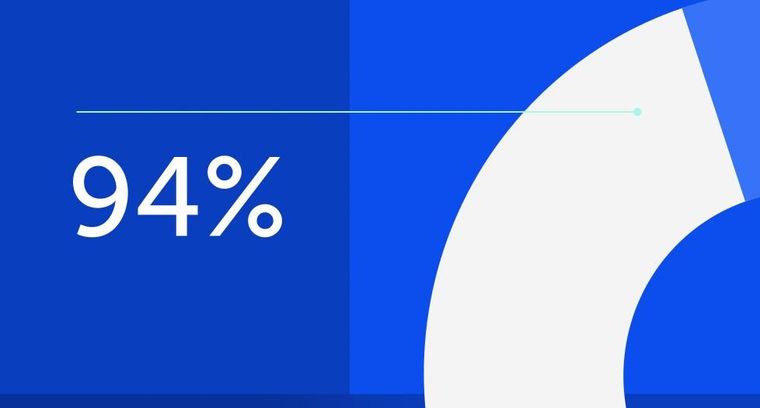
94% of researchers rate our articles as excellent or good
Learn more about the work of our research integrity team to safeguard the quality of each article we publish.
Find out more
ORIGINAL RESEARCH article
Front. For. Glob. Change, 04 December 2023
Sec. Forest Disturbance
Volume 6 - 2023 | https://doi.org/10.3389/ffgc.2023.1294552
This article is part of the Research TopicRemote Sensing of Environmental Changes in the Neotropical RegionView all 8 articles
The Brazilian Amazon land cover changes rapidly due to anthropogenic and climate drivers. Deforestation and forest disturbances associated with logging and fires, combined with extreme droughts, warmer air, and surface temperatures, have led to high tree mortality and harmful net carbon emissions in this region. Regional attempts to characterize land cover dynamics in this region focused on one or two anthropogenic drivers (i.e., deforestation and forest degradation). Land cover studies have also used a limited temporal scale (i.e., 10–15 years), focusing mainly on global and country-scale forest change. In this study, we propose a novel approach to characterize and measure land cover dynamics in the Amazon biome. First, we defined 10 fundamental land cover classes: forest, flooded forest, shrubland, natural grassland, pastureland, cropland, outcrop, bare and impervious, wetland, and water. Second, we mapped the land cover based on the compositional abundance of Landsat sub-pixel information that makes up these land cover classes: green vegetation (GV), non-photosynthetic vegetation, soil, and shade. Third, we processed all Landsat scenes with <50% cloud cover. Then, we applied a step-wise random forest machine learning algorithm and empirical decision rules to classify intra-annual and annual land cover classes between 1985 and 2022. Finally, we estimated the yearly land cover changes in forested and non-forested ecosystems and characterized the major change drivers. In 2022, forest covered 78.6% (331.9 Mha) of the Amazon biome, with 1.4% of secondary regrowth in more than 5 years. Total herbaceous covered 15.6% of the area, with the majority of pastureland (13.5%) and the remaining natural grassland. Water was the third largest land cover class with 2.4%, followed by cropland (1.2%) and shrubland (0.4%), with 89% overall accuracy. Most of the forest changes were driven by pasture and cropland conversion, and there are signs that climate change is the primary driver of the loss of aquatic ecosystems. Existing carbon emission models disregard the types of land cover changes presented in the studies. The twenty first century requires a more encompassing and integrated approach to monitoring anthropogenic and climate changes in the Amazon biome for better mitigation, adaptation, and conservation policies.
The primary interface with the atmosphere is the land cover, composed of plants, topsoil, rock, water layers, and built-up infrastructure. It is also where people and a wide variety of species live. Mapping and monitoring land cover dynamics are essential to characterizing biological and geophysical attributes for hydrological and geochemical modeling of energy balance and carbon exchange with soil and atmosphere (DeFries et al., 1999; Claussen et al., 2001; Roberts et al., 2003; Jones, 2008; De Sousa-Neto et al., 2017). It is also essential for sustaining biodiversity and the growing global socio-economic demands (Krausmann et al., 2013).
While several global land cover mappings (GLCMs) exist, these maps can rarely be directly applied to regional studies of land cover dynamics, given their high level of uncertainty (Congalton et al., 2014; Pendrill and Martin Persson, 2017; Galiatsatos et al., 2020), and thematic classification infrequently satisfies regional users (Grekousis et al., 2015). The existing GLCMs do not provide land cover change estimates such as deforestation and vegetation regrowth to support policy and decision-making for conservation and planning. Anthropogenic and climatic change are the main factors influencing the land cover dynamic in the Amazon biome (Marengo et al., 2018), and the GLCMs do not provide adequate accuracy and consistency to assess these factors (Nemani and Running, 1995).
Satellite imagery is vital to mapping and characterizing land cover dynamics. The 50-year Landsat data archive (Wulder et al., 2022) and the new cloud computing capability, notably by Earth Engine (Gorelick et al., 2017), have driven the proliferation of medium spatial resolution (i.e., 30 m pixel size) land cover maps at global, continental, and regional scales. The most common approach to mapping land cover using Earth Engine is based on the pixel spectral characterization of the land attributes from the spectral bands and spectral and textural indices with machine learning classification algorithms, mainly random forest, according to a systematic literature review (Pérez-Cutillas et al., 2023). Object-based classification has also brought attention to remote sensing map makers (Ma et al., 2017). With the novelty of cloud computing, time-series analysis (Friedl et al., 2022), and deep learning (Brown et al., 2022), image classification algorithms for handling large data sets are emerging. The MapBiomas initiative focuses on mapping at the biome scale to deal with their intrinsic biophysical and climatological characteristics. MapBiomas uses annual synthetic spectral bands and indices to obtain less cloudy (i.e., < 5.0%) Landsat mosaics to map annual land cover and land use (Souza et al., 2020), an approach replicated in several countries.
Several studies have also demonstrated that the compositional information within the Landsat pixel is valuable in characterizing land cover classes (Roberts et al., 1993; Adams et al., 1995; Asner, 2009). The pixel composition can be estimated from the multispectral bands of Landsat sensors in terms of the abundance of green vegetation (GV), non-photosynthetic vegetation (NPV), soil, and shade, which make up the land cover classes in the Amazon biome (Souza et al., 2005). For example, grasslands are composed of a mixture of GV, NPV, and soil uniquely different from dense forests (with high shade and GV and no soil) (Asner and Heidebrecht, 2002). Sub-pixel information is also essential to detect and characterize disturbances leading to ecosystem degradation (Veraverbeke and Hook, 2013; Salih et al., 2017). Therefore, pixel compositional information can potentially separate ambiguous land cover classes (e.g., pasture, bare soil, rock, and impervious surface), improving land cover mapping and characterizing ongoing climate change in land cover interactions (Song et al., 2018).
Most studies in the Amazon biome have focused on deforestation and drivers of forest degradation to characterize land cover dynamics (e.g., Souza et al., 2013; Bullock et al., 2020b; Potapov et al., 2020) or cover a few Landsat scenes and a short temporal scale (Roberts et al., 2002). Another essential demand is using land cover maps to estimate changes, such as deforestation, vegetation regrowth (Nunes et al., 2020), and water dynamics (Souza et al., 2019), directly from the land cover map series.
The objectives of this study are 4-fold: i) improve the detection and mapping of the main land cover classes of the Amazon biome using Landsat sub-pixel compositional information; (ii) characterize the annual land cover change in forest, non-forested vegetation, and aquatic ecosystems; (iii) assess how the land cover change drivers varied between 1985 and 2022; and (iv) estimate annual loss and gain among the land cover types during this period. This study is organized as follows: Section 2 presents the methods applied to process the Landsat time series to map and characterize land change. Section 3 presents the results, focusing on the annual land cover change and land change drivers. Then, we discuss the mapping uncertainty and summarize the findings of future research steps.
The Amazon is the world's largest tropical rainforest, spanning the borders of eight South American nations. Its environmental functions are crucial for preserving the equilibrium of the world's climate, its vast biodiversity, and the homeplace of the original Amazonian people. Our study area, the Brazilian Amazon Biome (Figure 1), comprises more than 421 million hectares, or 50% of the Pan-Amazon extension. The Amazon biome occupies 49.5% of the nation compared to Brazilian land.
Figure 1. Brazilian Amazon and Landsat scenes of Collection 1 during 1985 and 2022 with <50% of cloud cover.
We selected all Landsat images from Collection 1 Tier 1 in the Google Earth Engine (Gorelick et al., 2017) catalog with < 50% cloud cover. More than 86k Landsat scenes were used to build the Amazon annual mapping between 1985 and 2022. We assessed the number of Landsat scenes available per year and identified three distinct periods of imagery availability (Figure 2). The first was from 1985 to 1998 when the Landsat 5 TM sensor was the only operational. In that period, we found almost 15k available images, with an average of 1,070 per year. The second period was between 1999 and 2011, when Landsat 5 and 7 operated simultaneously, duplicating the total number of scenes compared with the previous period. In the second period, we selected 34k Landsat images (an average of 2,638 per year). In 2012, we observed a drop in image availability because Landsat 5 stopped acquiring data, with only Landsat 7 operational, reducing the number of scenes to 1,753 images this year.
The last period of Landsat scene availability was between 2013 and 2021. We had Landsat 7, 8, and 9 available in this period, raising the number of images per year relative to the previous periods, reaching an average of 3,358 Landsat scenes per year. For 2021, we used the Landsat 9 Collection 2 Tier 1 (n = 67 scenes) because Collection 1 data was unavailable. Including Landsat Collection 2 in the Landsat time series does not affect the annual classification results because the scenes are processed individually. In 2022, we reached almost 5k Landsat scenes (Figure 2), which may inaugurate a new period of image availability for Landsat 9.
We also assessed how the number of Landsat scenes varies across the study area (Figures 1, 2). The southern region showed 400–600 Landsat scenes over the entire period (i.e., 1985–2021; Figure 1), whereas the northern Atlantic region had fewer scenes available (<100). The central area had approximately 300 scenes, and the western and central northern areas had approximately 200. The number of scenes may affect the classification results, which are further investigated in this study.
Defining a classification scheme remains challenging for remote sensing of terrestrial land cover mapping (Yang et al., 2017). Land use is related to how people interact with land surface types (i.e., classes). In contrast, land cover relates to the properties (biophysical and chemical) of the Earth's surface (Lambin et al., 2001). We selected the most relevant land cover classes in our mapping efforts to measure change in the Brazilian Amazon, including forest, flooded forest, shrubland, natural grassland, pastureland, cropland, outcrop, bareland and impervious, wetland, and water (Table 1). We decided to include pastureland and cropland, which are associated with land use, because their extent is significant in the Brazilian Amazon, and both have distinct spectral, compositional, and phenological characteristics from natural grassland and are the main drivers of change in the region. Detailed descriptions of the land cover classes are presented in Table 1.
Our approach to mapping and characterizing land cover dynamics in the Brazilian Amazon Biome with Landsat time series follows five steps: (i) data preprocessing; (ii) spectral mixture analysis (SMA); (iii) land cover classification; (iv) post-classification; and (v) accuracy assessment (Figure 3). We present below the detailed methodology for each of these steps.
i) Data preprocessing
We used Landsat Collection 1 Tier preprocessed and ready for analysis (Dwyer et al., 2018), which fulfills standard geometric and radiometric quality criteria, supporting consistent time series applications (Qiu et al., 2018). Detailed information about Landsat scene selection and availability is presented in Section 2.1. Once the scenes were selected, the next step was to apply cloud and shadow masks. We used the Temporal Dark Outlier Mask (TDOM) algorithm and the Band Quality Assessment (BQA) band available in the Landsat Collection for that purpose, presented in detail in the code script (Supplemental material 1).
We also used annual Landsat cloud-free mosaics, obtained by applying a median reducer to all scenes selected every year from 1985 to 2022, as inputs to classify outcrops, bareland, and impervious classes. The Landsat quality assessment (QA) band was used to remove the shadow and cloud from each Landsat scene used to build the annual mosaics. Furthermore, we calculated the median value of the stacked bands for each year, removing the remaining cloud and shade not filtered by the QA band. We also used ancillary data to assist in mapping the outcrop class. The Global 30 meters height above the nearest drainage (HAND) (Rennó et al., 2008) dataset was used for that, combined with the sub-pixel compositional information generated with spectral mixture analysis (see Section 2.5.1 for more detail). HAND offers a worldwide depiction of elevation relative to the closest drainage point at a resolution of 30 meters, which helps detect regions with steep slopes and ridges associated with outcrops. The SRTM data are also an input to map the outcrop class.
ii) Spectral mixture analysis
We estimated the pure spectral composition's sub-pixel proportion (or fraction), named endmember of green vegetation (GV), soil, non-photosynthetic vegetation (NPV), and cloud in each pixel using a generic SMA model (Souza et al., 2005). The SMA assumes that the reflectance of each pixel in each band of the Landsat image can be modeled as a linear combination of the fraction image Fi of the endmember components and its additional residual:
where ρb is a reflectance of the nth mixed, in band b, i is the reflectance of the pure component i, in band b, Fi the fraction (or proportion) of the endmember component i, and εb the residual error of each band. The fractional values of the endmember in the pixel i sum up to one (i.e., 100%) so that,
The SMA is solved to estimate the unknown fraction values Fi of each endmember component within the Landsat image pixel, thus obtaining sub-pixel proportions of vegetation, NPV, soil, and cloud. The shade fraction is obtained as a complement of the sum of all fractions for each pixel. In this case, the shade endmember is set to zero (i.e., photometric shade) in all Landsat bands. The endmember values used in this study have been published elsewhere (Souza et al., 2005). Fractional information of each was also used to calculate additional SMA indices, including the normalized difference fraction index (NDFI) (Souza et al., 2005) and the canopy shade factor index (CSFI), a novel fractional index presented in this study. These sub-pixel compositional fractional and derived indices had been used in time series analysis (Bullock et al., 2020a), and in this study, we used them to classify the land cover classes targeted in this study. Detailed information to calculate the SMA fractions and indices using Google Earth Engine is presented in Supplementary material 1.
iii) Land cover classification
The land cover classification steps included: (i) defining the reference dataset to train, calibrate, and test the map results; (ii) applying data augmentation to train and calibrate RF models for each Landsat scene; (iii) building the RF classification model and applying it to each Landsat scene to generate annual land cover maps; (iv) applying post-classification algorithms to remove classification errors and artifacts; and (v) assessing the map accuracy.
We used the point reference data generated by the MapBiomas Project (Parente et al., 2021; Sales et al., 2021), which contains tens of thousands of sample pixels. The reference dataset was built annually from 1985 to 2021 with colocated land cover classifications over Brazil. Sample pixels were randomly selected using topography and land change proportion strata. Several trained interpreters carefully assigned land cover and land use classes to Landsat pixels through visual interpretation, spectral information from Landsat and Modis, and higher spatial resolution imagery (when available). The reference dataset classification was harmonized with the classification legend of this study (Table 1). We used all 35,000 pixels that fell inside the borders of the Amazon Biome and split them into training and calibration (10k samples; 29%) and test datasets (25k samples; 71%).
Because our land cover classification approach is applied to all Landsat scenes, we had to implement a data augmentation technique to get enough samples per class to train and calibrate the RF algorithm. First, we segment the Landsat scene using the simple non-iterative clustering (SNIC) algorithm, using six Landsat bands (red, green, blue, NIR, SWIR-1, and SWIR-2). Then, we overlay the point reference data sample with the segmented Landsat scene matching the year. The image segments with more than one land cover class were excluded, leaving only segments with a single class. We assessed the level of class purity within the image segment using existing land cover maps (i.e., MapBiomas Collection 7). Finally, we generate random samples within the selected image segment (i.e., polygon region) and assign the same thematic classification to the reference data sample used to select the image segment. This process guarantees enough samples for the RF training and calibration for the majority of the thematic classes (Table 1). This approach was applied in the +86k Landsat scenes selected for the Amazon biome through the time series.
We used the random forest (RF) classifier available in Google Earth Engine (Gorelick et al., 2017) using as input data the SMA sub-pixel fractional information, which included: green vegetation (GV) soil, non-photosynthetic vegetation (NPV), cloud, shade, NDFI, and CSFI. This selection was based on the feature importance analysis, which pointed out that the fraction bands were in the top 10 list of the most important features to classify the Landsat scenes together with the NIR and SWIR bands. The number of input features selected was the default value for this parameter (i.e., mtree, which is given by the square root of the number of features as defined for each biome). The RF algorithm was set to run 50 to 100 iterations, which allows for the lowest calibration error.
The land cover classes wetland, outcrop, bareland, and impervious did not have enough reference sample data (i.e., classified pixels) for training and calibrating the RF classifier. To overcome that, we classified these classes independently and integrated them into the post-classification step (Figure 3). Wetlands can be found in three land cover types: forest, shrubland, and natural grassland (Table 1). These classes can be permanently or sporadically flooded. We randomly selected stratified samples using the MapBiomas Surface Water dataset to train and calibrate the RF model to classify wetland (Supplementary material 2). Then, we used SRTM, HAND, and SMA fractions as inputs to the RF to classify wetland. We combined the wetland binary map with the forest class to obtain the flooded forest (i.e., overlapping with wetland). The areas where shrubland and natural grassland overlapped with wetland were reclassified to the latter class.
Outcrop has a unique spectro-temporal behavior, with a stable compositional trend of soil, NPV, and GV. We empirically defined the outcrop compositional trend and used empirical rules to detect and map this class. Additionally, we included information about altitude, steep slopes, and geomorphological features such as escarpments and the top of hills using SRTM and HAND. Then, we selected stratified samples (with outcrop and no outcrop) to train and calibrate an RF model to map outcrop.
Similarly, we identified the compositional trend of bareland and impervious surfaces using SMA fraction images and derived indices. We used the dynamic world (Brown et al., 2022) dataset available in the Google Earth Engine to randomly select stratified samples for the classes impervious/bareland and others. Then, RF was used to generate annual binary maps of impervious/bareland to update the annual land cover maps. Detailed information on how these classes were mapped can be found in Supplementary material 3.
iv) Post-classification
In this step, we generated the final annual land cover and land cover change maps using the Landsat scenes classified individually. For each year, we calculated intra-annual map metrics to measure classification stability, class changes, and classification errors, as proposed by Pontius et al. (2017). Then, we trained and calibrated RF with the samples used to classify each Landsat scene to generate the annual land cover maps (Figure 4). More details about the annual classification are provided in Supplementary material 1.
Finally, we applied spatial and temporal filters to generate the final annual land cover map collection, which we used to measure land cover change (Figure 3). The spatial filter uses a majority-neighborhood logic to replace single pixels or a group of pixels. The mode of the neighborhood classes replaces areas smaller than 1 hectare. This approach helps to eliminate undesirable transitions deriving from classification. The temporal filter is a set of rules for non-allowed transitions applied to each image classified in a given year. For example, it was possible to remove clouds and correct non-allowed transitions. A total of 50 temporal rules, distributed in 3 groups, were used: (a) rules for cases not observed in the first year; (b) rules for cases not observed in the final year; and (c) rules for examples of implausible transitions or those not observed for intermediate years (Supplementary material 5).
v) Map accuracy
We evaluated the mapping accuracy and area estimation using the good practices protocol (Olofsson et al., 2014) based on 25,000 independent test samples obtained with reference data splitting (Figure 3). Then, we estimated each class's overall accuracy and the user's and producer's accuracy with 95% confidence. We then assessed each year's map accuracy using the reference samples. Because not all samples could be utilized annually due to data cloud coverage or the inability to classify a sample pixel, the sample size varied annually.
Figure 3. Image processing steps and algorithms applied to map land cover dynamics in the Brazilian Amazon biome at the Landsat sub-pixel level.
Figure 4. Example of the intra-annual land cover classification for a subset (256 × 256 pixels) of the Landsat 227/062, in the Santarém-PA region; (A) the Landsat scenes processed for 2022 (n = 28); (B) the classification metrics used in the RF post-classification; and (C) the annual land cover classification. The cropland extent was larger in the more recent images; the area increased in the mode metric; however, crop occurrence is concentrated in small areas along the year. The reference training and calibration samples showed a higher concentration of pastureland in this area, making the RF model classify most of the areas in this land cover class.
The Landsat annual map time series generated in this study allowed us to assess land cover change and the dynamics of the Brazilian Amazon biome. We present first the changes that happened between the initial date (1985) of the Landsat time series analyzed and the end date (2022) (Figure 5). In 1985, the Amazon Biome comprised 97% of natural land cover classes (forest, shrubland, natural grassland, and water). We expect some level of anthropic disturbance in the natural land cover during this period. For example, forests are affected by logging, fire, and fragmentation; fires in shrubland and natural grassland and damming water bodies affected these land cover classes by 1985. Pasture covered only 2.9% (i.e., 12.10 Mha) of the biome with a tiny relative area of cropland (400 thousand ha) (Table 2).
Figure 5. Amazon biome land cover in 1985 and 2022. The main change was pastureland replacing forest and cropland expansion along the southern Mato Grosso border with the Cerrado biome.
Astonishingly, over the 37 years of our Landsat time series analysis, we found extensive land cover change, affecting primarily the forest class. The most extensive land cover change was the conversion of forest to pastureland. We estimated that 46.6 Mha (11% of the forest in 1985) of forests were lost over the 37 years analyzed, which represents an average loss of 1.254 Mha/year (Table 2). Pastureland increased by 44.7 Mha (10.6% increase relative to 1985), which means that forest conversion to pastureland dominates in the Amazon (95.7%). The comparison of the initial and end maps of the land cover time series also shows that forest loss no longer concentrates in the so-called arc of deforestation region, which is in the southern fringe between the Amazonia and Cerrado biomes, going up north along the eastern border. In 2022, we found three new hot spots of forest loss: in the midlands of Pará, along the BR-163 road connecting Santarém-PA to Cuiabá-MT, and in the southern Amazonas (Figure 5).
This section explores the yearly land cover change over the entire time series between 1985 and 2022. We investigated the trend (Figure 6) and breaking points (Figure 7) in the time series that reveal major shifts in change among the land cover classes. We applied the Loess function to the annual land cover maps to estimate the changing trend (Figure 6).
Figure 6. Land cover area yearly trend during 1985 and 2022 detected by the Landsat time series at the sub-pixel level. The red line is the Loess function time series trend.
Figure 7. Annual area change and the Loess trend line, with uncertainty interval, during 1985 and 2022 for the land cover classes of the Amazon Biome.
The forest class steadily decreased between 1985 and 2022, from 362.0 Mha to 315.6 Mha, which reduced the forest cover in the Brazilian Amazon biome approximately to 75% (Table 2). When inspecting the annual forest cover change, we observed four temporal breaking points associated with forest loss (Figure 7). The first breaking point period, between 1985 and 2004, showed the fasted rate of deforestation (1.62 Mha/year). The second period entered the deforestation control phase between 2004 and 2012, when forest loss reached a low annual rate in 2012, with an average annual rate of 0.94 Mha/year. The year 2013 marked the start of a new period of increasing deforestation that will extend until 2022, the end of our time series analysis. We also found that some years showed forest gain due to secondary regrowth [not captured in Brazil's official forest monitoring system (Valeriano et al., 2012)]. From 2013 to 2022, the average forest loss was 1.04 Mha/year (Figure 6). Natural grassland and shrubland land cover classes showed a monotonous temporal trend, with some years of decrease in extent, possibly associated with droughts and fires (i.e., in 2000 and 2010). We identified two possible decadal change cycles, marked in 2004, in these land cover classes, which deserve further in-depth analysis with climate and other variables (not the subject of this study).
The land cover classes associated with water land cover classes (i.e., water, wetlands, and flooded forests) showed a decreasing trend in annual extent (Figure 6). Surface water started reducing in 1998, with several years below the average annual change, notoriously 2004–2005, 2010, 2016, and 2020, possibly associated with extreme droughts (Marengo et al., 2023) (Figures 6, 7). The annual change in the flooded forest class showed a similar temporal trend as in water. However, wetlands exhibited a steeper loss after 2010, a hallmark year of consecutive dry years in the Amazon region (Parrens et al., 2023). As expected, the outcrop class showed no significant change over the 37-year-time series due to its long-term geologic stability. Our outcrop mapping approach minimized the vegetation phenological changes expected in the land cover class because we used dry season imagery mosaics to map it.
The annual time series trend analysis also revealed temporal breaking points associated with pastureland, cropland, and bareland and impervious land cover classes (Figures 6, 7). A detailed inspection of the annual area change behavior showed three temporal patterns of pastureland expansion (Figure 7). First, between 1985 and 2000, the pastureland area expanded to 22.66 Mha at an annual average rate of 1.51 Mha/year; then decelerated to 0.99 Mha/year between 2000 and 2013, and slightly increased until 2022 at 1.0 Mha/year. Cropland expansion was marginal between 1985 and 1996 at an annual average of 2,550 ha/year (Figure 6). Cropland annual area changes exhibited three distinct temporal rate patterns (Figure 7). The trend Loess function revealed that 1996 was the temporal breaking point for cropland faster expansion in the Amazon Biome, with two periods: 1996–2008 and 2008–2022. In the first period, the cropland annual average expansion rate was 158,500/year, and in the second one, 233,000 ha/year (Figures 6, 7).
Bareland and impervious are a mixed land cover class, including urban areas and infrastructure (roads and large mining infrastructure) for the impervious surfaces, and abandoned pastures with unvegetated topsoil (i.e., bareland), also found in most rural towns. We also found three breaking point periods for this land cover class: 1985–2000, with an expansion of 21,682 ha at a rate of 1,457 ha/year; 2000–2013, expanding almost 95,000 ha (at 7,271 ha/year); and 2013–2022 gaining 143,929 ha (at 15,992 ha/year) (Figures 6, 7).
We used the annual land cover trend analysis presented above to estimate the annual area change in each mapped class and to define temporal breaking points that indicated how fast or slow the changes happened. In the section, we used the breaking points to understand the land cover class transitions in forest and aquatic ecosystems (Figure 8).
Figure 8. Land cover class transitions in the Amazon Biome at the temporal breaking points associated with forest conversion (A) and in the water ecosystem (B).
The annual land cover change analysis identified three key breaking points along the 1985–2022 time series associated with forest loss: 1998, 2004, and 2013. These breaking points are temporal markers of deforestation, mainly due to pastureland expansion (Figure 8A). We also detected, to a smaller extent, pastureland-to-cropland conversion and pasture abandonment (followed by potentially secondary growth). We also estimated that 2 million hectares of pastures were abandoned and potentially entered into secondary vegetation growth, and that 27 thousand hectares were shifted to cropland (Figure 8A). Forest directly converted to cropland was more than four times larger (i.e., 127 thousand hectares) relative to pastureland conversion to this class. A tiny area (600 hectares) of cropland was abandoned and followed secondary growth. Interestingly, 28.7 thousand hectares of cropland returned to pastureland in this period (6.2% more than the opposite). Only 10.1 thousand hectares remained as cropland in the following period, i.e., 35% (Figure 8A).
Between 1998 and 2004, it was also a period with very high annual deforestation rates, with 2004 considered the second largest annual rate of forest loss in the region, according to the official deforestation statistics in Brazil (Valeriano et al., 2012). The annual rate of pastureland abandonment also increased by 15% (2.3 Mha), and pastureland converted to cropland increased almost 20-fold (532 thousand hectares) relative to the previous one, which marks the acceleration of crop expansion in the Brazilian Amazon. We detected approximately five times as much direct forest loss to cropland (i.e., 536 thousand hectares) relative to the previous period. Cropland shift to secondary forest regrowth was 1.18 thousand hectares (Figure 8A).
The 2004–2012 period was recognized as the ‘golden' period of deforestation control in the Brazilian Amazon (West and Fearnside, 2021). However, our analysis showed that the annual deforestation rate in the first 3 years of this period was not different from 1985 to 1998. Lowering deforestation started after 2008 and lasted until 2012. Cropland expansion over pastureland reached 1.0 Mha, and 153 thousand hectares were the opposite. This type of land use shift occurs in farms as a soil management practice in crop-livestock systems (Monteiro et al., 2024). The Pastureland and Cropland convertions to secondary growth forest were 2.4 Mha and 590 thousand hectares, respectively (Figure 8A).
The 2013–2022 period is notable for a lack of deforestation control in the Brazilian Amazon biome (Gatti et al., 2023). Annual forest loss rates to pastureland and cropland were 1.44 Mha/year and 44.2 thousand hectares/year (Figure 8A). The total direct forest to cropland conversion area summed 385 thousand hectares, and pastureland shifted to 3.3 Mha, establishing extensible crop fields in southern Mato Grosso (Figures 5, 8A).
The aquatic ecosystems showed two prominent temporal breaking points: 1998 and 2010 (Figure 7). Surface water was reduced by 10 thousand ha between 1985 and 2022, with distinct dynamic patterns in the breaking point periods. In the 1985–1998 period, water changed among the water-influenced land cover classes (i.e., water, flooded forest, and wetland). We found that 3.36 thousand ha of water class changed to wetland, indicating that surface water was reduced to the point that soil and vegetation mixed with water (Figure 8B). The change from water to natural grassland was 1.2 thousand ha and 0.74 thousand ha to flooded forest, indicating surface water loss. Conversely, flooded forest and wetland were covered by water in this period, with a total change of 4,62 thousand ha (96% to wetland). This may indicate that new areas were permanently flooded. Overall, ~4.22 thousand ha of surface water (i.e., water class) mapped in 1985 was displaced, and new surface water was gained, extending approximately 100 thousand ha. From 1998 to 2010, the change in water to wetland almost doubled to 6.42 thousand ha, relative to the previous period (Figure 8B). A similar trend was observed from water to natural grassland, with a change of 2.70 thousand ha.
We also mapped the spatial distribution of the land cover change along the temporal breaking points (Figure 9). The forest-to-pastureland conversion concentrates on the arc of deforestation between 1985 and 2004, along the fringe of the Amazon biome with the Cerrado and Caatinga biomes in the southern and eastern borders, respectively. After that, pastureland replaced forest, mostly toward the core of the Amazon (Figure 9A). Cropland expansion was concentrated in the southern Mato Grosso states, with less extent in the eastern Amazon. Secondary growth vegetation occurred along the regions where pastureland is concentrated, with more concentration in the northeast of the Amazon biome. The water loss occurred along the Amazon River margins in its estuary on the Marajó-PA Island (Figure 9D). The varzea region on the northern Amazon border with Roraima also showed large areas of water loss, as did the southern border of the Amazon biome with Pantanal and in the Eastern Amazon. Water gains are mostly associated with damming for hydroelectric power, with less extent flooding in Marajó-PA Island in the estuary of the Amazon River.
The quantification, characterization, and mapping of land cover change indicated that pastureland expansion is the major change driver in the Amazon biome, followed by cropland expansion, and that water losses, especially after the 2010 severe drought followed by more frequent similar events (Marengo et al., 2018), suggest that climate change is a newer driver affecting water ecosystems in the region.
We estimated annually the mapping accuracy between 1985 and 2021 (there was no reference data for 2022) using the independent reference test sampled (25k). The mean overall accuracy during these years was 89% ± 0.0023. In this study, we present the users' accuracy per class, which is more meaningful because it measures how each land cover class mapped agrees with the reference data (Stehman and Czaplewski, 1998) (Supplementary material 5 presents all accuracy metrics per year).
The forest class had the largest users' accuracy, ranging from 94 to 95%, and the largest test samples along the time series (18,657, sd = 1,061; 74.6%). Pastureland had the second largest test samples (2,796, SD = 827), and its users' accuracy ranged from 57 to 86%. The user's accuracy of pastureland increased over time, reaching 71% in 1995 and above 80% in 1999. Cropland (186 samples, SD = 175) had users' accuracy below 50% until 2004, but after that, it ranged between 51 and 68% (mean = 62%). Natural grassland (756 samples, SD = 42) exhibited users' accuracy of 54–58% between 1986 and 2012, increasing to 63–70% after 2013; and shrubland (299 samples, SD = 17) had the lowest one (mean = 11%).
For the aquatic land cover classes, water had the best users' accuracy, ranging from 89 to 93% (mean = 91%, 373 samples); wetland (232 samples, SD = 8) was 49–64% (mean = 57%). Flooded forest (373 samples, SD = 8) had users' accuracy below 50% along the annual mapping time series (mean of 44%). It was not possible to estimate the users' accuracy for bareland, impervious, and outcrop because these classes had < 50 test samples per year.
The reference sample we chose for this study was designed to train a different land cover classification scheme. We harmonized the reference samples to the Amazonia biome, which is further integrated into the final MapBiomas mapping product. The reference test sample estimated the overall accuracy with a margin of error of 5%. It is crucial to highlight that the sample size for reference data in several classes is small, as described above, requiring another sampling design, even though we decided to present the area error estimate using good practices (Olofsson et al., 2014) (Figure 10).
Figure 10. Pixel counting and area adjustment for the land cover classes mapped. The area adjustment for 2022 was not presented because the reference data were not available.
Comparing the land cover area estimates with pixel counting and areas adjusted using the accuracy error matrix (Figure 10) reveals that our mapping efforts to reconstruct the 37-time series presented in this study can identify the major land change drivers in the Amazon biome. The forest class showed similar annual area estimates using these two approaches, with pixel counting accounting for 1.5% less area on average. Our mapping approach underestimates the area of Pastureland by 14% (SD = 8%), with more difference between pixel counting and area adjusted in 1985–1994 (26%), reducing to 10.5% in 1995–2018, and converging (2.7%) in the last 3 years of the time series (2019–2022). Pixel counting overestimated the cropland area along the time series relative to the area adjusted, except for 1985 and 1986, but showed a similar area expansion until 2012 (27% larger on average in 1987–2012). After 2013, these area estimates showed different trends, with the area-adjusted estimate below 400 thousand ha (Figure 10).
Our mapping approach also underestimated the area of flooded forest by 28% on average (SD = 4%) and the wetland class by 42% (SD = 3%). Natural grassland was under-mapped on average by 5% until 2011, increasing to 17% after 2012. Shrubland was over-mapped with pixel counting along the entire time series at 13% (SD = 6%) but within the confidence interval (CI = 95%) in most of the years. Even though we estimated that the area adjusted to bareland, impervious, and outcrop, the results must be disregarded due to the limited number of samples needed to estimate the mapping accuracy (Figure 10).
The low mapping accuracy of the classes above did not affect the land cover change analysis because most of the changes occurred within the most predominant classes, and these classes showed higher accuracy (i.e., forest, pastureland, cropland, and water). Additionally, the area estimates of these main land cover classes showed similar results using pixel counting, and the area was adjusted with the accuracy metrics according to “Good Practices” (Olofsson et al., 2014), allowing us to infer the land cover change drivers in the Amazon Biome (presented in Section 3.4). The remaining cloud and shade after applying the TDOM algorithm did not affect the land cover mapping results because the SMA model handled them. However, we could not assess possible remaining atmospheric contamination (e.g., haze) in the Collection 1 Tier preprocessed Landsat data and its impact on the mapping uncertainty because we processed more than 86k Landsat scenes, and assessing individual scenes was unfeasible.
Given the high accuracy of mapping the forest class (93–94%), we designed a methodology to estimate annual deforestation directly from the land cover time series. We also applied a methodology to map the annual extent of secondary growth based on the methodology proposed by Nunes et al. (2020). We combined the annual forest losses and gains to estimate the annual forest balance and the annual deforestation of old-growth forests and secondary forests ≥ 5 years old (Supplementary material 6).
The total deforestation estimate in the Amazon biome was 52.7 Mha between 1985 and 2022 (Figure 11), and the total forest loss in this period was 46.4 Mha, less than the total deforestation because the forest class includes secondary forests. Subtracting the secondary growth forest (> 5 years old, because 1–5 years old is considered fallow land) gain of 5.7 Mha in 2022, we get 47 Mha of forest loss in 2022, meaning that the Amazon Biome lost 52.7 Mha over the 37 years analyzed, and 5.7 Mha is under secondary vegetation growth. The annual deforestation method used masked out forest loss annually, and to unmask it, we had to apply another method (Nunes et al., 2020) (Supplementary material 6). Therefore, we present the annual deforestation of old-growth forests and secondary vegetation (> 5 years old).
Figure 11. Spatial distribution of annual deforestation between 1986 and 2022 (A) and annual deforestation rate of old-growth forest and secondary vegetation > 5 years old (B).
We compared the annual deforestation rate for old-growth forests with other sources (Supplementary material 6, Figure 2). Our total deforestation estimate was 9.3% greater than the Prodes deforestation estimate (48.2 Mha) for the Amazon region from 1988 to 2022 (period overlapped with Prodes; Figure 2, Supplementary Material 6). We observed more annual differences in our estimate with the Prodes one between 1991 and 2000 and less difference between 2000 and 2012. After 2012, our deforestation estimate was 18.9% larger than Prodes. We attribute the overall differences to the spatial resolution of our maps (i.e., 30m sub-pixel) compared to Prodes, which used 6.5 ha. Our mapping approach used all useful Landsat (>50% of cloud cover) scenes, whereas Prodes uses only one scene per year.
Another innovation brought by our land cover change analysis was to estimate the deforestation of secondary growth forests (Figure 11b). The tree loss cover product (Hansen et al., 2013), which considers both old-growth and secondary forests in the deforestation estimation analysis, does not separate these two deforestation classes. The annual deforestation from the tree loss cover product showed similar results to our estimates until 2013, increasing over most of the year until 2021. The deforestation in secondary forests > 5 years old adds up to 7 Mha between 1992 and 2022. It is important to highlight that secondary forest deforestation happens in areas previously deforested. When considering the total deforestation (i.e., in old-growth and secondary forests > 5 years old), the area of forest loss increases to 63.7 Mha from 1985 to 2022.
In this study, we presented the land cover dynamics of the Brazilian Amazon biome between 1985 and 2022 and the associated mapping uncertainties. The novelty of our mapping approach was to process more than 86 thousand Landsat scenes to extract sub-pixel compositional information as input to machine learning and empirical rules to map the 10 most significant land cover classes in the biome. The annual land cover maps revealed that forest loss is mainly associated with pastureland for cattle ranching, followed by cropland expansion. It was also possible to derive annual deforestation and secondary growth vegetation maps and statistics, with estimates compatible with Brazil's official deforestation and global deforestation products. We also characterized non-forested ecosystems, including shrubland and natural grassland classes, and assessed their anthropogenic change. It was also possible to identify water loss in aquatic ecosystems, which may be associated with extreme and frequent droughts, extended summer seasons, and higher temperatures. Future steps for our research will focus on the measure of forest disturbances related to forest degradation drivers, which include selective logging, fires, and forest fragmentation, based on this study's land cover mapping efforts. The 37-year improved spatial-temporal characterization of land cover change and drivers in the Amazon, revealed in this study, offers crucial details about the threats to the Amazon biome and how human intervention and, possibly, climate change have changed the region.
The datasets presented in this study can be found in online repositories. The names of the repository/repositories and accession number(s) can be found in the article/Supplementary material.
CS: Conceptualization, Data curation, Formal analysis, Funding acquisition, Methodology, Supervision, Writing – original draft, Writing – review & editing. LO: Conceptualization, Data curation, Formal analysis, Methodology, Supervision, Validation, Visualization, Writing – review & editing. JSo: Data curation, Methodology, Software, Validation, Visualization, Writing – review & editing. BF: Data curation, Methodology, Software, Validation, Visualization, Writing – review & editing. AF: Data curation, Methodology, Software, Validation, Visualization, Writing – review & editing. JSi: Data curation, Methodology, Software, Validation, Visualization, Writing – review & editing.
The author(s) declare financial support was received for the research, authorship, and/or publication of this article. This study was funded by the MapBiomas Initiative through the Arapyaú Foundation (#CT936).
The authors thank the MapBiomas Initiative for providing constructive feedback to improve the Amazon biome's mapping products, Imazon for offering the working space and support to carry on this study, and the donors who financed this study.
The authors declare that the research was conducted in the absence of any commercial or financial relationships that could be construed as a potential conflict of interest.
All claims expressed in this article are solely those of the authors and do not necessarily represent those of their affiliated organizations, or those of the publisher, the editors and the reviewers. Any product that may be evaluated in this article, or claim that may be made by its manufacturer, is not guaranteed or endorsed by the publisher.
The Supplementary Material for this article can be found online at: https://www.frontiersin.org/articles/10.3389/ffgc.2023.1294552/full#supplementary-material
Adams, J. B., Sabol, D. E., Kapos, V., Almeida, R., Roberts, D. A., Smith, M. O., et al. (1995). Classification of multispectral images based on fractions of endmembers - application to land-cover change in the Brazilian amazon. Remote Sens. Environ. 52, 137–154. doi: 10.1016/0034-4257(94)00098-8
Asner, G. P. (2009). Automated mapping of tropical deforestation and forest degradation: CLASlite. J. Appl. Remote Sens. 12, 3223675. doi: 10.1117/1.3223675
Asner, G. P., and Heidebrecht, K. B. (2002). Spectral unmixing of vegetation, soil and dry carbon cover in arid regions: comparing multispectral and hyperspectral observations. Int. J. Remote Sens. 23, 3939–3958. doi: 10.1080/01431160110115960
Brown, C. F., Brumby, S. P., Guzder-Williams, B., Birch, T., Hyde, S. B., Mazzariello, J., et al. (2022). Dynamic World, near real-time global 10 m land use land cover mapping. Sci. Data 9, 1–17. doi: 10.1038/s41597-022-01307-4
Bullock, E. L., Woodcock, C. E., and Olofsson, P. (2020a). Monitoring tropical forest degradation using spectral unmixing and Landsat time series analysis. Remote Sens. Environ. 238, 11. doi: 10.1016/j.rse.2018.11.011
Bullock, E. L., Woodcock, C. E., Souza, C., and Olofsson, P. (2020b). Satellite-based estimates reveal widespread forest degradation in the Amazon. Glob. Chang. Biol. 26, 2956–2969. doi: 10.1111/gcb.15029
Claussen, M., Brovkin, V., and Ganopolski, A. (2001). Biophysical versus biogeochemical feedbacks of large-scale land cover change. Geophys. Res. Lett. 28, 1011–1014. doi: 10.1029/2000GL012471
Congalton, R., Gu, J., Yadav, K., Thenkabail, P., and Ozdogan, M. (2014). Global land cover mapping: a review and uncertainty analysis. Remote Sens. 6, 12070–12093. doi: 10.3390/rs61212070
De Sousa-Neto, D., Gomes, E. R., Nascimento, L., and Pacheco, N. F., and Ometto, J. P. (2017). “Land use and land cover transition in brazil and their effects on greenhouse gas emissions,” in Soil Management and Climate Change: Effects on Organic Carbon, Nitrogen Dynamics, and Greenhouse Gas Emissions, eds M. A. Muñoz, and R. Zornoza (London: Academic Press), 309–321.
DeFries, R. S., Field, C. B., Fung, I., Collatz, G. J., and Bounoua, L. (1999). Combining satellite data and biogeochemical models to estimate global effects of human-induced land cover change on carbon emissions and primary productivity. Global Biogeochem. Cycles 13, 803–815. doi: 10.1029/1999GB900037
Dwyer, J. L., Roy, D. P., Sauer, B., Jenkerson, C. B., Zhang, H. K., Lymburner, L., et al. (2018). Analysis ready data: enabling analysis of the Landsat archive. Remote Sens. 10, 1363. doi: 10.3390/rs10091363
Friedl, M. A., Woodcock, C. E., Olofsson, P., Zhu, Z., Loveland, T., Stanimirova, R., et al. (2022). Medium spatial resolution mapping of global land cover and land cover change across multiple decades from landsat. Front. Remote Sens. 3, 894571. doi: 10.3389/frsen.2022.894571
Galiatsatos, N., Donoghue, D. N. M., Watt, P., Bholanath, P., Pickering, J., Hansen, M. C., et al. (2020). An assessment of global forest change datasets for national forest monitoring and reporting. Remote Sens 1, 1790. doi: 10.3390/rs12111790
Gatti, L. V., Cunha, C. L., Marani, L., Cassol, H. L. G., Messias, C. G., Arai, E., et al. (2023). Increased Amazon carbon emissions mainly from decline in law enforcement. Nature 2023, 1–6. doi: 10.1038/s41586-023-06390-0
Gorelick, N., Hancher, M., Dixon, M., Ilyushchenko, S., Thau, D., Moore, R., et al. (2017). Google earth engine: planetary-scale geospatial analysis for everyone. Remote Sens Environ. 2017, 18–27. doi: 10.1016/j.rse.2017.06.031
Grekousis, G., Mountrakis, G., and Kavouras, M. (2015). An overview of 21 global and 43 regional land-cover mapping products. Int. J. Remote Sens. 36, 5309–5335. doi: 10.1080/01431161.2015.1093195
Hansen, M. C., Potapov, P. V., Moore, R., Hancher, M., Turubanova, S. A., Tyukavina, A., et al. (2013). High-resolution global maps of 21st-century forest cover change. Science 342, 850–853. doi: 10.1126/science.1244693
Jones, K. B. (2008). “Importance of land cover and biophysical data in landscape-based environmental assessments,” in North America Land Cover Summit, eds J. C. Campbell, K. B. Jones, J. H. Smith, and M. T. Koeppe (Washington, DC: Association of American Geographers), 215–249.
Krausmann, F., Erb, K. H., Gingrich, S., Haberl, H., Bondeau, A., Gaube, V., et al. (2013). Global human appropriation of net primary production doubled in the 20th century. Proc. Natl. Acad. Sci. U. S. A. 110, 10324–10329. doi: 10.1073/pnas.1211349110
Lambin, E. F., Turner, B. L., Geist, H. J., Agbola, S. B., Angelsen, A., Bruce, J. W., et al. (2001). The causes of land-use and land-cover change:moving beyond the myths. Global Environ. Change 11, 261–269. doi: 10.1016/S0959-3780(01)00007-3
Ma, L., Li, M., Ma, X., Cheng, L., Du, P., Liu, Y., et al. (2017). A review of supervised object-based land-cover image classification. ISPRS J. Photogrammetr. Remote Sens. 130, 277–293. doi: 10.1016/j.isprsjprs.2017.06.001
Marengo, J. A., Ambrizzi, T., Reboita, M. S., Costa, M. H., Dereczynski, C., Alves, L. M., et al. (2023). Climate Variability and Change in Tropical South America. Cham: Springer International Publishing, 15–44.
Marengo, J. A., Souza Jr, C. M., Thonicke, K., Burton, C., Halladay, K., Betts, R. A., et al. (2018). Changes in climate and land use over the Amazon region: current and future variability and trends. Front. Earth Sci. 6, 228. doi: 10.3389/feart.2018.00228
Monteiro, A., Barreto-Mendes, L., Fanchone, A., Morgavi, D. P., Pedreira, B. C., Magalhães, C. A. S., et al. (2024). Crop-livestock-forestry systems as a strategy for mitigating greenhouse gas emissions and enhancing the sustainability of forage-based livestock systems in the Amazon biome. Sci. Total Environ. 906, 167396. doi: 10.1016/j.scitotenv.2023.167396
Nemani, R. R., and Running, S. W. (1995). Satellite monitoring of global land cover changes and their impact on climate. Clim. Change 31, 395–413. doi: 10.1007/BF01095154
Nunes, S., Oliveira, L., Siqueira, J., Morton, D. C., and Souza, C. M. (2020). Unmasking secondary vegetation dynamics in the Brazilian Amazon. Environ. Res. Lett. 15, 034057. doi: 10.1088/1748-9326/ab76db
Olofsson, P., Foody, G. M., Herold, M., Stehman, S. V., Woodcock, C. E., Wulder, M. A., et al. (2014). Good practices for estimating area and assessing accuracy of land change. Remote Sens. Environ. 148, 42–57. doi: 10.1016/j.rse.2014.02.015
Parente, L., Silva, A. P., Baumann, L., Lopes, V., Silva, E., Nogueira, S., et al. (2021). Multi-Decade Land Use and Land Cover Samples for Brazil Based in a Stratified Sampling Design and Visual Interpretation of Landsat Data (1985 — 2018). Silver Spring, MD: Zenodo.
Parrens, M., Al-Bitar, A., and Fleischmann, A. S. (2023). Monitoring extreme floods and droughts in the amazon basin with surface-water-based indices. J. Hydrometeorol. 24, 1417–1436. doi: 10.1175/JHM-D-22-0170.1
Pendrill, F., and Martin Persson, U. (2017). Combining global land cover datasets to quantify agricultural expansion into forests in Latin America: limitations and challenges. PLoS ONE 12, e181202. doi: 10.1371/journal.pone.0181202
Pérez-Cutillas, P., Pérez-Navarro, A., Conesa-García, C., Zema, D. A., and Amado-Álvarez, J. P. (2023). What is going on within google earth engine? A systematic review and meta-analysis. Remote Sens. Appl. 29, 100907. doi: 10.1016/j.rsase.2022.100907
Pontius, R. G., Krithivasan, R., Sauls, L., Yan, Y., and Zhang, Y. (2017). Methods to summarize change among land categories across time intervals. J. Land Use Sci. 12, 218–230. doi: 10.1080/1747423X.2017.1338768
Potapov, P., Hansen, M. C., Kommareddy, I., Kommareddy, A., Turubanova, S., Pickens, A., et al. (2020). Landsat analysis ready data for global land cover and land cover change mapping. Remote Sens. 12, 426. doi: 10.3390/rs12030426
Qiu, S., Lin, Y., Shang, R., Zhang, J., Ma, L., Zhu, Z., et al. (2018). Making Landsat time series consistent: evaluating and improving Landsat analysis ready data. Remote Sens. 11, 51. doi: 10.3390/rs11010051
Rennó, C. D., Nobre, A. D., Cuartas, L. A., Soares, J. V., Hodnett, M. G., Tomasella, J., et al. (2008). HAND, a new terrain descriptor using SRTM-DEM: mapping terra-firme rainforest environments in Amazonia. Remote Sens. Environ. 112, 3469–3481. doi: 10.1016/j.rse.2008.03.018
Roberts, D. A., Adams, J. B., and Sabol, D. E. (1993). Remote sensing of vegetation in Amazonia, ecological implications of spectral mixtures. Bullet. Ecol. Soc. Am. 74, 412.
Roberts, D. A., Keller, M., and Soares, J. V. (2003). Studies of land-cover, land-use, and biophysical properties of vegetation in the large scale biosphere atmosphere experiment in Amazonia. Remote Sens. Environ. 87, 377–388. doi: 10.1016/j.rse.2003.08.012
Roberts, D. A., Numata, I., Holmes, K., Batista, G., Krug, T., Monteiro, A., et al. (2002). Large area mapping of land-cover change in rondonia using multitemporal spectral mixture analysis and decision tree classifiers. J. Geophys. Res. Atmosph. 107, LBA−40. doi: 10.1029/2001JD000374
Sales, M. H. R., Bruin, d. e., and Souza, S. C., and Herold, M. (2021). Land use and land cover area estimates from class membership probability of a random forest classification. IEEE Trans. Geosci. Remote Sens. 18, 1–11. doi: 10.1109/TGRS.2021.3080083
Salih, A. A. M., Ganawa, E. T., and Elmahl, A. A. (2017). Spectral mixture analysis (SMA) and change vector analysis (CVA) methods for monitoring and mapping land degradation/desertification in arid and semiarid areas (Sudan), using Landsat imagery. The Egypt. J. Remote Sens. Space Sci. 20, S21–S29. doi: 10.1016/j.ejrs.2016.12.008
Song, X. P., Hansen, M. C., Stehman, S. V., Potapov, P. V., Tyukavina, A., Vermote, E. F., et al. (2018). Global land change from 1982 to 2016. Nature 560, 639–643. doi: 10.1038/s41586-018-0411-9
Souza, C. M., Kirchhoff, F. T., Oliveira, B. C., Ribeiro, J. G., and Sales, M. H. (2019). Long-term annual surface water change in the Brazilian Amazon Biome: potential links with deforestation, infrastructure development and climate change. Water 11, 566. doi: 10.3390/w11030566
Souza, C. M., Shimbo, J. Z., Rosa, M. R., Parente, L. L., Alencar, A. A., Rudorff, B. F. T. T., et al. (2020). Reconstructing three decades of land use and land cover changes in Brazilian biomes with landsat archive and earth engine. Remote Sens. 12, 2735. doi: 10.3390/rs12172735
Souza, C. M., Siqueira, J. V., Sales, M. H., Fonseca, A. V., Ribeiro, J. G., Numata, I., et al. (2013). Ten-year landsat classification of deforestation and forest degradation in the brazilian amazon. Remote Sens. 5, 5493–5513. doi: 10.3390/rs5115493
Souza Jr, C. M, Roberts, D. A., and Cochrane, M. A. (2005). Combining spectral and spatial information to map canopy damage from selective logging and forest fires. Remote Sens. Environ. 98, 329–343. doi: 10.1016/j.rse.2005.07.013
Stehman, S. V., and Czaplewski, R. L. (1998). Design and analysis of thematic map accuracy assessment: fundamental principles. Remote Sens. Environ. 64, 331–344. doi: 10.1016/S0034-4257(98)00010-8
Valeriano, D. M., Mello, E. M. K., Moreira, J. C., Shimabukuro, Y. E., and Duarte, V. (2012). Monitoring tropical forest from space: the PRODES digital project. Photogrammetr. Remote Sens. Spatial Inf. Sci. 12, 1–3.
Veraverbeke, S., and Hook, S. J. (2013). Evaluating spectral indices and spectral mixture analysis for assessing fire severity, combustion completeness and carbon emissions. Int. J. Wildland Fire 22, 707–720. doi: 10.1071/WF12168
West, T. A. P., and Fearnside, P. M. (2021). Brazil's conservation reform and the reduction of deforestation in Amazonia. Land Use Policy 100:105072. doi: 10.1016/J.LANDUSEPOL.2020.105072
Wulder, M. A., Roy, D. P., Radeloff, V. C., Loveland, T. R., Anderson, M. C., Johnson, D. M., et al. (2022). Fifty years of Landsat science and impacts. Remote Sens. Environ. 280, 113195. doi: 10.1016/j.rse.2022.113195
Keywords: land cover, land cover change, Amazon, Landsat, earth engine
Citation: Souza CM Jr, Oliveira LA, de Souza Filho JS, Ferreira BG, Fonseca AV and Siqueira JV (2023) Landsat sub-pixel land cover dynamics in the Brazilian Amazon. Front. For. Glob. Change 6:1294552. doi: 10.3389/ffgc.2023.1294552
Received: 14 September 2023; Accepted: 30 October 2023;
Published: 04 December 2023.
Edited by:
Celso H. L. Silva-Junior, Instituto de Pesquisa Ambiental da Amazonia (IPAM), BrazilReviewed by:
Yan Gao, Universidad Nacional Autonoma de Mexico, MexicoCopyright © 2023 Souza, Oliveira, de Souza Filho, Ferreira, Fonseca and Siqueira. This is an open-access article distributed under the terms of the Creative Commons Attribution License (CC BY). The use, distribution or reproduction in other forums is permitted, provided the original author(s) and the copyright owner(s) are credited and that the original publication in this journal is cited, in accordance with accepted academic practice. No use, distribution or reproduction is permitted which does not comply with these terms.
*Correspondence: Carlos M. Souza Jr., c291emFqckBpbWF6b24ub3JnLmJy
Disclaimer: All claims expressed in this article are solely those of the authors and do not necessarily represent those of their affiliated organizations, or those of the publisher, the editors and the reviewers. Any product that may be evaluated in this article or claim that may be made by its manufacturer is not guaranteed or endorsed by the publisher.
Research integrity at Frontiers
Learn more about the work of our research integrity team to safeguard the quality of each article we publish.