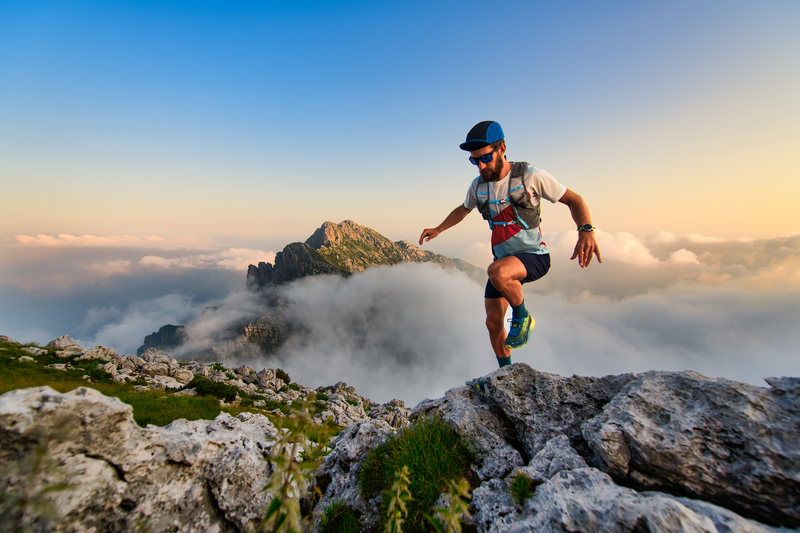
95% of researchers rate our articles as excellent or good
Learn more about the work of our research integrity team to safeguard the quality of each article we publish.
Find out more
ORIGINAL RESEARCH article
Front. For. Glob. Change , 21 March 2024
Sec. People and Forests
Volume 6 - 2023 | https://doi.org/10.3389/ffgc.2023.1243649
Background: High-quality in-forest landscapes are very important when landscape designers and foresters are planning and managing forest landscape resources. Understanding people’s visual behavior toward in-forest landscapes plays an important role in creating high-quality in-forest landscapes.
Methods: People’s visual information about in-forest landscapes with different landscape uniformity (IFLDLU) was visualized and digitized by eye-tracking technology and virtual reality method of picture, and the data were statistically analyzed by MANOVA, one-way ANOVA, Spearman’s rho correlation analysis, and linear regression in SPSS.
Purpose: This study aimed to discuss the influence of landscape uniformity on people’s visual behavior, satisfaction preference, and re-viewing intention, and to reveal the main reasons for the spatial cognitive characteristics of in-forest scenes that arouse their visual attention and re-viewing intention.
Main results: (1) The landscape uniformity of the in-forest landscape (IFL) affects people’s observation mode; (2) People’s visual attention differs across in-forest landscape uniformity, as mainly reflected in visual span and pupil diameter; (3) Overall, people prefer a cluster distribution of in-forest landscapes, for which they have higher re-viewing intentions; (4) Spatial cognitive characteristics that arouse participants’ visual behavior, satisfaction preference, and re-viewing intention vary with the landscape uniformity of in-forest landscapes. Among them, many spatial cognitive characteristics should be optimized in random distribution for IFL, arousing higher satisfaction preference and re-viewing intention. Therefore, when planning, designing, and managing in-forest landscapes, we suggest that spatial cognitive characteristics should be improved and optimized based on the landscape uniformity of the IFL to arouse participants’ positive visual attention, enhance their place identity, make them “reluctant to bid farewell,” and further arouse their place attachment.
With the acceleration of urbanization and the increase in forest leisure demand, people’s cognition of forests is changing (Wilkes-Allemann et al., 2017). Compared with the economic value of wood, people are inclined to pay increasing attention to the recreational functions of forests, including healthcare, leisure, and entertainment. Meanwhile, forest tourism has gradually become a leisure activity for people during their leisure time (Deng, 2009; Deng et al., 2013; Gao et al., 2020; Khachatryan et al., 2020; Wu et al., 2021; Shi et al., 2022). Based on the statistics of the China People’s Network in 2021, the number of forest tourists in China reached 7.5 billion during the 13th Five-Year Plan period. In the second half of 2020, the impact of the epidemic gradually weakened and the number of tourists nationwide gradually increased to 84.2% in 2019, which not only promoted the development of forest tourism in China but also made tourists start to focus on higher quality and high-level forest landscape spaces (China People’s Network, 2021).
However, in China, most forest parks are developed from state-owned forest farms with rich plantation resources, so early scholars focused on studying in-forest landscapes in terms of tree growth (Deng, 2009; Deng et al., 2013). For example, Liu claimed that the spatial structure of forests determines the competitive advantage and spatial niche among trees, which greatly affects the health and stability of the forest (Liu et al., 2014). The Voronoi diagram is used to study forest spaces and their distribution patterns. However, whether different landscape uniformity affects people’s preference evaluation and which forest landscape elements can attract tourists’ visual attention need to be further discussed.
As forest tourism develops and tourists pursue high-quality landscapes, it is increasingly important to discuss the public’s demand and preference for IFL and the spatial cognitive characteristics of high-quality landscapes (Oku and Fukamachi, 2006; Wu et al., 2019; Zhang et al., 2022). Previous studies have noted that arbor forest is absolutely dominant in forest landscapes, and its area ratio is relatively large, which means that to upgrade the overall quality of forest landscapes, arbor forest makes a great contribution (Bosun et al., 2007). High-quality in-forest landscapes can increase the attraction of a forest park itself, and after meeting the basic recreational conditions of tourists, it can also make more tourists feel attached to the scene (Wu et al., 2019).
Therefore, it is a subject worth in-depth study for planners and managers of landscape resources to create high-quality in-forest landscape spaces that conform to the public esthetic.
As Dachary-Bernard and Rambonilaza (2012) pointed out, esthetics greatly influence people’s cognitive preference for landscapes and their characteristics. At present, there are many studies on subjective evaluation and these studies have mainly used questionnaires and interviews (Chen and Qiao, 2015; Wu et al., 2021). With the development of research, these methods overly rely on the subjective evaluation of respondents, making their reliability relatively low (Daniel, 2001; Zhang et al., 2017a,b; Gao et al., 2020). Therefore, scholars tend to use quantitative research and direct evaluation to intervene in related fields. As the research of Dupont et al. (2015) pointed out, eye tracking technology can quickly and accurately catch changes in eye movement, such as pupil diameter and visual span, when people observe landscapes or objects. Meanwhile, Sun et al. (2018) pointed out that eye-tracking technology can be used to explore people’s psychological activities and perceptions of scenes when viewing landscape spaces. Compared with the subjective evaluation method, the multi-module analysis technology represented by eye-tracking technology can present more precise experimental data from more dimensions, which is conducive to researchers gaining a deeper understanding of the mechanism by which people and landscape interact, thus providing a decision-making theoretical basis for a high-quality landscape environment.
In addition, scholars have also proven that eye-movement technology has become a mature technology that can be feasibly used in landscape research (Zadegan et al., 2008; Wang et al., 2016; Franěk et al., 2018; Li et al., 2020; Ding et al., 2022; Li and Huang, 2022). The eye collects external information, and 80% of environmental information can be obtained through the eye, thus eye movement is the basis of external environment perception (Gratzer and McDowell, 1971; Huang and Lin, 2019). As early as the 1990s, scholars introduced eye-tracking technology into research on landscape preference (de Lucio et al., 1996; Duchowski, 2002).
Eye-movement technology and subjects’ cognitive preference for landscapes usually depend on their perception of landscapes. Eye-tracking technology is an important and effective means to study landscape preferences. The degree of attention to the landscape can help provide a deeper understanding of how people appreciate and view landscape space (Khachatryan et al., 2020). In other words, eye tracking can be used to more accurately understand the factors that attract people’s attention and viewing mode, thus helping designers and managers better plan and manage forest landscapes (Amati et al., 2018).
A study by Zhang et al. (2020) found that the visual heatmap of the forest landscape was relatively scattered in the boundary of various ecological elements. At the same time, when people view landscape photos, they are more likely to be attracted by the main buildings of the landscape or some prominent landscape elements (Guo et al., 2017). A higher fixation count and shorter average fixation time make it easier to extract spatial information and search more. A higher average fixation time of open landscapes means that it is difficult to extract information. A uniform landscape is associated with less fixation, saccades, and longer and faster eye movement, which indicates that visual exploration is weak in landscapes with monotonous characteristics (Dupont et al., 2014).
Furthermore, the intensity of landscape design is significantly related to visual quality (Xu et al., 2018). Landscape with proper design density can increase people’s perceptions of visual beauty, and landscape with moderate design intensity is more favored by Lothian (1999), Kurdoglu and Kurdoglu (2010), and Wu et al. (2021).
In addition, recent research results show that people spend a long time exploring the surroundings (top, bottom, left, and right sides), while they spend a long time fixated on the center of urban parks (Li and Huang, 2022). Plant form and species richness significantly affect people’s fixation behavior, while the arrangement of plants has no significant effect (Shi et al., 2022).
Amati et al. (2018) pointed out that manmade objects (lampposts, buildings, and benches) can be easily perceived and viewed, even if they only take up a small part of the scene. Sun et al. (2018) pointed out that advantageous buildings (such as pavilions and garden paths) can attract tourists’ attention. At the same time, when tourists view the plant community, the intersection of trees and shrubs can produce more fixation, and the density of plants also causes visual behavior changes. A study by Ding et al. (2022) found that people prefer to fixate on plant leaves, followed by flowers and fruits. Zhou et al. (2022) pointed out that people should spend more time identifying complex elements and proportions of elements in the process of place cognition.
Wang et al. (2018) found that tourists mainly focus on buildings, boundaries, places, and areas of the square. Research by Sang Ode et al. (2016) showed that fences, grass, marginal areas, and pastures of wooded vegetation attract the attention of tourists. Zhang et al. (2022) confirmed that cognitive indicators such as color brightness, spatial openness, uniformity, and layering of in-forest landscapes affect people’s visual appeal to varying degrees.
In summary, we found that in landscape research, at present, scholars mostly use eye-tracking technology to study landscape attractiveness, landscape elements, landscape facilities, and landscape perception (Gratzer and McDowell, 1971; Juristo et al., 2007; Chen and Qiao, 2015; Huang and Lin, 2019; Gao et al., 2020).
On the other hand, Hands and Brown (2002) pointed out that the number of colors and the diversity of landscapes are the main driving factors that significantly affect people’s visual preference in industrial wasteland landscapes. Kendal et al. (2012) confirmed that the esthetic and visual characteristics of plants (leaf color, leaf shape, flower size, etc.) significantly affect people’s preferences through their subjective evaluation of garden plants. Huang and Lin (2019) discussed the influence of people’s visual behavior on landscapes based on landscape color, complexity, and preference. People’s visual appeal is influenced by hue and chromaticity, and there is a certain correlation between people’s preferences and color. People pay more attention to photos with large color changes, especially magenta mountain landscapes. Gao et al. (2020) found that landscape type significantly influenced visual behavior and preference, while iconic elements and interesting landscape elements of scenes easily aroused people’s visual appeal. Zheng et al. (2022) pointed out that the visual attributes of green plants strongly influence people’s attention preference. People have a high subjective rating for trees + shrubs + grass, blue flowers, red flowers, pink flowers, broad-leaved trees, and bamboo.
Research on the evaluation of people’s preference for landscape spatial elements and their characteristics has gradually attracted the attention of experts.
However, we think that the composition of landscape space does not depend on only the type of space and its constituent elements. The landscape uniformity also affected people’s cognition (Deng et al., 2013). As Kaplan R. and Kaplan S. (1989) and Kaplan S. and Kaplan R. (1989) pointed out, people’s visual attention to landscape is closely related to the structure of the landscape itself. Meanwhile, Wu et al. (2019) also verified that stands with different characteristics (such as tree species, undergrowth density, and layout) have different influences on people’s esthetic preferences. In other words, people’s preferences for in-forest landscapes vary with landscape characteristics.
Throughout the previous studies, scholars have done more research on the environmental characteristics of forest landscapes and people’s preferences for them from the perspective of spatial content. However, how do the spatial structure characteristics (landscape uniformity) of in-forest landscape space affect people’s visual behavior; what spatial cognitive factors will affect the evaluation of people’s satisfaction preference; and what is the mechanism among in-forest landscape environmental characteristics, visual behavior, and preference evaluation remain to be further discussed. The specifics are as follows:
1. How does the landscape uniformity of the in-forest landscape affect people’s visual behavior?
2. How does the landscape uniformity of the in-forest landscape affect people’s satisfaction preference?
3. How does the landscape uniformity of the in-forest landscape affect people’s visual behavior, satisfaction preference, and people’s spatial cognitive characteristics?
Therefore, from characteristics of in-forest landscape environment visual behavior-preference evaluation, this study discusses the mechanism of the landscape uniformity of in-forest landscape environment on people’s vision and cognition. Through these analyses, planners and managers can grasp the preferences and needs of participants for in-forest landscape, thus to better plans and promote the effective utilization of in-forest landscape resources.
First, Cottet et al. (2018) pointed out that evaluations of perception and visual behavior do not differ significantly between landscape photos and actual scenes. Research using landscape photos has been proven to be highly reliable by scholars (Huang and Lin, 2019; Zhang et al., 2021, 2022; Shi et al., 2022).
Meanwhile, we hope that the experiment simulates the perception of landscapes in real life as closely as possible and that the photos used for the eye-movement experiment are as neat as possible to avoid the edited or simulated landscapes from causing patterned eye movement among participants.
Therefore, in our study, original photos of forest parks were selected as experimental materials. After on-the-spot investigation and screening, based on spatial representativeness and universality, Shenyang National Forest Park, Heyi National Forest Park, and Liberation Forest in Caohekou in northern China were finally selected as the research objects (Figure 1). IFL includes coniferous, broad-leaved, and mixed-forest landscape space.
Research by White et al. (2014) showed that landscape preference is often influenced by weather and seasonal conditions. Therefore, all the experimental materials (50 photos) in this study were collected at a consistent time (maple season in 2020, sunny day, and morning), with the same standard (height 170 cm) and the same equipment (Olympus EM5; Dupont et al., 2016, 2017).
In addition, for landscape uniformity, Hui and Gadow proposed the notion of the uniform angle index (Wi; Hui et al., 1999). According to the value of Wi, IFLDLU can be divided into an even distribution (W: <0.475), a random distribution (W: 0.475–0.517), and a cluster distribution (W: >0.517). The calculation formula is as follows:
Wi means the angle index of the ith reference tree; n means the number of adjacent trees of the reference tree, and n = 4 in this article; When the jth angle α < 72°, Zij = 1; otherwise, Zij = 0.
According to the above formula, we measured and statistically analyzed the tree height, DBH, crown width, and coordinates (X, Y) in our sample plot (20 m × 20 m), and calculated the uniform angle index of each sample plot by Winkelmass software. Finally, the 50 collected pictures are divided into three grades, namely even distribution, random distribution, and cluster distribution. Among 10 experts and 20 non-experts, 9 representative photos were selected as experimental materials (numbered A-I in Figure 2).
Figure 2. (A–I) represents the nine forest landscape scenes we have chosen. Because this picture mainly expresses whether each scene belongs to uniform distribution, random distribution or cluster distribution. So, we have not introduced the nine scenes of (A–I) in detail. Meanwhile here (A–I) is more like 1–9 to express an order.
Finally, through Python, the distribution of trees (20 m × 20 m) in various plots is intuitively reflected by the Voronoi diagram of the point set (Qin et al., 2008; Liu et al., 2014), see Figure 2.
Average fixation count (AFC), average lateral visual (ALV) span, average portrait visual (APV) span, average first fixation duration (FFD), and average pupil diameter (APD) are five eye-movement indicators commonly used in previous studies to analyze whether there are statistical differences in the participants’ visual observation modes of IFLDLU (Sang Ode et al., 2016; Guo et al., 2017; Sun et al., 2018; Xu et al., 2018; Wu et al., 2019, 2021). Therefore, we also chose these as our eye-movement index (Figure 3).
Our questionnaire consists of three parts (Figure 3).
Part 1: Assesses four basic attributes: the participants’ student number, gender, age, and major.
Part 2: Refers to domestic and foreign research literature to analyze the characteristics of the research object based on the principles of comprehensiveness, representativeness, and designability (Berto et al., 2008), accounting for the characteristics of the survey space in this study and avoiding the psychological burden on participants during the experiment, to establish an evaluation system of spatial cognitive characteristics. We used a 7-point Likert scale to quantify each spatial cognitive feature.
Finally, three first-level indicators of landscape change, color cognition, and spatial cognition, and eight second-level indicators of RPS, RLC, etc., were selected as the participants’ spatial cognitive characteristics surveyed.
Part 3: The overall evaluation of every scene includes two secondary indicators: SAP and RVI.
First, Blasco et al. (2009) proved that students’ esthetic abilities are extensive and that it is representative and feasible to use students for relevant eye-movement experiments. Therefore, we recruited 60 university students who had no eye diseases.
Second, the participants completed the eye-movement experiment at the Environmental Psychology Laboratory A of Forestry College of Shenyang Agricultural University. The experimental materials were shown using PowerPoint (PPT) projection, and the participants sat 3 m in front of the projection. The indoor environment is controlled to be noiseless and dimly lit to ensure that the participants are not affected by the outside world and can clearly observe the experimental materials. The experiment was carried out by using a German head-mounted SMI Glass2 eye-movement instrument. Before the experiment, 5-point calibration was used. The participant viewed one interference picture, nine experimental photos, and nine blank photos via PPT, and single-person eye-movement data were obtained at approximately 180 s/person. The specific experimental process, PPT playing diagram, and participant experimental diagram are shown in Figure 4.
Finally, the participants who completed the eye-movement experiment played the 9 pictures again and completed the corresponding spatial cognitive characteristics questionnaire for each scene in Environmental Psychology Laboratory B.
The above experiments were conducted after obtaining informed consent from all the participants and were in line with the ethical statement.
In our study, we recruited 60 undergraduate and graduate students (18–24 years), yielding 477 valid data, including 53 data for each landscape uniformity subtype (Figure 5).
Figure 5. Participants’ attributes of IFLDLU. EVD, In-forest landscape space with different layout of even distribution; RAD, In-forest landscape space with different layout of random distribution; CLD, In-forest landscape space with different layout of cluster distribution.
For each landscape uniformity scene, we chose three plots as experimental materials. Previous studies have shown that gender and major can affect people’s visual behavior. Therefore, to eliminate the differences caused by the interior of the landscape uniformity (subtype) and the attributes (gender and major) of participants, we used MANOVA to detect the main influences of landscape uniformity subtype, gender, and major on the eye movement and preference index of IFLDLU (Table 1).
We can see that the interaction effects of subtype, gender, and major on each measurement index are not significant (p < 0.05). Therefore, in this study, we did not explore the impact of landscape subtype, gender, and major on participants’ visual attention and satisfaction preference.
We visualized the fixation point position and fixation duration of participants viewing IFLDLU to obtain a map of the fixation point spatial distribution and fixation sequence path of the landscape (Figure 6). The following can be seen:
1 IFLDLU affects people’s attention. In even distributed IFL space, participants’ fixation behavior is scattered (X: 1,500–3,500 pixels; Y: 1,000–3,000 pixels), and the portrait viewing range is basically the same as the lateral viewing range. When appreciating the randomly distributed IFL space, the participants’ fixation behavior is relatively concentrated (X: 1,500–3,500 pixels; Y: 1,500–2,500 pixels), mainly reflected in the portrait viewing range. In addition, when participants view the cluster distributed IFL space, their visual behavior is more concentrated (X: 1,500–2,000 pixels; Y: 1,000–1,500 pixels and 2,000–2,500 pixels).
Figure 6. Spatial fixation distribution map and fixation sequence path map of IFLDLU. (1) The fixation point spatial distribution map is formed by the fixation points in the horizontal (X-axis) and vertical (Y-axis) and the fixation duration (Z-axis) of each fixation point in the scene. At the same time, we divide nine forest landscape scenes into three categories according to the size of the angular scale, so three figures are finally formed. (2) The fixation sequence path map is the participant’s fixation path when viewing the scene, in which 1, 2, 3, and other numbers represent the fixation order (the first fixation position, the second fixation position…), and the size of the circle represents the fixation duration. In our analysis, it is found that even the landscape scenes with the same plant layout will have different gaze fixation, so nine pictures are formed.
When participants view the IFL space, their visual behavior is changed based on plant distribution.
2 From the viewing sequence, generally speaking, people present three viewing orders when appreciating the IFL space: from top to bottom (Figures 6A-2,A-3,B-2,B-3,C-3), from middle to outside (Figures 6B-1,C-2), and from bottom to top (Figure 6A-1).
In addition, the fixation sequence presented different trends in each landscape uniformity space. We think this is the result of the characteristics of the constituent spatial elements.
From the viewing content, the random and even fixation points are mainly concentrated in the part of the canopy, trunk, and under forest, while the fixation points of cluster distribution of IFL are concentrated in the canopy and foreground plants (Figures 6C-1,C-3).
The visual data of participants viewing IFLDLU were analyzed using the one-way ANOVA (Table 2). Table 3 shows that the eye-movement index varies with landscape uniformity (p < 0.05). Participants’ fixation behavior differed when viewing IFLDLU, which is mainly reflected in the indicators of ALV, APV, and APD (p < 0.05).
Table 3. Relationships among landscape uniformity, eye-movement data, satisfaction preference, and spatial cognitive characteristics of the IFL space.
From these results, we can see that, overall, the ALV is larger than the APV in three spatial layouts of in-forest landscapes. In the random distribution, the ALV and APV of participants both show larger values (627.326, 37.602), which are significantly different from even and cluster distribution (p < 0.01). Compared with the even distribution, the APV of cluster distribution is larger (334.602 and 32.498), and the ALV is smaller (589.434 and 579.511).
Overall, when people appreciate the IFL space, they are more inclined to the “large-scale” horizontal visual processing mode. However, due to the characteristics of the space itself, especially the arrangement of plants, participants have large lateral and portrait exploration visual breadth in RAD. This means that participants’ viewing mode tends to “overall processing” of the scene in RAD. In addition, compared with the RAD, participants tend to view portraits in the CLD, that is, they tend to view the vertical changes in the space.
When participants view IFLDLU, their APD is different. This means that their psychological load, cognitive level, and processing load on the scene are different (p < 0.05). Among them, EVD (3.943) > RAD (3.167) > CLD (2.950).
The participants show a larger APD (3.943) when viewing EVD. When participants cognize the EVD, they have a heavy psychological load and need to invest more energy to cognize the scene, so the processing degree is relatively difficult. However, in CLD, the APD is smaller (2.950), so the cognitive level is relatively easy, and the processing load of the eyes in the process of recognizing the scene is also relatively small. This may be because plants with a cluster distribution are more concentrated than those with an even distribution of an in-forest landscape.
Although the FFD of participants is different when they view IFLDLU, CLD (3624.420) > RAD (2718.563) > EVD (2668.846), there is no significant difference (p > 0.05). This shows that when participants first appreciate IFL, their sensitivity to the space for IFL will not be significantly different due to the change in landscape uniformity.
In the above analysis, we find a phenomenon: the difference in information sources contained in the landscape uniformity space affects people’s cognitive processing mode of landscape visual interfaces.
A one-way ANOVA was used to analyze participants’ SAP and RVI when viewing IFLDLU. In addition, SAP as the independent variable and RVI as the dependent variable were used to analyze the relationship between SAP and RVI of the space for IFL through linear regression analysis (Figure 7).
Figure 7. Satisfaction preference, re-viewing intention, and differences of IFLDLU. EVD, In-forest landscape space with different layout of even distribution; RAD, In-forest landscape space with different layout of random distribution; CLD, In-forest landscape space with different layout of cluster distribution. SAP, satisfaction preference; RVI, Re-viewing intention. *Significant correlation with p < 0.05; **Significant correlation with p < 0.01.
First, we found that participants’ overall satisfaction with in-forest landscape space fluctuated within the range of 3.000–4.500, and there were differences in participants’ SAP and RVI in the IFLDLU. Participants’ satisfaction with the IFL space is not high, which also indicates that the landscape quality of IFLDLU still needs to be improved to various degrees.
Second, IFLDLU with high participants’ satisfaction has a higher RVI, and there is a significant linear relationship between them (Adj.R2 = 0.618, Sig. = 0.000). Participants’ satisfaction with the space for IFL is consistent with their RVI, and a higher SAP of the scene can lead to a higher RVI. People prefer to view the landscape that they think looks good, rather than those they dislike.
At the same time, from the average value of participants’ overall satisfaction with IFLDLU, we can see that, participants tend to view CLD (4.283, 4.113), and their satisfaction (3.686) and re-viewing intention (3.491) in RAD are low.
We used Spearman’s rho correlation analysis to explore relationships among landscape uniformity type, eye-movement data, satisfaction preference, and spatial cognitive characteristics when people view the IFL (Table 3).
First, the landscape uniformity of the IFL was significantly correlated with visual span and many spatial cognitive indicators (p < 0.005). When participants view IFLDLU, the APV and APD of participants are mainly affected by plant species, landscape richness, color richness and brightness, permeability, and uniformity of landscape space.
Second, the cognitive indicators that cause participants’ ALV mainly include the richness of landscape content and the three-dimensional sense of landscape; APV is mainly caused by plant richness, landscape content, and color; FFD is mainly caused by the sense of permeability; and APD is mainly caused by the richness of landscape content and the uniformity of space (p < 0.005).
Participants’ sensitivity to the scene is mainly influenced by the sense of penetration. When participants view the space of the IFL, they present a large ALV and APV. The stronger the three-dimensional sense of the scene, the more participants tend to laterally view the scene. The richer the plants and colors in the scene, the more participants tend to portrait view the scene. At the same time, if the richness of the landscape scene is too high and the space is more orderly, participants will have a greater psychological load, thus increasing the time spent cognitively processing the scene.
As there is a significant correlation between landscape uniformity and cognitive indicators, ALV, APD, etc. (p < 0.005), we analyzed the correlation among eye-movement indicators, SAP, and other spatial cognitive indicators of participants in EVD, RAD, and CLD. The SAP of each IFL spacer was taken as an independent variable and RVI as a dependent variable to explore the relationship between SAP and RVI in each type of landscape uniformity (Figure 8).
Figure 8. Relationships among eye movement index, satisfaction preference, and spatial cognitive characteristics of IFLDLU. *Significant correlation with p < 0.005; **Significant correlation with p < 0.01; −0.163** indicate that SHI is significantly related to APV in even distribution of in-forest landscape, other values are interpreted in the same way. AFC, Average fixation count; ALV, Average lateral visual span; FFD, Average first fixation duration; APD, Average pupil diameter; RPS, Richness of plant species; PLC, Richness of landscape content; NSI, Near-far stereoscopic impression; RCO, Richness of color; BCO, Brightness of color,; SPE, Spatial permeability, SUN, Spatial uniformity; SHI, sense of hierarchy; SAP, Satisfaction preference; RVI, Re-viewing intention.
First, Figure 8 shows that the spatial cognitive indicators that arouse participants’ visual behavior and satisfaction preference are different in IFLDLU. There is a significant linear relationship between the participants’ RVI and SAP (Adj. R2 is more than 60%, Sig. = 0.000).
Second, in EVD, RPS and APD mainly arouse changes in the participants’ FFD and APD for the scenes, and the hierarchy of sense itself has a significant negative correlation with APV (p < 0.05). The stronger the hierarchy in such scenes, the more they can arouse participants’ small-scale portrait observation mode.
Therefore, it is possible to arouse participants’ positive visual behavior and small-scale and local viewing mode by improving the hierarchy, plant richness, and spatial neatness degree to increase participants’ satisfaction with the scene and further arouse their re-viewing intention.
Furthermore, participants’ ALV and APV are mainly aroused by the cognitive characteristics of each space in RAD (p < 0.05). In RAD with varied landscape contents, such as a strong three-dimensional sense of the landscape and bright and transparent colors, participants tend to observe and appreciate the scene laterally in a large range; while in scenes with high plant richness, varied landscape contents, rich and bright color, and strong uniformity, it will trigger a wide range of portrait appreciation modes of participants or arouse/induce people’s overall cognition of the scene.
This means that spatial cognitive characteristics such as RPS, RLC, NSI, RCO, BCO, SPE, and SUN can be improved, thus affecting people’s overall cognition of a scene, improving the SAP of the scene, and arousing participants’ RVI.
In addition, SPE is negatively correlated with AFC, SHI is positively correlated with ALV, and both SPE and SHI are positively correlated with participants’ SAP in CLD (p < 0.05). The SPE and SHI of scenes can be improved to arouse participants’ fixation duration and large-scale viewing behavior, thus improving participants’ SAP and arousing participants’ RVI of the scene.
Kaplan S. and Kaplan R. (1989) pointed out that two dimensions of spatial configuration and content jointly influence people’s landscape visual experience. Our research found that different layouts of the IFL space can arouse people’s different visual observation modes, which leads to differences in their overall evaluation of space, and then affects their re-viewing intention or reuse of landscape.
People mainly arouse different visual behaviors through their cognition of IFLDLU, so that they have a sense of identity with the scene, which is manifested in their SAP for the scene. Then, they have different degrees of dependence inducing different re-viewing intentions. Meanwhile, optimizing the spatial cognitive characteristics of scenes can arouse participants’ “attachment” to scenes with different layouts. In other words, people’s appreciation of the landscape is a process of gradual understanding, and it is also a process of gradual scene perception. Figure 9 shows the cognitive mechanism of viewing in different spatial layouts.
Previous findings are consistent with some of our results of this mechanism:
Attributes of scenes (type, shape, density, etc.) affect people’s visual behavior patterns (Kaplan S. and Kaplan R., 1989; Liu et al., 2014; Wu et al., 2019; Gao et al., 2020). The plant structural characteristics, growth status, plant coverage, seasonal changes, hierarchical structure, and even the selection of characteristics of individual plants and special plants affect participants’ visual behavior. In our analysis results, people tend to explore laterally in EVD mode, and their visual attention is scattered; in RAD mode, people’s attention is concentrated, and they tend to overall process in “lateral + portrait” mode; while when people’s attention is more concentrated, they tend to portrait explore in CLD mode.
These findings may be due to the relatively uniform arrangement of plants, single tree species (coniferous forest), rich exposed ground, small shrubs, roots, and other interesting elements in EVD mode. Therefore, participants constantly laterally search for interesting objects instead of enjoying the portrait changes of plants when they view in-forest landscapes.
In RAD, plants are distributed in a concentrated way, and the scene looks chaotic. Therefore, participants need to constantly laterally explore and portrait search for information sources.
In CLD, plants are concentrated in layout with colorful broad-leaved trees. Therefore, people’s attention to the scene is concentrated. In addition, fallen leaves on the ground and portrait changes of plants in the scene easily attract the visual attention of participants.
This is in line with the behavioral characteristics of people in scenes in environmental psychology (“extensive exploration” and “special exploration” models). People look for interesting information in extensive exploration and understand the content of information in special exploration (Hu and Lin, 2016). The distribution of visual attention in the IFL space presents a “multiregional exploration mode” (represented by RAD and EVD) and a “concentrated exploration mode” (represented by CLD). Participants extensively explore interesting information in EVD and RAD with scattered landscape information, while they are willing to pay more attention and spend more time understanding the contents and meanings of the space in CLD. This point can also be explained by the finding that FFD is the longest and the visual span is the smallest in CLD, while FFD is the shortest and the visual span is the longest in RAD.
In addition, previous research has confirmed that visual exploration is weak and cognitive difficulty is low for uniform landscapes with monotonous characteristics (Dupont et al., 2014). This is contrary to our results: participants’ cognitive load of scenes present EVD (3.943) > RAD (3.167) > CLD (2.950). The reason for this difference may be that, although the even distributed scenes look monotonous, they are also rich in interesting elements such as bare ground, small shrubs, and roots, and the distribution is scattered. Therefore, participants’ cognitive load of scenes will increase. However, plants are concentrated and the components are single (mainly the colorful trees in autumn) in CLD, so participants can effectively identify and lock the areas of interest in the scene. Therefore, it is a cognitive process with less load.
Previous studies have found that landscapes with poor spatial structure have a negative experience effect. This means that a disorderly environment is esthetically annoying (Palmer, 2004; Zhang et al., 2021). Wu et al. (2019) also pointed out that participants’ preferences are influenced by the characteristics of stands. Similarly, the “prospect-refuge” theory points out that if people can watch and avoid an environment, it benefits their survival, and an environment with the characteristics of a prospect is loved by Palmer (2004). People like better lookout scenes, to a certain extent, because such scenes allow them to see the surrounding situation, thus enhancing people’s sense of security (Baran et al., 2018; Tabrizian et al., 2018).
This is consistent with our research results: people’s preference for IFL is influenced by plant layout. On the one hand, all the IFL space that we choose have certain prospect, but the prospect of EVD and RAD is relatively poor compared with those of CLD. Therefore, people present a high preference for CLD. On the other hand, people’s satisfaction is the lowest in RAD because random distribution is understood as disorderly landscape space to some extent (Wang et al., 2022).
In addition, the cognitive theory of emotion holds that people make adjustments to adapt to the environment while accepting the stimuli of the environment (Lasarus, 1991). On this basis, Bagozzi (1992) proposed the self-regulating attitude and noted that people’s attitudes toward a scene can be divided into three stages: the assessment of the scene, the emotion toward the scene, and the behavior in the scene. Liu et al. (2019) also pointed out that people’s place identity will arouse their place dependence. This means that increasing people’s satisfaction with the place can help stimulate their place attachment, thus changing their attitude toward the landscape, that is, increasing their RVI.
This coincides with our research results. First, our research shows that higher participants’ satisfaction with the scene aroused higher re-viewing intention. Second, we find that people’s cognition of scene shows a process of “cognition → evaluation → emotional response → emotional initiation.” When people view the landscape space, it is a process of recognition, thus stimulating the corresponding visual behavior. The continuous process of cognition also involves scene evaluation (place identity), which leads to different satisfaction preferences. The cognitive process will also stimulate people’s corresponding emotions (place dependence: make people’s attitude toward scenes change from “gone forever” to “be reluctant to bid farewell,” to arouse people’s attachment to the place emotionally), and further influence people’s behavior, that is, arouse people’s re-viewing intention (Figure 10).
Bourassa (1990) raised the possibility that people’s exploration of scenes includes not only rational cognition but also some instinctive perceptual cognition. However, in landscape scenes, we do not know what role people’s emotions play in scene cognition. The cognitive process of “rationality + sensibility” in the landscape will be an interesting topic and worthy of in-depth discussion.
There is a close relationship between visual esthetic preference and landscape change. People expect to see, live in, and visit places for which they have high visual esthetic preference, that is, beautiful landscapes (Gobster et al., 2007). The “biophilia hypothesis” states that abundant plants in forests are attractive to humans. Huang and Lin (2019) pointed out that high color change was positively correlated with preference, and high hue and chromaticity change can promote visual change. Our previous study also found that the cognitive factors that arouse people’s visual appeal vary by scene (Zhang et al., 2022). This phenomenon is also found in our analysis (Table 3; Figure 8).
First, the complexity (plant richness, landscape content, and color), color brightness, permeability, and space uniformity of in-forest scenes have higher participants’ satisfaction.
Then, in different spatial layouts, people’s visual attention and satisfaction preferences are driven by different spatial cognitive characteristics. However, regardless of the layout of a scene, their visual attention and satisfaction preference for the scene are influenced by multiple spatial cognitive characteristics.
The landscape space that is favored and attracts people’s attention varies with spatial layout, and it is not the function of a single factor, but the joint function of several factors. This means that in both urban green and forest landscapes, the main factors that affect participants’ preferences and visual behavior are the result of multiple factors. In the future planning and utilization of landscape resources, we should balance various factors to improve people’s sense of experience and even attachment.
Above all, we agree that people’s viewing mode of the IFL space and their cognition and understanding of landscape space are influenced by the layout of spatial elements. Meanwhile, people’s understanding of landscape space is a process from “what is in space” to “what is space.” Of course, after people understand the landscape, they appropriately enhance or weaken the characteristics of the space itself, to make appropriate judgments on the satisfaction preference and re-viewing intention of the scene.
First, regarding the experimental purpose, this study discussed the relationship between viewers’ visual attention and cognitive evaluation under different layout structures based on the spatial structure of IFL.
Regarding experiments, eye-movement technology is a relatively mature technology that has been widely studied in many fields. Meanwhile, the collection and design of experimental data in this study are based on previous research, and appropriate adjustments are made according to the particularity of the experimental plots. The experiments are also carried out with the consent of the participants.
Finally, some of our research results have been supported by previous studies, and we further found that the spatial structure of in-forest landscapes has different inducing effects on visual behavior.
Therefore, we believe that our experiment is feasible and repeatable.
First, this study mainly discussed and analyzed the peak tourist season in the north temperate zone (northern China): the maple season. However, research groups should further analyze whether the layout of different spatial structures affects viewers’ visual behavior characteristics and re-viewing intention differently across seasons. This will better reveal which structure of the IFL space is more suitable for people’s viewing or is well received in a completely natural cycle. This will also help managers of forest scenic resources expand and publicize scenic resources and tourist markets more pertinently, to achieve the sustainable utilization of scenic resources.
Second, this study explored the preference evaluation and visual behavior characteristics of college students. However, as our previous studies have shown, there are “professional effects” when discussing the landscape. “Ten people ten colors” is an eternal problem that designers need to face, which means that demographic characteristics are inevitable variables. Therefore, it is interesting and meaningful to explore the visual attention tendencies, preference evaluation, and re-viewing intention of participants from different backgrounds when the spatial structure changes.
Next, this study analyzes and discusses visual behavior and preference evaluation based only on the IFL space. However, the composition of forest landscape resources is diverse. Therefore, it is necessary to further explore various types of element layouts or spatial composition forms, which will help to further expand and deepen the landscape field, especially natural landscape research represented by forest landscape resources, which will contribute to the accurate planning, design and sustainable utilization of landscape resources.
Finally, this study collected relevant experimental materials from a real in-forest scene. Because of the strong naturalness of the in-forest landscape, it is difficult for us to find sample plots with different layouts under the same stand conditions during the sample plot collection process. Similarly, it is difficult for us to find the same subtype landscape space under different spatial layouts in the actual forest landscape (for example, each spatial layout includes coniferous, broad-leaved, and mixed-forest landscape spaces). However, it is easy to find such plots in urban park landscapes. Therefore, in future research, we will further search for such plots in urban landscapes and conduct in-depth research.
In this study, in northern China, nine landscape layout IFL subtypes in three spatial layouts of three forest parks were taken as examples. From the relationships among visual attention, spatial cognitive characteristics, satisfaction preference, and re-viewing intention, we clarify the visual attention and satisfaction preference for the IFL space with different spatial layouts, and the spatial cognitive characteristics that affect them. The main results are as follows:
1 In terms of visual attention, spatial layouts of IFL affect people’s observation mode. The fixation of the EVD is scattered, while that of the CLD is concentrated.
2 In terms of visual-behavior differences, there are differences in arousing people’s eye-movement behavior when across types of in-forest landscapes, mainly reflected in visual span and pupil diameter.
In addition, although participants’ visual behavior varied across IFL, there was no significant difference in the sensitivity to scenes.
3 In terms of satisfaction preference and re-viewing intention of the scene, overall satisfaction of the space of IFL fluctuates from 3.000 to 4.500. Moreover, people prefer CLD and have a higher expectation of re-viewing, and they tend to have the lowest satisfaction with RAD, with a lower willingness to re-viewing.
4 In terms of the main factors affecting visual attention, satisfaction preference, and re-viewing intention, spatial cognitive characteristics that arouse participants’ visual behavior and satisfaction preference are different in the IFLDLU. That means there are some differences in spatial cognitive characteristics of optimizing the visual quality and satisfaction preference of IFLDLU to arouse people’s higher re-viewing intention.
These results show that when planning and designing the landscape space of an in-forest scene, we should improve and optimize the spatial cognitive characteristic according to the spatial layout of IFL, to arouse participants’ positive visual attention, enhance their place identity (satisfaction preference), and make them “be reluctant to bid farewell” to the scene, thus improving their place dependence (re-viewing intention). Spatial cognitive characteristics of place should be improved to create high-quality IFL with different layouts so that participants not only have positive visual behavior and high satisfaction but also arouse attachment to the place.
The raw data supporting the conclusions of this article will be made available by the authors, without undue reservation.
Written informed consent was obtained from the individual(s) for the publication of any potentially identifiable images or data included in this article.
YG designed and conducted the experiments, analyzed data, and wrote and modified the manuscript. XS modified the manuscript and provided suggestions for this manuscript. ZZ, WZ, and HM provided suggestions for this manuscript. TZ collected the experimental materials, designed the experiments, and modified the manuscript. All authors contributed to the article and approved the submitted version.
The research was supported by the Educational Commission of Liaoning Province of China (LJK0687) and the Natural Science Foundation of China (31971714).
The authors would like to thank the reviewers for their comments on the study, which helped them better improve the ideas of the study. We would like to thank the visitors in the experiment and AJE (https://secure.aje.com/r/HVPMKW56) for their linguistic assistance during the preparation of this manuscript.
The authors declare that the research was conducted in the absence of any commercial or financial relationships that could be construed as a potential conflict of interest.
All claims expressed in this article are solely those of the authors and do not necessarily represent those of their affiliated organizations, or those of the publisher, the editors and the reviewers. Any product that may be evaluated in this article, or claim that may be made by its manufacturer, is not guaranteed or endorsed by the publisher.
Amati, M., Ghanbari Parmehr, E., McCarthy, C., and Sita, J. (2018). How eye-catching are natural features when walking through a park? Eye-tracking responses to videos of walks. Urban For. Urban Green. 31, 67–78. doi: 10.1016/j.ufug.2017.12.013
Bagozzi, R. P. (1992). The self-regulation of attitudes, intentions and behavior. Soc. Psychol. Q. 55, 178–204. doi: 10.2307/2786945
Baran, P. K., Tabrizian, P., Zhai, Y., Smith, J. W., and Floyd, M. F. (2018). An exploratory study of perceived safety in a neighborhood park using immersive virtual environments. Urban For. Urban Green. 35, 72–81. doi: 10.1016/j.ufug.2018.08.009
Berto, R., Massaccesi, S., and Pasini, M. (2008). Do eye movements measured across high and low fascination photographs differ? Addressing Kaplan’s fascination hypothesis. J. Environ. Psychol. 28, 185–191. doi: 10.1016/j.jenvp.2007.11.004
Blasco, E., González-Olabarria, J. R., Rodriguéz-Veiga, P., Pukkala, T., Kolehmainen, O., and Palahí, M. (2009). Predicting scenic beauty of forest stands in Catalonia (north-East Spain). J. Forest. Res. 20, 73–78. doi: 10.1007/s11676-009-0013-3
Bosun, W., Shaolin, P., Luo, G., and Youhua, Y. (2007). Diversity of tropical forest landscape types in Hainan Island, China. Acta Ecol. Sin. 27, 1690–1695. doi: 10.1016/S1872-2032(07)60040-7
Bourassa, S. C. (1990). A paradigm for landscape aesthetics. Environ. Behav. 22, 787–812. doi: 10.1177/0013916590226004
Chen, X. Y., and Qiao, L. F. (2015). Applicable prospects of eye tracking technology in the research of landscape visual perception. Int J Multimedia Ubiquit Engineer. 10, 111–118. doi: 10.14257/ijmue.2015.10.10.12
China People’s Network. (2021). Available at: http://travel.people.com.cn/n1/2021/0119/c41570-32004084.html (Accessed 19 January 2021.
Cottet, M., Vaudor, L., Tronchère, H., Roux-Michollet, D., Augendre, M., and Brault, V. (2018). Using gaze behavior to gain insights into the impacts of naturalness on city dwellers’ perceptions and valuation of a landscape. J. Environ. Psychol. 60, 9–20. doi: 10.1016/j.jenvp.2018.09.001
Dachary-Bernard, J., and Rambonilaza, T. (2012). Choice experiment, multiple programmes contingent valuation and landscape preferences: how can we support the land use decision making process? Land Use Policy 29, 846–854. doi: 10.1016/j.landusepol.2012.01.002
Daniel, T. C. (2001). Whither scenic beauty? Visual landscape quality assessment in the 21st century. Landsc. Urban Plan. 54, 267–281. doi: 10.1016/S0169-2046(01)00141-4
de Lucio, J. V., Mohamadian, M., Ruiz, J. P., Banayas, J., and Bernaldez, F. G. (1996). Visual landscape exploration as revealed by eye movement. Landsc. Urban Plan. 34, 135–142. doi: 10.1016/0169-2046(95)00208-1
Deng, S. Q. (2009). Effects of thinning intensities on landscape qualities of suburb ecological landscape forest-a case study in Nanjing Wuxiangsi National Forestry Park. Nanjing, China: Nanjing Forestry University.
Deng, S. Q., Yin, N., Guan, Q. W., and Katoh, M. (2013). Short–term effects of thinning intensity on scenic beauty values of different stands. J. For. Res. 18, 209–219. doi: 10.1007/s10310-012-0342-5
Ding, N. N., Zhong, Y. D., Li, J. X., and Xiao, Q. (2022). Study on selection of native greening plants based on eye-tracking technology. Sci. Rep. 12:1092. doi: 10.1038/s41598-022-05114-0
Duchowski, A. T. (2002). A breadth-first survey of eye-tracking applications. Behav. Res. Methods Instrum. Comput. 34, 455–470. doi: 10.3758/BF03195475
Dupont, L., Antrop, M., and Van Eetvelde, V. (2014). Eye-tracking analysis in landscape perception research: influence of photograph properties and landscape characteristics. Landsc. Res. 39, 417–432. doi: 10.1080/01426397.2013.773966
Dupont, L., Antrop, M., and Van Eetvelde, V. (2015). Does landscape related expertise influence the visual perception of landscape photographs? Implications for participatory landscape planning and management. Landsc. Urban Plan. 141, 68–77. doi: 10.1016/j.landurbplan.2015.05.003
Dupont, L., Ooms, K., Antrop, M., and van Eetvelde, V. (2016). Comparing saliency maps and eye-tracking focus maps: the potential use in visual impact assessment based on landscape photographs. Landsc. Urban Plan. 148, 17–26. doi: 10.1016/j.landurbplan.2015.12.007
Dupont, L., Ooms, K., Antrop, M., and van Eetvelde, V. (2017). Testing the validity of a saliency-based method for visual assessment of constructions in the landscape. Landsc. Urban Plan. 167, 325–338. doi: 10.1016/j.landurbplan.2017.07.005
Franěk, M., Šefara, D., Petružálek, J., Cabal, J., and Myška, K. (2018). Differences in eye movements while viewing images with various levels of restorativeness. J. Environ. Psychol. 57, 10–16. doi: 10.1016/j.jenvp.2018.05.001
Gao, Y., Zhang, T., Zhang, W. K., Meng, H., and Zhang, Z. (2020). Research on visual behavior characteristics and cognitive evaluation of different types of forest landscape spaces. Urban For. Urban Green. 54:126788. doi: 10.1016/j.ufug.2020.126788
Gobster, P. H., Nassauer, J. I., Daniel, T. C., and Fry, G. (2007). The shared landscape: what do aesthetics have to do with ecology? Landsc. Ecol. 22, 959–972. doi: 10.1007/s10980-007-9110-x
Gratzer, M. A., and McDowell, R. D. (1971). Adaptation of an eye movement recorder to esthetic environmental mensuration. Storrs agricultural experimental station. Connecticut, United States: The University of Connecticut.
Guo, S. L., Zhao, N. X., Zhang, J. X., Xue, T., Liu, P. X., Xu, S., et al. (2017). Landscape visual quality assessment based on eye movement: college student eye-tracking experiments on tourism landscape pictures. Resourc Sci. 39, 1137–1147. doi: 10.18402/resci.2017.06.13
Hands, D. E., and Brown, R. D. (2002). Enhancing visual preference of ecological rehabilitation sites. Landsc. Urban Plan. 58, 57–70. doi: 10.1016/S0169-2046(01)00240-7
Hu, Z. F., and Lin, Y. L. (2016). Environmental psychology. 3rd Edn. Beijing, China: China Building Industry Press.
Huang, A. S.-H., and Lin, Y.-J. (2019). The effect of landscape colour, complexity and preference on viewing behaviour. Landsc. Res. 45, 214–227. doi: 10.1080/01426397.2019.1593336
Hui, G. Y., Gadow, K. V., and Albert, M. (1999). The neighbourhood pattern-a new structure parameter for describing distribution of forest tree position. Sci Silvae Sinicae. 35, 37–42.
Juristo, N., Moreno, A. M., and Sanchez-Segura, M.-I. (2007). Analyzing the impact of usability on software design. J. Syst. Softw. 80, 1506–1516. doi: 10.1016/j.jss.2007.01.006
Kaplan, R., and Kaplan, S. (1989). The experience of nature: A psychological perspective. Cambridge, United States: Cambridge University Press.
Kaplan, S., and Kaplan, R. (1989). The visual environment: public participation in design and planning. J. Soc. Issues 45, 59–86. doi: 10.1111/j.1540-4560.1989.tb01533.x
Kendal, D., Williams, K., and Williams, N. (2012). Plant traits link people’s plant preferences to the composition of their gardens. Landsc. Urban Plan. 105, 34–42. doi: 10.1016/j.landurbplan.2011.11.023
Khachatryan, H., Rihn, A., Hansen, G., and Clem, T. (2020). Landscape aesthetics and maintenance perceptions: assessing the relationship between homeowners’ visual attention and landscape care knowledge. Land Use Policy 95:104645. doi: 10.1016/j.landusepol.2020.104645
Kurdoglu, O., and Kurdoglu, B. C. (2010). Determining recreational, scenic, and historical–cultural potentials of landscape features along a segment of the ancient silk road using factor analyzing. Environ. Monit. Assess. 170, 99–116. doi: 10.1007/s10661-009-1219-8
Li, Y., Gao, X. H., Chen, H. P., et al. (2020). A review of tourists’ behavior based on visual perception Xiamen University.
Li, C., and Huang, X. H. (2022). Differences in visual attraction between historical garden and urban park walking scenes. Land. 11:1766. doi: 10.3390/land11101766
Liu, S., Wu, S. C., Wang, H., Zhang, J., Li, J. J., Wang, C. L., et al. (2014). The stand spatial model and pattern based on voronoi diagram. Acta Ecol. Sin. 34, 1436–1443. doi: 10.5846/stxb201210131416
Liu, Q. Y., Wu, Y., Xiao, Y. H., Huang, Q. T., and Lan, S. R. (2019). The inherent psychological mechanism of perceived restoration of urban parks—an perspective from environmental preference and place attachment theory. Chin Landscape Architec 35, 39–44. doi: 10.19775/j.cla.2019.06.0039
Lothian, A. (1999). Landscape and the philosophy of aesthetics: is landscape quality inherent in the landscape or in the eye of the beholder? Landscape and urban planning. Landsc. Urban Plan. 44, 177–198. doi: 10.1016/S0169-2046(99)00019-5
Oku, H., and Fukamachi, K. (2006). The differences in scenic perception of forest visitors through their attributes and recreational activity. Landsc. Urban Plan. 75, 34–42. doi: 10.1016/j.landurbplan.2004.10.008
Palmer, J. F. (2004). Using spatial metrics to predict scenic perception in a changing landscape: Dennis, Massachusetts. Landsc. Urban Plan. 69, 201–218. doi: 10.1016/j.landurbplan.2003.08.010
Wilkes-Allemann, J., Pütz, M., and Hanewinkel, M. (2017). Forest recreation as a governance problem: four case studies from Switzerland. Eur. J. For. Res. 136, 511–526. doi: 10.1007/s10342-017-1049-0
Qin, X. W., Zhang, S. Q., Li, X. F., Na, X. D., and Liu, C. Y. (2008). Spatial pattern of red-crowned crane nest-sites based on Voronoi diagram. Chin J Ecol. 27, 2118–2122.
Sang Ode, Å., Tveit, M. S., Pihel, J., and Hägerhäll, C. M. (2016). Identifying cues for monitoring stewardship in Swedish pasture landscapes. Land Use Policy 53, 20–26. doi: 10.1016/j.landusepol.2015.09.020
Shi, Y., Zhang, J., Shen, X., Chen, L., Xu, Y., Fu, R., et al. (2022). Designing perennial landscapes: plant form and species richness influence the gaze perception associated with aesthetic preference. Land. 11:1860. doi: 10.3390/land11101860
Sun, L., Shao, H., Li, S. Y., Huang, X., and Yang, W. (2018). Integrated application of eye movement analysis and beauty estimation in the visual landscape quality estimation of urban waterfront park. Int. J. Patt. Recogn. Artif. Intell. 32:1856010. doi: 10.1142/S0218001418560104
Tabrizian, P., Baran, P. K., Smith, W. R., and Meentemeyer, R. K. (2018). Exploring perceived restoration potential of urban green enclosure through immersive virtual environments. J. Environ. Psychol. 55, 99–109. doi: 10.1016/j.jenvp.2018.01.001
Wang, M., Wang, Y. X., Huang, H. Y., and Yinsheng, T. (2018). A visual analysis on the urban open spaces: with an eye-tracking experiment on the case study of the Flower City square, Guangzhou. Tropic Geogr. 38, 741–750. doi: 10.13284/j.cnki.rddl.003081
Wang, X. B., Zhang, L., and Shi, Y. X. (2022). Interpretation of Forest therapy from the perspective of healing landscape. In: Proceedings of 2017 5th international conference on physical education and society management(ICPESM 2017)V71.Ed. Singapore management and sports science institute, 2017, 326–330.
Wang, R. H., Zhao, J. W., and Liu, Z. Y. (2016). Consensus in visual preferences: the effects of aesthetic quality and landscape types. Urban For. Urban Green. 20, 210–217. doi: 10.1016/j.ufug.2016.09.005
White, M. P., Cracknell, D., Corcoran, A., Jenkinson, G., and Depledge, M. H. (2014). Do preferences for waterscapes persist in inclement weather and extend to sub-aquatic scenes? Landsc. Res. 39, 339–358. doi: 10.1080/01426397.2012.759919
Wu, J. Z., Zhong, Y. D., and Deng, J. Y. (2019). Assessing and mapping forest landscape quality in China. J. For. 10:648. doi: 10.3390/f10080684
Wu, Y., Zhuo, Z., Liu, Q., Yu, K., Huang, Q., and Liu, J. (2021). The relationships between perceived design intensity, preference, restorativeness and eye movements in designed urban green space. Int. J. Environ. Res. Public Health 18:10944. doi: 10.3390/ijerph182010944
Xu, W., Zhao, J., Huang, Y., and Hu, B. (2018). Design intensities in relation to visual aesthetic preference. Urban For. Urban Green. 34, 305–310. doi: 10.1016/j.ufug.2018.07.011
Zadegan, Y. R., Behe, B. K., and Gough, R. (2008). Consumer preferences for native plants in Montana residential landscapes and perceptions for naturalistic designs. J. Environ. Hortic. 26, 109–114. doi: 10.24266/0738-2898-26.2.109
Zhang, Z., Gao, Y., Zhou, S., Zhang, T., Zhang, W., and Meng, H. (2022). Psychological cognitive factors affecting visual behavior and satisfaction preference for forest recreation space. Forests 13:136. doi: 10.3390/f13020136
Zhang, Z., Qie, G. F., Wang, C., Jiang, S., Li, X., and Li, M. (2017b). Relationship between Forest color characteristics and scenic beauty: case study analyzing pictures of mountainous forests at sloped positions in Jiuzhai Valley, China. Forests 8:63. doi: 10.3390/f8030063
Zhang, Z., Qie, G. F., Wang, C., Jiang, S. S., Li, X., Li, M. X., et al. (2017a). Application of eye-tracking assistive technology in forest landscape evaluation. J. World Forestry Res. 30, 19–23. doi: 10.13348/j.cnki.sjlyyj.2017.0043
Zhang, C., Wang, H., and Wang, C. (2020). Visual quality evaluation and distance change analysis of urban forest landscape based on eye movement. J Chin Urban Forest. 18, 6–12.
Zhang, G. C., Yang, J., Wu, G. W., and Hu, X. (2021). Exploring the interactive influence on landscape preference from multiple visual attributes: openness, richness, order, and depth. Urban For. Urban Green. 65:127363. doi: 10.1016/j.ufug.2021.127363
Zheng, J., Huang, Y., Chen, Y., Guan, L., and Liu, Q. (2022). Subjective preference and visual attention to the attributes of ornamental plants in urban green space: an eye-tracking study. Forests 13:1871. doi: 10.3390/f13111871
Keywords: forest landscape, landscape uniformity, visual behavior, spatial cognitive characteristic, re-viewing intention
Citation: Gao Y, Sun X, Zhang Z, Zhang W, Meng H and Zhang T (2024) How does the landscape uniformity in different forest landscapes affect the visual behavior and preference evaluation intention of participants—a case study of forest landscape in northern China (Liaoning). Front. For. Glob. Change. 6:1243649. doi: 10.3389/ffgc.2023.1243649
Received: 21 June 2023; Accepted: 04 December 2023;
Published: 21 March 2024.
Edited by:
Zhonghua Gou, Wuhan University, ChinaCopyright © 2024 Gao, Sun, Zhang, Zhang, Meng and Zhang. This is an open-access article distributed under the terms of the Creative Commons Attribution License (CC BY). The use, distribution or reproduction in other forums is permitted, provided the original author(s) and the copyright owner(s) are credited and that the original publication in this journal is cited, in accordance with accepted academic practice. No use, distribution or reproduction is permitted which does not comply with these terms.
*Correspondence: Tong Zhang, dG9uZ3poYW5nQHN5YXUuZWR1LmNu
Disclaimer: All claims expressed in this article are solely those of the authors and do not necessarily represent those of their affiliated organizations, or those of the publisher, the editors and the reviewers. Any product that may be evaluated in this article or claim that may be made by its manufacturer is not guaranteed or endorsed by the publisher.
Research integrity at Frontiers
Learn more about the work of our research integrity team to safeguard the quality of each article we publish.