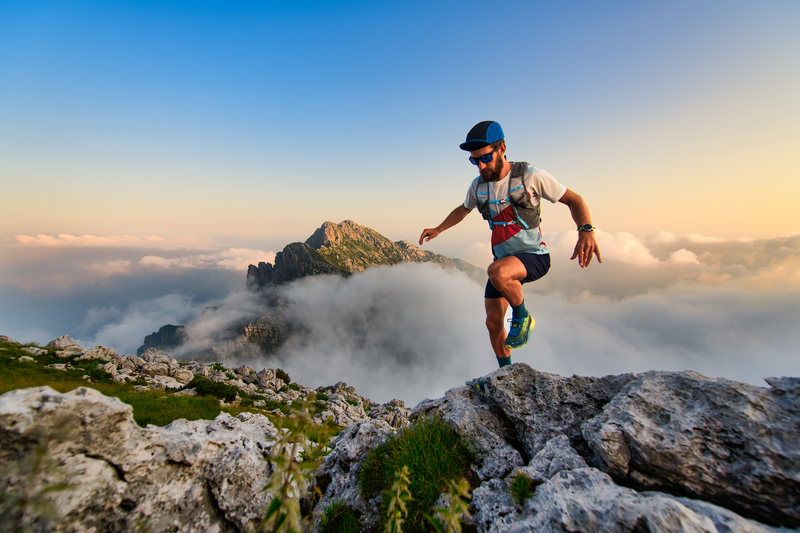
95% of researchers rate our articles as excellent or good
Learn more about the work of our research integrity team to safeguard the quality of each article we publish.
Find out more
ORIGINAL RESEARCH article
Front. For. Glob. Change , 11 January 2024
Sec. People and Forests
Volume 6 - 2023 | https://doi.org/10.3389/ffgc.2023.1322660
This article is part of the Research Topic Agroforestry for biodiversity and ecosystem services View all 8 articles
Inappropriate management of land use systems is one of the main factors that leads to soil quality degradation and its quantification is crucial to their sustainable utilization planning. The objective of the research is to evaluate how various land-use systems, viz., natural forest, tree plantations of Tectona grandis, Terminalia bellirica, Swietenia macrophylla, Artocarpus hirsutus, Melia dubia based agroforestry system, horticulture (Mangifera indica) and agriculture systems impact the soil physicochemical and biological characteristics in semi-arid climatic conditions of India. Principal component analysis followed by linear and non-linear scoring methods was employed to compute the soil quality index (SQI). The soil attributes viz., dehydrogenase activity, acid phosphatase activity, soil available nitrogen, potassium, calcium, porosity, and soil available iron emerged as significant indicators for assessing the soil quality. Among different SQIs, non-linear weighted SQI can efficiently assess soil quality. Based on the non-linear weighted SQI, the order of the systems studied was natural forest (0.973) > Swietenia macrophylla (0.756) > agroforestry (0.737) > agriculture (0.556) > Tectona grandis (0.416) > Terminalia bellirica (0.373) > Artocarpus hirsutus (0.343) > Mangifera indica (0.208). The study concludes that converting natural forests into different land-use systems deteriorated the soil quality. Identifying soil indicators will help rapidly diagnose soil degradation, assess soil-based ecosystem services, and design appropriate land management practices in the future.
Soil is one of the vital component of the terrestrial ecosystem that has an indirect and direct impact on all living forms. Soil health has been one of the critical deciding factors in the success or failure of human civilization. There has been wide-scale deforestation over the years to meet the growing needs of food, fodder, and timber due to the increasing population and urbanization. Continuous deforestation converted natural forests into different land uses like tree plantations, horticulture, agroforestry, agriculture, etc. To meet the production goals, the farming community has opted for improper soil management and land use techniques, such as monoculture, intensive cropping patterns with heavy mechanization, and injudicious usage of agri-chemicals (Chandel et al., 2018). Soil health and sustainability have always been out of context in this due course of development. Unscientific agricultural intensification to achieve self-sufficiency has damaged soils and accelerated its degradation (Jinger et al., 2023). The two main forms of degradation linked with poor soil management practices in semi-arid environments are soil nutrient depletion and soil physical deterioration (Jien and Wang, 2013; Ghaemi et al., 2014; Trivedi et al., 2016; Chemeda et al., 2017; Jinger et al., 2023). Different vegetation types can significantly alter the physical, chemical, and biological aspects of the soil (Tauqeer et al., 2022). They can have long-term effects on soil characteristics (Li et al., 2019), and these changes may be used as a crucial tool to evaluate the changes in soil quality (SQ) (Liu et al., 2018). Therefore, SQ assessments are necessary to understand the state of the soil and create more effective management strategies (Qi et al., 2009).
The soil has to be assessed regularly to monitor the change in different SQ parameters. To assess SQ, it is essential to measure every soil property that indicates a quantifiable soil characteristic impacting the soil’s capacity to fulfill a particular function (Karlen et al., 1997). Developing a soil quality index (SQI) with a minimal set of characteristics has been found to indicate the capability of the soil to perform owing to changes in the management techniques, such as land-use changes (Doran and Parkin, 1994; Karlen and Stott, 1994; Raiesi, 2017). There are fewer studies comparing the SQ under various land use systems (LUSs). Rashkow (2014) investigated the SQ of forested, converted, and cropland areas and noted that forested areas exhibited the highest level of SQ, followed by converted and it was least in cropland areas. Chandel et al. (2018) observed that the SQI in the forest was highest than any other LUS in Submontane Punjab. Zou et al. (2021) suggested that SQI was better in primary rain forest followed by rubber based agroforestry and least in monoculture rubber plantations. In another study it was observed that, soil qualities were less affected by the conversion of natural forests into tree plantations than by the conversion of cultivated areas (Zarafshar et al., 2020). A minimum dataset (MDS) of soil indicator as SOC, bulk density, CEC, pH, available potassium, and available phosphorous was developed by Hinge et al. (2019) to determine SQ under various land use and soil management and recorded the highest SQ in dense forest. Based on the MDS values of silt, pH, CEC, exchangeable potassium, and soil organic matter, Mesfin et al. (2023) determined that grassland had higher soil quality in comparison to both natural forest and cultivated land.
The SQIs are developed by different scoring techniques, such as linear and non-linear. A weighted additive SQI using MDS could quantify the adverse impacts of forest conversion to dry farming on SQ (Davari et al., 2020). Dry farming and forest removal lowered the SQ by 44.5%. A non-linear scoring of soil indicators showed a decrease in SQI due to deforestation(Nabiollahi et al., 2018). Sinha et al. (2014) identified the weighted non-linear index as the most sensitive for all agroecosystems and proposed it as a future evaluation index. Research suggests that SQIs are a valuable technique to determine the impact of land degradation and changes in land use on SQ. However, the most robust SQI method suitable to assess the effect of various LUS is still a question to be answered. Therefore, there is a need for further investigation to explore the various SQI indexing techniques in different LUSs. Many studies on SQ indexing focus on short-term effects, but there is limited research on the long-term impacts of land use change on SQ. It is crucial to comprehend the long term viability and sustainability of different LUSs. It is hypothesized that conversion of natural forests to agricultural land will deteriorate SQ. Understanding the significance of SQ, the present study was conducted with the aims (1) to assess the soil physicochemical and biological characteristics across various land-use systems and (2) to develop MDS of soil indicators and compare soil quality indexing approaches to assess SQ under tree-based LUSs.
The study was carried out at the research farm, Gandhi Krishi Vigyana Kendra, University of Agricultural Sciences (UAS), Bengaluru, Karnataka, India (Latitude: 13° 05′ North, Longitude: 77° 34′ East and Altitude: 924 msL). The mean annual rainfall of the study region is 920 mm, with a maximum temperature of 29.6°C and a minimum temperature of 18.2°C. The climate of the study region is semi-arid. As per USDA classification, soils are classified as fine, kaolinitic, isohyperthermic, Typic Kandiustalf. The soil texture varied from sandy clay to sandy clay loam. Eight prevalent land-use systems were considered to study their long-term influence on soil characteristics and SQ.
Among the eight different and distinct LUSs studied, seven were tree-based LUSs viz., natural forest, tree plantations of Tectona grandis, Terminalia bellirica, Swietenia macrophylla, Artocarpus hirsutus, Melia dubia-based agroforestry system (Melia dubia-finger millet), horticulture system (Mangifera indica) and one agriculture based LUS (finger millet) were considered for the study. These land-use systems (LUSs) were studied under similar climatic conditions with the respective crops for more than 30 years, except for the agroforestry system (around 10 years). The experimental setup provides an unique opportunity to understand the long-term influence of LUSs and management on SQ under disturbed and undisturbed conditions. The forest in the study is classified as a dry deciduous (Champion and Seth, 1968). The tree plantations (Tectona grandis, Terminalia bellirica, Swietenia macrophylla, and Artocarpus hirsutus) were established in 1986 with a spacing of 2 m × 2 m. The Melia dubia – finger millet-based agroforestry system was established 2010 at a spacing of 8 m × 5 m, and mango was planted at a spacing of 10 m × 10 m between 1974 and 1977. Agricultural land was under cultivation of finger millet (Eleusine coracana) continuously from 1974 onwards. Area under different land use systems were 1,500, 1,280, 1,600, 640 and 2,000 m2 for tree plantations, agroforestry, mango, finger millet and natural forest, respectively.
Representative soil samples from eight different LUSs were collected from 0 to 0.6 m depth following standard protocols using motorised auger. In each land use system, soil samples were collected from three random locations and in each location, soils were collected from three different depths (0–20 cm, 20–40 cm and 40–60 cm). The average value of the three depths was considered in this study. The representative soil sample collected was divided into two subsets. One subset of the sample was air-dried and passed through a 2 mm sieve. The samples were stored in a moisture-free environment for further analysis. The second subset of the sample was placed in refrigerated conditions and utilized to determine the biological properties of the soil.
The physicochemical and biological properties of soil samples were determined using standard analytical procedures. The soil pH and electrical conductivity (EC) were measured using a pH meter and a conductivity meter in 1:2 soil-to-water ratio (Jackson, 2005). The soil organic carbon (SOC) was estimated using the wet digestion method (Jackson, 1967). The soil available nitrogen (N), phosphorus (P), and potassium (K) were determined by the alkaline potassium permanganate distillation (Subbiah and Asija, 1956), Bray’s method (Bray and Kurtz, 1945) and ammonium acetate extractant method (Jackson, 2005), respectively. Soil available calcium, magnesium, sulfur, iron, zinc, copper, and manganese were determined using inductively coupled plasma optical emission spectrometry (ICP-OES) (Spectra Genesis, Germany manufacture). The Keen Raczkowski cup method was used to determine the pore space and maximum water holding capacity (MWHC) (Piper, 1966). According to the established protocol, soil dehydrogenase activity (DHA) was measured by converting 2, 3, and 5 triphenyl tetrazolium chloride (TTC) to triphenyl formazan (TPF) (Tabatabai, 1994). Acid phosphatase activity (AcidPhos) was measured using the p-nitrophenyl phosphate technique (Tabatabai and Bremner, 1969). Soil microbial biomass carbon (SMBC) was measured by chloroform fumigation and incubation method (Carter, 1991).
SQ is assessed in three steps (Andrews et al., 2002): selecting the minimum data set (MDS) using PCA and correlation, scoring soil indicators, and integrating scores to develop SQI (Figure 1). The variables with significant differences (p < 0.05) between LUSs were selected for PCA and MDS selection. The PCA was performed to analyze the relationship between these indicators using the varimax rotation technique. The PCs that explained at least 5% of the variation in the data and had eigenvalues greater than one were considered for indicator selection (Brejda et al., 2000).
In each PC, indicators with weighted loading values within 10% of the highest weighted loading were chosen to screen the MDS regardless of sign (Rezaei et al., 2006). A multivariate correlation was utilized to eliminate data redundancy when multiple factors were retained within a single PC. Among the highly-correlated variables (>0.60), one with higher values is only considered for MDS. Each highly weighted variable was considered significant for the MDS when they were not correlated.
For the SQI computation each observation of the MDS indicator was normalized. The normalized indicator value is referred as the “indicator score” (Si). Both linear (SL) and non-linear (SNL) scoring functions were employed to compute Si, so that the indicator values ranged between 0 and 1 (Andrews et al., 2002). In both methods, each indicator was categorized as “more is better,” “less is better,” or “optimum is better.” For “optimum is better,” indicator observations were scored as “more is better” up to the threshold level and then scored as “less is better.” Linear normalization (SL) was performed using the maximum (Xmax) and minimum (Xmin) for each soil indicator (X) (Eqs 1 and 2).
Non-linear scores (SNL) were calculated using the average indicator value (X0) (Eq. 3)
Where, b is the slope assumed to be −10.5 for the positive function and +10.5 for negative function (Sinha et al., 2009; Zhang et al., 2011).
After assigning scores to each indicator, the weight was calculated using PCA’s output. To compute the weight factor for each soil indicator in a PC, the percent variation explained by the PC with the highest loading was divided by the total variation explained by all the PCs with an eigenvalue greater than 1 (Bhardwaj et al., 2011; Singh et al. 2014). The SQI is computed by multiplying each indicator’s value by its weight and integrating the result (Eq. 4).
In addition, the following equations were utilised to compute the additive SQI (Eq. 5) (Andrews et al., 2002)
Where, Wi is the indicator’s PCA-based weight, Si is the indicator’s score, and n is the number of indicators. So, in the present study, four SQIs were developed.
The following equation (Eq. 6) (Masto et al., 2008) was used to compute the sensitivity of the SQI for detecting the effects of land use change on SQ
Where, SQImax represents the maximum SQI and SQImin represents the minimum SQIs observed under each indexing procedure.
The statistical analysis of the data pertaining to various soil attributes was conducted using R software version 4.2.2, employing a randomized block design (RBD). Means of the soil properties of different LUSs were compared by using the Tukey HSD procedure (Steel and Torrie, 1960). Pearson’s correlation coefficient determined the strength of SQ properties. The PCA was carried out using Statistical software SPSS 20.0 and the results were further used to develop the MDS by PCA for SQI development. Descriptive statistics and one-way analysis of variance (ANOVA) were applied to the SQI under different land uses. A radar plot presents the percentage contribution of each indicator to the SQI in different LUSs.
The soil pH of natural forest (6.73) and S. macrophylla (6.54) was neutral, whereas that of agriculture and agroforestry soils was acidic (4.93 and 5.03, respectively) (Table 1). The soil pH values of other tree-based systems varied between 5.03 and 6.24. The addition of litter in tree-based systems has influenced the soil pH, which aligns with many previous reports (Tang and Yu, 1999; Marschner and Noble, 2000; Xu et al., 2006; Rukshana et al., 2011). It can also be observed that as the tree-based LUSs age, the soil pH is becoming more neutral, which is evident from the order Agroforestry (5.03) < M. indica (5.83) < Tree plantation (6.06) < Natural forest (6.73). Hong et al. (2018) stated that afforestation reduces pH in relatively alkaline soil and can also increase in relatively acidic soil leading to neutralization over long durations(Hong et al., 2018). This might be due to the creation of an overall balance of the hydrogen ions generated in the soil during the nutrient cycle and various other processes (litter decomposition, microbial enzyme activities, root exudates, etc.) that occur in the soil ecosystem (De Schrijver et al., 2012; Rukshana et al., 2014). Natural forests recorded significantly higher EC levels (0.09 dS m−1) than other LUS (Table 1). This may be caused by the weathering and decomposition of litter, which results in the enrichment of soil minerals by basic salts. Previous studies (Verma et al., 2001; Alam et al., 2018) have reported a higher EC in tree-based systems than in arable land.
The study revealed that the different LUSs significantly affected soil organic carbon (SOC) levels. The SOC was higher under natural forests (0.98%) and lower in agriculture systems (0.36%) (Table 1). The findings are consistent with prior research studies (Pal et al., 2012; Singh and Sharma, 2012; Nanganoa et al., 2019; Tesfahunegn and Gebru, 2020). The tree-based LUSs are associated with increased SOC levels, which can be attributed to the incorporation of varying quantities of litter and roots, and differential rates of organic matter breakdown added by different tree species. Removing crop residues from cultivated land and frequent soil tillage may reduce SOC. Depleting SOC may decrease soil fertility, land degradation, and potential desertification.
Higher N was recorded in the agriculture system, while P and K was highest under agroforestry (Table 1). The increased availability of N, P, and K in managed agricultural system and agroforestry may be attributed to the consistent and frequent additions of synthetic fertilizers, whereas, in natural forest and tree plantations, there were no such additions. Among the unmanaged tree-based systems, the highest N, P, and K were recorded under natural forests, stating that diversity in litter quality also affects the soil nutrient availability compared to monoculture.
Higher Ca and Mg was recorded in natural forest (Table 1). Earlier studies have reported that natural forests have a higher concentration of exchangeable bases (Ca, Mg) in their soil than other LUSs (Muche et al., 2015; Tesfahunegn and Gebru, 2020). The higher Ca and Mg content in tree-based systems can be ascribed to the addition of a larger quantity of litter and addition of substantial amounts of organic matter and nutrients to the soil. Exchangeable bases vary due to leaching losses, a low concentration in the parent rock, and clay mineral content. According to Muche et al. (2015), the continual cultivation and use of inorganic fertilizers resulted in the depletion of exchangeable Ca and Mg.
Higher Zn content was recorded in natural forest (1.34 ppm), followed by S. macrophylla (1.16 ppm), and it was least in agriculture soils (0.18 ppm), which is on par with the horticulture system (0.22 ppm) (Table 1). The highest concentration of iron was recorded in A. hirsutus (18.15 ppm), which was on par with agriculture, S. macrophylla and T. bellirica, while the least was recorded in M.indica (10.49 ppm). Cu concentration was higher in A. hirsutus tree plantations (1.54 ppm), which was on par with T. bellirica, natural forest, T. grandis, agroforestry, and S. macrophylla while the M.indica (0.74 ppm) had the lowest. The highest concentration of Mn was recorded in natural forests (36.34 ppm), lowest was recorded in M.indica (22.28 ppm). The variability in micronutrient content among different tree species can be attributed to variations in concentration, litter decomposition rate, the quantity of litter added, and soil organic matter build-up (Dhaliwal et al., 2019).
Higher DHA was recorded in natural forest (40.44 μg TPF g−1 soil 24 h−1) while agriculture soil (1.61 μg TPF g−1 soil 24 h−1) had the least (Table 1). Higher DHA in the natural forest may be attributable to greater substrate availability and higher SOC levels. Błońska et al. (2017) and Yu et al. (2012) also found higher DHA in natural forest. Additional litter biomass, dead root cells, and rhizosphere secretions may boost carbon, nutrient and higher rhizospheric microbial activity (Uthappa et al., 2015; Woźniak et al., 2022). There was a considerable difference in DHA between the tree-based LUSs. Cultivated soils generally have less organic matter, weaker structure, and fewer microbes.
Agroforestrty exhibited higher AcidPhos activity (142.53 μg PNP g−1 soil h−1), similar to that of agriculture, T. grandis, T.bellirica, and natural forest, and significantly lower phosphatase activity was recorded in M.indica (73.21 μg PNP g−1 soil h−1) which was comparable to S. macrophylla, T.bellirica and A.hirsutus (Table 1). The regulation of phosphorus cycling in soil, especially in soils deficient in phosphorus, is significantly influenced by phosphatase activity (Janes-Bassett et al., 2022). Phosphatase activity is associated with SOM, P, and N availability. The highest AcidPhos activity in agroforestry might have increased organic-P hydrolysis into inorganic-P, increasing the available P (Radersma and Grierson, 2004).
The SMBC was the highest in natural forest (429.38 μg g−1), whereas agriculture system (247.85 μg g−1) and M. indica (263.28 μg g−1) had the least values (Table 1). The difference in SMBC amongst land uses is due to SOC content, management of the LUS, litter quality, and quantity (Lepcha and Devi, 2020). Continuous and higher organic matter deposition via leaf litter resulted in higher SMBC in natural forest. A strong positive association (r = 0.857) was observed between SOC and SMBC. The results are consistent with previously reported studies (Chen et al., 2017; Padalia et al., 2018; Lepcha and Devi, 2020). The imbalanced use of fertilizers and application of fertilizers, particularly N, resulted in a considerable decline in SMBC (Lu et al., 2011; Qiu et al., 2016).
Higher maximum water holding capacity was recorded in natural forest (31.80%), which was on par with S. macrophylla, T. bellirica, T. grandis and agroforestry while the agriculture (23.76%) was least, which was on par with M.indica, T. grandis and A. hirsutus (Table 1). S. macrophylla recorded the highest pore space (42.33%), which was on par with natural forest (41.99%) and T. bellirica (41.15%), and lowest was recorded in agriculture (34.85%) (Table 1). Trees maintain soil structure and minimize soil crust formation by adding organic matter, promoting soil macro and micropores, and retaining moisture. Agricultural soils may have become more compact due to cultivation practices and minimal organic manure additions. The soil pore space is inversely related to soil bulk density. Bizuhoraho et al. (2018) and Zhang et al. (2022) found greater soil porosity in tree-based land use than in cultivated land. Soil organic matter and tree root systems accelerate soil aggregate formation (Jiao et al., 2020) and improve soil structure (Wang et al., 2018).
The soil properties that exhibited significant variation among the LUSs were chosen for PCA. The four PCs with an eigenvalue greater than one were selected, which explained 80.53% of the cumulative variance (Table 2). Varimax rotation was performed to optimally distribute the variance in the selected four PCs. PC1 with an eigenvalue of 5.13, explained a variation of about 30.16%. It included DHA with a positive factor loading of 0.918 and Ca (0.858). The PC2 explained a variation of 22.61% and an eigenvalue of 3.84. It included K (0.884), AcidPhos (0.829), N (0.822), and Mg (0.798) with positive factor loading. The PC3 explained 18.80% of the variation with an eigenvalue of 3.20. In this PC, pore space has the highest factor loading (0.864), followed by MWHC (0.846). The PC4 had the highest factor loading of 0.891, contributed by Fe, with an eigenvalue of 1.52 and a variation of 8.96%.
From each PC, indicators within 10% of the highest factor loading were selected for the MDS (Rezaei et al., 2006). If a PC included more than one soil indicator, the correlation coefficient (r < 0.60) was utilized to determine whether variables were redundant and should be removed (Legaz et al., 2017) (Figure 2). In the first PC, two indicators were within 10% of the highest factor loading (Table 2). From the PC1, the two indicators were DHA and Ca, with a correlation coefficient of 0.71. Since both are important variable and represents two different aspects of soil, i.e., biological and chemical properties (major secondary nutrients), both were considered.
In PC2, K, AcidPhos, N, and Mg were within 10% of the highest factor loading. The K was positively correlated with N (r = 0.71), AcidPhos (r = 0.63), and Mg (0.68) (Figure 2). Since the soils of semi-arid tropics have low fertility, particularly N, it was considered essential and retained from a soil fertility point of view. It was also considered because the study area’s soils were poor in N and it is a limiting nutrient for growth and plant functions. The AcidPhos represents the P availability in the soil, so AcidPhos was also considered from PC2.
In PC3, pore space and MWHC were within 10% of the highest factor loading. This PC focuses on soil physical parameters based on loading factor pattern and size. Pore space and MWHC were highly correlated (r = 0.97) (Figure 2). Therefore, only pore space was selected to represent the PC3 for MDS. In PC4, Fe was selected, representing the soil’s available micronutrients. Overall, the MDS comprised of the indicators chosen as K, N, Ca, DHA, AcidPhos, pore space, and Fe, which represented the soil’s physical, chemical, and biological properties.
In the current study, DHA and AcidPhos were chosen as indicators of MDS among various soil biological properties. Due to their higher sensitivity, soil enzyme activity has often been used in forest soils to evaluate the consequences of land use change (Lucas-Borja et al., 2010; Bini et al., 2013). DHA was considered one of the important SQ indicator (Mandal et al., 2011; Biswas et al., 2017; Klimkowicz-Pawlas et al., 2019). The DHA reflects soil organic matter quality, nutrient availability, microbial activity related to N cycling, and organic compound oxidation (Bünemann et al., 2018), making it an important indicator of SQ (Chaudhury et al., 2005). Phosphatase activity plays a crucial role in the regulation and maintenance of P cycling in the soil, particularly in phosphorus-deficient soils (Janes-Bassett et al., 2022). According to Lemanowicz (2018) phosphatase activity can indicate soil biological and organic phosphorus mineralization capacity. Mahajan et al. (2021) also identified acid phosphatase as one of the MDS in controlling the SQ.
Among the macro nutrients N, K and Ca were selected for MDS. N is an important nutrient for optimal plant growth and development and it has been selected as MDS to evaluate the SQ by Mahajan et al. (2021). It plays an essential role in several vital activities, including growth, the increase of leaf area, and biomass production (Anas et al., 2020). A lack of N can inhibit plant growth and development. Adequate N supply improves above-ground biomass, grain yields, root development (volume, area, diameter, length, mass), nutrient uptake, nutritional balance, and dry mass production (Good et al., 2004; Diaz et al., 2006). K is also frequently used as an SQ indicator in earlier studies (Bünemann et al., 2018; Shi et al., 2021; Maini et al., 2022; Brar et al., 2023). While evaluating SQ under different land use systems Vashisht et al. (2020) and Shao et al. (2020) also identified K as one of the MDS. The K is vital in plants’ osmotic regulations that help regulate moisture stress. Another study concluded that available K and Fe are the most important parameters to assess soil health (Idowu et al., 2008). According to Phillip and Martin, 2003, Ca is a secondary macronutrient essential to plants, and its concentrations in the shoot can range from 0.1 to 5% dry weight. It functions as a secondary messenger and structural component of cell walls and membranes (Phillip and Martin, 2003). Ca contributes to soil fertility through clay flocculation and good aeration (Norton, 2013) and by improving the physical condition of soils. Calcium-rich soils are often more friable and have better water infiltration capabilities. Studies in different tree based systems also identified Ca as MDS while evaluating the SQ (Mulyono et al., 2019; Leul et al., 2023). Tesfahunegn (2014) included Fe as an important SQ indicator in assessing SQ under different LUSs. Through its involvement in the electron transport chain, iron serves as essential for practically all living species since it is involved in many physiological, biochemical, and metabolic activities (Gyana and Sahoo, 2015). Soil porosity is widely recognized as the best indicator of SQ. Characterization of the pore system helps comprehend water and air retention and movement in the soil, evaluate LUS effects, and measure soil deterioration such as compaction and crusting (Pagliai and Vignozzi, 2006). Cardoso et al. (2013) stated that soil porosity is important parameters for assessing SQ status. The other soil properties had low factor loading; thus, these were excluded from the MDS. The “more is better” approach was followed for DHA, AcidPhos, N, K, and Ca. For Fe and pore space “optimum is better” approach was followed.
The SQI was developed by transforming soil properties into scores using linear and non-linear scoring methods, and the weights calculated from PCA are presented in Figure 3. All four SQI: linear additive (SQI-1), linear weighted (SQI-2), non-linear additive (SQI-3), non-linear weighted (SQI-4) were able to identify SQ changes in different LUS, implying that they could be used to monitor SQ. However, a sensitivity test revealed the differences among the SQIs (Figure 4). The SQI-4 had the highest sensitivity to detect SQ changes under different LUSs, while the least sensitive was SQI-1. The order of sensitivity of SQIs tested was SQI-4 > SQI-3 > SQI-2 > SQI-1. Thus, SQI-4 derived through a non-linear weighted method could be successfully used to assess SQ, as it is the most sensitive index for assessing different agroecosystems (Sinha et al., 2014) and represents the system function more accurately than the linear technique (Andrews et al., 2002; Mahajan et al., 2020).
Figure 3. Effect of different land use systems on the soil quality index developed using different indexing method.
Figure 4. Sensitivity values of different soil quality indices developed using different indexing methods.
Radar plots illustrate how MDS soil indicators contributed to SQI in various scoring methodologies (Figure 5). In the linear additive method, all the selected MDS indicators had equal contributions except for DHA. In the linear weighted method, all the parameters had equal contributions except for Fe and DHA. In both the non-linear additive and weighted methods, the contribution of all the parameters was significantly different. It also agrees with the sensitivity analysis (Figure 4), where non-linear scoring methods were more sensitive than linear scoring methods.
Figure 5. Radar diagrams of the contribution of selected indicators of the minimum dataset to soil quality indices developed using different indexing method under different land uses.
The SQI calculated for different land uses using the non-linear scoring weighted method was significantly higher under natural forest (0.973), followed by S. macrophylla (0.756) and agroforestry (0.737). Significantly lowest SQI was recorded in M. indica (0.208). Based on the SQI, the order of the systems studied was natural forest (0.973) > S. macrophylla (0.756) > agroforestry (0.737) > agriculture (0.556) > T. grandis (0.416) > T. bellirica (0.373) > A. hirsutus (0.343) > M. indica (0.208). The natural forest soils are rich in organic matter, harbours rich soil biodiversity (Bardgett and Van Der Putten, 2014; Uthappa, 2021) and have high biotic activity (Osman and Osman, 2013), which has positive effects on soil physicochemical and biological properties as reflected by the highest SQI. Chandel et al. (2018) found that the SQI was the highest in forest ecosystems, followed by grassland, horticulture, cultivated, and bare land. They also stated that the improved SQI in forested areas is due to the higher organic matter content of the soil. According to Tesfahunegn (2014) natural forest areas have a better SQ than uncultivated marginal land systems and forests maintained higher SQI values than agroforestry and agriculture (Mandal et al., 2013). The S. macrophylla based tree plantation and agroforestry system, has emerged as second best LUSs. Practising agroforestry can be viable and sustainable option to maintain soil health (Fahad et al., 2022; Jinger et al., 2023). The monoculture of forest trees, horticulture trees, and agriculture proved to be the third best option compared to natural and agroforestry systems. It is worth mentioning that except in agriculture and agroforestry system, no other systems received fertilizers or soil amendments. The trees (S. macrophylla, A. hirsutus, T. bellirica and T. grandis) were also planted at a very closer spacing (2 × 2 m) which would have led to exhaustion of soil nutrients.
The results of the present investigation revealed that the converting natural forests into different LUSs as tree plantations, horticulture, agroforestry and agriculture deteriorates the SQ. However, increasing population and demographic changes exert tremendous pressure on existing arable land and forest for 5Fs (food, fodder, fuelwood, fibre, fertilizer). Thus, sustainable utilization of natural forests and its conversion into different appropriate LUSs demands scientific approaches to quantify the effects on natural resources, particularly soils. The present study identified a minimum number of soil indicators as MDS and a non-linear weighted SQ indexing approach (SQI-4) as a robust method to detect the changes in the SQ. The method reduces the cost and time of sampling, analysis and enables rapid estimation of SQ. Further, the output of this study could be of immense significance to designing appropriate land management practices for sustainable utilization of converted land uses.
The research findings indicate that the LUS has a substantial long-term impact on the physical, chemical, and biological characteristics of the soil in semi-arid climatic conditions.An SQI comprising of soil indicators viz., DHA, AcidPhos, N, K, Ca, porosity, and Fe was developed to assess SQ changes under different LUSs. Of the four SQI approaches, the non-linear weighted approach was the best to assess SQ. In each of the four SQIs, the natural forest emerged as the best land use system, signifying that it is the most SQ-compliant LUS. The conversion of natural forests into other alternative LUSs decreased the SQ, indicating soil deterioration at varying scales. Further, intensive cultivation and unmanaged tree plantations may reduce SQ. SQ assessment using MDS indicators could also reduce the time and cost under similar agro-climatic situations. Therefore, the approach of the SQI using MDS in the current study could be helpful for proper scientific planning and sustainable utilization of natural resources.
The original contributions presented in the study are included in the article/supplementary material, further inquiries can be directed to the corresponding authors.
AU: Conceptualization, Data curation, Formal analysis, Investigation, Methodology, Visualization, Writing – original draft. AD: Conceptualization, Methodology, Supervision, Writing – original draft. BD: Methodology, Software, Writing – review & editing. GM: Writing – review & editing. SC: Software, Writing – review & editing. DJ: Methodology, Writing – review & editing. PJ: Writing – review & editing. PK: Writing – review & editing. AK: Formal analysis, Investigation, Methodology, Writing – review & editing. RK: Methodology, Supervision, Writing – review & editing. NM: Formal analysis, Investigation, Writing – review & editing. CD: Formal analysis, Investigation, Writing – review & editing. IfA: Visualization, Writing – review & editing. SE: Funding acquisition, Writing – review & editing. IbA: Funding acquisition, Writing – review & editing. ME: Funding acquisition, Writing – review & editing. SF: Visualization, Writing – review & editing.
The author(s) declare financial support was received for the research, authorship, and/or publication of this article. The authors extend their appreciation to Researchers Supporting Project number (RSP2024R298), King Saud University, Riyadh, Saudi Arabia.
AU is thankful for the permission and support of Indian Council of Agricultural Research, New Delhi, India and ICAR-Central Agroforestry Research Institute, Jhansi, Uttar Pradesh, India to conduct the study. AU is also grateful to University of Agricultural Sciences, Bangalore, India for providing permission and facilities to conduct this study. The authors extend their appreciation to Researchers Supporting Project number (RSP2024R298), King Saud University, Riyadh, Saudi Arabia.
The authors declare that the research was conducted in the absence of any commercial or financial relationships that could be construed as a potential conflict of interest.
All claims expressed in this article are solely those of the authors and do not necessarily represent those of their affiliated organizations, or those of the publisher, the editors and the reviewers. Any product that may be evaluated in this article, or claim that may be made by its manufacturer, is not guaranteed or endorsed by the publisher.
Alam, B., Singh, R., Uthappa, A. R., Chaturvedi, M., Singh, A. K., Newaj, R., et al. (2018). Different genotypes of Dalbergia sissoo trees modified microclimate dynamics differently on understory crop cowpea (Vigna unguiculata) as assessed through ecophysiological and spectral traits in agroforestry system. Agric. For. Meteorol. 249, 138–148. doi: 10.1016/j.agrformet.2017.11.031
Anas, M., Liao, F., Verma, K. K., Sarwar, M. A., Mahmood, A., Chen, Z.-L., et al. (2020). Fate of nitrogen in agriculture and environment: agronomic, eco-physiological and molecular approaches to improve nitrogen use efficiency. Biol. Res. 53, 1–20. doi: 10.1186/s40659-020-00312-4
Andrews, S. S., Karlen, D. L., and Mitchell, J. P. (2002). A comparison of soil quality indexing methods for vegetable production systems in northern California. Agric. Ecosyst. Environ. 90, 25–45. doi: 10.1016/S0167-8809(01)00174-8
Bardgett, R. D., and Van Der Putten, W. H. (2014). Belowground biodiversity and ecosystem functioning. Nature 515, 505–511. doi: 10.1038/nature13855
Bhardwaj, A. K., Jasrotia, P., Hamilton, S. K., and Robertson, G. P. (2011). Ecological management of intensively cropped agro-ecosystems improves soil quality with sustained productivity. Agric. Ecosyst. Environ. 140, 419–429. doi: 10.1016/j.agee.2011.01.005
Bini, D., dos Santos, C. A., Carmo, K. B., Kishino, N., Andrade, G., Zangaro, W., et al. (2013). Effects of land use on soil organic carbon and microbial processes associated with soil health in southern Brazil. Eur. J. Soil Biol. 55, 117–123. doi: 10.1016/j.ejsobi.2012.12.010
Biswas, S., Hazra, G. C., Purakayastha, T. J., Saha, N., Mitran, T., Roy, S. S., et al. (2017). Establishment of critical limits of indicators and indices of soil quality in rice-rice cropping systems under different soil orders. Geoderma 292, 34–48. doi: 10.1016/j.geoderma.2017.01.003
Bizuhoraho, T., Kayiranga, A., Manirakiza, N., and Mourad, K. A. (2018). The effect of land use systems on soil properties: a case study from Rwanda. Sustain. Agric. Res. 7:30. doi: 10.5539/sar.v7n2p30
Błońska, E., Lasota, J., and Zwydak, M. (2017). The relationship between soil properties, enzyme activity and land use. For. Res. Pap. 78, 39–44. doi: 10.1515/frp-2017-0004
Brar, S. K., Dhaliwal, S. S., Sharma, V., Sharma, S., and Kaur, M. (2023). Soil quality assessment in diversified long-term experimentation under different agriculturally based cropping systems. J. Soil Sci. Plant Nutr. 23, 1727–1739. doi: 10.1007/s42729-023-01134-5
Bray, R. H., and Kurtz, L. T. (1945). Determination of total, organic, and available forms of phosphorus in soils. Soil Sci. 59, 39–46. doi: 10.1097/00010694-194501000-00006
Brejda, J. J., Moorman, T. B., Karlen, D. L., and Dao, T. H. (2000). Identification of regional soil quality factors and indicators I. Central and southern high plains. Soil Sci. Soc. Am. J. 64, 2115–2124. doi: 10.2136/sssaj2000.6462115x
Bünemann, E. K., Bongiorno, G., Bai, Z., Creamer, R. E., De Deyn, G., de Goede, R., et al. (2018). Soil quality–a critical review. Soil Biol. Biochem. 120, 105–125. doi: 10.1016/j.soilbio.2018.01.030
Cardoso, E. J. B. N., Vasconcellos, R. L. F., Bini, D., Miyauchi, M. Y. H., dos Santos, C. A., Alves, P. R. L., et al. (2013). Soil health: looking for suitable indicators. What should be considered to assess the effects of use and management on soil health? Sci. Agric. 70, 274–289. doi: 10.1590/S0103-90162013000400009
Carter, M. R. (1991). Ninhydrin-reactive N released by the fumigation-extraction method as a measure of microbial biomass under field conditions. Soil Biol. Biochem. 23, 139–143. doi: 10.1016/0038-0717(91)90126-5
Champion, H. G., and Seth, S. K. (1968). A revised survey of the forest types of India. Delhi: Manager Publications.
Chandel, S., Hadda, M. S., and Mahal, A. K. (2018). Soil quality assessment through minimum data set under different land uses of submontane Punjab. Commun. Soil Sci. Plant Anal. 49, 658–674. doi: 10.1080/00103624.2018.1425424
Chaudhury, J., Mandal, U. K., Sharma, K. L., Ghosh, H., and Mandal, B. (2005). Assessing soil quality under long-term rice-based cropping system. Commun. Soil Sci. Plant Anal. 36, 1141–1161. doi: 10.1081/CSS-200056885
Chemeda, M., Kibret, K., and Fite, T. (2017). Influence of different land use types and soil depths on selected soil properties related to soil fertility in Warandhab area, Horo Guduru Wallaga zone, Oromiya, Ethiopia. Int. J. Environ. Sci. Nat. Resour. 4:555634. doi: 10.19080/IJESNR.2017.04.555634
Chen, C., Liu, W., Jiang, X., and Wu, J. (2017). Effects of rubber-based agroforestry systems on soil aggregation and associated soil organic carbon: implications for land use. Geoderma 299, 13–24. doi: 10.1016/j.geoderma.2017.03.021
Davari, M., Gholami, L., Nabiollahi, K., Homaee, M., and Jafari, H. J. (2020). Deforestation and cultivation of sparse forest impacts on soil quality (case study: West Iran, Baneh). Soil Tillage Res. 198:104504. doi: 10.1016/j.still.2019.104504
De Schrijver, A., De Frenne, P., Staelens, J., Verstraeten, G., Muys, B., Vesterdal, L., et al. (2012). Tree species traits cause divergence in soil acidification during four decades of postagricultural forest development. Glob. Chang. Biol. 18, 1127–1140. doi: 10.1111/j.1365-2486.2011.02572.x
Dhaliwal, S. S., Naresh, R. K., Mandal, A., Singh, R., and Dhaliwal, M. K. (2019). Dynamics and transformations of micronutrients in agricultural soils as influenced by organic matter build-up: a review. Environ. Sustain. Indic. 1-2:100007. doi: 10.1016/j.indic.2019.100007
Diaz, C., Saliba-Colombani, V., Loudet, O., Belluomo, P., Moreau, L., Daniel-Vedele, F., et al. (2006). Leaf yellowing and anthocyanin accumulation are two genetically independent strategies in response to nitrogen limitation in Arabidopsis thaliana. Plant Cell Physiol. 47, 74–83. doi: 10.1093/pcp/pci225
Doran, J. W., and Parkin, T. B. (1994). “Defining and assessing soil quality” in Defining soil quality for a sustainable environment. eds. J. W. Doran, D. C. Coleman, D. F. Bezdicek, and B. A. Stewart (Madison, WI: SSSA), 3–21.
Fahad, S., Chavan, S. B., Chichaghare, A. R., Uthappa, A. R., Kumar, M., Kakade, V., et al. (2022). Agroforestry systems for soil health improvement and maintenance. Sustainability 14:14877. doi: 10.3390/su142214877
Ghaemi, M., Astaraei, A. R., Emami, H., Nassiri Mahalati, M., and Sanaeinejad, S. H. (2014). Determining soil indicators for soil sustainability assessment using principal component analysis of Astan Quds-east of Mashhad-Iran. J. Soil Sci. Plant Nutr. 14, 1005–1020. doi: 10.4067/S0718-95162014005000077
Good, A. G., Shrawat, A. K., and Muench, D. G. (2004). Can less yield more? Is reducing nutrient input into the environment compatible with maintaining crop production? Trends Plant Sci. 9, 597–605. doi: 10.1016/j.tplants.2004.10.008
Gyana, R. R., and Sahoo, S. (2015). Role of iron in plant growth and metabolism. Rev. Agric. Sci. 3, 1–24. doi: 10.7831/ras.3.1
Hinge, G., Surampalli, R. Y., and Goyal, M. K. (2019). Effects of land use and soil management on soil quality in India’s northeastern Himalayas. J. Environ. Eng. 145:4019007. doi: 10.1061/(ASCE)EE.1943-7870.0001507
Hong, S., Piao, S., Chen, A., Liu, Y., Liu, L., Peng, S., et al. (2018). Afforestation neutralizes soil pH. Nat. Commun. 9, 1–7. doi: 10.1038/s41467-018-02970-1
Idowu, O. J., Van Es, H. M., Abawi, G. S., Wolfe, D. W., Ball, J. I., Gugino, B. K., et al. (2008). Farmer-oriented assessment of soil quality using field, laboratory, and VNIR spectroscopy methods. Plant Soil 307, 243–253. doi: 10.1007/s11104-007-9521-0
Jackson, M. L. (2005). Soil chemical analysis: advanced course. Madison, WI: UW-Madison Libraries Parallel Press.
Janes-Bassett, V., Blackwell, M. S. A., Blair, G., Davies, J., Haygarth, P. M., Mezeli, M. M., et al. (2022). A meta-analysis of phosphatase activity in agricultural settings in response to phosphorus deficiency. Soil Biol. Biochem. 165:108537. doi: 10.1016/j.soilbio.2021.108537
Jiao, S., Li, J., Li, Y., Xu, Z., Kong, B., Li, Y., et al. (2020). Variation of soil organic carbon and physical properties in relation to land uses in the Yellow River Delta, China. Sci. Rep. 10, 1–12. doi: 10.1038/s41598-020-77303-8
Jien, S.-H., and Wang, C.-S. (2013). Effects of biochar on soil properties and erosion potential in a highly weathered soil. Catena 110, 225–233. doi: 10.1016/j.catena.2013.06.021
Jinger, D., Kaushal, R., Kumar, R., Paramesh, V., Verma, A., Shukla, M., et al. (2023). Degraded land rehabilitation through agroforestry in India: achievements, current understanding, and future prospectives. Front. Ecol. Evol. 11:1088796. doi: 10.3389/fevo.2023.1088796
Karlen, D. L., Parkin, T. B., and Eash, N. S. (1997). “Use of soil quality indicators to evaluate conservation reserve program sites in Iowa” in Methods for assessing soil quality. eds. J. Doran and A. J. Jones (Madison, WI: SSSA), 345–355.
Karlen, D. L., and Stott, D. E. (1994). “A framework for evaluating physical and chemical indicators of soil quality” in Defining soil quality for a sustainable environment. eds. J. W. Doran, D. C. Coleman, D. F. Bezdicek, and B. A. Stewart (Madison, WI: SSSA), 53–72.
Klimkowicz-Pawlas, A., Ukalska-Jaruga, A., and Smreczak, B. (2019). Soil quality index for agricultural areas under different levels of anthropopressure. Int. Agrophys. 33, 455–462. doi: 10.31545/intagr/113349
Legaz, B. V., De Souza, D. M., Teixeira, R. F. M., Antón, A., Putman, B., and Sala, S. (2017). Soil quality, properties, and functions in life cycle assessment: an evaluation of models. J. Clean. Prod. 140, 502–515. doi: 10.1016/j.jclepro.2016.05.077
Lemanowicz, J. (2018). Dynamics of phosphorus content and the activity of phosphatase in forest soil in the sustained nitrogen compounds emissions zone. Environ. Sci. Pollut. Res. 25, 33773–33782. doi: 10.1007/s11356-018-3348-5
Lepcha, N. T., and Devi, N. B. (2020). Effect of land use, season, and soil depth on soil microbial biomass carbon of eastern Himalayas. Ecol. Process. 9, 1–14. doi: 10.1186/s13717-020-00269-y
Leul, Y., Assen, M., Damene, S., and Legass, A. (2023). Effects of land use types on soil quality dynamics in a tropical sub-humid ecosystem, western Ethiopia. Ecol. Indic. 147:110024. doi: 10.1016/j.ecolind.2023.110024
Li, H., Liao, X., Zhu, H., Wei, X., and Shao, M. (2019). Soil physical and hydraulic properties under different land uses in the black soil region of Northeast China. Can. J. Soil Sci. 99, 406–419. doi: 10.1139/cjss-2019-0039
Liu, D., Huang, Y., An, S., Sun, H., Bhople, P., and Chen, Z. (2018). Soil physicochemical and microbial characteristics of contrasting land-use types along soil depth gradients. Catena 162, 345–353. doi: 10.1016/j.catena.2017.10.028
Lu, M., Yang, Y., Luo, Y., Fang, C., Zhou, X., Chen, J., et al. (2011). Responses of ecosystem nitrogen cycle to nitrogen addition: a meta-analysis. New Phytol. 189, 1040–1050. doi: 10.1111/j.1469-8137.2010.03563.x
Lucas-Borja, M. E., Bastida, F., Nicolás, C., Moreno, J. L., del Cerro, A., and Andrés, M. (2010). Influence of forest cover and herbaceous vegetation on the microbiological and biochemical properties of soil under Mediterranean humid climate. Eur. J. Soil Biol. 46, 273–279. doi: 10.1016/j.ejsobi.2010.05.003
Mahajan, G. R., Das, B., Manivannan, S., Manjunath, B. L., Verma, R. R., Desai, S., et al. (2021). Soil and water conservation measures improve soil carbon sequestration and soil quality under cashews. Int. J. Sediment Res. 36, 190–206. doi: 10.1016/j.ijsrc.2020.07.009
Mahajan, G., Das, B., Morajkar, S., Desai, A., Murgaokar, D., Kulkarni, R., et al. (2020). Soil quality assessment of coastal salt-affected acid soils of India. Environ. Sci. Pollut. Res. 27, 26221–26238. doi: 10.1007/s11356-020-09010-w
Maini, A., Sharma, V., Sharma, S., Arora, R., and Dhaliwal, S. S. (2022). Soil biochemical properties of various land use under rainfed conditions in Shiwalik foothills of Punjab, India. Arch. Agron. Soil Sci. 68, 688–703. doi: 10.1080/03650340.2020.1851683
Mandal, D., Dhyani, B. L., Kumar, A., Singh, C., Bihari, B., Muruganandam, M., et al. (2013). Impact of different land use systems on soil quality in northwest Himalayan region. Indian J. Soil Conserv. 41, 200–205.
Mandal, U. K., Ramachandran, K., Sharma, K. L., Satyam, B., Venkanna, K., Udaya Bhanu, M., et al. (2011). Assessing soil quality in a semiarid tropical watershed using a geographic information system. Soil Sci. Soc. Am. J. 75, 1144–1160. doi: 10.2136/sssaj2009.0361
Marschner, B., and Noble, A. D. (2000). Chemical and biological processes leading to the neutralisation of acidity in soil incubated with litter materials. Soil Biol. Biochem. 32, 805–813. doi: 10.1016/S0038-0717(99)00209-6
Masto, R. E., Chhonkar, P. K., Singh, D., and Patra, A. K. (2008). Alternative soil quality indices for evaluating the effect of intensive cropping, fertilisation and manuring for 31 years in the semi-arid soils of India. Environ. Monit. Assess. 136, 419–435. doi: 10.1007/s10661-007-9697-z
Mesfin, D., Assefa, E., and Simane, B. (2023). Soil quality index under different land-use types: the case of Choke Mountain agroecosystems, upper Blue Nile Basin, Ethiopia. Can. J. Soil Sci. 103, 285–296. doi: 10.1139/cjss-2022-0053
Muche, M., Kokeb, A., and Molla, E. (2015). Assessing the physicochemical properties of soil under different land use types. J. Environ. Anal. Toxicol. 5:1. doi: 10.4172/2161-0525.1000309
Mulyono, A., Suriadikusumah, A., Harriyanto, R., and Djuwansah, M. R. (2019). Soil quality under agroforestry trees pattern in upper Citarum watershed, Indonesia. J. Ecol. Eng. 20, 203–213. doi: 10.12911/22998993/93942
Nabiollahi, K., Golmohamadi, F., Taghizadeh-Mehrjardi, R., Kerry, R., and Davari, M. (2018). Assessing the effects of slope gradient and land use change on soil quality degradation through digital mapping of soil quality indices and soil loss rate. Geoderma 318, 16–28. doi: 10.1016/j.geoderma.2017.12.024
Nanganoa, L. T., Okolle, J. N., Missi, V., Tueche, J. R., Levai, L. D., and Njukeng, J. N. (2019). Impact of different land-use systems on soil physicochemical properties and macrofauna abundance in the humid tropics of Cameroon. Appl. Environ. Soil Sci. 2019, 1–9. doi: 10.1155/2019/5701278
Norton, R. (2013) Focus on calcium: its role in crop production. GRDC Updates Pap. Available at: https://grdc.com.au/resources-and-publications/grdc-update-papers/tab-content/grdc-updatepapers/2013/02/focus-on-calcium-its-role-in-crop-production (Accessed September 25, 2020).
Padalia, K., Bargali, S. S., Bargali, K., and Khulbe, K. (2018). Microbial biomass carbon and nitrogen in relation to cropping systems in central Himalaya, India. Curr. Sci. 115, 1741–1750. doi: 10.18520/cs/v115/i9/1741-1750
Pagliai, M., and Vignozzi, N. (2006). Soil porosity as an indicator of soil health. Ann. Arid Zone 45:259.
Pal, S., Panwar, P., and Bhardwaj, D. R. (2012). Soil quality under forest compared to other landuses in acid soil of North Western Himalaya, India. Ann. For. Res. 56, 187–198.
Phillip, J. W., and Martin, R. B. (2003). Calcium in plants. Ann. Bot. 92, 487–511. doi: 10.1093/aob/mcg164
Qi, Y., Darilek, J. L., Huang, B., Zhao, Y., Sun, W., and Gu, Z. (2009). Evaluating soil quality indices in an agricultural region of Jiangsu Province, China. Geoderma 149, 325–334. doi: 10.1016/j.geoderma.2008.12.015
Qiu, S., Gao, H., Zhu, P., Hou, Y., Zhao, S., Rong, X., et al. (2016). Changes in soil carbon and nitrogen pools in a Mollisol after long-term fallow or application of chemical fertilizers, straw or manures. Soil Tillage Res. 163, 255–265. doi: 10.1016/j.still.2016.07.002
Radersma, S., and Grierson, P. F. (2004). Phosphorus mobilization in agroforestry: organic anions, phosphatase activity and phosphorus fractions in the rhizosphere. Plant Soil 259, 209–219. doi: 10.1023/B:PLSO.0000020970.40167.40
Raiesi, F. (2017). A minimum data set and soil quality index to quantify the effect of land use conversion on soil quality and degradation in native rangelands of upland arid and semiarid regions. Ecol. Indic. 75, 307–320. doi: 10.1016/j.ecolind.2016.12.049
Rashkow, E. D. (2014). Perfumed the axe that laid it low: the endangerment of sandalwood in southern India. Indian Econ. Soc. Hist. Rev. 51, 41–70. doi: 10.1177/0019464613515553
Rezaei, S. A., Gilkes, R. J., and Andrews, S. S. (2006). A minimum data set for assessing soil quality in rangelands. Geoderma 136, 229–234. doi: 10.1016/j.geoderma.2006.03.021
Rukshana, F., Butterly, C. R., Baldock, J. A., and Tang, C. (2011). Model organic compounds differ in their effects on pH changes of two soils differing in initial pH. Biol. Fertil. Soils 47, 51–62. doi: 10.1007/s00374-010-0498-0
Rukshana, F., Butterly, C. R., Xu, J.-M., Baldock, J. A., and Tang, C. (2014). Organic anion-to-acid ratio influences pH change of soils differing in initial pH. J. Soils Sediments 14, 407–414. doi: 10.1007/s11368-013-0682-6
Shao, G., Ai, J., Sun, Q., Hou, L., and Dong, Y. (2020). Soil quality assessment under different forest types in the mount tai, central eastern China. Ecol. Indic. 115:106439. doi: 10.1016/j.ecolind.2020.106439
Shi, Z., Bai, Z., Guo, D., and Chen, M. (2021). Develop a soil quality index to study the results of black locust on soil quality below different allocation patterns. Land 10:785. doi: 10.3390/land10080785
Singh, B., and Sharma, K. N. (2012). Depthwise distribution of soil organic carbon and nutrients under some tree species after seventeen years of plantation. J. Indian Soc. Soil Sci. 60, 198–203.
Singh, A. K., Bordoloi, L. J., Kumar, M., Hazarika, S., and Parmar, B. (2014). Land use impact on soil quality in eastern Himalayan region of India. Environ. Monit. Assess. 186:2013–2024. doi: 10.1007/s10661-013-3514-7
Sinha, N. K., Chopra, U. K., and Singh, A. K. (2014). Cropping system effects on soil quality for three agro-ecosystems in India. Exp. Agric. 50, 321–342. doi: 10.1017/S001447971300046X
Sinha, S., Masto, R. E., Ram, L. C., Selvi, V. A., Srivastava, N. K., Tripathi, R. C., et al. (2009). Rhizosphere soil microbial index of tree species in a coal mining ecosystem. Soil Biol. Biochem. 41, 1824–1832. doi: 10.1016/j.soilbio.2008.11.022
Steel, R. G. D., and Torrie, J. H. (1960). Principles and procedures of statistics. McGraw-Hill Book Company, New York.
Subbiah, B. V., and Asija, G. L. (1956). A rapid method for the estimation of nitrogen in soil. Curr. Sci. 26, 259–260.
Tabatabai, M. A. (1994). “Soil enzymes” in Methods of soil analysis: part 2 microbiological and biochemical properties. eds. R. W. Weaver, J. S. Angle, and P. S. Botttomley (Madison, WI: The American Society of Agronomy), 775–833.
Tabatabai, M. A., and Bremner, J. M. (1969). Use of p-nitrophenyl phosphate for assay of soil phosphatase activity. Soil Biol. Biochem. 1, 301–307. doi: 10.1016/0038-0717(69)90012-1
Tang, C., and Yu, Q. (1999). Impact of chemical composition of legume residues and initial soil pH on pH change of a soil after residue incorporation. Plant Soil 215, 29–38. doi: 10.1023/A:1004704018912
Tauqeer, H. M., Turan, V., Farhad, M., and Iqbal, M. (2022). “Sustainable agriculture and plant production by virtue of biochar in the era of climate change” in Managing plant production under changing environment. eds. M. Hasanuzzaman, G. J. Ahammed, and K. Nahar (Singapore: Springer), 21–42.
Tesfahunegn, G. B. (2014). Soil quality assessment strategies for evaluating soil degradation in northern Ethiopia. Appl. Environ. Soil Sci. 2014, 1–14. doi: 10.1155/2014/646502
Tesfahunegn, G. B., and Gebru, T. A. (2020). Variation in soil properties under different cropping and other land-use systems in dura catchment, Northern Ethiopia. PloS One 15:e0222476. doi: 10.1371/journal.pone.0222476
Trivedi, P., Delgado-Baquerizo, M., Anderson, I. C., and Singh, B. K. (2016). Response of soil properties and microbial communities to agriculture: implications for primary productivity and soil health indicators. Front. Plant Sci. 7:990. doi: 10.3389/fpls.2016.00990
Uthappa, A. R. (2021). Assessment of ecosystem services under different land use Systems in Semiarid Conditions. University of Agricultural Sciences, GKVK.
Uthappa, A. R., Bana, O. P. S., Kumar, M., and Kanwal, M. (2015). Soil physico-bio-chemical properties as influenced by varying tree densities in poplar (Populus deltoides Bartr. Ex marsh.) based agroforestry system. Indian J. Agrofor. 17, 81–90.
Vashisht, B. B., Maharjan, B., Sharma, S., and Kaur, S. (2020). Soil quality and its potential indicators under different land use systems in the Shivaliks of Indian Punjab. Sustainability 12:3490. doi: 10.3390/su12083490
Verma, R. K., Shadangi, D. K., and Totey, N. G. (2001). Analysis of biodiversity and improvement in soil quality under plantations on degraded land. Indian J. For. 24, 21–28.
Wang, J., Qin, Q., and Bai, Z. (2018). Characterizing the effects of opencast coal-mining and land reclamation on soil macropore distribution characteristics using 3D CT scanning. Catena 171, 212–221. doi: 10.1016/j.catena.2018.07.022
Woźniak, M., Gałązka, A., Siebielec, G., and Frąc, M. (2022). Can the biological activity of abandoned soils be changed by the growth of Paulownia elongata× Paulownia fortunei?—preliminary study on a young tree plantation. Agriculture 12:128. doi: 10.3390/agriculture12020128
Xu, J. M., Tang, C., and Chen, Z. L. (2006). Chemical composition controls residue decomposition in soils differing in initial pH. Soil Biol. Biochem. 38, 544–552. doi: 10.1016/j.soilbio.2005.06.006
Yu, Y., Shen, W., Yin, Y., Zhang, J., Cai, Z., and Zhong, W. (2012). Response of soil microbial diversity to land-use conversion of natural forests to plantations in a subtropical mountainous area of southern China. Soil Sci. Plant Nutr. 58, 450–461. doi: 10.1080/00380768.2012.708645
Zarafshar, M., Bazot, S., Matinizadeh, M., Bordbar, S. K., Rousta, M. J., Kooch, Y., et al. (2020). Do tree plantations or cultivated fields have the same ability to maintain soil quality as natural forests? Appl. Soil Ecol. 151:103536. doi: 10.1016/j.apsoil.2020.103536
Zhang, Y., Wang, L., Jiang, J., Zhang, J., Zhang, Z., and Zhang, M. (2022). Application of soil quality index to determine the effects of different vegetation types on soil quality in the Yellow River Delta wetland. Ecol. Indic. 141:109116. doi: 10.1016/j.ecolind.2022.109116
Zhang, C., Xue, S., Liu, G.-B., and Song, Z.-L. (2011). A comparison of soil qualities of different revegetation types in the loess plateau, China. Plant Soil 347, 163–178. doi: 10.1007/s11104-011-0836-5
Keywords: tree-based land-use systems, soil degradation, soil indicators, soil quality index, soil health
Citation: Uthappa AR, Devakumar AS, Das B, Mahajan GR, Chavan SB, Jinger D, Jha PK, Kumar P, Kokila A, Krishnamurthy R, Mounesh NV, Dhanush C, Ali I, Eldin SM, Al-Ashkar I, Elshikh MS and Fahad S (2024) Comparative analysis of soil quality indexing techniques for various tree based land use systems in semi-arid India. Front. For. Glob. Change. 6:1322660. doi: 10.3389/ffgc.2023.1322660
Received: 16 October 2023; Accepted: 06 December 2023;
Published: 11 January 2024.
Edited by:
Avijit Ghosh, Indian Grassland and Fodder Research Institute (ICAR), IndiaReviewed by:
Gopal Shukla, Uttar Banga Krishi Viswavidyalaya, IndiaCopyright © 2024 Uthappa, Devakumar, Das, Mahajan, Chavan, Jinger, Jha, Kumar, Kokila, Krishnamurthy, Mounesh, Dhanush, Ali, Eldin, Al-Ashkar, Elshikh and Fahad. This is an open-access article distributed under the terms of the Creative Commons Attribution License (CC BY). The use, distribution or reproduction in other forums is permitted, provided the original author(s) and the copyright owner(s) are credited and that the original publication in this journal is cited, in accordance with accepted academic practice. No use, distribution or reproduction is permitted which does not comply with these terms.
*Correspondence: A. R. Uthappa, YXJ1dGhhcHBhQGdtYWlsLmNvbQ==; Shah Fahad, c2hhaGZhaGFkQGF3a3VtLmVkdS5waw==
Disclaimer: All claims expressed in this article are solely those of the authors and do not necessarily represent those of their affiliated organizations, or those of the publisher, the editors and the reviewers. Any product that may be evaluated in this article or claim that may be made by its manufacturer is not guaranteed or endorsed by the publisher.
Research integrity at Frontiers
Learn more about the work of our research integrity team to safeguard the quality of each article we publish.