- 1College of Life and Environmental Science, Central South University of Forestry and Technology, Changsha, Hunan, China
- 2Huitong National Field Station for Scientific Observation and Research of Chinese Fir Plantation Ecosystem in Hunan Province, Huitong, China
- 3National Engineering Laboratory for Applied Technology of Forestry & Ecology in South China, Changsha, China
- 4Faculty of Science, Central South University of Forestry and Technology, Changsha, Hunan, China
- 5Hunan Forest & Grassland Fire Monitoring, Dispatch and Evaluation Center, Changsha, Hunan, China
Introduction: The behavior of forest fire is a complex phenomenon, and accurate simulation of forest fire is conducive to emergency response management after ignition. In order to further understand the characteristics of forest fire spread and the applicability of WRF-Fire in China, which is a coupled fire-atmospheric wildfire model, this study simulated a high-intensity forest fire event that occurred on October 17, 2022 in Xintian County, southern Hunan Province.
Methods: Based on the fire-atmosphere coupled WRF-Fire model, we used high-resolution geographic information, meteorological observation and fuel classification data to analyze the forest fire behavior. At the same time, the simulation results are compared with the fire burned area observed by satellite remote sensing forest fire monitoring data.
Results: The study found that, the simulated wind speed, direction and temperature trends are similar to the observation results, but the simulated wind speed is overestimated, the dominant wind direction is N, and the temperature is slightly underestimated. The simulated wind field is close to the actual wind field, and the simulation results can show the spatial and temporal variation characteristics of the local wind field under complex terrain while obtaining the high-resolution wind field. The simulated fire burned area is generally overestimated, spreading to the north and southwest compared with the observed fires, but it can also capture the overall shape and spread trend of the fire well.
Discussion: The results show that the model can accurately reproduce the real spread of fire, and it is more helpful to forest fire management.
1 Introduction
Forest fire is one of the most dangerous natural disasters at present (Zhang et al., 2023). Forest fire not only destroys the forest ecosystem, but also causes great loss to life, property and ecological environment (Turco et al., 2019; Sakellariou et al., 2020; Zong et al., 2022). Therefore, it is of great significance to prevent the occurrence of forest fires and control the harm of fires to maintain the sustainable development of social economy. We are also carrying out various efforts and research. Recently, the prediction of forest fires and the scheduling of fire extinguishing resources have attracted the attention of many scholars. Many researchers are committed to establishing accurate forest fire spread prediction models, and guide the formulation of correct fire extinguishing measures (Williams et al., 2013; Jellouli and Bernoussi, 2022; Michael et al., 2022; Xu et al., 2022). However, forest fire spread is a complex process, which involves many chemical changes, and it is difficult to accurately analyze and predict forest fire spread (Muñoz-Esparza et al., 2018).
The main surface forest fire spread models in the world are Rothermel model (USA), McArthur model (Australia), Canadian forest fire spread model and physical wildland fire model. The Rothermel model is a physical model that follows the energy conservation law. It mainly studies the spread of the flame front without considering the continuous combustion of the fire scene (Rothermel, 1972). The McArthur model is a mathematical description of the McArthur’s fire danger meters by Noble I R. It is a quantitative relationship between forest fire spreading speed and various parameters derived from multiple ignition experiments, which belongs to a semi-mechanism and semi-statistical model. Although it can predict fire weather and some important fire behavior parameters, its scope of application is extremely limited, only suitable for grassland and eucalyptus forest (Noble et al., 1980). The Canadian Forest Fire Danger Rating System was developed based on the theory of Canadian forest fire behavior. It is through hundreds of experiments and observations, derived forest fire rate of spread (ROS) equation. It consists of four subsystems: forest fire behavior prediction (FBP), forest fire weather index (FWI), forest fire occurrence prediction (FOP), and fuel moisture (AFM). The spreading speed equations are different for different types of fuels, and each equation is independently dependent on the initial spread index (ISI) (Forestry Canada Fire Danger Group, 1992). The physical wildland fire model is based on the basic physical laws to establish a mathematical representation of fire propagation. The physical laws captures the critical and general behavior of the fire, as well as its interaction with the surrounding environment, and enables predictions of its behavior and spread under arbitrary conditions to be made (Sullivan, 2019). In these four models, the Rothermel model comprehensively considers the forest material, fuel humidity, wind speed and other factors, which has high reference value and has been widely used (Rothermel, 1972). Based on the Rothermel model, the more widely used forest fire spread models include FARSITE (Finney, 1998), BehavePlus (Andrews, 1986, 2007; Heinsch and Andrews, 2010), HFire (Fei and Jimy, 2001; Morais, 2001), and WRF-Fire (Mandel et al., 2011; Kochanski et al., 2021).
The above research work shows that the meteorological conditions as driving factors are provided to the combustion model in an offline form, which cannot characterize the feedback mechanism between the fire field and the environmental field. This directly limits the application of these models in the operational prediction of fire spread, and the coupling of the WRF and the fire spread model (WRF-Fire) solves this problem (Coen et al., 2013; Bakhshaii and Johnson, 2018; Jellouli and Bernoussi, 2022). WRF-Fire uses the Rothermel model with a wide range of applications. The Rothermel model follows the energy conservation law, and the model has a high degree of abstraction. It basically includes all the factors that can affect the fire behavior, and considers various physical and chemical details of forest fire spread (Rothermel, 1972). The WRF-Fire numerical model uses the Anderson fuel model classification method (three Grass and grass-dominated, four Chaparral and shrub fields, three Timber litter and three Slash) (Anderson, 1982). This method measures the physical parameters of 13 types of fuels through experiments, such as different component loads, water content, so as to obtain the combustion heat and determine the spreading speed of the fire line (Coen et al., 2013). WRF-Fire can also homogenize the fuel in space by using the same fuel category for the whole region, so as to change the fuel characteristics and add custom fuel categories. For real data, the spatial heterogeneity of fuel categories can be mapped to the region from the fuel mapping datasets. It can set the number of ignitions, time, location and shape, and the moisture content of the fuel, which is an important factor affecting fire behavior (Jiménez et al., 2018; Li et al., 2022). Based on this model system, a series of research results have been obtained. Chang-Bok Rim et al. demonstrated the use of WRF-Fire model to establish a real-time forest fire forecasting system and its application potential in a certain area of North Korea based on the existing conditions and the meteorological conditions of the fire monitoring station (Rim et al., 2018). Beezley et al. simulated the forest fire process in Colorado, indicating that the model has the ability to simulate the spread of real fire (Beezley et al., 2010). José et al. evaluated the influence of input values on the accuracy of WRF-Fire simulation by simulating fire behavior in Spain (José et al., 2014). The results confirmed that the use of accurate meteorological data and fuel moisture content model is the key to accurately simulate fire behavior. Dobrinkova et al. simulated the forest fire process in Bulgaria, and the results show that WRF-Fire has greatly improved the simulation of forest fires when the data is not very sufficient (Dobrinkova et al., 2010). High spatial resolution fuel classification data is the basis for quantitative simulation and prediction of fire spread. For example, the United States has publicly released basic database with 30 m spatial resolution fuel classification, and constantly updated.1 However, there is still a lack of fuel type standards and basic databases at the national and regional levels in China (Liu et al., 2018). At the same time, there are few reports on the interaction between fire and meteorological conditions.
Therefore, in order to improve the accuracy of forest fire spread prediction, this paper utilizes high-resolution surface cover data mapped with Anderson fuel model, and then determines specific fuel type based on the survey data of forest fuel fixed sample plots in the hilly area of southern Hunan. The WRF-Fire numerical model is used to study the spread process of Xintian forest fire, which provides theoretical support and technical guidance for forest fire spread prediction and the suppression of fires in the hilly area of southern China.
2 Materials and methods
2.1 The study region
Xintian is a county under the jurisdiction of Yongzhou City, Hunan Province. It is located between 112°02′ ~ 112°23′E and 25°40′ ~ 26°06′N, with a total area of 1022.4 km2. Xintian is located in the northern part of the Nanling Mountains, and the terrain is low, with an altitude of 200–1,080 m. The county is surrounded by mountains, the terrain is high in the northwest and low in the southeast, and the mountainous area accounts for 36.56%. The county is rich in forest resources, and the dominant tree species on the surface are mainly Pinus massoniana Lamb. with more oil, and the forest coverage rate is 55.60% (Qiu et al., 2022). Xintian is a subtropical continental monsoon climate, the annual average sunshine hours are between 1384.1 ~ 1688.0 h. The spring forest fire prevention period is from March to June each year, and the autumn forest fire prevention period is mainly from September to November each year. During this period, the climate is dry, and the airflow field is complex. Due to the frequent occurrence of historical fires, we simulated the spread process of the ‘10·17’ fatal forest fire in Xintian. The forest fire was reported at 16: 00 on October 17, 2022, and the safety of surrounding human beings was seriously threatened. The forest fire was successfully extinguished on October 25.
The forest fire area was continuously dry and rainless, the surface vegetation and leaves were dry, and the wind speed was high in the early stage; after the fire occurs, the spread speed was fast, the local terrain is complex, and burning points is many, thus resulting in huge losses. Based on Sentinel-2 satellite data2 as monitoring scene, we used 4, 3, 2 band RGB synthetic images to verify the shape of the burned area. Sentinel-2 is a sun-synchronous orbit satellite with a revisit period of 5 days, covering 13 spectral bands. The ground resolutions of Sentinel-2 satellite data are 10 m, 20 m and 60 m, respectively (Liu et al., 2023).
2.2 Description of the Xintian fire event
On 17rd of October 2022, a forest fire broke out in the Yao nationality township (southern Hunan), under the Xintian County, Yongzhou City, Hunan Province, under the conditions of strong northerly wind flow, high temperature and low relative humidity. According to the National Meteorological Science Data Center,3 wind speeds were more than 7 m s−1 in several locations of Yongzhou that day. At approximately 0730 UTC, a forest fire was reported in Banziqiao village (Figure 1), in the northern part of Xintian County. The local settlements were evacuated, but despite the efforts of the Fire Service, the fire was active until the late hours of the 26th of October 2022, totally burning two settlements and partially another one.
About 2 hours later, at 0940 UTC on 17 October, 2022, a fire spot was found in the settlement of Banziqiao village of Jiufeng Mountain. The gusts recorded by the automatic weather station (AWS) in Yongzhou (near the ignition point of the fire event) reached 5 m s−1 between 0730 and 0930 UTC. It quickly became obvious that the people were unable to operate due to the strong wind field. Under such extreme wind conditions, the fire quickly spread southward. In just 2 days, the fire spread to several surrounding towns. In addition, due to the drought in Hunan Province during this period, as well as its unique fuel types (mainly coniferous mixed forests, such as Cunninghamia lanceolata (Lamb.) Hook. and Pinus massoniana Lamb.), assisted the intensification of the event. At about 0100 UTC on 21 October, the fire on the mountain in Xintian County has been roughly extinguished, and requiring firefighters to go up the mountain to extinguish the small sparks and prevent re-ignition. At 0142 UTC fire rekindled and caused two lives. The fire was completely extinguished on 26 October, 2022, the total burned area of Xintian fire was observed to be 10520.6 ha, according to the Fire Department.
2.3 The fire prediction model
2.3.1 Model description
WRF (Weather Research and Forecast) is a new generation of non-hydrostatic balanced, high-resolution mesoscale numerical weather model system,4 developed by the US National Centers for Environmental Prediction (NCEP) and the National Center for Atmospheric Research (NCAR). It is widely used in regional and global weather, climate simulation research and operational forecasting. In the calculation process of WRF model, firstly, the static terrain data and meteorological data need to be interpolated to the established calculation grid, and the initial data and boundary data needed by the main module are generated by the real process. Then, the main module program is run to perform integral operation on the set research area and the physical process adopted, and the calculation results are output. Finally, the post-processing software is used to process and analyze the simulation results (Michalakes et al., 2001; Skamarock et al., 2005, 2008). Since version 3.2 (released in April 2010), WRF couples the fire spread model (WRF-Fire), which can simulate the occurrence of forest fire and dynamic feedback with the atmosphere, realizing the two-way feedback between fire and weather field (Coen and Schroeder, 2015). WRF-Fire is a wildland fire physics module within the WRF model that allows explicit treatment of the effects of fire on atmospheric dynamics and feedback to fire behavior; thus the simulated fire can “create its own weather.” The properties of surface fuels, local altitude and wind speed determine the speed and direction of fire propagation, while the release of heat and water vapor into the surrounding air by combustion in turn changes the meteorological elements of the near-ground (Mandel et al., 2011; Kochanski et al., 2013a; Mandel et al., 2014).
WRF-Fire adopts the Rothermel model with a wide range of applications. The Rothermel model (Rothermel, 1972) follows the heat conduction law and is a physical mechanism model based on the energy conservation law. The model is abstract, basically includes all factors that can affect fire behavior, and considers various physical and chemical details of forest fire spread. Its basic idea is that the spread of forest fire is actually the process of continuous ignition of unlit fuels in front of the flame. When the unlit fuels absorb heat and reach the ignition point of fuels, these fuels are ignited (Rothermel, 1972).
2.3.2 Model configuration and parameterization
We used the 4.2 version of the WRF model coupled with the SFIRE model to complete the atmosphere-fire simulation in this study. WRF supports a multi-grid nesting configuration, and the standard coarse-to-fine grid ratio is 3. The coupled atmosphere-fire model is run in only the finest mesh. In this case, it required a great deal of time to generate a forecast. Thus, we used a two-way nesting run configuration. The construction of nested grid domains was shown in Figure 2. The NCEP FNL analysis data of 1° × 1° four times a day are used as the initial field and boundary conditions, and the four model domains with two-way nesting are used with grid spacings of 18 km, 6 km, 2 km and 666.67 m. The fourth layer of the study area terrain data using ASTER GDEM (Advanced Space Borne Thermal Emission and Reflection Radiometer Global Digital Elevation Model), the grid resolution is about 30 m. The detailed configurations of the WRF-Fire modeling domains are given in Table 1. All of the domains are centered at coordinates (112°12′E, 26°3′36″N). There are 41 sigma vertical levels. A 180-s time step is used for the outermost domain. In the innermost grid, the fire mesh is 10 times finer than the atmospheric mesh to allow for gradual heat release into the atmosphere, even if fuel and topography data might not be available at such fine resolution (Mandel et al., 2011).
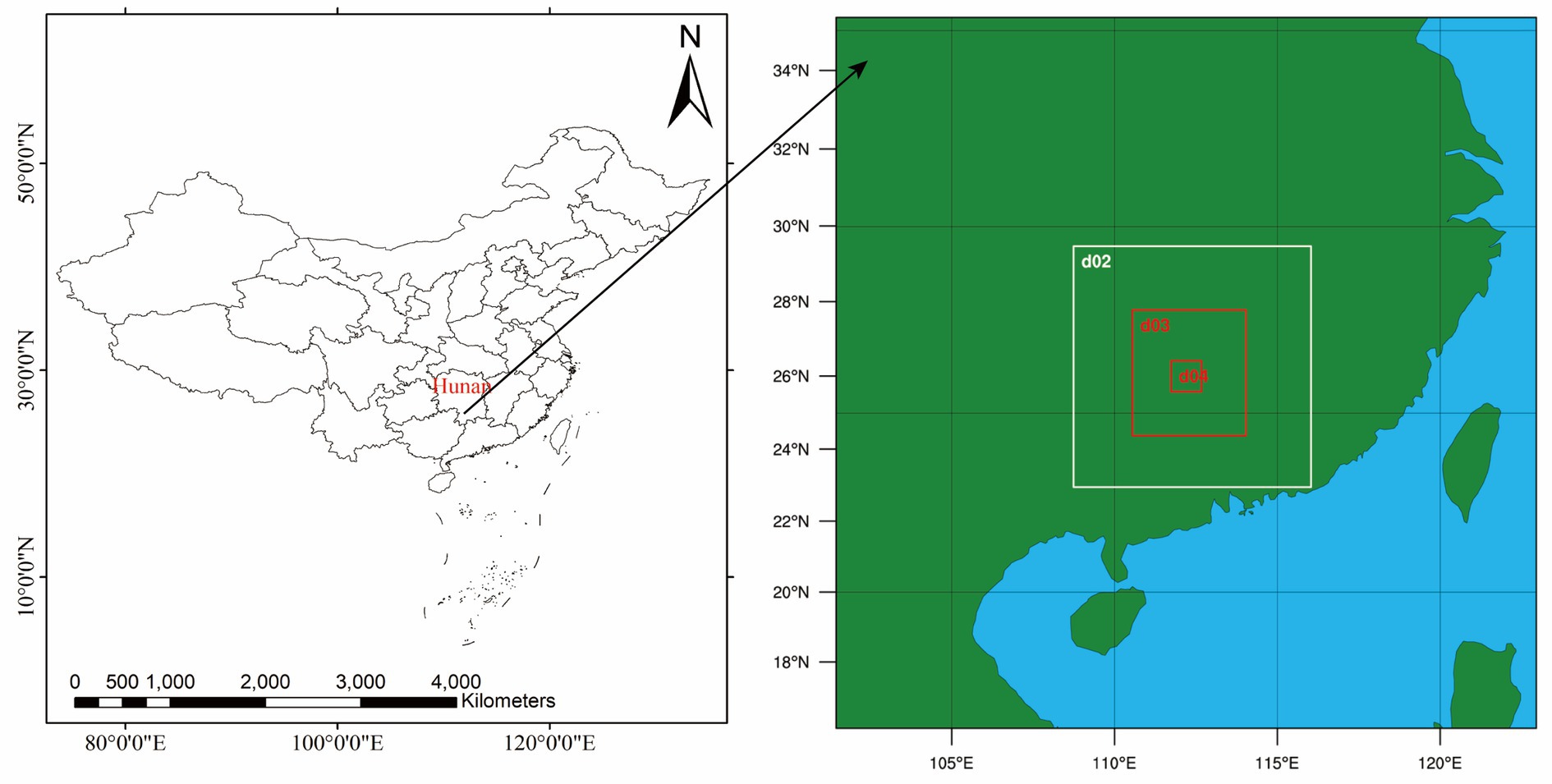
Figure 2. The map showing the nested domain configuration that was used in the WRF-Fire experiments. The configuration has coarsest 18 km grid spacing for the outermost domain1 with other nested domains getting finer by one thirds.
The physical parameterization schemes used in all of the model domains are the Dudhia shortwave radiation (Dudhia, 1989), rapid radiative transfer model (RRTM) longwave radiation (Mlawer et al., 1997), WRF Single-Moment 6-class (WSM6) microphysics (Hong et al., 2004), Monin-Obukhov near-surface layer scheme (Janji, 1994), Noah land-surface scheme (Fei and Jimy, 2001). The Yonsei University (YSU) parameterisation of the planetary boundary layer scheme is used in the all domains (Troen and Mahrt, 1986; Hu et al., 2013). A Kain-Fritsch (Kain, 2004) cumulus scheme is applied only in the first and second domain. A summary of the physical parameterization schemes were provided in Table 2. The WRF-Fire simulation started on October 17 at 0000 UTC and was run until 1800 UTC Oct.26.
2.3.3 Data sources and preprocessing
Running the WRF mode requires a meteorological data to driven, and the meteorological data is FNL reanalysis data, which mainly includes three separate data sets: 3d, flx and sfc. After downloading the files of the corresponding date, it needed to be extracted in the file system. The FNL (Final Operational Global Analysis) data is a global reanalysis data provided by the National Center for Environmental Prediction (NCEP) and National Center for Atmospheric Research (NCAR). Using the state-of-the-art global data assimilation system and well-established database, they carried out quality control and assimilation of observation data from various sources, and obtained a complete set of reanalysis datasets. In addition, the topography of the Fire model is a sub-grid form of the atmospheric model grid defined by WRF through terrain independent of the atmospheric model, as the basic data of the key elements of forest fire spread calculation. The ASTER GDEM (Advanced Spaceborne Thermal Emission and Reflection Radiometer Global Digital Elevation Model) V3 used in this study was jointly released by NASA and METI (Ministry of Economy, Trade and Industry) on August 5, 2019, which can be downloaded through Geospatial Data Cloud.5 The spatial resolution of DEM data is 30 m × 30 m, and the topographical conditions of the simulated area are shown in Figure 3.
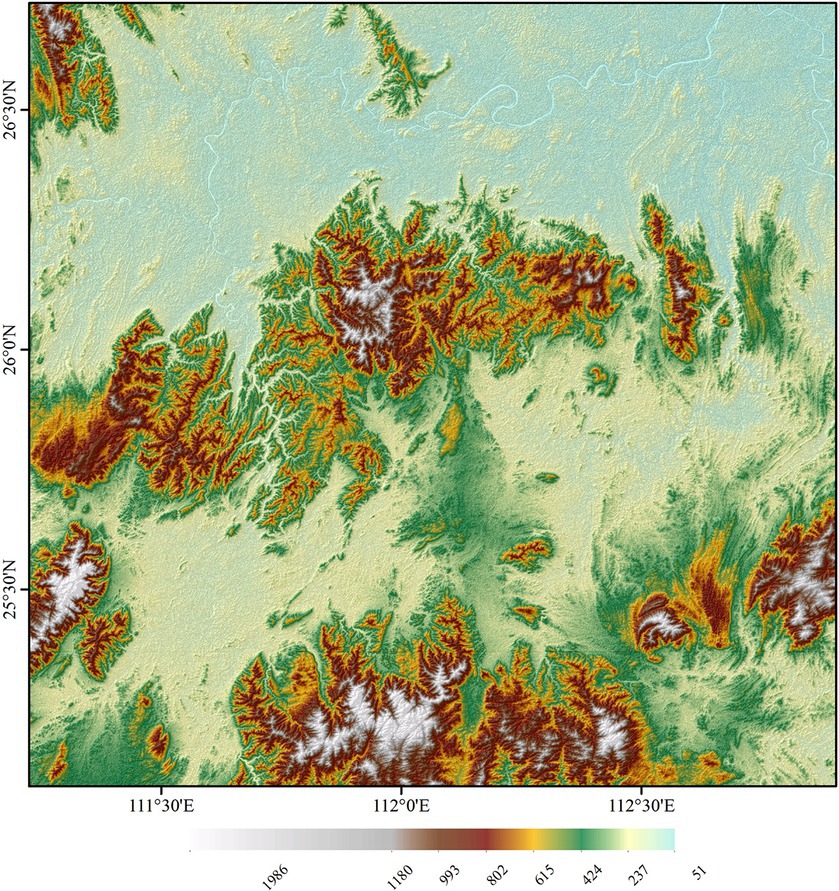
Figure 3. The topographical conditions of the simulated area (Domain Four in Figure 2). Unit of elevation: m above sea level.
In the WRF-Fire model system, forest fire fuel data is embedded into the forest fire grid, which is composed of sub-grids of each atmospheric grid. In this way, the fuel type defined on the sub-grid, together with the terrain and the ground wind, are the core element that determines the fire behavior characteristics such as the direction and speed of the spread of a forest fire, and the flame intensity. The fuel data of WRF-Fire is category data, which is constructed into 13 fuel categories using Anderson’s classification system (Anderson, 1982). In Table 3, the constants that determine the combustion characteristics of each fuel are summarized. This study follows the 13 categories of constant values used in WRF-Fire. In this study, the fuel classification data is based on the global land cover data from-glc (Finer Resolution Observation and Monitoring of Global Land Cover),6 which is mapped with Anderson fuel model, and the specific fuel categories are determined according to the survey data of forest fuel fixed sample plots in the hilly area of central Hunan.
The from-glc is the first 30-meter resolution global land cover map produced using Landsat Thematic Mapper and Enhanced Thematic Mapper Plus data. The data set is divided into 10 categories. First, after a series of processing such as reclassification, resampling, and projection transformation, the results are converted into ASCII code files, and then compiled to generate a.out using write_geogrib.c. After running, the required binary format fuel classification data can be generated.
3 Results
3.1 Simulated atmospheric conditions
The prevailing synoptic conditions, which greatly influenced the behavior and evolution of the fatal forest fire events in the Xintian on 17 October 2022, are presented along with the available surface observations.
As shown in Figure 4, the synoptic system and its changes in this region were very stable and simple, and they were in the situation of high temperature, low humidity and strong wind, which was a necessary and important condition for fire. According to the satellite data, the fire was located at the front part of a ridge. The wind was steady from the northeast at 0600 UTC, Oct.17 (Figure 4A). After the ignition began at about 0730 UTC, Oct.17, the center of high pressure was located above the Yao nationality township (Figure 4B). At 1800 UTC, Oct.17 (Figure 4C), the high pressure system moved to the southeast of Xintian county, the wind direction gradually shifted from north east wind to north wind. As can be observed in Figure 4, in general, the wind direction was mostly north wind and the wind speed was relatively high, and the temperature was high during the whole fire spread period.
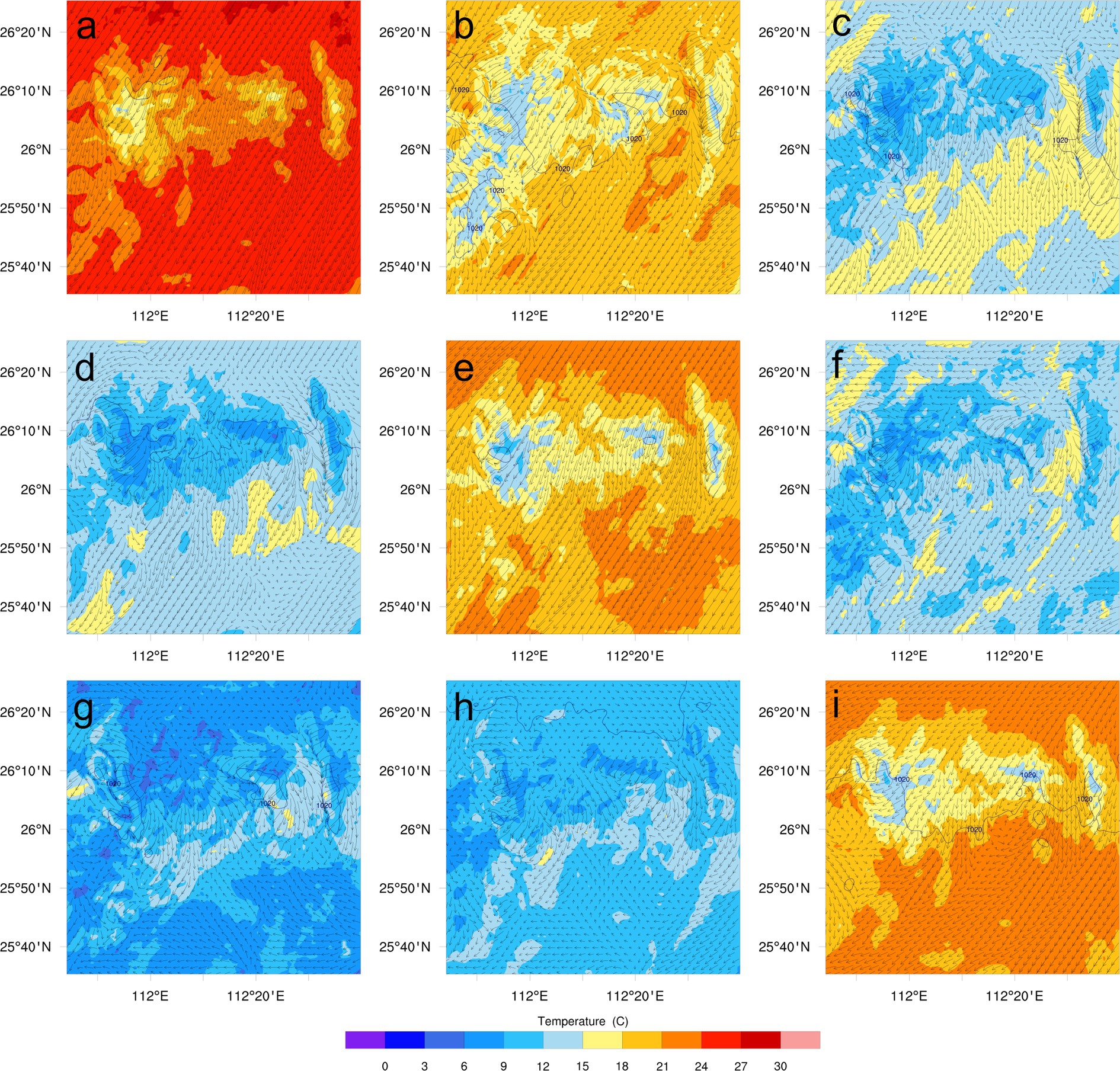
Figure 4. The synoptic pattern variation based on WRF-d04 values. (The contours are sea level pressure, unit: h Pa, and temperature (shaded), unit:°C, arrow is wind, unit: m s−1). [(A) 0600 UTC, Oct.17, 2022; (B) 1200 UTC, Oct.17, 2022; (C) 1800 UTC, Oct.17, 2022; (D) 0000 UTC,Oct.18, 2022; (E) 0600 UTC, Oct.18, 2022; (F) 1200 UTC, Oct.18, 2022; (G) 1800 UTC, Oct.18, 2022; (H) 0000 UTC,Oct.19, 2022; (I) 0600 UTC, Oct.19, 2022].
3.1.1 Wind
The Xintian fire was ignited in Banziqiao Village, Yao Township. Due to the continuous drought in the area, the surface vegetation and leaves were numerous, and the fire broke out almost 8 h after the simulation began. At that time, the wind speed was high and the fire spread rapidly. The wind can not only accelerate the evaporation of fuel moisture, make the fuel dry and flammable, while continuously replenish new oxygen, increase the combustion conditions and accelerate the combustion process. Wind is one of the most important and rapidly changing environmental factors that influence fire behavior, the higher wind speeds produce larger fire spreading rates over a wide range of wind speeds and fuels (Coen et al., 2013). An evaluation of the fire spread forecast by WRF-Fire starts therefore with an evaluation of the wind forecast by WRF. The first 6 hours were not accounted in the results and the meteorological forecast was performed from 0600 UTC on 17 October 2022 to 1800 UTC, on 26 October 2022. We obtained the every 3 h wind field distribution during forest fire spread by WRF model. In this study, we selected the Yongzhou meteorological stations closest to the fire site for verification.
A comparison of the wind speed and direction time series for all simulations and observations was shown in Figure 5. As can be seen from the figure, the variation trend of simulated wind speed is similar to the observed results, but the wind speed at a height of 10 meters was slightly overestimated (Figure 5A). At the beginning of the experiment, the wind blew from the northeast and then moved slowly to the north, and the dominant wind direction was N, which proved that the simulated wind direction was in good agreement with the observed results (Figure 5B). Through the comparison of wind speed and wind direction, it was found that WRF model was used to simulate the wind field, and the simulated wind field was close to the measured wind field. The simulation results can reflect the real wind field while obtaining high-resolution wind field.
In the fire-fighting operation, it is very important to understand the abrupt change of wind direction and wind speed. In order to observe the horizontal distribution and evolution of wind fields during the spread of forest fire, we focused on extracting the simulated wind field distribution at six moments in the study area during the fire rekindle period (October 21, 2022), as shown in Figure 6. At 0000 UTC Oct.21, the wind field of Yao nationality township was relatively uniform, the overall northerly wind, and the wind speed was large, with the highest wind speed reaching 2.7 m s−1 (Figure 6A). At 0300 UTC Oct.21, the wind field of Yao nationality township was still north wind, and the wind speed increases, with the highest wind speed reaching 4.3 m s−1 (Figure 6B), which was very beneficial to the rekindle of the forest fire. At 0600 UTC Oct.21, the wind speed continued to increase to 5.0 m s−1, which accelerated the spread of the forest fire (Figure 6C). In general, it can be observed from Figure 6 that the wind speed increases during the rekindle of the forest fire, and the wind direction was mainly north wind in the spreading area. In summary, WRF can present the temporal and spatial variation of local wind fields under complex terrain.
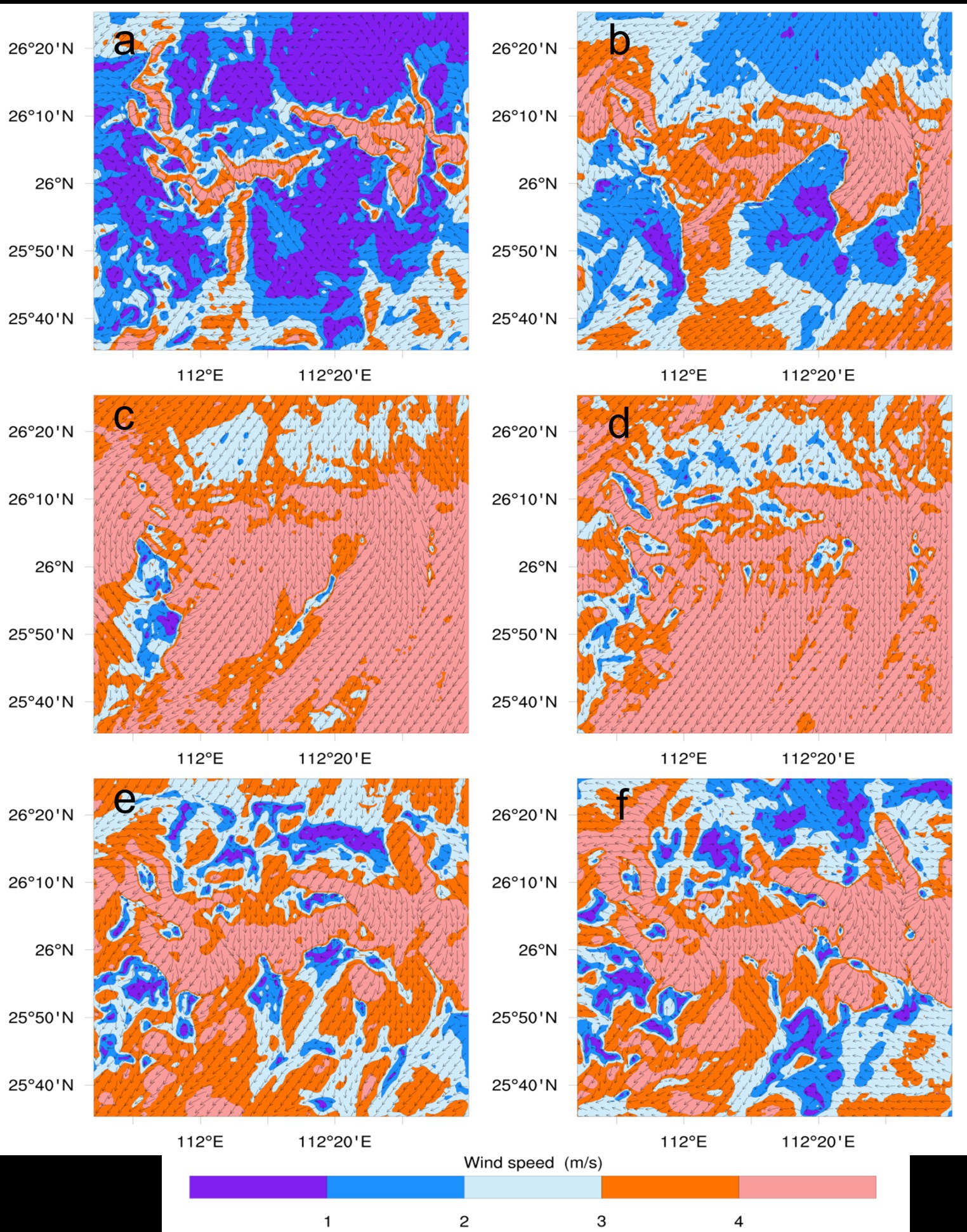
Figure 6. Spatial distribution of the simulated wind field. [(A) 0000 UTC, Oct.21, 2022; (B) 0300 UTC, Oct.21, 2022; (C) 0600 UTC, Oct.21, 2022; (D) 0900 UTC,Oct.21, 2022; (E) 1,200 UTC, Oct.21, 2022; (F) 1500 UTC, Oct.21, 2022].
3.1.2 Temperature
The simulated temperatures averaged from 0600 UTC on 17 October 2022 to 1800 UTC, on 26 October 2022 near the fire area are compared with the observations, and the comparisons of the simulated and observed temperature time series are shown in Figure 7. As can be seen from the figure, the overall change trend of the simulated temperature was similar to the observations, but the simulated temperature was underestimated (Figure 7). We also extracted the simulated temperature distribution at the six moments during the re-fire period, and the temperature in the study area gradually increased with the fire was rekindled (Figure 8). From the perspective of the simulated wind and temperature, the model better simulated the characteristics of this fire, so the spread characteristics of forest fire can be studied based on the simulation results.
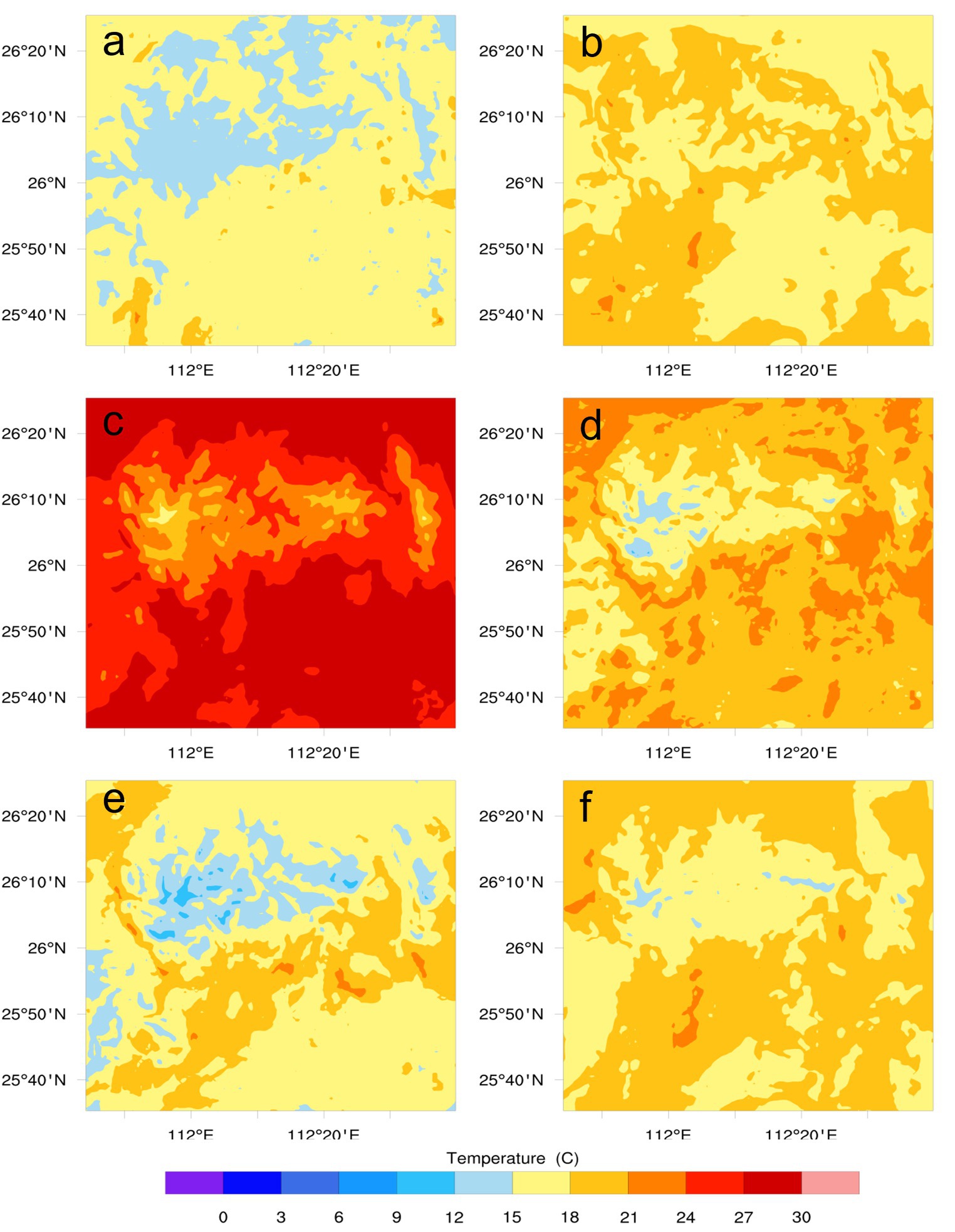
Figure 8. Spatial distribution of the simulated temperature. [(A) 1800 UTC, Oct.20, 2022; (B) 0000 UTC, Oct.21, 2022; (C) 0600 UTC, Oct.21, 2022; (D) 1200 UTC,Oct.21, 2022; (E) 1800 UTC, Oct.21, 2022; (F) 0000 UTC, Oct.22, 2022].
3.2 Simulated fire behavior and evolution
Due to the drought in Hunan Province during this period and its unique forest type (mainly coniferous mixed forest), the Xintian fire was ignited at 0730 UTC on October 17, 2022 and eventually burned 10,520.6 hectare (Figure 1). It must be mentioned that the time period varies between simulated and observed fire events, and the lack of temporal observation data on the actual burn area. Thus we have only the fire perimeter at the end of the fire event. In addition, it is known that fire-fighters have made numerous attacks on the fire in order to constrain the fire. As there is no precise data available on these attacks, we ran the simulation without taking into account the effect of the fire-fighters.
We divided the Xintian fire into two stages according to the rekindle of the fire. The results of the first stage of the dynamic simulation of forest fire spread are shown in Figure 9. The forest fire mainly spread southward from the fire point. Figure 9 shows the fire spread area of Xintian fire at different times and at intervals of 6 h. At 0900 UTC Oct.21, the fire spread southward with the northerly wind (Figure 9A). At 1500 UTC Oct.21, the wind direction of the fire field was northwest wind, and the fire quickly spread southeast and burned violently (Figure 9B). Figure 9C shows that the wind direction is to the northeast and the fire spreads to the southwest. From 0300 UTC Oct.18 to 0300 UTC Oct.19, the wind direction was relatively stable, all of which were northeast wind, and the fire spread to the southwest (Figures 9D–H). Since 0900 UTC Oct.18, the wind speed decreased, the wind direction was relatively chaotic, and the fire spread speed slowed down (Figures 9I–L). The ROS is closely linked to the wind, and the rise of the ambient wind speed will increase the diffusion rate of the fire line contacting fresh fuel and air along its surroundings.
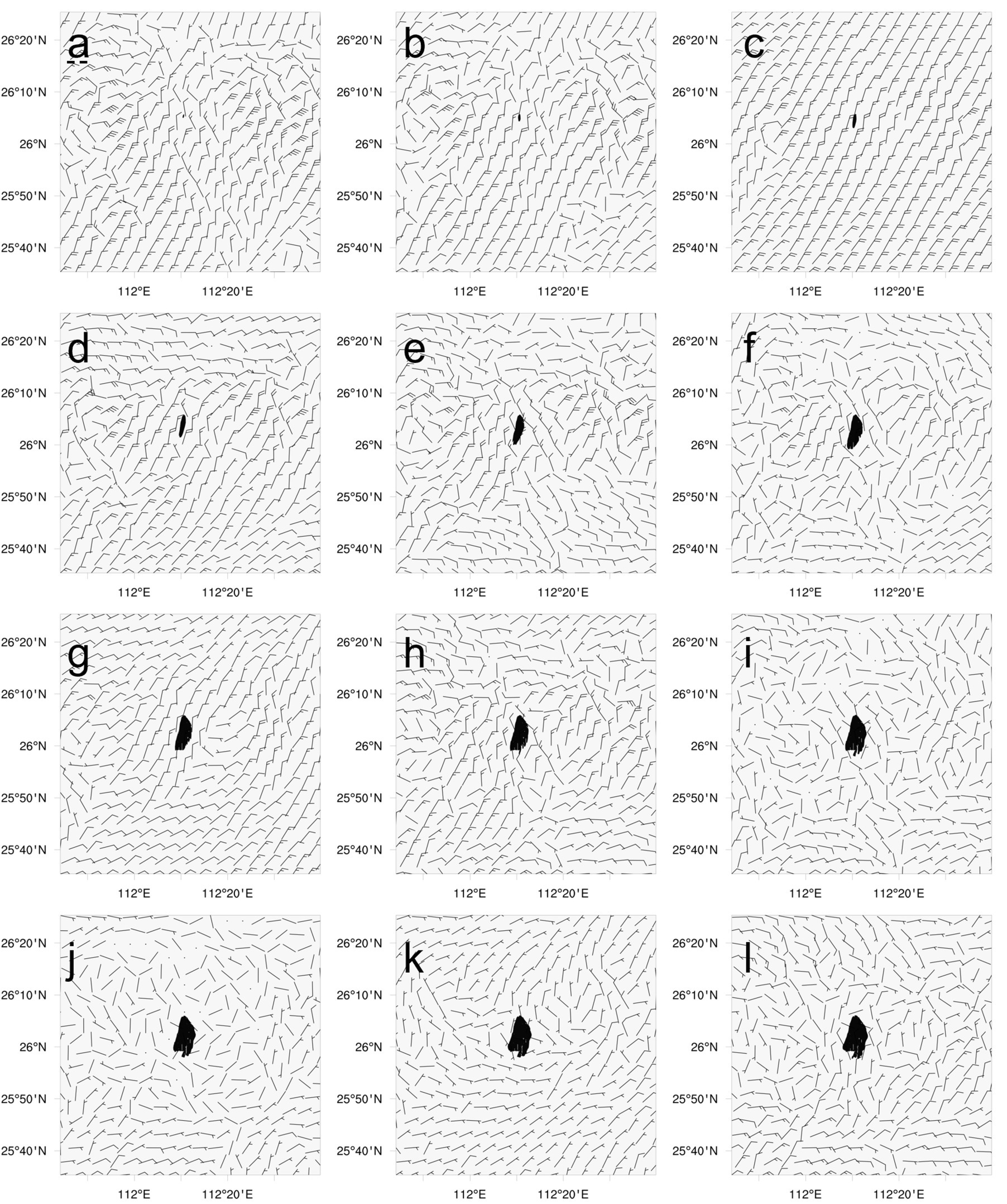
Figure 9. Evolution of the simulated burnt area (black shadow) and wind field. The figure shows every 6 h from 0900 UTC Oct.17, 2022 to 0300 UTC Oct.20, 2022. [(A) 0900 UTC, Oct.17, 2022; (B) 1500 UTC, Oct.17, 2022; (C) 2100 UTC, Oct.17, 2022; (D) 0300 UTC, Oct.18, 2022; (E) 0900 UTC, Oct.18, 2022; (F) 1500 UTC, Oct.18, 2022; (G) 2100 UTC, Oct.18, 2022; (H) 0300 UTC, Oct.19, 2022; (I) 0900 UTC, Oct.19, 2022; (J) 1500 UTC, Oct.19, 2022; (K) 2100 UTC, Oct.19, 2022; (L) 0300 UTC, Oct.20, 2022].
The results of the second stage of the dynamic simulation of forest fire spread are shown in Figure 10. At 0000 UTC Oct.21 (before the fire reignited), there are low wind speed and disorderly wind direction (Figure 10A). At 0600 UTC Oct.21 (after the fire reignited), the wind speed increases, the wind direction is northward, and the fire spread slowly (Figure 10B). From 1,200 UTC Oct.21 to 0000 UTC Oct.22, the wind direction is relatively stable, all of which are northeast wind, and the fire spread to the southwest (Figures 10C–E). From 0600 UTC Oct.22 to 1800 UTC Oct.23, the wind direction in the fire area is mostly north wind, and the fire will spread slowly to the south (Figures 10F–L). As the fuels were consumed, the fire slowed and the combustion was gradually extinguished on the 26rd.
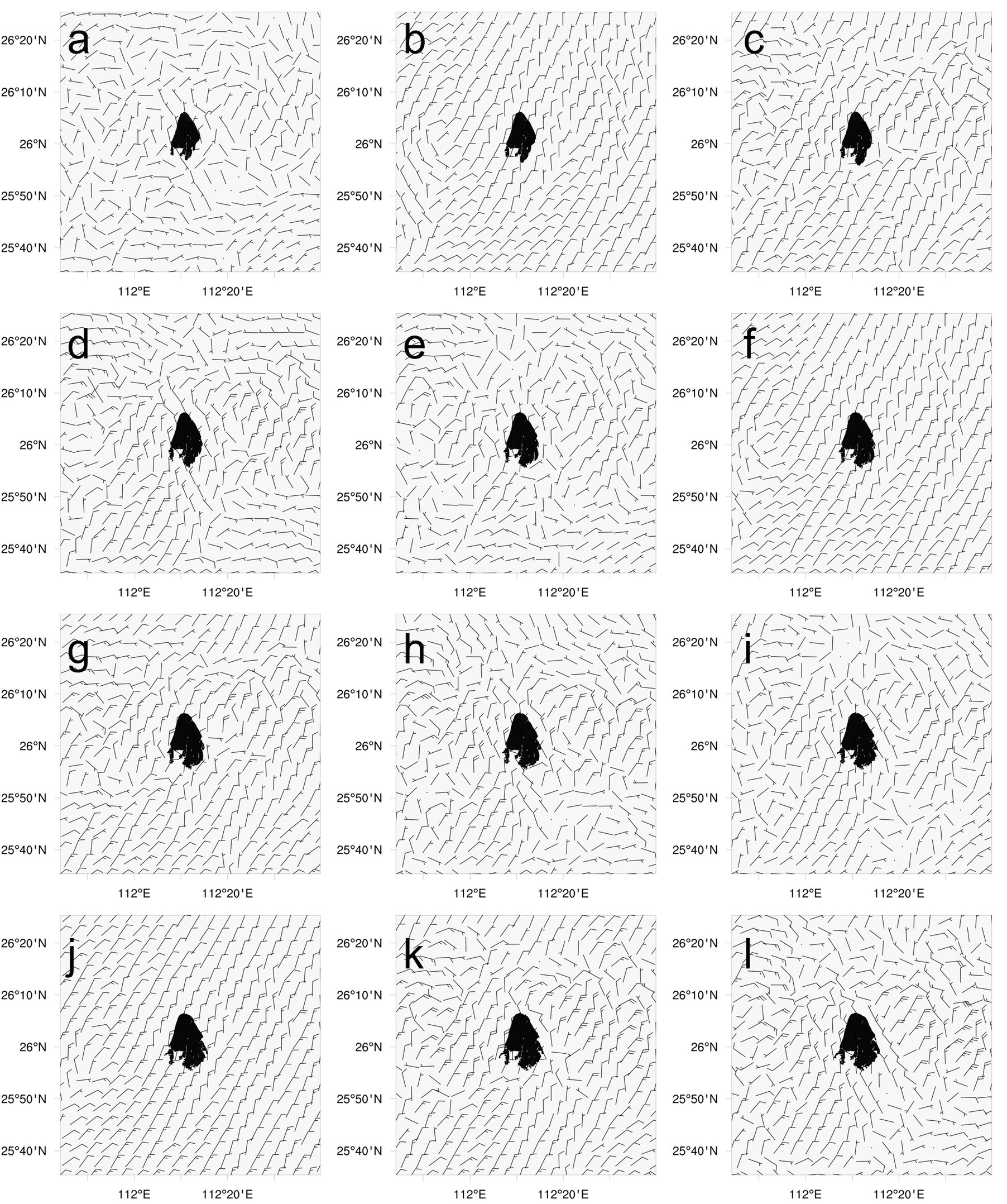
Figure 10. Evolution of the simulated burnt area (black shadow) and wind field. The figure shows every 6 h from 0000 UTC Oct.21, 2022 to 1800 UTC Oct.23, 2022. [(A) 0000 UTC, Oct.21, 2022; (B) 0600 UTC, Oct.21, 2022; (C) 1200 UTC, Oct.21, 2022; (D) 1800 UTC, Oct.21, 2022; (E) 0000 UTC, Oct.22, 2022; (F) 0600 UTC, Oct.22, 2022; (G) 1200 UTC, Oct.22, 2022; (H) 1800 UTC, Oct.22, 2022; (I) 0000 UTC, Oct.23, 2022; (J) 0600 UTC, Oct.23, 2022; (K) 1200 UTC, Oct.23, 2022; (L) 1800 UTC, Oct.23, 2022].
According to the WRF-Fire simulation system (from 0730 UTC Oct.17, 2022 to 1800 UTC Oct.26, 2022), the total burned area of Xintian fire was 20718.1 ha (Figure 11), which was about twice the observed area (10520.6 ha). It can be seen from Figures 9–11 that although the simulated fire area was generally overestimated, it can also capture the overall shape and the spread trend of the fire well.
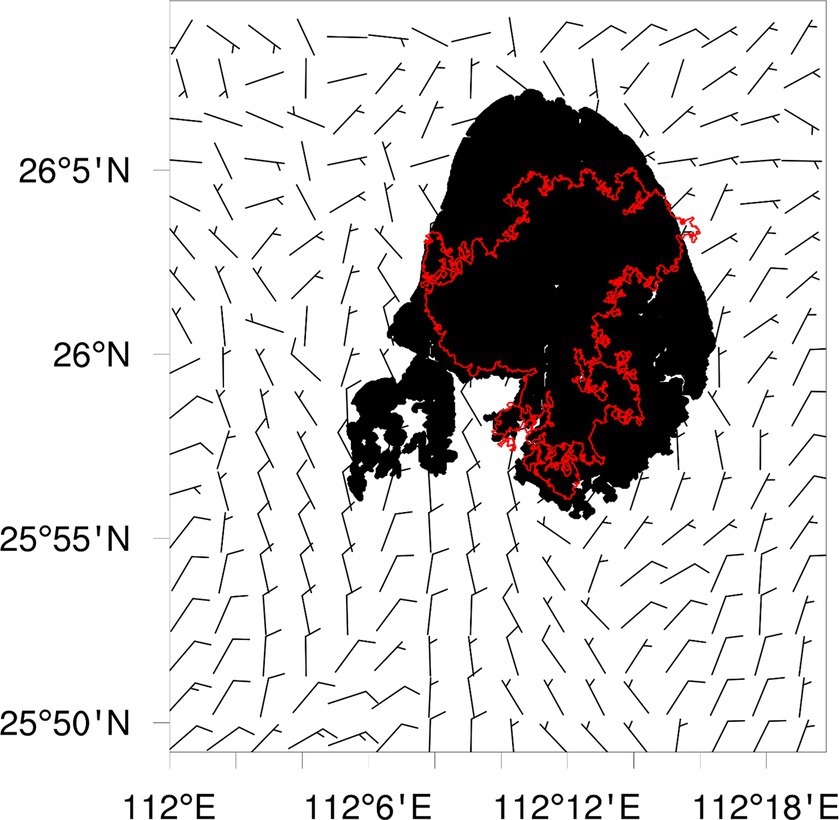
Figure 11. Comparison between the simulated fire areas of WRF-Fire (black shadow) and observed the fire areas of sentinel data (red lines).
4 Discussion and conclusion
In this study, the coupled atmosphere-fire simulation was performed using WRF-Fire. In the coupled atmosphere-fire simulation, the difference between the simulated fire boundary and the observed fire boundary depends on the accuracies of the meteorological and fire components of the model. Wind speed and wind direction are the key parameters to control fire propagation in forest fires simulation, which can affect both the ROS of fire and the direction of fire propagation (Shamsaei et al., 2023). Therefore, unrealistic wind forecasts may lead to incorrect fire spread prediction, even if the fire model itself provides a perfect fire spread prediction. In addition, fuel is another key factor in forest fire (Seong et al., 2013), and inaccurate fuel maps and fuel models can also lead to different fire propagation processes, even with a perfect weather forecast.
This study presented the prevailing synoptic and surface weather conditions from Oct.17, 2022 to Oct.26, 2022. The simulated wind and temperature of in the fire area are sensitive to the horizontal boundary conditions, and if these boundary conditions are inaccurate, the simulated field in the fire area will deteriorate (Peace et al., 2015). As shown in Section 3.2, there is a good agreement between WRF simulated wind direction and observed wind direction. The variation tendency of simulated wind speed by WRF is similar to the observed results, which can well simulate the change of wind speed. However, the comparison with the existing observed results shows that the wind intensity predicted by WRF is generally overestimated, which is consistent with the results of Lai et al. (2019) and Kartsios et al. (2021). The overall change trend of WRF simulated temperature is similar to the observed results, but the simulated temperature is underestimated, which is consistent with the results of Lai et al. (2019) and Li et al. (2023). The initialization error caused by insufficient description of terrain and land use may be some of the reasons for the above differences (Seong et al., 2013; Wang et al., 2023). The second reason may be that the predictability of the WRF model decreases significantly after 36 h, with model errors accumulating over time to the point of undermining the results (Kochanski et al., 2013b). The third reason may be that the meteorological numerical simulation is performed at a high resolution (wrf-d04: dx = dy = 0.666 km), where predictability decreases as one approaches the threshold resolution of an explicit simulation of boundary layer process deterministically (Mukherjee et al., 2016).
The 1 to 10 ratio between atmospheric and fire mesh, led to a horizontal discretization of approximately 60 m × 60 m for the fire model. Although the resolution is very high, certain physical processes related to atmosphere-fire interactions have not been resolved. The accuracy of the WRF-Fire simulation is judged based on a comparison between the observed and predicted final fire perimeter. In this study, the simulated fire perimeter and area are larger, and the simulated fire spreads to the north and southwest than the observed fire.
Wherever the model overestimates the burned area, widespread overestimation of wind speeds in some areas may be responsible for the mismatch. And the hourly variation of wind direction also affects the prediction of fire spread. It is worth noted that the modeled fire perimeter is very sensitive to the wind forecasts. When the Rothermel formula pushes the fire forward a certain distance based on the wind simulated by WRF-Fire, it can never move the fire back to its previous position. That is, prediction errors in simulated ROS accumulate over time (Kochanski et al., 2013b). Nevertheless, the fire spread data from the center section proved that WRF-Fire simulations also correctly captured the general direction of forest fire spread during the Xintian Fire, as Shamsaei et al. (2023).
Obviously, the preventing effect of human fire-fighting activities on forest fire spread is not considered in the forest fire spread model. In the case of forest fire in this study, we also dispatched firefighting helicopters and a large number of personnel to carry out active firefighting work. Although it is impossible to quantitatively determine the effect of fire suppression on diffusion, the burned area of the natural state can be greatly reduced under the same burned time.
This study evaluated the performance and applicability of WRF-Fire, a coupled fire-atmospheric forest fire model, in simulating the 2022 Xintian Fire by comparing the simulated and observed fire perimeter and burned area. The comparison of the results showed that there are significant differences between the simulated and observed results in terms of fire spread direction and fire perimeter. Compared to the observed values, the simulated fire was overestimated by twice and spreaded north and southwest. It is a big problem to verify the accuracy of the existing fire spread model. The factors causing the burned zone error are summarized as follows: the generally overestimated wind speed in this study has a greater impact on the simulated fire behavior (Clarke et al., 2013); the WRF-Fire model only represents fire propagation as surface fire, and lacks a mechanism to allow canopy fire modeling (Mandel et al., 2011); the static fuel data used in the study is a fuel code produced by referring to the land cover category from_glc, and there are inevitable error factors in this process; finally, the fire model does not take into account the fire firefighting actions.
Although there are differences between observed and simulated final fire perimeters, the findings suggest that WRF-Fire has the potential for operational applications as an operational predictive model for forest fire behavior that correctly captures the general direction of forest fire spread. In the next study, we will use higher resolution fuel data and meteorological data to further improve the accuracy of the model simulation results, and carry out a large number of forest fire simulation experiments in southern hilly areas to provide more detailed guidance for forest fire fighting. More accurate or reliable predictions and propagation of fire behavior and propagation of forecasts through WRF-Fire will be more helpful for forest fire management. These measures include: protecting people and guiding evacuation planning; predicting the possible size, duration and complexity of forest fire under the changes of in local meteorological and topographic conditions; developing and applying firefighting strategies and tactics, and to deploy air and ground resources effectively and safely.
Data availability statement
The original contributions presented in the study are included in the article/supplementary material, further inquiries can be directed to the corresponding authors.
Author contributions
HH: Writing – review & editing, Writing – original draft, Visualization, Validation, Software, Project administration, Methodology, Investigation, Formal analysis, Data curation, Conceptualization. XD: Writing – review & editing, Supervision, Project administration, Methodology, Investigation, Conceptualization. GZ: Writing – review & editing, Software, Formal analysis, Data curation. LF: Writing – review & editing, Methodology, Formal analysis. JL: Writing – review & editing, Investigation, Validation, Methodology. ZL: Writing – review & editing, Validation, Investigation, Formal analysis. YZ: Writing – review & editing, Investigation, Validation, Methodology. YW: Writing – review & editing, Methodology, Investigation, Conceptualization, Formal analysis, Data curation.
Funding
The author(s) declare financial support was received for the research, authorship, and/or publication of this article. This work was supported by the National Natural Science Foundation Project [32271879]; the Special Project of Forest and Grassland Fire Risk Survey of Forestry Bureau of Shaoyang County, Hunan Province, China [XYXLYJSLFH-2021-004].
Acknowledgments
We would like to acknowledge high-performance computing support from Cheyenne (doi: 10.5065/D6RX99HX) provided by NCAR’s Computational and Information Systems Laboratory, sponsored by the National Science Foundation.
Conflict of interest
The authors declare that the research was conducted in the absence of any commercial or financial relationships that could be construed as a potential conflict of interest.
Publisher’s note
All claims expressed in this article are solely those of the authors and do not necessarily represent those of their affiliated organizations, or those of the publisher, the editors and the reviewers. Any product that may be evaluated in this article, or claim that may be made by its manufacturer, is not guaranteed or endorsed by the publisher.
Footnotes
References
Anderson, H. E. (1982). Aids to determining fuel models for estimating fire behavior. Sidney: USDA Forest Service.
Andrews, P. L. (1986). BEHAVE: Fire behavior prediction and fuel modeling system-BURN subsystem, part 1. Ogden, UT: U.S. Department of Agriculture, Forest Service, Intermountain Research Station.
Andrews, P. L. (2007). BehavePlus fire modeling system: past, present, and future. Seventh Symposium on Fire and Forest Meteorology.
Bakhshaii, A., and Johnson, E. A. (2018). A review of a new generation of wildfire-atmosphere modeling. Can. J. For. Res. 49:138. doi: 10.1139/cjfr-2018-0138
Beezley, J., Kochanski, A., Kondratenko, V., Mandel, J., and Sousedík, B. (2010). Simulation of the Meadow Creek fire using WRF-fire. Washington, D.C.: American Geophysical Union.
Clarke, H., Evans, J. P., and Pitman, A. J. (2013). Fire weather simulation skill by the weather research and forecasting (WRF) model over south-East Australia from 1985 to 2009. Int. J. Wildland Fire 22, 739–756. doi: 10.1071/wf12048
Coen, J. L., Cameron, M., Michalakes, J., Patton, E. G., Riggan, P. J., and Yedinak, K. M. (2013). WRF-fire: coupled weather–wildland fire modeling with the weather research and forecasting model. J. Appl. Meteorol. Climatol. 52, 16–38. doi: 10.1175/jamc-d-12-023.1
Coen, J. L., and Schroeder, W. (2015). The High Park fire: coupled weather-wildland fire model simulation of a windstorm-driven wildfire in Colorado's front range. J. Geophys. Res. Atmos. 120, 131–146. doi: 10.1002/2014jd021993
Dobrinkova, N., Jordanov, G., and Mandel, J. (2010). “WRF-fire applied in Bulgaria” in Numerical methods and applications. eds. I. Dimov, S. Dimova, and N. Kolkovska (Berlin Heidelberg: Springer), 133–140.
Dudhia, J. (1989). Numerical study of convection observed during the winter monsoon experiment using a mesoscale two-dimensional model. J. Atmos. Sci. 46, 3077–3107. doi: 10.1175/1520-0469(1989)046<3077:NSOCOD>2.0.CO;2
Fei, C., and Jimy, D. (2001). Coupling an advanced land surface-hydrology model with the Penn State-NCAR MM5 modeling system. Part I: model implementation and sensitivity. Mon. Weather Rev. 129, 569–585. doi: 10.1175/1520-0493(2001)129<0569:CAALSH>2.0.CO;2
Finney, M. A. (1998). FARSITE: Fire Area Simulator-model development and evaluation. Ogden, UT: U.S. Department of Agriculture, Forest Service, Rocky Mountain Research Station.
Forestry Canada Fire Danger Group (1992). Development and structure of the Canadian forest fire behavior prediction system. Ottawa, Ontario, Canada: Forestry Canada, Science and Sustainable Development Directorate.
Heinsch, F. A., and Andrews, P. L. (2010). BehavePlus fire modeling system, version 5.0: design and features. Fort Collins, CO: U.S. Department of Agriculture, Forest Service, Rocky Mountain Research Station.
Hong, S. Y., Dudhia, J., and Chen, S. H. (2004). A revised approach to ice microphysical processes for the bulk parameterization of clouds and precipitation. Mon. Weather Rev. 132, 103–120. doi: 10.1175/1520-0493(2004)132<0103:Aratim>2.0.Co;2
Hu, X. M., Klein, P. M., and Xue, M. (2013). Evaluation of the updated YSU planetary boundary layer scheme within WRF for wind resource and air quality assessments. J. Geophys. Res. Atmos. 118, 10490–10505. doi: 10.1002/jgrd.50823
Janji, Z. I. (1994). The Step-Mountain eta coordinate model: further developments of the convection, viscous sublayer, and turbulence closure schemes. Mon. Weather Rev. 122, 927–945. doi: 10.1175/1520-0493(1994)122<0927:TSMECM>2.0.CO;2
Jellouli, O., and Bernoussi, A. S. (2022). The impact of dynamic wind flow behavior on forest fire spread using cellular automata: application to the watershed BOUKHALEF (Morocco). Ecol. Model. 468:109938. doi: 10.1016/j.ecolmodel.2022.109938
Jiménez, P., Muñoz-Esparza, D., and Kosović, B. (2018). A high resolution coupled fire–atmosphere forecasting system to minimize the impacts of wildland fires: applications to the chimney tops II wildland event. Atmosphere 9:197. doi: 10.3390/atmos9050197
José, R. S., Pérez, J. L., González, R. M., Pecci, J., and Palacios, M. (2014). Analysis of FIRE behaviour simulations over Spain with WRF-FIRE. Int. J. Environ. Pollut. 55, 148–156. doi: 10.1504/IJEP.2014.065919
Kain, J. S. (2004). The Kain-Fritsch convective parameterization: an update. J. Appl. Meteorol. 43, 170–181. doi: 10.1175/1520-0450(2004)043<0170:TKCPAU>2.0.CO;2
Kartsios, S., Karacostas, T., Pytharoulis, I., and Dimitrakopoulos, A. P. (2021). Numerical investigation of atmosphere-fire interactions during high-impact wildland fire events in Greece. Atmos. Res. 247:105253. doi: 10.1016/j.atmosres.2020.105253
Kochanski, A. K., Herron-Thorpe, F., Mallia, D. V., Mandel, J., and Vaughan, J. K. (2021). Integration of a coupled fire-atmosphere model into a regional air quality forecasting system for wildfire events. Front. For. Glob. Change 4:728726. doi: 10.3389/ffgc.2021.728726
Kochanski, A. K., Jenkins, M. A., Mandel, J., Beezley, J. D., Clements, C. B., and Krueger, S. (2013a). Evaluation of WRF-SFIRE performance with field observations from the FireFlux experiment. Geosci. Model Dev. 6, 1109–1126. doi: 10.5194/gmd-6-1109-2013
Kochanski, A. K., Jenkins, M. A., Mandel, J., Beezley, J. D., and Krueger, S. K. (2013b). Real time simulation of 2007 Santa Ana fires. For. Ecol. Manag. 294, 136–149. doi: 10.1016/j.foreco.2012.12.014
Lai, S., Chen, H., He, F., and Wu, W. (2019). Sensitivity experiments of the local wildland fire with WRF-fire module. Asia-Pac. J. Atmos. Sci. 56, 533–547. doi: 10.1007/s13143-019-00160-7
Li, B., Liu, G., and Shu, L. (2022). Simulation study on surface fire behavior of main forest types in Mentougou District, Beijing. J. Beijing For. Univ. 44, 96–105. doi: 10.12171/j.1000−1522.20210204
Li, C., Meng, Q., and Cui, W. (2023). Impact of dynamic wind flow behavior on Forest fire spread using WRF model: a case study of “3·30” Forest fire in Xichang. Sci. Technol. Eng. 23, 4579–4585. doi: 10.12404/j.issn.1671-1815.2023.23.11.04579
Liu, P., Liu, Y., Guo, X., Zhao, W., Wu, H., and Xu, W. (2023). Burned area detection and mapping using time series Sentinel-2 multispectral images. Remote Sens. Environ. 296:113753. doi: 10.1016/j.rse.2023.113753
Liu, F., Zhang, Y., Man, Z., and Sun, L. (2018). Classifying Forest fuel types by photo classification and image recognition. J. Cent. South Univ. For. Technol. 46, 51–57. doi: 10.13759/j.cnki.dlxb.2018.11.010
Mandel, J., Amram, S., Beezley, J. D., Kelman, G., Kochanski, A. K., Kondratenko, V. Y., et al. (2014). Recent advances and applications of WRF–SFIRE. Nat. Hazards Earth Syst. Sci. 14, 2829–2845. doi: 10.5194/nhess-14-2829-2014
Mandel, J., Beezley, J. D., and Kochanski, A. K. (2011). Coupled atmosphere-wildland fire modeling with WRF 3.3 and SFIRE 2011. Geosci. Model Dev. 4, 591–610. doi: 10.5194/gmd-4-591-2011
Michael, Y., Kozokaro, G., Brenner, S., and Lensky, I. M. (2022). Improving WRF-fire wildfire simulation accuracy using SAR and time series of satellite-based vegetation indices. Remote Sens. 14:2941. doi: 10.3390/rs14122941
Michalakes, J., Chen, S., Dudhia, J., Hart, L., Klemp, J., Middlecoff, J., et al. (2001). Development of a next generation regional weather research and forecast model. Dev. Teracomput. 1, 269–276. doi: 10.1142/9789812799685_0024
Mlawer, E. J., Taubman, S. J., Brown, P. D., Iacono, M. J., and Clough, S. A. (1997). Radiative transfer for inhomogeneous atmospheres: RRTM, a validated correlated-k model for the longwave. J. Geophys. Res. Atmos. 102, 16663–16682. doi: 10.1029/97jd00237
Morais, M. E. (2001). Comparing spatially explicit models of fire spread through chaparral fuels: a new algorithm based upon the Rothermel fire spread equation. Santa Barbara, CA: University of California.
Mukherjee, S., Schalkwijk, J., and Jonker, H. J. J. (2016). Predictability of dry convective boundary layers: an LES study. J. Atmos. Sci. 73, 2715–2727. doi: 10.1175/jas-d-15-0206.1
Muñoz-Esparza, D., Kosović, B., Jiménez, P. A., and Coen, J. L. (2018). An accurate fire-spread algorithm in the weather research and forecasting model using the level-set method. J. Adv. Model. Earth Syst. 10, 908–926. doi: 10.1002/2017ms001108
Noble, I. R., Bary, G. A. V., and Gill, A. M. (1980). McArthur's fire danger meters expressed as equations. Aust. J. Ecol. 5, 201–203. doi: 10.1111/j.1442-9993.1980.tb01243.x
Peace, M., Mattner, T., Mills, G., Kepert, J., and McCaw, L. (2015). Fire-modified meteorology in a coupled fire–atmosphere model. J. Appl. Meteorol. Climatol. 54, 704–720. doi: 10.1175/jamc-d-14-0063.1
Qiu, Z., Hu, X., Qian, H., Wei, B., and Liao, K. (2022). Analysis of spatial distribution characteristics of ancient and famous trees in Xintian County. J. Cent. South Univ. For. Technol. 42, 46–56. doi: 10.14067/j.cnki.1673-923x.2022.10.006
Rim, C. B., Om, K. C., Ren, G., Kim, S. S., Kim, H. C., and Kang Chol, O. (2018). Establishment of a wildfire forecasting system based on coupled weather–wildfire modeling. Appl. Geogr. 90, 224–228. doi: 10.1016/j.apgeog.2017.12.011
Rothermel, R. C. (1972). A mathematical model for predicting fire spread in wild land fuels. Sidney: USDA Forest Service.
Sakellariou, S., Parisien, M. A., Flannigan, M., Wang, X., de Groot, B., Tampekis, S., et al. (2020). Spatial planning of fire-agency stations as a function of wildfire likelihood in Thasos, Greece. Sci. Total Environ. 729:139004. doi: 10.1016/j.scitotenv.2020.139004
Seong, J. H., Han, S. O., Jeong, J. H., and Kim, K. H. (2013). Study on sensitivities and fire area errors in WRF-fire simulation to different resolution data set of fuel and terrain, and surface wind. Atmosphere 23, 485–500. doi: 10.14191/Atmos.2013.23.4.485
Shamsaei, K., Juliano, T. W., Roberts, M., Ebrahimian, H., Kosovic, B., Lareau, N. P., et al. (2023). Coupled fire-atmosphere simulation of the 2018 camp fire using WRF-fire. Int. J. Wildland Fire 32, 195–221. doi: 10.1071/wf22013
Skamarock, W. C., Klemp, J. B., Dudhia, J., Gill, D. O., and Powers, J. G. (2005). A description of the advanced research WRF version 2. Boulder: NCAR.
Skamarock, W. C., Klemp, J. B., Dudhia, J., Gill, D. O., and Powers, J. G. (2008). A description of the advanced research WRF version 3. Boulder: NCAR.
Sullivan, A. L. (2019). “Physical modelling of wildland fires” in Encyclopedia of wildfires and wildland-urban Interface (WUI) fires. ed. S. Manzello (Cham: Springer).
Troen, I. B., and Mahrt, L. (1986). A simple model of the atmospheric boundary layer; sensitivity to surface evaporation. Bound. -Layer Meteorol. 37, 129–148. doi: 10.1007/BF00122760
Turco, M., Jerez, S., Augusto, S., Tarín-Carrasco, P., Ratola, N., Jiménez-Guerrero, P., et al. (2019). Climate drivers of the 2017 devastating fires in Portugal. Sci. Rep. 9:13886. doi: 10.1038/s41598-019-50281-2
Wang, Y., Zhao, L., Yang, X., and Liang, L. (2023). Study on the influence of atmosphere and topographic on wildfire spread - A wildfire in Shanxi province as a case study. J. Catastr. 38, 118–125. doi: 10.3969/j.issn.1000-811X.2023.03.019
Williams, B. J., Song, B., and Williams, T. M. (2013). Visualizing mega-fires of the past: a case study of the 1894 Hinckley fire, east-Central Minnesota, USA. For. Ecol. Manag. 294, 107–119. doi: 10.1016/j.foreco.2012.12.008
Xu, Y., Li, D., Ma, H., Lin, R., and Zhang, F. (2022). Modeling Forest fire spread using machine learning-based cellular automata in a GIS environment. Forests 13:1974. doi: 10.3390/f13121974
Zhang, B., Cai, D., Ai, S., Wang, H., and Zuo, X. (2023). Research on the influencing factors and prevention measures of long-term forest fire risk in Northeast China. Ecol. Indic. 155:110965. doi: 10.1016/j.ecolind.2023.110965
Keywords: forest fire, coupled fire-atmosphere model, fire behavior simulation, fire spread, WRF-FIRE, Xintian forest fire
Citation: Hu H, Deng X, Zhang G, Feng L, Long J, Li Z, Zhu Y and Wang Y (2024) Fire behavior simulation of Xintian forest fire in 2022 using WRF-fire model. Front. For. Glob. Change. 7:1336716. doi: 10.3389/ffgc.2024.1336716
Edited by:
Michael Vrahnakis, University of Thessaly, GreeceReviewed by:
Gianni Pagnini, Basque Center for Applied Mathematics, SpainBambang Hero Saharjo, IPB University, Indonesia
Lailan Syaufina, IPB University, Indonesia
Copyright © 2024 Hu, Deng, Zhang, Feng, Long, Li, Zhu and Wang. This is an open-access article distributed under the terms of the Creative Commons Attribution License (CC BY). The use, distribution or reproduction in other forums is permitted, provided the original author(s) and the copyright owner(s) are credited and that the original publication in this journal is cited, in accordance with accepted academic practice. No use, distribution or reproduction is permitted which does not comply with these terms.
*Correspondence: Xiangwen Deng, ZHh3ZnJlZUAxMjYuY29t; Yiying Wang, Z3JhaW53eXlAMTYzLmNvbQ==