and Gebremariam, E. (2018). Dynamics of land change: insights from a three-level intensity analysis of the Legedadie-dire catchments, Ethiopia. Environ. Monitor. Assess. 190:309. doi: 10.1007/s10661-018-6688-1
Aslami, F., and Ghorbani, A. (2018). Object-based land-use/land-cover change detection using Landsat imagery: a case study of Ardabil, Namin, and Nir counties in Northwest Iran. Environ. Monit. Assess. 190:376. doi: 10.1007/s10661-018-6751-y
Ayek, A. A., and Zerouali, B. (2025). Monitoring temporal changes of the Qattinah Lake surface area using Landsat data and Google earth engine. DYSONA Appl. Sci. 6, 126–133. doi: 10.30493/DAS.2024.476854
Bahar, A. (2018). Modeling the effect of fire frequency and vegetation cover on Mediterranean vegetation dynamics [doctoral dissertation, Hacettepe University]. Hacettepe University Institute of Science and Technology, Department of Biology. Available online at: http://www.openaccess.hacettepe.edu.tr:8080/xmlui/bitstream/handle/11655/4892/Anil_Bahar_TEZ.pdf?sequence=1andisAllowed=y (Accessed November 28, 2021)
Bailey, K. M., McCleery, R. A., Binford, M. W., and Zweig, C. (2016). Land-cover change within and around protected areas in a biodiversity hotspot. J. Land Use Sci. 11, 154–176. doi: 10.1080/1747423X.2015.1086905
Bayar, R. (2003). Landnutzung-der bevölkerung in Anamur. Turkish J. Geograph. Sci. 1, 97–116. doi: 10.1501/Cogbil_0000000023
Berdimbetov, T., Shelton, S., Pushpawela, B., Rathnayake, U., Koshim, A. G., Yegizbayeva, A., et al. (2024). Use of intensity analysis and transfer matrix to characterize land conversion in the Aral Sea basin under changing climate. Modeling Earth Syst. Environ. 10, 4717–4729. doi: 10.1007/s40808-024-02019-x
Bozdogan Sert, E., Kaya, E., Adiguzel, F., Cetin, M., Gungor, S., Zeren Cetin, I., et al. (2021). Effect of the surface temperature of surface materials on thermal comfort: a case study of Iskenderun (Hatay, Turkey). Theor. Appl. Climatol. 144, 103–113. doi: 10.1007/s00704-021-03524-0
Çavdar, B., and Yolasığmaz, H. (2015). Evaluation according to criteria and ındicators of sustainable forest management: Saçinka Forest planning unit. J. Artvin Coruh Univ. Faculty Forestry 16, 18–29. doi: 10.17474/acuofd.48359
Çelik, M. Ö., Fidan, D., Ulvi, A., and Yakar, M. (2023). Evaluation of forest fires using remote sensing and geographic information systems: a case study of Mersin province, Silifke district. Anatolian J. Forest Res. 9, 116–125. doi: 10.53516/ajfr.1302553
Chowdhury, M., Hasan, M. E., and Abdullah-Al-Mamun, M. M. (2020). Land use/land cover change assessment of Halda watershed using remote sensing and GIS. Egypt. J. Remote Sens. Space Sci. 23, 63–75. doi: 10.1016/j.ejrs.2018.11.003
Climate News (2019). İklim Değişikliği ve Arazi Kullanımının Etkileri. Tematik Bilgi Notu. İklim Haber 2019. Available online at: https://www.birbucukderece.com/assent/pdf/raporarazietkiler.pdf (Accessed May 2, 2024)
de Mello, K., Taniwaki, R. H., de Paula, F. R., Valente, R. A., Randhir, T. O., Macedo, D. R., et al. (2020). Multiscale land use impacts on water quality: assessment, planning, and future perspectives in Brazil. J. Environ. Manag. 270:110879. doi: 10.1016/j.jenvman.2020.110879
Dewan, A. M., and Yamaguchi, Y. (2009). Land use and land cover change in greater Dhaka, Bangladesh: using remote sensing to promote sustainable urbanization. Appl. Geogr. 29, 390–401. doi: 10.1016/j.apgeog.2008.12.005
Doaemo, W., Mohan, M., Adrah, E., Srinivasan, S., and Dalla Corte, A. P. (2020). Exploring forest change spatial patterns in Papua New Guinea: a pilot study in the Bumbu River basin. Land 9:282. doi: 10.3390/land9090282
Doyle, C., Beach, T., and Luzzadder-Beach, S. (2021). Tropical forest and wetland losses and the role of protected areas in northwestern Belize, revealed from Landsat and machine learning. Remote Sens. 13:379. doi: 10.3390/rs13030379
Duran, C. (2014). Spatial analysis of forest fires in Mersin according to their starting points (2001-2013). Forestry Res. J. 1, 38–49. doi: 10.17568/oad.87328
Ekumah, B., Armah, F. A., Afrifa, E. K., Aheto, D. W., Odoi, J. O., and Afitiri, A. R. (2020). Assessing land use and land cover change in coastal urban wetlands of international importance in Ghana using intensity analysis. Wetl. Ecol. Manag. 28, 271–284. doi: 10.1007/s11273-020-09712-5
European Comission (2024). Overview of EU pre-accession assistance for rural development. Available online at: https://agriculture.ec.europa.eu/international/international-cooperation/enlargement/pre-accession-assistance/overview_en#:~:text=The%20instrument%20for%20pre%2Daccession,the%20European%20Union%20(EU) (Accessed April 23, 2024)
Feng, Y., Lei, Z., Tong, X., Gao, C., Chen, S., Wang, J., et al. (2020). Spatially-explicit modeling and intensity analysis of China's land use change 2000–2050. J. Environ. Manag. 263:110407. doi: 10.1016/j.jenvman.2020.110407
Forestry Statistics (2024). Forestry statistics. Available online at: https://www.ogm.gov.tr/tr/e-kutuphane/resmi-istatistikler (Accessed March 26, 2024)
Garg, V., Nikam, B. R., Thakur, P. K., Aggarwal, S. P., Gupta, P. K., and Srivastav, S. K. (2019). Human-induced land use land cover change and its impact on hydrology. HydroResearch 1, 48–56. doi: 10.1016/j.hydres.2019.06.001
General Directorate of Agricultural Reform (2024). IPARD programme. Republic of Türkiye Ministry of Agriculture and Forestry general Directorate of Agricultural Reform. Available online at: https://ipard.tarimorman.gov.tr/prog-en (Accessed April 23, 2024)
Girma, H. M., and Hassan, R. M. (2014). Drivers of land-use change in the southern nations’ nationalities and people’s region of Ethiopia. African J. Agric. Resource Econ. 9, 148–164. doi: 10.22004/ag.econ.176515
Gogoi, P. P., Vinoj, V., Swain, D., Roberts, G., Dash, J., and Tripathy, S. (2019). Land use and land cover change effect on surface temperature over eastern India. Sci. Rep. 9, 8859–8810. doi: 10.1038/s41598-019-45213-z
Göksel, C., and Bektaş Balçık, F. (2019). Land use and land cover changes using spot 5 pansharpen images; a case study in Akdeniz District, Mersin-Turkey. Turkish J. Eng. 3, 32–38. doi: 10.31127/tuje.444685
Gong, Y., Li, J., and Li, Y. (2020). Spatiotemporal characteristics and driving mechanisms of arable land in the Beijing-Tianjin-Hebei region during 1990-2015. Socio Econ. Plan. Sci. 70:100720. doi: 10.1016/j.seps.2019.06.005
Gültekin, Y. S., and Gültekin, P. (2024). “Forest fire risk management at the country scale: the case of Turkey” in Fire Hazards: Socio-economic and Regional Issues. eds. J. Rodrigo-Comino and L. Salvati (Cham: Springer International Publishing), 43–52.
Halmy, M. W. A., Gessler, P. E., Hicke, J. A., and Salem, B. B. (2015). Land use/land cover change detection and prediction in the north-western coastal desert of Egypt using Markov-CA. Appl. Geogr. 63, 101–112. doi: 10.1016/j.apgeog.2015.06.015
Hassan, Z., Shabbir, R., Ahmad, S. S., Malik, A. H., Aziz, N., Butt, A., et al. (2016). Dynamics of land use and land cover change (LULCC) using geospatial techniques: a case study of Islamabad Pakistan. Springerplus 5, 812–811. doi: 10.1186/s40064-016-2414-z
Hersperger, A. M., Oliveira, E., Pagliarin, S., Palka, G., Verburg, P., Bolliger, J., et al. (2018). Urban land-use change: the role of strategic spatial planning. Glob. Environ. Chang. 51, 32–42. doi: 10.1016/j.gloenvcha.2018.05.001
Huang, H., Chen, Y., Clinton, N., Wang, J., Wang, X., Liu, C., et al. (2017). Mapping major land cover dynamics in Beijing using all Landsat images in Google earth engine. Remote Sens. Environ. 202, 166–176. doi: 10.1016/j.rse.2017.02.021
Huang, B., Huang, J., Pontius, R. G. Jr., and Tu, Z. (2018). Comparison of intensity analysis and the land use dynamic degrees to measure land changes outside versus inside the coastal zone of Longhai, China. Ecol. Indic. 89, 336–347. doi: 10.1016/j.ecolind.2017.12.057
Huang, J., Pontius, R. G. Jr., Li, Q., and Zhang, Y. (2012). Use of intensity analysis to link patterns with processes of land change from 1986 to 2007 in a coastal watershed of Southeast China. Appl. Geogr. 34, 371–384. doi: 10.1016/j.apgeog.2012.01.001
Islam, M. R., Miah, M. G., and Inoue, Y. (2016). Analysis of land use and land cover changes in the coastal area of Bangladesh using landsat imagery. Land Degrad. Dev. 27, 899–909. doi: 10.1002/ldr.2339
Jasim, A. T. (2025). Assessing LULC dynamics in Kirkuk City, Iraq using Landsat imagery and maximum likelihood classification. DYSONA-Appl. Sci. 6, 113–119. doi: 10.30493/DAS.2024.478315
John, L. R., Hambati, H., and Armah, F. A. (2014). An intensity analysis of land-use and land-cover change in Karatu District, Tanzania: community perceptions and coping strategies. African Geograph. Rev. 33, 150–173. doi: 10.1080/19376812.2013.838660
Jung, M., Scharlemann, J. P., and Rowhani, P. (2020). Landscape-wide changes in land use and land cover correlate with, but rarely explain local biodiversity change. Landsc. Ecol. 35, 2255–2273. doi: 10.1007/s10980-020-01109-2
Kale, M. P., Chavan, M., Pardeshi, S., Joshi, C., Verma, P. A., Roy, P. S., et al. (2016). Land-use and land-cover change in Western Ghats of India. Environ. Monit. Assess. 188, 387–323. doi: 10.1007/s10661-016-5369-1
Kaptan, S. (2021). Changes in forest areas and land cover and their causes using intensity analysis: the case of Alabarda forest planning unit. Environ. Monit. Assess. 193:387. doi: 10.1007/s10661-021-09089-9
Kaptan, S., Aksoy, H., Durkaya, B., and Aksoy Çavdar, Ş. (2019). Investigation of changes in land cover and use with geographic information systems (Akgöl forest management chief example) [paper presentation]. 8th international vocational schools symposium. Available online at: https://acikerisim.bartin.edu.tr/handle/11772/1947 (Accessed November 27, 2021)
Karimi, H., Jafarnezhad, J., Khaledi, J., and Ahmadi, P. (2018). Monitoring and prediction of land use/land cover changes using CA-Markov model: a case study of Ravansar County in Iran. Arab. J. Geosci. 11, 1–9. doi: 10.1007/s12517-018-3940-5
Kart Gölgeli, Ü. (2016). The importance of local foods in gastronomy tourism: Anamur [Master’s thesis, Mersin University]. Mersin University, Institute of Social Sciences, Department of Tourism Management. Available online at: https://tez.yok.gov.tr/UlusalTezMerkezi/tezDetay.jsp?id=0VHaK0WT1I8UY0VGE6IdKAandno=zVmw0Xw6Id8mh_WqqQUOjA (Accessed May 26, 2024)
Käyhkö, N., Fagerholm, N., Asseid, B. S., and Mzee, A. J. (2011). Dynamic land use and land cover changes and their effect on forest resources in a coastal village of Matemwe, Zanzibar, Tanzania. Land Use Policy 28, 26–37. doi: 10.1016/j.landusepol.2010.04.006
Keçecioğlu Dağlı, P. (2021). Evaluation of the change in urban land use within the context of strategic spatial planning: İstanbul Riva case [doctoral dissertation, Bartın university]. Bartın University, Institute of Postgraduate Education, Department of Landscape Architecture. Available online at: https://tez.yok.gov.tr/UlusalTezMerkezi/tezSorguSonucYeni.jsp (Accessed May 26, 2024)
Keçecioğlu Dağlı, P., and Cengiz, C. (2022). “A theoretical framework for land use change” in Landscape research II. eds. Ö. Demirel and E. Düzgüneş (Lyon: Livre de Lyon Publishing), 253–272.
Kolios, S., and Stylios, C. D. (2013). Identification of land cover/land use changes in the greater area of the Preveza peninsula in Greece using Landsat satellite data. Appl. Geogr. 40, 150–160. doi: 10.1016/j.apgeog.2013.02.005
Kourosh Niya, A., Huang, J., Karimi, H., Keshtkar, H., and Naimi, B. (2019). Use of intensity analysis to characterize land use/cover change in the biggest island of Persian gulf, Qeshm Island, Iran. Sustainability 11:4396. doi: 10.3390/su11164396
Li, C., Gong, P., Wang, J., Yuan, C., Hu, T., Wang, Q., et al. (2016). An all-season sample database for improving land-cover mapping of Africa with two classification schemes. Int. J. Remote Sens. 37, 4623–4647. doi: 10.1080/01431161.2016.1213923
Manzoor, S. A., Griffiths, G. H., Robinson, E., Shoyama, K., and Lukac, M. (2022). Linking pattern to process: intensity analysis of land-change dynamics in Ghana as correlated to past socioeconomic and policy contexts. Land 11:1070. doi: 10.3390/land11071070
Marshall, L., Biesmeijer, J. C., Rasmont, P., Vereecken, N. J., Dvorak, L., Fitzpatrick, U., et al. (2018). The interplay of climate and land use change affects the distribution of EU bumblebees. Glob. Chang. Biol. 24, 101–116. doi: 10.1111/gcb.13867
Martins, F., Alegria, C., and Gil, A. (2016). Mapping invasive alien Acacia dealbata link using ASTER multispectral imagery: a case study in central-eastern of Portugal. Forest Systems 25:e078. doi: 10.5424/fs/2016253-09248
Mersin Governorship (2024a). Anamur İlçesi. T.C. Mersin Valiliği. Available online at: http://www.mersin.gov.tr/anamur (Accessed November 20, 2024)
Mersin Governorship (2024b). Agriculture and rural development support institution supported 478 projects in our Mersin Province to date, 3.7 billion tl investment was made, 1,850 employment was provided. Available online at: http://www.mersin.gov.tr/tkdk-mersin-ilimizde-bugune-kadar-478-projeye-destek-verdi-37-milyar-tl-yatirim-yapildi-1850-istihdam-saglandi (Accessed April 23, 2024)
Meteorological Service (2024). 2024 areal precipitation report. Rebuplic of Türkiye, Ministry of Environment, urbanization and climate change, meteorological service. Available online at: https://www.mgm.gov.tr/veridegerlendirme/yagis-raporu.aspx?b=a#sfB (Accessed May 21, 2024)
Mialhe, F., Gunnell, Y., Ignacio, J. A. F., Delbart, N., Ogania, J. L., and Henry, S. (2015). Monitoring land-use change by combining participatory land-use maps with standard remote sensing techniques: showcase from a remote forest catchment on Mindanao, Philippines. Int. J. Appl. Earth Obs. Geoinf. 36, 69–82. doi: 10.1016/j.jag.2014.11.007
Nasiri, V., Darvishsefat, A. A., Rafiee, R., Shirvany, A., and Hemat, M. A. (2019). Land use change modeling through an integrated multi-layer perceptron neural network and Markov chain analysis (case study: Arasbaran region, Iran). J. For. Res. 30, 943–957. doi: 10.1007/s11676-018-0659-9
Nyamekye, C., Kwofie, S., Ghansah, B., Agyapong, E., and Boamah, L. A. (2020). Assessing urban growth in Ghana using machine learning and intensity analysis: a case study of the new Juaben municipality. Land Use Policy 99:105057. doi: 10.1016/j.landusepol.2020.105057
Obodai, J., Adjei, K. A., Odai, S. N., and Lumor, M. (2019). Land use/land cover dynamics using landsat data in a gold mining basin-the Ankobra, Ghana. Remote Sensing Appl. 13, 247–256. doi: 10.1016/j.rsase.2018.10.007
Organisation for Economic Co-operation and Development (OECD) (2018). Monitoring land cover change. Available online at: http://www.oecd.org/env/indicatorsmodelling-outlooks/brochure-land-cover-change-v2.pdf
Özcan, O., Musaoğlu, N., and Türkeş, M. (2018). Assessing vulnerability of a forest ecosystem to climate change and variability in the western Mediterranean sub-region of Turkey. J. For. Res. 29, 709–725. doi: 10.1007/s11676-017-0505-5
Özçelik, M. S. (2018). Water consumption measurements on forest trees by trunk heat balance method [doctoral dissertation, İstanbul university]. İstanbul university, Institute of Graduate Studies in science and engineering, Department of Forest Engineering. Available online at: https://tez.yok.gov.tr/UlusalTezMerkezi/tezSorguSonucYeni.jsp (Accessed November 29, 2024)
Patra, S., Sahoo, S., Mishra, P., and Mahapatra, S. C. (2018). Impacts of urbanization on land use/cover changes and its probable implications on local climate and groundwater level. J. Urban Manag. 7, 70–84. doi: 10.1016/j.jum.2018.04.006
Petropoulos, G. P., Kalivas, D. P., Georgopoulou, I. A., and Srivastava, P. K. (2015). Urban vegetation cover extraction from hyperspectral imagery and geographic information system spatial analysis techniques: case of Athens, Greece. J. Appl. Remote Sens. 9:96088. doi: 10.1117/1.JRS.9.096088
Piao, Y., Xiao, Y., Ma, F., Park, S., Lee, D., Mo, Y., et al. (2023). Monitoring land use/land cover and landscape pattern changes at a local scale: a case study of Pyongyang, North Korea. Remote Sensing 15:1592. doi: 10.3390/rs15061592
Pontius, Jr. R. G. (2024). Professor Robert Gilmore Pontius Jr‘s professional website. Available online at: https://wordpress.clarku.edu/rpontius/?_gl=1%2Agt2d8y%2A_gcl_au%2ANTI1Mjg2OTMxLjE3MzIxMDkwODU.%2A_ga%2AMTUxMTc1ODgwMS4xNzE5NDAzNTQz%2A_ga_8T1G50QTBE%2AMTczNDMzNTE5NC4zLjAuMTczNDMzNTE5Ni41OC4wLjc4NDgxMzkyOQ (Accessed December 04, 2019).
Rachman, F., Huang, J., Xue, X., and Marfai, M. A. (2024). Insights from 30 years of land use/land cover transitions in Jakarta, Indonesia, via intensity analysis. Land 13:545. doi: 10.3390/land13040545
Ren, Y., Lü, Y., Comber, A., Fu, B., Harris, P., and Wu, L. (2019). Spatially explicit simulation of land use/land cover changes: current coverage and future prospects. Earth Sci. Rev. 190, 398–415. doi: 10.1016/j.earscirev.2019.01.001
Rundel, P. W., Arroyo, M. T., Cowling, R. M., Keeley, J. E., Lamont, B. B., and Vargas, P. (2016). Mediterranean biomes: evolution of their vegetation, floras, and climate. Annu. Rev. Ecol. Evol. Syst. 47, 383–407. doi: 10.1146/annurev-ecolsys-121415-032330
Sakizadeh, M., and Milewski, A. (2024). Quantifying LULC changes in Urmia Lake Basin using machine learning techniques, intensity analysis and a combined method of cellular automata (CA) and artificial neural networks (ANN) (CA-ANN). Modeling Earth Syst. Environ. 10, 2011–2030. doi: 10.1007/s40808-023-01895-z
Şen, A. N. (2019). Flora of Anamur (Mersin) and its highlands [doctoral dissertation, Konya Selcuk university]. Konya Selcuk University, Institute of Science and Technology, Department of Biology. Available online at: https://tez.yok.gov.tr/UlusalTezMerkezi/tezSorguSonucYeni.jsp (Accessed November 26, 2021)
Sevinç, F., and Duran, E. (2018). Sürdürülebilir deniz turizmi ve tüketim paradoksu: Tüketirken tükenmek. J. Consumer Consumpt. Res. 10, 173–196. (in Turkish) Available at: https://betadergi.com/ttad/yonetim/icerik/makaleler/197-published.pdf
Shoyama, K., Braimoh, A. K., Avtar, R., and Saito, O. (2018). Land transition and intensity analysis of cropland expansion in northern Ghana. Environ. Manag. 62, 892–905. doi: 10.1007/s00267-018-1085-7
Siddika, S., and Sresto, M. A. (2025). Assessing urban resilience of Khulna City in response to environmental and socioeconomic challenges. DYSONA-Appl. Sci. 6, 134–144. doi: 10.30493/DAS.2024.481910
Sinha, S., Sharma, L. K., and Nathawat, M. S. (2015). Improved land-use/land-cover classification of semi-arid deciduous forest landscape using thermal remote sensing. Egypt. J. Remote Sens. Space Sci. 18, 217–233. doi: 10.1016/j.ejrs.2015.09.005
Sunkar, M., and Uysal, A. (2014). Anamur (Dragon) Çayı’nın (Mersin) hidrografik özellikleri ve ekonomik potansiyeli. J. Geogr. 28, 69–93. Available at: https://dergipark.org.tr/tr/pub/iucografya/issue/25073/264643
Tang, J., Li, Y., Cui, S., Xu, L., Ding, S., and Nie, W. (2020). Linking land-use change, landscape patterns, and ecosystem services in a coastal watershed of southeastern China. Global Ecol. Conserv. 23:e01177. doi: 10.1016/j.gecco.2020.e01177
Tasser, E., Leitinger, G., and Tappeiner, U. (2017). Climate change versus land-use change—what affects the mountain landscapes more? Land Use Policy 60, 60–72. doi: 10.1016/j.landusepol.2016.10.019
Terêncio, D. P. S., Cortes, R. M. V., Pacheco, F. A. L., Moura, J. P., and Fernandes, L. F. S. (2020). A method for estimating the risk of dam reservoir silting in fire-prone watersheds: a study in Douro river, Portugal. Water 12:2959. doi: 10.3390/w12112959
TUIK (2000). Turkish statistical institute, population of provinces, districts and lower level municipalities in 2000. Available online at: https://rapory.tuik.gov.tr/28-05-2024-13:35:14-7265930493387020521676134964.html? (Accessed April 23, 2024)
TUIK (2020). Turkish statistical institute, population of provinces, districts and lower level municipalities in 2020. Available online at: https://biruni.tuik.gov.tr/medas/?kn=95andlocale=tr (Accessed April 23, 2024)
TUIK (2023). Turkish statistical institute, City/district center town/village population and annual population growth rate by province. Available online at: https://biruni.tuik.gov.tr/medas/?kn=95andlocale=tr (Accessed April 23, 2024)
Turner, B., Meyer, W. B., and Skole, D. L. (1994). Global land-use/land-cover change: towards an integrated study. Ambio 23, 91–95.
Twisa, S., and Buchroithner, M. F. (2019). Land-use and land-cover (LULC) change detection in Wami river basin, Tanzania. Land 8:136. doi: 10.3390/land8090136
United States Geological Survey (USGS) (2021). Landsat Missions, Landsat 8. Available online at: https://www.usgs.gov/ (Accessed June 2, 2021)
Urgilez-Clavijo, A., Rivas-Tabares, D., Gobin, A., and de la Riva, J. (2024). Comprehensive framework for analysing the intensity of land use and land cover change in continental Ecuadorian biosphere reserves. Sustain. For. 16:1566. doi: 10.3390/su16041566
Varga, O. G., Pontius, R. G. Jr., Singh, S. K., and Szabó, S. (2019). Intensity analysis and the figure of merit’s components for assessment of a cellular automata–markov simulation model. Ecol. Indic. 101, 933–942. doi: 10.1016/j.ecolind.2019.01.057
Verburg, P. H., Crossman, N., Ellis, E. C., Heinimann, A., Hostert, P., Mertz, O., et al. (2015). Land system science and sustainable development of the earth system: a global land project perspective. Anthropocene 12, 29–41. doi: 10.1016/j.ancene.2015.09.004
Verburg, P. H., de Nijs, T. C., van Eck, J. R., Visser, H., and de Jong, K. (2004). A method to analyse neighbourhood characteristics of land use patterns. Comput. Environ. Urban. Syst. 28, 667–690. doi: 10.1016/j.compenvurbsys.2003.07.001
Verma, P., Raghubanshi, A., Srivastava, P. K., and Raghubanshi, A. S. (2020). Appraisal of kappa-based metrics and disagreement indices of accuracy assessment for parametric and nonparametric techniques used in LULC classification and change detection. Modeling Earth Syst. Environ. 6, 1045–1059. doi: 10.1007/s40808-020-00740-x
Verstegen, J. A., van der Laan, C., Dekker, S. C., Faaij, A. P., and Santos, M. J. (2019). Recent and projected impacts of land use and land cover changes on carbon stocks and biodiversity in East Kalimantan, Indonesia. Ecol. Indic. 103, 563–575. doi: 10.1016/j.ecolind.2019.04.053
Vivekananda, G. N., Swathi, R., and Avln, S. (2021). Multi-temporal image analysis for LULC classification and change detection. European J. Remote Sens. 54, 189–199. doi: 10.1080/22797254.2020.1771215
Watson, R. T., Noble, I. R., Bolin, B., Ravindranath, N. H., Verardo, D. J., and Dokken, D. J. (2000). Land use, land-use change and forestry: A special report of the intergovernmental panel on climate change. Cambridge, UK: Cambridge University Press.
Weng, Q. (2001). A remote sensing? GIS evaluation of urban expansion and its impact on surface temperature in the Zhujiang Delta, China. Int. J. Remote Sens. 22, 1999–2014. doi: 10.1080/713860788
Wu, J., Miao, C., Zheng, H., Duan, Q., Lei, X., and Li, H. (2018). Meteorological and hydrological drought on the loess plateau, China: evolutionary characteristics, impact, and propagation. J. Geophys. Res. Atmos. 123, 11–569. doi: 10.1029/2018JD029145
Xie, Q., Han, Y., Zhang, L., and Han, Z. (2023). Dynamic evolution of land use/land cover and its socioeconomic driving forces in Wuhan, China. Int. J. Environ. Res. Public Health 20:3316. doi: 10.3390/ijerph20043316
Xie, Z., Pontius, R. G. Jr., Huang, J., and Nitivattananon, V. (2020). Enhanced intensity analysis to quantify categorical change and to identify suspicious land transitions: a case study of Nanchang, China. Remote Sensing 12:3323. doi: 10.3390/rs12203323
Yang, Y., Liu, Y., Xu, D., and Zhang, S. (2017). Use of intensity analysis to measure land use changes from 1932 to 2005 in Zhenlai County, Northeast China. Chinese Geograph. Sci. 27, 441–455. doi: 10.1007/s11769-017-0876-8
Zadbagher, E. (2017). Modelling land use/cover change in Seyhan Basin, Turkey using remote sensing and geographic information system [master‘s thesis, Çukurova University]. Çukurova University, Institute of Natural and Applied Sciences, Department of Remote Sensing and Geographic Information Systems. Available online at: https://tez.yok.gov.tr/UlusalTezMerkezi/tezSorguSonucYeni.jsp (Accessed May 26, 2024)
Zadbagher, E., Becek, K., and Berberoglu, S. (2018). Modeling land use/land cover change using remote sensing and geographic information systems: case study of the Seyhan Basin, Turkey. Environ. Monitoring Assess. 190:494. doi: 10.1007/s10661-018-6877-y
Zdruli, P. (2014). Land resources of the Mediterranean: status, pressures, trends and impacts on future regional development. Land Degrad. Dev. 25, 373–384. doi: 10.1002/ldr.2150
Zheng, H. W., Shen, G. Q., Wang, H., and Hong, J. (2015). Simulating land use change in urban renewal areas: a case study in Hong Kong. Habitat Int. 46, 23–34. doi: 10.1016/j.habitatint.2014.10.008
Zhou, P., Huang, J., Pontius, R. G., and Hong, H. (2014). Land classification and change intensity analysis in a coastal watershed of Southeast China. Sensors 14, 11640–11658. doi: 10.3390/s140711640
Keywords: LULC, forest areas, intensity analysis, remote sensing, GIS
Citation: Aksoy H, Kaptan S, Keçecioğlu Dağli P and Atar D (2024) Unveiling two decades of forest transition in Anamur, Türkiye: a remote sensing and GIS-driven intensity analysis (2000–2020). Front. For. Glob. Change. 7:1498890. doi: 10.3389/ffgc.2024.1498890
Edited by:
Rishikesh Singh, Amity University, Mohali, IndiaReviewed by:
G. N. Tanjina Hasnat, University of Chittagong, BangladeshAmit Kumar Tiwari, Banaras Hindu University, India
Hazem Abdo, Tartous University, Syria
Copyright © 2024 Aksoy, Kaptan, Keçecioğlu Dağli and Atar. This is an open-access article distributed under the terms of the Creative Commons Attribution License (CC BY). The use, distribution or reproduction in other forums is permitted, provided the original author(s) and the copyright owner(s) are credited and that the original publication in this journal is cited, in accordance with accepted academic practice. No use, distribution or reproduction is permitted which does not comply with these terms.
*Correspondence: Sinan Kaptan, c2thcHRhbkBiYXJ0aW4uZWR1LnRy