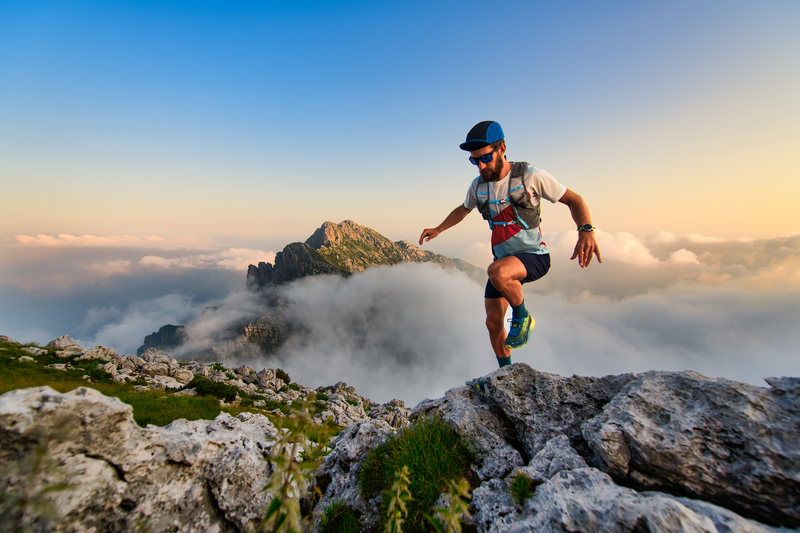
94% of researchers rate our articles as excellent or good
Learn more about the work of our research integrity team to safeguard the quality of each article we publish.
Find out more
ORIGINAL RESEARCH article
Front. For. Glob. Change , 20 November 2024
Sec. Forest Management
Volume 7 - 2024 | https://doi.org/10.3389/ffgc.2024.1449340
This article is part of the Research Topic Forest Assisted Migration View all 12 articles
Introduction: Global climate change and associated stressors threaten forest ecosystems due to the rapid pace of climate change, which could exceed the natural migration rate of some tree species. In response, there is growing interest to research and implement forest assisted migration (FAM). Here, we used a species-independent indicator based on climate analogy, according to the sigma (dis)similarity (σd) index, to match planting sites across the eastern US with (future) climatically-compatible seedlots (CCS).
Methods: We developed CCS for a grid composed of 1 × 1° of latitude and longitude. CCS were based on future climate analogs with ≤2σd analogy to ensure CCS were representative of future climate change. CCS were located for three time periods, 2030's, 2050's, and 2090's and three emissions scenarios (SSP2-4.5, SSP3-7.0, and SSP5-8.5) from the Coupled Model Intercomparison Project phase 6 database, using 12 climate variables.
Results: CCS were identified for the majority of 1 × 1° grids based on the SSP3-7.0 scenario. Approximately 28% of 1 × 1° grid's 2090's projections included future climate novelty. The 2030's, 2050's, and 2090's CCS were located on average 222, 358, and 662 km or 1, 2, and 3 eastern seed zones away from the 1 × 1° grids, respectively. CCS were also located further south-southwest (188–197°). In addition, the average forest cover of CCS was approximately 2%, 5%, and 10% less than that of the 1 × 1° grids.
Discussion: Our development and synthesis of CCS emphasized four key results: (i) average distances to 2030's and 2050's CCS were similar to seed-transfer guidelines for some tree species, but 2090's CCS exceeded current recommendations; (ii) south-southwesterly locations of CCS aligned with tree species habitat distribution dynamics; (iii) future climate novelty potentially challenges the conceptual basis of FAM if tree species are not adapted to climate change; and (iv) variation in forest cover among CCS presents potential opportunities and challenges due the presence or absence of forestland to source seed. Ultimately, our goal was to locate and synthesize CCS that could enable FAM decision support.
Global climate change and associated stressors represent some of the greatest challenges facing forest ecosystems today (Parmesan et al., 2022; Domke et al., 2023). This is exemplified by the rapid pace of climate change, which could exceed the natural migration rate of some species, leading to maladaptation and ecological and economic losses (Aitken et al., 2008; Mckenney et al., 2011; Price et al., 2013; Etterson et al., 2020; Prasad et al., 2020; Bisbing et al., 2021). One adaptation strategy increasingly discussed is Forest Assisted Migration (FAM), usually as a mechanism to accelerate natural species or genotype migration to compensate for anticipated ecological, economic, and cultural losses (Millar et al., 2007; Pedlar et al., 2012; Williams and Dumroese, 2013; Nagel et al., 2017; Palik et al., 2022). The emerging consensus is that as forest vulnerability to climate change increases, FAM will likely become an increasingly important strategy in forest conservation and management.
The anticipated prevalence of climate-driven migration lags and variety of FAM forms can be illustrated through the United States Forest Service (USFS) Climate Change Tree Atlas (https://www.fs.usda.gov/nrs/atlas/tree/) (Iverson et al., 2019a,b). The Tree Atlas quantifies potential distribution dynamics of current and projected future habitat of 125 eastern US tree species. For example, within the Northeast region, 65 of these species are expected to experience regional habitat increases by 2100 (under a very high emissions scenario), of which 24 are non-endemic to the Northeast and only seven may naturally migrate into the region by 2100, despite expected habitat outfilling. Specifically, whether tree species or genotypes are sourced and transplanted within their current range, just outside their range, or far from their range refers to assisted population expansion, assisted range expansion, or assisted species migration, respectively—exemplifying different levels of FAM intensity, endemism, and associated risk. However, practitioners in the eastern US lack the resources and confidence identifying the precise optimal seed sources for these species—a topic described in recent FAM literature (Park and Talbot, 2018; Palik et al., 2022; Royo et al., 2023)—because resources such as the Tree Atlas do not currently account for intraspecies variation (Leites et al., 2019; Prasad and Leites, 2022) and guidelines for selecting the best genetic sources are unavailable for most species (Pike et al., 2020), including recently developed universal response functions that integrate both genetic and environmental information (Wang et al., 2010; Chakraborty et al., 2015).
Implementing FAM inherently involves a degree of risk due to many interacting factors (Dumroese et al., 2015; Park and Talbot, 2018). Perceived short- and long-term challenges with respect to local vs. non-local seed source optimality to potentially novel environmental and changing climate conditions threaten the potential success of FAM. From a climate standpoint, local seed sources are expected to progressively respond negatively to intensifying climate change. On the other hand, non-local seed sources identified for their future adaptation are expected to increasingly respond favorably to climate change. However, non-local seed sources must survive current climate conditions in which they may be less adapted due to phenological mismatches. In addition to short-term responses to climate, non-local seed sources must also adapt to potentially novel plant-soil feedbacks (Refsland et al., 2023), browsing pressure (Champagne et al., 2021a), and moisture regimes (Champagne et al., 2021b) that local seed sources may be better adapted. Thus, early seedling survival is key to FAM success (Corlett and Westcott, 2013). Provenance tests have historically been critical to providing information on short-term acclimatization of non-local seed sources and the development of seed transfer distances for a select number of tree species. Recent provenance trials and early results from FAM studies have shown that non-local seed sources can be successful and even outperform local seed sources during this crucial period (Lu et al., 2014; Palik et al., 2022). Hence, identifying appropriate seed sources is key to developing practical knowledge on how to guide FAM efforts.
Researchers have instead developed generalizable, sometimes species-independent, approaches to identifying appropriate seed sources or seed-transfer guidelines when optimum species or genetic information is unavailable. For example, tree seed zones may be based on climatic thresholds related to drought or cold hardiness (Bower et al., 2014; Erickson and Halford, 2020; Pike et al., 2020). The US Eastern Seed Zone Forum, for example, identifies current eastern seed zones as a combination of cold hardiness zones and ecoregions that are intended to delineate key adaptive responses in which native seed may be collected and planted without deleterious effects due to phenological mismatches (http://www.easternseedzones.com/) (Pike et al., 2020). To facilitate FAM when species or genotypes have not been field tested or when genetic information is unavailable, the Seed Zone Forum recommends seed transfers within or between adjacent seed zones, i.e., ~1–2 seed zones, to enable adaptation to future climate change as a general rule (Pike et al., 2020). Transfer distances >2 seed zones, however, are thought to pose risk of genetic maladaptation, especially in the near term before the climate has changed in favor of individuals from warmer regions. Nonetheless, these recommendations recognize that accelerating climate change may warrant transfers exceeding conservative movements between adjacent seed zones (Pike et al., 2020).
Alternatively, researchers may identify optimal seed sources using climate distances between two points in a fixed area, in which seed may be sourced from locations where climates are most similar, i.e., climatic distances are minimized (Doherty et al., 2017; Shryock et al., 2018; St.Clair et al., 2022; Royo et al., 2023). As opposed to ad hoc migration prescriptions, which may be comprised of indiscriminately-sourced seed from more southern locations, climate-distance approaches offer a more geographically- and thus climatically-precise approach that researchers are increasingly advocating (Young et al., 2020; Royo et al., 2023). In addition, collections across fixed seed zones may be compromised if zones are too large, increasing maladaptation across large distances, vs. too small, necessitating unwarranted or excessive collections (O'Neill et al., 2014). Similarly, more climatically-precise methods in the eastern US are expected to better capture a greater range (besides temperature-related variables) of key climatic responses, e.g., moisture, in the adaptive profile of selected seed sources, such that climatically-compatible seedlots may also be located further west or east, e.g., drier or wetter, of a planting site, as observed in the distribution dynamics of tree species habitat in the Tree Atlas (Iverson et al., 2019a).
Here, we used a standardized climate-distance and species-independent index, sigma (dis)similarity (σd) (Mahony et al., 2017), to identify climate analogs—contemporary locations with climates similar to the anticipated future climate of a planting site (Williams et al., 2007; Grenier et al., 2013). We refer to these new locations as (future) climatically-compatible seedlots (CCS). The general premise of this approach is that CCS located within future climate analogs are expected to better minimize risk and maximize seedling adaptation potential because they originated from similar climates to the planting site's anticipated future climate (Young et al., 2020; St.Clair et al., 2022; Royo et al., 2023). Thus, the expectation is that they are pre-adapted to the anticipated future climate of a planting site. Climate analog modeling within a FAM context is growing in popularity. However, broad syntheses for regions such as the eastern US are lacking. The eastern US is an ideal candidate for such a synthesis because it contains some of the most forested regions in the US, coincides with existing adaptive resources, i.e., Tree Atlas and eastern seed zones, and is where FAM is actively being used in support of climate-adaptive forestry (Nagel et al., 2017; Palik et al., 2022).
Climate analogy and σd also provides a basis for the identification of future climate novelty. Novel climates are emerging conditions with no analog in the observational record. Future climate novelty could challenge the conceptual basis of FAM because FAM is partially dependent on the premise that successful seedling establishment of future-climate-adapted tree species or genotypes is based on the complementarity of their fundamental niche to the anticipated future climate space (Williams and Jackson, 2007). However, if no reference conditions exist for a future climate, FAM may be less reliable due to climate change exceeding the adaptive potential of prospective seed sources. Some estimates suggest 10%−40% of Earth's terrestrial surface, including portions of the eastern US, are expected to experience climate novelty by 2100 (Mahony et al., 2017). Identifying the emergence and locations at risk of developing future climate novelty may be an important consideration in FAM adaptive planning and risk assessment.
Our objectives were to use climate analogy according to σd to quantify potential CCS for the eastern US and provide a broad synthesis on CCS patterns that may enable FAM decision support. We used a grid of 1 × 1° latitude and longitude as our frame of reference. Our work synthesizes geographic patterns in CCS: (i) climate analogy of CCS, i.e., (dis)similarity of future climates; (ii) distance to CCS (both in geographic distance and minimum number of seed zones and plant hardiness zones between CCS and planting sites); (iii) direction to CCS (as degrees bearing); (iv) emergence of future climate novelty; and (v) variation in forest cover between 1 × 1° grid planting sites and CCS as an examination of potential socio-environmental opportunities or challenges due to the presence or absence of forestlands at desired, i.e., most-climatically matched, locations. We used climate models based on 2030's, i.e., current (2021–2040), 2050's, i.e., mid-century (2041–2060), and 2090's, i.e., late-century (2081–2100) periods, according to three emissions scenarios, SSP2-4.5, SSP3-7.0, and SSP5-8.5, derived from the Coupled Model Intercomparison Project phase 6 (CMIP6) database (IPCC, 2022).
We quantified CCS for a series of 494, 1 × 1° grids located in the eastern US (east of the 100th Meridian) developed as part of the USFS Climate Change Tree Atlas (Figure 1a). The geographic footprint provided a coarsely-summarized continuous grid of representative sites permitting sufficient climate observations from historical weather stations. In addition, the 1 × 1° grid focus complements the Tree Atlas, delivering a single information point enabling FAM decision support. Forest cover averaged 31.21 ± 2.14% (±95% CI) and ranged from 0.03 to 86.25% across the grid (NALC, 2020). CCS were located within a larger study domain encompassing the extent of the western hemisphere north of the equator as defined by the input climate data. The region was also partitioned into five geographic regions and 92 eastern US seed zones to assist in our synthesis (Iverson et al., 2008; Pike et al., 2020) (Figures 1b, c).
Figure 1. The eastern US 1 × 1° grid where we located (future) climatically-compatible seedlots (CCS) (a), including five geographic regions (b) and 92 eastern US seed zones (c). Note 1 × 1° grids are referenced by their southeastern corner, i.e., a coordinate of 45.89, −88.46 occurs in the S45_E88 1 × 1° grid.
We used climate analogy according to σd to locate CCS for each 1 × 1° grid (Mahony et al., 2017). This approach produces σd surfaces that depict geographic areas where current climate conditions are most similar, i.e., analogous, to each 1 × 1° grid's forecasted climate. σd is derived from standardized Mahalanobis distances and interpreted as a multivariate z-score metric (Mahony et al., 2017; Fitzpatrick and Dunn, 2019). The use of Mahalanobis distances reduces limitations in variable scaling, e.g., °C vs. mm, and variance inflation due to correlations. Mahalanobis distances were standardized with respect to local 1 × 1° grid climate variability and scaled according to the half-normal percentile of the associated chi distribution, where n df equaled the number of dimensions, i.e., climate variables, and 1σd, 2σd, and 4σd corresponded to the 68th, 95th, and 99.9th normal percentiles, respectively. A 0σd indicates identical climates, i.e., a 1 × 1° grid's future climate and its closest-matched contemporary climate analog are a perfect match. σd is both a measure of the strength of climate analogy and an indicator of future climate novelty. Hence, σd may serve as a proxy for seedling adaptation potential to future climate change, i.e., where analogy is strongest (σd lowest) seedling adaptation, e.g., survival, productivity, reproduction, may be greatest. Thus, we defined an upper threshold of 2σd (95th percentile), in which we confidently assigned CCS to each 1 × 1° grid's σd surface. This is because values >2σd generally indicate future climate novelty (Mahony et al., 2017). In other words, values >2σd indicate a 1 × 1° grid's future climate is dissimilar to any contemporary climate in the study domain, which we interpret as there being no CCS for that 1 × 1° grid's anticipated future climate.
We used 12 climate variables and three datasets to map σd values and locate CCS for each 1 × 1° grid. The 12 climate variables consisted of mean minimum and maximum temperature (°C) and total precipitation (mm) for each of the four climatological seasons; winter (December, January, and February), spring (March, April, and May), summer (June, July, and August), and autumn (September, October, and November). The variables were selected because we considered them to represent fundamental elements of future climate change and because they have been supported in previous climate analog mapping applications (Mahony et al., 2017; Fitzpatrick and Dunn, 2019).
The first dataset was a contemporary climate surface [A] that we converted into the σd surfaces, serving as the regional climate pool and potential CCS. We used gridded 1-km resolution climate data for North America provided by the AdaptWest Project and generated using ClimateNA v7.3 software (AdaptWestProject, 2022). The geographic extent of [A] defined the larger study domain across North America. These data were based on downscaled PRISM (PRISMClimateGroup, 2022) and WordClim data (Fick and Hijmans, 2017), estimated over a 30-year period from 1981–2010.
The second group of datasets were projections of each 1 × 1° grid's future climate [B], we spatially related to [A]. Future climate data in [B] were averaged within the extent of each 1 × 1° grid for the 12 climate variables. We used climate models derived from the Coupled Model Intercomparison Project phase 6 (CMIP6) database (corresponding to the 6th IPCC Assessment Report) (IPCC, 2022) and the 8-model general-purpose ensemble selected by Mahony et al. (2022). The raster surfaces were provided by the AdaptWest Project and included ensemble-mean projections from models ACCESS-ESM1.5, CNRM-ESM2-1, EC-Earth3, GFDL-ESM4, GISS-E2-1-G, MIROC6, MPI-ESM1.2-HR, and MRI-ESM2.0 (see AdaptWestProject, 2022, for details on these projections).
Future climate projections were provided in 20-year averages for the time periods of 2021–2040, 2041–2060, and 2081–2100 to accommodate a spectrum of climate-adaptation options in [B]. We refer to these temporal projections as enabling “adaptation” to 2030's, 2050's, and 2090's predicted climates or current, mid-century, and late-century, respectively. We also included Shared Socioeconomic Pathways, SSP2-4.5 (intermediate warming limited to < 3°C), SSP3-7.0 (high warming limited to < 4°C), and SSP5-8.5 (very high warming exceeding >4°C), to assess a range of emissions scenarios. We focused our results on SSP3-7.0 here as our objectives were to provide a general pattern of CCS representative of potential challenges for FAM, while acknowledging the likelihood of a given scenario is challenging (Hausfather and Peters, 2020; Schwalm et al., 2020; Hausfather et al., 2022). In addition, we found similarities in the geographic locations of CCS across emissions scenarios, e.g., 72% of all 2050's CCS were located < 100 km from one another across SSP2-4.5, SSP3-7.0, and SSP5-8.5 (though these similarities declined in 2090's projections). We provided results for all three emissions scenarios in the Supplementary material, using tables and interactive web maps created with the leaflet R package (Cheng et al., 2024).
The third and final dataset was developed from NOAA weather station records to estimate historical interannual climate variability [C] within each 1 × 1° grid. σd standardizes climatic distances with respect to historical climate data. We estimated [C] for the 12 climate variables using the Global Summary of the Month (GSOM) dataset (Lawrimore et al., 2016). The GSOM dataset contains meteorological events at monthly resolution from 1763, composed primarily of daily Global Historical Climatology Network observations. We located all weather stations within each 1 × 1° grid that contained ≥20 years of observations over a reference period of 1961–2010 using the rnoaa R package (Chamberlain, 2020). We used the 50-year reference period and required a minimum of five unique weather stations per 1 × 1° grid to estimate [C] in order to ensure sufficient climate observations for calculating σd. Station records were averaged using inverse distance weighting to the center of each 1 × 1° grid and monthly records were aggregated to the four climatological seasons to match the climate data in [A] and [B]. For 1 × 1° grids (mostly located along coastlines with limited land area) without five weather stations or ≤ 20 years of observations, we searched outwards from the cell's centroid incorporating the nearest five weather stations with these criteria into the analyses, similarly using inverse distance weighting to calculate the 12 climate variables.
We located CCS for each 1 × 1° grid by converting [A] into surfaces of σd using future climate projections in [B] and scaling climatic distances with respect to local historical climate variability in [C]. All analyses were conducted in R (https://www.r-project.org/) and we adapted methods and R scripts provided in Mahony et al. (2017). We considered CCS to include ≤ 2σd analogy between [A] and [B] (in each 1 × 1° grid). We summarized the strength of analogy (or novelty), i.e., σd value, distance (km), and bearing (°) to the pixel with the least σd value. We provided summaries for 37 eastern US states and five geographic regions. The 1 × 1° grid cells were assigned to individual states, regions, seed zones, and plant hardiness zones using majority rule, i.e., the geographic feature with the greatest areal extent within a 1 × 1° grid was used. We used eastern seed zones version 2.2 and plant hardiness zones version 2023 (Pike et al., 2020; USDA Agricultural Research Service, 2023). The granularity and resolution of these maps can lead to over- or under-counting zones (from 1 × 1° grid to CCS) if they are spatially-disjoined and/or overly-small. For our purposes, we snapped the original maps to US counties to force small, spatially-disjoined sections of zones to be absorbed into larger zones (particularly in the case of plant hardiness zones) or be separated into individual zones (eastern seed zones). Finally, we estimated percent forest cover amount within each 1 × 1° grid and the landscape surrounding each CCS within a 50-km buffer radius (an area roughly similar in size to the average 1 × 1° grid). Forest cover was estimated with the 2020 North American Land Cover dataset provided in 30 m resolution, based on Landsat satellite imagery of Canada, Mexico, and the US (NALC, 2020).
We located CCS ≤ 2σd analogy for all (n = 494) of the 1 × 1° grids for the 2030's, i.e., current (2021–2040) period, all but one (n = 493) of the 1 × 1° grids for the 2050's, i.e., mid-century (2041–2060) period, and 356 (~72%) of the 1 × 1° grids for the 2090's, i.e., late-century (2081–2100) period, under the SSP3-7.0 emissions scenario (Figure 2, Table 1). In other words, 28% of 1 × 1° grids displayed emerging climate novelty under 2090's climate projections. Supplementary Tables S1–S9 and interactive web maps (Supplementary Figures S1–S3) summarize results for all three emissions scenarios (SSP2-4.5, SSP3-7.0, and SSP5-8.5). The fewer number of 2090's CCS resulted from accentuated >2σd novelty emerging throughout the southern portion of the eastern US, attributed to accelerated climate change and deficient land area further south, e.g., the Gulf of Mexico, to draw representative analogs.
Figure 2. Locations of (future) climatically-compatible seedlots (CCS) ≤2σd analogy for the eastern US 1 × 1° grid under the SSP3-7.0 emissions scenario and three adaptation planning periods: 2030's (2021–2040) (a), 2050's (2041–2060) (b), and 2090's (2081–2100) (c). Arrows point each 1 × 1° grid's CCS, omitting >2σd novelty (note only 50% of CCS in each period are shown for display purposes). The analogy (or novelty) of each 1 × 1° grid's future climate projection to its CCS is shown in color on each arrow and in inset maps (1 × 1° grids >2σd novelty are displayed in white color).
Table 1. Regional and US state-level statistics of (future) climatically-compatible seedlots (CCS) ≤2σd analogy for the three adaptation periods, 2030's (2021–2040), 2050's (2041–2060), and 2090's (2081–2100), and SSP3-7.0 emissions scenario, including the number (n) of CCS, i.e., 1 × 1° grids with CCS, and the mean (±95% CI) σd value, distance to CCS (km), bearing to CCS (°), number of seed zones from 1 × 1° grid to CCS, and difference (Δ) in forest cover (%) between 1 × 1° grid and CCS (note, only CCS located within established eastern US seed zones could be used in calculating the number of seed zones to CCS, generally excluding CCS west of the 100th Meridian).
The σd value, as a potential measure of CCS adaptation to climate change, was on average 0.03 ± 0.01 (±95% CI) σd (~2nd percentile) for the 2030's, 0.20 ± 0.03 σd (~16th percentile) for the 2050's, and 0.71 ± 0.06 σd (~52nd percentile) for the 2090's periods (noting that 0σd is a perfect match, ≤ 2σd is up to a moderate level of (dis)similarity, and >2σd is indicative of future climate novelty) (Mahony et al., 2017) (Table 1). Altogether, the total area representing ≤ 2σd analogy for each CCS was on average 451-, 351-, and 177-thousand km2 for the 2030's, 2050's, and 2090's planning periods, respectively (Supplementary Tables S2, S5, S8).
The geographic distance to CCS (from 1 × 1° grid planting cell's centroid to the pixel with the lowest σd value) was on average 222 ± 12 km for the 2030's, 358 ± 20 km for the 2050's, and 662 ± 35 km for the 2090's adaptation planning periods (Table 1). Regionally, 1 × 1° grids located in the Southeast and South Central regions generally displayed the greatest distances to CCS across the three adaptation periods, e.g., 353 ± 30 and 408 ± 87 for 2050's South Central and Southeast CCS, respectively, whereas 1 × 1° grids of the Great Plains region largely displayed the smallest distances to CCS, e.g., 293 ± 25 for 2050's CCS (Table 1).
Geographic distances to CCS, on average, roughly corresponded to 1, 2, and 3 seed zones (specifically 1.19 ± 0.09, 1.71 ± 0.10, and 2.94 ± 0.13 seed zones) from the 1 × 1° grid's native seed zone to the seed zone of its CCS for the 2030's, 2050's, and 2090's periods, respectively (Table 1). The distance to CCS also averaged 1–2 plant hardiness zones (specifically 0.92 ± 0.08, 1.27 ± 0.08, and 2.24 ± 0.10 plant hardiness zones) for the 2030's, 2050's, and 2090's periods, respectively (Supplementary Tables S2, S5, S8). In addition, CCS were located on average 2.18 ± 0.15, 3.41 ± 0.21, and 6.05 ± 0.39 1 × 1° grid cells from the 1 × 1° grid planting site for the 2030's, 2050's, and 2090's periods, respectively (Supplementary Tables S2, S5, S8). The CCS were also located 0.73 ± 0.07, 1.02 ± 0.08, and 1.83 ± 0.11 US states from the 1 × 1° grid planting site (based on majority feature) for the 2030's, 2050's, and 2090's periods, respectively (Supplementary Tables S2, S5, S8). This is important to note if statutes preclude the selling or procuring of seedlings from other jurisdictions, i.e., non-local sources.
The bearing (representing the angle in degrees measured clockwise from north) to CCS was on average 191 ± 2°, indicating that CCS were generally located south-southwest of each 1 × 1° grid, with an increasing tendency for more southwesterly locations over extended planning periods, e.g., 2090's (Table 1). Specifically, CCS were located on average 188 ± 4°, 189 ± 4°, and 197 ± 4° from each 1 × 1° grid's centroid for the 2030's, 2050's, and 2090's periods, respectively. Some regional patterns were also apparent; CCS (across 2030's, 2050's, and 2090's projections) for the North Central region were located on average 184° to 190° to the southwest of each 1 × 1° grid, whereas CCS of the Northeast averaged 182° to 203°.
The amount of CCS forest cover (based on % forest within a 50-km buffer radius) was on average −2.27 ± 1.31%, −4.77 ± 1.51%, and −9.56 ± 2.16% less than that of their associated 1 × 1° grid for the 2030s, 2050's, and 2090's periods, respectively (Table 1, Figure 3). The Northeast region displayed the greatest average difference in forest cover amount between 1 × 1° grids and CCS, e.g., −17.86 ± 4.13 for 2090's CCS. In contrast, the Great Plains region displayed the smallest average difference in forest cover amount between 1 × 1° grids and CCS, e.g., 0.48 ± 1.23 for 2090's CCS.
Figure 3. Color composite visualizing variation in forest cover (%) difference (ΔForest cover) between eastern US 1 × 1° grids and their (future) climatically-compatible seedlots (CCS) for the 2030's (2021–2040) (a), 2050's (2041–2060) (b), and 2090's (2081–2100) (c) periods. Forest cover values were transformed into channels of color in which 1 × 1° grid forest cover represented the green axis, CCS forest cover the blue axis, and a default value of 0 was set for the red axis. We provide seven examples for the 2090's period. Examples 1–2 (located in the greener color space) highlight potential challenges due the absence of forest cover (to source seed) at CCS (y axis in legend) relative to 1 × 1° grid (x axis). Examples 3–4 highlight dramatic differences in forest cover but still including CCS with >30% forest cover. Example 5 is neutral (1 × 1° grid and CCS both including >50% forest cover). Finally, examples 6–7 (in bluer color space) highlight potential opportunities for some 1 × 1° grids to source from regions with greater forest cover. Note 1 × 1° grids >2σd novelty are not displayed.
The majority of CCS (~96%−98%) ≤ 2σd analogy across the three adaptation periods were located within the eastern US (east of the 100th Meridian), whereas non-representative analogs (>2σd novelty) were more often located outside the eastern US (west of the 100th Meridian, including Mexico) (Figure 2); some of this is attributed to anticipated >2σd novelty observed in the southern US. CCS among the 2030's and 2050's periods were also generally located within the same geographic region as the 1 × 1° grid in which they represent, e.g., 75 and 70% of the Northeast region's CCS for the 2030's and 2050's planning periods were located within the Northeast, respectively (Figures 4a, b). However, only 54% of the Northeast region's 2090's CCS remained in the Northeast (Figure 4c). The majority (~30%) of the Northeast region's other 2090's CCS were located in the South Central region. This pattern is apparent for much of the 1 × 1° grid, especially in the Northeast and North Central regions, i.e., USFS Region 9. The South Central region is the only region to host CCS for all other geographic regions across each period, e.g., 37% of 2090's CCS (excluding the South Central region) were located within the South Central region (Figure 4c).
Figure 4. A flow diagram depicting the geographic region where 1 × 1° grids and their (future) climatically-compatible seedlots (CCS) are located under the SSP3-7.0 emissions scenario for the 2030's (2021–2040) (a), 2050's (2041–2060) (b), and 2090's (2081–2100) (c) periods. The size of the vertical bars represents the number of individual 1 × 1° grid cells (left) or CCS (right) and the width of the flows (left to right) indicates the number of connections.
There is a growing need to manage for climate-driven range shifts (Bonebrake et al., 2018). Novel strategies and frameworks aimed to conserve ecosystem integrity under climate change, including FAM, are being increasingly researched and implemented (Millar et al., 2007; Pedlar et al., 2012; Nagel et al., 2017; Palik et al., 2022; Royo et al., 2023). Challenges implementing FAM include identifying optimal seed sources and estimating seed-transfer guidelines when relevant genetic information is unavailable. However, due to the need to implement these strategies under a rapidly changing climate, researchers must develop fast and efficient approaches to guide targeted collections, such as with the use of climate data. Here, we used climate analogy according to σd, a species-independent and climate-distance approach, to locate CCS for the eastern US that may serve as a surrogate for genetic information. The goal of this work was to provide a broad synthesis of CCS patterns that may enable greater FAM decision support across the eastern US.
Average distances to CCS ≤ 2σd analogy were expectedly large (under the SSP3-7.0 emissions scenario), yet similar to maximum transfer distances (to avoid phenological mismatches) recommended for some species, as derived from provenance trials, common gardens, and other published resources (Pike and Haase, 2024). For example, maximum seed transfers of 100–200 miles (161–322 km) are commonly recommended for some species, e.g., sugar maple (Acer saccharum), and upwards of 300 miles (483 km) for others, e.g., red pine (Pinus resinosa), depending on the species (LaBonte, 2022; Pike and Bloese, 2022). These distances are similar to average distances to 2030's and 2050's CCS (222 and 358 km, respectively). Average distances across seed zones to 2030's and 2050's CCS (1 and 2 seed zones, respectively) were also similar to recommendations by the Eastern Seed Zone Forum (Pike et al., 2020). It is important to note that seed-transfer guidelines are different from CCS developed here—CCS show where to source seed and seed-transfer guidelines define the expected maximum distance seed may be moved before deleterious effects, which may help validate CCS or enable selection among species with least risk of maladaptation (see Tree Atlas section below).
In addition, recent studies in the eastern US show support for seed transfers and/or distances to assisted-migrated tree species' northern range termini of 100–200 km, including early survival results nearing 100% in one study (Muller et al., 2019). As a potential proxy to transfer distance, comparisons in survival among assisted population expansion vs. assisted range expansion plantings have also been examined, but results have been mixed (Muller et al., 2019; Palik et al., 2021, 2022; Clark et al., 2022)—likely because species vary in their tolerance to seed transfer (Leites et al., 2019). Nonetheless, these studies seemingly support seed transfers among CCS representative of adaptation to the next ~1.5–2 decades and ~200 km or 1–2 seed zones in distance, based on early survival results.
However, 2090's CCS average distances (662 km, ≥3 seed zones, and ≥2 plant hardiness zones) exceeded current seed transfer recommendations. It is important to note that geographic distance alone can sometimes be a poor correlate of adaptation to novel planting environments (Leimu and Fischer, 2008). Rather, it is the underlying differences in the abiotic, e.g., climate and soils, and biotic, e.g., competitors or pests or pathogens, environment that affects survival, which geographic distance may not always integrate because environmental gradients transition more gradually or sharply than expected, e.g., floristic tension zones between boreal and temperate forest types (Joyce and Rehfeldt, 2013). Some site-level differences may also be partially mediated by appropriately matching tree species to finer-scale habitat at the planting site (see below). Nonetheless, researchers are recognizing that more intense seed movements, strategies, and risk acceptance are needed to avoid the potential opportunity cost of FAM, which may include moving species or genotypes beyond current transfer recommendations—especially to enable adaptation to 2100 climate change, the risk of inaction may exceed FAM risks (Palik et al., 2022; Royo et al., 2023).
Second, CCS were located on average south-southwest of the 1 × 1° grid planting sites—a pattern generally resembling drier, continental climates. This is important because it likely associates with longitudinally-aligned traits influenced by precipitation, such that moving genotypes south-to-north may not necessarily minimize maladaptation nor maximize resiliency goals under FAM (Zhu et al., 2012; Fei et al., 2017; Gougherty et al., 2021). These subtle shifts in degrees bearing relative to orientation north can produce different patterns in CCS geographies relative to 1 × 1° grid origin, especially as distances increase. For example, a shift in bearing from 190° to 203° would result in the movement of a CCS by 150 km (or 143 km west and 42 km north) using an average geographic distance of 662 km. It is difficult to disentangle how specific climate features contribute to the joint distribution of (dis)similarity change. However, these results generally reflect the nature of climate change within the eastern US (Dobrowski et al., 2013). In addition, these results are similar to anticipated range shifts in the mean centers of suitable habitat of many eastern US tree species that are expected to shift in a northeast direction and originate from more southwestern locations (Iverson et al., 2019a).
Third, we also detected large areas of emergent climate novelty >2σd across the eastern US, particularly in the Southeast and South Central regions and for later-century projections, e.g., ~28% of 1 × 1° grids may experience climate novelty during the 2090's period. This is partially attributed to deficient land area further south at southern latitudes at the extent of terrestrial habitat along the Gulf of Mexico. In contrast, areas along the Appalachian range, e.g., state of Vermont in the Northeast, may be buffered from >2σd novelty due to increased topographic heterogeneity, enabling CCS to be drawn from lower elevations throughout the study domain. However, other regions may experience future climate novelty, including ~9% of 1 × 1° grids in the North Central region. The only region expected to not experience future climate novelty (at the 1 × 1° grid scale) under the SSP3-7.0 emissions scenario was the Northeast. It is important to note that results emphasized in our study were based on the high SSP3-7.0 emissions scenario, according to global warming trends limited to < 4°C. For the unmitigated, very-high SSP5-8.5 emissions scenario exceeding >4°C, 46% of 1 × 1° grids in the eastern US may experience future climate novelty (at the 1 × 1° grid scale) (Supplementary Table S9).
Future studies might examine the influence of climate novelty on FAM outcomes. We currently cannot predict how seed sources collected across a range of analogies, i.e., σd values, will respond to climate change. For example, what impacts (if any) to short- or long-term survival may occur if CCS with moderate levels of (dis)similarity, e.g., 0.5σd, vs. CCS with perfect alignment to climate change, i.e., 0σd, are used? Similarly, at the other end of the gradient and from a local climate change perspective, understanding tree species and genotype response across a range of σd values could assist in whether or not to pursue FAM and perhaps even FAM timing. In other words, it may be that local seed sources are better than non-local seed sources up to a minimum threshold, i.e., σdmin, in which local climate change has exceeded historical variability and the tolerance of local tree species and genotypes. This could function as a means to trigger FAM consideration and enhance confidence in non-local seed source establishment because the climate now aligns better with the CCS in which seed were sourced. It is important to note that even for the 2030's earliest planning period, the most closely-matched climate analogs and subsequent CCS were located 222 km on average from the 1 × 1° grid planting sites. Because of these distances, it likely indicates we have already met a minimum threshold to begin engaging in FAM practices, emphasizing a need to consider non-local seed sources in forest management planning sooner than later.
It is also possible that survival and productivity are less influenced by subtle differences in analogy, local climate departure, or even possibly moderate levels of climate novelty because slightly missing the optimal habitat of seed sources may not be that serious (Gray et al., 2011). In addition, some tree species show levels of plasticity that could suggest an ability to respond to climate novelty (Hamrick, 2004; Leimu and Fischer, 2008; Copenheaver et al., 2020). Short-term responses of seedlings sourced along gradients of (dis)similarity may be tested in common gardens, greenhouses, or other controlled environments (Bronson and Gower, 2010). Identifying key demographic parameters along these gradients could help (i) refine the selection of CCS, (ii) determine how much of the optimal climatic habitat may be missed (Gray et al., 2011), (iii) whether subtle ( ≤ 100 km) differences among emissions scenarios affect future adaptation potential (at least from a FAM decision standpoint in selecting among emissions scenarios to inform collections), and (iv) enable identification of population structures that facilitate climate change adaptation within species. Ultimately, from an operational standpoint, some level of moderate climate analog mismatch would need to be incorporated into the decision-making process due to the presence of seed sources and nursery practice (see below).
Fourth, we detected variation in forest cover amount among 1 × 1° grids and their CCS. Specifically, CCS were on average comprised of less (current) forest cover than that of the associated 1 × 1° grid. This is important because it may overestimate FAM confidence if seed sources are unavailable at CCS. In the provided examples, target 1 × 1° grids among the upper North Central region were comprised of 37 and 52% forest cover while their CCS were comprised of only 6 and 11% forest cover, respectively (Figure 3's examples 1–2). These differences were even more dramatic in other examples (3–4 highlight 1 × 1° grids comprised of >80% forest cover but with CCS comprised of at least 30%−50% forest cover) but neutral in another (example 5 highlights a 1 × 1° grid and CCS that were both comprised of 55% forest cover). Still, CCS may be located in landscapes with greater levels of forest cover (examples 6–7), presenting potential opportunities with increased area to locate seed sources. Our intention here was to highlight potential challenges and opportunities when identifying seed sources within CCS due to the presence or absence of forestlands, including the likelihood of missing ecologically, economically, or culturally important species.
The regional focus of our work was selected to coincide with the USFS Climate Change Tree Atlas. The CCS being located primarily within the eastern US and within the extent of the Tree Atlas allows for complementary information to be shared that either the Tree Atlas or CCS in this article do not provide individually (similar to seed-transfer guidelines). For example, the Tree Atlas predicts that post oak (Quercus stellata) and black hickory (Carya texana) may experience increasing habitat suitability within southeastern Ohio by 2100 (Peters et al., 2020). Here, CCS for this time period under the SSP3-7.0 emissions scenario were largely located across parts of the Ozarks in the state of Arkansas, also where these species occur likewise—though representing a large seed transfer of ~1,100 km. Hence, tree lists provided by the Tree Atlas support identification of future-climate-adapted tree species whereas CCS identify locations where these species may be sourced. Further refinement in FAM decision making may also incorporate seed-transfer guidelines (if available) or other information including seed zone or plant hardiness zone maps. In addition, the intersection of where within a species range climate analogs are located can provide more geographically-specific information to inform application of climate adaptation as well as a basic understanding to identify gradients of within species heterogeneity (Prasad and Potter, 2017; Gougherty et al., 2021).
Environmental data, particularly on soils, topography, and microbial communities and their interactions, are not currently incorporated directly into climate analog mapping, but these abiotic and biotic characteristics are known to impact the ability of tree species to adapt to new habitats (Leimu and Fischer, 2008; Lafleur et al., 2010; Refsland et al., 2023). Post-mapping environmental filters may be used to refine the selection of CCS where environmental conditions are agreeable to the planting site. In addition, FAM practitioners have the ability seek out suitable planting environments for specific tree species within the larger landscape, e.g., dry ridgetop vs. mesic bottomland (Iverson et al., 1997). Extreme weather events, particularly intense precipitation, that are expected to increase in frequency are also not easily incorporated into climate projections (Rastogi et al., 2020). Other challenges emerge if species currently occupy suboptimal habitat and are in climatic disequilibrium (Rehfeldt et al., 2018); this is particularly acute among species with narrow ranges (Seliger et al., 2021) and could affect FAM outcomes if species assemblages do not adequately reflect contemporary climate conditions. It is also unlikely to distinguish a species' pure climatic equilibrium because anthropogenic-climate forcing has been present throughout the observational record and tree species have been adapting to global change for a millennia (Davis, 1983; Hamrick, 2004; Prasad et al., 2024).
The selection of climate variables may also influence the location of CCS. However, our use of 12 seasonal climate variables, spanning a broad range of climate variation across the annual cycle, is likely to be applicable to most situations in a general way. In addition, these variables are fundamental, first-order variables that serve as the basis of more derived bioclimatic variables. Nonetheless, future work examining the effect of CCS analogy (or novelty) could also test a range of variables on seedling response because tree species are expected to respond independently to different climate features, including within-species variation (Joyce and Rehfeldt, 2013; Leites et al., 2019; Prasad and Leites, 2022). Species- or even population-specific CCS could be introduced through increased knowledge of inter- and intra-specific responses to different climate variables. In such a way, climate analog models could be tuned to the precise selection of species-relevant climate characteristics. While doing so may increase FAM confidence in enabling more species-specific CCS, the models do lose generality to be quickly applied across forest communities to rapidly enable fast and efficient seed collections. Still though, genetic information and field trials of provenance testing remain a critical step, including integration of genetic and environmental information into universal response functions (Wang et al., 2010; Chakraborty et al., 2015) to enhance basic understanding of physiological responses and inform management implementation. In addition, the coarse grain of the 1 × 1° grid likely masks fine-scale climate variation (within future climate projections) along topographic gradients or supplemental warming due to heat island effects. On the other hand, examining CCS at too a fine resolution could necessitate unwarranted or excessive collections (O'Neill et al., 2014), but may be useful in identifying terrain features or refugia that buffer against the most severe of anticipated impacts (Morelli et al., 2016; Stralberg et al., 2020). Our 1 × 1° grid focus ultimately balances some of these scale-based constraints by providing a discrete set 494 representative sites across the eastern US.
Climate-distance models offer a geographically- and thus climatically-precise approach to match CCS to planting sites. However, there is tension between current nursery practice and the desire to know the precise geographic origin of seed sources as this is not currently a common practice (Palik et al., 2022; Clark et al., 2023). In fact, it is unlikely that when the geographic origins of seed sources are known, it will match the precision of CCS in this study at 1 km resolution. Rather, climate-distance approaches may be treated as providing a general pattern in which CCS may be identified. The recent development of the eastern seed zone map is meant to in part define the origin of seed sources, i.e., “collections zones.” However, CCS may occur within smaller subsections of a seed zone, i.e., seed zones may be too large, or CCS may be distributed across more than one seed zone, providing opportunities to possibly blend these approaches in the future (see Supplementary Figures S1–S3). In addition to the challenges outlined throughout, nursery production and access is also broadly considered a potential key barrier to forest restoration and FAM operationalization (Fargione et al., 2021; Clark et al., 2023). It is also apparent that broad interregional cooperation is needed. For example, CCS were located on average ~1–2 US states away from the 1 × 1° grids across the three climate periods. As FAM increases, practitioners are expected to increasingly rely on CCS in other jurisdictions, potentially far from their region of comfort or within disallowed areas due to statutes that preclude selling seedlings from non-local sources. Of particular note is USFS Region 9's (North Central and Northeast) anticipated reliance on CCS located within Region 8 (Southeast and South Central).
Our development of (future) climatically-compatible seedlots (CCS) for the eastern US 1 × 1° grid and following synthesis emphasized four key results: (i) Average distances to 2030's and 2050's CCS were expectedly large, yet within range of maximum transfer distances recommended for some tree species. These distances also seemingly validate the Eastern Seed Zone Forum's recommendations of moving seed across 1–2 seed zones (as a general rule when species or genotype information is unavailable) to support adaptation to climate change (within this time frame). In contrast, average 2090's CCS' distances were larger than recommended, highlighting the magnitude of climate change and need for forest assisted migration (FAM) research that examines seed transfers this large. (ii) CCS were located south-southwest—not directly south—resembling the nature of climate change projections and mean centers of habitat shifts anticipated for eastern US tree species. (iii) Future climate novelty was present in future climate projections, potentially challenging the conceptual basis of FAM if tree species are not adapted to these changes. (iv) CCS typically occurred in areas with dissimilar levels of forest cover as the planting sites, presenting potential opportunities or challenges due to the presence or absence of forestlands at CCS. This also implies potential differences in forest assemblages between sites and future work may consider examining associated impacts to sourcing ecologically, economically, or culturally important species. The overall goal of this work was to provide a set of outputs that may enable FAM decision support. The need to implement forest climate change adaptation strategies designed to manage for tree species habitat redistribution under climate change is exceeding the knowledge base needed to inform management guidance. Climate-based and species-independent approaches can help bridge this gap and enable identification of CCS to support FAM.
Publicly available datasets were analyzed in this study. This data can be found at: https://adaptwest.databasin.org/.
BA: Conceptualization, Formal analysis, Funding acquisition, Writing – original draft, Writing – review & editing. AR: Conceptualization, Funding acquisition, Writing – review & editing. CK: Conceptualization, Funding acquisition, Writing – review & editing. DB: Conceptualization, Funding acquisition, Writing – review & editing. SM: Methodology, Resources, Writing – review & editing. AG: Writing – review & editing. AP: Methodology, Resources, Writing – review & editing. LI: Methodology, Resources, Writing – review & editing. ER: Writing – review & editing. PR: Writing – review & editing. CP: Writing – review & editing. JM: Writing – review & editing. AM: Writing – review & editing.
The author(s) declare financial support was received for the research, authorship, and/or publication of this article. Financial support was provided by the USDA Forest Service, Northern Research Station.
The authors wish to thank Carolyn Pike for helpful feedback and suggestions on the manuscript. We would also like to thank Tyler Refsland and Paula Marquardt for contributions toward the development of ideas presented in the manuscript. We wish to thank our home institutions for support. In addition, we would like to thank Wisconsin Department of Natural Resources for their support. Finally, we appreciate the feedback provided by two reviewers that improved this manuscript.
The authors declare that the research was conducted in the absence of any commercial or financial relationships that could be construed as a potential conflict of interest.
All claims expressed in this article are solely those of the authors and do not necessarily represent those of their affiliated organizations, or those of the publisher, the editors and the reviewers. Any product that may be evaluated in this article, or claim that may be made by its manufacturer, is not guaranteed or endorsed by the publisher.
The Supplementary Material for this article can be found online at: https://www.frontiersin.org/articles/10.3389/ffgc.2024.1449340/full#supplementary-material
AdaptWestProject (2022). Gridded current and projected climate data for North America at 1km resolution, generated using the ClimateNA v7.30 software (T. Wang et al., 2022). Available at: https://www.adaptwest.databasin.org (accessed February 27, 2024).
Aitken, S. N., Yeaman, S., Holliday, J. A., Wang, T., and Curtis-McLane, S. (2008). Adaptation, migration or extirpation: climate change outcomes for tree populations. Evol. Appl. 1, 95–111. doi: 10.1111/j.1752-4571.2007.00013.x
Bisbing, S. M., Urza, A. K., Buma, B. J., Cooper, D. J., Matocq, M., and Angert, A. L. (2021). Can long-lived species keep pace with climate change? Evidence of local persistence potential in a widespread conifer. Divers. Distrib. 27, 296–312. doi: 10.1111/ddi.13191
Bonebrake, T. C., Brown, C. J., Bell, J. D., Blanchard, J. L., Chauvenet, A., Champion, C., et al. (2018). Managing consequences of climate-driven species redistribution requires integration of ecology, conservation and social science. Biol. Rev. 93, 284–305. doi: 10.1111/brv.12344
Bower, A. D., Clair, J. B. S., and Erickson, V. (2014). Generalized provisional seed zones for native plants. Ecol. Appl. 24, 913–919. doi: 10.1890/13-0285.1
Bronson, D. R., and Gower, S. T. (2010). Ecosystem warming does not affect photosynthesis or aboveground autotrophic respiration for boreal black spruce. Tree Physiol. 30, 441–449. doi: 10.1093/treephys/tpq001
Chakraborty, D., Wang, T., Andre, K., Konnert, M., Lexer, M. J., Matulla, C., et al. (2015). Selecting populations for non-analogous climate conditions using universal response functions: the case of douglas-fir in central Europe. PLoS ONE 10, 1–21. doi: 10.1371/journal.pone.0136357
Chamberlain, S. (2020). rnoaa: “NOAA” Weather Data from R. R package version 1.2.0. Available at: https://cran.r-project.org/package=rnoaa (accessed February 27, 2024).
Champagne, E., Raymond, P., Royo, A. A., Speed, J. D. M., Tremblay, J. P., and Côté, S. D. (2021a). A review of ungulate impacts on the success of climate-adapted forest management strategies. Curr. For. Rep. 7, 305–320. doi: 10.1007/s40725-021-00148-5
Champagne, E., Turgeon, R., Munson, A. D., and Raymond, P. (2021b). Seedling response to simulated browsing and reduced water availability: insights for assisted migration plantations. Forests 12:1396. doi: 10.3390/f12101396
Cheng, J., Schloerke, B., Karambelkar, B., and Xie, Y. (2024). leaflet: Create interactive web maps with the JavaScript “Leaflet” library. R package version 2.2.2.9000. Available at: https://github.com/rstudio/leaflet, https://rstudio.github.io/leaflet/ (accessed February 27, 2024).
Clark, P. W., Amato, A. W. D., Palik, B. J., Woodall, C. W., Dubuque, P. A., Edge, G. J., et al. (2023). A lack of ecological diversity in forest nurseries limits the achievement of tree-planting objectives in response to global change. Bioscience 73, 575–586. doi: 10.1093/biosci/biad049
Clark, P. W., D'Amato, A. W., Evans, K. S., Schaberg, P. G., and Woodall, C. W. (2022). Ecological memory and regional context influence performance of adaptation plantings in northeastern US temperate forests. J. Appl. Ecol. 59, 314–329. doi: 10.1111/1365-2664.14056
Copenheaver, C. A., Shumaker, K. L., Butcher, B. M., Hahn, G. E., Perkins, L. P. J., Dukes, C. J., et al. (2020). Dendroclimatology of sugar maple (Acer saccharum): climate-growth response in a late-successional species. Dendrochronologia 63:125747. doi: 10.1016/j.dendro.2020.125747
Corlett, R. T., and Westcott, D. A. (2013). Will plant movements keep up with climate change? Trends Ecol. Evol. 28, 482–488. doi: 10.1016/j.tree.2013.04.003
Davis, M. B. (1983). Quaternary history of deciduous forests of Eastern North America and Europe. Ann. Missouri Bot. Gard. 70, 550–563. doi: 10.2307/2992086
Dobrowski, S. Z., Abatzoglou, J., Swanson, A. K., Greenberg, J. A., Mynsberge, A. R., Holden, Z. A., et al. (2013). The climate velocity of the contiguous United States during the 20th century. Glob. Chang. Biol. 19, 241–251. doi: 10.1111/gcb.12026
Doherty, K. D., Butterfield, B. J., and Wood, T. E. (2017). Matching seed to site by climate similarity: techniques to prioritize plant materials development and use in restoration. Ecol. Appl. 27, 1010–1023. doi: 10.1002/eap.1505
Domke, G. M., Fettig, C. J., Marsh, A. S., Baumflek, M., Gould, W. A., Halofsky, J. E., et al. (2023). “Ch. 7. Forests,” in Fifth National Climate Assessment, eds. A. R. Crimmins, C. W. Avery, D. R. Easterling, K. E. Kunkel, B. C. Stewart, and T. K. Maycock (Washington, DC: U.S. Global Change Research Program). doi: 10.7930/NCA5.2023.CH7
Dumroese, R. K., Williams, M. I., Stanturf, J. A., and Clair, J. B. S. (2015). Considerations for restoring temperate forests of tomorrow: forest restoration, assisted migration, and bioengineering. New For. 46, 947–964. doi: 10.1007/s11056-015-9504-6
Erickson, V. J., and Halford, A. (2020). Seed planning, sourcing, and procurement. Restor. Ecol. 28, S216–S224. doi: 10.1111/rec.13199
Etterson, J. R., Cornett, M. W., White, M. A., and Kavajecz, L. C. (2020). Assisted migration across fixed seed zones detects adaptation lags in two major North American tree species. Ecol. Appl. 30, 1–20. doi: 10.1002/eap.2092
Fargione, J., Haase, D. L., Burney, O. T., Kildisheva, O. A., Edge, G., Cook-Patton, S. C., et al. (2021). Challenges to the reforestation pipeline in the United States. Front. For. Glob. Change 4, 1–18. doi: 10.3389/ffgc.2021.629198
Fei, S., Desprez, J. M., Potter, K. M., Jo, I., Knott, J. A., and Oswalt, C. M. (2017). Divergence of species responses to climate change. Sci. Adv. 3:e1603055. doi: 10.1126/sciadv.1603055
Fick, S. E., and Hijmans, R. J. (2017). WorldClim 2: new 1-km spatial resolution climate surfaces for global land areas. Int. J. Climatol. 37, 4302–4315. doi: 10.1002/joc.5086
Fitzpatrick, M. C., and Dunn, R. R. (2019). Contemporary climatic analogs for 540 North American urban areas in the late 21st century. Nat. Commun. 10, 614. doi: 10.1038/s41467-019-08540-3
Gougherty, A. V., Keller, S. R., and Fitzpatrick, M. C. (2021). Maladaptation, migration and extirpation fuel climate change risk in a forest tree species. Nat. Clim. Chang. 11, 166–171. doi: 10.1038/s41558-020-00968-6
Gray, L. K., Gylander, T., Mbogga, M. S., Chen, P. Y., and Hamann, A. (2011). Assisted migration to address climate change: recommendations for aspen reforestation in western Canada. Ecol. Appl. 21, 1591–1603. doi: 10.1890/10-1054.1
Grenier, P., Parent, A. C., Huard, D., Anctil, F., and Chaumont, D. (2013). An assessment of six dissimilarity metrics for climate analogs. J. Appl. Meteorol. Climatol. 52, 733–752. doi: 10.1175/JAMC-D-12-0170.1
Hamrick, J. L. (2004). Response of forest trees to global environmental changes. For. Ecol. Manage. 197, 323–335. doi: 10.1016/j.foreco.2004.05.023
Hausfather, Z., Marvel, K., Schmidt, G. A., Nielsen-Gammon, J. W., and Zelinka, M. (2022). Climate simulations: recognize the ‘hot model' problem. Nature 605, 26–29. doi: 10.1038/d41586-022-01192-2
Hausfather, Z., and Peters, G. P. (2020). RCP8.5 is a problematic scenario for near-term emissions. Proc. Natl. Acad. Sci. U. S. A. 117, 27791–27792. doi: 10.1073/pnas.2017124117
IPCC (2022). Climate Change 2022: Impacts, Adaptation, and Vulnerability. Contribution of Working Group II to the Sixth Assessment Report of the Intergovernmental Panel on Climate Change, eds. H.-O. Pörtner, D. C. Roberts, M. Tignor, E. S. Poloczanska, K. Mintenbeck, A. Alegría, et al. (Cambridge: Cambridge University Press), 3056. doi: 10.1017/9781009325844
Iverson, L. R., Dale, M. E., Scott, C. T., and Prasad, A. (1997). A GIS-derived integrated moisture index to predict forest composition and productivity of Ohio forests (U.S.A.). Landsc. Ecol. 12, 331–348. doi: 10.1023/A:1007989813501
Iverson, L. R., Peters, M. P., Prasad, A. M., and Matthews, S. N. (2019a). Analysis of climate change impacts on tree species of the eastern US: results of DISTRIB-II modeling. Forests 10:302. doi: 10.3390/f10040302
Iverson, L. R., Prasad, A. M., Matthews, S. N., and Peters, M. (2008). Estimating potential habitat for 134 eastern US tree species under six climate scenarios. For. Ecol. Manage. 254, 390–406. doi: 10.1016/j.foreco.2007.07.023
Iverson, L. R., Prasad, A. M., Peters, M. P., and Matthews, S. N. (2019b). Facilitating adaptive forest management under climate change: a spatially specific synthesis of 125 species for habitat changes and assisted migration over the eastern United States. Forests 10:989. doi: 10.3390/f10110989
Joyce, D. G., and Rehfeldt, G. E. (2013). Climatic niche, ecological genetics, and impact of climate change on eastern white pine (Pinus strobus L.): guidelines for land managers. For. Ecol. Manage. 295, 173–192. doi: 10.1016/j.foreco.2012.12.024
LaBonte, N. (2022). Red pine: guidance for seed transfer within the eastern United States. Tree Plant. Notes 65, 63–68. Available at: https://rngr.net/publications/tpn/66-2 (accessed February 27, 2024).
Lafleur, B., Paré, D., Munson, A. D., and Bergeron, Y. (2010). Response of northeastern North American forests to climate change: will soil conditions constrain tree species migration? Environ. Rev. 18, 279–289. doi: 10.1139/A10-013
Lawrimore, J. H., Ray, R., Applequist, S., Korzeniewski, B., and Menne, M. J. (2016). Global Summary of the Month (GSOM), Version 1. NOAA National Centers for Environmental Information. Available at: https://doi.org/10.7289/V5QV3JJ5 (accessed February 27, 2024).
Leimu, R., and Fischer, M. (2008). A meta-analysis of local adaptation in plants. PLoS ONE 3, 1–8. doi: 10.1371/journal.pone.0004010
Leites, L. P., Rehfeldt, G. E., and Steiner, K. C. (2019). Adaptation to climate in five eastern North America broadleaf deciduous species: growth clines and evidence of the growth-cold tolerance trade-off. Perspect. Plant Ecol. Evol. Syst. 37, 64–72. doi: 10.1016/j.ppees.2019.02.002
Lu, P., Parker, W. H., Cherry, M., Colombo, S., Parker, W. C., Man, R., et al. (2014). Survival and growth patterns of white spruce (Picea glauca [Moench] Voss) rangewide provenances and their implications for climate change adaptation. Ecol. Evol. 4, 2360–2374. doi: 10.1002/ece3.1100
Mahony, C. R., Cannon, A. J., Wang, T., and Aitken, S. N. (2017). A closer look at novel climates: new methods and insights at continental to landscape scales. Glob. Chang. Biol. 23, 3934–3955. doi: 10.1111/gcb.13645
Mahony, C. R., Wang, T., Hamann, A., and Cannon, A. J. (2022). A global climate model ensemble for downscaled monthly climate normals over North America. Int. J. Climatol. 5871–5891. doi: 10.1002/joc.7566
Mckenney, D. W., Pedlar, J. H., Rood, R. B., and Price, D. (2011). Revisiting projected shifts in the climate envelopes of North American trees using updated general circulation models. Glob. Change Biol. 17, 2720–2730. doi: 10.1111/j.1365-2486.2011.02413.x
Millar, C. I., Stephenson, N. L., and Stephens, S. L. (2007). Climate change and forests of the future: managing in the face of uncertainty. Ecol. Appl. 17, 2145–2151. doi: 10.1890/06-1715.1
Morelli, T. L., Daly, C., Dobrowski, S. Z., Dulen, D. M., Ebersole, J. L., Jackson, S. T., et al. (2016). Managing climate change refugia for climate adaptation. PLoS ONE 11:e0159909. doi: 10.1371/journal.pone.0159909
Muller, J. J., Nagel, L. M., and Palik, B. J. (2019). Forest adaptation strategies aimed at climate change: assessing the performance of future climate-adapted tree species in a northern Minnesota pine ecosystem. For. Ecol. Manage. 451:117539. doi: 10.1016/j.foreco.2019.117539
Nagel, L. M., Palik, B. J., Battaglia, M. A., D'Amato, A. W., Guldin, J. M., Swanston, C. W., et al. (2017). Adaptive silviculture for climate change: a national experiment in manager-scientist partnerships to apply an adaptation framework. J. For. 115, 167–178. doi: 10.5849/jof.16-039
NALC (2020). 2020 North American Land Cover at 30 m spatial resolution. Produced by NRCan/CCMEO, USGS, INEGI, CONABIO, and CONAFOR. Available at: http://www.cec.org/north-american-environmental-atlas/land-cover-30m-2020/ (accessed February 27, 2024).
O'Neill, G. A., Stoehr, M., and Jaquish, B. (2014). Quantifying safe seed transfer distance and impacts of tree breeding on adaptation. For. Ecol. Manage. 328, 122–130. doi: 10.1016/j.foreco.2014.05.039
Palik, B. J., Clark, P. W., D'Amato, A. W., Swanston, C. W., and Nagel, L. M. (2022). Operationalizing forest assisted migration in the context of climate change adaptation: examples from the eastern USA. Ecosphere 13:e4260. doi: 10.1002/ecs2.4260
Palik, B. J., D'Amato, A. W., Slesak, R. A., Kastendick, D., Looney, C., and Kragthorpe, J. (2021). Eighth-year survival and growth of planted replacement tree species in black ash (Fraxinus nigra) wetlands threatened by emerald ash borer in Minnesota, USA. For. Ecol. Manage. 484:118958. doi: 10.1016/j.foreco.2021.118958
Park, A., and Talbot, C. (2018). Information underload: ecological complexity, incomplete knowledge, and data deficits create challenges for the assisted migration of forest trees. Bioscience 68, 251–263. doi: 10.1093/biosci/biy001
Parmesan, C., Morecroft, M. D., Trisurat, Y., Adrian, R., Anshari, G. Z., Arneth, A., et al. (2022). “Terrestrial and freshwater ecosystems and their services,” in Climate Change 2022: Impacts, Adaptation and Vulnerability. Contribution of Working Group II to the Sixth Assessment Report of the Intergovernmental Panel on Climate Change, eds. H. O. Pörtner, D. C. Roberts, M. Tignor, E. S. Poloczanska, K. Mintenbeck, A. Alegría, et al. (Cambridge: Cambridge University Press), 197–377. doi: 10.1017/9781009325844.004
Pedlar, J. H., McKenney, D. W., Aubin, I., Beardmore, T., Beaulieu, J., Iverson, L., et al. (2012). Placing forestry in the assisted migration debate. Bioscience 62, 835–842. doi: 10.1525/bio.2012.62.9.10
Peters, M. P., Prasad, A. M., Matthews, S. N., and Iverson, L. R. (2020). Climate Change Tree Atlas (Version 4). Delaware, OH: USFS, NRS NIACS. Available at: https://www.fs.usda.gov/nrs/atlas/ (accessed February 27, 2024).
Pike, C., Potter, K. M., Berrang, P., Crane, B., Baggs, J., Leites, L., et al. (2020). New seed-collection zones for the eastern United States: the eastern seed zone forum. J. For. 118, 444–451. doi: 10.1093/jofore/fvaa013
Pike, C. C., and Bloese, P. (2022). Sugar maple: guidance for seed transfer within the eastern United States. Tree Plant. Notes 65, 21–28.
Pike, C. C., and Haase, D. L, . (eds). (2024). Seed Transfer Guidelines for important tree species of the eastern United States. Agricultural Handbook No. 801. Washington, DC: USDA Forest Service, 135.
Prasad, A., and Leites, L. (2022). Ecological analysis of intraspecific variability of eastern white pine (Pinus strobus) under climate change by combining provenance and demographic data. Landsc. Ecol. 37, 109–128. doi: 10.1007/s10980-021-01333-4
Prasad, A., Pedlar, J., Peters, M., Matthews, S., Iverson, L., McKenney, D., et al. (2024). “Understanding climate change dynamics of tree species: implications for future forests,” in Future Forests: Mitigation and Adaptation to Climate Change, ed. S. G. McNulty (Amsterdam: Elsevier), 151–175. doi: 10.1016/B978-0-323-90430-8.00002-2
Prasad, A., Pedlar, J., Peters, M., McKenney, D., Iverson, L., Matthews, S., et al. (2020). Combining US and Canadian forest inventories to assess habitat suitability and migration potential of 25 tree species under climate change. Divers. Distrib. 26, 1142–1159. doi: 10.1111/ddi.13078
Prasad, A. M., and Potter, K. M. (2017). Macro-scale assessment of demographic and environmental variation within genetically derived evolutionary lineages of eastern hemlock (Tsuga canadensis), an imperiled conifer of the eastern United States. Biodivers. Conserv. 26, 2223–2249. doi: 10.1007/s10531-017-1354-4
Price, D. T., Alfaro, R. I., Brown, K. J., Flannigan, M. D., Fleming, R. A., Hogg, E. H., et al. (2013). Anticipating the consequences of climate change for Canada's boreal forest ecosystems. Environ. Rev. 21, 322–365. doi: 10.1139/er-2013-0042
PRISMClimateGroup (2022). Oregon State University. Available at: https://prism.oregonstate.edu (accessed February 27, 2024).
Rastogi, D., Touma, D., Evans, K. J., and Ashfaq, M. (2020). Shift toward intense and widespread precipitation events over the United States by mid-21st Century. Geophys. Res. Lett. 47:e2020GL089899. doi: 10.1029/2020GL089899
Refsland, T. K., Adams, B., Bronson, D., Kern, C. C., Marquardt, P., McGraw, A. M., et al. (2023). Synthesis of plant-soil feedback effects on eastern North American tree species: implications for climate-adaptive forestry. Front. Ecol. Evol. 11:1073724. doi: 10.3389/fevo.2023.1073724
Rehfeldt, G. E., Leites, L. P., Joyce, D. G., and Weiskittel, A. R. (2018). Role of population genetics in guiding ecological responses to climate. Glob. Chang. Biol. 24, 858–868. doi: 10.1111/gcb.13883
Royo, A. A., Raymond, P., Kern, C. C., Adams, B. T., Bronson, D., Champagne, E., et al. (2023). Desired REgeneration through Assisted Migration (DREAM): implementing a research framework for climate-adaptive silviculture. For. Ecol. Manage. 546:121298. doi: 10.1016/j.foreco.2023.121298
Schwalm, C. R., Glendon, S., and Duffy, P. B. (2020). Reply to hausfather and peters: RCP8.5 is neither problematic nor misleading. Proc. Natl. Acad. Sci. USA. 117, 27793–27794. doi: 10.1073/pnas.2018008117
Seliger, B. J., McGill, B. J., Svenning, J. C., and Gill, J. L. (2021). Widespread underfilling of the potential ranges of North American trees. J. Biogeogr. 48, 359–371. doi: 10.1111/jbi.14001
Shryock, D. F., DeFalco, L. A., and Esque, T. C. (2018). Spatial decision-support tools to guide restoration and seed-sourcing in the Desert Southwest. Ecosphere 9:e02453. doi: 10.1002/ecs2.2453
St.Clair, J. B., Richardson, B. A., Stevenson-Molnar, N., Howe, G. T., Bower, A. D., Erickson, V. J., et al. (2022). Seedlot selection tool and climate-smart restoration tool: web-based tools for sourcing seed adapted to future climates. Ecosphere 13, 1–18. doi: 10.1002/ecs2.4089
Stralberg, D., Arseneault, D., Baltzer, J. L., Barber, Q. E., Bayne, E. M., Boulanger, Y., et al. (2020). Climate-change refugia in boreal North America: what, where, and for how long? Front. Ecol. Environ. 18, 261–270. doi: 10.1002/fee.2188
USDA Agricultural Research Service (2023). USDA Plant Hardiness Zone Map. Available at: https://planthardiness.ars.usda.gov/ (accessed February 27, 2024).
Wang, T., O'Neill, G. A., and Aitken, S. N. (2010). Integrating environmental and genetic effects to predict responses of tree populations to climate. Ecol. Appl. 20, 153–163. doi: 10.1890/08-2257.1
Williams, J. W., and Jackson, S. T. (2007). Novel climates, no-analog communities, and ecological surprises. Front. Ecol. Environ. 5, 475–482. doi: 10.1890/070037
Williams, J. W., Jackson, S. T., and Kutzbach, J. E. (2007). Projected distributions of novel and disappearing climates by 2100 AD. Proc. Natl. Acad. Sci. USA. 104, 5738–5742. doi: 10.1073/pnas.0606292104
Williams, M. I., and Dumroese, R. K. (2013). Preparing for climate change: forestry and assisted migration. J. For. 111, 287–297. doi: 10.5849/jof.13-016
Young, D. N., Blush, T. D., Landram, M., Wright, J. W., Latimer, A. M., and Safford, H. D. (2020). Assisted gene flow in the context of large-scale forest management in California, USA. Ecosphere 11:e03001. doi: 10.1002/ecs2.3001
Keywords: forest assisted migration, climatically-compatible seedlot, climate analog, adaptive silviculture, climate change
Citation: Adams BT, Royo AA, Kern CC, Bronson DR, Matthews SN, Gougherty AV, Prasad AM, Iverson LR, Rehm EM, Raymond P, Périé C, Miesel JR and McGraw AM (2024) Identifying climatically-compatible seedlots for the eastern US: building the predictive tools and knowledge to enable forest assisted migration. Front. For. Glob. Change 7:1449340. doi: 10.3389/ffgc.2024.1449340
Received: 14 June 2024; Accepted: 21 October 2024;
Published: 20 November 2024.
Edited by:
Debojyoti Chakraborty, Bundesforschungs- und Ausbildungszentrum für Wald, Naturgefahren und Landschaft (BFW), AustriaReviewed by:
Katharina Julie Liepe, Thünen Institute of Forest Genetics, GermanyCopyright © 2024 Adams, Royo, Kern, Bronson, Matthews, Gougherty, Prasad, Iverson, Rehm, Raymond, Périé, Miesel and McGraw. This is an open-access article distributed under the terms of the Creative Commons Attribution License (CC BY). The use, distribution or reproduction in other forums is permitted, provided the original author(s) and the copyright owner(s) are credited and that the original publication in this journal is cited, in accordance with accepted academic practice. No use, distribution or reproduction is permitted which does not comply with these terms.
*Correspondence: Bryce T. Adams, YnJ5Y2UuYWRhbXNAdXNkYS5nb3Y=
Disclaimer: All claims expressed in this article are solely those of the authors and do not necessarily represent those of their affiliated organizations, or those of the publisher, the editors and the reviewers. Any product that may be evaluated in this article or claim that may be made by its manufacturer is not guaranteed or endorsed by the publisher.
Research integrity at Frontiers
Learn more about the work of our research integrity team to safeguard the quality of each article we publish.