- 1Guangxi Key Laboratory of Mangrove Conservation and Utilization, Guangxi Academy of Marine Science (Guangxi Mangrove Research Center), Guangxi Academy of Science, Beihai, China
- 2College of Design and Creativity, Guilin University of Electronic Technology, Beihai, China
- 3College of Architecture and Urban Planning, Guangzhou University, Guangzhou, China
- 4College of Design and Creativity, Fujian Jiangxia University, Fuzhou, China
- 5Department of Landscape Architecture, College of Landscape Architecture and Art, Fujian Agriculture and Forestry University, Fuzhou, China
- 6State Key Laboratory of Remote Sensing Science, Faculty of Geographical Science, Beijing Normal University, Beijing, China
Introduction: Mangroves are an important component of coastal ecosystems, and determining the spatial dispersion of prevalent mangrove species and the most suitable land-use source for mangrove growth is of great importance for judicious restoration and effective conservation approaches. Maximum entropy (MaxEnt) models are well suited for this task; however, the default parameterization such models for distribution prediction has limitations and may produce results with low accuracy, requiring elucidation of useful parameter settings. Further, a focus on predicting only the mangrove distribution is insufficient for mangrove restoration, and clarification of suitable habitats is required. Here, we examined the geographical distribution of six mangrove species in Beihai, China (Aricennia marina, Aegiceras corniculatum, Kandelia obovata, Rhizophora stylosa, Bruguiera gymnorrhiza, and Acanthus ilicifolius).
Methods: We used the ENMTools tool to select 16 variables from environmental factors, including bioclimate, terrain, sediment type, land-use classification, and sea-surface salinity and temperature. Using the selected variables and mangrove distribution data, a MaxEnt model optimized using the “kuenm” package in R was used to establish a mangrove prediction distribution model for Beihai City. Transition analyses of land-use types within suitable zones further clarified their current and potential functional roles.
Results and discussion: The spatial occurrences of A. marina, A. corniculatum, and K. obovata were strongly driven by topographical features, those of R. stylosa and B. gymnorrhiza mostly depended on bioclimatic variables, and that of A. ilicifolius was driven mostly by edaphic conditions, notably the substrate type. The predicted optimal suitable area for mangrove growth in Beihai City was 50.76 km2, of which 55.04% are currently officially protected. Unprotected areas suitable for mangrove growth were mainly located in Lianzhou Bay, Tieshangang Bay, Dafengjiang, and Xicun Port. The majority of these regions were derived from land-use transitions from wetlands and aquaculture ponds to forested ecosystems. We suggest that careful development of selected wetland ecosystems and transmutation of aquaculture ponds into forested landscapes are crucial for effective mangrove restoration. Our results will assist in selecting suitable species for mangrove restoration sites and improving mangrove restoration efficiency.
1 Introduction
Mangroves are woody plant communities composed of evergreen trees and shrubs (Li and Dai, 2015). As an important marine wetland type (Jia et al., 2023), mangroves are mainly distributed in tropical and subtropical coastal intertidal zones (Valiela et al., 2001). Natural and human factors, such as coastal development (He et al., 2007) and climate change (Bertness et al., 2002), have resulted in mangrove wetland losses (Renzi et al., 2019). The global mangrove wetland area has declined by 35% since approximately 1980 and continues to decrease at a rate of 1–2% per year (Polidoro et al., 2010; Curnick et al., 2019). Global temperature increases affect mangrove growth, such as in areas where sea levels are rising rapidly, and mangroves have become extinct in some regions (Alongi, 2015). In China, the total mangrove forest area decreased by nearly 50% from 1950 to 2001 (Wang W. et al., 2020).
Therefore, mangrove restoration has become an important topic in ecological protection worldwide (Thomas et al., 2017; Hai et al., 2020); however, some reforestation actions lack scientific guidance in selecting locations and tree species, which is an important reason for the low survival rate of restored mangroves (Hu et al., 2020a). Mangrove growth is influenced by factors such as elevation, salinity, substrate, and temperature (Hu W. J. et al., 2020); however, the dominant factors influencing the growth of different mangrove types vary. In addition to considering local species, the main environmental factors driving mangrove growth need to be considered. An efficient approach to select suitable mangrove species and determine suitable land sources for mangrove restoration remains an unsolved issue (Balke and Friess, 2016; Fan and Mo, 2018). The majority of studies examining habitat suitability for mangrove restoration have not specified species using geographic information system methods (Jumawan and Macandog, 2021); direct planting remains the most commonly used mangrove restoration method (Gerona-Daga and Salmo, 2022). Therefore, the mangrove survival rate is often uncertain. Furthermore, restoration work cannot be effectively carried out if the land-use type of a potential mangrove area is not specified.
Employing a maximum entropy (MaxEnt) model and accounting for land-use transfer can solve these problems. At present, classic ecological niche models include MaxEnt, Rule Set Genetic Algorithm (GARP), support vector machine (SVM), and decision tree (DT) models, among other approaches (Peterson et al., 2007). Among these approaches, the MaxEnt model is more suitable for predicting the potential habitats of species in complex forests, featuring higher accuracy than SVM and DT models (Tu et al., 2012). The MaxEnt model outperformed the GARP model in predicting the potential mangrove distribution (Hu et al., 2020b). The MaxEnt model employs maximum entropy principles to simulate and predict suitable habitats for species based on species distribution records and environmental variables using machine-learning methods (Liu et al., 2017; Mukul et al., 2019; Wan et al., 2019; Bao et al., 2022). As the MaxEnt model is easy to implement (Wang et al., 2007), it has been widely used in species distribution modeling (Cobben et al., 2015; West et al., 2016; Liu et al., 2019).
Global-scale studies have used species distribution models to predict the response of 30 mangrove species to future climate conditions (Record et al., 2013); however, analysis on the impact of factors such as land use and sea-surface salinity is lacking. At the regional scale, Charrua et al. (2020) used the MaxEnt model to predict the distribution of Aricennia marina and Rhizophora mucronate. The capacity of species distribution modeling to predict the geographic distribution of the mangrove community in Mexico was also evaluated (Rodríguez-Medina et al., 2020). Banerjee et al. (2022) analyzed the predicted distribution of 10 mangrove species in the Indian Western Pacific region at temporal and spatial scales. Research on predicting the mangrove distribution in China has been conducted at the national scale (Hu et al., 2020a) and for specific locations such as Guangdong (Chao et al., 2021), Fujian (Hu W. J. et al., 2020), and the Beibu Gulf (Li L. F. et al., 2023). All of these studies adopted default system parameters for modeling and did not perform any optimization (Wang W. et al., 2020). Furthermore, overfitting may occur under default settings (Radosavljevic and Anderson, 2014), which negatively affects the prediction accuracy. Therefore, this study differed from previous research that predicted the distribution of mangroves in the following two aspects. First, we used the “kuenm” package (Cobos et al., 2019) in R to balance the fitting and complexity of the MaxEnt model by optimizing the regularization multiplier (RM) and feature class (FC) parameters. We used the optimized MaxEnt model, which served as the computational framework for elucidating the spatial configuration, potential distribution, and critical environmental requirements for an ensemble of six important mangrove species: A. marina, Aegiceras corniculatum, Kandelia obovata, Rhodophora stylosa, Bruguiera gymnorrhiza, and Acanthus ilicifolius (Figure 1). Second, existing research has only determined suitable locations for mangroves; however, an in-depth analysis on the specific sources of areas suitable for the growth of mangroves has not been conducted. In this study, we used a land-use transfer model to identify the land types most suitable for the distribution of mangroves, providing a basis for the selection of mangrove restoration areas. The purpose of this study was to determine suitable species and planting sites for the restoration of different mangrove species, aiming to improve the efficiency of mangrove restoration.
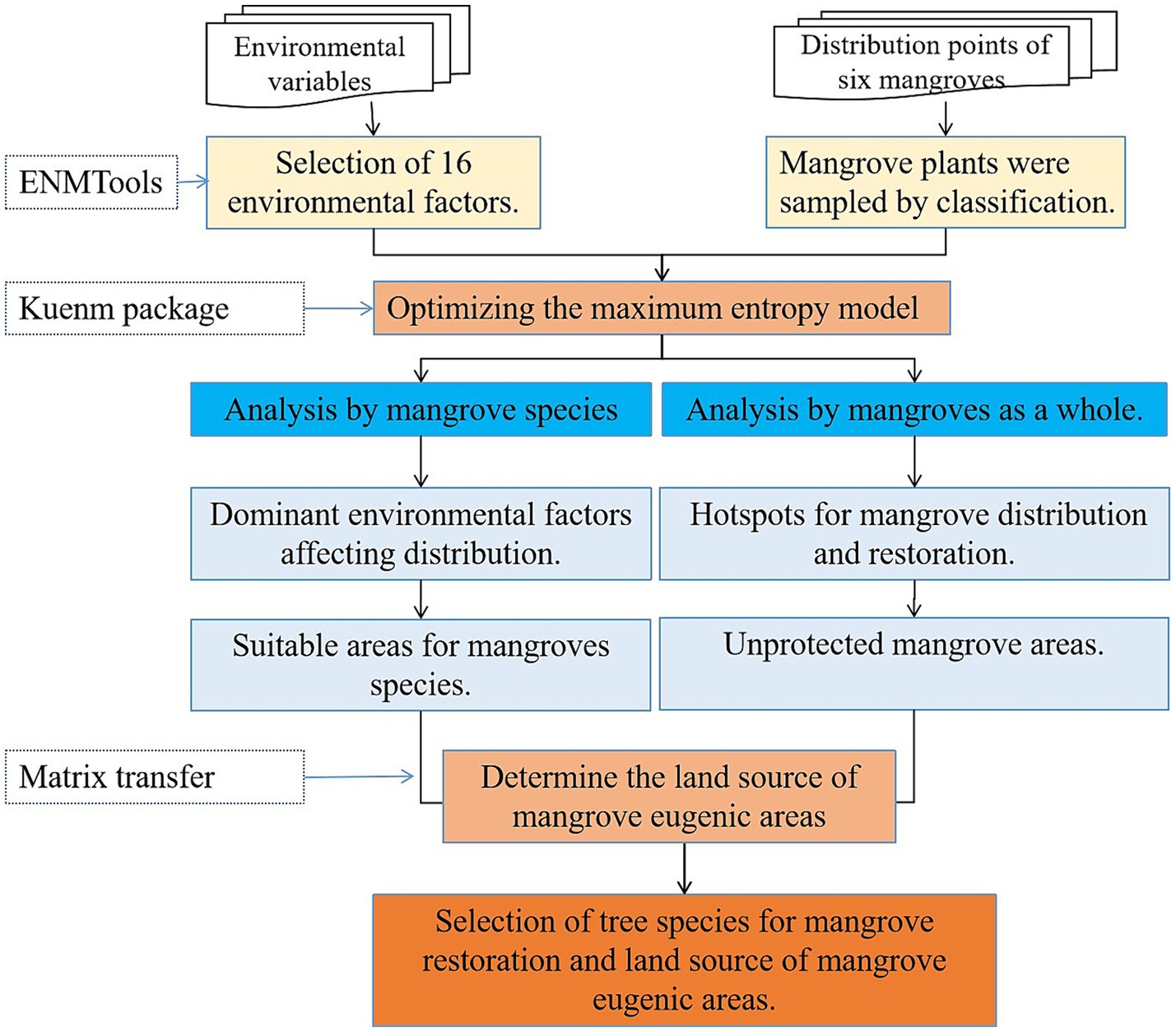
Figure 1. Analysis framework used to predict the potential distribution of mangrove species for restoration purposes.
2 Materials and methods
2.1 Study area
Beihai City is located in the southern Guangxi Zhuang Autonomous Region in south China (108°50′–109°47′E, 20°26′–2 l°55′N, Figure 2). It is characterized by a marine monsoon climate, with an annual average temperature of 22.9°C and an annual precipitation of 1,670 mm. The total length of the continental coastline is 553.08 km, accounting for approximately one third of the length of the coastline in Guangxi. The study area contains many natural bays, including Tieshangang Bay, Lianzhou Bay, and Yingluo Port, and features a mangrove nature reserve and a national wetland park. We mainly focused on the predicted mangrove distribution along the continental coastline. The dominant mangrove species were A. marina, A. corniculatum, K. obovata, B. gymnorrhiza, R. stylosa, and A. ilicifolius, and the top six mangrove communities in terms of area were A. marina, A. corniculatum, K. obovata, A. marina + A. corniculatum, B. gymnorrhiza + A. marina, and R. stylosa + A. corniculatum. The A. marina, K. obovata, and A. corniculatum communities accounted for 47.16, 28.18, and 11.65%, respectively, of the total area (Tao et al., 2017).
We selected Beihai City as the model area, mainly because the Beihai mangrove area accounts for 48.73% of the total mangrove area in the Beibu Gulf in Guangxi, China. Mangroves in the Beihai Sea play an important role in balancing the coastal environment, significantly affecting the local ecosystem. Urban development continues to impinge on the mangrove forests on the east coast of Beihai City. The cumulative survival rate of mangrove artificial afforestation in Guangxi from 2002 to 2015 was only 33.6% (Fan and Mo, 2018). This suggests that modeling and predicting the mangrove tree species distribution in Beihai City and determining suitable areas for different mangrove types is of great significance for improving the success rate of local mangrove restoration.
2.2 Mangrove distribution
We have been conducting research on mangroves for several years and are familiar with the distribution of mangroves in Beihai City. Photos were taken of each patch using drones, which allows confirmation of various mangrove species. Mangrove distribution data for Beihai were visually interpreted based on 2020 Google Earth images (0.61–2.4-m resolution). To avoid misclassification, we extracted various mangrove sample points using the following steps: (1) we visually interpreted and extracted 438 small mangrove patches in Beihai City; (2) the mangrove species were differentiated based on the appearance of patches and image color (Table 1; Liu et al., 2021; Li L. F. et al., 2023); the mangrove species were registered in the aforementioned mangrove patches. In doubtful instances, photos and targeted field surveys were used for confirmation; (3) the Fishnet tool in the ArcGIS 10.4 software package (Environmental Systems Research Institute, Redlands, CA, USA) was used to resample the interpreted mangroves (sampling accuracy of 300 m). The plant names and coordinates of mangrove sampling points were recorded, forming a distribution dataset of six mangrove plants. In total, 1,030 sampling and coordinate data points were obtained from the study area, consisting of 362, 537, 43, 36, 28, and 24 sampling points for A. corniculatum, A. marina, K. obovata, B. gymnorrhiza, R. stylosa, and A. ilicifolius, respectively.
2.3 Environmental data
Environmental data can be used to determine differences in growth factors among mangrove species. Environmental factors such as sea-surface temperature (SST), sea-surface salinity temperature, salinity, and distance from the coastline are important indicators of potential mangrove growth and distribution (Peng et al., 2016). As mangroves grow in sea–land ecotones, their fitness is affected by both continental and marine environmental factors; thus, we combined terrestrial and marine environmental data. To estimate the mangrove distribution in Beibu Gulf, the study area boundary was fixed using the coastline as a reference. The study area was determined based on the distribution pattern of mangroves and referring to existing research on mangrove distribution prediction (Hu et al., 2020a; Hu W. J. et al., 2020). The estimated study area enclosed a 10-km inland buffer zone (Hu et al., 2020a) and a 5-m isobath in the sea. Resampling was carried out in ArcGIS v.10.4 to extract environmental data, and Kriging interpolation was conducted to expand the data to sea or land and to integrate marine and terrestrial data. The sources of environmental data are shown in Table 2. The data accuracy was normalized to 30″ using ArcGIS, and the dataset was saved in ASCII format.
We sourced 29 descriptive variables of environmental data (19 parameters), terrain (2 parameters), SST data (3 parameters), sea-surface salinity (3 parameters), substrate type (1 parameter), and land use (1 parameter). Pearson correlation analysis (Li L. F. et al., 2023) was used to screen environmental variables to detect collinearity. We conducted this analysis using ENMTools (Li D. X. et al., 2023; https://github.com/danlwarren/ENMTools) as this approach does not rely on species distribution data and provides stable analysis results (Figure 3).
The final dataset included 16 bioclimatic variables: mean diurnal range (mean monthly [max. Temp–min. temp]; bio2), isothermality ([bio2/bio7] × 100; bio3), temperature seasonality (standard deviation × 100; bio4), temperature of the warmest month (bio5), mean temperature of the warmest quarter (bio10), precipitation seasonality (coefficient of variation; bio15), precipitation in the warmest quarter (bio18), precipitation in the coldest quarter (bio19), topographic elevation (alt18), wetland index (WTI), mean sea-surface salinity in the coldest season (c_sss), mean sea-surface salinity in the warmest season (w_sss), mean SST in the coldest season (c_sst), mean SST in the warmest season (w_sst), substrate type (substrate), and land-use type (land-use).
2.4 Model parameter settings
The “kuenm” package1 was used to optimize the RM and FC parameters in R (version 3.6.3; https://www.rproject.org/; Warren and Seifert, 2011). These two parameters were essential for building a species distribution model using MaxEnt version 3.4.4.2 During modeling, 75% of the data were used as the training set. In total, 1,160 candidate models were evaluated with parameters reflecting 40 RM setting combinations (from 0.1 to 4, with an interval of 0.1) and 29 FC combinations. Model selection was based on statistical significance (partial receiver operating characteristic), predictive ability (low omission rates), and complexity (we used Akaike’s information criterion with small sample size correction [AICc] to evaluate the model fit). First, the set of models was reduced using the omission rate criterion (<5% when possible). Second, the models with a delta AICc value <2 were selected from the resulting candidate set (Cobos et al., 2019; Figure 4). Model parameters for MaxEnt analysis were configured in alignment with FC and RM specifications (Table 3). Among the six mangrove plant species, A. marina and R. stylosa had a feature class of quadratic (Q), and the determined feature class settings for the other four mangrove plants differed. Bruguiera gymnorrhiza and R. stylosa had regulation multipliers of 0.5, and the other four species had different values.
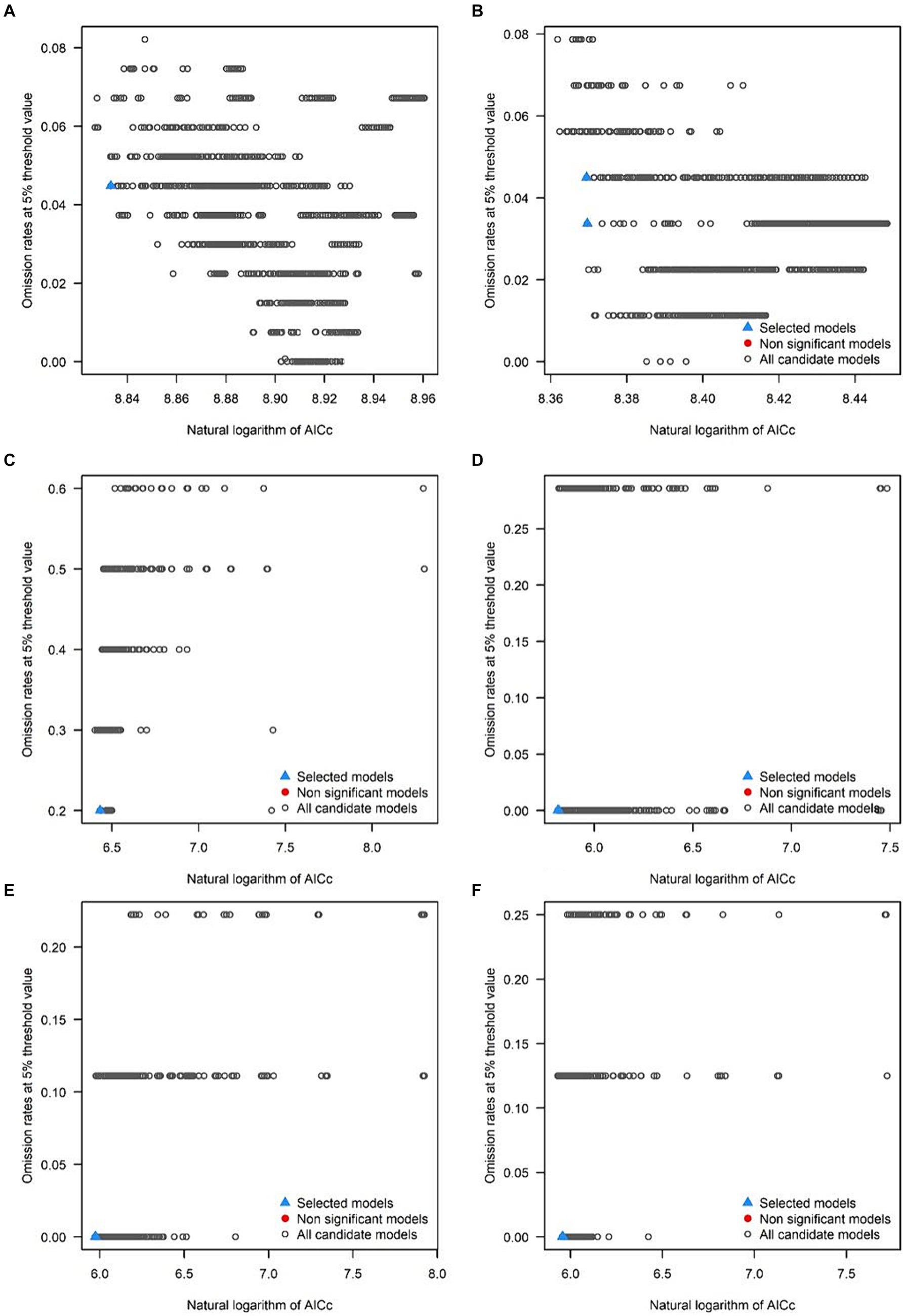
Figure 4. Omission rates and AICc values for all, non-significant, and selected “best” candidate models for: (A) A. marina, (B) A. corniculatum, (C) K. obovate, (D) R. stylosa, (E) B. gymnorrhiza, and (F) A. ilicifolius.
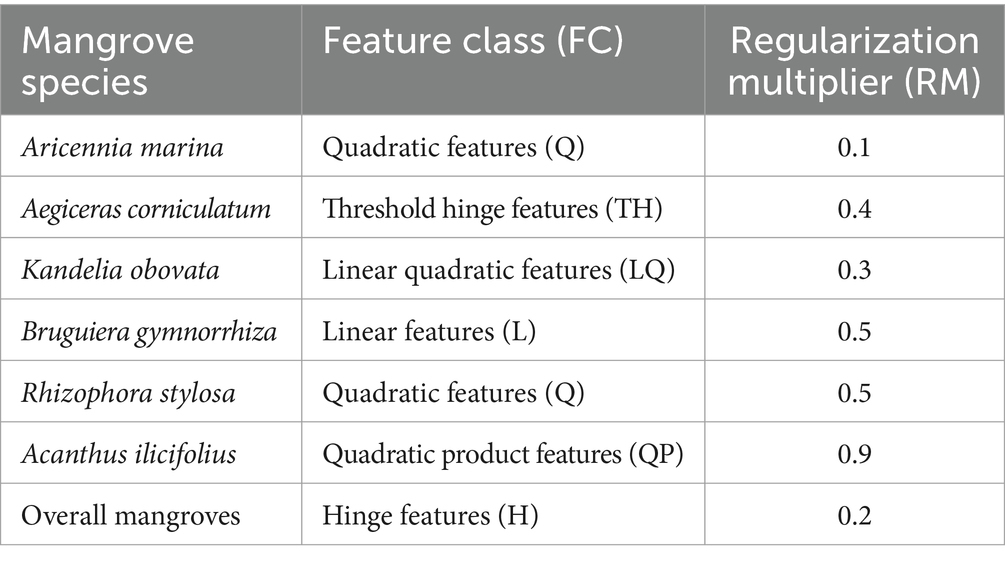
Table 3. MaxEnt parameter settings for mangroves based on optimization using the “kuenm” package in R for each species.
2.5 Land-use transfer
The land source for the optimal mangrove growth area was obtained using the land-use transfer method. We used a transfer matrix of land types to analyze the structural characteristics of the change direction of different land-use types (Shi et al., 2000) and ArcGIS 10.4 to obtain the land-use type transfer matrix.
2.6 Division of suitable areas for mangrove growth
Stratification of habitat fitness was conducted utilizing the natural breakpoint algorithm, with 0–0.2 denoting unfit conditions, 0.2–0.5 denoting low fitness, 0.5–0.7 denoting medium fitness, and values >0.7 denoting optimal fitness as mangrove habitat (Chao et al., 2020). Based on these results, potential mangrove distribution hotspots were calculated using kernel density estimation (Cai et al., 2012).
3 Results
3.1 Suitable areas for mangroves in Beihai, Guangxi
Optimal fitness areas were located in the Guangxi Shankou Mangrove Reserve, Tieshangang Bay, Lianzhou Bay, and Beihai National Wetland Park. The optimal area for A. marina was 33.51 km2 in size (Table 4), with the highest fitness areas mainly distributed in Jinhai Bay and Tieshangang Bay (Figure 5A); that for A. corniculatum was 22.07 km2 and was mainly distributed in Lianzhou Bay and Dafengjiang (Figure 5B); that for K. obovate was 40.87 km2 and was along Dafeng River and in Guangxi Shankou Mangrove Reserve (Figure 5C); that for B. gymnorrhiza was 9.81 km2 and was located in the Dandou Sea area (Figure 5D); that for R. stylosa was 21.25 km2 and mainly distributed in the Dandou Sea area and Yingluo Port (Figure 5E); and that for A. ilicifolius was 6.54 km2 and primarily located in regions with low estuarine salinity in Lianzhou Bay (Figure 5F).
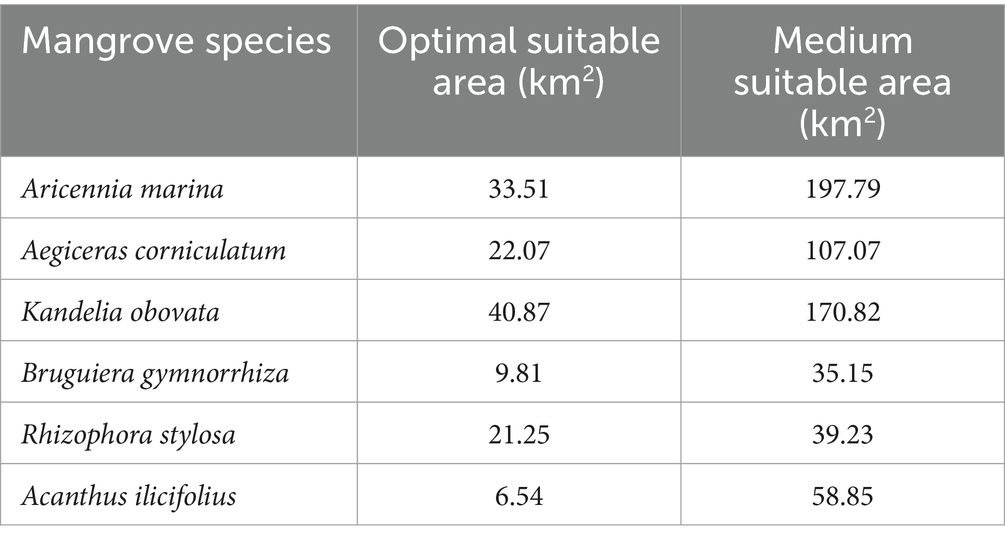
Table 4. Suitable mangrove areas in the study area as determined by MaxEnt analysis for each species.
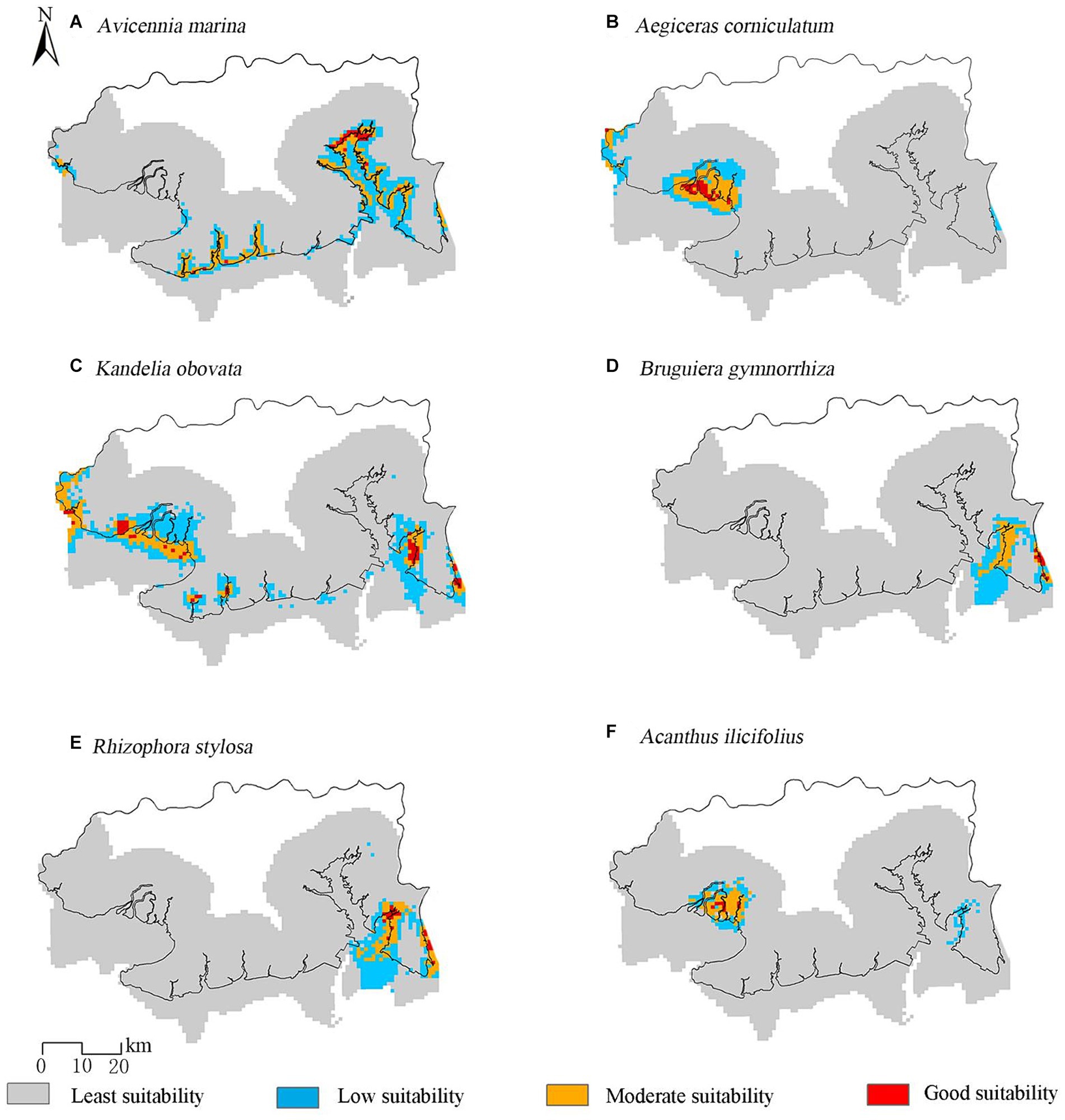
Figure 5. Distribution of suitable habitats for mangroves in Beihai for: (A) A. marina, (B) A. corniculatum, (C) K. obovate, (D) R. stylosa, (E) B. gymnorrhiza, and (F) A. ilicifolius.
3.2 Contribution of environmental factors affecting the mangrove distribution
Contributions of variables are based on interactions between different environmental variables (Liu et al., 2019; Hu et al., 2020a). The contributions of the six topographical factors were greater than those of the other factors (Figure 6). The influence of these factors on individual species decreased in the order A. marina > K. obovate > A. corniculatum > A. ilicifolius > R. stylosa > B. gymnorrhiza. Topographic factors contributed significantly to predicting the A. marina distribution, with a contribution value >50%.
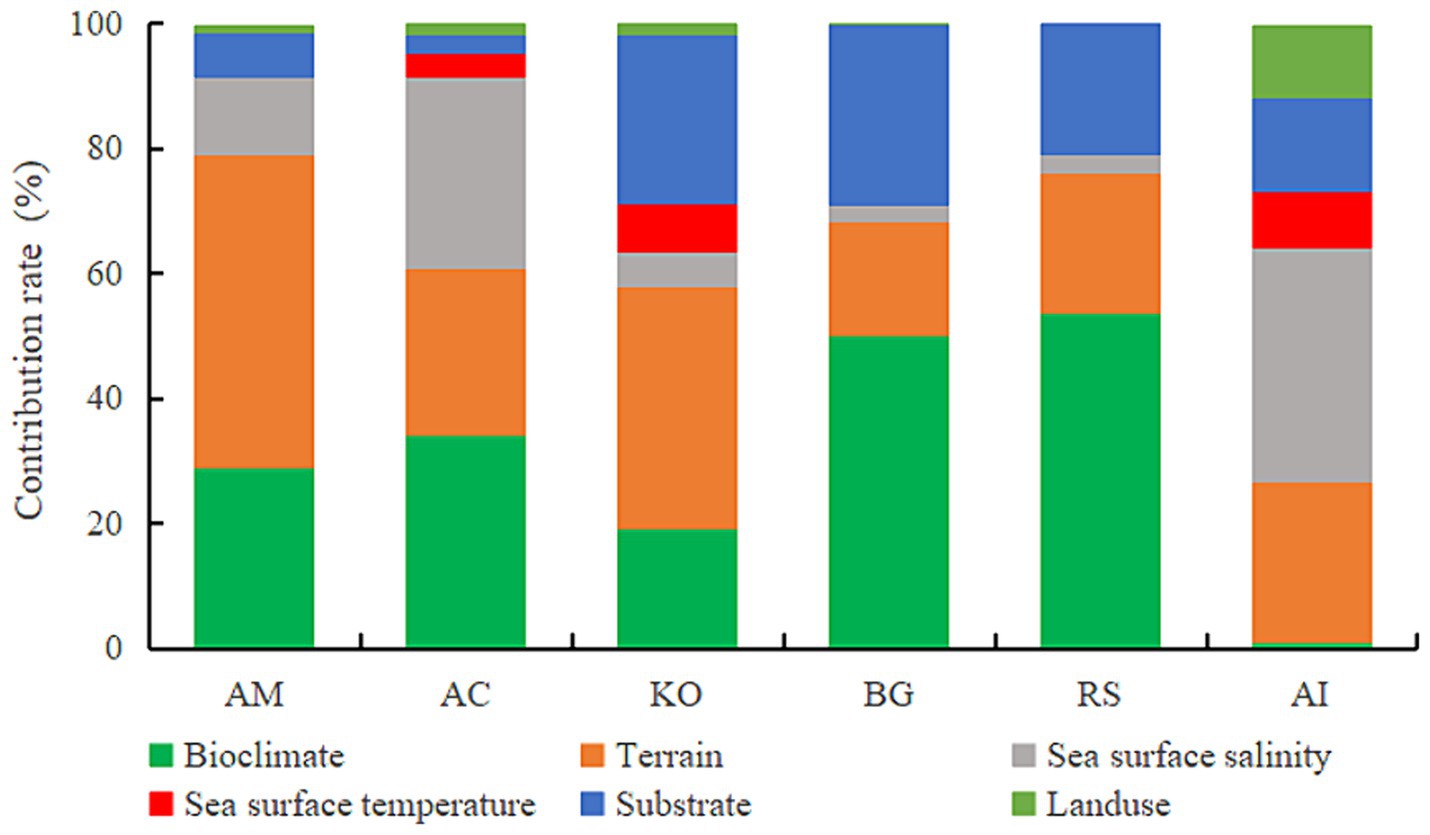
Figure 6. Contribution of the six investigated environmental variables in predicting the distribution of mangrove species (A. marina: AM, A. corniculatum: AC, K. obovata: KO, B. gymnorrhiza: BG, R. stylosa: RS, and A. ilicifolius: AI).
The contribution of bioclimatic factors to the R. stylosa and B. gymnorrhiza distribution was 53.5 and 49.9%, respectively. Sea-surface salinity was among the top-three influencing factors for A. ilicifolius, A. corniculatum, and A. marina, with a relative contribution of 37.4 and 30.7%, respectively, for A. ilicifolius and A. corniculatum, indicating that these species are sensitive to salinity.
3.3 Land-use analysis of suitable areas for mangroves based on land-use transfer
The land-use map for 2020 and the potential distribution grade map of the six mangrove species were used as the area transfer matrix to analyze the land source of potentially suitable mangrove areas that could provide the basis for mangrove restoration sites. Only a very small proportion of the coastal land area in Beihai City was transformed into the optimal habitat for the six mangrove species (Figure 7). The optimal mangrove habitat in Beihai mainly originated from wetlands and aquaculture ponds (Figure 8). The area transferred from wetlands to the optimal habitat for K. obovata was the largest (15.71 km2), and that for A. marina was 11.78 km2. The area transferred from aquaculture ponds to eugenic areas for mangroves was the largest for A. corniculatum (13.63 km2) and mainly distributed in Lianzhou Bay (Figure 9); this area was 11.33 km2 for K. obovata. This indicated that suitable wetlands can be developed in the region for mangrove restoration, particularly for K. obovata and A. marina. Furthermore, restoring aquaculture ponds to forest could constitute the main measure for mangrove restoration, with A. corniculatum and K. obovate as well-suited restoration species.
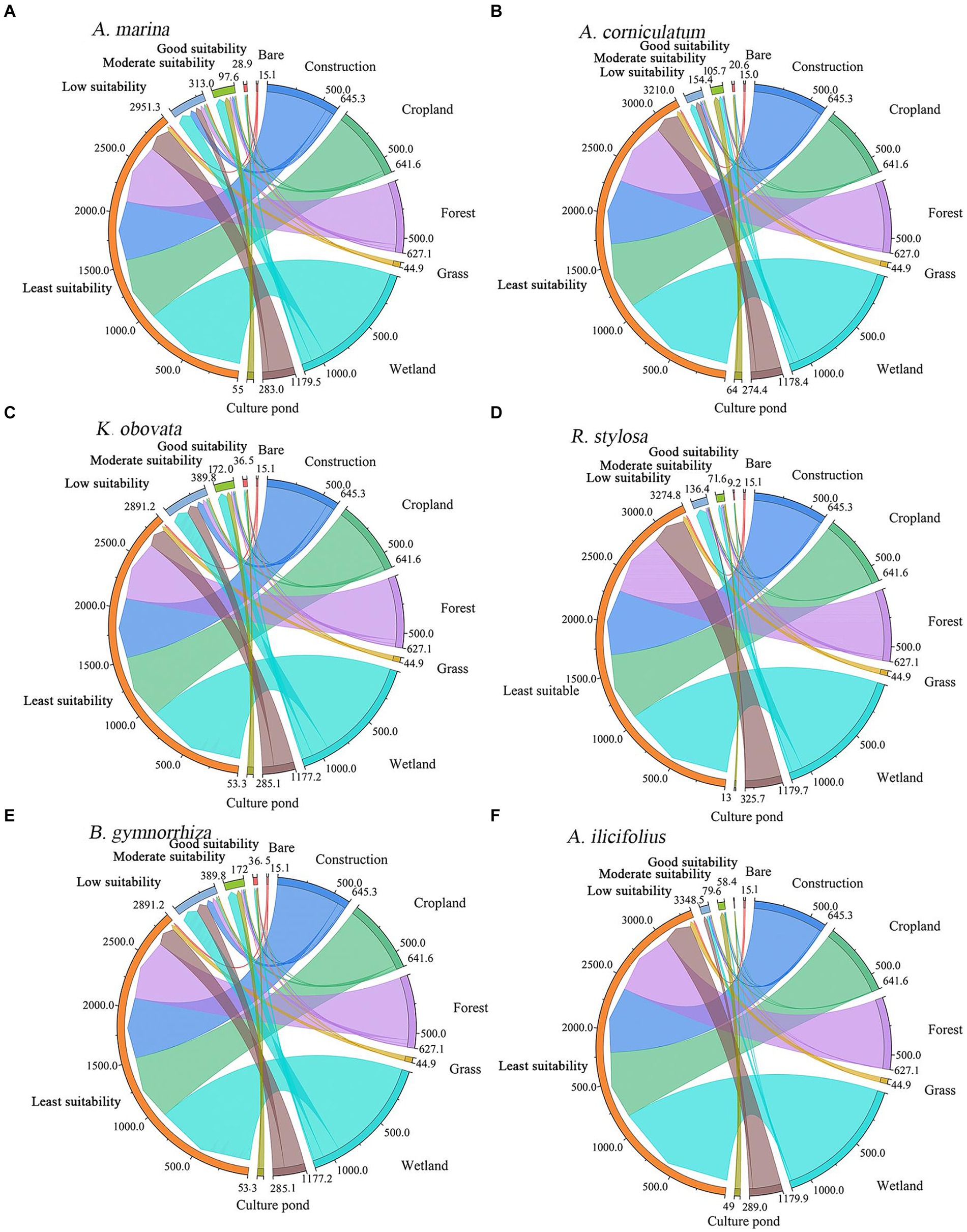
Figure 7. Transfer direction between different mangrove habitats and landscape types for: (A) A. marina, (B) A. corniculatum, (C) K. obovate, (D) R. st ylosa, (E) B. gymnorrhiza, and (F) A. ilicifolius.
3.4 Protection status and priorities
Based on the superposition of the distribution of protected and potentially suitable mangrove areas, 55.04% of the most suitable localities were included within protected areas (Figure 10). Six areas were identified as priority areas for mangrove protection and restoration: Dafeng, Lianzhou, Jinhai, Xicun, Tieshangang Bay, and Shankou mangrove reserves. The unprotected mangrove areas in Beihai were primarily distributed in Lianzhou Bay, Dafeng River, Xicun Port, and Tieshangang Bay.
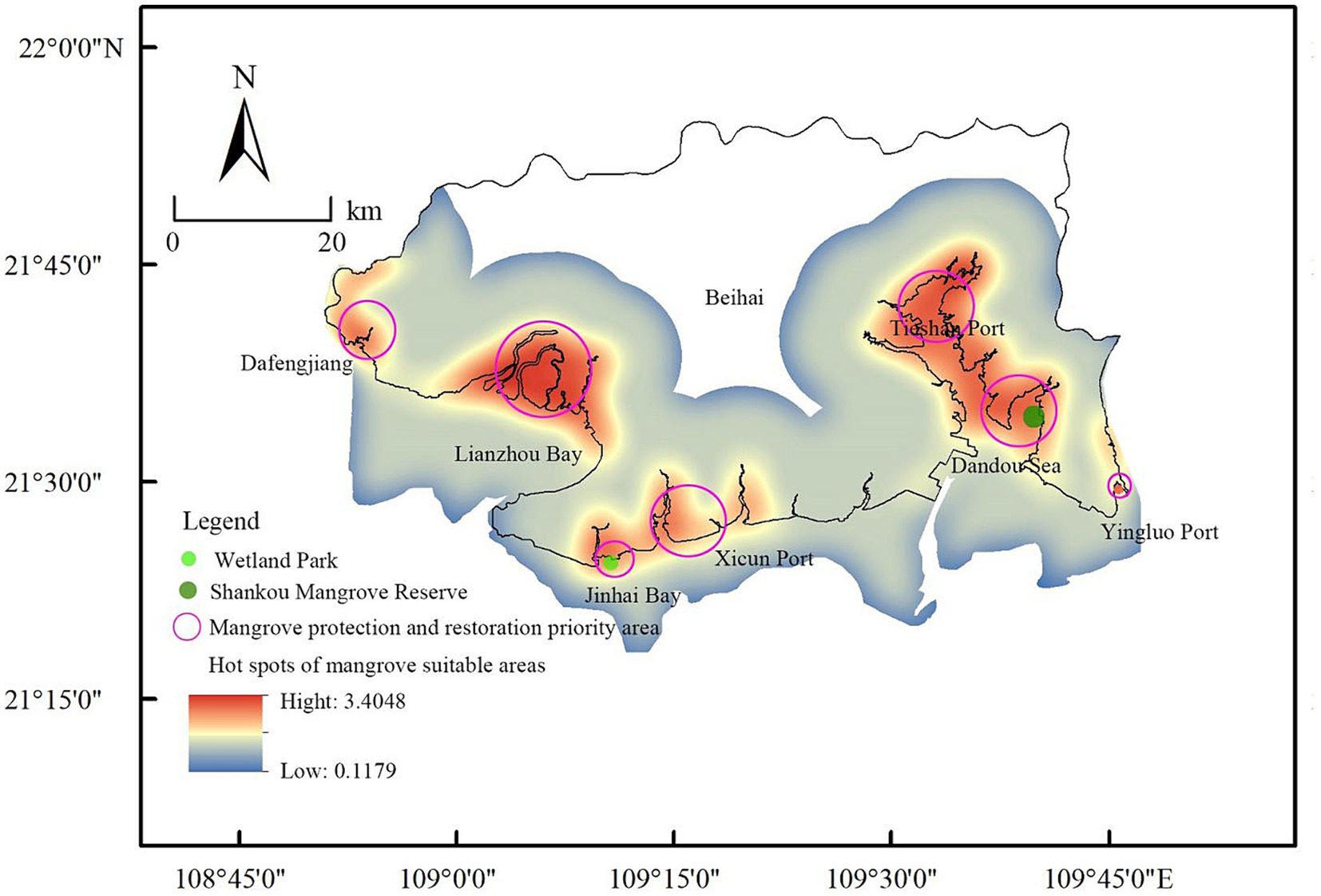
Figure 10. Mangrove suitability hotspot map and priority area of mangrove conservation and restoration.
4 Discussion
Previous studies have focused on predicting the mangrove distribution and paid minimal attention to the potentially available land sources for mangrove eugenic areas (Wang et al., 2021). On the basis of predicting the mangrove distribution, this study explored suitable land sources for mangrove growth to effectively guide mangrove protection, restoration, and management.
4.1 Dominant environmental factors affecting suitability for mangroves
Analysis of the combined mangrove forests distributed along the coast of Beihai City indicated that the influence of bioclimatic factors was likely to be less significant than that of topographic factors. In contrast, a previous study on factors predicting the mangrove distribution in Fujian found that bioclimate and SST were the most relevant (Hu et al., 2020a), which may have been due to differences in geographical latitude between the two regions. The limited regional geographic latitude span of the present study (20°26′–21°55′34″N) resulted in a small SST range; consequently, its influence on the mangrove distribution was relatively weak.
The distribution of Rhizophora stylosa and B. gymnorrhiza was more sensitive to bioclimatic factors than that of other mangroves, which constituted 49.9 and 53.3% of the factor influence, respectively. The sensitivity of these two species to bioclimatic factors has previously been empirically demonstrated (Li et al., 2023), which is consistent with our prediction of their distribution in Beibu Gulf.
Elevation greatly affects the mangrove distribution (Leong et al., 2018). The contribution of terrain factors to the predicted habitat suitability for A. marina and K. obovata was greater than that of other factors, indicating that these species were more sensitive to this type of habitat characteristics. The effect of topographical factors on the mangrove distribution decreased in the order A. marina > K. obovate > A. corniculatum > A. ilicifolius > R. stylosa > B. gymnorrhiza. Related studies have shown that A. marina is more tolerant of low intertidal altitude than K. obovata (Chen and Ye, 2013), which is consistent with the conclusions of the present study.
Sea-surface salinity is an important factor affecting the mangrove distribution (Barik et al., 2018; Sinsin et al., 2021). In this study, the predicted distribution areas of A. corniculatum and A. ilicifolius were mainly in Lianzhou Bay, which has low salinity. Aricennia marina was distributed in Tieshan gang Bay, Beihai Jinhai Bay Mangrove Reserve, and the southern coast of Beihai National Wetland Park, where salinity is relatively high. Among these three species, A. marina was previously found to have the highest salt tolerance, and A. ilicifolius was found to have the lowest salt tolerance (Ye et al., 2004), which is consistent with the findings of the present study.
4.2 Model applicability
We applied an optimized MaxEnt model in this study to predict the potential spatial distribution pattern of mangroves in Beihai city. The evaluation results can be compared with the current distribution status of mangroves and the area of eugenic zones.
According to the Beihai Mangrove Resource Protection Plan (2020–2030), the suitable area for planting mangroves is 49.52 km2 (Beihai Municipal People’s Government, 2021). These data were sourced from the third land survey of China, which mainly employed remote-sensing image interpretation, on-site investigation, and digital image processing techniques. The model results in the present study predicted a similar optimal area for mangrove growth (50.76 km2); thus, the modeled mangrove distribution was consistent with the current situation, which confirmed the accuracy of the optimized MaxEnt model for predicting suitable areas for mangrove growth.
4.3 Main land sources for mangrove restoration
Aquaculture ponds have been a focus of attention as potential mangrove restoration sites worldwide (Winterwerp et al., 2005; Matsui et al., 2012). Related research suggests that at a regional-scale in China, approximately 6,400 ha of ponds are used for aquaculture, accounting for 38% of the theoretically recoverable area (Hu et al., 2020b). Loss of mangroves can be attributed to conversion of agriculture/aquaculture and dock and road construction, with pond aquaculture being the main cause of global mangrove destruction (Thomas et al., 2017; Elwin et al., 2019), as shrimp pond pollution affects mangrove growth (Zhang and Liao, 2022).
We focused on the overall mangrove forest in our land-use transfer analysis and found that transfer of aquaculture ponds could provide the largest optimal habitat area. The area of aquaculture ponds suited for mangrove growth was 23.71 km2, while that of wetlands suitable for mangrove growth was 12.25 km2 (Figure 9), indicating that aquaculture ponds constitute primary and promising candidate areas for mangrove restoration, which is consistent with the findings of a previous study (Su et al., 2022). We analyzed six mangrove species separately (Figure 8) and found that the transfer of aquaculture ponds was the most suitable for the growth of A. corniculatum (with 13.63 km2). Therefore, A. corniculatum can be selected as the primary plant when ecologically restoring aquaculture ponds.
The factors that affected mangrove growth included bioclimate, terrain, substrate type, sea-surface salinity, land-use classification, and SST. However, our assessment was limited in that chemical properties of the soil at the site (e.g., potassium, phosphorus, and lead contents) and hydrodynamic force were not considered. Significant differences may exist between soil samples from natural mangroves and traditional or non-traditional shrimp ponds in terms of pH, conductivity, and phosphorus, lead, and chromium contents (Krishnapriya et al., 2023). In the long term, changes in these soil chemical parameters will affect organisms and limit further mangrove restoration. Related studies have shown that ponds located on peat soil are unsustainable and require complete restoration if they are to be converted to mangrove forests, and that ponds on other soil types can be restored into a mixed system of mangrove ponds (Bosma et al., 2012). Owing to differences in soil chemical properties, abandoned ponds may be restored for shrimp and fish production or become targets for mangrove restoration (Aslan et al., 2021). It is difficult to accurately obtain hydrological measurements in mangrove coastal areas, and certain key physical quantities are difficult to parameterize and especially difficult to generalize in hydrodynamic calculations. Thus, in future research, the impact of hydrodynamic force factors on mangroves should be investigated.
4.4 Mangrove protection and restoration strategies
(1) In 2013 and 2021, the mangrove forest area in Beihai City was 32.64 km2 (Tao et al., 2017) and 41.93 km2, respectively. Overall, a growing trend in the area of mangrove forests was observed in Beihai, and local mangroves have been well protected. The model results of this study predict that the suitable forest area for mangrove growth is 50.76 km2. Notably, although a large area of suitable forest land remains, the development of shrimp pond aquaculture in the area is taking up the space suitable for the growth of mangrove forests. The proportion of the mangrove breeding shoreline adjacent to Guangxi is far higher than that of natural and reclamation shorelines, and a large area of coastal mudflats has been reclaimed, resulting in the continuous occupation of suitable mangrove forest area (Peng et al., 2016). However, returning ponds to forests is also likely to cause economic losses and livelihood issues for coastal villagers who previously engaged in aquaculture (Tang et al., 2023). To address these conflicts, relevant departments should prioritize mangrove restoration in shrimp ponds suitable for mangrove cultivation.
(2) Based on the vacancy analysis results, priority should be given to the protection and restoration of mangroves in Lianzhou Bay, Dafeng River, Xichun Port, and Tieshangang Bay. According to literature records, mangroves are present in these areas (Tao et al., 2017). Currently, artificial mangroves, rather than seagrass or salt marshes, are developed. The sedimentary layer of Lianzhou Bay is mainly composed of muddy soil (Wang R. M. et al., 2020). One national level mangrove protection area (Shankou Mangrove Forest Protection Area) is located near Tieshangang Bay. In 2013, the area of mangroves in Tieshangang Bay was 11.12 km2 (Tao et al., 2017). The wetlands suitable for transfer into a moderately suitable area for mangrove growth are mainly located in the coastal region of Tieshangang Bay. However, there are only few tree species in Tieshangang Bay, mainly A. marina, which is consistent with previous research (Tao et al., 2017). Therefore, we suggest using A. marina in Tieshangang Bay for restoration. New wetland parks or protected areas can be constructed in the above-mentioned areas or incorporated into existing protected areas to protect the existing mangroves, and selection of sites within the optimal mangrove habitat should be prioritized to conduct mangrove restoration.
A limitation of this study was that soil chemical properties were not considered among the model’s environmental factors. Therefore, in future research, chemical soil parameters in shrimp ponds suitable for mangrove growth should be monitored. Soil properties should be added as influencing factors in model analysis for improving the accuracy of distribution prediction. The present study focused on mangrove species indigenous to Beihai and did not cover the invasive species Sonneratia apetala. Future studies should aim to incorporate such invasive taxa, thereby broadening the taxonomic scope and the utility of the predictive model for both conservation and ecological restoration efforts.
5 Conclusion
Mangrove distribution prediction and identification of suitable land sources are important in mangrove restoration. In this study, we evaluated the suitable environmental conditions for the six main mangrove tree species in Beihai City, Guangxi Province. The results indicated that topographical factors were the strongest drivers of the spatial distribution of A. marina, A. corniculatum, and K. obovata, and that soil type was more important for A. ilicifolius. We identified the land sources suited as the optimal growth areas for mangroves, and transformation of aquaculture ponds into mangrove habitats appeared to be the most effective ecosystem restoration approach. The aquaculture ponds located in Lianzhou Bay are an important mangrove restoration area, and A. corniculatum should be the priority tree species.
The model predictions suggested strategies for targeted mangrove restoration. For example, A. marina exhibited a marked preference for littoral zones such as Tieshangang Bay and Jinhai Bay, and A. ilicifolius preferred low-salinity environments in Lianzhou Bay. The distribution prediction map of the six main mangrove plants in Beihai City created in this study provides a basis for mangrove restoration and protection. Areas such as Lianzhou Bay, Tieshangang Bay, and Xicun Port were particularly suitable as mangrove habitats. Notably, 55.04% of these areas are already protected.
Our findings have potential to provide guidance for the demarcation of future or expanded protection areas, thereby improving effective reforestation management. Our results provide new insights and a theoretical basis for future mangrove protection and restoration.
Data availability statement
The original contributions presented in the study are included in the article/supplementary material, further inquiries can be directed to the corresponding author.
Author contributions
LL: Methodology, Writing – original draft, Writing – review & editing. LW: Funding acquisition, Methodology, Writing – original draft. WM: Methodology, Writing – review & editing, Software. CS: Investigation, Software, Writing – review & editing. LF: Investigation, Software, Writing – review & editing. XX: Investigation, Writing – review & editing. TY: Investigation, Writing – review & editing. XY: Investigation, Writing – review & editing. JW: Investigation, Writing – review & editing.
Funding
The author(s) declare financial support was received for the research, authorship, and/or publication of this article. This work was supported by the National Natural Science Foundation of China (grant numbers U21A2022 and 32060282), the Research Fund of Program of Guangxi Key Lab of Mangrove Conservation and Utilization (GKLMC-20A06) and Innovation and Development fund of Guangxi Academy of Sciences (2024YGFZ504-101).
Acknowledgments
We would like to thank Guangxi Key Lab of Mangrove Conservation and Utilization for providing financial support.
Conflict of interest
The authors declare that the research was conducted in the absence of any commercial or financial relationships that could be construed as a potential conflict of interest. The author(s) declared that they were an editorial board member of Frontiers, at the time of submission. This had no impact on the peer review process and the final decision.
Publisher’s note
All claims expressed in this article are solely those of the authors and do not necessarily represent those of their affiliated organizations, or those of the publisher, the editors and the reviewers. Any product that may be evaluated in this article, or claim that may be made by its manufacturer, is not guaranteed or endorsed by the publisher.
Footnotes
1. ^https://github.com/marlonecobs/kuenm
2. ^http://biodiversityinformatics.amnh.org/open_source/maxent/
References
Alongi, D. M. (2015). The impact of climate change on mangrove forests. Curr. Clim. Chang. Rep. 1, 30–39. doi: 10.1007/s40641-015-0002-x
Aslan, A., Rahman, A. F., Robeson, S. M., and Ilman, M. (2021). Land-use dynamics associated with mangrove deforestation for aquaculture and the subsequent abandonment of ponds. Sci. Total Environ. 791:148320. doi: 10.1016/j.scitotenv.2021.148320
Balke, T., and Friess, D. A. (2016). Geomorphic knowledge for mangrove restoration: a pan-tropical categorization. Earth Surf. Process. Landf. 41, 231–239. doi: 10.1002/esp.3841
Banerjee, A. K., Feng, H., Lin, Y. T., Liang, X. R., Wang, J. K., and Huang, Y. L. (2022). Setting the priorities straight - species distribution models assist to prioritize conservation targets for the mangroves. Sci. Total Environ. 806:150937. doi: 10.1016/j.scitotenv.2021.150937
Bao, R., Li, X., and Zheng, J. (2022). Feature tuning improves MAXENT predictions of the potential distribution of Pedicularis longiflora Rudolph and its variant. PeerJ 10:e13337. doi: 10.7717/peerj.13337
Barik, J., Mukhopadhyay, A., Ghosh, T., Mukhopadhyay, S. K., Chowdhury, S. M., and Hazra, S. (2018). Mangrove species distribution and water salinity: an indicator species approach to Sundarban. J. Coast. Conserv. 22, 361–368. doi: 10.1007/s11852-017-0584-7
Beihai Municipal People’s Government . (2021). Protection plan for mangrove resources in Beihai City. [EB/OL]. Available at: https://W020221229416771871178.pdf.beihai.gov.cn (Accessed May 21, 2024).
Bertness, M. D., Ewanchuk, P. J., and Silliman, B. R. (2002). Anthropogenic modification of New England salt marsh landscapes. Proc. Natl. Acad. Sci. USA 99, 1395–1398. doi: 10.1073/pnas.022447299
Bosma, R., Sidik, A. S., van Zwieten, P., Aditya, A., and Visser, L. (2012). Challenges of a transition to a sustainably managed shrimp culture agro-ecosystem in the Mahakam Delta, East Kalimantan, Indonesia. Wetl Ecol. Manag. 20, 89–99. doi: 10.1007/s11273-011-9244-0
Cai, X. J., Wu, Z. F., and Cheng, J. (2012). Analysis of road network pattern and landscape fragmentation based on kernel density estimation. Chin. J. Ecol. 31, 160–166. doi: 10.13292/j.1000-4890.2012.0035
Chao, B. X., Hu, W. J., Chen, B., Zhang, D., Chen, G. C., Yu, W. W., et al. (2020). Potential suitable habitat of mangroves and conservation gap analysis in Guangdong Province with MaxEnt modeling. Chin. J. Ecol. 39, 3785–3794. doi: 10.13292/j.1000-4890.202011.009
Chao, B. X., Wang, Y. Y., Yu, W. W., Ma, Z. Y., Chen, G. C., Chen, B., et al. (2021). Predicting mangrove forest distribution driven by land uses in Guangdong Province. China Environ. Sci. 41, 5282–5291. doi: 10.19674/j.cnki.issn1000-6923.20210416.001
Charrua, A. B., Bandeira, S. O., Catarino, S., Cabral, P., and Romeiras, M. M. (2020). Assessment of the vulnerability of coastal mangrove ecosystems in Mozambique. Ocean Coast. Manag. 189:105145. doi: 10.1016/j.ocecoaman.2020.105145
Chen, Y., and Ye, Y. (2013). Growth and physiological responses of saplings of two mangrove species to intertidal elevation. Mar. Ecol. Prog. Ser. 482, 107–118. doi: 10.3354/meps10274
Cobben, M. M. P., van Treuren, R., Castañeda-Álvarez, N. P., Khoury, C. K., Kik, C., and van Hintum, T. J. L. (2015). Robustness and accuracy of Maxent niche modelling for Lactuca species distributions in light of collecting expeditions. Plant Genet. Resour. 13, 153–161. doi: 10.1017/S1479262114000847
Cobos, M. E., Peterson, A. T., Barve, N., and Osorio-Olvera, L. (2019). Kuenm: an R package for detailed development of ecological niche models using Maxent. PeerJ 7:e6281. doi: 10.7717/peerj.6281
Curnick, D. J., Pettorelli, N., Amir, A. A., Balke, T., Barbier, E. B., Crooks, S., et al. (2019). The value of small mangrove patches. Science 363:239. doi: 10.1126/science.aaw0809
Elwin, A., Bukoski, J. J., Jintana, V., Robinson, E. J. Z., and Clark, J. M. (2019). Preservation and recovery of mangrove ecosystem carbon stocks in abandoned shrimp ponds. Sci. Rep. 9:18275. doi: 10.1038/s41598-019-54893-6
Fan, H. Q., and Mo, Z. C. (2018). The history, achievements and lessons learnt for mangrove restoration in Guangxi, China. Guangxi Sci. 25, 363–371. doi: 10.13656/j.cnki.gxkx.20180918.001
Gerona-Daga, M. E. B., and Salmo, S. G. A. (2022). A systematic review of mangrove restoration studies in Southeast Asia: challenges and opportunities for the United Nation’s Decade on Ecosystem Restoration. Front. Mar. Sci. 9:987737. doi: 10.3389/fmars.2022.987737
Hai, N. T., Dell, B., Phuong, V. T., and Harper, R. J. (2020). Towards a more robust approach for the restoration of mangroves in Vietnam. Ann. Forest Sci. 77:18. doi: 10.1007/s13595-020-0921-0
He, B. Y., Lai, T. H., Chen, J. F., and Qiu, G. L. (2007). Studies of the tolerance of Avicennia marina and Aegiceras corniculatum to seawater immersion in Guangxi, China. Acta Ecol. Sin. 27, 1131–1138.
Hu, W. J., Chao, B., Wang, Y., Dong, P., Zhang, D., Yu, W., et al. (2020). Assessing the potential distributions of mangrove forests in Fujian Province using MaxEnt model. China Environ. Sci. 40, 4029–4038. doi: 10.19674/j.cnki.issn1000-6923.2020.0448
Hu, W., Wang, Y., Dong, P., Zhang, D., Yu, W., Ma, Z., et al. (2020a). Predicting potential mangrove distributions at the global northern distribution margin using an ecological niche model: determining conservation and reforestation involvement. Forest Ecol. Manag. 478:118517. doi: 10.1016/j.foreco.2020.118517
Hu, W., Wang, Y., Zhang, D., Yu, W., Chen, G., Xie, T., et al. (2020b). Mapping the potential of mangrove forest restoration based on species distribution models: a case study in China. Sci. Total Environ. 748:142321. doi: 10.1016/j.scitotenv.2020.142321
Jia, M. M., Wang, Z. M., Mao, D. H., Ren, C. Y., Song, K. S., Zhao, C. P., et al. (2023). Mapping global distribution of mangrove forests at 10-m resolution. Sci. Bull. 68, 1306–1316. doi: 10.1016/j.scib.2023.05.004
Jumawan, J. H., and Macandog, D. M. (2021). GIS weighted suitability analysis as decision support tool for mangrove rehabilitation in Oriental Mindoro, Philippines. J. Ecosyst. Sci. Eco-Gov. 3, 1–13.
Krishnapriya, P., Bijith, P., and Sandeep, S. (2023). Physicochemical characteristics of shrimp ponds on mangrove ecosystems in Kannur District of Kerala, India. Wetl. Ecol. Manag. 31, 287–296. doi: 10.1007/s11273-023-09916-5
Lan, W. L., Li, T. S., and Han, L. J. (2014). Distribution and seasonal variation of nutrition in the adjacent waters of Tieshangang Bay in Guangxi. Mar. Sci. 38, 63–69. doi: 10.11759/hykx20100812001
Leong, R. C., Friess, D. A., Crase, B., Lee, W. K., and Webb, E. L. (2018). High-resolution pattern of mangrove species distribution is controlled by surface elevation. Estuar. Coast. Shelf Sci. 202, 185–192. doi: 10.1016/j.ecss.2017.12.015
Li, C. G., and Dai, H. B. (2015). Mechanism analysis of temporal dynamics in mangrove spatial distribution in Guangxi, China: 1960–2010. Acta Ecol. Sin. 35, 5992–6006. doi: 10.5846/stxb201401160119
Li, D. X., Li, Z. X., Liu, Z. W., Yang, Y. J., Khoso, A. G., Wang, L., et al. (2023). Climate change simulations revealed potentially drastic shifts in insect community structure and crop yields in China’s farmland. J. Pest. Sci. 96, 55–69. doi: 10.1007/s10340-022-01479-3
Li, L. F., Liu, W., Ai, J., Cai, S. J., and Dong, J. (2023). Predicting mangrove distributions in the Beibu Gulf, Guangxi, China, using the MaxEnt model: determining tree species selection. Forests 14:149. doi: 10.3390/f14010149
Liu, W. A., Li, L. F., Xue, Y. H., and Tao, Y. C. (2021). Study on the population size and distribution of Rhizophora stylosa in Lan'gen village of Beihai City based on Google earth high image. J. Guangxi Acad. Sci. 37:264269. doi: 10.13657/j.cnki.gxkxyxb.20210928.010
Liu, X., Liu, H., Gong, H., Lin, Z., and Lv, S. (2017). Appling the one-class classification method of Maxent to detect an invasive plant Spartina alterniflora with time-series analysis. Remote Sens. 9:112011. doi: 10.3390/rs9111120
Liu, H., Qi, X., Gong, H., Li, L., Zhang, M., Li, Y., et al. (2019). Combined effects of global climate suitability and regional environmental variables on the distribution of an invasive marsh species spartina alterniflora. Estuar. Coasts 42, 99–111. doi: 10.1007/s12237-018-0447-y
Matsui, N., Morimune, K., Meepol, W., and Chukwamdee, J. (2012). Ten year evaluation of carbon stock in mangrove plantation reforested from an abandoned shrimp pond. Forests 3, 431–444. doi: 10.3390/f3020431
Mukul, S. A., Alamgir, M., Sohel, M. S. I., Pert, P. L., Herbohn, J., Turton, S. M., et al. (2019). Combined effects of climate change and sea-level rise project dramatic habitat loss of the globally endangered Bengal tiger in the Bangladesh Sundarbans. Sci. Total Environ. 663, 830–840. doi: 10.1016/j.scitotenv.2019.01.383
Peng, Y., Zheng, M., Zheng, Z., Wu, G., Chen, Y., Xu, H., et al. (2016). Virtual increase or latent loss? A reassessment of mangrove populations and their conservation in Guangdong, southern China. Mar. Pollut. Bull. 109, 691–699. doi: 10.1016/j.marpolbul.2016.06.083
Peterson, A. T., Papeş, M., and Eaton, M. (2007). Transferability and model evaluation in ecological niche modeling: a comparison of GARP and Maxent. Ecography 30, 550–560. doi: 10.1111/j.0906-7590.2007.05102.x
Polidoro, B. A., Carpenter, K. E., Collins, L., Duke, N. C., Ellison, A. M., Ellison, J. C., et al. (2010). The loss of species: mangrove extinction risk and geographic areas of global concern. PLoS One 5:e10095. doi: 10.1371/journal.pone.0010095
Radosavljevic, A., and Anderson, R. P. (2014). Making better Maxent models of species distributions: complexity, overfitting and evaluation. J. Biogeogr. 41, 629–643. doi: 10.1111/jbi.12227
Record, S., Fitzpatrick, M. C., Finley, A. O., Veloz, S., and Ellison, A. M. (2013). Should species distribution models account for spatial autocorrelation? A test of model projections across eight millennia of climate change: projecting spatial species distribution models. Glob. Ecol. Biogeogr. 22, 760–771. doi: 10.1111/geb.12017
Renzi, J. J., He, Q., and Silliman, B. R. (2019). Harnessing positive species interactions to enhance coastal wetland restoration. Front. Ecol. Evol. 7:131. doi: 10.3389/fevo.2019.00131
Rodríguez-Medina, K., Yañez-Arenas, C., Peterson, A. T., Euán Ávila, J., and Herrera-Silveira, J. (2020). Evaluating the capacity of species distribution modeling to predict the geographic distribution of the mangrove community in Mexico. PLoS One 15:e0237701. doi: 10.1371/journal.pone.0237701
Shi, P. J., Chen, J., and Pan, Y. Z. (2000). Landuse change mechanism in Shenzhen City. Acta Geograph. Sin. 55, 151–160. doi: 10.3321/j.issn:0375-5444.2000.05.003
Sinsin, C. B. L., Salako, K. V., Fandohan, A. B., Zanvo, M. G. S., Kouassi, K. E., and Glèlè Kakaï, R. L. G. (2021). Pattern of seedling emergence and early growth in Avicennia germinans and Rhizophora racemosa along an experimental salinity gradient. Afr. J. Ecol. 59, 1013–1022. doi: 10.1111/aje.12889
Su, J., Yin, B., Chen, L., and Gasparatos, A. (2022). Priority areas for mixed-species mangrove restoration: the suitable species in the right sites. Environ. Res. Lett. 17:065001. doi: 10.1088/1748-9326/ac6b48
Tang, D. B., Xin, C. L., Chen, N., Liu, X. J., Zhang, L., and Liu, X. J. (2023). Mangrove forest fragmentation and its ecological service value in Tongming Sea of Zhanjiang, Guangdong. Chin. J. Appl. Ecol. 34, 415–422. doi: 10.13287/j.1001-9332.202302.022
Tao, Y. C., Ge, W. B., Liu, W. A., Pan, L. H., Qiu, G. L., Wang, X., et al. (2017). A survey on the spatial distribution and community types of mangroves in Guangxi based on high—resolution satellite imageries. J. Nat. Resour. 32, 1602–1614. doi: 10.11849/zrzyxb.20160803
Thomas, N., Lucas, R., Bunting, P., Hardy, A., Rosenqvist, A., and Simard, M. (2017). Distribution and drivers of global mangrove forest change, 1996–2010. PLoS One 12:e0179302. doi: 10.1371/journal.pone.0179302
Tu, C. H., Lo, N. J., Chang, W. I., and Huang, K. Y. (2012). Evaluating the novel methods on species distribution modeling in complex forest. Int. arch. Photogramm. Remote Sens. Spat. Inf. Sci. ISPRS Arch. 39, 77–82. doi: 10.5194/isprsarchives-XXXIX-B2-77-2012
Valiela, I., Bowen, J. L., and York, J. K. (2001). Mangrove forests: one of the world’s threatened major tropical environments. Bioscience 51, 807–815. doi: 10.1641/0006-3568(2001)051[0807:MFOOTW]2.0.CO;2
Wan, J. Z., Wang, C. J., and Yu, F. H. (2019). Effects of occurrence record number, environmental variable number, and spatial scales on MaxEnt distribution modelling for invasive plants. Biologia 74, 757–766. doi: 10.2478/s11756-019-00215-0
Wang, Y., Chao, B., Dong, P., Zhang, D., Yu, W., Hu, W., et al. (2021). Simulating spatial change of mangrove habitat under the impact of coastal land use: coupling MaxEnt and dyna-CLUE models. Sci. Total Environ. 788:147914. doi: 10.1016/j.scitotenv.2021.147914
Wang, R. M., Dai, Z. J., Huang, H., Li, S. S., Hu, B. Q., Zhou, X. Y., et al. (2020). Spatial patterns of the mangrove along the riverine estuaries, Nanliujiang River and Dafengjiang River of the Beibu Gulf. Haiyang Xuebao 42, 54–61. doi: 10.3969/j.issn.0253−4193.2020.12.006
Wang, W., Fu, H., Lee, S. Y., Fan, H., and Wang, M. (2020). Can strict protection stop the decline of mangrove ecosystems in China? From rapid destruction to rampant degradation. Forests 11:55. doi: 10.3390/f11010055
Wang, Y., Xie, B., Wan, F., Xiao, Q., and Dai, L. (2007). Application of ROC curve analysis in evaluating the performance of alien species’ potential distribution models. Biodivers. Sci. 15, 365–372. doi: 10.1360/biodiv.060280
Warren, D. L., and Seifert, S. N. (2011). Ecological niche modeling in Maxent: the importance of model complexity and the performance of model selection criteria. Ecol. Appl. 21, 335–342. doi: 10.1890/10-1171.1
Wei, M. X., He, B. M., and Tong, W. P. (2006). Frontal characteristic of the salinity and its relations to environmental factors in Nanliujiang Estuary, Guangxi. J. Oceanogr. Taiwan Strait. 25, 526–532. doi: 10.3969/j.issn.1000-8160.2006.04.011
West, A. M., Kumar, S., Brown, C. S., Stohlgren, T. J., and Bromberg, J. (2016). Field validation of an invasive species Maxent model. Ecol. Inform. 36, 126–134. doi: 10.1016/j.ecoinf.2016.11.001
Winterwerp, J. C., Borst, W. G., and De Vries, M. B. (2005). Pilot study on the erosion and rehabilitation of a mangrove mud coast. J. Coast. Res. 212, 223–230. doi: 10.2112/03-832A.1
Ye, Y., Lu, C. Y., Hu, H. Y., and Tang, F. Y. (2004). Comparisons of tolerances to salt stress among three salt-secreting mangrove species. Acta Ecol. Sin. 24, 2444–2450. doi: 10.3321/j.issn:1000-0933.2004.11.014
Keywords: maximum entropy model, Beihai, mangrove, habitat suitability, restoration
Citation: Lifeng L, Wenai L, Mo W, Shuangjiao C, Fuqin L, Xiaoling X, Yancheng T, Yunhong X and Weiguo J (2024) Analysis of mangrove distribution and suitable habitat in Beihai, China, using optimized MaxEnt modeling: improving mangrove restoration efficiency. Front. For. Glob. Change. 7:1293366. doi: 10.3389/ffgc.2024.1293366
Edited by:
Jiang Jiang, Nanjing Forestry University, ChinaReviewed by:
Prashant Sharma, Dr. Yashwant Singh Parmar University of Horticulture and Forestry, IndiaPhoebe Jan Stewart-Sinclair, National Institute of Water and Atmospheric Research, New Zealand
Xu Shusheng, South China Sea Institute of Planning and Environment Research, China
Copyright © 2024 Lifeng, Wenai, Mo, Shuangjiao, Fuqin, Xiaoling, Yancheng, Yunhong and Weiguo. This is an open-access article distributed under the terms of the Creative Commons Attribution License (CC BY). The use, distribution or reproduction in other forums is permitted, provided the original author(s) and the copyright owner(s) are credited and that the original publication in this journal is cited, in accordance with accepted academic practice. No use, distribution or reproduction is permitted which does not comply with these terms.
*Correspondence: Wenai Liu, liuwenai@mangrove.org.cn