- 1School of Economics and Management, Northeast Forestry University, Harbin, Heilongjiang, China
- 2School of Tourism Ecology and Environment, Guilin Tourism University, Guilin, Guangxi, China
This paper proposes a theoretical framework for assessing ecological protection and restoration from the perspective of ecological efficiency. We applied the super-efficiency Slack-based measure model to examine the social and economic impacts of ecological resource consumption transformation in Heilongjiang Province, China. Additionally, a convergence analysis was used to evaluate and test the impact of the standard deviation ellipse method on regional sustainability. The results indicated that the land use structure was unstable; the conversion rate of resource consumption was low; and the average Ecological efficiency was only 0.343 in terms of the land use structure. Funds for forest ecological restoration have a significant impact on the effectiveness of ecological resource transformation. Implementing the Chinese ecological restoration project improves the ecological efficiency level of the communities. The center of gravity of ecological efficiency moved greatly in the years when forestry investment increased. Technological transfer and diffusion, experience imitation in environmental regulation, and eventually convergent steady-state levels of the ecological efficiency of different regions are necessary to improve the economic and social development level of regions with low environmental quality efforts should be made to reduce resource consumption intensity, increase fund utilization efficiency, and form a comprehensive and systematic system of ecological environment governance through reasonable enhancement of regional environmental regulations, increased investment in technological advancement, and funds for ecological protection and restoration.
1 Introduction
Ecological restoration (ER) originated in the early 20th century in European and American nations (Higgs, 2003). The goal is to assist ecosystems in restoring their original structure and function after being damaged (Hira et al., 2023). ER involves assisting ecosystems to sustain their healthy historical development path (Gann et al., 2019). Land degradation can be reversed and biodiversity and ecosystem services can be improved through ecological restoration (ER). Global land degradation and environmental change are substantial risks to biodiversity and ecosystem services, as reported by Pandit et al. (2020). Wortley et al. (2013) state that these issues have been widely integrated into local and global natural resource management methods.
International organizations are currently focusing on the implementation of systematic protection and restoration principles in ecological restoration processes (Rey Benayas et al., 2009). These practices include the Brazilian Atlantic Tropical Forest Landscape Restoration (Chazdon and Uriarte, 2016), South Africa’s National Parks Restoration Project (Moyo et al., 2021), and the wide-ranging restoration of riparian systems in the western United States (Oppenheimer et al., 2015). Forest restoration efforts in Nepal utilize the approaches mentioned by Laudari et al. (2022). Chinese governments have made significant investments and offered financial support in order to address the environmental crisis caused by massive land usage (Li et al., 2020; Bi et al., 2021; Yin and Cao, 2022). China has carried out 16 major ecological restoration initiatives since the 1980s, such as restoring farmland back into forests and safeguarding natural forests, resulting in substantial advancements (Qu et al., 2020; Worlanyo and Jiangfeng, 2021; Zhang et al., 2022). However, most countries find it challenging to simultaneously achieve rapid economic development and enhanced ecosystem services (Wang et al., 2021).
Previous studies on ecological restoration evaluation primarily focused on assessing vegetation structure, ecological processes, and biodiversity indicators, contributing to the limited universality and applicability of the ecosystem restoration evaluation index system (Yang et al., 2013). Hence, it is necessary to enhance research on multi-object and multi-scale ecological restoration in order to improve the evaluation system of ecological restoration effects. Researchers are turning their attention on the significance of evaluating ecological restoration in the field of ecology. Consequently, the obstacles encountered in ecological restoration evaluation research are gaining more recognition (Ding and Zhao, 2014). Researchers have conducted numerous ecological restoration evaluation studies regarding China’s ecological protection and restoration projects. However, due to variations in research methodology, objectives, and perspectives, research areas encompass biodiversity, ecosystem services, climate change, land use, and ecological restoration theories and technologies. This diversity has led to a wide range of research outcomes and a scarcity of universally applicable summaries of ecological restoration experiences. Summary of a study on ecological restoration with general applicability (Gao and Yang, 2015).
Ecological attributes are the most widely utilized post-implementation indicators in China and other regions, which represent 94% of cases, but research on social and economic indicators is relatively limited at 3.5%. Ecological conservation and restoration research primarily emphasizes high-income countries over countries with significant deforestation rates (Wortley et al., 2013), leading to a notable scarcity of knowledge summaries for regions requiring extensive restoration efforts. Only a limited number of studies have endeavored to assess restoration from a social and economic perspective (Zheng and Zhuang, 2021). It must be emphasized that there is a significant absence of empirical information concerning the social and economic results of restoration (Pandit et al., 2020). Socio-economic factors should be included when assessing ecological conservation and restoration efforts.
Extensive research has been conducted on various ecosystems in China, such as rivers, wetlands, forests, grasslands, agricultural lands, and post-mining lands. However, there remains a need for a comprehensive understanding of ecological restoration practices across all terrestrial ecosystems (Cui et al., 2021). Although urbanization and ER conflict still persist during economic development (Wang et al., 2019), there are various issues that need to be addressed, including insufficient understanding of the systematicity and flowability of ecosystem elements, a lack of coordination between socio-economic and environmental factors, insufficient recognition of ecosystem nature, and a lack of long-term cooperation among departments (Xu et al., 2006; Qu et al., 2020). This has resulted in a lack of coordination, capacity, and adaptability among elements of the national spatial system. As research advances in different areas of ecological restoration, and as more ecologists reflect on large-scale ecosystem changes, ecological restoration programs should act as a bridge between complex and unpredictable changes, helping to restore meaningful and tangible relationships between humans and ecosystems (Ives et al., 2017). A new theory of progressive ecological restoration has also been proposed by scholar Liu (2020), emphasizing the importance of monitoring and dynamic assessment. In order to obtain the long-term effects of ecological restoration programs, ecological restoration projects must be integrated with some form of economic development. In order to optimize the effectiveness of ecological restoration efforts, it is essential to conduct research on evaluating ecological protection and restoration (Erbaugh et al., 2020), establish geographically specific attributes and management strategies (Edrisi and Abhilash, 2021), compile lessons learned, and formulate restoration models suitable for various scales.
China has made ecological conservation, restoration, and optimization national strategic priorities (Li et al., 2020). China proposed integrated restoration of mountain-river-forest-farmland-lake-grassland (MRFFLG) ecosystems in 2013 as an innovative approach to advancing territorial space protection using a systemic and holistic approach (Gao et al., 2022). It requires beginning by optimizing territorial space layout, adjusting land use structure and relationships, and conducting overall protection, systematic restoration, and comprehensive governance in accordance with the integrity, systematicity, and regularity of the ecosystem (Wang et al., 2022). China has implemented three batches (25 in total) of pilot projects since 2016 for the conservation and restoration of MRFFLG, which cover the majority of Chinese provinces (autonomous regions and municipalities). It is imperative to develop quantitative methods to determine whether or not projects are successful in order to rationalize and improve such methods. However, empirical research in ecological conservation and restoration lacks methods for analyzing social and economic outcomes and summarizing successful experiences. This has resulted in a lack of coordination, capacity, and adaptability among various elements in the country’s spatial system. This has adversely affected the stability of the spatial structure.
There is no doubt that ER success is not simply determined by ecological progress but also by the ability to fully utilize its potential (Erbaugh et al., 2020), adapt to changes in the ecological environment, and improve the need for ER decision-making and policy (Wortley et al., 2013). Clearly, this promotes the development of an interdisciplinary research area aimed at assessing the effectiveness of restoration strategies from an interdisciplinary perspective and conducting a comprehensive analysis of both ecological and socioeconomic benefits (Abhilash, 2021).
It presents a theoretical framework for assessing biodiversity conservation and restoration based on multi-objective outputs and multi-factor inputs. Heilongjiang Province (HP) in China is used as an example for measuring and evaluating ecological efficiency (EE) of the MRFFLG ecosystem. EE is defined as “creating more goods and services through fewer resources and generating less waste and pollution” (Hitchens et al., 1998). This concept can also be used to describe “the effectiveness of ecological resources in meeting human needs” (Huang et al., 2023). The term EE refers to the efficiency with which MRFFLG natural resources are utilized to meet human needs. This creates more goods and services at a lower cost and less environmental pollution. It is intended to satisfy the multi-objective requirements of the ecosystem. In the context of eco-protection and socioeconomic growth, it is possible to achieve a win-win situation (Mao et al., 2019). Consequently, it is imperative that ecological resources are monitored and evaluated to ensure they are converted efficiently into economic and social outputs. This is to identify significant reasons for sluggish economic development, and to provide a basis for transforming natural resources into economic development opportunities.
This study aims to: (1) develop a framework for assessing the EE of the MRFFLG ecosystem from a social-ecological systems perspective, focusing on transforming ecological resources into economic and social development opportunities; (2) establish an indicator system that evaluates economic, social, and natural resources; (3) analyze the spatiotemporal evolution trend and convergence characteristics of the EE in the study area; and (4) offer policy recommendations based on the evaluation of changes in EE trends and spatial distribution patterns in regional areas.
2 Material
2.1 Study area
Heilongjiang Province (HP) is located in the northeastern forest zone of China and is the northernmost and easternmost province on mainland China (121°11′ W to 135°05′ E, 43°26′ S to 53°33′ N). It is also a key guarantee area for food safety and ecological security of China, with a total area of 473,000 km2. It is divided into 12 prefecture-level cities and one prefecture, as well as 121 county-level administrative divisions (Figure 1). It is estimated that HP has 47.3% forest coverage and an average annual rainfall of 608.5 mm, with an average annual temperature of 4.2°C in 2021. The HP landscape is characterized by various types of ecosystems, which include five-types of mountains, one-type of water body, one-type of grass field, and three-types of farmlands, while the MRFFLG landscape is distributed over a broad area. A pilot project for “ecological protection and restoration of MRFFLG” is underway in this area. Since HP is a resource-rich province, it has great potential to expand ecological space and serves as an important carrier of ecological security on a national level (Liu et al., 2022). Historical development and the exploitation of natural resources have left the ecosystem with a lot of historical debt. The quality of the ecosystem has not yet been transformed qualitatively. In contrast, HP has lagged behind other provinces in China in economic development. There appears to be a contradiction between urgent economic growth and ecological protection and restoration. Economies and social development in the region must also be balanced with ecological protection and restoration. It is difficult to infer successful experiences and challenges faced by ecological protection and restoration as there is insufficient information regarding spatial changes in land use cover and ecosystem services in HP, the need for economic growth and economic development, and the mismatch between limited resources in quantity, space, and time. In light of the fact that natural resources can be converted into financial efficiency, it is worthwhile to explore the process. Research is challenging due to the variability of degradation types in ecosystems and the specificity of restoration goals. In addition to technical progress in ecological preservation and restoration, a comprehensive goal-guided framework is urgently required.
The measurement of the ecological value of the MRFFLG life community (LC) in HP and the identification of its spatial–temporal differences and evolution trends have great practical relevance for enhancing regional ecological value and promoting harmony between humans and nature.
2.2 Data sources
Specifically, this study examined data for 11 typical ecological cities in HP from 2006 to 2019. This included the 11th Five-Year Plan for National Economic and Social Development (2006–2010), the 12th Five-Year Plan (2011–2015), and the 13th Five-Year Plan (2016–2020). At this time, China’s economic and social development entered an exciting phase. The contradiction between natural resources and the environment became more evident, indicating a significant gap between demand space and resource availability. The study uses statistical data collection and calculations based on geographical information as its data sources.
2.2.1 Statistical data
The urban gross domestic product (GDP) data is derived from the “China Urban Statistical Yearbook” (published by the China Statistical Publishing House); the public finance revenue and expenditure data (in ten thousand yuan), the pollution emissions data, the labor force population, and the area of green space per capita (in m2) of urban roads and parks are all reported. All other economic data from 2006 to 2019 are derived from the “Heilongjiang Statistical Yearbook” (published by China Statistical Publishing House); urban CO2 emissions data (in million tonnes) from 2006 to 2019 are derived from China city emission data in the Chinese Carbon Accounting Database1 (Shan et al., 2022).
2.2.2 Geographic information data
In order to obtain the HP 2019 elevation data (Digital Elevation Model, DEM), the Geospatial Data Cloud website2 sourced the SRTMDEM 90 M original elevation data; ESACCI (European Space Agency Climate Change Institute) provided the land use and land cover data from 2006 to 2019.
2.2.3 Extraction of geographic information data
In conjunction with the actual situation and research needs of HP, ArcGIS software was used to perform supervised classification and visual interpretation of remote sensing images. Land use types were reclassified and extracted based on the land classification standards of the third national land survey in China. Reorganizing “wetlands” as a primary land category alongside cultivated land, forest area, grassland and waters. For each prefecture-level city in HP, water area, forest area, cultivated land area, grassland area, construction land area, wetland area, and unused land area were calculated from 2006 to 2019. Specifically, forest types include deciduous forests, bamboo forests, shrub forests, and others. It consists of rivers, lakes, reservoirs, ponds, canals, water engineering land, glaciers, and permanent snow. Rice fields, irrigated lands, and dry lands constitute farmlands. Natural grassland, artificial grassland, marsh grassland, and other grasslands are mainly included in grassland. Construction land consists primarily of urban and rural areas. Unoccupied lands include fallow lands, terraced lands, and bare lands. Wetland habitats consist of mangrove forests, forested swamps, shrubby swamps, salt flats, marshland, and tidal flats. The specific classification criteria for the various types of land are shown in the Table 1. In cases where some land use types were found to be outside the main land use types expected to be classified during the actual classification process, they were corrected according to the actual utilization status, e.g., bare areas such as 200, 201, and 202 were classified as other types. The reclassification was done according to the above criteria through the ArcGIS Spatial Analysis tool, after which the area of each land type was extracted from the different areas in the area analysis module of the Spatial Analysis tool.
3 Research ideas and methods
3.1 The theoretical framework of research ideas
This article presents a detailed workflow (Figure 2). Assuming MRFFLG constitutes a LC from the perspective of EE, a theoretical framework is proposed that involves multi-objective outputs and multi-factor inputs in order to evaluate ecological protection and restoration.
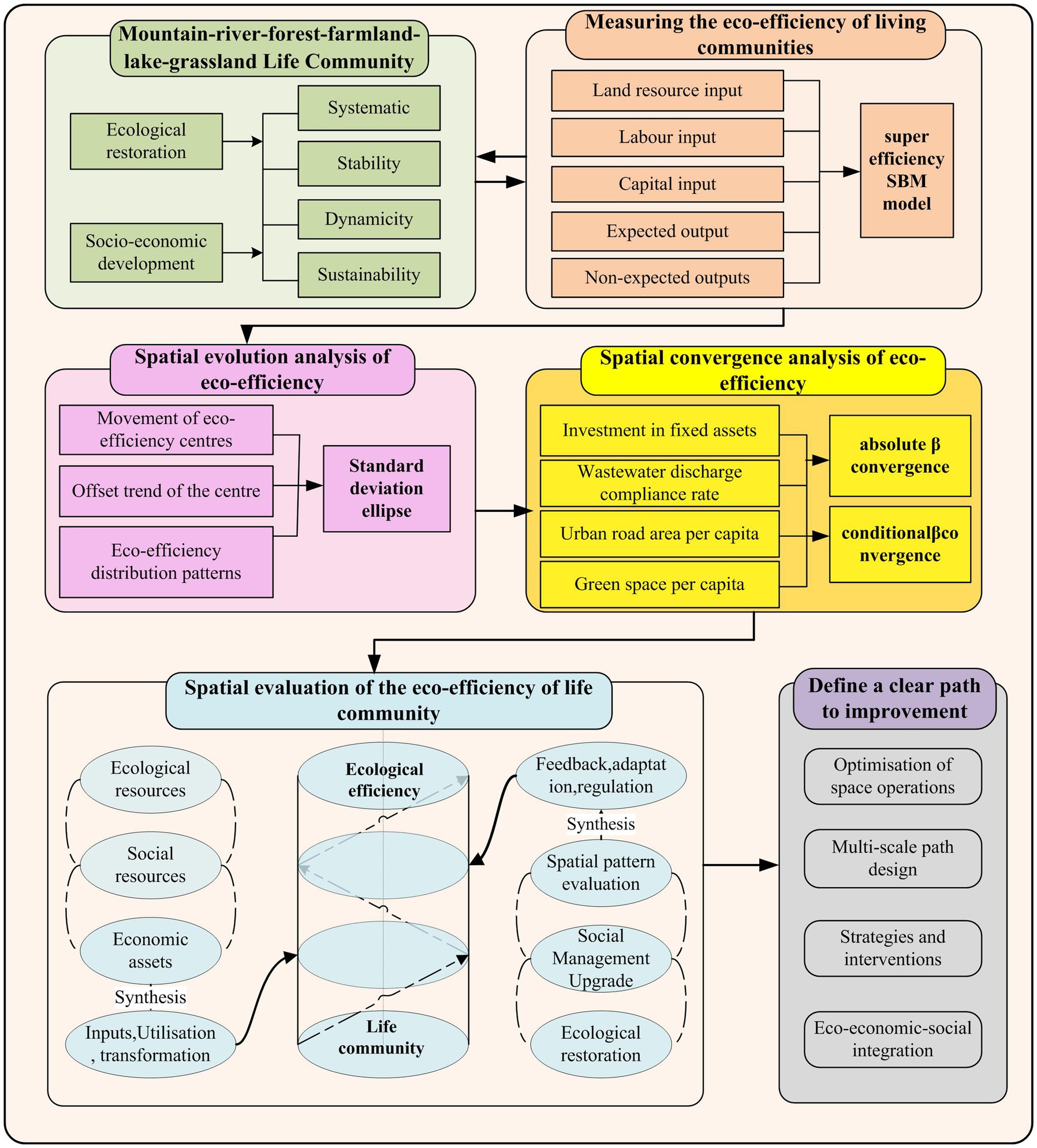
Figure 2. Framework for the evaluation of ecological protection and restoration that takes into account multi-objective outputs and multi-factor inputs.
3.2 Research methodology
3.2.1 Research methodology for measuring ecological efficiency in the community of life
The purpose of this paper is to measure EE in 11 cities in HP using the super-efficiency (SE) Slack-Based Measure (SBM) model, which has obvious improvements over traditional methods. In contrast to the radial SBM model, the SE-SBM model proposed by Tone (2001) integrates both the SE and SBM models, solving the problem that standard efficiency models cannot further compare the efficiency of decision-making units when multiple decision-making units are efficient. Ultimately, this article selects the SE-SBM model with undesirable outputs for research because it has stronger practicality for measuring the EE within the MRFFLG LC. Equation (1) illustrates the construction of the SE-SBM model.
,
In the SE-SBM model, represents the optimal solution of the model, which is the EE value of the LC calculated by the model, with a value range of 0 to 1. represents the number of input variables, which range from 1 to m, assuming there are m inputs. represents the slack variables of inputs, indicating the meaning of reduction if inputs are excessive. represents the decision unit. represents the output of the decision unit. is the evaluated object. is the weight variable. is a virtual effective decision unit that serves as a reference point for the optimal solution. Constraints on non-desired output b are added based on this reference point. The summation of represents the effective decision units constructed using different non-desired outputs, which is also a reference system. represents the slack variable of unexpected output, which is the amount that unexpected output needs to be reduced; represents the unexpected output of the decision unit. For the -th decision unit, if its unexpected output is to be effective, it needs to be reduced by , and if its expected output is to be effective, it must increase output by. It is also required that both the slack variables of inputs and outputs must be greater than or equal to 0. represents the assumption of constant returns to scale.
3.2.2 Methodology for constructing an ecological efficiency measurement indicator system
The purpose of this paper is to construct an EE input–output evaluation indicator system for the MRFFLG LC in HP in four dimensions (Table 2). Firstly, the indicator system emphasizes the systematization and integrity of communities by using four dimensions of inputs, such as natural resources, social and economic inputs, and unanticipated and expected outputs. Secondly, natural resource elements involved in production and living in the research area have been included in the data selection, thereby enriching the indicator system for assessing the effectiveness of ecological conservation and restoration. From the perspective of a socio-ecological system, this study constructs an evaluation framework and focuses on the consumption of ecological resources during the transformation of ecological resources into economic resources and the challenges that are associated with regional sustainable development. As a function of output, the study considers how efficiently natural resources are utilized under conditions of economic growth.
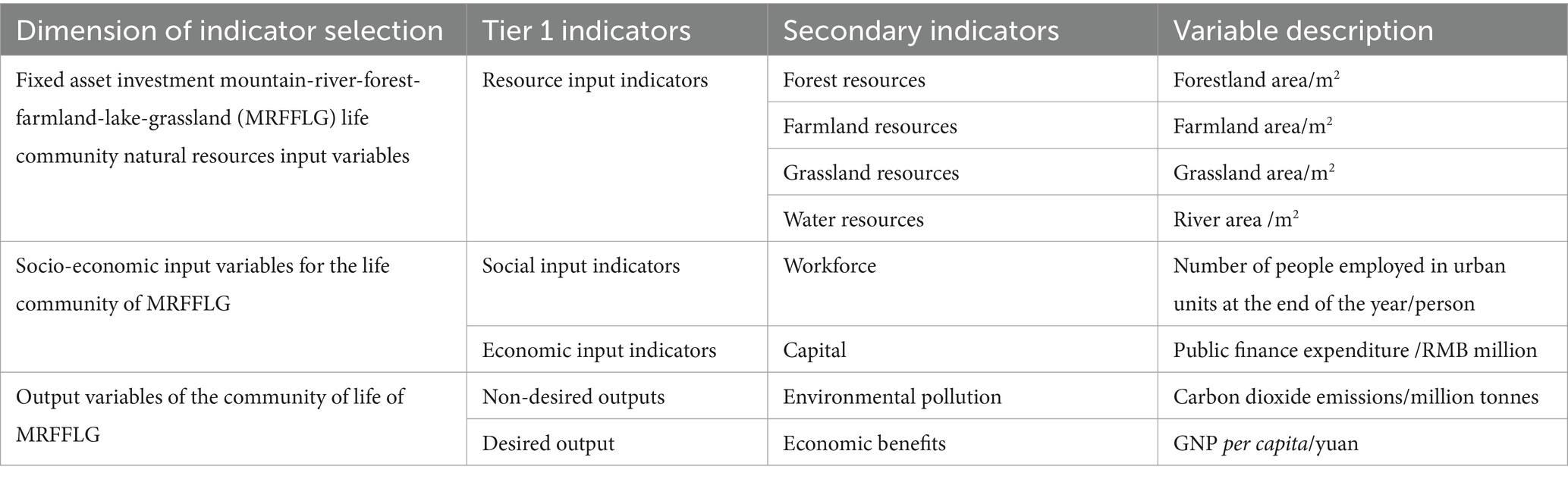
Table 2. The ecological efficiency evaluation index system of the life community of mountain-river-forest-farmland-lake-grassland (MRFFLG) systems.
A compelling argument is made by Kondo and Nakamura (2005) that the use of resources is the fundamental cause of environmental pressure and that policies designed to improve efficiency are often easier to adopt than policies that restrict economic activity levels. Although there are many input and output indicators for EE, few of these reflect the close relationship between economic, social, and natural ecological systems. It is difficult to directly apply the results of EE estimation to ecological system protection or restoration practices since indicator selection tends to focus more on the inputs and outputs of the social and economic system. GDP and CO2 emissions are commonly used as output indicators (Grand, 2016). The scale of material inputs is increasingly becoming more important when it comes to issues related to waste generation and emissions (Behrens et al., 2007). As a result, unexpected CO2 emissions have become an important indicator that cannot be ignored. Research on EE evaluation has shown that input indicators are diverse, with a greater emphasis on capital stock, labor quantity, and energy consumption being used as key input indicators for determining environmental efficiency (Kondo and Nakamura, 2005; Behrens et al., 2007; Yu et al., 2013). EE studies cannot be directly applied to ecological system governance if there is a lack of understanding of the local ecosystem and its close relationship with the natural ecological system (Hukkinen, 2001).
Several major resource types are considered in this article using a balanced multiple input approach, which includes natural elements such as MRFFLG and considers the importance of land demand in terms of ecological pressure for several major resource types. Combined with existing research on social and economic input indicators, CO2 emissions are added as an unwelcome output indicator that identifies the quality of the environment. This indicator system is intended to represent the direction of regional EE development while incorporating the system thinking of the MRFFLG LC and also to respond to the spillover effects of differential policies on space through interregional correlation. Stock indicators are used to highlight the overall input of the MRFFLG LC resources for the purpose of designing indicators. Natural resource input stock indicators in HP include the supply of several major resource types such as MRFFLG while social and economic input indicators include labor input and public fiscal expenditure. In summary, this article provides a comprehensive system of inputs and outputs for evaluating EE.
3.2.3 Method for spatial evaluation of ecological efficiency
In this study, the standard deviation ellipse method and the convergence analysis method are used to analyze and evaluate the spatial variation of the EE centroid. They are also used to determine whether there is any spatial convergence of EE based on the MRFFLG LC EE measurements.
3.2.3.1 Evaluation method for the spatial migration model of ecological efficiency centroid
This study utilizes the standard deviation ellipse method to determine how the EE centroid of the MRFFLG LC has migrated and distributed over time in HP between 2006 and 2019. Its advantages over previous research methods are that it overcomes the disadvantages that resulted from the division of administrative regions in previous research methods. An integral part of spatial statistics, it quantitatively describes global and spatial properties, including centrality, dispersion, directionality, spatial morphology, and timely spatial evolution processes within the distribution of the research object (Zhang et al., 2022). Among its advantages is its ability to compare spatial patterns over time within the same dimension and to visualize research indicators for each year in a manner that accurately and intuitively illustrates the main regions and relative trends in the spatial distribution of geographical elements over time.
We calculated the main parameters of the standard deviation ellipse of the EE of the MRFFLG LC in HP from 2006 to 2019 using the ArcGIS measure geographic distribution module to better understand its spatial evolution characteristics and accurately describe its spatial distribution trend. The EE is shown as an ellipse with the EE as the weight, and the general characteristics of the spatial distribution can be observed through changes in the parameters such as centroid, azimuth, major axis, and minor axis (Equation 2). Here is the formula for calculating each of the above parameters:
Azimuth:
X-axis standard deviation:
Y-axis standard deviation:
and are the average centroid coordinates of the elements; is the weight of the study unit; is the orientation angle of the ellipse; and are the central coordinates of the elements in each study area; and are the coordinate deviations of the centroid from the central coordinates in each study unit; and are the standard deviations along the X and Y axes, respectively; and is the total number of elements.
3.2.3.2 Evaluation methods for eco-efficient spatial convergence analysis models
This article employs the β-convergence and β-absolute convergence methods to examine EE convergence in a LC. Spatial convergence models are widely used to examine whether per capita GDP of countries or regions converges or diverges. This approach can also be used to test for EE convergence and divergence across different regions during the sample period. The application of the β-convergence concept is more widely adopted and has stronger policy implications in empirical analysis. Neoclassical growth models assume that all economies have the same technology, but their initial factor endowments differ (Yu et al., 2013). Green spaces have been found to enhance human health and well-being (Wheeler et al., 2015). The control variables in this study included the area of park green spaces per capita, the area of urban roads per capita, the compliance rate with pollution discharges, and the fixed asset investment as variables that impact EE.
β convergence refers to the phenomenon where the rate of EE growth in regions with low EE levels is higher than that in regions with high EE levels. Absolute β convergence requires strict assumptions, such as uniform environmental conditions across evaluation units, including the same fixed asset input and per capita green area. Over time, different regions’ EE will converge to the same level. The testing model for absolute beta convergence is as follows (Equation 3):
T represents the research period, represents the EE of the ecosystem of MRFFLG in the i-th area at the beginning and end of the research, represents the constant term, represents the regression coefficient, and represents the random disturbance term. When <0 and is significant, it indicates that the area with lower EE of the ecosystem of MRFFLG has a larger EE growth rate than the area with higher EE.
If the condition of -convergence holds, the conditional assumption is eliminated, and taking into account the differences between cities in population, income levels, fixed asset investment, per capita green area, and other aspects, the growth rate of the EE of the LC will converge to their respective steady-state levels over time through different growth paths. The testing model for -convergence is as follows (Equation 4):
T represents the research period, represents the EE of the MRFFLG LC in the i-th region at the beginning and end of the study, represents the constant term, represents the regression coefficient, represents the random disturbance term, and represents the regression coefficient of the added j-th control variable. When <0 and is significant, it indicates that the EE of the MRFFLG LC in the study area will converge to their respective stable levels based on their own conditional characteristics, and the difference in EE between different regions will persist in the long term.
Finally, this paper selects the Spatial Durbin Model to test absolute convergence and conditional convergence. Considering the spatial correlation of the study area, a spatial econometric model for the convergence of EE growth in the MRFFLG LC is constructed. The empirical test and analysis of the EE of the MRFFLG LC in HP are carried out, and the model is presented in Equation (5).
In the formula, represents the natural logarithm of the EE growth rate of the MRFFLG LC in the study area from to . and are the parameters to be estimated, represents absolute convergence. is an element in the spatial weight matrix, while and are spatial correlation coefficients, and reflects the influence of adjacent areas on the attribute values of the local area. represents the regression coefficient of the spatial interaction effect, and is the random error term. Based on previous research, this paper selects fixed asset investment, the rate of wastewater discharge compliance, per capita urban road area, and per capita park green area as control variables to determine absolute convergence and conditional convergence. If the coefficient of each control variable is 0, the above model is absolutely convergent, and if is not 0, it is conditionally convergent. The paper examines the level of environmental regulation, the intensity of government macro-control, the level of urban development, and the degree of urban greening.
4 Results
4.1 Results of EE level measurement under the time dimension
Based on the existing social and natural resource inputs, the average EE of the MRFFLG from 2006 to 2019 was only 0.34, suggesting there is still room for improvement. The input–output balance has reached the optimal level when EE is 1, ensuring an effective balance between environmental protection and resource conservation. When the EE is close to 0, it indicates a lack of coordination between economic development and ecological protection because of the imbalance between input and output. HP has a relatively low level of EE. Figure 3 shows the average EE for the MRFFLG in HP during the period 2006 to 2019.
The overall EE level of Heilongjiang Province in 2006–2019 showed an M-shaped fluctuating change, and despite the large level of fluctuation, on the whole, the EE level in recent years has still shown an increase. The level of EE fluctuates substantially under the existing social and natural resource inputs, and 2009, 2011, and 2014 are the 3 years that have seen the greatest growth in EE. Throughout the entire study period, the main years with large changes were 2009, 2011, and 2014, in which a complete cessation of commercial logging of natural forests was implemented in the key state-owned forest areas of HP in 2014, and a pilot project for ecological protection and restoration of MRFFLG was launched in HP after 2018, The EE level in 2019 was about 0.07 higher than that in 2016, and the fluctuation of EE has gradually become smaller since 2016, with the overall level of change showing a steady increase. While around 2011 was the period of implementation of the second phase of the natural forest protection project. It can be seen that with the gradual improvement of China’s ecological protection and restoration actions, the quality of the regional ecological environment has subsequently been improved.
4.2 Evaluation results of EE levels under the spatial dimension
The results show that the highest level of EE is located in the key state-owned forest areas, indicating that the level of EE is relatively higher in forest resource-rich areas. Table 3 shows the characteristics of the spatial distribution of the EE. It is clear from the center of gravity coordinates that the movement direction of the geographic center of gravity of HP’s biotic community in each year indicates the “high density” areas of HP, which vary between 128.75°E to 130.05°E and 46.10°N to 47.08°N, which indicate that HP is a key state-owned forest area. During the study period, there were mainly two stages of significant movement directions among the EE in HP. In particular, the trend was to the northwest during 2006–2008, 2009–2011, and 2015–2018, while it was to the southeast during 2011–2012, 2014–2015, and 2018–2019. Between 2010 and 2013, the EE in HP shifted to the northwest direction from the perspective of the development direction of EE, indicating a greater degree of improvement in EE in the northwest region of HP than in the southeast region during this period.
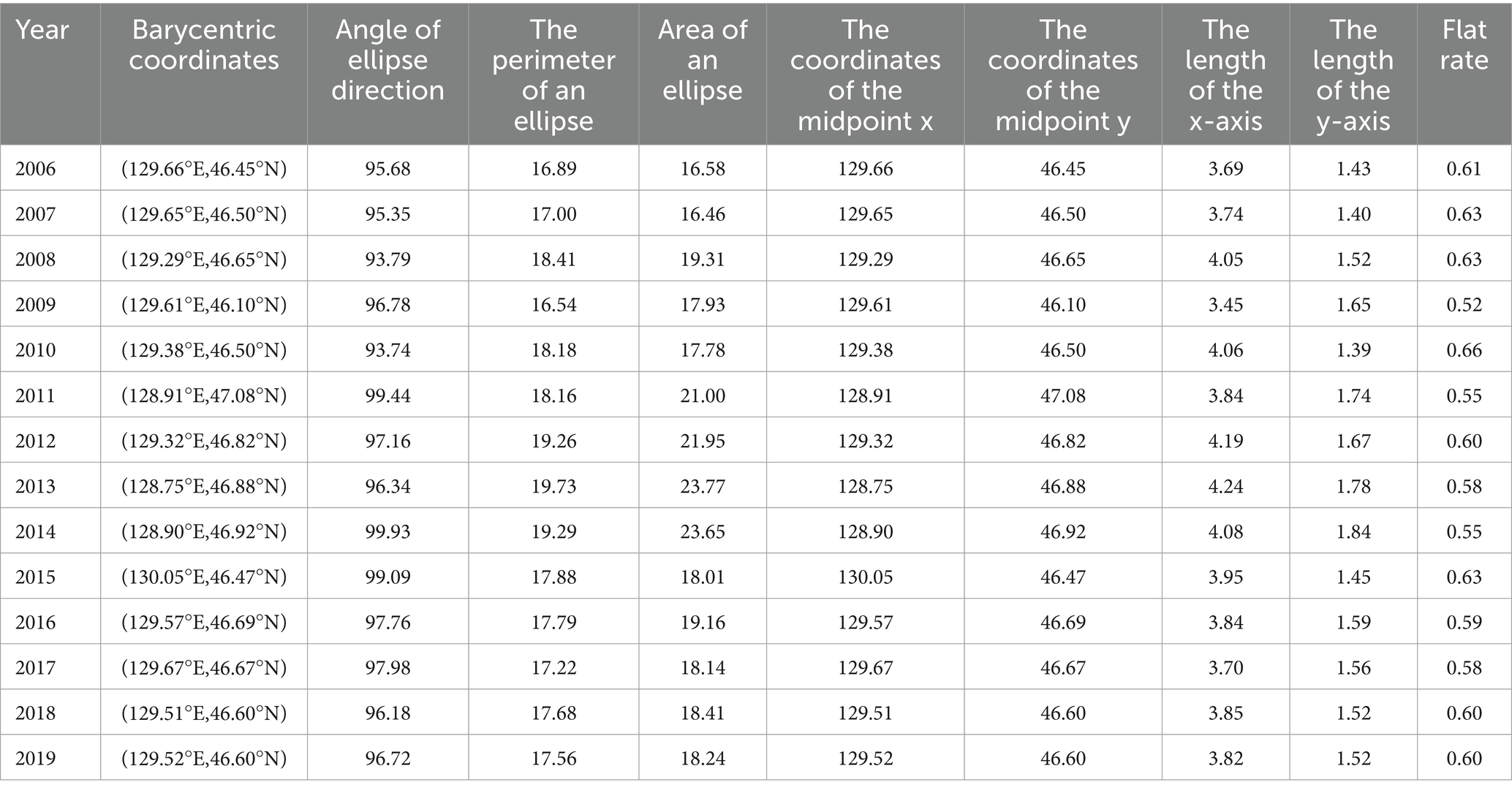
Table 3. Direction and distance of the shifting gravity center of ecological efficiency of (MRFFLG) systems in Heilongjiang Province (HP), China.
The MRFFLG of HP during the study period is not stable enough. The results show that the eastern and southern regions of HP have higher levels of EE. The years with the largest movement of the EE in terms of distance traveled are 2010, 2011, 2014, and 2015 (Figure 4). There was a noticeable shift in the EE between 2010 and 2011, with a transfer direction toward the northwest and a significant offset. In contrast, during the period of 2014–2015, there was a greater change in factors and a greater distance of center of gravity movement. In terms of distance and speed of movement, the EE shifted toward the southeast. In both the north–south and east–west directions, the center of gravity movement distance increased significantly, and the straight-line movement distance also increased significantly. Thus, the EE level in the eastern and southern regions of HP improved more significantly during this period than in the western and northern regions.
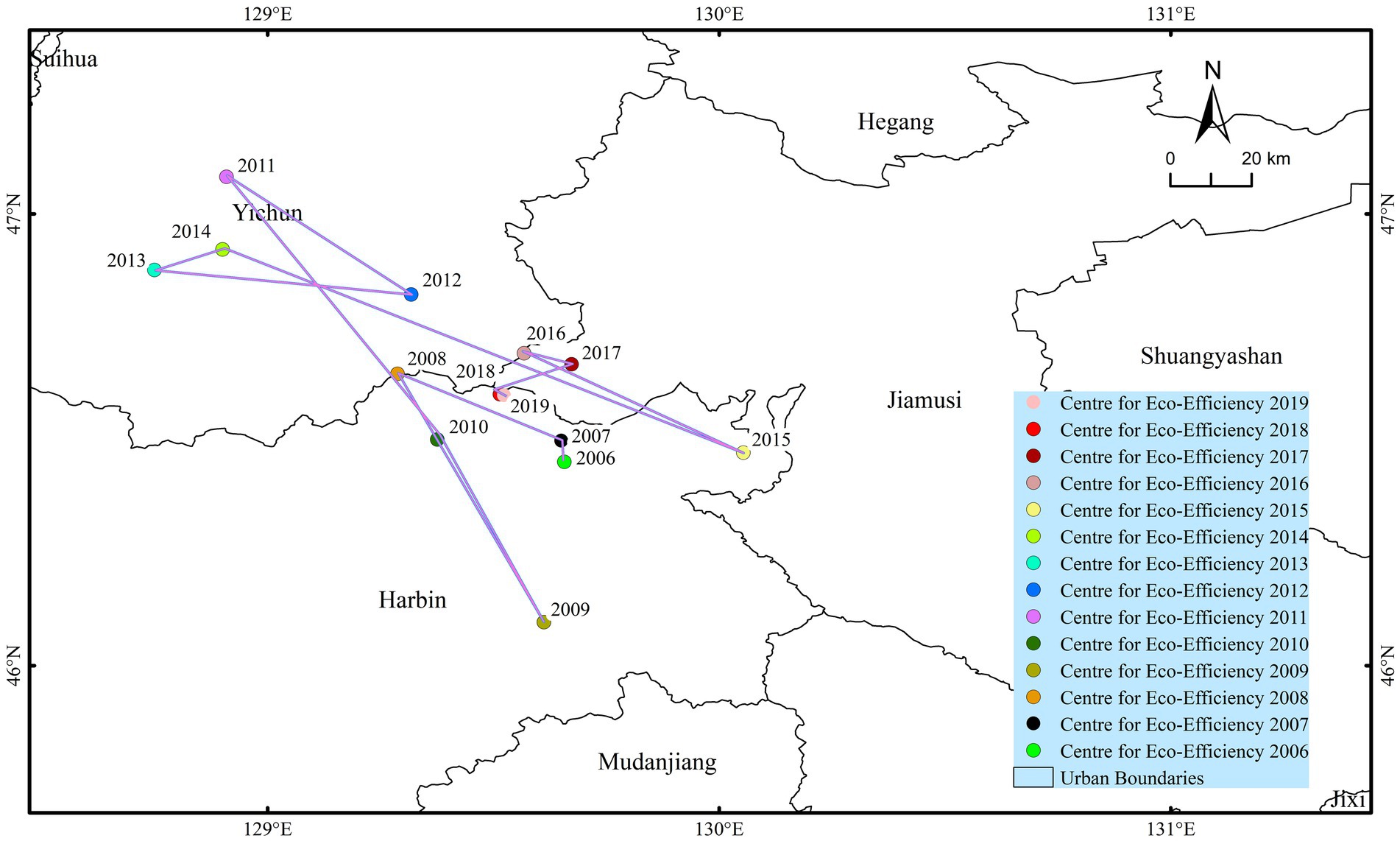
Figure 4. Migration trajectory of the ecological efficiency gravity center of (MRFFLG) systems from 2006 to 2019.
4.3 Results of standard deviation ellipse analysis of EE
The results from the standard deviation ellipse again verify that the spatial distribution pattern of EE levels is not stable enough. EE standard deviation ellipses in HP were mainly located in the southeastern part of the central region from 2006 to 2019 (Figure 5). This larger flattening ratio indicates that the development level of EE is unevenly distributed throughout the region. It was 2010 that had the most directional distribution of the ellipse, while other years did not exhibit much change in the flattening ratio. From 2006 to 2019, the area of the standard deviation ellipse generally increased first and then decreased. Over the years 2006 to 2014, the circumference and area of the standard deviation ellipse gradually increased and then gradually decreased. Additionally, the variation in the long and short semiaxes was also increasing in the beginning and then decreasing in the end, indicating that spatial agglomeration characteristics have gradually become more evident in the east–west direction since 2014, while the degree of spatial agglomeration has been gradually increasing in the north–south direction. In 2013 and 2014, the ellipse coverage area was significantly larger than in previous years, while in 2006 and 2007, the ellipse coverage area was the smallest. EE ranges in 2018 and 2019 were higher than those in 2006 and 2007. The rotation of the azimuth angle showed a large variation. In 2011 and 2014, the azimuth angle increased by 5.70° and 3.59°, respectively. This indicates that the spatial distribution pattern of EE is deviating in a noticeable manner. The spatial distribution pattern of EE in HP shifted from southeast to northwest by 5.70° in 2011, and from northwest to southeast by 3.59° in 2014 and 2015.
In the MRFFLG in southern cities of HP, the average EE of the LC is higher than that in northern cities based on the spatial distribution pattern and movement path of the EE center of the LC during the research period. In general, the spatial distribution of EE in the MRFFLG of HP exhibits a northwest-to-southeast pattern and a tendency to shift toward the southeast. Initially, the length of the X semi-axis decreases from the length of the major axis of the standard deviation ellipse, then increases, decreases again, and increases again, indicating that the EE of the LC in the MRFFLG of HP continues to vary. It appears that dispersion first occurs, followed by agglomeration, then dispersion again, followed by agglomeration. It can be seen that the length of the Y semi-axis increases and decreases over time, suggesting that the spatial distribution pattern of the EE center of the LC in the MRFFLG of HP during the study period is not stable enough, which indicates that the spatial spillover effect has not yet become apparent.
4.4 Results of spatial convergence analysis
The existence of spatial convergence pattern of EE of MRFFLG in HP was verified by convergence analysis method. First, the convergence analysis indicates that EE has reached absolute convergence in the MRFFLG research area of HP, which means that the levels of EE in the various research areas will eventually reach a steady state level. The second effect is that constraints on fixed asset investments and an increase in investments in environmental optimization have the potential to enable regions with lower EE to achieve relatively higher growth rates. As a result, the EE gap between regions is likely to gradually decrease in the future.
As shown in Table 4, all convergence models for AEEit have significant coefficients of negative convergence, and most of them pass the significance level test at 1%. The conditional β-convergence results in Table 4 indicate that overall, EE in the MRFFLG region of HP exhibits significant conditional convergence under the joint influence of environmental regulation, government macro-control, urban development level, and resource endowment level. In other words, EE will show a trend of annual decline with an increase in environmental regulations, an improvement in government macro-controls, an acceleration in urbanization, and changes in the endowment of resources per capita. It is worth mentioning that the amount of investment in fixed assets passed the negative significance test, indicating that the amount of investment in fixed assets has a negative impact on the improvement of EE. Among the items of fixed asset investment, the top ones according to the proportion from high to low are manufacturing industry, real estate industry, transportation, storage and postal industry and so on. It can be seen that some inputs that need to rely on consuming a lot of resources to promote industrial development may have a negative impact on EE. On the other hand, the increase of parks and green areas per capita has a promoting effect on the regional EE, indicating that the optimization of the ecological environment and the increase of public green areas can help to improve the Eco-output.
5 Discussion
5.1 The EE levels from a socio-economic perspective
The problem of high evaluation indexes is corrected by the super-efficiency SBM model, and the results show that the overall EE level of HP is low, and Analyzing the overall situation, the average value of the ecological efficiency of MRFFLG in the 11 cities of HP during the period of 2006–2019 is 0.34, which means that there is still a lot of room for improvement of the ecological efficiency with the existing inputs of social and natural resources, which indicates that the imbalance of inputs and outputs has led to the lack of a better coordinated development between economic development and ecological protection, and the EE of many cities in different periods still has obvious differences and shows certain spatial heterogeneity.
From the time distribution of the mean value of ecological efficiency of MRFFLG in HP, the overall fluctuating trend shows a large fluctuation trend, and the years in which changes in ecological efficiency have been substantially improved are 2009 (0.351), 2011 (0.532) and 2014 (0.483). A circular economy perspective reveals that the EE of an ecosystem reflects a separation between economic growth and environmental pressure (De Pascale et al., 2021; Liu et al., 2022). HP environmental pressures are still relatively high and cannot fully accommodate economic growth. Moreover, it falls within the area of ineffective EE in the ecosystem. The highest value recorded in previous years was only 0.523, indicating that ecological resources are not adequately converted into tangible advantages. Sustainable development in the region is threatened by ecological resource loss. Despite significant improvements in EE levels over the past 2 years (2011 and 2014), significant fluctuations have been observed in the overall trend. The reason is that China’s constraints on resource protection have been alleviated by some forestry subsidy funds. This has temporarily improved the HP ecosystem’s ecological efficiency. With time, policy investment and implementation will inevitably reach a point of fatigue, and EE will decrease until a new round of financial investment or strong policy constraints will once again lead to a significant increase in HP’s ecosystem’s EE in the short term. Clearly, this illustrates the dependence on government regulation and fund investment, which are unpredictable.
5.2 Spatial variation in the center of gravity of EE
From the coordinates of the geographic center of gravity of the EE on the map, the movement of the geographic center of gravity in each year points to the “high-density” part of the ecological efficiency, which varies between 128.75°E ~ 130.05°E, 46.10°N ~ 47.08°N. Although the center of gravity shows an irregular trajectory, its main moving range is located in the key state-owned forest area of HP. In terms of moving distance, the years with larger moving amplitude were in 2010, 2011, 2014, and 2015, which corresponded to the end of the first phase of the Practice and Prospect for Natural Forest Protection Projects, the start of the second phase of the natural forest protection project, and the implementation of the policy of stopping commercial logging of natural forests in the key state-owned forest areas of HP, respectively. The center of gravity moved the largest distance and the fastest speed from 2014 to 2015, and the magnitude of elemental changes was more obvious, indicating that the full cessation of commercial logging of natural forests in key state-owned forest areas of HP produced a relatively large spatial difference in the EE of the community of life of MRFFLG.
Over time, EE levels and ER investment funds are related. EE levels in the ecosystem show similar trends due to national investments in ecological protection and restoration funds. China is expected to increase its investment in ecological protection and restoration funds for forestry. This will directly affect the MRFFLG ecosystem. It is anticipated that this will result in an increase in EE levels. Consequently, the ecosystem of MRFFLG will be improved, making it more sustainable. The above results indicate that the government’s centralized investment in ecological protection and restoration has achieved positive results. In addition, the implementation of ecological protection and restoration actions concentrated in localized areas has led to large spatial differences in the overall level of EE in the study sample areas. Although the level of EE in HP has continued to improve, cities with lower levels of EE face challenges such as high investment in ecological resources, insufficient expected outputs, and excessive unintended outputs. The spatial distribution pattern of regional EE centers in HP shows a northwest-southeast bias. The spatial spillover effect has not yet appeared.
5.3 Characteristics of spatial convergence of EE
The MRFFLGs LCs in HP will eventually reach a steady-state level of EE, and regions with lower EE will experience relatively faster growth rates. It has been confirmed that the EE of the MRFFLGs LC in HP has passed the convergence test. Even though there are significant regional differences in the EE level of the LC, fixed asset investment has passed the negative significance test. This indicates that fixed asset investment negatively affects EE. Increasing per capita park and green space area through fixed asset investments promotes regional-EE. It is expected that the EE gap between regions will gradually narrow in the future.
Nevertheless, if fixed asset investments are increased in industries with high resource and pollution consumption, regional EE may be reduced although the overall level of EE in MRFFLGs LC in HP is low, places with significant levels of EE can provide experiences and references for places with lower levels of EE, including better government regulations and pollution limits.
According to the theory of technology transfer and diffusion, the greater the technological gap between different regions, the greater their technological reserves, and the diffusion of new products or technologies as well as cooperative innovations will lead to the rapid diffusion of technological advances and related experiences among different regions. This kind of experience imitation is less costly than spontaneous innovation, and the possibility of successful realization is higher. Therefore, regions with backward level of eco-efficiency will accumulate more experience imitation of eco-efficiency progress, and thus show faster improvement in practice, and can rapidly improve the eco-efficiency of their own ecological community of life in the MRFFLG by imitating the policies or measures of the neighboring regions at a low cost. Moreover, the ability of these regions to emulate and learn from each other is likely to grow over time, reducing the cost of borrowing more complex technologies, and may itself create “social capacity” in the development process, thus maintaining the level of eco-efficiency up to a certain level of sustainability and stability.
5.4 Research outlook
In the existing research on ecological restoration, the number of social science-based research fields is far lower than that of Science Technology, Physical Sciences and Life Sciences Bio-medicine research heat. As restoration ecologists increasingly respond to changes in large-scale ecosystems, a broader range of stakeholders will be included in the ecological restoration process, advancing the need for interdisciplinary communication. In the process of integrated ecosystem management there are bound to be conflicting contradictions between development and utilization and resource protection, and determining ecological protection and restoration management goals is a process that requires trade-offs. Assessing and managing new ecosystems from a more holistic perspective and considering socioeconomic factors that may have an impact on them will be worthwhile research in the future. Integrated ecosystem management inevitably involves conflicts between resource development and utilization and ecological protection and restoration. Managing both economic development and ecological protection is a multi-objective decision-making process, and different land use types have different ecosystem service functions. Currently, most research is focused on the relationship between changes in resource utilization types and ecosystem service functions, with little attention paid to the impact of alternative land use combinations. Research can be conducted in the future to investigate the relationship between the proportion of different resource utilization types and the improvement of ecosystem services.
6 Conclusion
In this study, the level of EE in HP was measured and evaluated. It presents a theoretical framework for evaluating ER based on multi-objective production and multi-factor inputs. EE indicators for ecological and socioeconomic aspects are included in the framework for assessing life communities’ EE. To provide a reference for constructing an evaluation index system of ecological restoration efficiency that also includes socioeconomic dimensions. The research findings that: (1) The overall EE level is generally low, with the highest value of 0.523 in all the years, and most of the time Heilongjiang Province still belongs to the EE non-effective region, but in recent years it has begun to show a steady upward trend. (2) The spatial distribution of the EE in HP demonstrates a northwest-southeast pattern. The pattern was not stable enough during the research period, and spatial spillover effects were not significant. (3) The implementation and enforcement of policies related to ecological protection and restoration in China have significantly promoted the improvement of EE. The migration trajectory of the center of gravity of ecological efficiency shows that its main moving range is located in the key state-owned forest area of HP, and during the implementation of the natural forest protection project. (4) There are obvious differences in the EE levels of different types of cities, with resource-mature cities generally higher. (5) There is spatial convergence of EE levels in HP. In the future, regions with lower EE levels will have relatively higher growth rates, so that the EE gap between regions will gradually narrow in the future.
Research results have important implications for regional ecological protection and restoration, enrich theoretical frameworks and feasible paths for assessing the operational efficiency of the MRFFLG system both theoretically and practically, and serve as valuable references for the practice of protecting, restoring, and governing ecological systems. Most of the current research focuses on the impact of resource utilization type shift on the relationship between ecosystem service functions, while there are fewer studies on the impact of the combination of different land categories, and in the future, we can consider increasing the empirical research on the relationship between the enhancement of ecosystem service functions under the study of the weight of different resource utilization types. On the other hand, due to the limitations of data availability and the study area and period, the effects of some factors are not yet significant; with the deepening of the ecological protection and restoration process, a longer-term study can be carried out through continuous follow-up surveys.
Data availability statement
Publicly available datasets were analyzed in this study. This data can be found here: In order to obtain the HP 2019 elevation data (Digital Elevation Model, DEM), the Geospatial Data Cloud website (https://www.gscloud.cn/) sourced the SRTMDEM 90M original elevation data; ESACCI (European Space Agency Climate Change Institute) provided the land use and land cover data from 2006 to 2019).
Author contributions
YR: Data curation, Funding acquisition, Investigation, Methodology, Software, Visualization, Writing – original draft, Formal analysis, Resources, Validation, Writing – review & editing. MA: Formal analysis, Methodology, Supervision, Validation, Writing – review & editing. YC: Conceptualization, Formal analysis, Funding acquisition, Methodology, Project administration, Supervision, Validation, Writing – review & editing. SZ: Data curation, Methodology, Software, Supervision, Validation, Writing – original draft, Writing – review & editing.
Funding
The author(s) declare financial support was received for the research, authorship, and/or publication of this article. This research was funded by the following three programs: National Social Science Foundation Youth Program (23CGL063): Study on the Path of Enhancing the Efficiency of Ecological Protection and Restoration in State-owned Forest Areas by Digital Empowerment; Heilongjiang Postdoctoral Fund (LBH-Z23052): Digital-enabled multi-subject multi-span synergistic governance mechanism for mountain-river-forest-farmland-lake-grassland in Heilongjiang province; Youth Fund for Humanities and Social Sciences Research, Ministry of Education (23YJC790036): Study on Dynamic Spatial Correlation, Synergistic Effect and Path Optimization of Pollution and Carbon Reduction in Urban Agglomerations.
Acknowledgments
We acknowledge various sources of financial and institutional support, especially the National Social Science Foundation Youth Program (23CGL063) and Humanities and Social Sciences Research, Ministry of Education (23YJC790036). With special thanks to the Northeast Forestry University Modern Forestry and Carbon Sink Economy Development Research Center Think Tank for their support.
Conflict of interest
The authors declare that the research was conducted in the absence of any commercial or financial relationships that could be construed as a potential conflict of interest.
Publisher’s note
All claims expressed in this article are solely those of the authors and do not necessarily represent those of their affiliated organizations, or those of the publisher, the editors and the reviewers. Any product that may be evaluated in this article, or claim that may be made by its manufacturer, is not guaranteed or endorsed by the publisher.
Footnotes
References
Abhilash, P. C. (2021). Restoring the unrestored: strategies for restoring global land during the UN decade on ecosystem restoration (UN-DER). Land 10:201. doi: 10.3390/land10020201
Behrens, A., Giljum, S., Kovanda, J., and Niza, S. (2007). The material basis of the global economy. Worldwide patterns of natural resource extraction and their implications for sustainable resource use policies. Ecol. Econ. 64, 444–453. doi: 10.1016/j.ecolecon.2007.02.034
Bi, W., Wang, K., Weng, B., Yan, D., and Liu, S. (2021). Does the returning farmland to forest program improve the ecosystem stability of rhizosphere in winter in alpine regions? Appl. Soil Ecol. 165:104011. doi: 10.1016/j.apsoil.2021.104011
Chazdon, R. L., and Uriarte, M. (2016). Natural regeneration in the context of large-scale forest and landscape restoration in the tropics. Biotropica 48, 709–715. doi: 10.1111/btp.12409
Cui, W., Liu, J., Jia, J., and Wang, P. (2021). Terrestrial ecological restoration in China: identifying advances and gaps. Environ. Sci. Eur. 33, 1–14. doi: 10.1186/s12302-021-00563-2
De Pascale, A., Arbolino, R., Szopik-Depczyńska, K., Limosani, M., and Ioppolo, G. (2021). A systematic review for measuring circular economy: the 61 indicators. J. Clean. Prod. 281:124942. doi: 10.1016/j.jclepro.2020.124942
Ding, J. Y., and Zhao, W. W. (2014). Progress and prospects on evaluation of ecological restoration: a review of the 5th world conference on ecological restoration. J. Appl. Ecol. 25, 2716–2722. doi: 10.13287/j.1001-9332.2014.0217
Edrisi, S. A., and Abhilash, P. C. (2021). Need of transdisciplinary research for accelerating land restoration during the UN decade on ecosystem restoration. Restor. Ecol. 29:e13531. doi: 10.1111/rec.13531
Erbaugh, J. T., Pradhan, N., Adams, J., Oldekop, J. A., Agrawal, A., Brockington, D., et al. (2020). Global forest restoration and the importance of prioritizing local communities. Nat. Ecol. Evol. 4, 1472–1476. doi: 10.1038/s41559-020-01282-2
Gann, G. D., McDonald, T., Walder, B., Aronson, J., Nelson, C. R., Jonson, J., et al. (2019). International principles and standards for the practice of ecological restoration. Restor. Ecol. 27, S1–S46. doi: 10.1111/rec.13035
Gao, J., and Yang, Z. (2015). Restoration of ecological functions: goal and orientation of ecological restoration in China. J. Ecol. Rural Environ. 31, 1–6.
Gao, M., Hu, Y., and Bai, Y. (2022). Construction of ecological security pattern in national land space from the perspective of the community of life in mountain, water, forest, field, lake and grass: a case study in Guangxi Hechi, China. Ecol. Indic. 139:108867. doi: 10.1016/j.ecolind.2022.108867
Grand, M. C. (2016). Carbon emission targets and decoupling indicators. Ecol. Indic. 67, 649–656. doi: 10.1016/j.ecolind.2016.03.042
Higgs, E. (2003). Nature by design: people, natural process, and ecological restoration. Massachusetts Institute of Technology.
Hira, A., Arif, M., Zarif, N., Gul, Z., Xiangyue, L., and Yukun, C. (2023). Effects of riparian buffer and stream channel widths on ecological indicators in the upper and lower Indus River basins in Pakistan. Front. Environ. Sci. 11:1113482. doi: 10.3389/fenvs.2023.1113482
Hitchens, D.M., Clausen, J., and Fichter, K. (1998). World business council for sustainable development-WBCSD/international environmental management benchmarks, Schritte zum nachhaltigen Unternehmen. Springer, Berlin.
Huang, L., Zhang, Y., Hong, Z., and Xu, X. (2023). Influencing factors on ecological efficiency: based on 11 cities in Zhejiang Province. IEAM 19, 139–151. doi: 10.1002/ieam.4610
Hukkinen, J. (2001). Eco-efficiency as abandonment of nature. Ecol. Econ. 38, 311–315. doi: 10.1016/S0921-8009(01)00217-8
Ives, C. D., Giusti, M., Fischer, J., Abson, D. J., Klaniecki, K., Dorninger, C., et al. (2017). Human–nature connection: a multidisciplinary review. Curr. Opin. Environ. Sustain. 26-27, 106–113. doi: 10.1016/j.cosust.2017.05.005
Kondo, Y., and Nakamura, S. (2005). Waste input-output linear programming model with its application to eco-efficiency analysis. Econ. Syst. Res. 17, 393–408. doi: 10.1080/09535310500283526
Laudari, H. K., Aryal, K., Maraseni, T., Pariyar, S., Pant, B., Bhattarai, S., et al. (2022). Sixty-five years of forest restoration in Nepal: lessons learned and way forward. Land Use Policy 115:106033. doi: 10.1016/j.landusepol.2022.106033
Liu, J. G., Cui, W. H., Tian, Z., and Jia, J. L. (2020). Stepwise ecological restoration theory. Chin. Sci. Bull. 66, 1014–1025. doi: 10.1360/TB-2020-1128
Liu, X., Arif, M., Wan, Z., and Zhu, Z. (2022). Dynamic evaluation of coupling and coordinating development of environments and economic development in key state-owned forests in Heilongjiang Province, China. Forests 13:2069. doi: 10.3390/f13122069
Li, W., Chen, J., and Zhang, Z. (2020). Forest quality-based assessment of the returning farmland to Forest program at the community level in SW China. For. Ecol. Manag. 461:117938. doi: 10.1016/j.foreco.2020.117938
Mao, D., He, X., Wang, Z., Tian, Y., Xiang, H., Yu, H., et al. (2019). Diverse policies leading to contrasting impacts on land cover and ecosystem services in Northeast China. J. Clean. Prod. 240:117961. doi: 10.1016/j.jclepro.2019.117961
Moyo, H., Slotow, R., Rouget, M., Mugwedi, L., Douwes, E., Tsvuura, Z., et al. (2021). Adaptive management in restoration initiatives: lessons learned from some of South Africa’s projects. S. Afr. J. Bot. 139, 352–361. doi: 10.1016/j.sajb.2021.03.016
Oppenheimer, J. D., Beaugh, S. K., Knudson, J. A., Mueller, P., Grant-Hoffman, N., Clements, A., et al. (2015). A collaborative model for large-scale riparian restoration in the western United States. Restor. Ecol. 23, 143–148. doi: 10.1111/rec.12166
Pandit, R., Parrotta, J. A., Chaudhary, A. K., Karlen, D. L., Vieira, D. L. M., Anker, Y., et al. (2020). A framework to evaluate land degradation and restoration responses for improved planning and decision-making. Ecosyst. People 16, 1–18. doi: 10.1080/26395916.2019.1697756
Qu, S., Wang, L., Lin, A., Yu, D., Yuan, M., and Li, C. (2020). Distinguishing the impacts of climate change and anthropogenic factors on vegetation dynamics in the Yangtze River Basin, China. Ecol. Indic. 108:105724. doi: 10.1016/j.ecolind.2019.105724
Rey Benayas, J. M., Newton, A. C., Diaz, A., and Bullock, J. M. (2009). Enhancement of biodiversity and ecosystem services by ecological restoration: a meta-analysis. Science 325, 1121–1124. doi: 10.1126/science.1172460
Shan, Y., Guan, Y., Hang, Y., Zheng, H., Li, Y., Guan, D., et al. (2022). City-level emission peak and drivers in China. Sci. Bull. 67, 1910–1920. doi: 10.1016/j.scib.2022.08.024
Tone, K. (2001). A slacks-based measure of efficiency in data envelopment analysis. Eur. J. Oper. Res. 130, 498–509. doi: 10.1016/S0377-2217(99)00407-5
Wang, L., Li, Z., Wang, D., Chen, J., Liu, Y., Nie, X., et al. (2021). Unbalanced social-ecological development within the Dongting Lake basin: inspiration from evaluation of ecological restoration projects. J. Clean. Prod. 315:128161. doi: 10.1016/j.jclepro.2021.128161
Wang, N., Zhu, P., Zhou, G., Xing, X., and Zhang, Y. (2022). Multi-scenario simulation of land use and landscape ecological risk response based on planning control. International Journal of Environmental Research and Public Health, 19:14289.
Wang, X., Shao, S., and Li, L. (2019). Agricultural inputs, urbanization, and urban-rural income disparity: Evidence from China. China Economic Review, 55, 67–84.
Wheeler, B. W., Lovell, R., Higgins, S. L., White, M. P., Alcock, I., Osborne, N. J., et al. (2015). Beyond greenspace: an ecological study of population general health and indicators of natural environment type and quality. Int. J. Health Geogr. 14:17. doi: 10.1186/s12942-015-0009-5
Worlanyo, A. S., and Jiangfeng, L. (2021). Evaluating the environmental and economic impact of mining for post-mined land restoration and land-use: a review. J. Environ. Manag. 279:111623. doi: 10.1016/j.jenvman.2020.111623
Wortley, L., Hero, J. M., and Howes, M. (2013). Evaluating ecological restoration success: a review of the literature. Restor. Ecol. 21, 537–543. doi: 10.1111/rec.12028
Xu, J., Yin, R., Li, Z., and Liu, C. (2006). China’s ecological rehabilitation: unprecedented efforts, dramatic impacts, and requisite policies. Ecol. Econ. 57, 595–607. doi: 10.1016/j.ecolecon.2005.05.008
Yang, Z. P., Gao, J. X., Zhou, K. X., Zheng, H., Wang, Y., and Li, H. M. (2013). Evaluation of ecological restoration: research progress. Chinese J. Ecol. 32:2494. doi: 10.13292/j.1000-4890.2013.0344
Yin, H., and Cao, Y. (2022). Test on the policy effect of natural forest protection project using double difference model from the perspective of forestry total factor productivity. Math. Probl. Eng. 2022, 1–7. doi: 10.1155/2022/9800727
Yu, Y., Chen, D., Zhu, B., and Hu, S. (2013). Eco-efficiency trends in China, 1978-2010: decoupling environmental pressure from economic growth. Ecol. Indic. 24, 177–184. doi: 10.1016/j.ecolind.2012.06.007
Zhang, X., Liu, K., Wang, S., Wu, T., Li, X., Wang, J., et al. (2022). Spatiotemporal evolution of ecological vulnerability in the Yellow River Basin under ecological restoration initiatives. Ecol. Indic. 135:108586. doi: 10.1016/j.ecolind.2022.108586
Keywords: Chinese green economy, mixed ecosystems, ecological efficiency, Slack-based measure (SBM) model, standard deviation ellipse, spatial convergence
Citation: Ren Y, Arif M, Cao Y and Zhang S (2024) Pathways to enhance the efficiency of forestry ecological conservation and restoration: empirical evidence from Heilongjiang Province, China. Front. For. Glob. Change. 7:1382198. doi: 10.3389/ffgc.2024.1382198
Edited by:
Gopal Shukla, Uttar Banga Krishi Viswavidyalaya, IndiaReviewed by:
Yongyang Wang, Guangdong University of Technology, ChinaKaushal Singh, Banda University of Agriculture and Technology, India
Copyright © 2024 Ren, Arif, Cao and Zhang. This is an open-access article distributed under the terms of the Creative Commons Attribution License (CC BY). The use, distribution or reproduction in other forums is permitted, provided the original author(s) and the copyright owner(s) are credited and that the original publication in this journal is cited, in accordance with accepted academic practice. No use, distribution or reproduction is permitted which does not comply with these terms.
*Correspondence: Shaopeng Zhang, cGVuZ3pzMTk5NEBuZWZ1LmVkdS5jbg==