- 1Faculty of Forestry and Wood Sciences, Czech University of Life Sciences Prague, Prague, Czechia
- 2ICAR-Indian Institute of Rice Research (IIRR), Hyderabad, India
- 3Department of Forest Botany, Dendrology and Geobiocoenology, Mendel University in Brno, Brno, Czechia
- 4Institute of Forest Ecology, Slovak Academy of Sciences, Zvolen, Slovakia
Accurate gene expression analysis in Norway spruce (Picea abies) under diverse stress conditions requires the identification of stable reference genes for normalization. Notably, the literature lacks reports on suitable reference genes in Norway spruce. Here, we aimed to address this gap by identifying suitable reference genes for quantitative real-time PCR in Norway spruce across various stress conditions (drought, heat, pathogen infection) in seedlings, tissues (needle, phloem, root), and developmental stages (seedlings, mature trees). We evaluated the stability of 15 candidate reference genes and assessed their expression stability using five statistical algorithms (ΔCt, geNorm, NormFinder, BestKeeper, and RefFinder). Our results highlight ubiquitin-protein ligase (SP1), conserved oligomeric Golgi complex (COG7), and tubby-like F-box protein (TULP6) as the most stable reference genes, while succinate dehydrogenase (SDH5) and heat shock protein 90 (HSP90) were the least stable under various experimental conditions. COG7 and TULP6 are novel candidate reference genes reported for the first time. The expression stability of the identified reference genes was further validated using dehydrin-like protein 5 (PaDhn5) under drought conditions in Norway spruce. Pairwise variation analysis suggests that two reference genes were sufficient to normalize gene expression across all sample sets. This study provides a comprehensive analysis of reference gene stability under different experimental conditions and a catalog of genes for each condition, facilitating future functional genomic research in Norway spruce and related conifers.
1 Introduction
Norway spruce [Picea abies (L.) Karst.] is one of the most ecologically and economically important coniferous tree species in Europe. Adapted to cool and wet conditions, its distribution extends from central and eastern Norway across Fennoscandia, the Baltic states, Belarus, Russia, and Central and Southeastern Europe (Danielsen et al., 2021). Over the past several decades, Norway spruce has been extensively planted in areas beyond its natural distribution range due to its excellent growth performance and desirable wood properties for commercial forestry (Caudullo et al., 2016; Hlásny et al., 2019). However, planting it outside its niche has led to health and vitality issues, increasing its susceptibility to biotic and abiotic stresses. For instance, Norway spruce forests in the Czech Republic experienced a dramatic increase in mortality in 2019, losing an average of 118 million m3 due to droughts, heatwaves, and bark beetle outbreaks (Ebner, 2020). Traditional management strategies, such as pheromone-based mass trapping, salvage logging, insecticides, and anti-attractants, have been largely ineffective in controlling mass bark beetle attacks (Dobor et al., 2020; Singh et al., 2024a). Consequently, there has been rapid exploration of functional genomics-based tools to develop effective management strategies. Notably, sequencing of the Norway spruce genome, the first among conifers (Nystedt et al., 2013), has significantly advanced gene expression studies aimed at understanding gene functions and molecular regulation under various stresses. However, the appropriate reference genes required for gene expression normalization in Norway spruce have not yet been comprehensively established. Therefore, identifying reliable reference genes is crucial for improving studies on gene expression profiling, providing novel insights into biological processes, and deepening the understanding of regulatory gene networks that contribute to spruce resistance, resilience, and survival (Wise et al., 2007).
The significance of differential gene expression analysis in understanding gene function and molecular regulation in response to various environmental factors is crucial. Real-time quantitative polymerase chain reaction (RT–qPCR) is a widely used technique for analyzing the expression of target genes owing to its high sensitivity, accuracy, specificity, reproducibility, and rapidity (Bustin, 2002; Gachon et al., 2004; Bustin et al., 2005; Takamori et al., 2017). However, the precision of RT–qPCR is highly dependent on using a reference gene as an internal control. Reference genes, also referred to as housekeeping genes, are consistently expressed in cells and are vital for fundamental cellular functions. These genes encode proteins that play crucial roles in essential cellular activities, including cell cycle regulation, DNA replication, and metabolism. An ideal reference gene maintains consistent expression levels across different experimental conditions and tissues and remains unaffected by developmental stages or the organism’s genotype (Han et al., 2012; Lu et al., 2018; Sen et al., 2021).
Several studies have identified and validated reference genes in crops, commercially valuable tree species, and herbs, including Solanum tuberosum L. (Nicot et al., 2005), Arabidopsis thaliana (L.) Heynh. (Czechowski et al., 2005), Oryza sativa L. (Jain et al., 2006), Triticum aestivum L. (Paolacci et al., 2009), Linum usitatissimum L. (Huis et al., 2010), Eucalyptus robusta Sm. (de Oliveira et al., 2012), Populus euphratica Oliv. (Wang et al., 2014), Solanum melongena L. (Mogilicherla et al., 2016), Bromus sterilis L. (Sen et al., 2021), Melissa officinalis L. (Bharati et al., 2023), and Populus tremula L. (Pastierovič et al., 2024). Recently, efforts have been made to identify and validate reference genes in coniferous species such as Chinese fir (Cunninghamia lanceolata (Lamb.) Hook.) and Masson pine (Pinus massoniana Lamb.) (Bao et al., 2016; Mo et al., 2019; Chen et al., 2019). However, these studies were limited to specific tissues and treatment conditions. Previous research on internal control genes in P. abies was limited to embryogenic cell lines (e.g., Vestman et al., 2011; de Vega-Bartol et al., 2013). In maritime pine (Pinus pinaster), genes such as GADPH, 18S rRNA, UBQ, and eIF4AII were found unsuitable as internal controls during embryo development due to the high variability in gene expression during embryogenesis and the involvement of these genes in both basal metabolism and other functions (Gonçalves et al., 2005). More recently, a study identified elongation factor 1-gamma, histone H1, GAPDH, and α-tubulin as stable reference genes for somatic embryogenesis tissues in Liriodendron hybrids, while elongation factor 1-gamma and actin were most reliable for germinative organ tissues (Li et al., 2021). These findings suggest that results from embryogenic cell lines may not accurately represent seedlings or mature trees under varying stress conditions. Therefore, establishing a set of stable reference genes for Norway spruce across different developmental stages and stress conditions is crucial for improving the accuracy and reliability of gene expression analysis, enhancing our understanding of its physiological processes and stress responses.
Here, we analyzed the expression profiles of 15 candidate reference genes in different conifer species based on published research and our in-house transcriptome data (de Vega-Bartol et al., 2013; Bao et al., 2016; Mo et al., 2019; Chen et al., 2019). These genes are known for performing conserved cellular functions in gymnosperms and, therefore, are expected to show stable expression across various conditions, developmental stages, and tissues. We evaluated the expression stability of ribosomal protein L26 (RPL26), serine/threonine-protein phosphatase (PP2A2), ribosomal protein L7Ae (RPL7Ae), ubiquitin-like domain-containing CTD phosphatase (UBCP), F-box protein (SKIP22), heat shock protein (HSP90), vacuolar fusion protein (CCZ1), succinate dehydrogenase (SDH5), 18S rRNA (RID2), putative lysine-specific demethylase (JMJ16), conserved oligomeric Golgi complex (COG7), ribosomal protein S10 (RSP10), ubiquitin-protein ligase (SP1), tubby-like F-box protein (TULP6), and actin-related protein (ARP9) across different tissues (needle, phloem and root), developmental stages (seedlings and mature trees), and under various biotic and abiotic stress conditions (drought, heat stress, and pathogen infection) in Norway spruce seedlings. The present study will enhance the accuracy of future gene expression studies and contribute to a more reliable evaluation of reference gene stability in Norway spruce. These reference genes are also expected to serve as templates for other related conifer species and should be further investigated.
2 Materials and methods
2.1 Plant materials and experimental conditions
2.1.1 Greenhouse experiment
Three-year-old containerized Norway spruce seedlings, approximately 40 cm high, were procured from a local nursery and planted in a moist peat/perlite mixture in May 2022. The seedlings were grown using seed material originating from the Bohemian-Moravian highlands (Czech Republic), characterized by 600–750 mm of annual rainfall and an altitude of 500–600 m above sea level. The seedlings were transferred to a greenhouse with a temperature range of 23 ± 2°C and 16-h/8-h light/dark photoperiod cycles at the Czech University of Life Sciences, Prague. Before any treatment, the plants were irrigated regularly with tap water for acclimation to the greenhouse conditions. A total of 16 plants were used for each treatment. The plants were grouped into sets of four, which were pooled to form one biological replicate. Consequently, each treatment comprised four biological replicates, with each replicate consisting of four plants. Control treatment: This group was irrigated regularly and was not subjected to any abiotic or biotic treatment. Water deficit treatment: One group was subjected to drought stress by gradually interrupting the water supply until the mean plant water potential decreased below −2.1 MPa. A water potential value of −2.1 MPa in Norway spruce is considered low, indicating water stress that may lead to embolism formation and a loss of hydraulic conductivity (Rosner et al., 2019). The degree of drought stress was quantified by measuring the predawn water potential using a Scholander pressure chamber (PMS Instruments, Corvallis, Oregon, United States). Heat stress: To induce heat stress, the seedlings were placed in a climate chamber (FytoScope FS-SI 3400, PSI, Drásov, Czech Republic) at 35°C for 10 days with a constant relative humidity of 80% and regular irrigation. Biotic stress: The seedlings were inoculated with the Ophiostomatoid fungus Ophiostoma flexuosum, and incubated for 1 month in a controlled climate chamber mentioned above. Throughout the treatment period, the plants were carefully maintained under optimal humidity, light, and nutrient conditions.
Samples were collected from three different tissues (needle, phloem, and roots) from all treatments, including abiotic stress (drought and heat), biotic stress (pathogen infection), and control (well-watered) treatments. Tissue samples were snap-frozen in liquid nitrogen immediately after collection and stored at −80°C for further processing.
2.1.2 Field experiment
In addition to the seedlings, samples from mature trees (90–100 years) were collected from research plots of the Czech University of Life Sciences, managed by the School Forest Enterprise (ŠLP) in Kostelec nad Černými lesy (49.9940° N, 14.8592° E) in the eastern district of the Central Bohemian region of the Czech Republic (Singh et al., 2023). The climate in this region is dry and warm in summer, with a vegetation season lasting 150–160 days and an average annual temperature and precipitation of 7–7.5°C and 600 mm, respectively. Norway spruce tissue samples (needle, phloem, and root) were collected from 16 trees in August 2022. The needle samples were collected by shooting branches with a shotgun (Burnett et al., 2021), while phloem and root samples were obtained using a 5 mm cork borer. The phloem samples were collected from the trunk at a height of 2 m. The cork borer was used to extract tissue cores that included both the phloem and cambium layers (Mao et al., 2019). For the root samples, lateral roots were selected from the same trees approximately 1 m from the trunk base (Kalyniukova et al., 2024). After carefully removing layers of soil and moss, the roots were exposed and sampled at 15 cm depth using the same 5 mm cork borer. All tissue samples were snap-frozen in liquid nitrogen immediately after collection and stored at −80°C for further processing. Similar to the greenhouse experiment, four biological replicates were used for each tissue type, with each replicate composed of four individual samples pooled together to reduce individual heterogenicity. The experimental design is schematically represented in Figure 1.
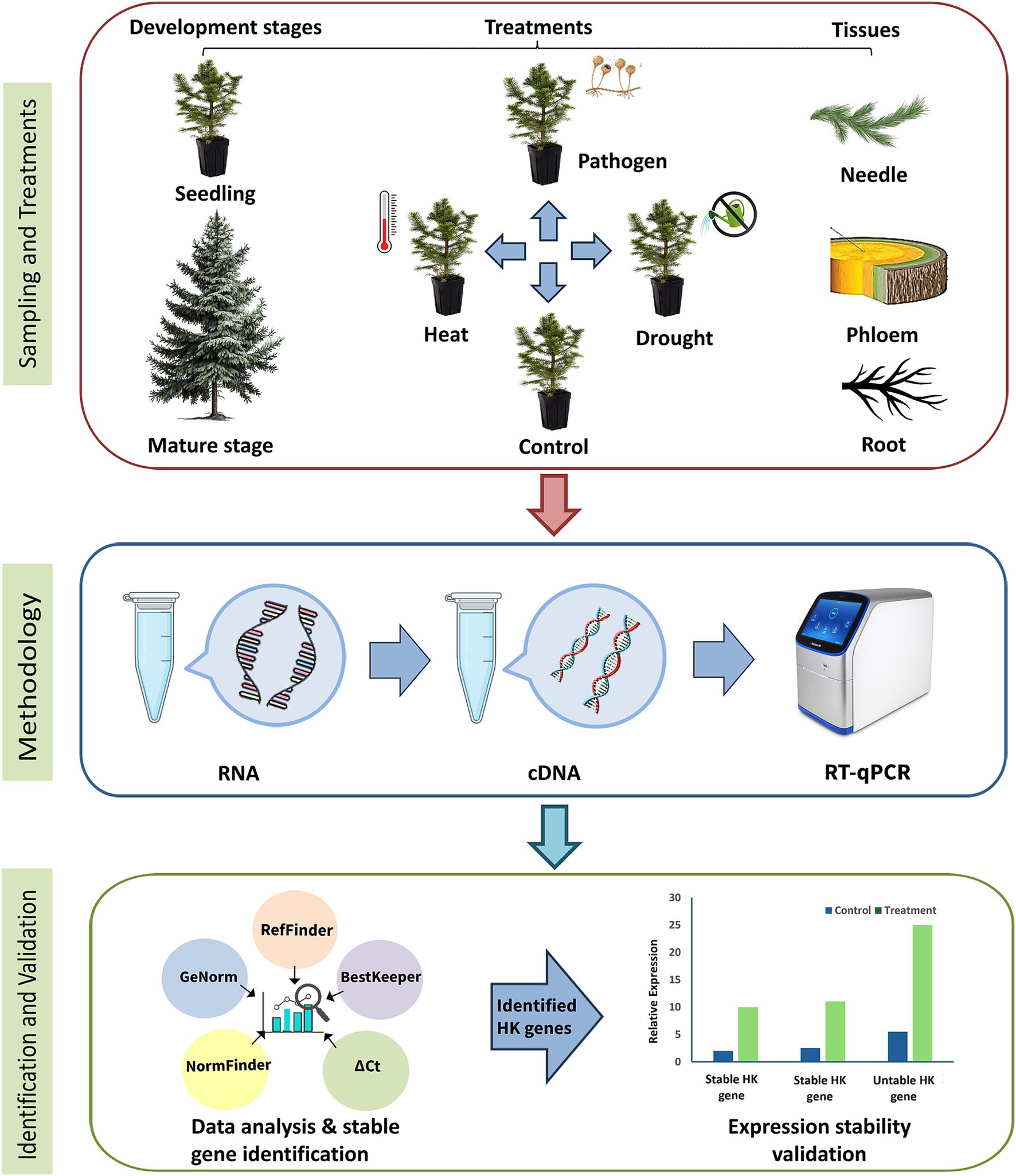
Figure 1. Schematic representation of the experimental design used to identify suitable reference genes in Norway spruce.
2.2 Selection of candidate reference genes for evaluation
A total of 15 candidate internal control genes, RPL26, PP2A2, RPL7Ae, UBCP, SKIP22, HSP90, CCZ1, SDH5, RID2, JMJ16, COG7, RPS10, SP1, TULP6, and ARP9 were selected for identifying the most suitable reference genes in Norway spruce (Table 1). The selected candidate genes were previously identified in other conifer species (de Vega-Bartol et al., 2013; Bao et al., 2016; Chen et al., 2019; Mo et al., 2019) and showed consistent expression in our in-house transcriptome data (Unpublished data). Primers for selected genes were designed through the PrimerQuest™ Tool (Integrated DNA Technologies, United States), and their primer efficiency and correlation coefficients (R2) were calculated (Table 1). The sequence used to design primers is provided in Supplementary material S1.
2.3 Total RNA extraction, cDNA synthesis, primer design, and quantitative RT–qPCR analysis
Total RNA from all three tissues (needle, phloem, and root) was isolated using a modified cetyltrimethylammonium bromide (CTAB) protocol described by Singh et al. (2024b). Briefly, 100 mg of homogenized tissue was added to the CTAB extraction buffer, followed by the addition of an equal amount of Chloroform:isoamyl alcohol (24:1) and subsequent centrifugation. The top aqueous layer was transferred to a new tube, and 0.5 volume of 5 M lithium chloride was added and incubated for 1 h at −20°C. Then, the mixture was centrifuged at 12,000 rpm for 10 min at 4°C, and the supernatant was discarded. The resulting RNA pellet was washed twice with 70% ethanol. The dried RNA pellet was eluted in 50 μL RNase-free water. The isolated RNA was further treated with the TURBO DNase Kit (Invitrogen, United States) to remove any genomic DNA contamination. RNA integrity and purity (260/280 and 260/230 nm absorbance ratios) were verified by electrophoresis on a 1.2% agarose gel and NanoDrop spectrophotometer (Thermo Scientific™, USA), respectively. Complementary DNA (cDNA) was synthesized from 1 μg of RNA using a High-Capacity cDNA Reverse Transcription Kit (Applied Biosystems, USA) according to the manufacturer’s protocol and stored at −20°C.
Before performing RT–qPCR, the cDNA samples were diluted by a factor of five. The RT–qPCR reaction mixture consisted of 5.0 μL of SYBR® Green PCR Master Mix (Applied Biosystems, United States), 1.0 μL of cDNA from fivefold dilutions, 10 μM of forward and reverse primers, and 3.0 μL of RNase-free water (Invitrogen, USA) to make up a total volume of 10.0 μL. Amplification was conducted with initial denaturation at 95°C for 10 min, followed by 40 cycles of 95°C for 15 s and 60°C for 1 min. The reactions were carried out using an Applied Biosystems™ StepOne™ Real-Time PCR System (Applied Biosystems, United States). To verify primer specificity, melting curve analysis was performed, ensuring gene-specific amplification with a gradual increase in temperature from 60 to 95°C. All RT–qPCR assays were conducted with four biological replicates per treatment and tissue, each including four technical replicates.
2.4 Statistical analyses
Five different algorithms and tools, i.e., geNorm, NormFinder, BestKeeper, ΔCt, and RefFinder were used to evaluate the gene expression stability of 15 candidate reference genes in Norway spruce. The comprehensive expression stability of candidate reference genes was evaluated using RefFinder, a web-based tool that combines the outputs of the former four algorithms. The geNorm algorithm calculates the expression stability value (M) and conducts pairwise variation (V) comparisons, where the lowest M value suggests the most stable expression (Vandesompele et al., 2002). NormFinder ranks reference genes based on their expression stability within a given set of samples (Andersen et al., 2004). BestKeeper, another freely available algorithm, assesses the standard deviation and correlation coefficient using the Cq (quantification cycle) values of all reference genes (Pfaffl et al., 2004). The ΔCt method directly evaluates the relative expression of gene pairs within each sample (Silver et al., 2006). The mean Cq values of each reference gene from each experiment served as input data and were processed using RefFinder, which combines widely used reference gene screening programs (geNorm, NormFinder, BestKeeper, and the ΔCt method) to calculate the geometric mean of their ranking values to provide an overall comprehensive ranking.
The optimum number of reference genes required under each experimental condition was determined by pairwise variation (V) analysis by calculating the variable value Vn/n + 1 based on the average expression stability (M) with a cutoff of 0.15 using the geNorm algorithm. The Vn/Vn + 1 value indicates the pairwise variation between two consecutive normalization factors. When the value falls below this threshold, adding the n + 1 reference gene becomes redundant.
2.5 Reference gene validation
Dehydrins are a part of the group 2 late embryogenesis abundant (LEA) protein family that protects conifers against osmotic stress in response to cold and drought conditions (Stival Sena et al., 2018; Čepl et al., 2020; Krokene et al., 2023). To validate the selected reference genes, the relative expression levels of the dehydrin gene PaDhn5 were analyzed according to the 2−ΔΔCt method (Livak and Schmittgen, 2001) using the most and the least stable reference genes. The data was checked for normality using the Kolmogorov–Smirnov test, and mRNA expression levels of target genes were assessed via single factor ANOVA using XLSTAT cloud (ver. 1.0), and a significance level of α = 0.05 was used as the cutoff to indicate significant differences among the control and treatment groups.
3 Results
3.1 Amplification efficiency and specificity of candidate reference genes
Each primer pair produced a single amplicon with an expected amplicon length, as shown by agarose gel electrophoresis (Supplementary material S2). Melting curve analysis revealed a single peak, indicating no primer dimer was present (Supplementary material S3). The amplification efficiency of each primer pair ranged from 91.19 to 114.86%, and the correlation coefficient (R2) values were greater than or equal to 0.879 (Table 1 and Supplementary material S4). The average cycle threshold (Ct) value was 28.14 (Supplementary material S5), while SDH5, SP1, RID2, and ARP9 showed the highest transcript abundance across most experimental conditions. In contrast, RPL7Ae and RPL26 were the least expressed reference genes. The remaining nine reference genes exhibited moderate expression levels.
3.2 Expression stability of candidate reference genes
3.2.1 Tissuewise stability
In needle tissues, the three most stable genes were SP1, JMJ16, and RID2 according to the comprehensive ranking by RefFinder and by ΔCt, while UBCP, HSP90, and SDH5 were the least stable genes (Table 2). The BestKeeper and NormFinder rankings were inconsistent with those of RefFinder and ΔCt, suggesting that PP2A2 and UBCP are the most stable genes, respectively. The geNorm ranking suggested a combination of RID2/ TUPL6 as the most stable gene (Supplementary material S6).
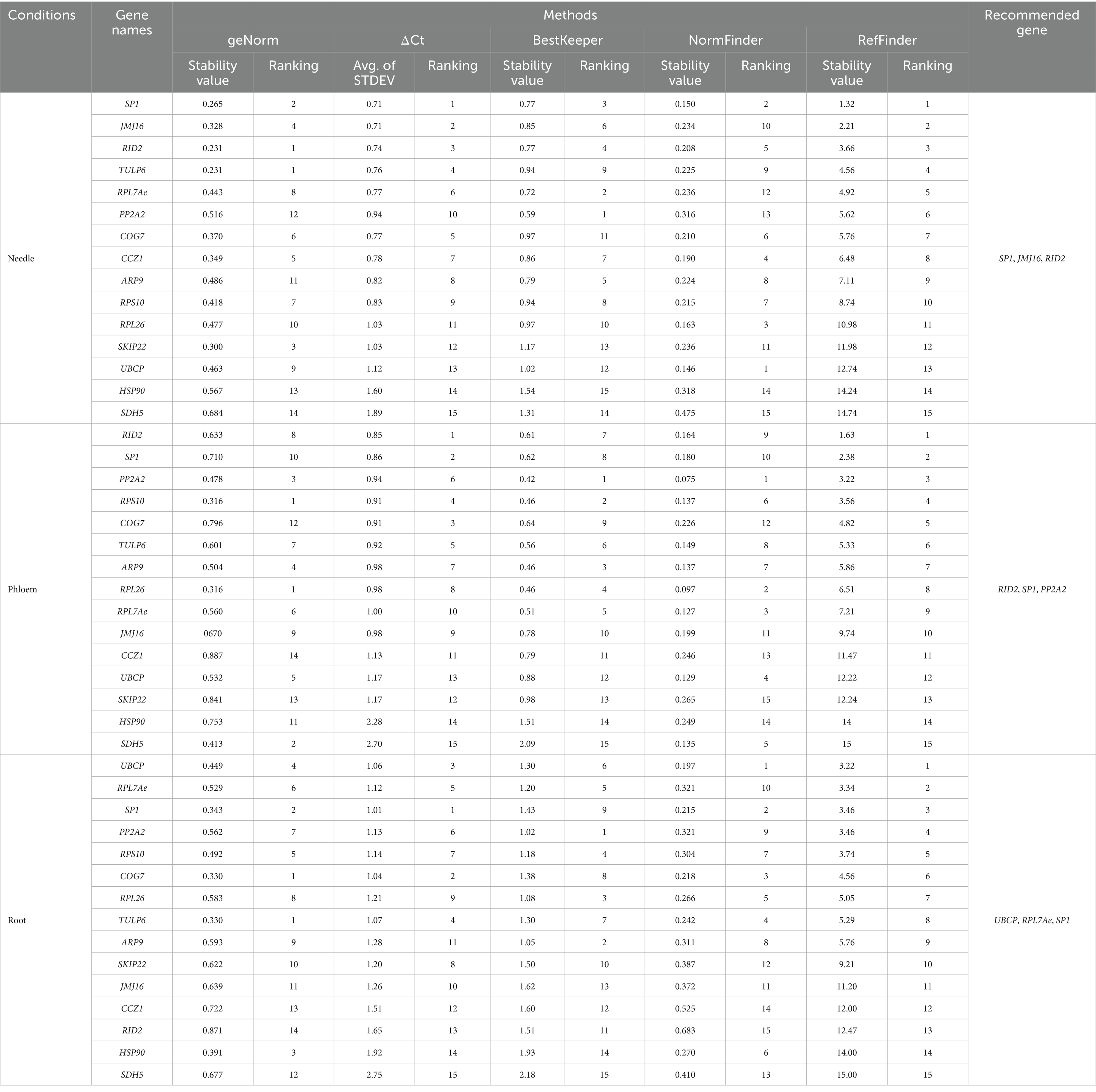
Table 2. Tissue-wise rankings of candidate reference genes based on stability value calculated by ΔCt, BestKeeper, NormFinder, and RefFinder. Each tissue group represent 4 biological replicates, each individually consisting of 4 plants pooled together.
For phloem tissues, RID2, SP1, and PP2A2 were the most stable genes according to RefFinder, while the least stable genes were SKIP22, HSP90, and SDH5. This ranking was consistent with that of the ΔCt method, except that ΔCt ranked COG7 as the third most stable gene. The BestKeeper and NormFinder algorithms ranked PP2A2 as the most stable gene in phloem tissues. According to geNorm, the combination of RPL26/RPS10 was the most stable gene in phloem tissues (Supplementary material S6).
In root tissues, the BestKeeper, ΔCt, and NormFinder rankings for the three most stable genes were not consistent with those of RefFinder. UBCP, RPL7Ae, and SP1 were suggested to be the three most stable genes based on the comprehensive rankings of RefFinder (Table 2). A combination of COG7/TULP6 was the most stable reference gene in root tissues according to geNorm (Supplementary material S6). The least stable reference genes by the RefFinder comprehensive ranking were SDH5, HSP90, and RID2.
3.2.2 Treatmentwise stability
3.2.2.1 Control conditions
According to the comprehensive rankings of RefFinder, the three most stable genes under control conditions were RPS10, RPL26, and SP1, while CCZ1, SDH5, and HSP90 were the least stable (Table 3). BestKeeper ranked RID2 as the most stable gene, whereas NormFinder ranked COG7 as the most stable gene. The ΔCt value indicated that RPS10 was the most stable gene, which is consistent with the RefFinder ranking. The best combination of reference genes according to geNorm was PP2A2/TULP6 under control conditions (Supplementary material S7). The HSP90 gene was the least stable, as determined by geNorm analysis and the other four algorithms.
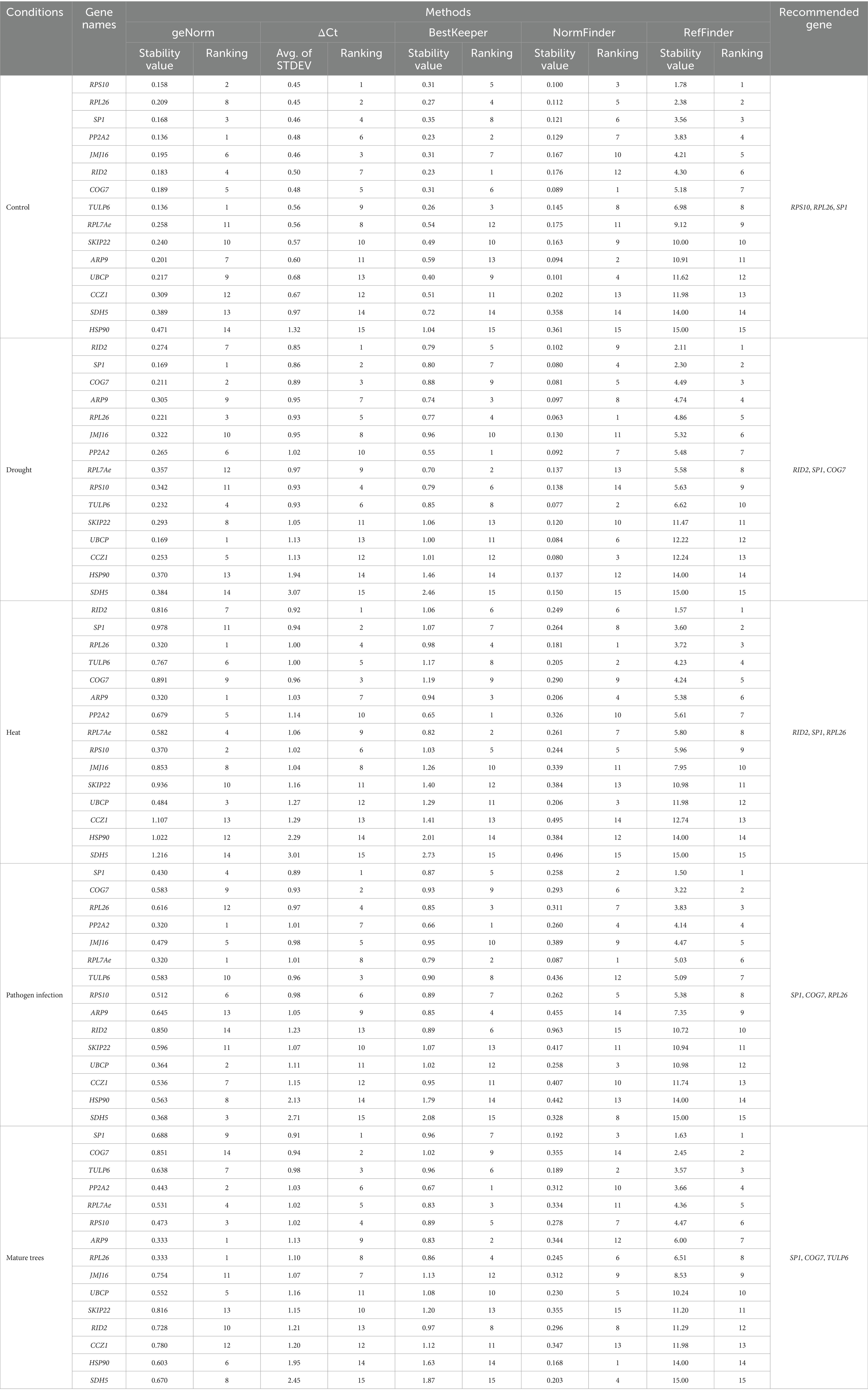
Table 3. Expression stability rankings of the reference genes, calculated by ΔCt, BestKeeper, NormFinder, and RefFinder across various treatment conditions in seedlings and untreated mature trees. Each treatment group represents 4 biological replicates, each individually consisting of 4 plants pooled together.
3.2.2.2 Drought stress conditions
Under drought conditions, RID2, SP1, and COG7 were identified as the three most stable reference genes by the RefFinder algorithm, while a combination of UBCP/SP1 was the most stable as per geNorm (Supplementary material S7). BestKeeper and NormFinder identified PP2A2 and RPL26 as the most stable genes, respectively. Both geNorm and other algorithms (ΔCt, BestKeeper, NormFinder, and RefFinder) identified SDH5 as the least stable reference gene under drought conditions.
3.2.2.3 Heat stress conditions
For studies under heat stress conditions, RID2, SP1, and RPL26 were identified as the most stable genes by comprehensive ranking by the RefFinder program, whereas BestKeeper and NormFinder ranked PP2A2 and RPL26 as the most stable genes, respectively. A combination of RPL26/ARP9 was ranked as the most stable gene according to geNorm (Supplementary material S7). Unanimously, SDH5 was suggested as the least stable gene under heat stress conditions by geNorm and other algorithms.
3.2.2.4 Pathogen infection conditions
The topmost three stable genes after Ophiostomatoid fungus inoculation were SP1, COG7, and RPL26 based on the rankings of the RefFinder algorithm, whereas the combination of PP2A2/RPL7Ae was the most stable according to geNorm (Table 2; Supplementary material S7). BestKeeper and NormFinder ranked PP2A2 and RPL26 as the most stable genes, respectively, which was not consistent with ΔCt and RefFinder rankings. The gene SDH5 was the least stable reference gene after fungal inoculation as per four algorithms, and geNorm ranked RID2 as the least stable reference gene.
3.2.2.5 Mature tree
In mature tree samples, the comprehensive ranking algorithm RefFinder identified SP1, COG7, and TULP6 as the most stable reference genes and SDH5 as the least stable gene. Similar to previous observations, the BestKeeper and NormFinder rankings were not consistent with that of RefFinder. BestKeeper and NormFinder ranked PP2A2 and HSP90, respectively, as the most stable genes. In contrast, a combination of RPL26/ARP9 was suggested to be the most stable gene and COG7 as the least stable reference gene by geNorm analysis (Supplementary material S7).
3.3 Optimal reference gene selection for normalization
To achieve more accurate and reliable gene expression results, it is often recommended to use multiple reference genes. According to Vandesompele et al. (2002), if the Vn/Vn + 1 value is below 0.15, the addition of an additional reference gene (n + 1) is deemed unnecessary, suggesting that the initial reference gene is sufficient for normalizing target gene expression. Using the geNorm algorithm, we determined the optimal number of reference genes for each condition. Our analysis revealed that at least two reference genes were necessary for accurate normalization in (mature trees and combined conditions), as indicated by the pairwise comparison values (Figure 2).
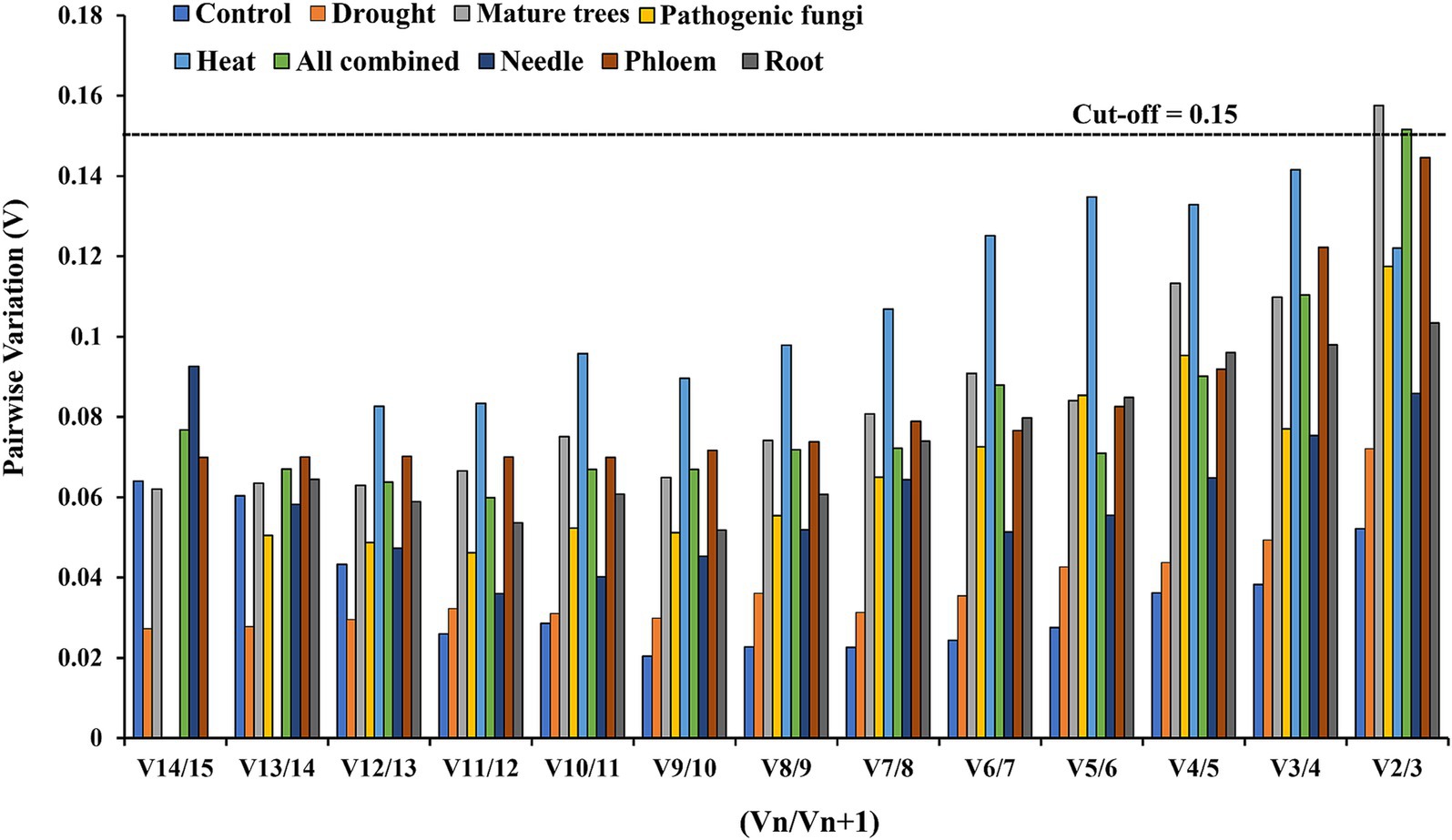
Figure 2. Pairwise variation analysis to determine the optimal number of reference genes required for normalization. The recommended cutoff value is 0.15, under which another gene is not required for normalization.
3.4 Validation of reference gene selection
Based on the RefFinder comprehensive analysis, SP1 and COG7 were identified as the most stable genes, whereas SDH5 was identified as the least stable gene for gene expression studies in Norway spruce samples under drought conditions. To validate the reliability of the candidate reference genes, the relative expression of the target gene PaDhn5 under drought stress was normalized with the most stable reference genes (SP1 and COG7) and the least stable reference gene (SDH5). The results indicated that the expression levels of PaDhn5 in drought-treated samples increased by 8-fold and 6-fold (p-values = 0.040 and 0.045), respectively, when normalized with the stable reference genes SP1 and COG7, compared to the control. In contrast, normalization with the least stable reference gene SDH5 resulted in an almost 20-fold increase (p = 0.031) in expression (Figure 3).
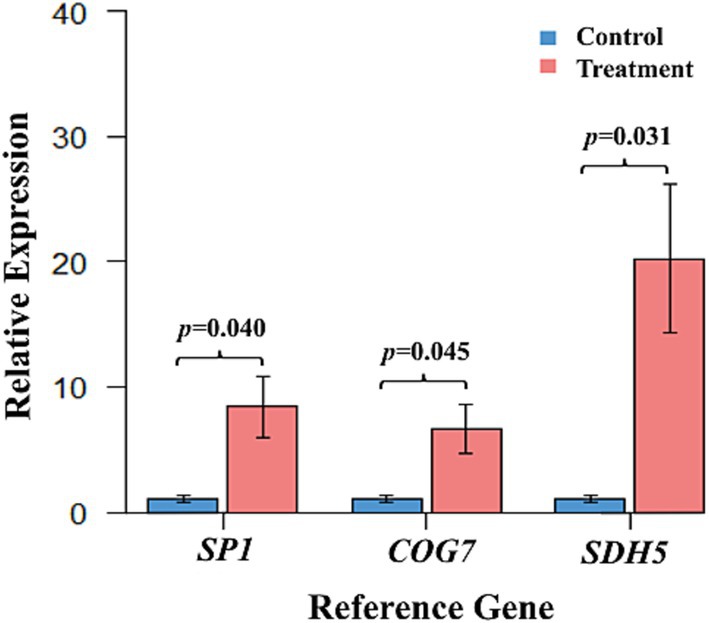
Figure 3. Relative expression levels of the target gene PaDhn5 in drought-treated and control phloem samples after normalization with the most stable and the least stable gene. Normalization was performed using the most stable genes, SP1 and COG7, and the least stable gene, SDH5, under drought conditions. The data represent the mean ± SD values of four biological replicates. Single-factor ANOVA was performed to compare the control and treatment groups of each gene to statistically analyze the relative expression of PaDhn5. p-values for each were generated as 0.04, 0.045, and 0.031 for the expression of PaDhn5 when calculated against reference genes SP1, COG7, and SDH5, respectively.
4 Discussion
In recent years, forests worldwide have experienced climate change-driven droughts, storms, windthrows, and high-temperature disturbances, leading to extensive tree mortality. In Europe, one of the most economically important forest tree species is Norway spruce, which was widely planted outside its natural distribution area during the eighteenth and nineteenth centuries. Nevertheless, recent climatic conditions have led to a decline in its vitality, increasing susceptibility to stress and rendering the species particularly vulnerable to the tree-killing spruce bark beetle, Ips typographus and other Ips beetles (Biedermann et al., 2019; Singh et al., 2024a). These factors underscore the importance of understanding the molecular responses of Norway spruce to both abiotic and biotic stresses.
RT–qPCR is a highly effective and dependable technique for quantitatively studying the relative abundance of target genes. This method is widely employed to investigate plant-pathogen interactions and uncover underlying molecular mechanisms (Vijayakumar and Sakuntala, 2024). RT–qPCR is a relatively less expensive and faster method for exploring target genes under specific conditions. Despite its frequent use, there are concerns that researchers may not always adhere to recommended protocols, which can lead to misleading results (Bustin et al., 2009). Housekeeping genes, commonly referred to as reference genes, are a group of genes that are consistently expressed in cells and are crucial for fundamental cellular functions. They are stable throughout a species irrespective of treatments but can be specific to a particular tissue or developmental stage (Lin and Lai, 2010; Li et al., 2021). For accurate measurement of relative gene expression, it is crucial to use multiple reference genes with stable expression under specific biological conditions (Dai et al., 2018; Wang et al., 2021). While RT–qPCR is effective and reliable for quantifying mRNA levels across different experimental conditions, several factors, such as RNA extraction, cDNA synthesis, primer design, and material handling, can impact the results (Schmittgen and Zakrajsek, 2000; Bustin et al., 2005; Huggett et al., 2005). Reliable reference genes help mitigate these variations, necessitating the evaluation of the stability of reference genes for each experimental condition to ensure precise and reliable data interpretation. Recent studies have evaluated reference genes in various coniferous species (e.g., Bao et al., 2016; Mo et al., 2019; Chen et al., 2019); however still, such studies on Norway spruce are limited to the embryogenic developmental stages (e.g., Vestman et al., 2011; de Vega-Bartol et al., 2013).
Among the 15 evaluated candidates, SP1, COG7, and TULP6 were the most stable reference genes, making them suitable for gene expression normalization in Norway spruce. The SP1 ubiquitin-protein ligase plays a crucial role in regulating chloroplast protein import, essential for plant development (Ling and Jarvis, 2015). It interacts with the translocon at the outer envelope membrane of chloroplasts (TOC) to recognize and import client proteins. SP1 ubiquitin-protein ligase associates with TOC complexes and mediates the ubiquitination of TOC components, leading to their degradation through the ubiquitin-proteasome system (UPS) and reorganization of the TOC machinery. Ubiquitin-protein ligase (ubiquitin-conjugating enzyme) genes have been identified as suitable reference genes in various tissues of Brazilian rubber trees (Hevea brasiliensis) (Li et al., 2011), in alfalfa (Medicago sativa) cultivars under different stress conditions (Castonguay et al., 2015), in Arabidopsis thaliana (Czechowski et al., 2005), Brachypodium distachyton (Hong et al., 2008), Vernicia fordii (Han et al., 2012), Capsicum annuum (Wan et al., 2011) and Brachiaria brizantha (Silveira et al., 2009). Our findings are in accordance with these studies, showing stable expression of SP1 in various tissue types and under various environmental conditions (Tables 2–4). Nevertheless, not all ubiquitin conjugating enzymes can be used as internal controls for normalization (Wan et al., 2011).
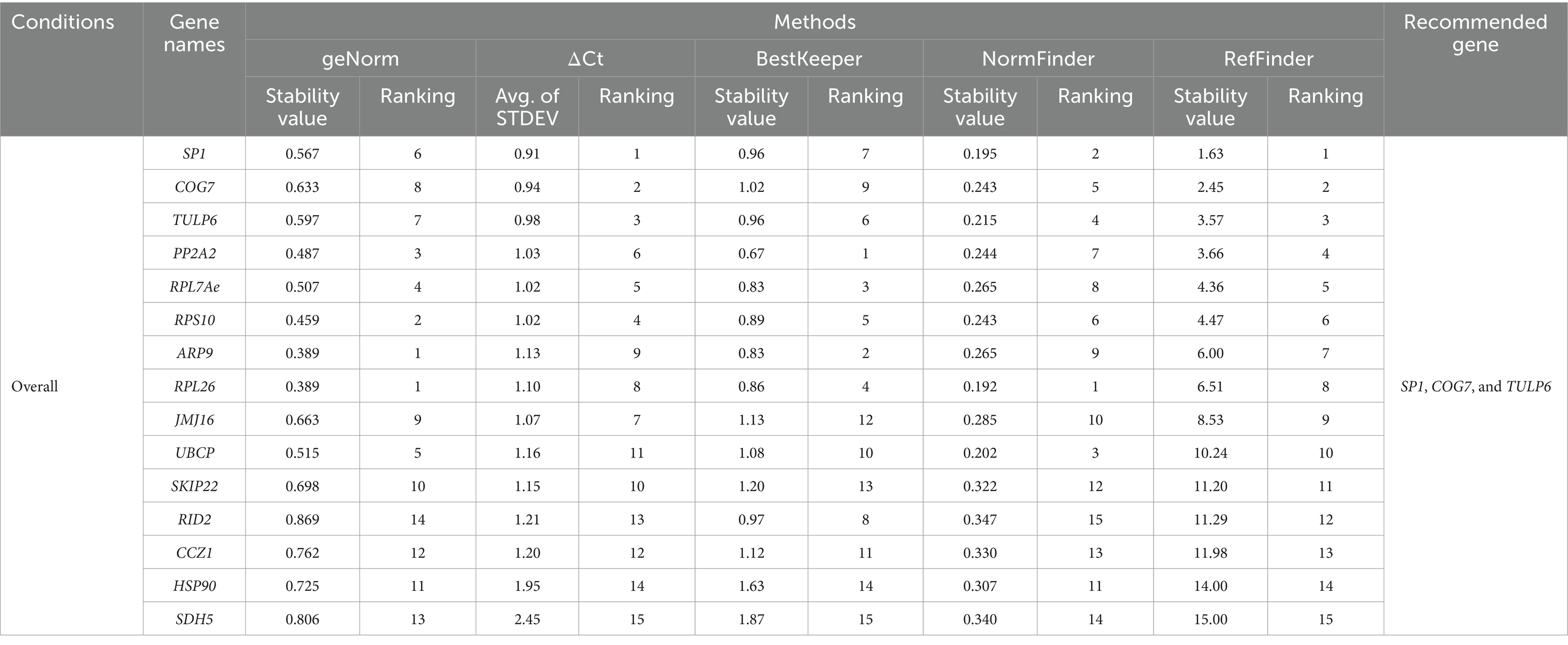
Table 4. Overall expression stability rankings of the reference genes throughout the tissues across all stress treatments, tissue types, and developmental stages as calculated by geNorm, ΔCt, BestKeeper, NormFinder, and RefFinder.
Conserved oligomeric Golgi complex subunit 7 (COG7) is a crucial component of the COG complex and is essential for maintaining the normal morphology and function of the Golgi apparatus (Klink et al., 2022). Specifically, COG7 is part of lobe B of the COG complex, which contains COGs5-8 (Ungar et al., 2002; Blackburn et al., 2019). This protein plays a vital role in various developmental processes, including embryo development, pigmentation, cell and organ expansion, and the formation of the organized shoot apical meristem (Ishikawa et al., 2008; Vukašinović et al., 2017; Rui et al., 2020, 2021). Despite its unstable expression across different tissue types, COG7 was ranked second in overall expression stability after SP1, according to RefFinder analysis. This implies that COG7 could be a viable reference gene for gene expression normalization, particularly in experiments investigating mechanisms of resistance to drought and pathogens (Tables 3, 4). To our knowledge, no previous studies have considered COG7 as a candidate reference gene.
Tubby-like F-box proteins (TULPs) were first discovered in mice and are highly conserved across a wide range of organisms. In plants, these Tubby-like proteins play a role in stress signaling pathways (Lai et al., 2004; Reitz et al., 2013). To date, 11 Tubby genes have been identified in Arabidopsis, 14 in rice, and 11 in poplar (Yang et al., 2008; Hong et al., 2015). Most plant TULPs possess a conserved F-box domain at the N-terminus and a Tubby domain at the C-terminus (Yang et al., 2008). Although TULPs are believed to function as transcription factors, their exact mechanisms of action remain largely unknown (Yulong et al., 2016). TULP6, while exhibiting variable expression across treatments, was the third most stable gene after SP1 and COG7 according to RefFinder analysis, indicating its potential as a reference gene for normalizing gene expression, especially in studies involving developmental stages (Tables 3, 4). Notably, TULPs have not been previously considered as reference genes.
To validate the identified reference genes, we evaluated the expression of dehydrin-like protein 5 (PaDhn5), a stress-responsive protein, across various Norway spruce tissues, developmental stages, and stress conditions. Dehydrins, part of the late embryogenesis abundant (LEA) family, accumulate in response to abiotic stresses and play a role in cryoprotection and membrane protection from reactive oxygen species (Close, 1997; Nylander et al., 2001; Rorat et al., 2006; Sun et al., 2021). Our results revealed that using the least stable reference gene (SDH5) led to erroneous data, showing a 20-fold increase in PaDhn5 expression under stress conditions. In contrast, the most stable reference genes, SP1 and COG7, resulted in more consistent PaDhn5 expression levels, with an 8-fold and 6-fold increase, respectively (Figure 3). The significant discrepancy between the most and least stable reference genes highlights the importance of using stable reference genes to prevent bias in RT-qPCR normalization. Based on our findings, we recommend using the identified stable reference genes and their combinations (Figure 4) for accurate normalization in RT-qPCR analyses of gene expression in Norway spruce, which will improve the sensitivity and reproducibility of the results.
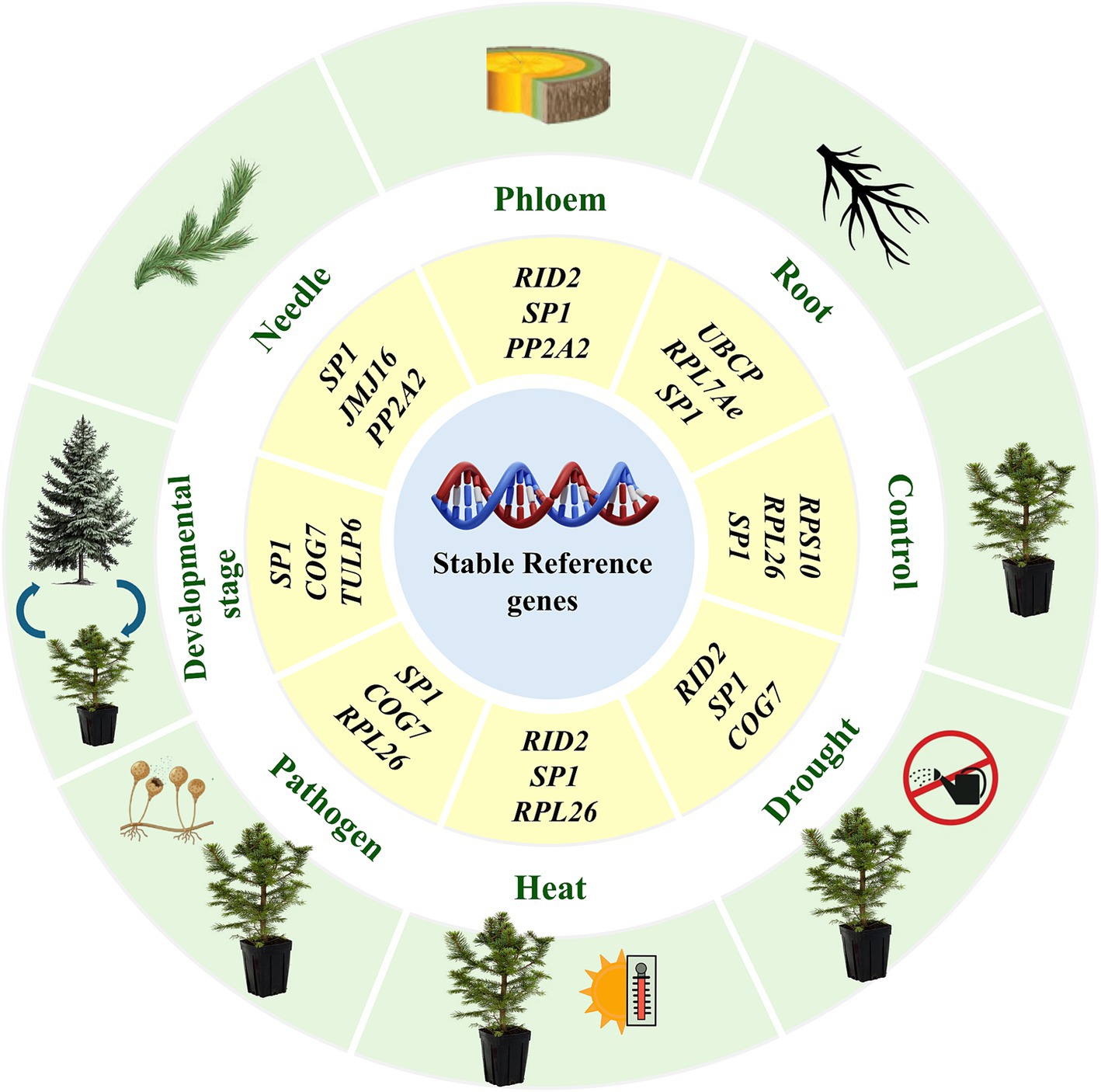
Figure 4. Recommended reference genes under different experimental conditions, tissues, and developmental stages.
5 Conclusion
This study provides a catalog of genes that have been reported in various conifer species and validated for Norway spruce for the first time. We evaluated 15 candidate reference genes in various tissues and developmental stages under different environmental conditions using RT–qPCR and systematically assessed their expression stability to identify the most suitable reference gene for each condition. The study identified ubiquitin-protein ligase (SP1), conserved oligomeric Golgi complex (COG7), and tubby-like F-box protein (TULP6) as the most suitable candidate reference genes, while succinate dehydrogenase (SDH5) was found to be the least stable. Notably, this is the first report testing COG7 and TULP6 as candidates for reference genes and confirming their stable expression. In addition, normalizing RT–qPCR data with both stably and unstably expressed genes showed that PaDhn5 expression aligns with the current knowledge of Norway spruce physiology. The fluctuations in gene expression between stable and unstable gene normalizations emphasize the need for validating reference genes for reliable RT–qPCR results. In summary, the identified and recommended reference genes and their combinations for normalizing gene expression in Norway spruce under various experimental conditions will benefit future gene expression and functional genomics studies in Norway spruce and related conifer species.
Data availability statement
The original contributions presented in the study are included in the article/Supplementary material, further inquiries can be directed to the corresponding author.
Author contributions
VVS: Conceptualization, Methodology, Data curation, Formal analysis, Visualization, Resources, Project administration, Funding acquisition, Writing – original draft, Writing – review & editing. AN: Methodology, Data curation, Formal analysis, and Writing – review & editing. GS: Methodology, Data curation, Formal analysis, Writing – review & editing. KM: Methodology, Writing – review & editing. RG: Methodology, Writing – review & editing. AR: Methodology, Resources, Writing – review & editing. RJ: Writing – review & editing.
Funding
The author(s) declare that financial support was received for the research, authorship, and/or publication of this article. This work was funded by the Internal Grant Agency (IGA; grant no. 43950/1312/3123 awarded to VVS) at the Faculty of Forestry and Wood Sciences, Czech University of Life Sciences, Prague, Czech Republic and the Ministry of Education, Youth and Sports of the Czech Republic (grant INTER-TRANSFER LTT20017).
Acknowledgments
AN, GS, and AR acknowledge support by Excellent Team Grants from the Faculty of Forestry and Wood Sciences, Czech University of Life Sciences, Prague, Czech Republic. KM acknowledges the Ramalingaswami Re-entry Fellowship (BT/RLF/Re-entry/11/2023) from the Department of Biotechnology, Government of India. The authors thank Dr. Ivana Tomášková and Dr. Kateřina Hamouzová for providing supporting facilities, and Dr. Madhab Kumar Sen for his assistance and consultations. The authors also thank the editor and reviewers for their helpful suggestions, which improved the quality of this work.
Conflict of interest
The authors declare that the research was conducted in the absence of any commercial or financial relationships that could be construed as a potential conflict of interest.
Publisher’s note
All claims expressed in this article are solely those of the authors and do not necessarily represent those of their affiliated organizations, or those of the publisher, the editors and the reviewers. Any product that may be evaluated in this article, or claim that may be made by its manufacturer, is not guaranteed or endorsed by the publisher.
Supplementary material
The Supplementary material for this article can be found online at: https://www.frontiersin.org/articles/10.3389/ffgc.2024.1458554/full#supplementary-material
References
Andersen, C. L., Jensen, J. L., and Orntoft, T. F. (2004). Normalization of real-time quantitative reverse transcription-PCR data: a model-based variance estimation approach to identify genes suited for normalization, applied to bladder and colon cancer data sets. Cancer Res. 64, 5245–5250. doi: 10.1158/0008-5472.can-04-0496
Bao, W., Qu, Y., Shan, X., and Wan, Y. (2016). Screening and validation of housekeeping genes of the root and cotyledon of Cunninghamia lanceolata under abiotic stresses by using quantitative real-time PCR. Int. J. Mol. Sci. 17:1198. doi: 10.3390/ijms17081198
Bharati, R., Sen, M. K., Kumar, R., Gupta, A., Žiarovská, J., Fernández-Cusimamani, E., et al. (2023). Systematic identification of suitable reference genes for quantitative real-time PCR analysis in Melissa officinalis L. Plants 12:470. doi: 10.3390/plants12030470
Biedermann, P. H., Müller, J., Grégoire, J. C., Gruppe, A., Hagge, J., Hammerbacher, A., et al. (2019). Bark beetle population dynamics in the Anthropocene: challenges and solutions. Trends Ecol. Evol. 34, 914–924. doi: 10.1016/j.tree.2019.06.002
Blackburn, J. B., D’Souza, Z., and Lupashin, V. V. (2019). Maintaining order: COG complex controls Golgi trafficking, processing, and sorting. FEBS Lett. 593, 2466–2487. doi: 10.1002/1873-3468.13570
Burnett, A. C., Serbin, S. P., Lamour, J., Anderson, J., Davidson, K. J., Yang, D., et al. (2021). Seasonal trends in photosynthesis and leaf traits in scarlet oak. Tree Physiol. 41, 1413–1424. doi: 10.1093/treephys/tpab015
Bustin, S. A. (2002). Quantification of mRNA using real-time reverse transcription PCR (RT-PCR): trends and problems. J. Mol. Endocrinol. 29, 23–39. doi: 10.1677/jme.0.0290023
Bustin, S. A., Benes, V., Garson, J. A., Hellemans, J., Huggett, J., Kubista, M., et al. (2009). The MIQE guidelines: minimum information for publication of quantitative real-time PCR experiments. Clin. Chem. 55, 611–622. doi: 10.1373/clinchem.2008.112797
Bustin, S. A., Benes, V., Nolan, T., and Pfaffl, M. W. (2005). Quantitative real-time RT-PCR–a perspective. J. Mol. Endocrinol. 34, 597–601. doi: 10.1677/jme.1.01755
Castonguay, Y., Michaud, J., and Dubé, M. P. (2015). Reference genes for RT-qPCR analysis of environmentally and developmentally regulated gene expression in alfalfa. Am. J. Plant Sci. 6, 132–143. doi: 10.4236/ajps.2015.61015
Caudullo, G., Tinner, W., and de Rigo, D. (2016). “Picea abies in Europe: distribution, habitat, usage and threats” in European atlas of forest tree species. eds. J. San-Miguel-Ayanz, D. de Rigo, G. Caudullo, T. Houston Durrant, and A. Mauri (Luxembourg: Publication Office of the European Union), 114–116.
Čepl, J., Stejskal, J., Korecký, J., Hejtmánek, J., Faltinová, Z., Lstibůrek, M., et al. (2020). The dehydrins gene expression differs across ecotypes in Norway spruce and relates to weather fluctuations. Sci. Rep. 10:20789. doi: 10.1038/s41598-020-76900-x
Chen, R., Chen, W., Tigabu, M., Zhong, W., Li, Y., Ma, X., et al. (2019). Screening and evaluation of stable reference genes for quantitative real-time polymerase chain reaction (qRT-PCR) analysis in Chinese fir roots under water, phosphorus, and nitrogen stresses. Forests 10:1087. doi: 10.3390/f10121087
Close, T. J. (1997). Dehydrins: a commonalty in the response of plants to dehydration and low temperature. Physiol. Plant. 100, 291–296. doi: 10.1111/j.1399-3054.1997.tb04785.x
Czechowski, T., Stitt, M., Altmann, T., Udvardi, M. K., and Scheible, W. R. (2005). Genome-wide identification and testing of superior reference genes for transcript normalization in arabidopsis. Plant Physiol. 139, 5–17. doi: 10.1104/pp.105.063743
Dai, F., Zhao, X., Tang, C., Wang, Z., Kuang, Z., Li, Z., et al. (2018). Identification and validation of reference genes for qRT-PCR analysis in mulberry (Morus alba L.). PLoS One 13:e0194129. doi: 10.1371/journal.pone.0194129
Danielsen, J. S., Morgado, L., Mundra, S., Nybakken, L., Davey, M., and Kauserud, H. (2021). Establishment of spruce plantations in native birch forests reduces soil fungal diversity. FEMS Microbiol. Ecol. 97:fiab074. doi: 10.1093/femsec/fiab074
de Oliveira, L. A., Breton, M. C., Bastolla, F. M., Camargo, S. D., Margis, R., Frazzon, J., et al. (2012). Reference genes for the normalization of gene expression in Eucalyptus species. Plant Cell Physiol. 53, 405–422. doi: 10.1093/pcp/pcr187
de Vega-Bartol, J. J., Santos, R. R., Simões, M., and Miguel, C. M. (2013). Normalizing gene expression by quantitative PCR during somatic embryogenesis in two representative conifer species: Pinus pinaster and Picea abies. Plant Cell Rep. 32, 715–729. doi: 10.1007/s00299-013-1407-4
Dobor, L., Hlásny, T., and Zimová, S. (2020). Contrasting vulnerability of monospecific and species-diverse forests to wind and bark beetle disturbance: the role of management. Ecol. Evol. 10, 12233–12245. doi: 10.1002/ece3.6854
Ebner, G. (2020). Significantly more damaged wood in 2019. Available at: https://www.timberonline.net/log_wood/2020/02/significantly-more-damagedwood-in-2019.html (Accessed May 12, 2024).
Gachon, C., Mingam, A., and Charrier, B. (2004). Real-time PCR: what relevance to plant studies? J. Exp. Bot. 55, 1445–1454. doi: 10.1093/jxb/erh181
Gonçalves, S., Cairney, J., Maroco, J., Oliveira, M. M., and Miguel, C. (2005). Evaluation of control transcripts in real-time RT-PCR expression analysis during maritime pine embryogenesis. Planta 222, 556–563. doi: 10.1007/s00425-005-1562-0
Han, X., Lu, M., Chen, Y., Zhan, Z., Cui, Q., and Wang, Y. (2012). Selection of reliable reference genes for gene expression studies using real-time PCR in tung tree during seed development. PLoS One 7:e43084. doi: 10.1371/journal.pone.0043084
Hlásny, T., Krokene, P., Liebhold, A., Montagné-Huck, C., Müller, J., Qin, H., et al. (2019). Living with bark beetles: Impacts, outlook and management options. Joensuu: European Forest Institute.
Hong, M. J., Kim, D. Y., and Seo, Y. W. (2015). Interactions between wheat tubby-like and SKP1-like proteins. Genes Genet. Syst. 90, 293–304. doi: 10.1266/ggs.14-00084
Hong, S. Y., Seo, P. J., Yang, M. S., Xiang, F., and Park, C. M. (2008). Exploring valid reference genes for gene expression studies in Brachypodium distachyon by real-time PCR. BMC Plant Biol. 8, 1–11. doi: 10.1186/1471-2229-8-112
Huggett, J., Dheda, K., Bustin, S., and Zumla, A. (2005). Real-time RT-PCR normalisation; strategies and considerations. Genes Immun. 6, 279–284. doi: 10.1038/sj.gene.6364190
Huis, R., Hawkins, S., and Neutelings, G. (2010). Selection of reference genes for quantitative gene expression normalization in flax (Linum usitatissimum L.). BMC Plant Biol. 10:71. doi: 10.1186/1471-2229-10-71
Ishikawa, T., Machida, C., Yoshioka, Y., Ueda, T., Nakano, A., and Machida, Y. (2008). EMBRYO YELLOW gene, encoding a subunit of the conserved oligomeric Golgi complex, is required for appropriate cell expansion and meristem organization in Arabidopsis thaliana. Genes Cells 13, 521–535. doi: 10.1111/j.1365-2443.2008.01186.x
Jain, M., Nijhawan, A., Tyagi, A. K., and Khurana, J. P. (2006). Validation of housekeeping genes as internal control for studying gene expression in rice by quantitative real-time PCR. Biochem. Biophys. Res. Commun. 345, 646–651. doi: 10.1016/j.bbrc.2006.04.140
Kalyniukova, A., Várfalvyová, A., Płotka-Wasylka, J., Majchrzak, T., Makoś-Chełstowska, P., Tomášková, I., et al. (2024). Deep eutectic solvent-based shaking-assisted extraction for determination of bioactive compounds from Norway spruce roots. Front. Chem. 12:1385844. doi: 10.3389/fchem.2024.1385844
Klink, V. P., Lawaju, B. R., Niraula, P. M., Sharma, K., McNeece, B. T., Pant, S. R., et al. (2022). The conserved oligomeric Golgi (COG) complex, a window into plant-pathogen interactions. J. Plant Interact. 17, 344–360. doi: 10.1080/17429145.2022.2041743
Krokene, P., Børja, I., Carneros, E., Eldhuset, T. D., Nagy, N. E., Volařík, D., et al. (2023). Effects of combined drought and pathogen stress on growth, resistance and gene expression in young Norway spruce trees. Tree Physiol. 43, 1603–1618. doi: 10.1093/treephys/tpad062
Lai, C. P., Lee, C. L., Chen, P. H., Wu, S. H., Yang, C. C., and Shaw, J. F. (2004). Molecular analyses of the Arabidopsis TUBBY-like protein gene family. Plant Physiol. 134, 1586–1597. doi: 10.1104/pp.103.037820
Li, H., Qin, Y., Xiao, X., and Tang, C. (2011). Screening of valid reference genes for real-time RT-PCR data normalization in Hevea brasiliensis and expression validation of a sucrose transporter gene HbSUT3. Plant Sci. 181, 132–139. doi: 10.1016/j.plantsci.2011.04.014
Li, T., Yuan, W., Qiu, S., and Shi, J. (2021). Selection of reference genes for gene expression analysis in Liriodendron hybrids’ somatic embryogenesis and germinative tissues. Sci. Rep. 11:4957. doi: 10.1038/s41598-021-84518-w
Lin, Y. L., and Lai, Z. X. (2010). Reference gene selection for qPCR analysis during somatic embryogenesis in longan tree. Plant Sci. 178, 359–365. doi: 10.1016/j.plantsci.2010.02.005
Ling, Q., and Jarvis, P. (2015). Regulation of chloroplast protein import by the ubiquitin E3 ligase SP1 is important for stress tolerance in plants. Curr. Biol. 25, 2527–2534. doi: 10.1016/j.cub.2015.08.015
Livak, K. J., and Schmittgen, T. D. (2001). Analysis of relative gene expression data using real-time quantitative PCR and the 2−ΔΔCT method. Methods 25, 402–408. doi: 10.1006/meth.2001.1262
Lu, J., Yang, C., Zhang, Y., and Pan, H. (2018). Selection of reference genes for the normalization of RT-qPCR data in gene expression studies in insects: a systematic review. Front. Physiol. 9:1560. doi: 10.3389/fphys.2018.01560
Mao, J., He, Z., Hao, J., Liu, T., Chen, J., and Huang, S. (2019). Identification, expression, and phylogenetic analyses of terpenoid biosynthesis-related genes in secondary xylem of loblolly pine (Pinus taeda L.) based on transcriptome analyses. PeerJ 7:e6124. doi: 10.7717/peerj.6124
Mo, J., Xu, J., Jin, W., Yang, L., Yin, T., and Shi, J. (2019). Identification of reference genes for quantitative gene expression studies in Pinus massoniana and its introgression hybrid. Forests 10:787. doi: 10.3390/f10090787
Mogilicherla, K., Solanke, A. U., Prabhakaran, N., Ahmad, I., Dhandapani, G., Jayabalan, N., et al. (2016). Evaluation of suitable reference genes for normalization of qPCR gene expression studies in brinjal (Solanum melongena L.) during fruit developmental stages. Appl. Biochem. Biotechnol. 178, 433–450. doi: 10.1007/s12010-015-1884-8
Nicot, N., Hausman, J. F., Hoffmann, L., and Evers, D. (2005). Housekeeping gene selection for real-time RT-PCR normalization in potato during biotic and abiotic stress. J. Exp. Bot. 56, 2907–2914. doi: 10.1093/jxb/eri285
Nylander, M., Svensson, J., Palva, E. T., and Welin, B. V. (2001). Stress-induced accumulation and tissue-specific localization of dehydrins in Arabidopsis thaliana. Plant Mol. Biol. 45, 263–279. doi: 10.1023/A:1006469128280
Nystedt, B., Street, N. R., Wetterbom, A., Zuccolo, A., Lin, Y. C., Scofield, D. G., et al. (2013). The Norway spruce genome sequence and conifer genome evolution. Nature 497, 579–584. doi: 10.1038/nature12211
Paolacci, A. R., Tanzarella, O. A., Porceddu, E., and Ciaffi, M. (2009). Identification and validation of reference genes for quantitative RT-PCR normalization in wheat. BMC Mol. Biol. 10:11. doi: 10.1186/1471-2199-10-11
Pastierovič, F., Mogilicherla, K., Hradecký, J., Kalyniukova, A., Dvořák, O., Roy, A., et al. (2024). Genome-wide transcriptomic and metabolomic analyses unveiling the defence mechanisms of Populus tremula against sucking and chewing insect herbivores. Int. J. Mol. Sci. 25:6124. doi: 10.3390/ijms25116124
Pfaffl, M. W., Tichopad, A., Prgomet, C., and Neuvians, T. P. (2004). Determination of stable housekeeping genes, differentially regulated target genes and sample integrity: BestKeeper-excel-based tool using pairwise correlations. Biotechnol. Lett. 26, 509–515. doi: 10.1023/b:bile.0000019559.84305.47
Reitz, M., Pai, S., Imani, J., and Schafer, P. (2013). New insights into the subcellular localization of tubby-like proteins and their participation in the Arabidopsis-Piriformospora indica interaction. Plant Signal. Behav. 8:e25198. doi: 10.4161/psb.25198
Rorat, T., Szabala, B. M., Grygorowicz, W. J., Wojtowicz, B., Yin, Z., and Rey, P. (2006). Expression of SK3-type dehydrin in transporting organs is associated with cold acclimation in Solanum species. Planta 224, 205–221. doi: 10.1007/s00425-005-0200-1
Rosner, S., Johnson, D. M., Voggeneder, K., and Domec, J. C. (2019). The conifer-curve: fast prediction of hydraulic conductivity loss and vulnerability to cavitation. Ann. For. Sci. 76:82. doi: 10.1007/s13595-019-0868-1
Rui, Q., Tan, X., Liu, F., Li, Y., Liu, X., Li, B., et al. (2021). Syntaxin of plants31 (SYP31) and SYP32 is essential for Golgi morphology maintenance and pollen development. Plant Physiol. 186, 330–343. doi: 10.1093/plphys/kiab049
Rui, Q., Wang, J., Li, Y., Tan, X., and Bao, Y. (2020). Arabidopsis COG6 is essential for pollen tube growth and Golgi structure maintenance. Biochem. Biophys. Res. Commun. 528, 447–452. doi: 10.1016/j.bbrc.2020.05.189
Schmittgen, T. D., and Zakrajsek, B. A. (2000). Effect of experimental treatment on housekeeping gene expression: validation by real-time, quantitative RT-PCR. J. Biochem. Biophys. Methods 46, 69–81. doi: 10.1016/S0165-022X(00)00129-9
Sen, M. K., Hamouzová, K., Košnarová, P., Roy, A., and Soukup, J. (2021). Identification of the most suitable reference gene for gene expression studies with development and abiotic stress response in Bromus sterilis. Sci. Rep. 11:13393. doi: 10.1038/s41598-021-92780-1
Silveira, É. D., Alves-Ferreira, M., Guimarães, L. A., da Silva, F. R., and Carneiro, V. T. D. C. (2009). Selection of reference genes for quantitative real-time PCR expression studies in the apomictic and sexual grass Brachiaria brizantha. BMC Plant Biol. 9:84. doi: 10.1186/1471-2229-9-84
Silver, N., Best, S., Jiang, J., and Thein, S. L. (2006). Selection of housekeeping genes for gene expression studies in human reticulocytes using real-time PCR. BMC Mol. Biol. 7:33. doi: 10.1186/1471-2199-7-33
Singh, V. V., Naseer, A., Mogilicherla, K., Trubin, A., Zabihi, K., Roy, A., et al. (2024a). Understanding bark beetle outbreaks: exploring the impact of changing temperature regimes, droughts, forest structure, and prospects for future forest pest management. Rev. Environ. Sci. Biotechnol. 23, 257–290. doi: 10.1007/s11157-024-09692-5
Singh, V. V., Naseer, A., Sellamuthu, G., and Jakuš, R. (2024b). An optimized and cost-effective RNA extraction method for secondary metabolite-enriched tissues of Norway spruce (Picea abies). Plants 13:389. doi: 10.3390/plants13030389
Singh, V. V., Zabihi, K., Trubin, A., Cudlín, P., Korolyova, N., Jakuš, R., et al. (2023). Effect of diurnal solar radiation regime and tree density on sap flow of Norway spruce (Picea abies [L.] karst.) in fragmented stands. Preprint. Available online: https://www.researchsquare.com/article/rs-3262723/v1
Stival Sena, J., Giguère, I., Rigault, P., Bousquet, J., and Mackay, J. (2018). Expansion of the dehydrin gene family in the Pinaceae is associated with considerable structural diversity and drought-responsive expression. Tree Physiol. 38, 442–456. doi: 10.1093/treephys/tpx125
Sun, Z., Li, S., Chen, W., Zhang, J., Zhang, L., Sun, W., et al. (2021). Plant dehydrins: expression, regulatory networks, and protective roles in plants challenged by abiotic stress. Int. J. Mol. Sci. 22:12619. doi: 10.3390/ijms222312619
Takamori, L. M., Pereira, A. V. C., Maia Souza, G., Vieira, L. G. E., and Ferreira Ribas, A. (2017). Identification of endogenous reference genes for RT-qPCR expression analysis in Urochloa brizantha under abiotic stresses. Sci. Rep. 7:8502. doi: 10.1038/s41598-017-09156-7
Ungar, D., Oka, T., Brittle, E. E., Vasile, E., Lupashin, V. V., Chatterton, J. E., et al. (2002). Characterization of a mammalian Golgi-localized protein complex, COG, that is required for normal Golgi morphology and function. J. Cell Biol. 157, 405–415. doi: 10.1083/jcb.200202016
Vandesompele, J., De Preter, K., Pattyn, F., Poppe, B., Van Roy, N., De Paepe, A., et al. (2002). Accurate normalization of real-time quantitative RT-PCR data by geometric averaging of multiple internal control genes. Genome Biol. 3, RESEARCH0034–RESEARCH0012. doi: 10.1186/gb-2002-3-7-research0034
Vestman, D., Larsson, E., Uddenberg, D., Cairney, J., Clapham, D., Sundberg, E., et al. (2011). Important processes during differentiation and early development of somatic embryos of Norway spruce as revealed by changes in global gene expression. Tree Genet. Genomes 7, 347–362. doi: 10.1007/s11295-010-0336-4
Vijayakumar, S., and Sakuntala, M. (2024). Validation of reference gene stability for normalization of RT-qPCR in Phytophthora capsici Leonian during its interaction with Piper nigrum L. Sci. Rep. 14:7331. doi: 10.1038/s41598-024-58139-y
Vukašinović, N., Oda, Y., Pejchar, P., Synek, L., Pečenková, T., Rawat, A., et al. (2017). Microtubule-dependent targeting of the exocyst complex is necessary for xylem development in Arabidopsis. New Phytol. 213, 1052–1067. doi: 10.1111/nph.14267
Wan, H., Yuan, W., Ruan, M., Ye, Q., Wang, R., Li, Z., et al. (2011). Identification of reference genes for reverse transcription quantitative real-time PCR normalization in pepper (Capsicum annuum L.). Biochem. Biophys. Res. Commun. 416, 24–30. doi: 10.1016/j.bbrc.2011.10.105
Wang, H. L., Chen, J., Tian, Q., Wang, S., Xia, X., and Yin, W. (2014). Identification and validation of reference genes for Populus euphratica gene expression analysis during abiotic stresses by quantitative real-time PCR. Physiol. Plant. 152, 529–545. doi: 10.1111/ppl.12206
Wang, W., Hu, S., Cao, Y., Chen, R., Wang, Z., and Cao, X. (2021). Selection and evaluation of reference genes for qRT-PCR of Scutellaria baicalensis Georgi under different experimental conditions. Mol. Biol. Rep. 48, 1115–1126. doi: 10.1007/s11033-021-06153-y
Wise, R. P., Moscou, M. J., Bogdanove, A. J., and Whitham, S. A. (2007). Transcript profiling in host-pathogen interactions. Annu. Rev. Phytopathol. 45, 329–369. doi: 10.1146/annurev.phyto.45.011107.143944
Yang, Z., Zhou, Y., Wang, X., Gu, S., Yu, J., Liang, G., et al. (2008). Genomewide comparative phylogenetic and molecular evolutionary analysis of tubby-like protein family in Arabidopsis, rice, and poplar. Genomics 92, 246–253. doi: 10.1016/j.ygeno.2008.06.001
Keywords: RT–qPCR, housekeeping genes/reference genes, gene expression, developmental stages and tissues, abiotic and biotic stress
Citation: Singh VV, Naseer A, Sellamuthu G, Mogilicherla K, Gebauer R, Roy A and Jakuš R (2024) Robust reference gene selection in Norway spruce: essential for real-time quantitative PCR across different tissue, stress and developmental conditions. Front. For. Glob. Change. 7:1458554. doi: 10.3389/ffgc.2024.1458554
Edited by:
Louis Bernier, Laval University, CanadaReviewed by:
Laurel Haavik, Southern Research Station, Forest Service (USDA), United StatesDavid Lázaro Gimeno, University of Helsinki, Finland
Copyright © 2024 Singh, Naseer, Sellamuthu, Mogilicherla, Gebauer, Roy and Jakuš. This is an open-access article distributed under the terms of the Creative Commons Attribution License (CC BY). The use, distribution or reproduction in other forums is permitted, provided the original author(s) and the copyright owner(s) are credited and that the original publication in this journal is cited, in accordance with accepted academic practice. No use, distribution or reproduction is permitted which does not comply with these terms.
*Correspondence: Vivek Vikram Singh, c2luZ2h2QGZsZC5jenUuY3o=