- 1National Research Council of Italy, Institute of BioEconomy (CNR IBE), Sassari, Italy
- 2Department of Agricultural and Forest Sciences and Engineering, University of Lleida, Lleida, Spain
- 3Faculty of Agriculture and Natural Resources, University of Mohaghegh Ardabili, Ardabil, Iran
The growing threats posed by wildfires in Southern Europe are calling for the development of comprehensive and sound management and risk assessment strategies. In this work, we present the application of wildfire simulation modeling based on the minimum travel time (MTT) algorithm to assess fine-scale (100-m resolution) wildfire hazard, transmission, and exposure to communities in the Italy-France Maritime cooperation area (Sardinia, Corsica, Tuscany, Liguria and Provence-Alpes-Côte d’Azur), which cover about 72,000 km2 of land. We simulated thousands of wildfires considering the current landscape and characterized and measured fine-scale wildfire risk factors and profiles by taking into account historical fire regimes, fuels, winds and fuel moisture conditions associated with the occurrence of the largest wildfires (>100 ha) that affected the study area in the last 20 years. Individual fires were simulated at 100-m resolution, consistent with the input files. Modeled annual burn probability and ignition probability revealed that Sardinia was the Region most affected by wildfires. The wildfire simulation outputs were then combined with main land uses and building footprint locations to characterize wildfire transmission and exposure to communities, and were summarized for main vegetation types and Regions. This study presents a cross-boundary and standardized approach based on wildfire spread modeling to analyze and quantify wildfire risk profiles in Southern Europe. The stochastic wildfire modeling systems we implemented used harmonized sets of data for a vast, fire-prone Mediterranean area, where previous similar studies were conducted at coarser resolutions and covered lower extent of lands. The approach presented in this work can be used as a reference pillar for the development and implementation of a common wildfire risk monitoring, management, and governance plan in the study area. The methods and findings of this study can be replicated in neighboring Mediterranean and other regions threatened by wildfires.
Introduction
Wildfires are a growing and significant threat to ecological, social, and economic values in the Euro-Mediterranean region, as they can cause relevant damages to natural values, ecosystem services, and human activities, and even loss of lives (Keeley et al., 2012; Molina-Terrén et al., 2019; Forzieri et al., 2021). More than 80% of the annual area burned in Europe is typically concentrated in Southern countries, which are characterized by vast portions of fire-prone lands that, under extreme environmental conditions, are able to sustain the propagation of fast-spreading, large and severe wildfires (San-Miguel-Ayanz et al., 2016; FAO and Plan Bleu, 2018; Galizia et al., 2022). According to San-Miguel-Ayanz et al. (2023), in the last 6 years the EU territory faced 3 of the worst wildfire seasons in terms of area burned of its history. In fact, a number of converging driving factors have promoted variations in wildfire regime and risk: these drivers include fuel buildup and land abandonment, climate-induced drought, high wind intensity events, and WUI expansion into fire-prone areas (Marlon et al., 2008; Moreira et al., 2011; Pausas and Fernández-Muñoz, 2012; Fernandes et al., 2014; Viedma et al., 2018; Turco et al., 2019; Pereira et al., 2020; Ruffault et al., 2020; Ganteaume et al., 2021).
The growing incidence of wildfires and related losses calls for strong actions to analyze and map wildfire hazard, transmission, and exposure, and to propose solutions for mitigating risks (Calkin et al., 2014; Ager et al., 2019; Alcasena et al., 2019). Nevertheless, accounting for the risks posed by large wildfires involves adequate consideration of their propagation and behavior potential in complex terrain and large landscapes, while taking into account several factors such as ignition patterns and fire regime, slope, fuel properties and moisture, historical weather and winds (Ager et al., 2011). In this paper, we will adopt the definitions of wildfire hazard (danger), exposure and risk of Chuvieco et al. (2023), and the definition of transmission of Ager et al. (2014).
Nowadays, a large number of wildfire spread and behavior models and simulators are available (Finney, 2004, 2006; Tolhurst et al., 2008; Balbi et al., 2009; Tymstra et al., 2010; Andrews, 2014; Kalabokidis et al., 2016; Arca et al., 2019; Monedero et al., 2019; Trucchia et al., 2020). Promising results to assess wildfire risk, exposure, and transmission potential have been obtained by new wildfire spread models based on Monte Carlo methods (Scott et al., 2018; Palaiologou et al., 2022). In this context, several burn probability modeling approaches are based on the MTT algorithm (Finney, 2002), a compact fire simulation algorithm that allows to simulate thousands of wildfires over large areas and is parallelized for multi-threaded processing. The MTT fire behavior library is also integrated in a number of software and command line applications, as for instance FlamMap (Finney, 2006), FconstMTT (Ager et al., 2017) or FSim (Finney et al., 2011). The application of the MTT algorithm and the abovementioned systems has emerged as a useful tool for a wide range of applications in Southern Europe, including fire hazard evaluation and mapping (Mitsopoulos et al., 2015; Alcasena et al., 2019; Sá et al., 2022), trade-off analysis among management strategies (Palaiologou et al., 2020; Benali et al., 2021; Aparício et al., 2022), fire management potential (Alcasena et al., 2019; Castellnou et al., 2019), impacts on communities and values of interest (Alcasena et al., 2015, 2021; Mallinis et al., 2016; Palaiologou et al., 2022), or post-fire disturbances (Salis et al., 2019). Even if the modeling approaches based on the MTT were developed and mostly tested in North America and Southern Europe (Miller and Ager, 2013; Parisien et al., 2020), various successful applications can be also found in a range of other regions and ecosystem types (Gomez et al., 2015; Jahdi et al., 2020; Galizia et al., 2021; Oliveira et al., 2021).
Several studies highlighted the need for systematic and standardized large-scale evaluations to map potential cross-boundary wildfire hazard and risk, with the final aim to target and prioritize the most critical areas where specific cross-boundary planning and management activities are necessary (Palaiologou et al., 2018; Ager et al., 2019; Thompson et al., 2020). This was one of the main objectives of the cluster of wildfire projects [a strategic project (MED-Star) and four associated projects (MED-PSS, MEDCoopFire, MEDForeste, InterMED)] recently financed by the Interreg Italy-France Maritime Programme (EU IFM, 2023), funded by the European Regional Development Fund (ERDF) and aimed at promoting territorial cooperation in the EU. The IFM Programme assists in facilitating and supporting cooperation projects between Italy, specifically the territories of Sardinia, Tuscany, and Liguria, and France, specifically Corsica and the Provence-Alpes-Côte d’Azur areas. This cooperation area shares a wealth of cultural, social, and economic values, as well as comparable environmental conditions and disturbances. Among these disturbances, wildfires are one of the most significant disruptive factors: each year, particularly in the summer season, the IFM area is affected by wildfires that threaten several anthropogenic and ecological values, as well as human lives. In this context, the EU IFM Programme has promoted a collaborative effort among regional institutions, research agencies, and policymakers aiming to develop strategies that implement operational and shared solutions for wildfire risk assessment and prevention.
To support the implementation of cross-boundary wildfire risk assessment and large-fire scenario analysis, we built on previous work carried out in Sardinia and applied wildfire simulation modeling based on the minimum travel time (MTT) algorithm. The goal of this study is to assess fine-scale (100-m resolution) current patterns of wildfire exposure, transmission, and exposure to communities in the IFM cooperation area (about 72,000 km2 of land). In order to assess wildfire risk profiles for the whole study area, we simulated thousands of large wildfire events by holding the current landscape, replicating the weather and fuel moisture conditions associated with fire events above 100 ha and running individual fires at 100-m resolution, consistent with the input files. This study presents a cross-boundary and standardized approach based on wildfire spread modeling to analyze and quantify wildfire hazard, transmission and exposure profiles in a relevant fire-prone area of Southern Europe. The wildfire modeling approach presented in this work supports the development of strategic decision support tools that can be used as a reference pillar for the definition and implementation of a common wildfire risk monitoring, management, and governance plan in the IFM area: for instance, wildfire hazard and exposure outputs can guide the coordinated design of landscape fuel treatment projects devoted to community protection or highly exposed forests and natural values. Furthermore, the methods and findings of this study are extendable and can be replicated in neighboring Mediterranean areas and other fire-prone regions.
Methods
Study area
The study area, extending approximately 72,000 km2 of land, is located between 39°00′ and 45°00′ N latitude and 5°00′ and 12°00′ E longitude (Figure 1). This encompasses the Italy-France Maritime (IFM) cooperation area, which involves four regions: Sardinia, Tuscany, and Liguria in Italy, and Corsica in France. Furthermore, it includes the French Departments of Var and Alpes-Maritimes, located within the region known as Provence-Alpes-Côte d’Azur, or PACA. Approximately 9 million people reside in this area, with a significant proportion living near the coastlines and major towns. During the summer, these coastal regions witness a substantial influx of tourists, leading to a considerable increase in the resident population. Overall, the average terrain elevation is approximately 550 m above sea level (a.s.l.), with a range from 0 to 3,040 m a.s.l. (Figure 1A). The highest peaks are found in the Alps, which broadly separate France from Italy. The primary plains are situated in Tuscany and Sardinia, whereas Liguria and Corsica exhibit a minimal presence of flat areas. Climate conditions fluctuate based on latitude, elevation, and distance from the Mediterranean Sea. This leads to several distinctions in climatic and weather conditions both among and within regions. In fact, the average annual temperatures range from about −6°C in the highest mountains to approximately 17°C in the warmest coastal areas of Sardinia, Corsica, and Tuscany. The average annual precipitation varies between roughly 400 mm and about 2,300 mm (Table 1).
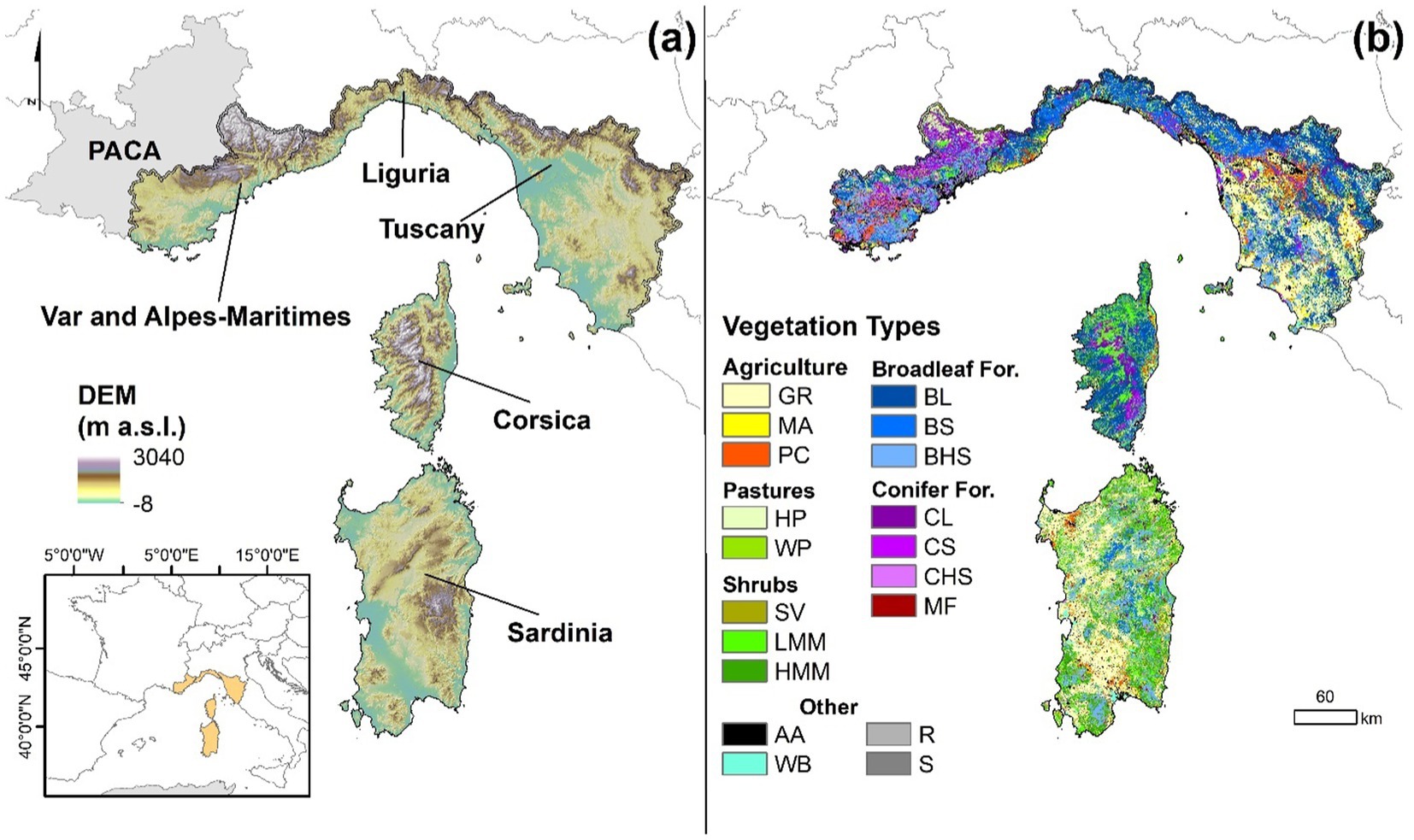
Figure 1. Digital Elevation Model (DEM) of Italy-France Maritime cooperation area (A), which includes four Regions (Sardinia, Tuscany, and Liguria, in Italy, and Corsica, in France) and two French Departments (Var and Alpes-Maritimes, located in the Provence-Alpes-Côte d’Azur (PACA) Region), and vegetation types of the study area (B). GR, grasslands; MA, mixed agricultural areas; PC, permanent crops; HP, herbaceous pastures; WP, wooded pastures; SV, sparse vegetation; LMM, low Mediterranean maquis; HMM, high Mediterranean maquis; BL, broadleaf with litter understory; BS, broadleaf with shrub understory; BHS, broadleaf with high shrub understory; CL, conifer with litter understory; CS, conifer with shrub understory; CHS, conifer with high shrub understory; MF, mixed forests; AA, anthropic areas; WB, water bodies; R, rocks; S, sands.
To derive a common map of vegetation types for the study area, we used as inputs the regional forest type geodatasets of four Regions [Corsica and PACA, (IGN, 2023), Liguria, (Agriligurianet, 2023); Tuscany, (GEOscopio, 2023)]. Apart from some differences in the forest classifications, all geodatasets provided spatial information on the main forest species and canopy cover (Supplementary Figure 1). These data were combined with the Corine Land Cover 2012 (EEA, 2017) to characterize non-forested areas. Regarding Sardinia, due to the absence of an updated forest-type map, we used the Sardinia Land Use Map (Sardegna Geoportale, 2023) and the Sardinia Bioclimatic map (Canu et al., 2015). Then, we involved regional stakeholders and experts with meetings, surveys and questionnaires in order to characterize other properties of the main forest species [i.e., type of understory (litter; shrubs; high shrubs); canopy characteristics (e.g., stand height, base height); fire behavior] that were not included in the forest datasets (Supplementary Figures 1, 2). Afterwards, we incorporated in the regional datasets the information obtained from the regional experts and we assembled a common and standardized spatial geodatabase covering the whole study area. We also summarized the different vegetation classes into a set of 19 standardized vegetation types, organized into 6 main vegetation types (Agriculture; Pastures; Shrubs; Broadleaf Forests; Conifer Forests; Other) (Figure 1B). We finally converted the geodatabase in a 100-m vegetation type raster map of the IFM cooperation area (Figure 1B, Supplementary Figure 1, and Supplementary Table 1). The most relevant vegetation types of the study area (Table 1 and Figure 1B) are represented by broadleaf forests (~36%) [mostly Quercus spp. (Q. ilex L., Q. pubescens Willd., Q. cerris L., Q. robur L., Q. suber L.), Castanea sativa Mill., Fagus sylvatica L., Ostrya carpinifolia Scop.], Mediterranean maquis (~16%), grasslands (~15%), and conifer forests (~8%) [mainly Pinus spp. (P. pinaster Aiton, P. nigra J.F.Arnold, P. sylvestris L., P. pinea L., P. halepensis Mill.)]. Broadleaf forests are the dominant forest type of the IFM Regions except for Sardinia, where Mediterranean maquis is the most significant vegetation type in the wildlands. Broadleaves are particularly relevant in Corsica and Liguria, where they account for 60 and 50% of the total regional area, respectively. In the PACA Departments, also conifer forests occupy vast areas (about 30% of the total area). Mediterranean maquis covers about 30% of Corsica and Sardinia islands, while on the contrary the lowest values can be found in Tuscany (~5%).
Wildfire regime and data
To characterize wildfires in the study area, we used the 1998–2019 Promethée database for the French regions (PROMÉTHÉE 2, 2023) and the database provided by each Italian Region (1998–2019 for both Sardinia and Tuscany; 1998–2016 for Liguria). Excluding wildfires with a size lower than 1 ha, the study area was affected by about 34,000 wildfires (0.022 fire ignitions km−2 year−1): approximately 2/3 of the ignitions were in Sardinia. Regarding the area burned (AB), in the study period about 600,000 hectares of land were burned, with an annual average of 0.38 ha km−2 and an evident peak in Sardinia, which accounted for about 62% of the total AB in the study area (Table 2). In more detail, two Regions, PACA, and Tuscany, showed relatively low levels of average annual AB, with 0.21 and 0.08 ha km−2, respectively, while higher values were presented by Sardinia, Liguria, and Corsica (0.70, 0.48, and 0.46 ha km−2, respectively). Overall, large wildfires were the main source of risk in the study area: about 60% of the total AB was due to a limited number of events (2.5% over the total fire ignitions) larger than 100 ha. In this regard, the incidence of large wildfires in terms of the total AB ranged from a minimum of 34.2% in Tuscany to little less than 80% in Corsica and the two PACA Departments (Table 2). Only about 0.5% of the total fire number in the IFM area originated fires with size above 500 ha, which accounted for about 36% of the total AB, with peaks in Corsica and PACA Departments (≈60% of the total AB) and the lowest relevance in Tuscany (≈9% of the total AB). Wildfires were largely concentrated in the May–October months, which accounted for about 90% of the total AB in the study area; however, Liguria and Alpine areas also presented significant wildfires in late fall and winter periods.
Input data
We used Arcfuels (Ager et al., 2011; Vaillant et al., 2013) to assemble and process, for the whole modeling domain, spatial data on topography and fuels and produce a 100-m resolution gridded landscape file, as required by FlamMap (Finney, 2006). The wildfire modeling domain (approximately 74,500 km2) covered the study area and also included a 2-km buffer around PACA Departments, Liguria, and Toscana to take into account incoming fires from neighboring areas and remove the “edge effect” (Palaiologou et al., 2022). Topography layers were obtained from the 25-m digital elevation data of Europe (EEA, 2017). Regarding fuels, starting from the standardized geodatabase described in the previous section, we attributed to each vegetation type and/or subtype (e.g., considering deciduousness, species, understory type) a specific surface fuel model, either standard (Anderson, 1982; Scott and Burgan, 2005) or customized (Arca et al., 2009; Duce et al., 2012; Ascoli et al., 2020; Supplementary Figure 1; Figure 2A; Table 3; Supplementary Table 2).
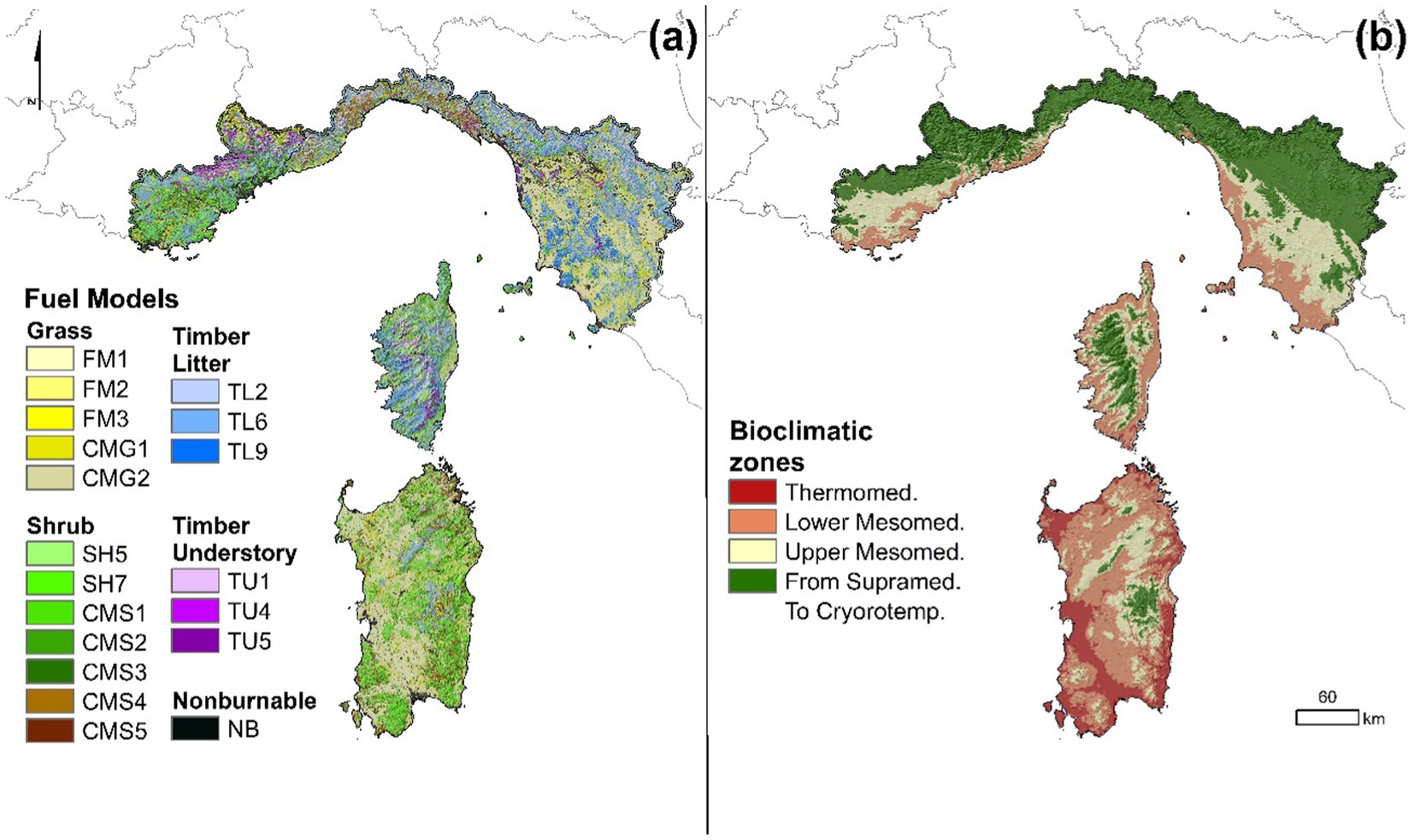
Figure 2. Surface fuel model map of the study area (A) (fuel model codes, parameters, and incidence at the Regional and study area level are provided in Table 3); bioclimatic map of the study area (B) [classes derived from Pesaresi et al. (2014)].
Dead and live fuel moisture content (FMC) was set focusing on the driest periods of the summer season and using as reference moisture data collected in previous studies and projects carried out in southern Europe (Pellizzaro et al., 2007; Salis et al., 2015; Martin-StPaul et al., 2018; Yebra et al., 2019; Pellizzaro, personal communication; Massaiu, personal communication). To account for FMC differences among and within Regions, we split the study area into four homogeneous bioclimatic zones, as presented in Figure 2B. The above four bioclimatic zones were determined starting from the spatial datasets of bioclimatic conditions of Pesaresi et al. (2014). In more detail, according to the abovementioned measured data, the dead FMC values ranged from the low moisture scenario of Scott and Burgan (2005), which was adopted in the areas characterized by the hottest bioclimatic conditions, to the moderate moisture scenario of Scott and Burgan (2005), adopted in the coldest bioclimatic contexts. Intermediate dead FMC values were selected in the remaining portions of the study area. Regarding live FMC, values ranged from 75 to 90% in timber understory and mature shrub fuel models, and from 50 to 65% in degraded shrubs. Also, in this case, we adopted a live FMC gradient moving from hot bioclimatic zones to cold areas and mountains based on observed data.
Dominant wind directions for wildfire simulations were set considering the wildfires larger than 200 ha (Table 4) and gathering the main wind direction observed in the weather station closest to each large wildfire on the day of the fire event. Wind speed was set to 35 km h−1 and remained constant during each wildfire simulation and for all wind direction scenarios.
A smoothed annual fire ignition probability grid was determined taking into account all wildfire ignitions with size larger than or equal to 1 ha, as reported in the historical databases, considering the reference study period. The ignition probability grid was created with ArcGIS 10.8 (Esri Inc.) using the Point Density method with a 10-km bandwidth. The above radius was the minimum value that would generate a nearly continuous map of fire ignition probabilities for the whole study area. Fire ignition probability grids derived from historical patterns, rather than the application of randomly assigned ignitions, allowed to replicate recent observed fire occurrence and to adequately take into account current hot-spot zones in the IFM study area, as well as to guarantee a minimum ignition likelihood in those remote areas that did not experience fires during the study period. Furthermore, to derive the probability grids, we did not restrict our selection only to ignitions that originated fires above 100 ha: this would have substantially reduced the sample size and would have caused the definition of coarse ignition probability grids (>30 km bandwidth) to saturate the landscape and avoid the exclusion of simulations in those areas that did not record large fires in their vicinity, particularly in Tuscany and PACA Departments. The ignition probability grid was held as constant input for all wildfire simulations.
Wildfire simulation modeling
Wildfire simulations were performed by using the minimum travel time (MTT) algorithm, as implemented in the “RANDIG” command-line version (Finney, 2002). The MTT algorithm calculates a two-dimensional fire growth, while the weather is held constant, following the Huygens’ principle, by searching for the pathways with minimum spread time from nodes or cell corners at a given resolution set by the user (Finney, 2002). The MTT is implemented to simulate thousands of potential wildfire ignitions and several fuel moisture and wind conditions, but cannot consider vegetation succession or fire suppression efforts. This algorithm has been widely used in previous research and studies aimed at assessing wildfire exposure and transmission and evaluating fire risk mitigation strategies over large and complex landscapes (Palaiologou et al., 2019; Parisien et al., 2020; Alcasena et al., 2021). Fire spread is predicted by the surface fire spread model of Rothermel (1972), fire intensity is converted to flame length using the equation of Byram (1959), and crown fire initiation is predicted through Van Wagner (1977) methods as implemented by Scott and Reinhardt (2001).
Fire ignition points were distributed within the burnable fuels of the modeling domain, according to the ignition probability grid originated from the historical fire data. Then, each fire was independently modeled randomly drawing from the frequency distribution of wind directions of the Region where the ignition was located. Overall, 145,000 fire events burning under extreme weather were simulated at 100 m resolution, consistent with the input data resolution, with constant fuel moisture and wind intensity (35 kmh−1), and a burning period of 480 min; fire suppression was not considered. The total simulated area burned by the wildfire simulations was close to 150 million hectares, which in the IFM cooperation area correspond to the area burned by wildfires in about 5,300 fire seasons.
Wildfire hazard, transmission, and exposure analysis
For the whole modeling domain, the wildfire simulations generated burn probability (BP) rasters, frequency distributions of flame lengths (FL) in twenty 0.5-m intervals for each pixel, fire size (FS) list, and simulated wildfire perimeter polygons. The annual burn probability (aBP) represents the annual likelihood of burning at each specific pixel given the current modeling domain, landscape characteristics and extreme weather conditions; it is calculated as the ratio of the number of times a given pixel burned to the modeled fire seasons (i.e., 5,300 in our study). aBP can range from 0 (the pixel never burns) to 1 (the pixel burns every year). The simulated average aBP was then summarized at the regional scale and for the whole study area by calculating the average value from the aBP raster file, for each Region and for the whole IFM area.
The fire size list, which includes the simulated fire ignition coordinates, allowed us to characterize the cumulative simulated ignition point densities (IPd) in the modeling domain and to compare this output with the historical data: the IPd was quantified in terms of annual number of events per km2, and was therefore indicated as aIPd (annual ignition point density). From the flame length distribution outputs, we derived the maximum flame length (FLM) of a surface fire burning a pixel.
Wildfire transmission for the modeling domain was first of all quantified using the wildfire source-sink ratio (SSR), which is the ratio, on a logarithmic scale, between average simulated FS and average simulated aBP: in relative terms, the cells with high burn probability but unable to generate large fires from an ignition act as wildfire sinks, while wildfire sources are those cells that have low burn probability but can generate large fires when an ignition occurs (Ager et al., 2012). In addition, following the methods proposed by Ager et al. (2014) and Palaiologou et al. (2019), wildfire transmission was estimated by intersecting simulated wildfire perimeters and ignition points with a hexnet of 1,000-ha hexcells (n = 8,050) for the whole IFM area and with vegetation type polygons (Figure 1B); we then assigned the wildfire origin (i.e., source hexcell or vegetation type) based on the ignition location. We then cross-tabulated the total area burned (AB) in each of the resulting intersected perimeter fragments, which were divided into (1) incoming fire (i.e., AB in a given vegetation type from a fire ignited outside of that vegetation type), (2) outgoing fire (i.e., AB outside the vegetation type where the fire was ignited), and (3) self-burning (i.e., a fire ignited in a vegetation type and entirely burned within it). This analysis allowed us to map wildfire transmission for the study area and to quantify the role played by each hexcell and vegetation type, and at the regional level, in terms of annual wildfire transmitting potential, in hectares per year, and of percentage of transmission types.
Finally, we estimated the structures threatened by wildfires in the study area. For this purpose, we used the Open Street Map (OSM) buildings shapefiles (2022). This structure footprint database provided accurate locations and contained all structures (approximately 4.1 million footprints) in the whole study area including residential housing, commercial buildings, farms, large stores, industrial buildings, and religious structures. We first derived the building centroids from each building polygon, which was classified at the municipal level. Then, we intersected building centroids and polygons with all wildfire perimeters simulated in the modeling domain. This allowed us to assess the structures threatened by wildfires in terms of the average annual number of threatened structures and relative terms (i.e., the annual number of threatened buildings in the unit area over the total number of buildings in that unit area), and to quantify the structure exposure to wildfires at the regional and provincial levels. To summarize and provide maps of wildfire threatened structures for the whole IFM area, we used the hexnet of 1,000-ha hexcells.
Results
Wildfire hazard
The output data and maps for annual burn probability (aBP) and annual ignition point density (aIPD) are presented in Table 5 and Figures 3A,B and showed specific patterns across the study area. Not surprisingly, aBP and aIPD outputs confirmed that Sardinia is by far the area most threatened by wildfires in terms of both probability of burning and probability of having ignitions. Conversely, Tuscany was the less affected Region, with the lowest mean values of aBP and aIPD.
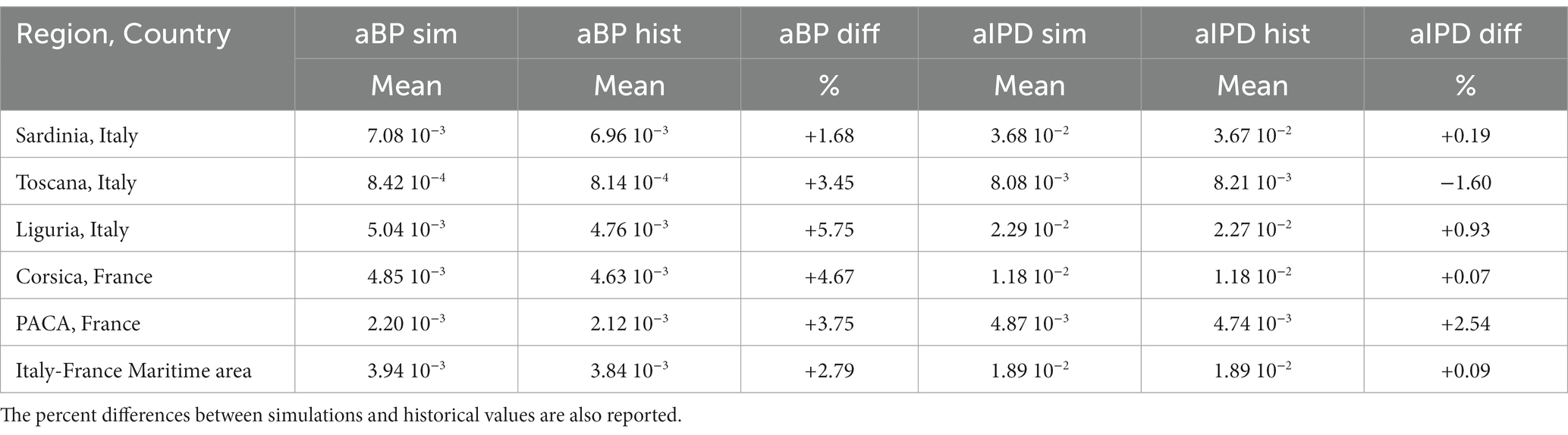
Table 5. Mean values of simulated and historical annual burn probability (aBP) and annual ignition point density (aIPD) at the regional level and for the whole study area.
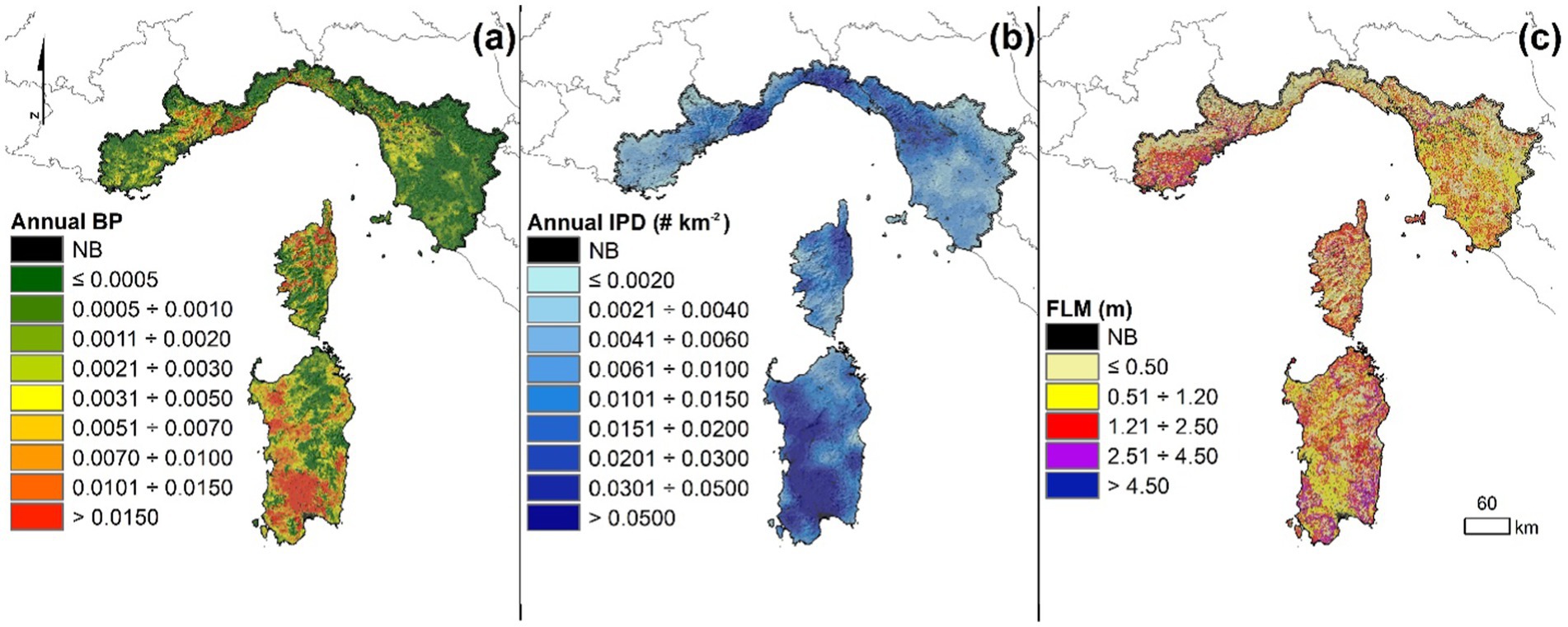
Figure 3. Maps of annual burn probability (aBP) (A), annual fire ignition point density (aIPD) (B), and maximum flame length (FLM) (C), which is the maximum flame length of a surface fire in a given pixel, of the Italy-France Maritime cooperation area, at 100-m resolution, derived from the combination of wildfire simulations, as described in the Methods.
In more detail, excluding non-burnable fuels, wildfire simulations for the study area generated a mean aBP value of 3.94 10−3, which corresponds to about 28,200 hectares burned per year and is slightly higher than the historical value (about 27,500 ha yr−1) observed in the study period. aBP ranged from about 0–1.31 10−1, with the highest peaks (aBP > 0.1) concentrated in small areas of central-southern Sardinia and of the province of Imperia, in Liguria. This output revealed large spatial variations within the study area and each Region (Figure 3A and Table 5). At the regional level, Sardinia presented the highest mean aBP (7.08 10−3), which is almost double than the mean value obtained for the IFM cooperation area. Liguria and Corsica also showed relevant mean aBP values (4.76 10−3 and 4.63 10−3, respectively), which are slightly higher than the mean value for the study area. By contrary, the lowest mean aBP values were observed in PACA Departments (2.12 10−3) and primarily in Tuscany (8.14 10−4, about 4.5 times lower than the mean value of the study area). About 11% of the IFM area exhibited aBP higher than 1.00 10−2 (Figure 3A), with the most evident hot spot areas observed in Sardinia, Corsica, and Liguria (with 22.5, 14.5, and 12.1% of the regional area characterized by aBP ≥ 1.00 10−2, respectively); the incidence of high aBP areas was much lower in PACA Departments and Tuscany (about 4.0 and 0.4% of the regional area, respectively). On the contrary, about 52% of the study area presented mild aBP conditions, with values below 1.0 10−3, even if large variations can be observed among and within Regions. Again, Tuscany and PACA showed vast areas with low aBP (about 75.1 and 62.8% of the regional area, respectively); conversely, the regional area with a low probability of fire occurrence and spread was approximately 30.2, 45.3, and 51.9% in Sardinia, Corsica, and Liguria, respectively. As a result, the estimated annual AB ranged from a maximum of about 17,100 ha in Sardinia, which accounted for about 60.5% of the annual AB in the study area, to a minimum of about 1,940 ha in Tuscany. The annual AB at the regional level from our simulations was moderately overestimated in all Regions with respect to the observed values: the highest overestimations were observed in Liguria (about +5.8%) and Corsica (+4.7%).
Even if the simulated aBP values were close to the observed data at the regional scale and for the whole study area, we noticed some significant differences between historical and simulated values at the local and province/department levels. The most relevant underestimation of aBP was observed in northern Sardinia, where the annual area burned by our simulations was about 1,400 and 1,700 ha lower than that observed during the study period in Sassari and Nuoro provinces, respectively; on the contrary, a large overestimation was observed in the Sud Sardegna province (about +2,500 ha burned per year). Observed and simulated aBP in Liguria and the PACA Departments also presented some important differences: we overestimated aBP in the Imperia province (1.41 10−2 vs. 7.91 10−3) and in the Alpes-Maritimes Department (3.64 10−3 vs. 1.31 10−3), while we underestimated aBP in the Var Department (1.17 10−3 vs. 2.69 10−3). Less significant differences between historical and simulated aBP were observed in Corsica and Tuscany provinces.
Focusing on fire events with size not lower than 1 ha, the mean simulated aIPd for the study area was about 1.89 10−2 ignitions km−2 (Table 5), which corresponds to the historical value observed in the period under investigation. Overall, the aIPd values of the whole IFM area ranged from 0 to about 0.27 ignitions km−2, while the historical data ranged from 0 to about 0.26 ignitions km−2. With respect to the historical values, our simulations presented a slight underestimation of aIPD in Tuscany (−1.60%), while the most relevant overestimation was observed in the two PACA Departments (+2.54%). The modeling outputs highlighted that the highest concentration of annual wildfire ignitions (>0.03 fires km−2) was in specific hot-spot areas, mostly located in Sardinia, Liguria, and Corsica, with about 37.1, 26.8, and 5.1% of the regional territory characterized by high likelihood of fire ignitions, respectively (Figure 3B). This pattern is confirmed also in terms of mean aIPD at the regional scale: Sardinia exhibited the highest mean aIPD, which was about 3.68 10−2 ignitions km−2 and preceded Liguria, with approximately 2.29 ignitions km−2. As a matter of fact, about 45% of the simulated fire ignitions in the study area were distributed in Sardinia. The other Regions (Corsica, PACA, and Tuscany) presented mean aIPD lower than the mean value of the study area, with the lowest values observed in Tuscany and PACA (8.08 10−3 and 4.87 10−3 ignitions km−2, respectively). The smallest probabilities of fire ignitions were primarily observed in PACA and Tuscany, which presented about 29.4 and 45.4% of the regional territory characterized by aIPD below 4.00 10−3 fires km−2, respectively.
The wildfire simulations produced values of maximum flame length (FLM) in the range 0–9.75 m. Considering the whole study area, a significant percentage of lands (about 66% of the total area) showed FLM values below 1.20 m, particularly in the most managed and productive agricultural zones and in the coldest bioclimatic conditions (Figure 3C). The Regions with the highest incidence of low FLM levels were Liguria and Tuscany. On the other hand, about 6.5% of the IFM territory exhibited the potential to originate high-intensity surface fires, especially in areas where the presence of high or mature shrubs is more prominent. The Regions with FLM greater than 2.50 m were mainly located in Sardinia, particularly in the eastern belt, in the southwestern part of the PACA Departments, and in the coastal areas of Corsica (Figure 3C).
Wildfire transmission
We then examined the wildfire transmission for the study area and started with the analysis of the source-sink ratio (SSR). About 31% of the entire study area presented SSR values below 5.5 and acted as wildfire sinks (Figure 4A). Specifically, the areas that presented the lowest SSR values were mainly located in Liguria, Sardinia, and Corsica. In these areas, overall, we found high aBP values (often also associated with high aIPD values), which corresponded to relatively low mean FS values. In contrast, the simulations evidenced SSR values above 6.5 in about 15% of the modeling domain: these areas, which can be classed as wildfire sources, were particularly relevant in Tuscany and PACA Departments (Figure 4A). Wildfire sources correspond to areas where the potential to originate large wildfires is high, although the probability of wildfire ignition and propagation is relatively limited.
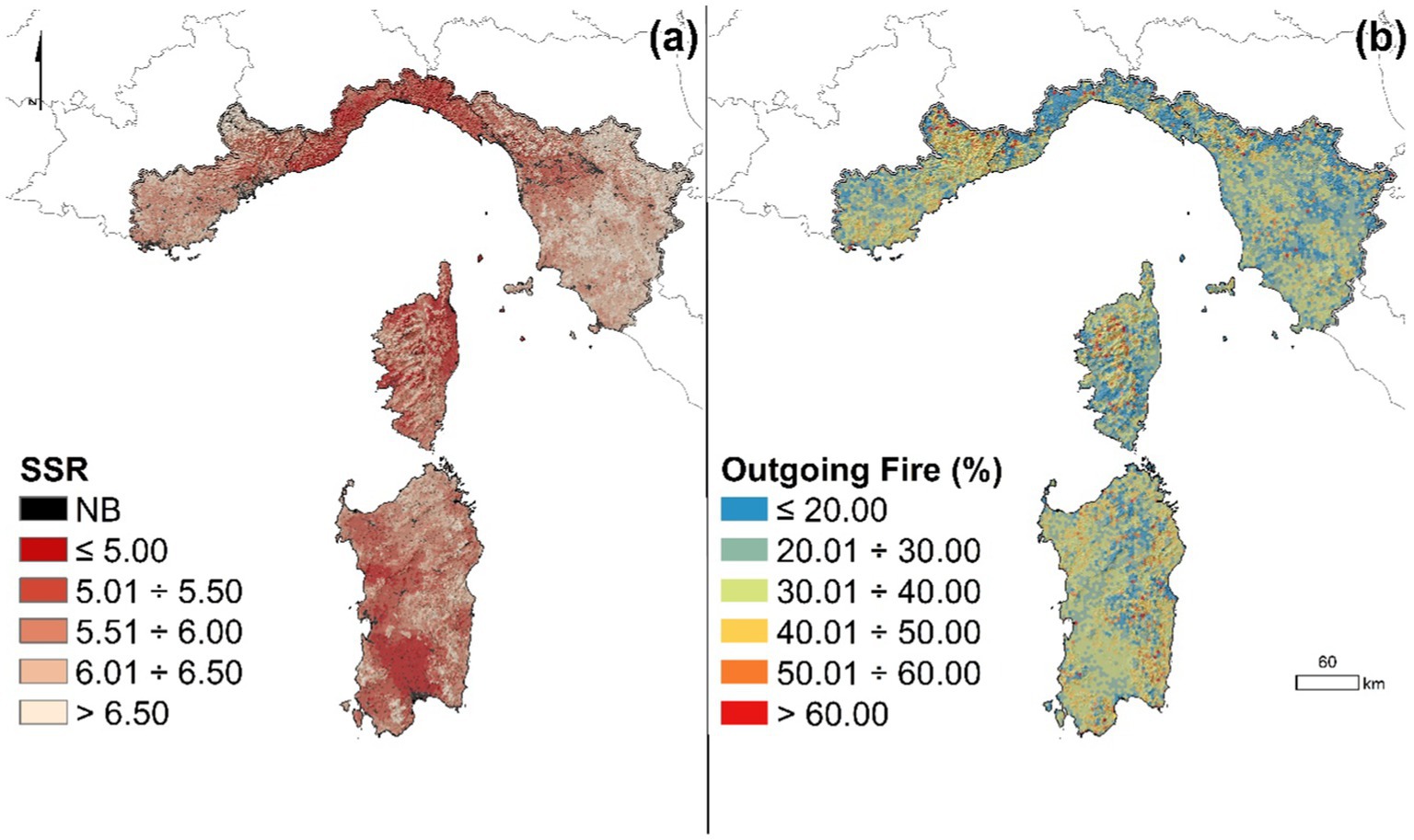
Figure 4. Maps of source-sink ratio (SSR), which measures the ratio (on a logarithmic scale) between simulated FS and simulated aBP (A), and percentage of outgoing wildfires (B), using a net of 1,000-ha hexcells.
The average incidence of outgoing wildfires in the IFM area, split into a net of hexcells with 1,000-ha size, is presented in Figure 4B. The incidence of outgoing wildfires in a given hexcell was calculated as the ratio between the AB outside the cell where the fire was ignited and the total AB (by self-burning, incoming wildfires and outgoing wildfires) in that cell. On the whole, about 4% of hexcells evidenced a high percentage (> 60%) of outgoing wildfires. These areas were mostly concentrated in mountains and steep zones, characterized by low burn and ignition probability, but able to produce large events with the potential to affect the neighboring cells given an ignition. About 48% of the study area presented a low percentage (<30%) of outgoing wildfires. In several cases, these areas were also characterized by low incoming wildfires but significant amount of self-burning wildfires, which were related to a high number of fire events with limited size and unable to spread toward other cells. The Regions that presented the lowest percentages of outgoing wildfires were Liguria and Tuscany, for which values below 30% were observed in about 70 and 60% of their hexcells (Figure 4B).
The modeling outputs revealed that agricultural areas, shrubs, and broadleaf forests were the most relevant transmitters of wildfires in the study area, with about 38, 25, and 20% of the total annual AB (about 27,600 ha per year) which was related with the above three main vegetation types, respectively (Figure 5A).
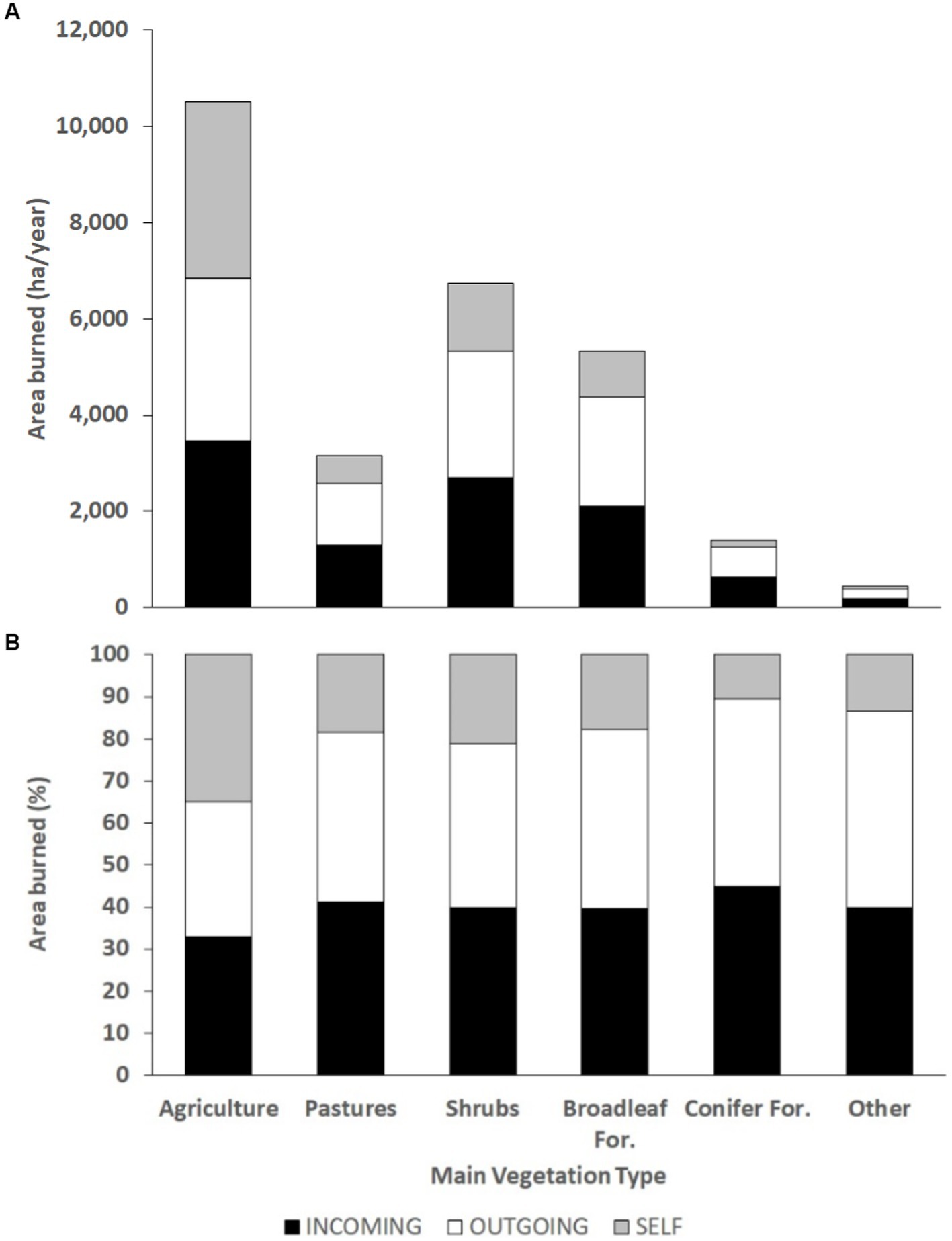
Figure 5. Simulated wildfire area burned in the main vegetation types (agriculture, pastures, shrubs, broadleaf forests, conifer forests, and other) as a function of the wildfire transmission types (incoming, outgoing, self-burning) in the whole study area. The above data report the annual wildfire transmission potential (A), and the percentage of wildfire transmission type of each main vegetation type (B).
Regarding agricultural lands, which cover about 24% of the IFM area, approximately 10,500 ha were annually burned in the study area by the wildfire simulations: about 80% (approximately 8,500 ha per year) of this AB was observed in Sardinia (Figures 5A, 6D). The other two Regions which presented relatively high annual area burned in agricultural areas were Tuscany and Liguria, with about 880 and 690 ha annually burned, respectively. With respect to the total AB at the regional scale, in general, the transmission type analysis for the agricultural areas vegetation type showed a substantial balance among outgoing (about 3,370 ha per year), incoming (about 3,470 ha per year), and self-burning fires (about 3,670 ha per year) at the IFM area level. However, two Regions presented a different pattern: Corsica and PACA Departments showed very low values of self-burning, about 13 and 10% of the total transmission for this main vegetation type, respectively, and on the other hand, exhibited a percentage of outgoing fires close to 50% (Figures 5B, 6A–E). This can be explained by the fact that the AB in agricultural lands in these Regions is quite limited, and this main vegetation types in both Regions is fragmented and characterized by small-size parcels, so wildfires tend to spread to other main vegetation types.
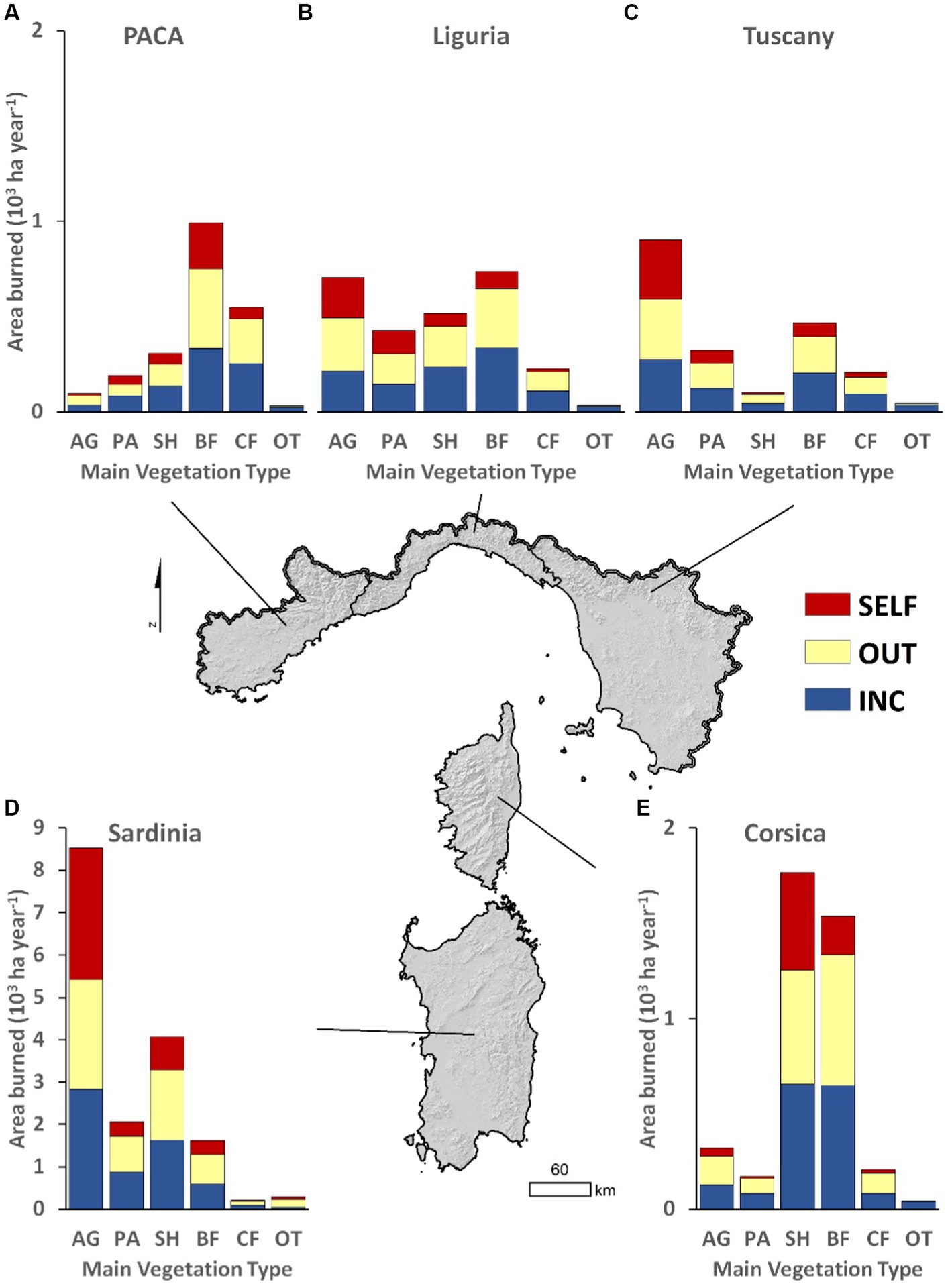
Figure 6. Annual area burned by main vegetation types and incidence of the diverse transmission types (outgoing, self-burning, and incoming fires) in the five Regions (A = PACA Departments; B = Liguria; C = Tuscany; D = Sardinia; E = Corsica) of the study area. AG, agriculture; PA, pastures; SH, shrubs; BF, broadleaf forests; CF, conifer forests; OT, other.
Shrubs, with about 6,750 ha burned annually in the study area, are the second largest main vegetation type contributing to wildfires in the 5 Regions under investigation. Again, the most significant territory was represented by Sardinia, which concentrated approximately 60% of the annual area of shrubs affected by wildfires in the IFM area. Likewise, an important contribution to the area of shrubs affected by wildfires was made by Corsica, for which the simulations estimated about 1,750 ha burned annually. Tuscany, Liguria, and PACA Departments accounted for little of the total annual shrubs AB (about 1.5 percent, 7.5 percent, and 4.5 percent, respectively) in the IFM zone. Focusing on wildfire transmission types, the main sources of transmission were incoming and outgoing fires, which for shrubs were roughly equivalent (around 40%). In addition, at the study area level, the self-burning percentage for shrubs was generally limited and close to 20%. The Regions with the highest self-burning values were Corsica (particularly in the northern area) and Sardinia (in the eastern area), i.e., the areas where the presence of shrubs is most continuous and covers large portions of the territory.
Broadleaf forests accounted for about 20% of the total annual AB in the study area, although they are the most important fuel category for the IFM area, with roughly 36% of the territory occupied by this main vegetation type. Our simulations estimated an average annual AB for broadleaf forests of about 5,300 ha. Sardinia and Corsica were the regions with the highest annual AB in broadleaf zones, with about 1,600 and 1,550 ha, respectively, followed by PACA Departments with about 1,000 ha per year. In the latter case, wildfires in broadleaf forests accounted for about 45% of the total AB in that Region. Of the total area affected by wildfires on broadleaf forests, the most relevant transmission type for the whole study area was represented by outgoing fires, which accounted for about 2,300 ha per year, or nearly 43% of the total; in the 5 Regions, this value remained relatively stable and ranged from a low of 41% in Tuscany to a high of 45% in Corsica. With about 2,100 hectares per year, incoming fires were the second fire transmission form for broadleaf forests in the IFM area; on average, for Tuscany and Liguria incoming fires were more relevant than outgoing fires in wildfire transmission on this main vegetation type.
Pastures, which occupy about 8% of the IFM lands, affected the surfaces burned in the study area by about 3,150 ha per year, which is about 11.5% of the total AB. Sardinia was the Region with the greatest influence on this, with approximately 2,050 ha burned per year, which corresponds to about 65% of the AB in pastures considering the entire study area.
About 5% of the surfaces affected by wildfires in the IFM area, roughly equal to 1,400 ha per year, involved conifer forests. In this case, the areas most disturbed were located in the PACA Departments, which accounted for 40% of the total conifer AB in the study area. Among the main vegetation types, conifers presented the lowest values of transmission by self-burning (10.5% at the study area scale). Finally, a very small proportion of wildfire events, about 450 ha per year, affected lands characterized by other fuels.
Wildfire exposure to communities
We observed substantial variations of wildfire exposure to communities across the study area (Figures 7A,B and Table 6). Overall, our simulations revealed that on average about 8,800 structures were annually threatened by wildfires in the IFM area (Table 6). Liguria and Sardinia accounted for about 65% of the total annual wildfire exposure to communities, with approximately 3,070 and 2,670 threatened structures each year, respectively. The most exposed hexcells (>16 threatened structures yr−1 per hexcell) were mainly located in Liguria, where about 6.5% of the regional hexcells presented high exposure conditions (Figure 7A). The Liguria province with the highest exposure to community was Imperia, which presented about 2,150 structures threatened by wildfires per year and a normalized value of annually threatened structures of about 1.64% (Table 6). In the remaining Regions, high exposure values (>16 threatened structures yr−1 per hexcell) were observed in much less areas and ranged from about 0.7% of the hexcells in Corsica to 0.2% in the PACA Departments (Figure 7A). On the other hand, on average approximately 78.8% of the study area presented less than 1 threatened structure yr−1 per hexcell. In this case, the highest incidence of low-exposure hexcells was observed in Tuscany (88.2%) and Corsica (82.3%), while Liguria exhibited the lowest percentage (60.1%).
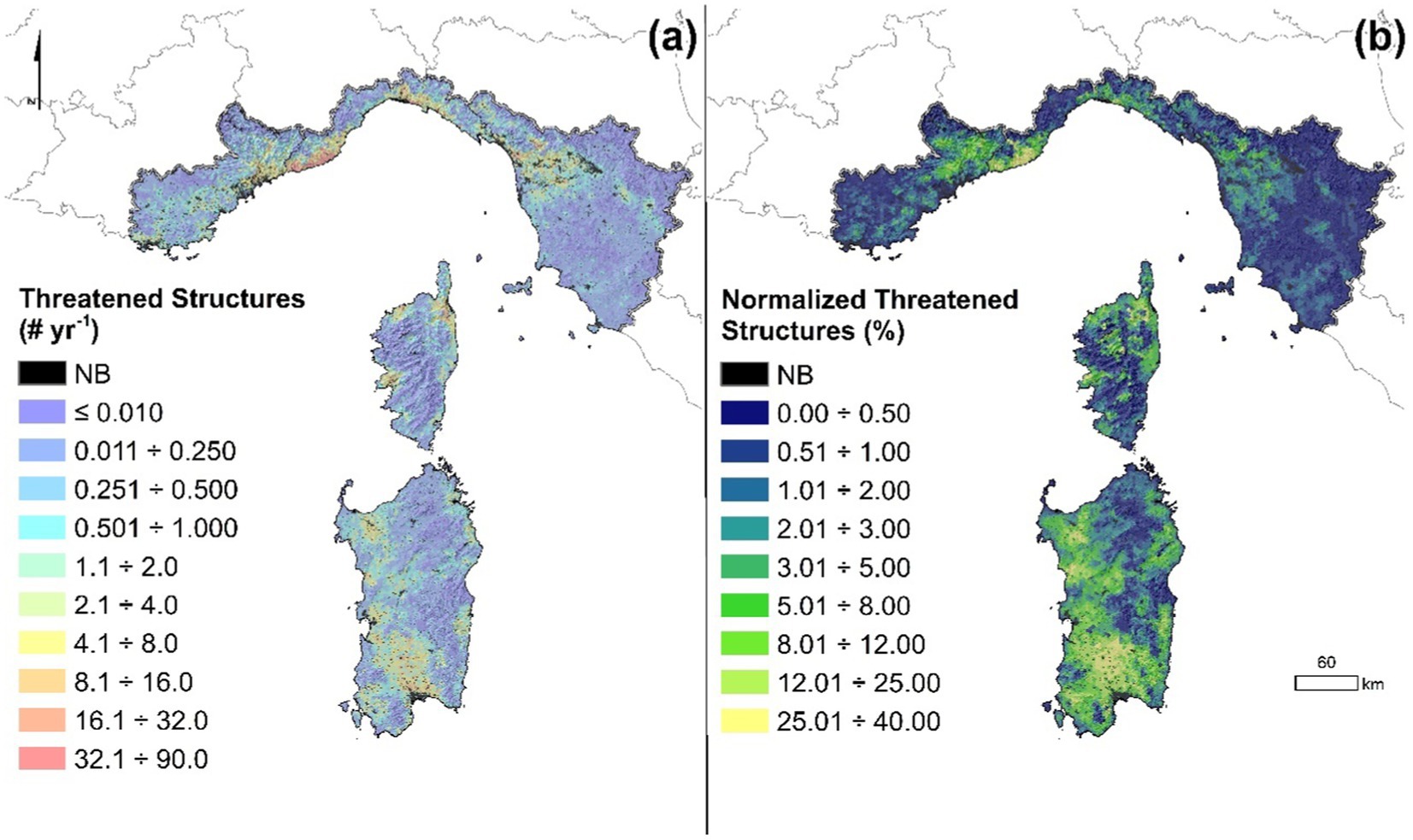
Figure 7. Annual number of threatened structures across the study area (A). Normalized threatened structures, in percentage, are derived from the ratio between the annual number of threatened buildings over the total number of buildings of a given hexcell (B). The study area was divided into 1,000-ha hexcells (n = 8,050).
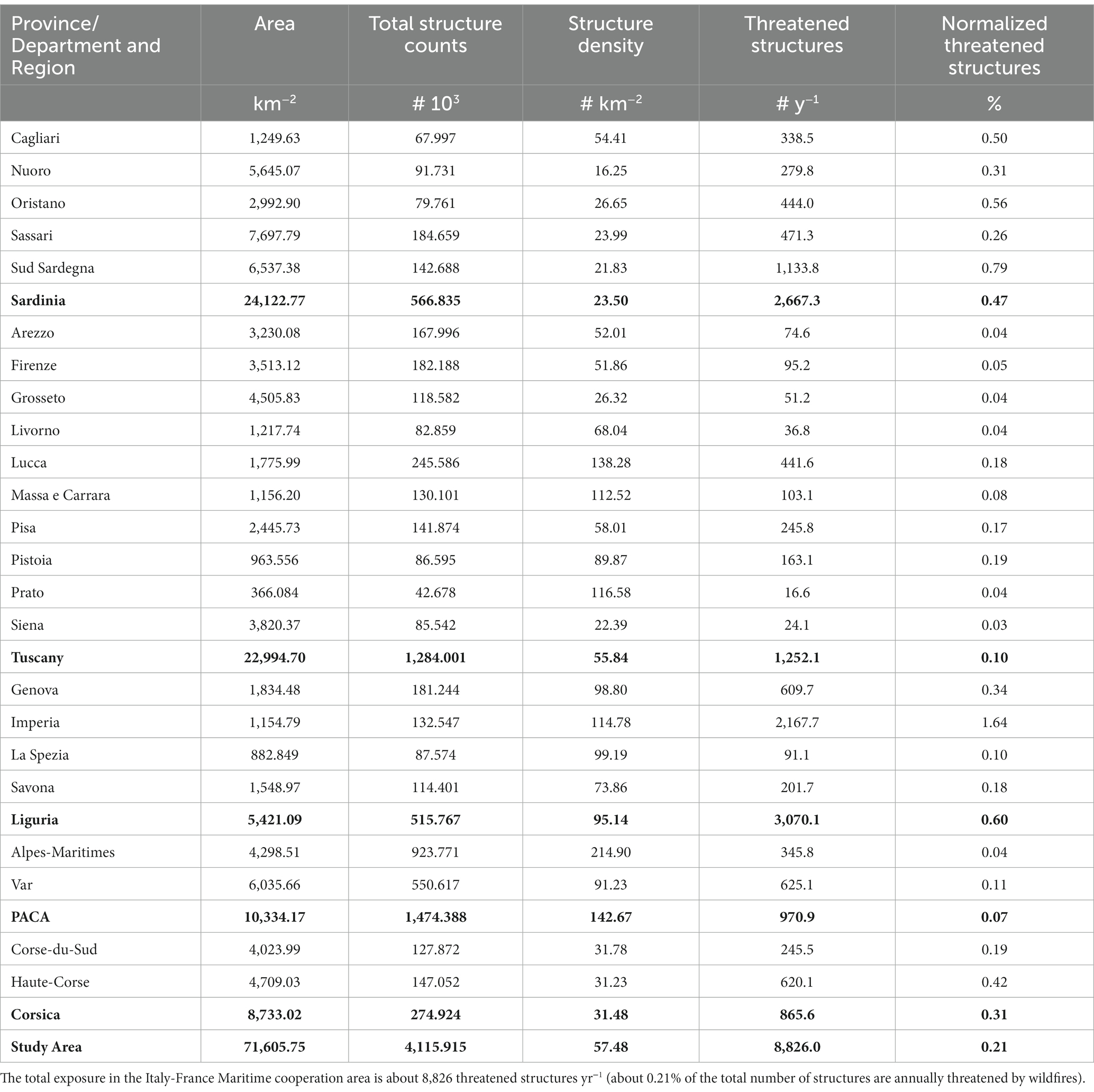
Table 6. Number of structures threatened by wildfires per year for the Regions and the Provinces/Departments included in the study area.
In terms of normalized structures threatened, that is the ratio between annual structures exposed to fires and total structures per hexcell, about 0.5% of the hexcells in the study area presented values in the range 4–8% (Figure 7B): in other words, only 0.5% of the IFM area presented a fire return interval nearby communities approximately between 12 and 25 years. The Regions which highlighted the most significant incidence of normalized structures threatened higher than 4% were Sardinia (about 1.1%, particularly in the southern part of the island) and Liguria (1.7%, largely in the western board of the Region), while in the PACA Departments and Tuscany, this exposure value was observed in less than 0.1% of their territories (Figure 7B). Regarding Liguria, it is noteworthy to evidence that this Region exhibited the highest value of normalized structures threatened, about 0.6%, which was largely determined by the province of Imperia, with average values of 1.64%, while the other provinces presented values below 0.30% (Table 6). Besides, a huge group of hexcells (about 49.7%) in the study area showed values of normalized structures threatened lower than 0.1%, which indicates very limited probabilities of wildfires impacting or menacing anthropic structures (Figure 7B). Again, Tuscany highlighted vast portions (75.7%) of the regional area with so low exposure to communities, followed by the PACA Region (58.3%). On the contrary, in Sardinia only 23.3% of the hexcells presented normalized structures threatened below 0.1%: in this Region, the average value of normalized structures threatened was one of the highest and was close to 0.5%, with the highest peaks observed in the provinces of Sud Sardegna and Oristano (Table 6).
Discussion and conclusion
In this study, we implemented a wildfire simulation modeling system to evaluate cross-boundary wildfire hazard, transmission, and exposure to communities in the so-called Italy-France Maritime (IFM) cooperation area, which covers about 72,000 km2 of land and includes four Regions (Sardinia, Tuscany, and Liguria, in Italy, and Corsica, in France) and two French Departments [Var and Alpes-Maritimes, located in the Provence-Alpes-Côte d’Azur (PACA) Region]. We were able to characterize and measure fine-scale (100-m resolution) wildfire risk factors by taking into account historical fire regimes, current fuels, weather, and fuel moisture conditions associated with the occurrence of wildfires above 100 ha. Through the iterative modeling of thousands large wildfires, we captured a large number of possible combinations of ignition sources and fire-weather scenarios in the study area. This comprehensive approach enabled the generation of a broad set of potential wildfire footprints, thereby helping to reduce some of the uncertainties in modeled predictions of exposure to communities and overall hazard (for instance, by using historically-based ignition probability grids and weighted weather scenarios). Furthermore, stochastic wildfire modeling systems, such as the one we implemented in this study, facilitate predictions of future large events and the exposure odds of severe wildfires impacting forest and rural areas, anthropic values and human communities (Ramirez et al., 2019; Ager et al., 2021). These predictions offer an assessment of expected wildfires in terms of potential hazard and transmission, and form the basis for developing consistent risk management strategies in the IFM cooperation area or elsewhere. The estimation of wildfire risk or exposure stem from the potential effects of a large set of plausible large wildfire episodes and aim at quantitative assessments rather than qualitative indices (Calkin et al., 2011; Oom et al., 2022; Chuvieco et al., 2023). To date, only a few studies have employed comparable wildfire modeling techniques across such a vast domain to explore wildfire hazard, transmission, and exposure at fine resolutions in the Euro-Mediterranean area (Alcasena et al., 2019, 2021): our study is among this small group, encompassing numerous communities and providing a level of detail not often seen within the Euro-Mediterranean area.
On the whole, our simulations were quite consistent with the historical wildfire data for the whole study area. Focusing on the annual area burned (AB) and burn probability (BP), we obtained a good agreement in each of the Regions, even if a limited overestimation of the annual AB was observed (about +3% for the whole study area). Similarly, an overestimation of the simulated annual AB compared to the historical data was reported by previous works (e.g., Sá et al., 2022). The simulated annual ignition point density (aIPd) was in line with the historical data, and the most relevant differences with respect to the observed data were observed in the PACA Departments (+2.5%) and Tuscany (−1.6%). We highlighted relevant variations among and within the Regions under investigation in terms of wildfire hazard, exposure to communities, and transmission. These differences are related to several factors, which include wildfire regime and frequency, bioclimatic conditions, occurrence of weather conditions conducive to large events, and socio-economic factors. In this work, we tried to keep into account these specific regional characteristics: for instance, fuel moisture values were tuned depending on bioclimatic conditions, and this allowed to obtain more realistic modeling outputs. However, the spatial maps of BP for Sardinia presented some relevant differences in the identification of the hot-spot zones with respect to a previous study (Salis et al., 2021). We also highlighted some relevant differences in historical vs. simulated aBP for some other provinces or Departments in PACA and Liguria. This can be explained by the fact that in this work the wildfire simulations for each Region were not stratified considering homogeneous zones and adopting specific weather conditions for each zone as done in the abovementioned study; moreover, in Salis et al. (2021) special attention was given to fire ignition patterns on large and very large wildfire days (above 200 ha), rather than considering the whole set of fire ignitions above 1 ha. The much lower incidence of wildfire ignitions, and particularly of large and very large wildfires in the other Regions under investigation, did not allow to replicate the same methodology previously applied in Sardinia, as the number of historical events to characterize BP and wildfire hazard for given weather scenarios was not able to adequately inform the wildfire modeling approach and to saturate the IFM study area. This resulted in a general overestimation of the simulated aBP in those areas and provinces/Departments which exhibited high values of ignition probability. In this regard, future work will refine the wildfire simulation approach proposed in this study to further calibrate the input and output data at more local scales by identifying homogeneous areas in terms of weather and fire regime. Yet, in terms of identification of the most significant hot-spot and high BP zones, our results were in line with previous studies carried out in some of the IFM Regions (Tonini et al., 2020; Pimont et al., 2021).
We evidenced the role played by herbaceous fuels and shrubs in wildfire spread and transmission, as well as the relatively low potential of forests in acting as wildfire sources. Similar results were highlighted by other works carried out in the Euro-Mediterranean area (Nunes et al., 2005; Oliveira et al., 2013; Salis et al., 2021). In general, herbaceous areas are characterized by the presence of fine-sized fuel particles (with varying loads depending on the level of management and grazing) which generally exhibits high surface area-to-volume ratios and are fully or nearly cured during the wildfire season: in combination with strong winds and low fuel moisture, herbaceous fuels can sustain the establishment of high wildfire spread and acceleration rates and the expansion potential of the fire fronts toward neighboring forests, shrublands and anthropic areas (Cheney et al., 1993; Cheney and Gould, 1995; Cruz et al., 2022). In this regard, the presence of agriculture and pastoral farms must be recognized as key factor for land management and fire risk reduction purposes, as it can limit continuity and load of flammable fuels (Catry et al., 2010; Aquilué et al., 2020; Colantoni et al., 2020; Spadoni et al., 2023). The current patterns of loss of agriculture, pastoralism, and silvicultural activities, with the inherent land abandonment and vegetation encroachment processes that are affecting a number of marginal and inner areas of the IFM territory, can increase the occurrence and severity of major wildfire events (Moreira et al., 2011; Sil et al., 2019; Salis et al., 2022). This risk could be further exacerbated by climate change, which is already impacting Southern Europe, and is expected to be responsible for a lengthening of the fire seasons and for increasing the risk and extent of wildfires in future years (Dupuy et al., 2020; Pimont et al., 2022; Venäläinen et al., 2022).
The wildfire modeling and exposure results from this study can guide the coordinated design of landscape fuel treatment projects across the IFM area. While our previous management-oriented works were limited to some study areas in Sardinia (Salis et al., 2016, 2018; Jahdi et al., 2023), we now have the required baseline information to extend the analysis to the entire cooperation area. For example, this expanded scope could facilitate the development of fuel reduction projects in high-priority areas, designed to protect communities in rural or forest areas where a declining population is leading to rapid fuel increase and pasture encroachment (Schuh et al., 2020). Furthermore, conducting landscape fuel treatment projects could benefit from the analysis of the wildfire transmission types (self-burning, outgoing, or incoming fires) to identify the best locations for contrasting the spread of large wildfires: significant reductions of the potential area burned could be obtained prioriting those areas (hexcells) with high values of outgoing fires, while a lower effectiveness would be guaranteed treating in self-burning locations. Plus, understanding and mapping the preferential fire propagation types for the main vegetation types can be valuable in orienting wildfire risk mitigation strategies: for instance, in case of herbaceous fuels with high transmission potential, grazing may result in the most appropriate and cheap fuel reduction strategy. On the other hand, hexcells with high propagation potential and high fuel loads, such as given forest areas with shrub understory, would likely require mechanical fuel treatments and more investments in terms of budget and personnel. In this context, using prioritization tools and building upon the modeling outputs, we can design the optimal mosaic of fuel treatment locations while considering the availability of local resources, existing environmental constraints, and operational limitations during treatment implementation (Alcasena et al., 2018; Palaiologou et al., 2020). Specifically, these projects could be integrated into ongoing community protection programs, where the use of prescribed fires and/or grazing is being incorporated to mitigate wildfire hazards, limit shrub encroachment and preserve grasslands, particularly in areas where extensive pastoral use remains a significant activity (Montiel and Kraus, 2010; Varela et al., 2018; Valkó and Deák, 2021; Cabiddu et al., 2023).
In wildland-urban interface (WUI) areas, our exposure results offer a valuable resource for providing a spatially explicit and quantitative measure of buildings threatened by wildfires and for enhancing existing WUI maps (Del Giudice et al., 2021; Bar-Massada et al., 2023), which aid in identifying areas where structures intermingle with hazardous fuels. However, previous studies have shown that high building density does not necessarily correlate with high wildfire exposure or losses (Alcasena et al., 2021, 2022), thus emphasizing the need of estimating the actual number of structures threatened by wildfires and provide policymakers with rough estimates of potential impacts during wildfires. These findings are crucial for insurance companies, raising awareness among the population, and guiding wildfire risk management efforts aimed at generating fire-adapted communities. It is important to note, however, that careful interpretation of our exposure results is necessary, as they represent a worst-case scenario without accounting for suppression efforts. In real-life fire scenarios, intervention teams on the ground and in the air work hard and diligently to limit damages in WUI areas.
On the whole, despite the escalating threat and increasing losses from unprecedented catastrophic wildfires occurred in recent years and likely occurring in future decades under changing climate conditions, wildfire risk assessment varies widely among EU countries and often, due to fire regime and environmental specificities, follows different national or even regional approaches not fully comparable (Oom et al., 2022). To address the lack of a comprehensive pan-European modeling and risk assessment system, a number of EU regulations and projects are progressively emphasizing the need for an EU-scale approach, as well as for a harmonization of procedures for wildfire risk assessment and for improving the actions of EU member states and cross-border regions in preventing and managing wildfires (Chuvieco et al., 2023). We advocate for the need to predict potential large and severe wildfires and assess potential hazard and exposure over vast domains in order to manage wildfires more effectively and prioritize risk prevention and mitigation strategies. However, current wildfire management budgets and efforts at EU level are mostly focused on wildfire detection, monitoring, and suppression: when weather and drought conditions are extreme or in case of concomitant events, real-time fire monitoring and suppression alone have proven to be inefficient and unable to contrast the propagation and impacts of large wildfires (Calkin et al., 2014; Thompson et al., 2017). For instance, a single large wildfire that occurred in the Montiferru area (western Sardinia) in July 2021, under extreme weather, burned about 13,000 hectares, damaged around 600 structures and threatened approximately 1,300 more. Such large and severe episodes, which exhibit rapid growth and extreme fire behavior, can easily overwhelm terrestrial forces suppression efforts, and often even the effectiveness of aerial resources is limited by a number of environmental conditions or constraints (e.g., topography, strong winds, low visibility, smoke, atmospheric turbulence, proximity to water resources), as well as by the priority goals of protecting lives and values of interest rather than focusing on contrasting the major wildfire paths (Plucinski et al., 2012; Tedim et al., 2018; Rodrigues et al., 2020). In other words, wildfire containment only becomes feasible when weather, environmental and civil protection conditions offer a reprieve, rendering the process largely opportunistic (Costa Alcubierre et al., 2011; Castellnou et al., 2019). As an alternative, wildfire hazard and transmission assessment at operational scales provides the anticipation necessary to implement large-scale fuel treatments or risk prevention and reduction programs and allows to identify high-priority areas or safe zones (Ager et al., 2017; Thompson et al., 2022). It would be therefore crucial to implement cross-border and transregional projects and policies that strengthen cooperation and support mechanisms, starting with standardized and harmonized wildfire management and planning strategies and including the adoption of virtuous examples of land and fuel management or prevention activities, as neighboring southern EU territories share similar problems and contexts and are highly fire-prone. In this regard, this work lays the foundation for the identification of the main critical issues of the IFM area in terms of wildfire hazard, transmission, and exposure to communities.
There are numerous limitations to the current study. Even if fire spread models are used with good success in several areas after adequate calibration and validation, the modeling equations and variables involved in the spatiotemporal simulation of fire propagation and behavior have inherent limitations and assumptions that should be taken into consideration (Sullivan, 2009a,b; Mell et al., 2010; Alexander and Cruz, 2013). In addition, fire models based on Rothermel’s equation (Rothermel, 1972) present some weaknesses in accounting for the coupled effects of topography and wind on fire propagation and behavior, particularly in areas with complex topography (Viegas, 2004; Viegas and Simeoni, 2011; Rodrigues et al., 2013).
In terms of fuels, we used previously validated standard and custom models and associated them with standardized vegetation types for the IFM area to generate fuel model maps. Future works could refine the mapping of vegetation types and the related fuel models using spatially explicit local information and more accurate datasets. For instance, LiDAR-derived data and metrics could be used to improve the assessment and mapping of canopy fuels, which are crucial for assessing the occurrence of crown fires, as well as for estimating canopy understory (Gonzalez-Ferreiro et al., 2017; Mauro et al., 2021; Marino et al., 2022; Labenski et al., 2023). Likewise, more accurate mapping of herbaceous fuels (i.e., considering their level of agricultural management or grazing regimes) and shrublands (i.e., determining height, density, canopy cover) would improve the assignment of specific fuel models, with relevant effects in the fuel map quality and reliability for several fire-prone contexts (García et al., 2011; Marino et al., 2016; Huesca et al., 2019; Bright et al., 2022). In this sense, a pan European freely available LiDAR-data coverage based on common and standardized methodologies and products, as well as the definition of standard formats, coordinate systems, resolution and update timing, would be strategic.
To minimize fire ignition locations and fire-weather uncertainty, we performed a large number of simulations based on historic ignition densities and on randomly sampled weather conditions from observed probabilities during the wildfire season, focusing on the days characterized by large fire spread. However, we did not consider containment effects, which vary a lot based on myriad factors such as fire simultaneity, resource availability and environmental constraints (Finney et al., 2009; Plucinski et al., 2012; Plucinski, 2019): including containment effects would exceedingly complicate the analysis of fire exposure, transmission and hazard, and therefore was out of the scopes of this work. Consequently, we are aware that our results may have overestimated the burned areas close to communities or highly valued resources, where suppression efforts are prioritized and concentrated during wildfires. Nevertheless, considering all limitations, the modeled outcomes showed relatively good correspondence with the observed fire regime and conditions, and the feedbacks received from the Regional Partners involved in this project were overall positive.
Data availability statement
The raw data supporting the conclusions of this article will be made available by the authors, without undue reservation.
Author contributions
MS and FA-U: writing. MS, LG, FA-U, and BA: methodology. RJ, BA, GP, and CS: validation. MS, LG, FA-U, and BA: conceptualization. PD: supervision and funding acquisition. All authors contributed to the article and approved the submitted version.
Funding
This work was carried out in the framework of the following projects: MED-Star (grant no. E88H19000120007), Med-Foreste (grant no. B85I1900010007) and Med-Coopfire (grant no. B81I1900010007) projects, supported by the European Union under the cross-border Programma Italia-Francia Marittimo 2014–2020; the “FOE2019—Climate Change: risk mitigation for sustainable development” (Ministerial Decree no. 856/19) project, funded by the Italian Ministry of Education, University and Research (MIUR); the FirEUrisk (Grant agreement ID: 101003890) project, funded by the EU under the “H2020-EU.3.5.—SOCIETAL CHALLENGES—Climate action, Environment, Resource Efficiency and Raw Materials” Programme.
Acknowledgments
We would like to thank the Institutions and Partners involved in the MED-Star project for collaborating in this study with data and suggestions and for contributing to the calibration phase and analysis of the simulation modeling approach. We are particularly grateful to Office National de Foret (ONF), Regione Sardegna, Regione Toscana, Regione Liguria, Regione PACA and Collectivité de Corse for actively contributing to the preparation of the standardized maps and data on vegetation types and fuel models. We would also like to thank Alan Ager of the USDA Forest Service and Stuart Brittain of Alturas Solutions for adapting the fire behavior library to this case study. Finally, the authors would like to thank the three reviewers who helped improving the quality of this work.
Conflict of interest
The authors declare that the research was conducted in the absence of any commercial or financial relationships that could be construed as a potential conflict of interest.
Publisher’s note
All claims expressed in this article are solely those of the authors and do not necessarily represent those of their affiliated organizations, or those of the publisher, the editors and the reviewers. Any product that may be evaluated in this article, or claim that may be made by its manufacturer, is not guaranteed or endorsed by the publisher.
Supplementary material
The Supplementary material for this article can be found online at: https://www.frontiersin.org/articles/10.3389/ffgc.2023.1241378/full#supplementary-material
References
Ager, A. A., Day, M. A., Alcasena, F. J., Evers, C. R., Short, K. C., and Grenfell, I. (2021). Predicting paradise: modeling future wildfire disasters in the western US. Sci. Total Environ. 784:147057. doi: 10.1016/j.scitotenv.2021.147057
Ager, A. A., Day, M. A., Finney, M. A., Vance-Borland, K., and Vaillant, N. M. (2014). Analyzing the transmission of wildfire exposure on a fire-prone landscape in Oregon, USA. For. Ecol. Manag. 334, 377–390. doi: 10.1016/j.foreco.2014.09.017
Ager, A. A., Day, M. A., Palaiologou, P., Houtman, R., Ringo, C., and Evers, C. (2019). Cross-boundary wildfire and community exposure: a framework and application in the western US. Fort Collins, CO: USDA Forest Service, Rocky Mountain Research Station.
Ager, A. A., Evers, C. R., Day, M. A., Preisler, H. K., Barros, A. M., and Nielsen-Pincus, M. (2017). Network analysis of wildfire transmission and implications for risk governance. PLoS One 12:e0172867. doi: 10.1371/journal.pone.0172867
Ager, A. A., Vaillant, N. M., and Finney, M. A. (2011). Integrating fire behavior models and geospatial analysis for wildland fire risk assessment and fuel management planning. J. Combust. 2011:e572452, 1–19. doi: 10.1155/2011/572452
Ager, A. A., Vaillant, N. M., Finney, M. A., and Preisler, H. K. (2012). Analyzing wildfire exposure and source–sink relationships on a fire prone forest landscape. For. Ecol. Manag. 267, 271–283. doi: 10.1016/j.foreco.2011.11.021
Agriligurianet (2023). Cartografia dei tipi forestali 2013. Available at: https://www.agriligurianet.it/it/impresa/politiche-di-sviluppo/tutela-economia-bosco/cartografia-dei-tipi-forestali.html (Accessed June 6, 2023).
Alcasena, F. J., Ager, A. A., Bailey, J. D., Pineda, N., and Vega-Garcia, C. (2019). Towards a comprehensive wildfire management strategy for Mediterranean areas: framework development and implementation in Catalonia, Spain. J. Environ. Manage. 231, 303–320. doi: 10.1016/j.jenvman.2018.10.027
Alcasena, F., Ager, A. A., Belavenutti, P., Krawchuk, M., and Day, M. A. (2022). Contrasting the efficiency of landscape versus community protection fuel treatment strategies to reduce wildfire exposure and risk. J. Environ. Manag. 309:114650. doi: 10.1016/j.jenvman.2022.114650
Alcasena, F., Ager, A. A., Le Page, Y., Bessa, P., Loureiro, C., and Oliveira, T. (2021). Assessing wildfire exposure to communities and protected areas in Portugal. Fire 4:82. doi: 10.3390/fire4040082
Alcasena, F. J., Ager, A. A., Salis, M., Day, M. A., and Vega-Garcia, C. (2018). Optimizing prescribed fire allocation for managing fire risk in Central Catalonia. Sci. Total Environ. 621, 872–885. doi: 10.1016/j.scitotenv.2017.11.297
Alcasena, F. J., Salis, M., Ager, A. A., Arca, B., Molina, D., and Spano, D. (2015). Assessing landscape scale wildfire exposure for highly valued resources in a Mediterranean area. Environ. Manag. 55, 1200–1216. doi: 10.1007/s00267-015-0448-6
Alexander, M. E., and Cruz, M. G. (2013). Are the applications of wildland fire behaviour models getting ahead of their evaluation again? Environ. Model. Softw. 41, 65–71. doi: 10.1016/j.envsoft.2012.11.001
Anderson, H. E. (1982). Aids to determining fuel models for estimating fire behavior. Ogden, UT: USDA Forest Service, Intermountain Forest and Range Experiment Station.
Andrews, P. L. (2014). Current status and future needs of the BehavePlus fire modeling system. Int. J. Wildland Fire 23, 21–33. doi: 10.1071/WF12167
Aparício, B. A., Santos, J. A., Freitas, T. R., Sá, A. C. L., Pereira, J. M. C., and Fernandes, P. M. (2022). Unravelling the effect of climate change on fire danger and fire behaviour in the transboundary biosphere Reserve of Meseta Ibérica (Portugal-Spain). Clim. Chang. 173:5. doi: 10.1007/s10584-022-03399-8
Aquilué, N., Fortin, M.-J., Messier, C., and Brotons, L. (2020). The potential of agricultural conversion to shape Forest fire regimes in Mediterranean landscapes. Ecosystems 23, 34–51. doi: 10.1007/s10021-019-00385-7
Arca, B., Bacciu, V., Pellizzaro, G., Salis, M., Ventura, A., Duce, P., et al. (2009). Fuel model mapping by IKONOS imagery to support spatially explicit fire simulators. Proceedings of the 7th international workshop on advances in remote sensing and GIS applications in forest fire management towards an operational use of remote sensing in forest fire management. Matera, Italy.
Arca, B., Ghisu, T., Casula, M., Salis, M., and Duce, P. (2019). A web-based wildfire simulator for operational applications. Int. J. Wildland Fire 28:99. doi: 10.1071/wf18078
Ascoli, D., Vacchiano, G., Scarpa, C., Arca, B., Barbati, A., Battipaglia, G., et al. (2020). Harmonized dataset of surface fuels under Alpine, temperate and Mediterranean conditions in Italy. A synthesis supporting fire management. IForest Biogeosci. For. 13, 513–522. doi: 10.3832/ifor3587-013
Balbi, J. H., Morandini, F., Silvani, X., Filippi, J. B., and Rinieri, F. (2009). A physical model for wildland fires. Combust. Flame 156, 2217–2230. doi: 10.1016/j.combustflame.2009.07.010
Bar-Massada, A., Alcasena, F., Schug, F., and Radeloff, V. C. (2023). The wildland – urban interface in Europe: spatial patterns and associations with socioeconomic and demographic variables. Landsc. Urban Plan. 235:104759. doi: 10.1016/j.landurbplan.2023.104759
Benali, A., Sá, A. C. L., Pinho, J., Fernandes, P. M., and Pereira, J. M. C. (2021). Understanding the impact of different landscape-level fuel management strategies on wildfire Hazard in Central Portugal. Forests 12:522. doi: 10.3390/f12050522
Bright, B. C., Hudak, A. T., McCarley, T. R., Spannuth, A., Sánchez-López, N., Ottmar, R. D., et al. (2022). Multitemporal lidar captures heterogeneity in fuel loads and consumption on the Kaibab Plateau. Fire Ecol. 18:18. doi: 10.1186/s42408-022-00142-7
Byram, G. M. (1959). “Combustion of forest fuels” in Forest fire: control and use. ed. K. P. Davis (New York: McGraw-Hill), 61–89.
Cabiddu, S., Cuccu, G. M., Pinna, T. M., Magari, L., Putzolu, G., Pala, E., et al. (2023). El uso cultural del fuego y sus efectos positivos en regiones agropastorales Mediterráneas. Rev. Incendio Riesgos Nat 10, 35–38.
Calkin, D. E., Cohen, J. D., Finney, M. A., and Thompson, M. P. (2014). How risk management can prevent future wildfire disasters in the wildland-urban interface. PNAS 111, 746–751. doi: 10.1073/pnas.1315088111
Calkin, D. E., Finney, M. A., Ager, A. A., Thompson, M. P., and Gebert, K. M. (2011). Progress towards and barriers to implementation of a risk framework for US federal wildland fire policy and decision making. Forest Policy Econ. 13, 378–389. doi: 10.1016/j.forpol.2011.02.007
Canu, S., Rosati, L., Fiori, M., Motroni, A., Filigheddu, R., and Farris, E. (2015). Bioclimate map of Sardinia (Italy). J. Maps 11, 711–718. doi: 10.1080/17445647.2014.988187
Castellnou, M., Prat-Guitart, N., Arilla, E., Larrañaga, A., Nebot, E., Castellarnau, X., et al. (2019). Empowering strategic decision-making for wildfire management: avoiding the fear trap and creating a resilient landscape. Fire Ecol. 15:31. doi: 10.1186/s42408-019-0048-6
Catry, F. X., Rego, F. C., Silva, J. S., Moreira, F., Camia, A., Ricotta, C., et al. (2010). “Fire starts and human activities” in Towards integrated fire management - outcomes of the European project fire paradox European forest institute research report. eds. J. S. Silva, F. Rego, P. Fernandes, and E. Rigolot (Joensuu, Finland: EFI), 9–22.
Cheney, N. P., and Gould, J. S. (1995). Fire growth in grassland fuels. Int. J. Wildland Fire 5, 237–247. doi: 10.1071/WF9950237
Cheney, N. P., Gould, J. S., and Catchpole, W. R. (1993). The influence of fuel, weather and fire shape variables on fire-spread in grasslands. Int. J. Wildland Fire 3, 31–44. doi: 10.1071/WF9930031
Chuvieco, E., Yebra, M., Martino, S., Thonicke, K., Gómez-Giménez, M., San-Miguel, J., et al. (2023). Towards an integrated approach to wildfire risk assessment: when, where, what and how may the landscapes burn. Fire 6:215. doi: 10.3390/fire6050215
Colantoni, A., Egidi, G., Quaranta, G., D’Alessandro, R., Vinci, S., Turco, R., et al. (2020). Sustainable land management, wildfire risk and the role of grazing in Mediterranean urban-rural interfaces: a regional approach from Greece. Land 9:21. doi: 10.3390/land9010021
Costa Alcubierre, P., Ribau, M. C., de Egileor, A. L. O., Bover, M. M., and Kraus, P. D. (2011). Prevention of large wildfires using the fire types concept. Generalitat de Catalunya Barcelona: Spain.
Cruz, M. G., Alexander, M. E., and Kilinc, M. (2022). Wildfire rates of spread in grasslands under critical burning conditions. Fire 5:55. doi: 10.3390/fire5020055
Del Giudice, L., Arca, B., Scarpa, C., Pellizzaro, G., Duce, P., and Salis, M. (2021). The wildland-anthropic interface raster data of the Italy-France maritime cooperation area (Sardinia, Corsica, Tuscany, Liguria, and Provence-Alpes-Côte d'Azur). Data Brief 38:107355. doi: 10.1016/j.dib.2021.107355
Duce, P., Pellizzaro, G., Arca, B., Ventura, A., Bacciu, V., Salis, M., et al. (2012). “Fuel types and potential fire behavior in Sardinia and Corsica islands: a pilot study” in Modelling fire behaviour and risk. eds. D. Spano, V. Bacciu, M. Salis, and C. Sirca (Italy: Nuova StampaColor Muros)
Dupuy, J., Fargeon, H., Martin-StPaul, N., Pimont, F., Ruffault, J., Guijarro, M., et al. (2020). Climate change impact on future wildfire danger and activity in southern Europe: a review. Ann. For. Sci. 77:35. doi: 10.1007/s13595-020-00933-5
EEA (2017). Corine Land Cover CLC 2012 Version 1851. Available at: https://land.copernicus.eu/pan-european/corine-land-cover/clc-2012?tab=download (Accessed August 4, 2023).
EU IFM (2023). Interreg Italy-France Maritime Programme. Available at: https://interreg-maritime.eu/it/web/pc-marittimo/home (Accessed June 6, 2023).
FAO and Plan Bleu (2018). State of Mediterranean Forests 2018. Food and Agriculture Organization of the United Nations, Rome and Plan Bleu, Marseille. Available at: https://www.fao.org/documents/card/fr/c/CA2081EN/
Fernandes, P. M., Loureiro, C., Guiomar, N., Pezzatti, G. B., Manso, F. T., and Lopes, L. (2014). The dynamics and drivers of fuel and fire in the Portuguese public forest. J. Environ. Manag. 146, 373–382. doi: 10.1016/j.jenvman.2014.07.049
Fick, S. E., and Hijmans, R. J. (2017). WorldClim 2: new 1-km spatial resolution climate surfaces for global land areas. Int. J. Climatol. 37, 4302–4315. doi: 10.1002/joc.5086
Finney, M. A. (2002). Fire growth using minimum travel time methods. Can. J. For. Res. 32, 1420–1424. doi: 10.1139/x02-068
Finney, M. A. (2004). FARSITE: fire area simulator-model development and evaluation. Ft. Collins, CO: U.S. Department of Agriculture, Forest Service, Rocky Mountain Research Station.
Finney, M. A. (2006). “An overview of FlamMap fire modeling capabilities” in Fuels management-how to measure success, proceedings RMRS-P-41. eds. P. L. Andrews and B. W. Butler (Fort Collins, CO: USDA Forest Service, Rocky Mountain Research Station), 213–220.
Finney, M. A., Grenfell, I. C., and McHugh, C. W. (2009). Modeling containment of large wildfires using generalized linear mixed-model analysis. For. Sci. 55, 249–255. doi: 10.1093/forestscience/55.3.249
Finney, M. A., McHugh, C. W., Grenfell, I. C., Riley, K. L., and Short, K. C. (2011). A simulation of probabilistic wildfire risk components for the continental United States. Stoch. Environ. Res. Risk Assess. 25, 973–1000. doi: 10.1007/s00477-011-0462-z
Forzieri, G., Girardello, M., Ceccherini, G., Spinoni, J., Feyen, L., Hartmann, H., et al. (2021). Emergent vulnerability to climate-driven disturbances in European forests. Nat. Commun. 12:1081. doi: 10.1038/s41467-021-21399-7
Galizia, L. F., Alcasena, F., Prata, G., and Rodrigues, M. (2021). Assessing expected economic losses from wildfires in eucalypt plantations of western Brazil. Forest Policy Econ. 125:102405. doi: 10.1016/j.forpol.2021.102405
Galizia, L. F., Curt, T., Barbero, R., and Rodrigues, M. (2022). Understanding fire regimes in Europe. Int. J. Wildland Fire 31, 56–66. doi: 10.1071/WF21081
Ganteaume, A., Barbero, R., Jappiot, M., and Maillé, E. (2021). Understanding future changes to fires in southern Europe and their impacts on the wildland-urban interface. J. Saf. Sci. Resil. 2, 20–29. doi: 10.1016/j.jnlssr.2021.01.001
García, M., Riaño, D., Chuvieco, E., Salas, J., and Danson, F. (2011). Multispectral and LiDAR data fusion for fuel type mapping using support vector machine and decision rules. Remote Sens. Environ. 115, 1369–1379. doi: 10.1016/j.rse.2011.01.017
GEOscopio (2023). Reg. Toscana Inventar. For. 2009. Available at: https://www502.regione.toscana.it/geoscopio/download/tematici/ift/index.html
Gomez, C., Mangeas, M., Curt, T., Ibanez, T., Munzinger, J., Dumas, P., et al. (2015). Wildfire risk for main vegetation units in a biodiversity hotspot: modeling approach in New Caledonia, South Pacific. Ecol. Evol. 5, 377–390. doi: 10.1002/ece3.1317
Gonzalez-Ferreiro, E., Arellano-Pérez, S., Castedo-Dorado, F., Hevia, A., Vega, J. A., Vega-Nieva, D. J., et al. (2017). Modelling the vertical distribution of canopy fuel load using national forest inventory and low-density airbone laser scanning data. PLoS One 12:e0176114. doi: 10.1371/journal.pone.0176114
Huesca, M., Riaño, D., and Ustin, S. L. (2019). Spectral mapping methods applied to LiDAR data: application to fuel type mapping. Int. J. Appl. Earth Obs. Geoinf. 74, 159–168. doi: 10.1016/j.jag.2018.08.020
IGN (2023). Produire Diffus. Données Géographiques For. En Fr. – DB Foret 2015. Available at: https://geoservices.ign.fr/bdforet (Accessed August 4, 2023).
In(see 2023). Recens. Population 2020. Available at: https://www.insee.fr/fr/statistiques?theme=0 (Accessed June 6, 2023).
ISTAT (2023). Censimento Della Pop. 2020. Available at: http://dati.istat.it/Index.aspx?DataSetCode=DCIS_POPRES1# (Accessed June 6, 2023).
Jahdi, R., Del Giudice, L., Melis, M., Lovreglio, R., Salis, M., Arca, B., et al. (2023). Assessing the effects of alternative fuel treatments to reduce wildfire exposure. J. For. Res. 34, 373–386. doi: 10.1007/s11676-022-01504-2
Jahdi, R., Salis, M., Alcasena, F. J., Arabi, M., Arca, B., and Duce, P. (2020). Evaluating landscape-scale wildfire exposure in northwestern Iran. Nat. Hazards J. Int. Soc. Prev. Mitig. Nat. Hazards 101, 911–932. doi: 10.1007/s11069-020-03901-4
Kalabokidis, K., Ager, A., Finney, M., Athanasis, N., Palaiologou, P., and Vasilakos, C. (2016). AEGIS: a wildfire prevention and management information system. Nat. Hazards Earth Syst. Sci. 16, 643–661. doi: 10.5194/nhess-16-643-2016
Keeley, J. E., Bond, W. J., Bradstock, R. A., Pausas, J. G., and Rundel, P. W. (2012). Fire in Mediterranean ecosystems: ecology, evolution and management. New York: Cambridge University Press.
Labenski, P., Ewald, M., Schmidtlein, S., Heinsch, F. A., and Fassnacht, F. E. (2023). Quantifying surface fuels for fire modelling in temperate forests using airborne lidar and Sentinel-2: potential and limitations. Remote Sens. Environ. 295:113711. doi: 10.1016/j.rse.2023.113711
Mallinis, G., Mitsopoulos, I., Beltran, E., and Goldammer, J. (2016). Assessing wildfire risk in cultural heritage properties using high spatial and temporal resolution satellite imagery and spatially explicit fire simulations: the case of holy Mount Athos, Greece. Forests 7:46. doi: 10.3390/f7020046
Marino, E., Ranz, P., Tomé, J. L., Noriega, M. A., Esteban, J., and Madrigal, J. (2016). Generation of high-resolution fuel maps from discrete airborne laser scanner data and Landsat-8 OLI: a low-cost and highly updated methodology for large areas. Remote Sens. Environ. 187, 267–280. doi: 10.1016/j.rse.2016.10.020
Marino, E., Tomé, J. L., Hernando, C., Guijarro, M., and Madrigal, J. (2022). Transferability of airborne LiDAR data for canopy fuel mapping: effect of pulse density and model formulation. Fire 5:126. doi: 10.3390/fire5050126
Marlon, J. R., Bartlein, P. J., Carcaillet, C., Gavin, D. G., Harrison, S. P., Higuera, P. E., et al. (2008). Climate and human influences on global biomass burning over the past two millennia. Nat. Geosci. 1, 697–702. doi: 10.1038/ngeo313
Martin-StPaul, N., Pimont, F., Dupuy, J. L., Rigolot, E., Ruffault, J., Fargeon, H., et al. (2018). Live fuel moisture content (LFMC) time series for multiple sites and species in the French Mediterranean area since 1996. Ann. For. Sci. 75:57. doi: 10.1007/s13595-018-0729-3
Mauro, F., Hudak, A. T., Fekety, P. A., Frank, B., Temesgen, H., Bell, D. M., et al. (2021). Regional modeling of forest fuels and structural attributes using airborne laser scanning data in oregon. Remote Sens. 13:261. doi: 10.3390/rs13020261
Mell, W. E., Manzello, S. L., Maranghides, A., Butry, D., and Rehm, R. G. (2010). The wildland-urban interface fire problem—current approaches and research needs. Int. J. Wildland Fire 19, 238–251. doi: 10.1071/WF07131
Miller, C., and Ager, A. A. (2013). A review of recent advances in risk analysis for wildfire management. Int. J. Wildland Fire 22, 1–14. doi: 10.1071/WF11114
Mitsopoulos, I., Mallinis, G., and Arianoutsou, M. (2015). Wildfire risk assessment in a typical Mediterranean wildland–urban Interface of Greece. Environ. Manag. 55, 900–915. doi: 10.1007/s00267-014-0432-6
Molina-Terrén, D. M., Xanthopoulos, G., Diakakis, M., Ribeiro, L., Caballero, D., Delogu, G. M., et al. (2019). Analysis of forest fire fatalities in Southern Europe: Spain, Portugal, Greece and Sardinia (Italy). Int. J. Wildland Fire 28, 85–98. doi: 10.1071/wf18004
Monedero, S., Ramirez, J., and Cardil, A. (2019). Predicting fire spread and behaviour on the fireline. Wildfire analyst pocket: a mobile app for wildland fire prediction. Ecol. Model. 392, 103–107. doi: 10.1016/j.ecolmodel.2018.11.016
Montiel, C., and Kraus, D. T. (2010). Best practices of fire use: prescribed burning and suppression: fire programmes in selected case-study regions in Europe. (European Forest Institute: Porvoo, Finland).
Moreira, F., Viedma, O., Arianoutsou, M., Curt, T., Koutsias, N., Rigolot, E., et al. (2011). Landscape – wildfire interactions in southern Europe: implications for landscape management. J. Environ. Manag. 92, 2389–2402. doi: 10.1016/j.jenvman.2011.06.028
Nunes, M. C. S., Vasconcelos, M. J., Pereira, J. M. C., Dasgupta, N., Alldredge, R. J., and Rego, F. C. (2005). Land cover type and fire in Portugal: do fires burn land cover selectively? Landsc. Ecol. 20, 661–673. doi: 10.1007/s10980-005-0070-8
Oliveira, S., Moreira, F., Boca, R., San-Miguel-Ayanz, J., Pereira, J. M. C., Oliveira, S., et al. (2013). Assessment of fire selectivity in relation to land cover and topography: a comparison between Southern European countries. Int. J. Wildland Fire 23, 620–630. doi: 10.1071/WF12053
Oliveira, U., Soares-Filho, B., de Souza Costa, W. L., Gomes, L., Bustamante, M., and Miranda, H. (2021). Modeling fuel loads dynamics and fire spread probability in the Brazilian Cerrado. For. Ecol. Manag. 482:118889. doi: 10.1016/j.foreco.2020.118889
Oom, D., De, R. D., Pfeiffer, H., Branco, A., Ferrari, D., Grecchi, R., et al. (2022). Pan-European wildfire risk assessment, EUR 31160 EN. Luxembourg: Publications Office of the European Union.
OSM (2022). Open Str. Map OSM. Available at: https://www.geofabrik.de/ (Accessed June 6, 2023).
Palaiologou, P., Ager, A. A., Evers, C. R., Nielsen-Pincus, M., Day, M. A., and Preisler, H. K. (2019). Fine-scale assessment of cross-boundary wildfire events in the western United States. Nat. Hazards Earth Syst. Sci. 19, 1755–1777. doi: 10.5194/nhess-19-1755-2019
Palaiologou, P., Ager, A. A., Nielsen-Pincus, M., Evers, C., and Kalabokidis, K. (2018). Using transboundary wildfire exposure assessments to improve fire management programs: a case study in Greece. Int. J. Wildland Fire 27, 501–513. doi: 10.1071/WF17119
Palaiologou, P., Kalabokidis, K., Ager, A. A., and Day, M. A. (2020). Development of comprehensive fuel management strategies for reducing wildfire risk in Greece. Forests 11:789. doi: 10.3390/f11080789
Palaiologou, P., Kalabokidis, K., Day, M. A., Ager, A. A., Galatsidas, S., and Papalampros, L. (2022). Modelling fire behavior to assess community exposure in Europe: combining open data and geospatial analysis. ISPRS Int. J. Geo Inf. 11:198. doi: 10.3390/ijgi11030198
Parisien, M.-A., Dawe, D. A., Miller, C., Stockdale, C. A., and Armitage, O. B. (2020). Applications of simulation-based burn probability modelling: a review. Int. J. Wildland Fire 28, 913–926. doi: 10.1071/WF19069
Pausas, J. G., and Fernández-Muñoz, S. (2012). Fire regime changes in the Western Mediterranean Basin: from fuel-limited to drought-driven fire regime. Clim. Chang. 110, 215–226. doi: 10.1007/s10584-011-0060-6
Pellizzaro, G., Duce, P., Ventura, A., Zara, P., Pellizzaro, G., Duce, P., et al. (2007). Seasonal variations of live moisture content and ignitability in shrubs of the Mediterranean Basin. Int. J. Wildland Fire 16, 633–641. doi: 10.1071/WF05088
Pereira, M. G., Parente, J., Amraoui, M., Oliveira, A., and Fernandes, P. M. (2020). “3 - the role of weather and climate conditions on extreme wildfires” in Extreme wildfire events and disasters. eds. F. Tedim, V. Leone, and T. K. McGee (Amsterdam: Elsevier), 55–72.
Pesaresi, S., Galdenzi, D., Biondi, E., and Casavecchia, S. (2014). Bioclimate of Italy: application of the worldwide bioclimatic classification system. J. Maps 10, 538–553. doi: 10.1080/17445647.2014.891472
Pimont, F., Fargeon, H., Opitz, T., Ruffault, J., Barbero, R., Martin-StPaul, N., et al. (2021). Prediction of regional wildfire activity in the probabilistic Bayesian framework of Firelihood. Ecol. Appl. 31:e02316. doi: 10.1002/eap.2316
Pimont, F., Ruffault, J., Opitz, T., Fargeon, H., Barbero, R., Castel-Clavera, J., et al. (2022). Future expansion, seasonal lengthening and intensification of fire activity under climate change in southeastern France. Int. J. Wildland Fire 32, 4–14. doi: 10.1071/WF22103
Plucinski, M. P. (2019). Fighting flames and forging firelines: wildfire suppression effectiveness at the fire edge. Curr. Forestry Rep. 5, 1–19. doi: 10.1007/s40725-019-00084-5
Plucinski, M. P., McCarthy, G. J., Hollis, J. J., and Gould, J. S. (2012). The effect of aerial suppression on the containment time of Australian wildfires estimated by fire management personnel. Int. J. Wildland Fire 21, 219–229. doi: 10.1071/WF11063
PROMÉTHÉE 2 (2023). For. Fires database Mediterr. Area Fr. – 1998-2019. Available at: https://www.promethee.com/incendies (Accessed June 6, 2023).
Ramirez, J., Monedero, S., Silva, C. A., and Cardil, A. (2019). Stochastic decision trigger modelling to assess the probability of wildland fire impact. Sci. Total Environ. 694:133505. doi: 10.1016/j.scitotenv.2019.07.311
Rodrigues, M., Alcasena, F., Gelabert, P., and Vega-García, C. (2020). Geospatial modeling of containment probability for escaped wildfires in a Mediterranean region. Risk Anal. 40, 1762–1779. doi: 10.1111/risa.13524
Rodrigues, A., Ribeiro, C., Raposo, J., Viegas, D. X., and André, J. (2013). Effect of canyons on a fire propagating laterally over slopes. Front. Mech. Eng. 5:41. doi: 10.3389/fmech.2019.00041
Rothermel, R. C. (1972). A mathematical model for predicting fire spread in wildland fuels. Ogden, UT: USDA Forest Service, Intermountain Forest and Range Experiment Station.
Ruffault, J., Curt, T., Moron, V., Trigo, R. M., Mouillot, F., Koutsias, N., et al. (2020). Increased likelihood of heat-induced large wildfires in the Mediterranean Basin. Sci. Rep. 10:13790. doi: 10.1038/s41598-020-70069-z
Sá, A. C. L., Aparicio, B., Benali, A., Bruni, C., Salis, M., Silva, F., et al. (2022). Coupling wildfire spread simulations and connectivity analysis for hazard assessment: a case study in Serra da Cabreira, Portugal. Nat. Hazards Earth Syst. Sci. 22, 3917–3938. doi: 10.5194/nhess-22-3917-2022
Salis, M., Ager, A. A., Alcasena, F. J., Arca, B., Finney, M. A., Pellizzaro, G., et al. (2015). Analyzing seasonal patterns of wildfire exposure factors in Sardinia, Italy. Environ. Monit. Assess. 187:4175. doi: 10.1007/s10661-014-4175-x
Salis, M., Arca, B., Del Giudice, L., Palaiologou, P., Alcasena-Urdiroz, F., Ager, A., et al. (2021). Application of simulation modeling for wildfire exposure and transmission assessment in Sardinia, Italy. Int. J. Disaster Risk Reduct. 58:102189. doi: 10.1016/j.ijdrr.2021.102189
Salis, M., Del Giudice, L., Arca, B., Ager, A. A., Alcasena-Urdiroz, F., Lozano, O., et al. (2018). Modeling the effects of different fuel treatment mosaics on wildfire spread and behavior in a Mediterranean agro-pastoral area. J. Environ. Manag. 212, 490–505. doi: 10.1016/j.jenvman.2018.02.020
Salis, M., Del Giudice, L., Jahdi, R., Alcasena-Urdiroz, F., Scarpa, C., Pellizzaro, G., et al. (2022). Spatial patterns and intensity of land abandonment drive wildfire Hazard and likelihood in Mediterranean Agropastoral areas. Land 11:1942. doi: 10.3390/land11111942
Salis, M., Del Giudice, L., Robichaud, P. R., Ager, A. A., Canu, A., Duce, P., et al. (2019). Coupling wildfire spread and erosion models to quantify post-fire erosion before and after fuel treatments. Int. J. Wildland Fire 28, 687–703. doi: 10.1071/wf19034
Salis, M., Laconi, M., Ager, A. A., Alcasena, F. J., Arca, B., Lozano, O., et al. (2016). Evaluating alternative fuel treatment strategies to reduce wildfire losses in a Mediterranean area. For. Ecol. Manag. 368, 207–221. doi: 10.1016/j.foreco.2016.03.009
San-Miguel-Ayanz, J., de Rigo, D., Caudullo, G., Durrant, T. H., and Mauri, A. (2016). European atlas of forest tree species. Publication Office of the European Union: Luxembourg.
San-Miguel-Ayanz, J., Durrant, T., Boca, R., Maianti, P., Liberta, G., Oom, D., et al. (2023). Advance report on forest fires in Europe, Middle East and North Africa 2022, EUR 31479 EN. Publications Office of the European Union, Luxembourg.
Sardegna Geoportale (2023). Carta Uso Suolo 2008. Available at: http://www.sardegnageoportale.it/index.php?xsl=2420&s=40&v=9&c=14480&es=6603&na=1&n=100&esp=1&tb=14401 (Accessed June 6, 2023).
Schuh, B., Andronic, C., Derszniak-Noirjean, M., Gaupp-Berghausen, M., Hui Hsiung, C., and Münch, A. (2020). Research for AGRI committee – The challenge of land abandonment after 2020 and options for mitigating measures. Brussels: European Parliament, Policy Department for Structural and Cohesion Policies.
Scott, J. H., and Burgan, R. E. (2005). Standard fire behavior fuel models: a comprehensive set for use with Rothermel’s surface fire spread model. Fort Collins, CO: USDA Forest Service, Rocky Mountain Research Station.
Scott, J. H., and Reinhardt, E. D. (2001). Assessing crown fire potential by linking models of surface and crown fire behavior. Ft. Collins, CO: U.S. Department of Agriculture, Forest Service, Rocky Mountain Research Station.
Scott, J. H., Short, K. C., Finney, M., Gilbertson-Day, J., and Vogler, K. C. (2018). FSim: the large-fire simulator — guide to best practices version 0.3.1.
Sil, Â., Fernandes, P. M., Rodrigues, A. P., Alonso, J. M., Honrado, J. P., Perera, A., et al. (2019). Farmland abandonment decreases the fire regulation capacity and the fire protection ecosystem service in mountain landscapes. Ecosyst. Serv. 36:100908. doi: 10.1016/j.ecoser.2019.100908
Spadoni, G. L., Moris, J. V., Vacchiano, G., Elia, M., Garbarino, M., Sibona, E., et al. (2023). Active governance of agro-pastoral, forest and protected areas mitigates wildfire impacts in Italy. Sci. Total Environ. 890:164281. doi: 10.1016/j.scitotenv.2023.164281
Sullivan, A. L. (2009a). Wildland surface fire spread modelling, 1990–2007. 2: empirical and quasi-empirical models. Int. J. Wildland Fire 18, 369–386. doi: 10.1071/WF06142
Sullivan, A. L. (2009b). Wildland surface fire spread modelling, 1990–2007. 3: simulation and mathematical analogue models. Int. J. Wildland Fire 18, 387–403. doi: 10.1071/WF06144
Tedim, F., Leone, V., Amraoui, M., Bouillon, C., Coughlan, M. R., Delogu, G. M., et al. (2018). Defining extreme wildfire events: difficulties, challenges, and impacts. Fire 1:9. doi: 10.3390/fire1010009
Thompson, M. P., Gannon, B. M., Caggiano, M. D., O’Connor, C. D., Brough, A., Gilbertson-Day, J. W., et al. (2020). Prototyping a geospatial atlas for wildfire planning and management. Forests 11:909. doi: 10.3390/f11090909
Thompson, M. P., O’Connor, C. D., Gannon, B. M., Caggiano, M. D., Dunn, C. J., Schultz, C. A., et al. (2022). Potential operational delineations: new horizons for proactive, risk-informed strategic land and fire management. Fire Ecol. 18:17. doi: 10.1186/s42408-022-00139-2
Thompson, M. P., Rodríguez y Silva, F., Calkin, D. E., and Hand, M. S. (2017). A review of challenges to determining and demonstrating efficiency of large fire management. Int. J. Wildland Fire 26, 562–573. doi: 10.1071/WF16137
Tolhurst, K., Shields, B., and Chong, D. (2008). Phoenix: development and application of a bushfire risk management tool. Aust. J. Emerg. Manag. 23, 47–54. doi: 10.3316/agispt.20091124
Tonini, M., D’Andrea, M., Biondi, G., Degli Esposti, S., Trucchia, A., and Fiorucci, P. (2020). A machine learning-based approach for wildfire susceptibility mapping. The case study of the Liguria region in Italy. Geosciences 10:105. doi: 10.3390/geosciences10030105
Trucchia, A., D’Andrea, M., Baghino, F., Fiorucci, P., Ferraris, L., Negro, D., et al. (2020). PROPAGATOR: an operational cellular-automata based wildfire simulator. Fire 3:26. doi: 10.3390/fire3030026
Turco, M., Jerez, S., Augusto, S., Tarín-Carrasco, P., Ratola, N., Jiménez-Guerrero, P., et al. (2019). Climate drivers of the 2017 devastating fires in Portugal. Sci. Rep. 9:13886. doi: 10.1038/s41598-019-50281-2
Tymstra, C., Bryce, R. W., Wotton, B. M., Taylor, S. W., and Armitage, O. B. (2010). Development and structure of Prometheus: the Canadian wildland fire growth simulation model. Available at: http://cfs.nrcan.gc.ca/publications?id=31775 (Accessed April 27, 2023).
Vaillant, N. M., Ager, A. A., and Anderson, J. (2013). ArcFuels10 system overview. Portland, OR: U.S. Department of Agriculture, Forest Service, Pacific Northwest Research Station.
Valkó, O., and Deák, B. (2021). Increasing the potential of prescribed burning for the biodiversity conservation of European grasslands. Curr. Opin. Environ. Sci. Heal. 22:100268. doi: 10.1016/j.coesh.2021.100268
Van Wagner, C. E. (1977). Conditions for the start and spread of crown fire. Can. J. For. Res. 7, 23–34. doi: 10.1139/x77-004
Varela, E., Górriz-Mifsud, E., Ruiz-Mirazo, J., and López-i-Gelats, F. (2018). Payment for targeted grazing: integrating local shepherds into wildfire prevention. Forests 9:464. doi: 10.3390/f9080464
Venäläinen, A., Ruosteenoja, K., Lehtonen, I., Laapas, M., Tikkanen, O.-P., and Peltola, H. (2022). “Climate change, impacts, adaptation and risk management” in Forest bioeconomy and climate change managing forest ecosystems. eds. L. Hetemäki, J. Kangas, and H. Peltola (Cham: Springer International Publishing), 33–53.
Viedma, O., Urbieta, I. R., and Moreno, J. M. (2018). Wildfires and the role of their drivers are changing over time in a large rural area of west-central Spain. Sci. Rep. 8:17797. doi: 10.1038/s41598-018-36134-4
Viegas, D. X. (2004). Slope and wind effects on fire propagation. Int. J. Wildland Fire 13, 143–156. doi: 10.1071/WF03046
Viegas, D. X., and Simeoni, A. (2011). Eruptive behaviour of forest fires. Fire. Technol 47, 303–320. doi: 10.1007/s10694-010-0193-6
Keywords: wildfire modeling, burn probability, MTT algorithm, wildfire management, wildfire risk, human communities, Mediterranean areas
Citation: Salis M, Del Giudice L, Alcasena-Urdiroz F, Jahdi R, Arca B, Pellizzaro G, Scarpa C and Duce P (2023) Assessing cross-boundary wildfire hazard, transmission, and exposure to communities in the Italy-France Maritime cooperation area. Front. For. Glob. Change. 6:1241378. doi: 10.3389/ffgc.2023.1241378
Edited by:
Juan Hernandez, University of Lisbon, PortugalReviewed by:
Nuno Guiomar, University of Évora, PortugalMatthew Thompson, Rocky Mountain Research Station, Forest Service (USDA), United States
Beatriz Duguy Pedra, University of Barcelona, Spain
Copyright © 2023 Salis, Del Giudice, Alcasena-Urdiroz, Jahdi, Arca, Pellizzaro, Scarpa and Duce. This is an open-access article distributed under the terms of the Creative Commons Attribution License (CC BY). The use, distribution or reproduction in other forums is permitted, provided the original author(s) and the copyright owner(s) are credited and that the original publication in this journal is cited, in accordance with accepted academic practice. No use, distribution or reproduction is permitted which does not comply with these terms.
*Correspondence: Michele Salis, michele.salis@ibe.cnr.it