- 1Forest Research and Training Centre (FRTC), Pokhara, Nepal
- 2Center for Earth Systems Research and Sustainability (CEN) and IWS-World Forestry, University of Hamburg, Hamburg, Germany
- 3Friends of Nature, Nepal (FON), Kathmandu, Nepal
Introduction: Many factors, such as climate, topography, forest management, or tree/forest attributes, influence soil organic carbon (SOC) and above-ground tree biomass (AGTB). This study focuses on assessing relationship between various predictor variables and response variables (SOC and AGTB) in the perspective of climate change scenario. The study was conducted throughout in Nepal using forest resource assessment data (2010–2014).
Methods: Our study applied a random forest model to assess the status of SOC and AGTB under future climate change scenarios using 19 bioclimatic variables accompanied by other variables such as altitude, aspect, basal area, crown cover development status, distance to settlement forest types, number of trees, macro-topography, management regime, physiographic zones, slope, and soil depth. The study used 737 (70%) samples as a training data for model development while 312 (30%) samples as a testing data for model validation.
Results and discussion: The respective RMSE, RMSE% and adjusted R2 of the Random Forest Model for SOC estimation were found to be 9.53 ton/ha, 15% and 0.746 while same for the AGTB were 37.55 ton/ha, 21.74% and 0.743. Particularly, changes in temperature and precipitation showed an effect on the amount of SOC and AGTB in the projected scenario i.e., CMIP6, SSP2 4.5 for 2040–2060. The study found the amount of SOC decreased by 3.85%, while AGTB increased by 2.96% in the projected scenario. The proposed approach which incorporates the effect of bioclimatic variables can be a better option for understanding the dynamics of SOC and AGTB in the future using climatic variables.
1. Introduction
Forest ecosystems are the largest carbon reservoirs storing ∼2 billion tons of CO2 per year (UNDESA and UNFFS, 2021). The 2006 Intergovernmental Panel on Climate Change (IPCC) guidelines for the national greenhouse gas inventories indicate three major carbon pools (biomass, dead organic matter, and soil) in the forest ecosystem (Eggleston et al., 2006; IPCC, 2006). Most of the forest carbon is found in soil organic matter (45%) followed by living biomass (44%) i.e., above-ground tree biomass (AGTB) and root biomass and remaining in dead organic matter, i.e., in dead wood and litter (FAO, 2020).
Several climatic and edaphic factors influence forest carbon storage (Hofhansl et al., 2020). AGTB is influenced by altitude (Powell et al., 2010; Van der Laan et al., 2014; Rajput et al., 2017), temperature and precipitation (Yan et al., 2015), water availability, soil nitrogen content, and tree cover (Requena Suarez et al., 2021). Similarly, soil organic carbon (SOC) is affected by the amount of above-ground litter fall and root turnover (Andivia et al., 2016), temperature and precipitation (Sun et al., 2019), soil conditions and vegetation (Reyna-Bowen et al., 2019), species diversity (Gamfeldt et al., 2013), soil properties and moisture (Hounkpatin et al., 2018), altitude (Zinn et al., 2018), slope aspect, and soil depths (Zhu et al., 2017).
Climate change is contributing to global warming due to the steady increase in temperature since the 1960s (NOAA, 2023). It is projected to increase the severity of impacts in both the natural and human systems (IPCC, 2023). Climate change, rising temperature particularly, in the future has shown to have a negative effect on AGTB (Larjavaara et al., 2021; Li Y. et al., 2022) and SOC (Kirschbaum, 2000; Zhao et al., 2021) while a positive effect of the rising temperature on AGTB and SOC has also been studied under different climate change scenarios (Fu et al., 2017; Azian et al., 2022). The carbon sink of the forest is sensitive to CO2 emission change resulting from increasing temperature, hydrological changes, and forest dynamics (Hubau et al., 2020).
Efficient estimation of above ground biomass and soil organic carbon is crucial for the study of carbon dynamics in forest ecosystems. Different assessment methods for the estimation of AGTB and SOC have been carried out. The 2006 IPCC guidelines have provisioned simple to robust method for the estimation of above and below carbon in Tier 1, Tier 2 and Tier 3 categories (IPCC, 2006). Design-based estimation (using ground-based sample plots) is one of the most used approaches for estimating AGTB and SOC (DFRS, 2014, 2015a,b; DFRS/FRA, 2014). Though it provides the precise evaluation of changes (stand structure, tree attributes) due to small standard error (Schadauer and Gabler, 2007), it is time-consuming, less cost- effective and difficult to implement in poorly accessible forest areas (Köhl et al., 2011; Kandel, 2013). Alternatively, a regression model (model-based estimation) has been used for the estimation of AGTB and SOC (Tian et al., 2014; Mohd Zaki et al., 2016; Pokhre, 2018; Li et al., 2019; Malla et al., 2022) that allows more flexibility to provide estimates outside the sample plots (Ståhl et al., 2016). Thus, model based estimation (regression model) is cost-effective and also able to estimate target variables of poorly accessible areas.
Recently, several studies have used a machine learning method such as random forest model (RFM) and gradient boosting (GB) for the prediction of AGTB and SOC (Powell et al., 2010; John et al., 2020; Lee et al., 2020; Li et al., 2020; López-Serrano et al., 2020; Nguyen and Kappas, 2020; Vorster et al., 2020). The RFM model uses machine learning algorithms for classification and regression based on decision trees (Jin et al., 2020). It is appropriate for large datasets with large numbers of variables, non-linear responses, both continuous and categorical variables and is less affected by the multicollinearity problem (Lu et al., 2016). Several studies found RFM superior to the regression model in terms of lowering mean squared error (Hounkpatin et al., 2018; Zhu et al., 2020; Xie et al., 2021), handling non-linear relations (Pahlavan Rad et al., 2014; Hengl et al., 2015), and indifference of assumptions of having probability distribution (normality) and no multicollinearity among independent variables (Lu et al., 2016; López-Serrano et al., 2016). Moreover, RFM does not require several numbers of sample plots, as in the case of design-based estimation, thus it is cost-effective. It can also estimate the target variable of the poorly accessible area in the presence of readily available independent variables (i.e., temperature, precipitation, slope, altitude, etc.).
Previous studies have used spectral values of satellite images as an independent variable to predict a response variable such as AGTB and SOC in the past period (Powell et al., 2010; Vicharnakorn et al., 2014; Angelopoulou et al., 2019; López-Serrano et al., 2020; Zhu et al., 2020; Kumar et al., 2022). However, the response of AGTB and SOC against change in climatic variables (temperature and precipitation) in the future has been lacking in the national scenario in Nepal. The influence of temperature and precipitation on the quantity of AGTB and SOC (Mehta et al., 2014; Bennett et al., 2020; Saimun et al., 2021) helps estimate these target variables in future climate change scenarios. Therefore, this study aims to answer the questions (1) Which are the variables (topographic, forest variables and climatic) significant to influence AGTB and SOC? (2) Are these variables likely to contribute to the amount of AGTB and SOC under the climate change scenario? The study covered all the forest covers of Nepal using forest resource assessment data. A RFM was used to better examine the influence of climatic, topographic and forest variables on the amount of AGTB and SOC. The research will improve our understanding of how climate change affects AGTB and SOC in the forests.
2. Materials and methods
2.1. Study area
For this study, we selected Nepal (Map 1) as a study site due to its varied site conditions. In Nepal, hilly region occupies a higher chunk of the land (∼86% of the total land area) while lowland (less than 300 m altitude) occupies only 14%. Wide altitudinal variations (<300–8,848 m), resulting in diverse climatic conditions, have produced different physiographic zones, i.e., Terai and Siwalik (lowlands), Mid-hills, High mountains and High Himal (LRMP, 1986), which influence the composition of flora and fauna (HMGN/MFSC, 2002). Stainton (1972) classified 35 forest types in Nepal that were further broadly categorized into 10 major groups based on the altitudinal range (HMGN/MFSC, 2002).
The climate of Nepal varies seasonally. For the last 30 years (1991–2020), the average monthly temperature ranges from ∼ 5°C in January to ∼18°C in July, whereas average rainfall ranges from ∼20 mm in November to ∼340 mm in July (ADB and WB, 2021). Nepal is likely to experience a higher rate of warming in two future periods (2016–2045 and 2036–2065) compared to the reference period, i.e., 1981 to 2010 (GoN/MoFE, 2021) and spatiotemporal changes in precipitation over the period from 1981 to 2010 (Karki et al., 2017). Diverse current and future climatic conditions within comparatively small areas (Dawadi, 2017) make Nepal an ideal place to study the effects of climate change on forests.
2.2. Data collection
The primary data used in this study were obtained from the third national forest inventory (NFI), which was carried out during 2010–2014. The NFI adopted a two-phase systematic sampling design, composed of 450 clusters containing 1,553 Permanent Sample Plots (PSPs)-after excluding inaccessible PSPs - in the real ground (See Figure 1). Data were collected only from the accessible PSPs (slope up to 100 % or 45°). On the sample plots tree related attributes such as diameter at breast height (DBH) and tree height were recorded for the analysis of growing stock, above ground tree biomass and carbon. The third NFI is the first assessment in Nepal that collected soil samples to analyze the SOC of the forests. Four soil pits were established in a cardinal direction in each PSP to collect soil samples. At each cardinal direction, soil pits of appropriate size were dug within the 2 m * 2 m area size at a 21 m distance from the PSP center. In each soil pit, soil samples were collected from three different horizons (1–10 cm, 10–20 cm, and 20–30 cm) up to the depth of 30 cm and were mixed together resulting in 3 soil samples representing three different soil horizons in each PSP (DFRS/FRA, 2014).
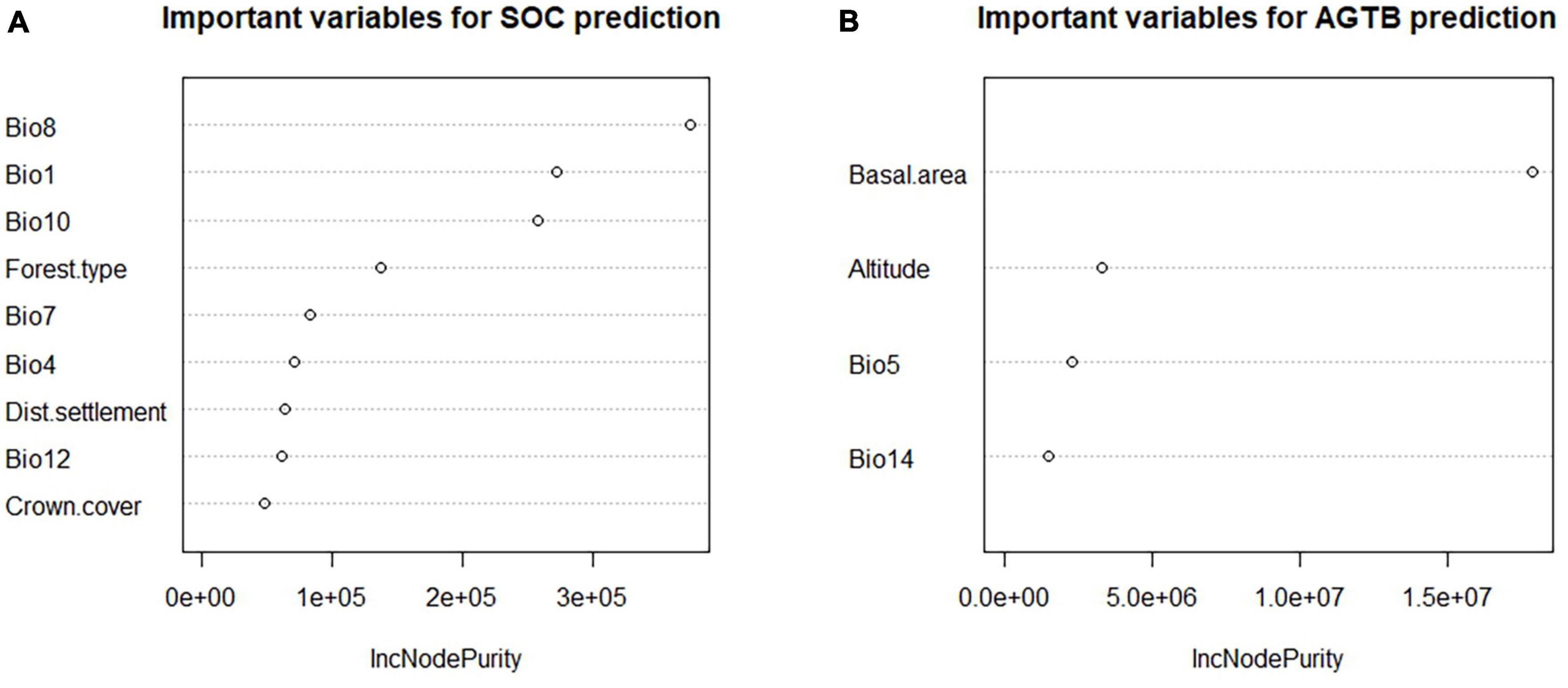
Figure 1. Order of variables based on its importance in the models for the prediction of SOC (A) and AGTB (B).
Besides forest inventory data, the study used 19 bioclimatic variables representing historic data (near current) representing average figures for the years 1970–2000 at 30 arc sec (∼1 km2) resolution (Fick and Hijmans, 2017). The study also used future climate data from the WorldClim data set1 at 30 arc sec (∼1 km2) resolution. representing Couple Modeled Inter-comparison Project Phase 6 (CMIP6) based on shared socio-economic pathways (SSP2 4.5) scenario from 2041 to 2060 (i.e., 2050 on average) with resulting global warming of 1.6 –2.5°C (IPCC, 2021). We used this scenario in the study because it is an intermediate scenario among five prescribed by Intergovernmental Panel on Climate Change (IPCC) and is based on the current level of CO2 emission until the middle of the century.
2.3. Soil organic carbon analysis
Altogether 1,049 PSPs out of 1,553 PSPs were used for SOC analysis. Data from 504 PSPs were removed for one or more of the factors: inappropriateness of the site condition e.g., presence of rock or boulder instead of soil, and missing data for important variables such as aspect, distance to settlement, etc. The Black wet combustion method (Walkley and Black, 1934) was applied in the Nepalese Department of Forest Research and Survey (DFRS) soil laboratory to analyze the SOC content. In addition, dry combustion and LECO CHN Analyzer were used in the Metla Soil Laboratory, Finland, to assure the quality of the laboratory test.
2.4. Above ground tree biomass analysis
Above-ground tree biomass was also estimated from the same PSPs used for SOC analysis. DBH of the tree greater than 5 cm was recorded from the PSPs. The stem volume of the tree was calculated using the equation given by Sharma and Pukkala (1990a).
where,
ln = Natural logarithm to the base 2.71828,
d = DBH in cm.
h = Total tree height in m.
a, b and c are parameters of the volume equation (Annex 1).
To get stem volume in a cubic meter, the model estimation must be divided by 1,000. According to Sharma and Pukkala (1990b), the air-dried wood densities of the tree species range from 352 kg/m3 for Trewia nudiflora L. to 960 kg/m3 for Acacia catechu (L.F.) wild.
In order to estimate AGTB, firstly stem biomass was calculated using following equation.
where,
Volume = Stem volume (m3).
Density = Air-dried wood density (kg/m3).
Branch biomass and foliage biomass of the trees were calculated using branch-to-stem and foliage-to-stem ratios, respectively based on tree species and three classes of the size of the stem (small = < 28 cm, medium = 28–53 cm and large = > 53 cm) at diameter at breast height (Sharma and Pukkala, 1990a). Finally, above ground tree biomass (AGTB) of each tree in the PSPs was calculated by using an equation (3). The individual tree biomass (Kg/m3) within PSP was calculated and it was further converted into ton/ha using the plot expansion factor.
2.5. Partition of data set
In order to have independent data sets for model development and model testing, the data were partitioned into two sets A total of 737 (70%) samples were used as training data and 312 (30%) were used as test data. The partitioning of the data was done by using the createDataPartition function in the “caret” package (Kuhn, 2008), which splits data randomly into two different sub-sets with different proportions.
2.6. Variables selection
Altogether 36 variables were identified for modeling purposes (Table 1). Out of these 36 variables, we conducted variable selection based on the importance of the variables in the model. To select the important variables, the function VSURF from the R package “VSURF” (Genuer et al., 2010) was used. This package selects important predictor variables for the model by step-wise analysis i.e., threshold, interpretation and prediction. Finally, the selected predictor variables were applied in the model development.
2.7. Estimation of SOC and AGTB using random forest model
Estimation of the SOC and AGTB was conducted (including all predictor variables and only important predictor variables) using a random forest model (RFM) by a function randomForest under the “randomForest” package in R software (version 4.2.1). RFM is a machine learning tool using bootstrap aggregating to develop models with an improved prediction (Jin et al., 2020). It is based on two parameters i.e., Number of predictor variables (Mtree) and the number of decision trees (Ntree). The random selection of predictor variables and the records in the data set to generate one decision tree helps to achieve higher accuracy in subsequent iterations. In this way, the RFM function generates many decision trees and averages to give an estimation for the response variable. Averaging a large number of decision trees helps to increase accuracy. Moreover, RFM generates IncNodePurity which is a total decrease in node impurities when splitting the predictor variables. An increase in the IncNodePurity value of the predictor variables indicates the higher importance of the variables. Furthermore, the partial dependence plot was plotted using the partialPlot function under the “randomForest” package in the R program. The plot shows the marginal effects of predictor variables on the response variable in the model (Friedman, 2001). It is generally used to evaluate whether the relationship between the predictor and response variable is linear, non-linear, or more complex.
2.8. Model validation
Observed data (test data) was plotted against predicted data (model output) to see their relationship for visual interpretation. Moreover, RMSE, RMSE% and R2 value was calculated to determine the efficiency of the model developed using the rmse function (“ModelMetrics” package), rmse_per function (“forestmangr” package) and summary function in the R program. The RMSE and RMSE% were calculated as follows.
Where,
i = the predicted SOC or AGTB on the ith plot,
yi = the observed SOC or AGTB on the ith plot,
= the average value of SOC or AGTB.
n = Number of samples.
3. Results
3.1. Variables used in the model
Altogether 35 independent variables were used for the prediction of SOC or AGTB in the study. Of which, nine variables were selected for the prediction of SOC (Bio1, Bio4, Bio7, Bio8, Bio10, Bio12, Forest type, Distance to settlement and Crown cover) and four variables for the prediction of AGTB (Basal area, Altitude, Bio5 and Bio14).
3.2. Variables importance in the model
The selected 9 and 4 Predictor variables for estimating SOC and AGTB, respectively showed different importance values in the models. The predictor variable “Bio8” was found to be the most important variable for the prediction of SOC followed by Bio1, Bio10, Forest type, Bio7, Bio4, Distance to settlement, Bio12 and Crown cover (Figure 1A) whereas Basal area showed its importance highest for the prediction of AGTB followed by Altitude, Bio5, and Bio14 (Figure 1B).
3.3. SOC and AGTB estimation
The random forest model was run in two ways. Firstly, all 35 predictor variables (RFM1 and RFM3) were used in the model (RMF1 and RMF3) for the estimation of SOC and AGTB. Secondly, only predictor variables with high-importance values were used in the model (RFM2 and RFM4) for the same estimation (Table 2). The root mean square error (RMSE), RMSE% and coefficient of determination (R2) were found similar for using all 35 predictor variables and using only 9 predictor variables for the estimation of SOC. On the other hand, the performance of the model for the estimation of AGTB was found slightly better while using 35 predictor variables compared to 4 predictor variables (Table 2).
3.4. Relation between number of decision trees and error in the model
The number of decision trees (or “trees”) in the Random forest model represents the number of sub-samples selected randomly from the original data set. Increasing the number of decision trees helps to reduce the error in the model. The error was sharply reduced when the number of sub-samples selected from the sample population increased from 1 to 100 and slowed down afterward in both the SOC (Figure 2A) and AGTB (Figure 2B) models.
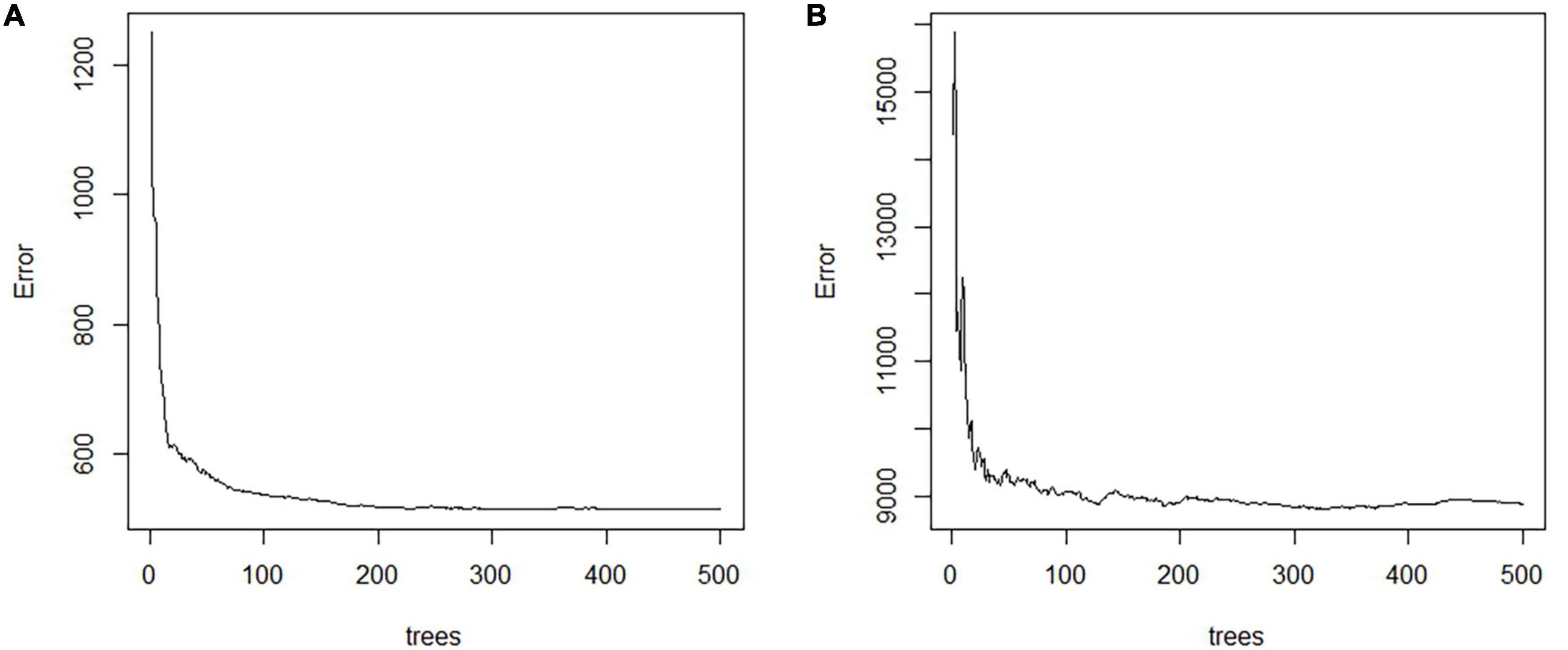
Figure 2. Reduction of error as the increase of number of decision trees (“trees”) in the RFM2 and RMF4 models for the estimation of SOC (A) and AGTB (B), respectively. “Trees” is a number of sub samples selected randomly from the sample population.
3.5. Accuracy assessment
Model performance varied in the estimation of SOC (RFM2) and AGTB (RFM4) using test data. RMSE% was found lower in the estimation of SOC as compared to the estimation of AGTB (Table 3).
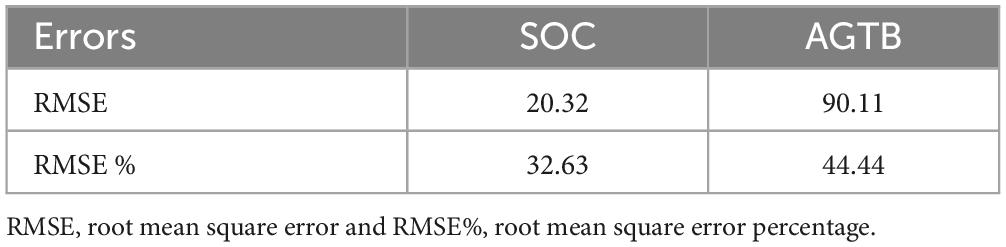
Table 3. Error assessment of the models (RFM2 and RFM4) developed to predict soil organic carbon (SOC) and above ground tree biomass (AGTB).
Moreover, the degree of fitness of the model calculated from the predicted value against the observed value for the estimation of SOC was found to be strong i.e., R2 = 0.759 and the relation was found significant (p < 0.05) (Figure 3A). A similar degree of fitness was also found in the case of AGTB estimation i.e., R2 = 0.762 and (p < 0.05) (Figure 3B).
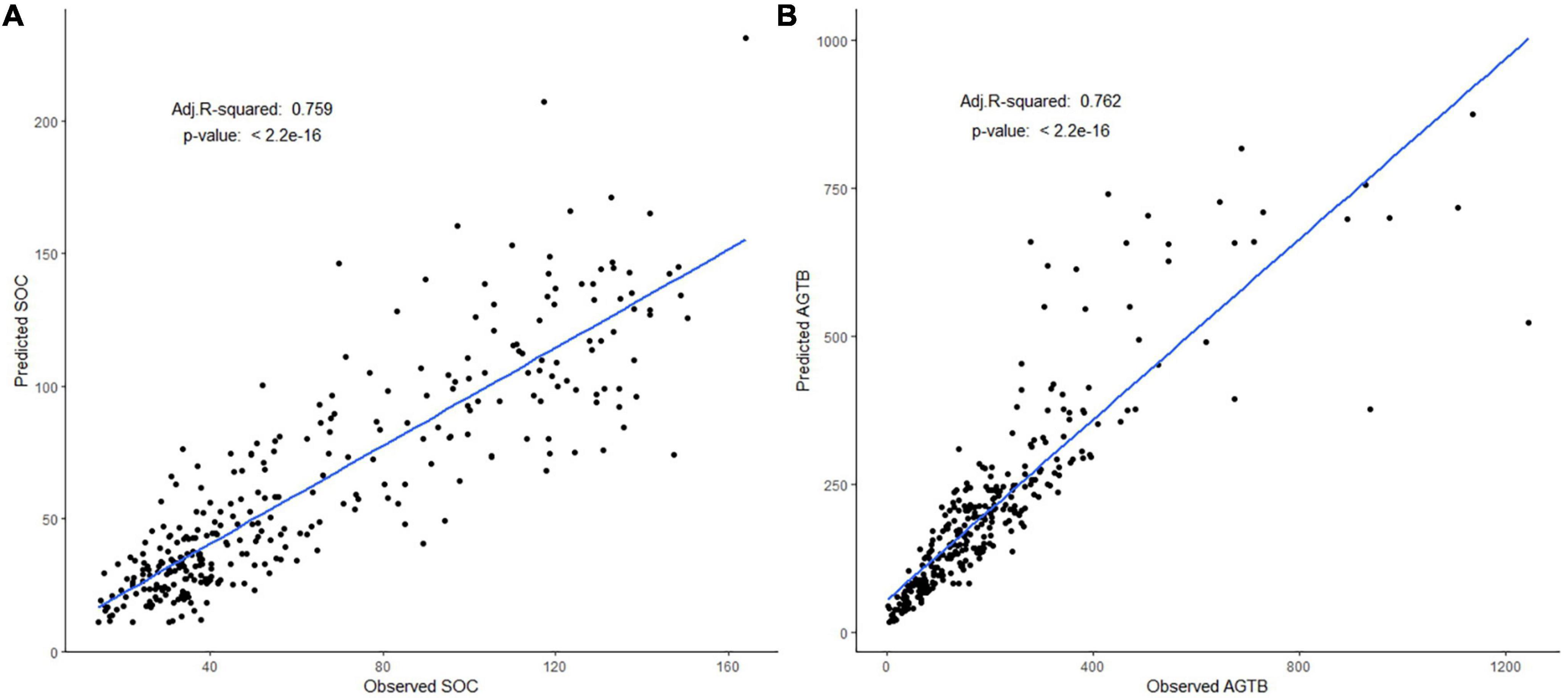
Figure 3. Validation of the models for Soil organic carbon (SOC) prediction (A) and Above ground tree biomass (AGTB) prediction (B) using predicted data and observed data with the help of independent data set.
3.6. Partial dependence plots (Response plots)
Partial dependence plots for each important predictor variable were plotted for both SOC (RFM2) and AGTB (RFM4) models. Our study found that the response variable SOC responded positively with Crown cover, Distance to settlement and Bio12, and responded negatively with Bio1, Bio7, Bio8 and Bio10, whereas it responded both ways (non-linear relation) with Bio4.
An increase in distance to settlement from the forests up to 8,000 m contributed to the increase in SOC, while for longer distances no effect on SOC was found. Similarly, an increase in crown cover and Bio12 also contributed to the increase in SOC. Furthermore, Bio1, Bio8 and Bio10 did not contribute to SOC up to the temperature of 12, 17, and 19°C, respectively. However, the increase in temperature after those limits contributed to a decrease in SOC. In contrast, Bio4 contributed to a decrease in SOC up to 500 mm and afterward, it contributed to an increase in SOC. Lastly, The comparison of forest types revealed that 1, 11, and 17 contributed more to SOC than the other forest types (Figure 4).
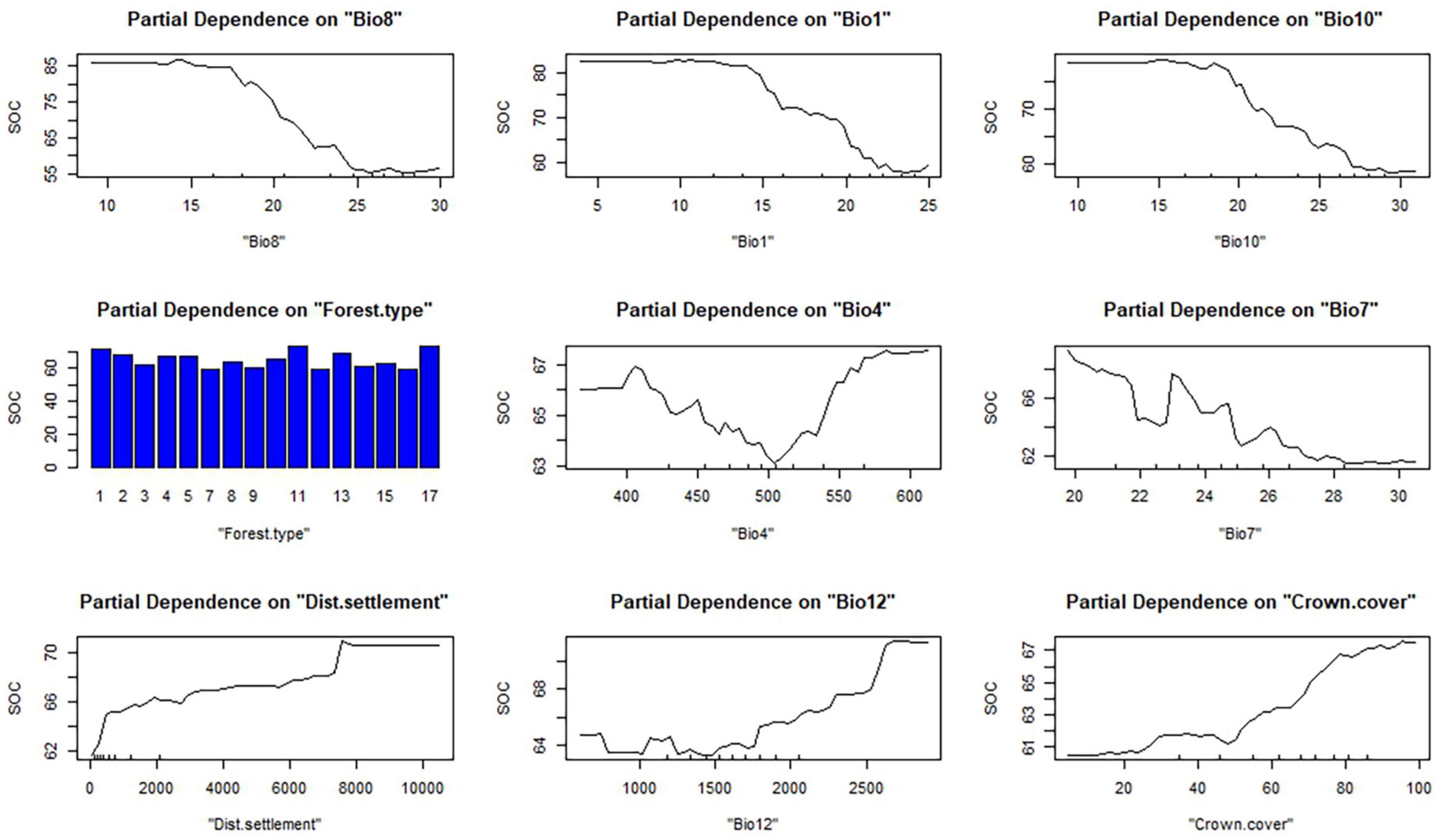
Figure 4. Predictor variables responding to Soil organic carbon (SOC) in the partial dependence plot of the random forest model (RFM2) where forest type represented by 1 = Abies spectabilis forest, 2 = Betula utilis forest, 3 = Cedrus deodara forest, 4 = Cupressus torulosa forest, 5 = Junifer wallichiana forest, 7 = Acacia catechu/Dalbergia sisso forest, 8 = Lower mixed hardwood (LMH) forest, 9 = Pinus roxburghii forest, 10 = Pinus wallichiana forest, 11 = Quercus sps forest, 12 = Shorea robusta forest, 13 = Picea smithiana forest, 14 = Shorea robusta TMH forest, 15 = Tsuga dumusa forest,16 = Terai mixed hardwood (TMH) forest, 17 = Upper mixed hardwood (UMH) forest.
Above-ground tree biomass responded differently with the four predicted variables (Basal area, Altitude, Bio5 and Bio14). Basal area and Bio5 showed a positive relation with AGTB, while Bio14 and Altitude showed both positive and negative (Figure 5). Basal area up to 80 m2/ha of the forests increased AGTB, and then the amount of AGTB stayed more or less stable, while an increase in Bio5 further increased AGTB. In contrast, altitude and Bio14 decreased AGTB up to 2,000 m and 7 mm, respectively, and after those limits, these variables increased AGTB.
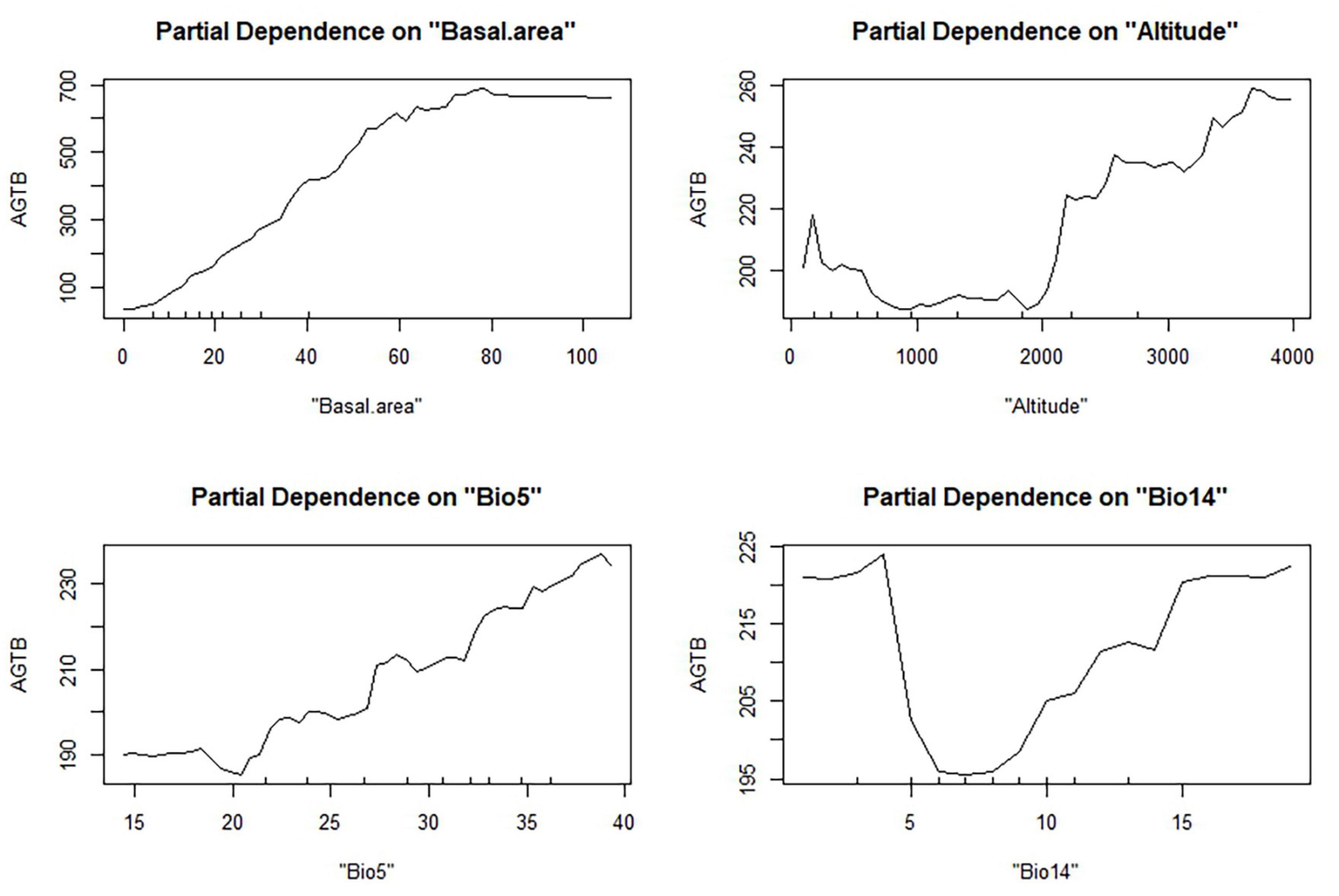
Figure 5. Predictor variables responding to above ground tree biomass (AGTB) in the partial dependence plot of the random forest model (RFM4).
3.7. Amount of soil organic carbon (SOC) and above ground tree biomass (AGTB) using climate change scenario (CMIP6, SSP2 4.5 for 2050)
The CMIP, SSP2 4.5 scenario showed an effect of climate change on SOC and AGTB, assuming other predictors to be the same. An average SOC stock of 63.6 tons/ha was found in the near current period, while it would decrease to 61.15 tons/ha in the future scenario. Unlikely, an average AGTB would increase to 210.57 tons/ha in the future scenario compared to the near current period (204.51 ton/ha). Our result shows that the amount of SOC would likely decrease by 3.85% while AGTB would likely increase by 2.96% in the future climate change scenario (Table 4).

Table 4. Changes in the amount of soil organic carbon (SOC) and above ground tree biomass (AGTB) in the near current period (1970–2000) and future scenario (2040–2060).
The SOC and AGTB were plotted over the individual PSP. The blue lines in both figures represent SOC/ATGB in the near current period (1970–2000) whereas red lines represent them in the future scenario (2040–2060). The blue line has exceeded the red line indicating decreasing trend of SOC in the future scenario (Figure 6A). But, for the amount of AGTB, a red line has exceeded the blue line indicating the trend of AGTB in the future (Figure 6B).
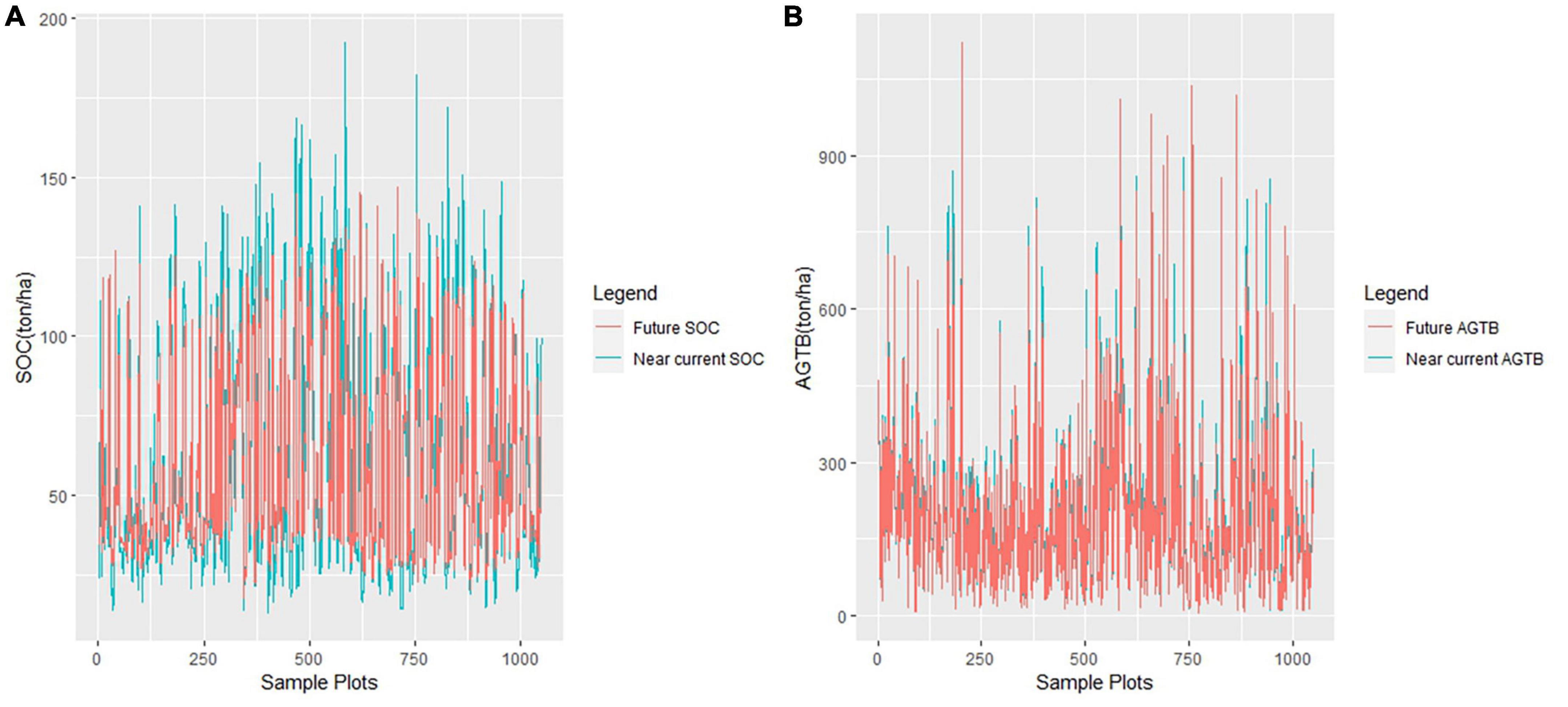
Figure 6. Amount of Soil organic carbon (SOC) changes in the future against near current period i.e., 1970–2000 (A) and amount of Above ground tree biomass (AGTB) changes in the future against near current scenario (B).
4. Discussion
4.1. Performance of the random forest models
A random forest model has been used in this study to estimate SOC and AGTB in the current and future climate change scenario. The RFM has been popular and considered to produce better accuracy than the multiple linear regression (Powell et al., 2010; Hounkpatin et al., 2018). The multiple linear regression approach is though popular, it does not well capture the complex relationships between the forest variables; and soil-landscape relationships subject to non-linear dynamics (Grimm et al., 2008; Chen et al., 2012). The coefficient of determination (R2 value) produced by our model for the estimation of AGTB is found strong, i.e., 0.74, which is higher than or similar to the other previous studies that used different predictor variables to predict AGTB using RFM (Powell et al., 2010; López-Serrano et al., 2020; Nguyen and Kappas, 2020; Li Z. et al., 2022). Similarly, the RMSE percent of the AGTB model in our study is slightly higher than the results reported by Musthafa and Singh (2022), Wai et al. (2022) and slightly lower than result of Zhu et al. (2020). These studies completely used other predictors (Image pixel value, age, crown density etc.) compared to our studies (especially temperature and precipitation). Moreover, R2 and RMSE% of the model for the estimation of SOC is smaller and higher, respectively than other studies (Hounkpatin et al., 2018; Lee et al., 2020). The possible reason could be the use of different independent variables in those studies than our study.
If we compare the estimated quantity of SOC and AGTB of the Random forest model with the forest resource assessment result (DFRS, 2015c) based on design based estimation, the quantity is found similar. The estimated average of SOC (63.6 ton/ha) in this study is 4.9% lower than the forest resource assessment result (66.88 ton/ha) whereas the average of AGTB (204.51 ton/ha) is 5.14% higher than the forest inventory result (i.e., 194.51 ton/ha). Though number of samples used in the model is lower than the samples used in design based approach, the Random forest model seems to be capable to produce better accuracy.
4.2. Factors influencing above ground tree biomass (AGTB)
Based on the previous studies, altitude, stand characteristics (tree age, density), slope, aspect, temperature and precipitation affect the AGTB (Powell et al., 2010; Van der Laan et al., 2014; Yan et al., 2015; Zhang et al., 2016; Rajput et al., 2017; Shen et al., 2018). Similar to the other studies (Wang et al., 2017; Bennett et al., 2020; Larjavaara et al., 2021), our study reports the effect of climate attributes on AGTB, particularly due to the maximum temperature of the warmest month (Bio5) and precipitation of the driest month (Bio14).
The RFM used in this study helps understand AGTB as functions of predictors such as altitude and climatic variables. Previous studies also used RFM to estimate AGTB, but were confined to a few predictor variables such as image pixel value, canopy height, topography, vegetation indices, and texture feature (Li Z. et al., 2022; Musthafa and Singh, 2022; Wai et al., 2022).
Our model shows an increase of AGTB under future climate change scenarios, a finding that is consistent with the results reported by Day et al. (2008), Saeed et al. (2019), Wang et al. (2019). Temperature is the most determining climatic factor that helps in accumulation of tree biomass particularly in the growth season (Devi et al., 2020). Similarly, an increase in precipitation in the driest months (Bio14) helps increase AGTB by lengthening the growing season that supports plant growth (Vaganov et al., 1999). Our results show a positive effect of Bio14 and warmer in the summer (similar to Bio5) with AGTB is consistent with the study conducted by Lewis et al. (2013), Devi et al. (2020), Noguchi et al. (2022). Unlike the forests in Nepal, rising temperature is likely to decrease above-ground biomass in the old-growth tropical forests (Larjavaara et al., 2021).
4.3. Factors influencing soil organic carbon (SOC)
Nine predictor variables, including topographic variables, climatic variables, forest types, distance to settlement and crown cover, are important to influence SOC distribution. Previous studies also report similar influencing variables for SOC, topography (altitude, slope and aspect), above-ground biomass, basal area, canopy cover, climate and forest types (Kara et al., 2008; Song et al., 2012; Mohammad and Rasel, 2013; Liu et al., 2016; Bangroo et al., 2017; Chaturvedi and Sun, 2018; Jakšić et al., 2021; Shapkota and Kafle, 2021). Apart from other variables, distance to settlement has also an effect on SOC. Our result shows that an increase in distance to settlement- which is likely to reduce human disturbances- results increase in SOC stock (Figure 4). SOC distribution is likely to be more in the area with less human disturbance (Mehta et al., 2008; Eshaghi Rad et al., 2018). Human disturbance such as logging and tree harvest result in a decrease in soil carbon and organic matter (Latty et al., 2004; Moreno et al., 2007).
Our study shows the mean temperature of the wettest quarter (Bio8) as a major predictor variable to estimate SOC in particular. In general, climatic variables are dominating other variables for the prediction of SOC. Similar to our study, previous studies have reported the effect of climate (temperature and precipitation) on SOC (Chen et al., 2015; Alani et al., 2017; Sun et al., 2019; Odebiri et al., 2020; Fang et al., 2022). But, other studies also found altitude as a major variable for SOC prediction (Dieleman et al., 2013; Odebiri et al., 2020). This is also true because altitude though does not directly influence SOC but is an indicator of various climatic functions that govern different vegetation and soil formation processes (Hanawalt and Wittaker, 1976). Thus, altitude can be used as a proxy of climatic variables (Malla et al., 2022).
Furthermore, our model shows a decrease in SOC amount in the future climate change scenario which is similar to the finding reported by Dimobe et al. (2018). Owing to global warming, surface temperature will continue to increase, at least, until 2050 under all emission scenarios (IPCC, 2021). The result shows an increase in temperature (in the future scenario) leads to a decrease in SOC amount, which is supported by other studies (Liu et al., 2021; Zhao et al., 2021). The possible reason could be an increase of soil microbial decomposition due to higher temperature resulting less SOC amount (Dong et al., 2021; Song et al., 2021). Similarly, the negative association of precipitation (in the future scenario) with SOC in our result is similar to the result reported by Alani et al. (2017). The higher amount of precipitation possibly causes to leach dissolved organic carbon of the soil resulting less SOC accumulation.
4.4. Implications of the study
4.4.1. Model implications
Our model shows the effect of climatic variables, topographic variables, forest variables, and distance to settlements on the amount of SOC and AGTB. Particularly, climatic variables (temperature and precipitation) have a direct relation with the formation process of SOC and AGTB. Mean annual precipitation is a driver of the amount of SOC and AGTB (Mehta et al., 2014). Precipitation influences soil moisture and hydrological processes (Heisler and Weltzin, 2006) which is an important factor in SOC cycling (Aanderud et al., 2010) and affects AGTB through functional traits (Cheng et al., 2021). Similarly, temperature also affects the amount of SOC (Zinn et al., 2018; Zhang et al., 2021) and the amount of AGTB (Poudel et al., 2011; Larjavaara et al., 2021). An increase in temperature helps soil microbial decomposition resulting in higher carbon emission or lower SOC accumulation (Dong et al., 2021; Song et al., 2021) whereas warming temperature enhances tree growth resulting in an increase in AGTB (Way and Oren, 2010).
However, most of the previous studies were focused on forest inventory data accompanied by satellite imageries to estimate AGTB and SOC of the latest period (Angelopoulou et al., 2019; López-Serrano et al., 2020). But for the future prediction of AGTB and SOC under climate change scenario, projected bioclimatic variables are necessary as input variables to produce a precise result. These projected bioclimatic variables have been widely used in species distribution modeling, and habitat suitability under different climate change scenarios (Fyllas et al., 2022; Khan et al., 2022; Shrestha et al., 2022) however, the use of these variables have been very limited for SOC prediction (Liu et al., 2021; Zhao et al., 2021).
Inclusion of Bio2 and Bio6 bioclimatic variables with inventory data helps estimate AGTB and SOC, respectively in a better way. Readily available bioclimatic variables not only improve the performance of the model but also reduce the cost of the model. Combining bioclimatic variables with other variables for the prediction of SOC and AGTB can be a viable option to understand the present scenario.
Moreover, using easily available projected bioclimatic variables under different climate change scenarios see text footnote 1 has benefited us in getting a better understanding the trend of SOC and AGTB in the future. Thus, our model shows an advantage over previous model to assess AGTB and SOC in the future climate change scenario using freely available climatic data.
4.4.2. Implications to Nepal
The forest policy of Nepal emphasizes managing forest resources largely through community participation. Almost half of the total forests have been managed under the broad regime of community-based forest management (Ghimire and Lamichhane, 2020). After the involvement of local people in forest resource management, Nepal has received positive changes in the forest condition. The forest cover of Nepal has been in an increasing trend reported by different assessments, i.e., 29% (DFRS, 1999), 40.36% (DFRS, 2015c), 41.69% (FRTC, 2022). Despite these facts, our model shows the amount of SOC is likely to be decreased in the future, whereas there will be a slight gain in the AGTB. In order to increase SOC in the future, the result highlights the need of management intervention to reduce forest degradation and deforestation through sustainable forest management in all the forests of Nepal to deal with climate change impact.
5. Conclusion
Climatic variables (temperature and precipitation) show an effect on the amount of SOC and AGTB in the future climate change scenario. However, the effect of climate on the SOC and AGTB is opposite (positive with AGTB while negative with SOC). Therefore, management intervention through sustainable forest management is crucial in all forest types to maintain SOC level in the future climate change scenario.
Our study proposed an approach for estimating the AGTB and SOC of Nepal using forest inventory data combined with world climate data (bioclimatic variables). Integrating readily available bioclimatic variables along with other predictor variables helps estimate SOC and AGTB in the near current and future scenario, leading to a better understanding of AGTB and SOC dynamics.
Data availability statement
The datasets presented in this article are not readily available because data sets are available from Forest Research and Training Center, Kathmandu, Nepal upon the request of the researchers, students or institutions. Requests to access the datasets should be directed to Forest Research and Training Center, info@frtc.gov.np.
Author contributions
RM contributes on data acquisition, data analysis and drafting manuscript. PN and MK contribute from draft stage to the final stage of the manuscripts. All authors discussed and revised the manuscript and read and approved the final manuscript.
Funding
This research was partially funded by the Deutsche Forschungsgemeinschaft (DFG, German Research Foundation) under Germany’s Excellence Strategy–EXC 2037 ‘CLICCS–Climate, Climatic Change, and Society’–Project Number: 390683824, contribution to the Center for Earth System Research and Sustainability (CEN) of Universität Hamburg.
Acknowledgments
We thank FRTC, Kathmandu for the provision of data, Sudiksha Joshi, Ph.D (USA) for proofreading, and the reviewers for their constructive comments and suggestions.
Conflict of interest
The authors declare that the research was conducted in the absence of any commercial or financial relationships that could be construed as a potential conflict of interest.
Publisher’s note
All claims expressed in this article are solely those of the authors and do not necessarily represent those of their affiliated organizations, or those of the publisher, the editors and the reviewers. Any product that may be evaluated in this article, or claim that may be made by its manufacturer, is not guaranteed or endorsed by the publisher.
Footnotes
References
Aanderud, Z. T., Richards, J. H., Svejcar, T., and James, J. J. (2010). A shift in seasonal rainfall reduces soil organic carbon storage in a cold desert. Ecosystems 13, 673–682. doi: 10.1007/s10021-010-9346-1
Alani, R., Odunuga, S., Andrew-Essien, N., Appia, Y., and Muyiolu, K. (2017). Assessment of the effects of temperature, precipitation and altitude on greenhouse gas emission from soils in Lagos metropolis. J. Environ. Protect. 08, 98–107. doi: 10.4236/jep.2017.81008
Andivia, E., Rolo, V., Jonard, M., Formánek, P., and Ponette, Q. (2016). Tree species identity mediates mechanisms of top soil carbon sequestration in a Norway spruce and European beech mixed forest. Ann. For. Sci. 73, 437–447. doi: 10.1007/s13595-015-0536-z
Angelopoulou, T., Tziolas, N., Balafoutis, A., Zalidis, G., and Bochtis, D. (2019). Remote sensing techniques for soil organic carbon estimation: A review. Remote Sens. 11, 1–18. doi: 10.3390/rs11060676
Azian, M., Nizam, M., Nik-Norafida, N., Ismail, P., Samsudin, M., and Noor-Farahanizan, Z. (2022). Projection of soil carbon changes and forest productivity for 100 years in Malaysia using dynamic vegetation model Lund-Potsdam-Jena. J. Trop. For. Sci. 34, 275–284. doi: 10.26525/jtfs2022.34.3.275
Bangroo, S. A., Najar, G. R., and Rasool, A. (2017). Effect of altitude and aspect on soil organic carbon and nitrogen stocks in the Himalayan Mawer Forest Range. Catena 158, 63–68. doi: 10.1016/j.catena.2017.06.017
Bennett, A. C., Penman, T. D., Arndt, S. K., Roxburgh, S. H., and Bennett, L. T. (2020). Climate more important than soils for predicting forest biomass at the continental scale. Ecography 43, 1692–1705. doi: 10.1111/ecog.05180
Chaturvedi, S. S., and Sun, K. (2018). Soil organic carbon and carbon stock in community forests with varying altitude and slope aspect in Meghalaya, India. Glob. Change Biol. 7:6.
Chen, G., Hay, G. J., and St-Onge, B. (2012). A GEOBIA framework to estimate forest parameters from lidar transects, Quickbird imagery and machine learning: A case study in Quebec, Canada. Int. J. Appl. Earth Observ. Geoinf. 15, 28–37. doi: 10.1016/j.jag.2011.05.010
Chen, X., Zhang, D., Liang, G., Qiu, Q., Liu, J., Zhou, G., et al. (2015). Effects of precipitation on soil organic carbon fractions in three subtropical forests in southern China. J. Plant Ecol. 9, 10–19. doi: 10.1093/jpe/rtv027
Cheng, H., Gong, Y., and Zuo, X. (2021). Precipitation variability affects aboveground biomass directly and indirectly via plant functional traits in the desert steppe of Inner Mongolia, Northern China. Front. Plant Sci. 12:674527. doi: 10.3389/fpls.2021.674527
Dawadi, B. (2017). Climatic records and linkage along an altitudinal gradient in the southern slope of Nepal Himalaya. J. Nepal Geol. Soc. 53, 47–56. doi: 10.3126/jngs.v53i0.23804
Day, T. A., Ruhland, C. T., and Xiong, F. S. (2008). Warming increases aboveground plant biomass and C stocks in vascular-plant-dominated Antarctic tundra. Glob. Change Biol. 14, 1827–1843. doi: 10.1111/j.1365-2486.2008.01623.x
Devi, N. M., Kukarskih, V. V., Galimova, ÀA., Mazepa, V. S., and Grigoriev, A. A. (2020). Climate change evidence in tree growth and stand productivity at the upper treeline ecotone in the Polar Ural Mountains. For. Ecosyst. 7:7. doi: 10.1186/s40663-020-0216-9
DFRS (1999). Forest resources of Nepal (1987–1998). Kathmandu: Department of Forest Research and Survey.
DFRS (2014). Churia forests of Nepal (2011-2013). Kathmandu: Department of Forest Research and Survey.
DFRS (2015a). High mountains and high Himalaya forests of Nepal. Kathmandu: Department of Forest Research and Survey.
DFRS (2015b). Middle mountains forests of Nepal: Forest resource assessment (FRA). Kathmandu: Department of Forest Research and Survey.
Dieleman, W. I. J., Venter, M., Ramachandra, A., Krockenberger, A. K., and Bird, M. I. (2013). Soil carbon stocks vary predictably with altitude in tropical forests: Implications for soil carbon storage. Geoderma 20, 59–67. doi: 10.1016/j.geoderma.2013.04.005
Dimobe, K., Kouakou, J. L. N., Tondoh, J. E., Zoungrana, B. J. B., Forkuor, G., and Ouédraogo, K. (2018). Predicting the potential impact of climate change on carbon stock in semi-arid West African Savannas. Land 7:124. doi: 10.3390/land7040124
Dong, X., Liu, C., Ma, D., Wu, Y., Man, H., Wu, X., et al. (2021). Organic carbon mineralization and bacterial community of active layer soils response to short-term warming in the great Hing’an Mountains of Northeast China. Front. Microbiol. 12:802213. doi: 10.3389/fmicb.2021.802213
Eggleston, S., Buendia, L., Miwa, K., Negara, T., and Tanabe, K. (2006). 2006 IPCC guidelines for national greenhouse gas inventories. Hayama: Institute for Global Environmental Strategies.
Eshaghi Rad, J., Valadi, G., Salehzadeh, O., and Maroofi, H. (2018). Effects of anthropogenic disturbance on plant composition, plant diversity and soil properties in oak forests, Iran. J. For. Sci. 64, 358–370. doi: 10.17221/13/2018-JFS
Fang, X., Lin Zhu, Y., Di Liu, J., Ping Lin, X., Zhao Sun, H., Hao Tan, X., et al. (2022). Effects of moisture and temperature on soil organic carbon decomposition along a vegetation restoration gradient of subtropical China. Forests 13, 1–16. doi: 10.3390/f13040578
FAO (2020). Global forest resource assessment 2020:Main report. Rome: FAO, doi: 10.4324/9781315184487-1
Fick, S. E., and Hijmans, R. J. (2017). WorldClim 2: New 1-km spatial resolution climate surfaces for global land areas. Int. J. Climatol. 37, 4302–4315. doi: 10.1002/joc.5086
Friedman, J. H. (2001). Greedy function approximation: A gradient boosting machine. Ann. Stat. 29, 1189–1232. doi: 10.1214/aos/1013203451
FRTC (2022). National Land Cover Monitoring System of Nepal. Kathmandu: Forest Research and Training Center.
Fu, L., Lei, X., Hu, Z., Zeng, W., Tang, S., Marshall, P., et al. (2017). Integrating regional climate change into allometric equations for estimating tree aboveground biomass of Masson pine in China. Ann. For. Sci. 74:42. doi: 10.1007/s13595-017-0636-z
Fyllas, N. M., Koufaki, T., Sazeides, C. I., Spyroglou, G., and Theodorou, K. (2022). Potential impacts of climate change on the habitat suitability of the dominant tree species in Greece. Plants 11:1616. doi: 10.3390/plants11121616
Gamfeldt, L., Snäll, T., Bagchi, R., Jonsson, M., Gustafsson, L., Kjellander, P., et al. (2013). Higher levels of multiple ecosystem services are found in forests with more tree species. Nat. Commun. 4:2328. doi: 10.1038/ncomms2328
Genuer, R., Poggi, J. M., and Tuleau-Malot, C. (2010). Variable selection using random forests. Pattern Recogn. Lett. 31, 2225–2236. doi: 10.1016/j.patrec.2010.03.014
Ghimire, P., and Lamichhane, U. (2020). Community based forest management in Nepal: Current status, successes and challenges. Grassroots J. Natl. Resour. 3, 16–29. doi: 10.33002/nr2581.6853.03022
GoN/MoFE (2021). Third National Communication to the United Nations. Kathmandu: Ministry of Forest and Soil Conservation (MFSC).
Grimm, R., Behrens, T., Märker, M., and Elsenbeer, H. (2008). Soil organic carbon concentrations and stocks on Barro Colorado Island — Digital soil mapping using Random Forests analysis. Geoderma 146, 102–113. doi: 10.1016/j.geoderma.2008.05.008
Hanawalt, R. B., and Wittaker, R. H. (1976). Altitudinally coordinated patterns of soils and vegetation in the San Jacinto Mountains, California. Soil Sci. 121, 114–124. doi: 10.1097/00010694-197602000-00007
Heisler, J. L., and Weltzin, J. F. (2006). Variability matters: Towards a perspective on the influence of precipitation on terrestrial ecosystems. N. Phytol. 172, 189–192. doi: 10.1111/j.1469-8137.2006.01876.x
Hengl, T., Heuvelink, G. B. M., Kempen, B., Leenaars, J. G. B., Walsh, M. G., Shepherd, K. D., et al. (2015). Mapping soil properties of Africa at 250 m resolution: Random forests significantly improve current predictions. PLoS One 10:e0125814. doi: 10.1371/journal.pone.0125814
HMGN/MFSC (2002). Nepal biodiversity strategy. Kathmandu: Ministry of Forests and Soil Conservation.
Hofhansl, F., Chacón-Madrigal, E., Fuchslueger, L., Jenking, D., Morera-Beita, A., Plutzar, C., et al. (2020). Climatic and edaphic controls over tropical forest diversity and vegetation carbon storage. Sci. Rep. 10:5066. doi: 10.1038/s41598-020-61868-5
Hounkpatin, O. K. L., Op, de Hipt, F., Bossa, A. Y., Welp, G., and Amelung, W. (2018). Soil organic carbon stocks and their determining factors in the Dano catchment (Southwest Burkina Faso). Catena 166, 298–309. doi: 10.1016/j.catena.2018.04.013
Hubau, W., Lewis, S. L., Phillips, O. L., Affum-Baffoe, K., Beeckman, H., Cuní-Sanchez, A., et al. (2020). Asynchronous carbon sink saturation in African and Amazonian tropical forests. Nature 579, 80–87. doi: 10.1038/s41586-020-2035-0
IPCC (2021). Climate Change 2021: The physical science basis – Summary for the Policymakers (Working Group I). Geneva: IPCC.
Jakšić, S., Ninkov, J., Milić, S., Vasin, J., Živanov, M., Jakšić, D., et al. (2021). Influence of slope gradient and aspect on soil organic carbon content in the region of Niš, Serbia. Sustainability 13:8332. doi: 10.3390/su13158332
Jin, Z., Shang, J., Zhu, Q., Ling, C., Xie, W., and Qiang, B. (2020). “RFRSF: Employee Turnover Prediction Based on Random Forests and Survival Analysis,” in Proceedings of the Lecture Notes in Computer Science (Including Subseries Lecture Notes in Artificial Intelligence and Lecture Notes in Bioinformatics), Amsterdam.
John, K., Isong, I. A., Kebonye, N. M., Ayito, E. O., Agyeman, P. C., and Afu, S. M. (2020). Using machine learning algorithms to estimate soil organic carbon variability with environmental variables and soil nutrient indicators in an alluvial soil. Land 9, 1–20. doi: 10.3390/land9120487
Kandel, P. (2013). Monitoring above-ground forest biomass: A comparison of cost and accuracy between LiDAR assisted multisource programme and field-based forest resource assessment in Nepal. Banko Janakari 23, 12–22. doi: 10.3126/banko.v23i1.9463
Kara, Ö, Bolat, I., Çakiroğlu, K., and Öztürk, M. (2008). Plant canopy effects on litter accumulation and soil microbial biomass in two temperate forests. Biol. Fertil. Soils 45, 193–198. doi: 10.1007/s00374-008-0327-x
Karki, R., Hasson, S., Schickhoff, U., Scholten, T., and Böhner, J. (2017). Rising precipitation extremes across Nepal. Climate 5:10004. doi: 10.3390/cli5010004
Khan, A. M., Li, Q., Saqib, Z., Khan, N., Habib, T., Khalid, N., et al. (2022). MaxEnt modelling and impact of climate change on habitat suitability variations of economically important Chilgoza Pine (Pinus gerardiana Wall.) in South Asia. Forests 13, 1–23. doi: 10.3390/f13050715
Kirschbaum, M. U. F. (2000). Will changes in soil organic carbon act as a positive or negative feedback on global warming? Biogeochemistry 48, 21–51. doi: 10.1023/A:1006238902976
Köhl, M., Lister, A., Scott, C. T., Baldauf, T., and Plugge, D. (2011). Implications of sampling design and sample size for national carbon accounting systems. Carbon Bal. Manage. 6, 1–20. doi: 10.1186/1750-0680-6-10
Kuhn, M. (2008). Building predictive models in R using the caret package. J. Stat. Softw. 28, 1–26. doi: 10.18637/jss.v028.i05
Kumar, M., Kumar, A., Thakur, T. K., Sahoo, U. K., Kumar, R., Konsam, B., et al. (2022). Soil organic carbon estimation along an altitudinal gradient of Chir-pine forests of Garhwal Himalaya, India: A Field Inventory to Remote Sensing Approach. Land Degrad. Dev. 33, 3387–3400. doi: 10.1002/ldr.4393
Larjavaara, M., Lu, X., Chen, X., and Vastaranta, M. (2021). Impact of rising temperatures on the biomass of humid old-growth forests of the world. Carbon Bal. Manage. 16, 1–9. doi: 10.1186/s13021-021-00194-3
Latty, E. F., Canham, C. D., and Marks, P. L. (2004). The effects of land-use history on soil properties and nutrient dynamics in northern hardwood forests of the Adirondack Mountains. Ecosystems 7, 193–207. doi: 10.1007/s10021-003-0157-5
Lee, S., Lee, S., Shin, J., Yim, J., and Kang, J. (2020). Assessing the carbon storage of soil and litter from national forest inventory data in South Korea. Forests 11, 1–15. doi: 10.3390/f11121318
Lewis, S. L., Sonké, B., Sunderland, T., Begne, S. K., Lopez-Gonzalez, G., van der Heijden, G. M. F., et al. (2013). Above-ground biomass and structure of 260 African tropical forests. Philos. Trans. R. Soc. B: Biol. Sci. 368:295. doi: 10.1098/rstb.2012.0295
Li, C., Li, Y., and Li, M. (2019). Improving forest aboveground biomass (AGB) estimation by incorporating crown density and using Landsat 8 OLI images of a subtropical forest in western Hunan in central China. Forests 10:104. doi: 10.3390/f10020104
Li, Y., Li, M., Li, C., and Liu, Z. (2020). Forest aboveground biomass estimation using Landsat 8 and Sentinel-1A data with machine learning algorithms. Sci. Rep. 10, 1–12. doi: 10.1038/s41598-020-67024-3
Li, Y., Li, M., and Wang, Y. (2022). Forest aboveground biomass estimation and response to climate change based on remote sensing data. Sustainability 14:14222. doi: 10.3390/su142114222
Li, Z., Bi, S., Hao, S., and Cui, Y. (2022). Aboveground biomass estimation in forests with random forest and Monte Carlo-based uncertainty analysis. Ecol. Indic. 142:109246. doi: 10.1016/j.ecolind.2022.109246
Liu, W., Zhu, M., Li, Y., Zhang, J., Yang, L., and Zhang, C. (2021). Assessing soil organic carbon stock dynamics under future climate change scenarios in the middle Qilian mountains. Forests 12:1698. doi: 10.3390/f12121698
Liu, Y., Li, S., Sun, X., and Yu, X. (2016). Variations of forest soil organic carbon and its influencing factors in east China. Ann. For. Sci. 73, 501–511. doi: 10.1007/s13595-016-0543-8
López-Serrano, P. M., Corral-Rivas, J. J., Díaz-Varela, R. A., Álvarez-González, J. G., and López-Sánchez, C. A. (2016). Evaluation of radiometric and atmospheric correction algorithms for aboveground forest biomass estimation using landsat 5 TM data. Remote Sens. 8:369. doi: 10.3390/rs8050369
López-Serrano, P. M., Domínguez, J. L. C., Corral-Rivas, J. J., Jiménez, E., López-Sánchez, C. A., and Vega-Nieva, D. J. (2020). Modeling of aboveground biomass with landsat 8 oli and machine learning in temperate forests. Forests 11, 1–18. doi: 10.3390/f11010011
LRMP (1986). Summary report: Land resources mapping project. Nepal: HMGN and Kenting Earth Sciences.
Lu, D., Chen, Q., Wang, G., Liu, L., Li, G., and Moran, E. (2016). A survey of remote sensing-based aboveground biomass estimation methods in forest ecosystems. Int. J. Digit. Earth 9, 63–105. doi: 10.1080/17538947.2014.990526
Malla, R., Neupane, P. R., and Köhl, M. (2022). Modelling soil organic carbon as a function of topography and stand variables. Forests 13:1391. doi: 10.3390/f13091391
Mehta, D. V. K., Sullivan, P. J., Walter, M. T., Krishnaswamy, J., and DeGloria, S. D. (2008). Impacts of disturbance on soil properties in a dry tropical forest in Southern India. Ecohydrology 1, 161–175. doi: 10.1002/eco.15
Mehta, N., Pandya, N. R., Thomas, V. O., and Krishnayya, N. S. R. (2014). Impact of rainfall gradient on aboveground biomass and soil organic carbon dynamics of forest covers in Gujarat, India. Ecol. Res. 29, 1053–1063. doi: 10.1007/s11284-014-1192-8
Mohammad, S., and Rasel, M. (2013). Effect of elevation and above ground biomass (AGB) on Soil Organic Carbon (SOC): A remote sensing based approach in Chitwan District, Nepal. Int. J. Sci. Eng. Res. 4, 1546–1553.
Mohd Zaki, N. A., Abd Latif, Z., Suratman, M. N., and Zainal, M. Z. (2016). Aboveground biomass and carbon stocks modelling using non-linear regression model. IOP Conf. Ser. Earth Environ. Sci. 37:12030. doi: 10.1088/1755-1315/37/1/012030
Moreno, G., Obrador, J. J., and García, A. (2007). Impact of evergreen oaks on soil fertility and crop production in intercropped dehesas. Agric. Ecosyst. Environ. 119, 270–280. doi: 10.1016/j.agee.2006.07.013
Musthafa, M., and Singh, G. (2022). Improving forest above-ground biomass retrieval using multi-Sensor L- and C- Band SAR data and multi-temporal spaceborne LiDAR Data. Front. For. Glob. Change 5:822704. doi: 10.3389/ffgc.2022.822704
Nguyen, T. D., and Kappas, M. (2020). Estimating the aboveground biomass of an evergreen broadleaf forest in Xuan Lien Nature Reserve, Thanh Hoa, Vietnam, using SPOT-6 data and the random forest algorithm. Int. J. For. Res. 2020:13. doi: 10.1155/2020/4216160
NOAA (2023). NOAA National Centers for Environmental information, Climate at a Glance: Global Time Series, published March 2023. Washington, DC: NOAA.
Noguchi, M., Hoshizaki, K., Matsushita, M., Sugiura, D., Yagihashi, T., Saitoh, T., et al. (2022). Aboveground biomass increments over 26 years (1993–2019) in an old-growth cool-temperate forest in northern Japan. J. Plant Res. 135, 69–79. doi: 10.1007/s10265-021-01358-5
Odebiri, O., Mutanga, O., Odindi, J., Peerbhay, K., Dovey, S., and Ismail, R. (2020). Estimating soil organic carbon stocks under commercial forestry using topo-climate variables in KwaZulu-Natal, South Africa. S. Afr. J. Sci. 116, 2–9. doi: 10.17159/sajs.2020/6339
Pahlavan Rad, M. R., Toomanian, N., Khormali, F., Brungard, C. W., Komaki, C. B., and Bogaert, P. (2014). Updating soil survey maps using random forest and conditioned Latin hypercube sampling in the loess derived soils of northern Iran. Geoderma 234, 97–106. doi: 10.1016/j.geoderma.2014.04.036
Pokhre, S. (2018). Assessment of above ground biomass and fire risk zonation in selected forest areas of Ludhikhola watershed, Gorkha Nepal. Remote Sens. Land 2, 47–64. doi: 10.21523/gcj1.18020104
Poudel, B. C., Sathre, R., Gustavsson, L., Bergh, J., Lundström, A., and Hyvönen, R. (2011). Effects of climate change on biomass production and substitution in north-central Sweden. Biomass Bioenergy 35, 4340–4355. doi: 10.1016/j.biombioe.2011.08.005
Powell, S. L., Cohen, W. B., Healey, S. P., Kennedy, R. E., Moisen, G. G., Pierce, K. B., et al. (2010). Quantification of live aboveground forest biomass dynamics with Landsat time-series and field inventory data: A comparison of empirical modeling approaches. Remote Sensing Environ. 114, 1053–1068. doi: 10.1016/j.rse.2009.12.018
Rajput, B. S., Bhardwaj, D. R., and Pala, N. A. (2017). Factors influencing biomass and carbon storage potential of different land use systems along an elevational gradient in temperate northwestern Himalaya. Agrofor. Syst. 91, 479–486. doi: 10.1007/s10457-016-9948-5
Requena Suarez, D., Rozendaal, D. M. A., De Sy, V., Gibbs, D. A., Harris, N. L., Sexton, J. O., et al. (2021). Variation in aboveground biomass in forests and woodlands in Tanzania along gradients in environmental conditions and human use. Environ. Res. Lett. 16, abe960. doi: 10.1088/1748-9326/abe960
Reyna-Bowen, L., Lasota, J., Vera-Montenegro, L., Vera-Montenegro, B., and Błońska, E. (2019). Distribution and factors influencing organic carbon stock in mountain soils in Babia Góra National Park, Poland. Appl. Sci. 9:1253. doi: 10.3390/app9153070
Saeed, S., Yujun, S., Beckline, M., Chen, L., Zhang, B., Ahmad, A., et al. (2019). Forest edge effect on biomass carbon along altitudinal gradients in Chinese Fir (Cunninghamia lanceolata): A study from Southeastern China. Carbon Manage. 10, 11–22. doi: 10.1080/17583004.2018.1537517
Saimun, M. S. R., Karim, M. R., Sultana, F., and Arfin-Khan, M. A. S. (2021). Multiple drivers of tree and soil carbon stock in the tropical forest ecosystems of Bangladesh. Trees For. People 5:100108. doi: 10.1016/j.tfp.2021.100108
Schadauer, K., and Gabler, K. (2007). Some approaches and designs of sample-based National Forest Inventories. Austrian J. For. Sci. 124, 105–133.
Shapkota, J., and Kafle, G. (2021). Variation in soil organic carbon under different forest types in Shivapuri Nagarjun National Park, Nepal. Scientifica 2021:1382687. doi: 10.1155/2021/1382687
Sharma, E. R., and Pukkala, T. (1990a). Volume equations and biomass prediction of forest trees in Nepal. Nepal: Forest Survey and Statistics Division.
Sharma, E. R., and Pukkala, T. (1990b). Volume tables for forest trees of Nepal. Nepal: Forest Survey and Statistics Division.
Shen, A., Wu, C., Jiang, B., Deng, J., Yuan, W., Wang, K., et al. (2018). Spatiotemporal variations of aboveground biomass under different terrain conditions. Forests 9:778. doi: 10.3390/f9120778
Shrestha, U. B., Lamsal, P., Ghimire, S. K., Shrestha, B. B., Dhakal, S., Shrestha, S., et al. (2022). Climate change– induced distributional change of medicinal and aromatic plants in the Nepal Himalaya. Ecol. Evolut. 12:e9204. doi: 10.1002/ece3.9204
Song, B., Niu, S., Zhang, Z., Yang, H., Li, L., and Wan, S. (2012). Light and heavy fractions of soil organic matter in response to climate warming and increased precipitation in a temperate steppe. PLoS One 7:e33217. doi: 10.1371/journal.pone.0033217
Song, Y., Liu, C., Song, C., Wang, X., Ma, X., Gao, J., et al. (2021). Linking soil organic carbon mineralization with soil microbial and substrate properties under warming in permafrost peatlands of Northeastern China. CATENA 203:105348. doi: 10.1016/j.catena.2021.105348
Ståhl, G., Saarela, S., Schnell, S., Holm, S., Breidenbach, J., Healey, S. P., et al. (2016). Use of models in large-area forest surveys: Comparing model-assisted, model-based and hybrid estimation. For. Ecosyst. 3:5. doi: 10.1186/s40663-016-0064-9
Sun, X., Tang, Z., Ryan, M. G., You, Y., and Sun, O. J. (2019). Changes in soil organic carbon contents and fractionations of forests along a climatic gradient in China. For. Ecosyst. 6, 1–12. doi: 10.1186/s40663-019-0161-7
Tian, X., Li, Z., Su, Z., Chen, E., van der Tol, C., Li, X., et al. (2014). Estimating montane forest above-ground biomass in the upper reaches of the Heihe River Basin using Landsat-TM data. Int. J. Remote Sensing 35, 7339–7362. doi: 10.1080/01431161.2014.967888
UNDESA and UNFFS. (2021). The Global Forest Goals Report. New York City, NY: United Nations Department of Economic and Social Affairs.
Vaganov, E. A., Hughes, M. K., Kirdyanov, A. V., Schweingruber, F. H., and Silkin, P. P. (1999). Influence of snowfall and melt timing on tree growth in subarctic Eurasia. Nature 400, 149–151. doi: 10.1038/22087
Van der Laan, C., Verweij, P. A., Quiñones, M. J., and Faaij, A. P. C. (2014). Analysis of biophysical and anthropogenic variables and their relation to the regional spatial variation of aboveground biomass illustrated for North and East Kalimantan, Borneo. Carbon Bal. Manage. 9:8. doi: 10.1186/s13021-014-0008-z
Vicharnakorn, P., Shrestha, R. P., Nagai, M., Salam, A. P., and Kiratiprayoon, S. (2014). Carbon stock assessment using remote sensing and forest inventory data in Savannakhet, Lao PDR. Remote Sens. 6, 5452–5479. doi: 10.3390/rs6065452
Vorster, A. G., Evangelista, P. H., Stovall, A. E. L., and Ex, S. (2020). Variability and uncertainty in forest biomass estimates from the tree to landscape scale: The role of allometric equations. Carbon Bal. Manage. 15, 1–20. doi: 10.1186/s13021-020-00143-6
Wai, P., Su, H., and Li, M. (2022). Estimating aboveground biomass of two different forest types in Myanmar from sentinel-2 data with machine learning and geostatistical algorithms. Remote Sens. 14:2146. doi: 10.3390/rs14092146
Walkley, A., and Black, I. A. (1934). An examination of the degtjareff method for determining soil organic matter, and a proposed modification of the chromic acid titration method. Soil Sci. 37, 29–38. doi: 10.1097/00010694-193401000-00003
Wang, W. J., He, H. S., Thompson, F. R., Fraser, J. S., and Dijak, W. D. (2017). Changes in forest biomass and tree species distribution under climate change in the northeastern United States. Landsc. Ecol. 32, 1399–1413. doi: 10.1007/s10980-016-0429-z
Wang, W. J., Thompson, F. R., He, H. S., Fraser, J. S., Dijak, W. D., and Jones-Farrand, T. (2019). Climate change and tree harvest interact to affect future tree species distribution changes. J. Ecol. 107, 1901–1917. doi: 10.1111/1365-2745.13144
Way, D. A., and Oren, R. (2010). Differential responses to changes in growth temperature between trees from different functional groups and biomes: A review and synthesis of data. Tree Physiol. 30, 669–688. doi: 10.1093/treephys/tpq015
Xie, X., Wu, T., Zhu, M., Jiang, G., Xu, Y., Wang, X., et al. (2021). Comparison of random forest and multiple linear regression models for estimation of soil extracellular enzyme activities in agricultural reclaimed coastal saline land. Ecol. Indic. 120:106925. doi: 10.1016/j.ecolind.2020.106925
Yan, F., Wu, B., and Wang, Y. (2015). Estimating spatiotemporal patterns of aboveground biomass using Landsat TM and MODIS images in the Mu Us Sandy Land, China. Agric. For. Meteorol. 200, 119–128. doi: 10.1016/j.agrformet.2014.09.010
Zhang, H., Song, T., Wang, K., Yang, H., Yue, Y., Zeng, Z., et al. (2016). Influences of stand characteristics and environmental factors on forest biomass and root–shoot allocation in southwest China. Ecol. Engineer. 91, 7–15. doi: 10.1016/j.ecoleng.2016.01.040
Zhang, Y., Ai, J., Sun, Q., Li, Z., Hou, L., Song, L., et al. (2021). Soil organic carbon and total nitrogen stocks as affected by vegetation types and altitude across the mountainous regions in the Yunnan Province, south-western China. Catena 196:104872. doi: 10.1016/j.catena.2020.104872
Zhao, F., Wu, Y., Hui, J., Sivakumar, B., Meng, X., and Liu, S. (2021). Projected soil organic carbon loss in response to climate warming and soil water content in a loess watershed. Carbon Bal. Manage. 16:24. doi: 10.1186/s13021-021-00187-2
Zhu, M., Feng, Q., Qin, Y., Cao, J., Li, H., and Zhao, Y. (2017). Soil organic carbon as functions of slope aspects and soil depths in a semiarid alpine region of Northwest China. Catena 152, 94–102. doi: 10.1016/j.catena.2017.01.011
Zhu, Y., Feng, Z., Lu, J., and Liu, J. (2020). Estimation of forest biomass in Beijing (China) using multisource remote sensing and forest inventory data. Forests 11, 1–17. doi: 10.3390/f11020163
Zinn, Y. L., Andrade, A. B., Araujo, M. A., and Lal, R. (2018). Soil organic carbon retention more affected by altitude than texture in a forested mountain range in Brazil. Soil Res. 56, 284–295. doi: 10.1071/SR17205
ANNEX
Keywords: biomass, carbon, climate change, random forest model, Nepal, precipitation, temperature
Citation: Malla R, Neupane PR and Köhl M (2023) Assessment of above ground biomass and soil organic carbon in the forests of Nepal under climate change scenario. Front. For. Glob. Change 6:1209232. doi: 10.3389/ffgc.2023.1209232
Received: 20 April 2023; Accepted: 22 August 2023;
Published: 05 September 2023.
Edited by:
Alessandra De Marco, Energy and Sustainable Economic Development (ENEA), ItalyReviewed by:
R. S. Rawat, Indian Council of Forestry Research and Education (ICFRE), IndiaHammad Gilani, Institute of Space Technology, Pakistan
Copyright © 2023 Malla, Neupane and Köhl. This is an open-access article distributed under the terms of the Creative Commons Attribution License (CC BY). The use, distribution or reproduction in other forums is permitted, provided the original author(s) and the copyright owner(s) are credited and that the original publication in this journal is cited, in accordance with accepted academic practice. No use, distribution or reproduction is permitted which does not comply with these terms.
*Correspondence: Rajesh Malla, raj_malla@yahoo.com