- 1Natural Resources Canada, Canadian Forest Service, Great Lakes Forestry Centre, Sault Ste. Marie, ON, Canada
- 2Natural Resources Canada, Canadian Forest Service, Northern Forestry Centre, Edmonton, AB, Canada
- 3Natural Resources Canada, Canadian Forest Service, Laurentian Forestry Centre, Québec, QC, Canada
- 4Hydro-Québec, Department of Environment, Montréal, QC, Canada
- 5Université du Québec à Rimouski, Department of Biology, Chemistry and Geography, Rimouski, QC, Canada
- 6Environment and Climate Change Canada, National Wildlife Research Centre, Ottawa, ON, Canada
In boreal forests of North America, land managers often carry out preventive treatments of forest fuel for the protection of human infrastructure from wildfires. However, these treatments may negatively affect other ecosystem services, such as the capacity to sustain wildlife populations. Here, we examine the efficacy of a strategy aimed at preserving a critical movement corridor for boreal woodland caribou (Rangifer tarandus caribou) in northern Québec, Canada, by raising high-voltage power line conductors above the forest canopy. To assess the interplay between the caribou protection objectives and a reduction in power line's exposure to wildfires, we developed an optimization model that combines the objectives of protecting the power line from wildfires via fuel treatments and maintaining a suitable movement corridor for caribou. The model combines a critical node detection (CND) problem with a habitat connectivity problem that allocates a minimum-resistance fixed-width habitat corridor between isolated wildlife refuges. Our results identify the best locations to perform fire fuel treatments to lessen the threat of fire damage to human infrastructure while maintaining a connectivity corridor for caribou in present and future climate scenarios. The selected fuel treatment locations aimed to mitigate wildfire exposure to a power line. In small-budget solutions, the exposure of power line infrastructure to wildfires was reduced by 36–39% in current climate conditions and by 20–31% in future climate, compared with no-treatment scenarios. Despite the detrimental effects of wildfire on both the industrial asset and caribou habitat, the approach provides strategies that help achieve a compromise between these two values. Such knowledge is timely to help mitigate the negative impacts of climate change on human livelihoods and natural ecosystems.
1. Introduction
Wildfires constitute the dominant natural disturbance agent in Canada, burning annually, on average, more than two million hectares (Hanes et al., 2019). Although it is a natural ecosystem process in many biomes, wildfires can cause significant damage to human infrastructure and industrial assets (Stocks et al., 2003; Williams and Bradstock, 2008; Thomas et al., 2017). Wildfires are thus recognized as a critical risk factor at the wildland–human interface (Theobald and Romme, 2007; Johnston and Flannigan, 2017). The protection of human infrastructure, such as settlements and power grids, is critical for maintaining public health and safety and economic productivity (Campbell and Lowry, 2012; Klinger et al., 2014). Decision-makers responsible for the protection of these values, including desired ecosystem services in areas of high wildfire risk, need tools to effectively reduce potential fire impacts to these values. Disrupting fuel continuity with fireguards, or through preventive fuel treatments, such as prescribed burns or targeted thinning of forest stands, aimed to reduce fire spread and intensity and hence the potential impacts to values of interest (e.g., human infrastructure). The ability of fuel treatments to successfully reduce the exposure to wildfires depends on many factors, such as fuel composition, weather, and the configuration and extent of treatments (Agee and Skinner, 2005; Barros et al., 2019). Planning fuel treatments for effective protection of values can be challenging due to the high cost of their practical implementation and insufficient economic resources available to decision-makers (Agee et al., 2000; Collins et al., 2010; Beverly et al., 2020).
Fuel treatments in forested ecosystems consist of modifications to the stand structure, composition, or both, to reduce fuel connectivity in the landscape around locations of concern and decrease their exposure to wildfires. However, the fragmentation and forest composition changes can potentially undermine other ecosystem services (Kalies and Kent, 2016). For instance, breaking the connectivity between forest patches may negatively affect wildlife species sensitive to habitat disturbances. This is the case for boreal populations of woodland caribou (Rangifer tarandus caribou), a species that is negatively affected by habitat loss and forest compositional changes that tend to favor its predators (such as gray wolves, Canis lupus) and increase competition with other large mammals, such as moose (Alces americanus) [James et al., 2004; Wittmer et al., 2005; Environment Canada (EC), 2012; Environment and Climate Change Canada (ECCC), 2017]. Woodland caribou is listed as threatened under the Canadian Species at Risk Act [Species at Risk Act (SARA), 2002] and vulnerable under Québec's Loi sur les espèces menacées ou vulnérables [Équipe de Rétablissement du Caribou Forestier du Québec (ÉRCFQ), 2013]. Recovery efforts to protect caribou populations are focused on landscape-level conservation of large areas of undisturbed old-growth coniferous forest and restoration of local habitat after disturbances (Rudolph et al., 2017; Lacerte et al., 2021, 2022), measures aimed at reducing the overlap with alternate prey species and predators [Environment Canada (EC), 2011].
Protecting extensive coniferous forest areas from human disturbance and preserving wildlife movement corridors with intact forest cover promote fuel continuity. Hence, the likelihood of large, high-intensity wildfires in such areas may also increase (Stockdale et al., 2019; Driscoll et al., 2021), leading to a conflict between caribou conservation and wildfire-protection objectives. Here, we consider a typical example of such conflict in the northeastern part of the province of Québec, Canada, where the public utility providing hydroelectricity in the province, Hydro-Québec, has committed to mitigate the impacts of a new 735-kV power line on caribou habitat connectivity in the vicinity of the Pipmuacan reservoir. Hydro-Québec has maintained a wildlife connectivity corridor within the footprint of this power line by raising the power line's conductors above the forest canopy, thus reducing the need for a forest clearing for ~10 km in an area used by caribou as a corridor between two areas of high-quality habitat (Dawe et al., 2022) (Figure 1). Retention of forest cover within this corridor is intended to facilitate animal movement across the power line, as linear features such as roads and pipelines are widely recognized as detrimental to caribou (Dyer et al., 2001, 2002; Vistnes and Nellemann, 2007; Lesmerises et al., 2013a). This forest retention under conductors contrasts with the standard practice of removing trees along the power line to minimize the risk of wildfire damage.
As climate will likely become more conducive to wildfire occurrence in Québec's boreal forest (Boulanger et al., 2014), the caribou corridor's exposure to large wildfires is anticipated to increase substantially (Dawe et al., 2022). There is thus a need to consider future conditions in wildfire mitigation strategies. The current and future threat of wildfires to power line infrastructure (Palm et al., 2022) can be mitigated using forest site treatments aimed to reduce the continuity of fuels, but at the risk of further fragmentation of critical caribou habitat, which would negate the benefits of the connectivity corridor. This necessitates a treatment plan that minimizes the exposure of human infrastructure to wildfires while causing the least possible impact to the quality and connectivity of caribou habitat.
Recently, optimization models have been proposed to prioritize fuel treatment decisions by minimizing fuel connectivity at the landscape scale (León et al., 2019; Matsyputa et al., 2019; Pais et al., 2021; Yemshanov et al., 2021a). In a related fashion, spatial optimization models have helped design habitat connectivity corridors by minimizing wildlife movement resistance (Dilkina et al., 2016; St. John et al., 2018; Yemshanov et al., 2022), estimating minimum-cost habitat corridors (Lai et al., 2011; Conrad et al., 2012), and controlling the habitat connectivity in a landscape using network optimization (Conrad et al., 2012; Dilkina et al., 2016; Yemshanov et al., 2021b,c). While no optimization-based fuel treatment models were proposed for northern boreal forests in Canada, optimization models have supported fuel treatment planning in the northern mountain conifer forests having an analogous forest structure and similar rates of occurrence of stand-replacing wildfires (Wei et al., 2008; Wei, 2012; Gannon et al., 2019; Yemshanov et al., 2021a).
Here, we present a linear programming model that allocates fuel treatments to minimize the wildfire exposure to the power line section intersecting the wildlife connectivity corridor (hereafter, the area of concern) while minimizing the negative effects to the wildlife corridor. Following Miller and Ager (2013), we considered wildfire exposure in the context of the spatial juxtaposition of values with fire behavior, such as likelihood and intensity, but without explicitly describing fire effects on those values. We combine a spatial fuel treatment placement problem that minimizes the likelihood of wildfire spread to the area of concern with the problem of maintaining a corridor between isolated refuges with minimum pass-through resistance for wildlife. Our methodology leverages recent advances in optimization to assist with wildfire prevention decisions (Martell et al., 1998; Wei et al., 2008; Rachmawati et al., 2015) and target the reduction in wildfire spread and intensity (Wei et al., 2008; Minas et al., 2014; Rachmawati et al., 2015, 2016; Minas and Hearne, 2016; Alcasena et al., 2018; Gannon et al., 2019). To find the optimal placement of fuel treatments, we incorporate the approach of Yemshanov et al. (2021a) where, for all possible pairs of locations in a forest landscape, we calculate the pairwise likelihoods of fire spread using a fire behavior simulation model. Our analyses aimed to support operational activities by finding the possible options to balance the power line exposure to wildfires against the wildlife habitat conservation objectives in current and future climates.
Our methodology builds upon our previous study (Yemshanov et al., 2021a) by adapting the critical node detection (CND) problem to degrade the connectivity of fuels in a landscape and minimize the risk of wildfire spread to the area of concern with human infrastructure. CND problem characterizes the vulnerability of networks after the removal of some nodes and has been applied in many disciplines (Houck et al., 2004; Matisziw and Murray, 2009; Walteros and Pardalos, 2012). In this study, we have modified the CND problem to incorporate probabilistic wildfire behavior information and linked this problem with the landscape-scale caribou habitat connectivity model.
2. Materials and methods
2.1. Case study
Our case study was located in the area surrounding Hydro-Québec's proposed connectivity corridor in northeastern Québec, Canada (Figure 1). The area included boreal forest (Saucier et al., 2009) with a disturbance regime dominated by periodic clear-cut harvesting (Fourrier et al., 2013) and high-intensity wildfires with return intervals averaging 400 years (Boulanger et al., 2014). This region includes a vast, poorly regenerated area that was burned by a wildfire in 1991 (Dawe et al., 2022). The wildfire's footprint intersects the caribou distribution range; this area did not experience fire or substantial human disturbances (such as road construction or logging) since the wildfire. Conservation officials in Québec anticipate that the use of this area by caribou will increase as coniferous trees mature. Therefore, Hydro-Québec is preserving a 10-km caribou connectivity corridor intersecting the soon-to-be-constructed power line (Figure 1).
2.2. Spatial placement of fuel treatments as a critical node detection problem
Consider a forest landscape as a network G of forest patches (nodes) I, where the connecting arcs (edges) E depict possible vectors of fire spread between adjacent patches (Figure 2A). Forest patches are covered by vegetation that could be ignited and support the spread of wildfires, called fuels in our context. Two nodes i and j with fuels, i, j ε I, are connected if the network G contains the path of connected nodes with fuels between them. The presence of a path between nodes i and j indicates a possible spread of a wildfire from i to j.
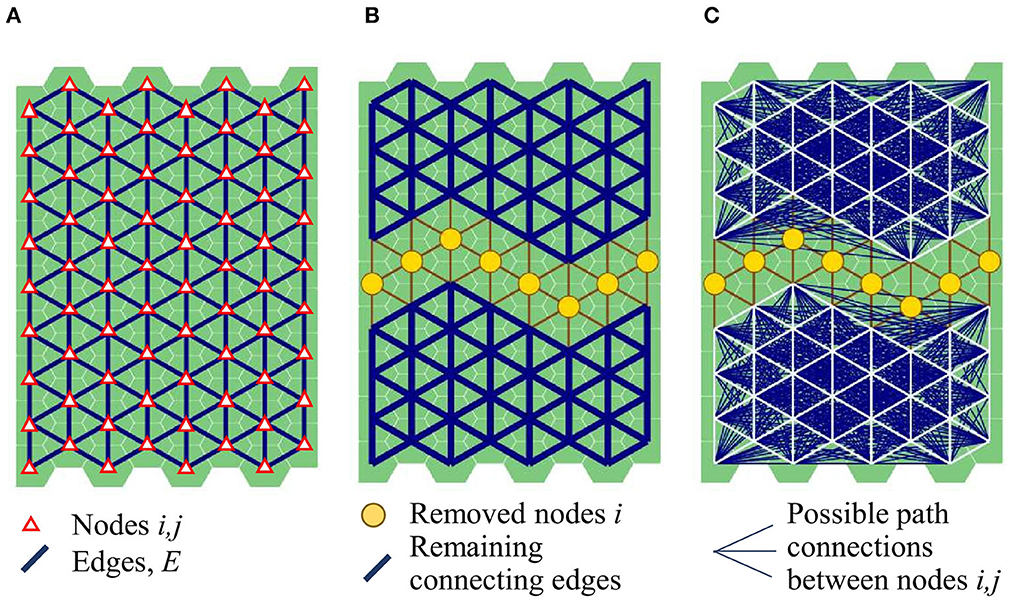
Figure 2. (A) A landscape network of connected patches (graph) G as a single connected component before interdiction. Network nodes i are shown as triangles and network edges E connecting nodes i are shown in dark blue; (B) an interdicted graph with two connected components. Large yellow dots denote the removed nodes; (C) pairwise connections uij between node pairs in the remaining connected components after interdiction (dark blue lines).
We conceptualize the fuel treatments in node i as an area where measures are implemented, aiming to fragment the fuels in the landscape and so reduce the likelihood of fire spread through i, so this impact is equivalent to a removal of that node and an interdiction of flow through i in network G (Figure 2B). To minimize the fuel connectivity in network G we solve a critical node detection (CND) problem that finds the key nodes whose removal (i.e., areas with fuel treatments) maximally degrades the connectivity of the fuel network according to a chosen criterion, such as the number of possible path connections between nodes (Arulselvan et al., 2009; Veremyev et al., 2014a,b, 2015).
A binary decision variable xi determines whether node i is not deleted from network G (xi=1 and xi=0 otherwise), and a binary variable uij, for every pair of nodes i,j, determines whether nodes i and j are not deleted from the network (uij=1 when xi = xj = 1) and there is a path connecting i and j (Figure 2C). The total number of nodes that can be removed from network G is limited by budget level B (see Table 1 for symbolic notations).
The CND problem evaluates the connections for every node pair i,j in the network (Figure 3A). While natural fire barriers, such as rock outcrops, can limit the spread of wildfires, fire behavior in forest landscapes can be limited by other factors, such as local fuel loads or weather (Miller et al., 2008; Parisien et al., 2011; Parks et al., 2011). In a spatial setting, the possible fire behavior can be defined as a set of plausible ways a fire may spread between all pairs of locations in network G. For each pair of nodes i and j, one needs to estimate the likelihood that a fire ignited in node i spreads to node j, pij. Incorporating this information directly into a contiguous fuel network is problematic, hence we adapted the approach from Yemshanov et al. (2021a), which does not alter the fuel network configuration. For each node i in network G, we defined an area (a subset Θi) of other nodes j that are potential spread destinations of fires ignited in i (Figures 3B, C). For each node i, we restricted the evaluation of path connections ij to nodes j—members of subset Θi. The configuration of subsets Θi defines the potential extent of fires that could spread from i and so the locations of critical nodes impacting that spread.
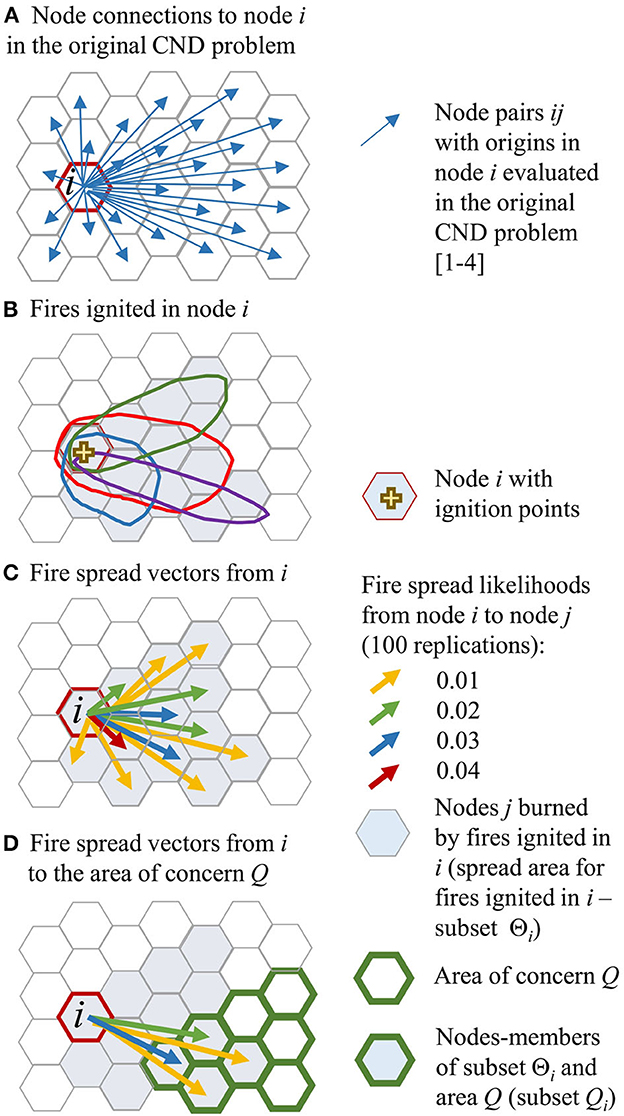
Figure 3. (A) Node pairs ij with the origin in node i that are evaluated in the basic CND problem; (B) potential spread area of fires ignited in location i. Nodes with the positive likelihoods of a fire spread from the ignition node i are shaded; (C) likelihoods of a fire spread from node i with the ignition points to nodes j, pij. Fire spread examples are based on 100 iterations; (D) likelihoods of a fire spread, pij from node i with the ignition points to node j in the area of concern Q.
We delineated the subsets Θi around each node i using a fire behavior simulation model (see Supplement S1). We used the subsets Θi to calculate the likelihoods of fires ignited in node i to spread to node j, pij (Figure 3C, see Supplement S2). For node i, subset Θi included the destination node j with the likelihoods of a fire spread from i, pij > 0. A removal of node with a larger subset Θi around i leads to a greater reduction of wildfire exposure to other nodes. Also, a node that belongs to a larger number of distinct subsets Θi is more likely to include a fire spread corridor and so its removal is beneficial for wildfire exposure reduction. In our infrastructure protection context, we only needed to consider the possible fire spread to nodes j located in the area of concern Q (Figure 3D). Hence, for each node i, we only needed to check for path connections to nodes that are members of both subsets Θi and Q (subset Qi hereafter) (Figure 3D).
To account for the uncertain fire behavior in the area, we weighted the binary decision variable uij that checks for the presence of a path between nodes i and j, by the estimated likelihood of fire spread between i and j, pij. Depending on how decision-maker may interpret the pij values, we formulated two management problems minimizing the spread of the most frequent (small) fires or the largest (rare) fires. The objective function [1] in our CND problem 1 minimizes the expected number of node pairs characterizing the possible spread of fires to the area of concern Q after the removal of B critical nodes in landscape network G, that is, as follows:
Problem 1:
The CND problem 1 is based on the formulation in Veremyev et al. (2014a). Constraint [2] limits the total treatment area; term ci denotes the node area; constraint [3] ensures, for adjacent nodes i and j, that nodes can be connected (i.e., uij = 1) if neither node is deleted, and constraint [4] ensures that nodes i and j are connected if there is a non-deleted node k, k ≠ j, in the neighborhood of i, ℵG(i), as well as nodes with valid path connections to i, such that k and j are connected. A large positive value M is needed to keep the right side of Equation [4] ≤ 1. Note that CND problem does not require defining the possible flow path(s) between node pairs i,j. Instead, constraints [3,4] check that i and j remain in the same connected portion of the network, which implies the presence of a flow path between i and j.
Weighting the decision variable uij in objective [1] by the fire spread likelihood pij prioritizes the interdiction of most frequent fire spread paths to nodes located in area Q. Wildfire spread depends on the distance between ignition and destination nodes i and j, which makes it dependent on the linear fire size. In forest landscapes, the fire-size distribution follows a power law (Cumming, 2001; Malamud et al., 2005); therefore, CND problem 1 prioritizes the interdiction of the most frequent but small fires.
In the alternative formulation (CND problem 2 hereafter), all positive pij values are replaced by unary fire spread indicators pijbin= 1, that is, as follows:
Problem 2:
subject to: constraints [2–4].
CND problem 2 considers the possible fires spreading to the area of concern Q within the subsets Θi (i.e., with pij > 0, j ∈ Q) regardless of their spread likelihood values pij. In gridded networks like forest landscapes, the number of connections between node pairs increases quadratically with the linear size of a connected component. Therefore, for each node, the number of possible long-distance fire spread paths exceeds the number of possible short-distance paths, and so the CND problem 2 minimizes the number of long-distance fire spread paths, which characterize large fires.
2.3. A wildlife corridor placement problem
In the fuel connectivity network, the node size is set with respect to the coarsest scale of fuel treatment decisions and the need to account for fine-scale fuel composition and natural fire barriers. In contrast, the caribou habitat network must consider larger patches (nodes) with a size sufficient to separate caribou from predators (Lesmerises et al., 2013a,b; Rempel and Hornseth, 2018). We defined the habitat network using 7-km2 nodes (Figure 4), assuming that the caribou corridor may begin in the area of the proposed Frégate Lake biodiversity reserve to the southeast of the power line and end in the region with abundant refuge habitat northwest of the power line (Figure 4B).
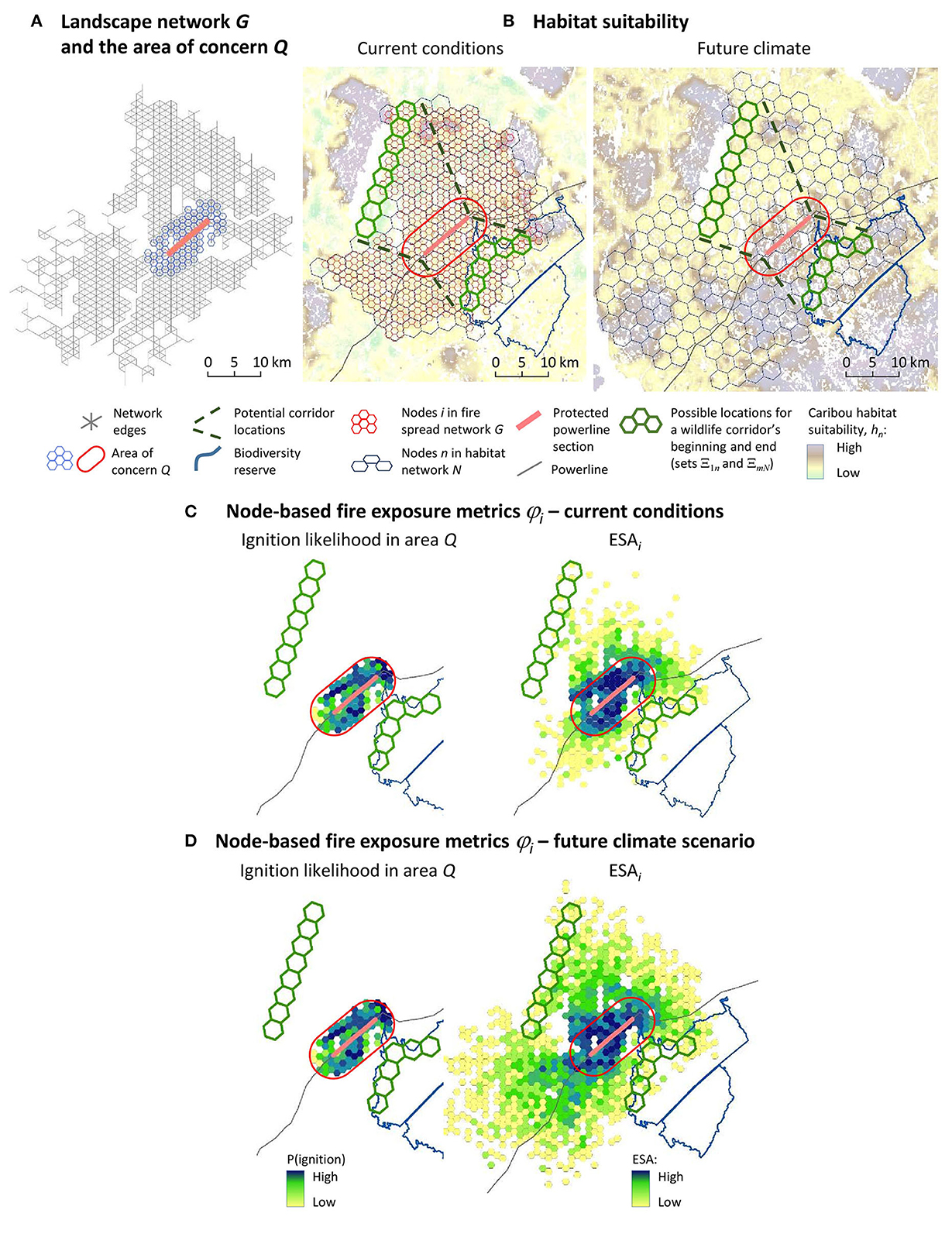
Figure 4. (A) Landscape network G and the area of concern Q; (B) caribou habitat suitability values in the current and future climate conditions. To improve the visibility of habitat suitability patterns, the fuel treatment network G in the future climate scenario is not shown. Node-based wildfire exposure metrics ϕi (i.e., the ESA and the ignition likelihood): (C) current conditions scenario; (D) future climate scenario.
We allocated a wildlife corridor using a landscape resistance concept, which characterizes the relative resistance of the environment to animal movement in a particular location (Zeller et al., 2012; Koen et al., 2014; Wade et al., 2015). Resistance can be depicted as a function of environmental or spatial attributes, such as the availability of habitat, or geographical distance to the closest population (Singleton et al., 2002; Royle et al., 2013). Previously, the resistance concept has been applied to the selection of wildlife corridors and protected areas in complex landscapes (Dilkina and Gomes, 2010; Conrad et al., 2012; Dilkina et al., 2016; Yemshanov et al., 2022).
We formulate a corridor placement problem as a network flow problem that minimizes total resistance for animals passing through the corridor. As we set the habitat patch size larger than the node size in the fuel network G and use different symbolic notations in our corridor placement problem, we depict a network N of connected patches (nodes) n with suitable wildlife habitat (Figure 4). Caribou habitat patches n, n ∈ N need to be large enough to ensure a separation of animals from predators (Vors and Boyce, 2009; Mumma et al., 2017).
Animals can move between neighboring nodes m and n in landscape N via the nodes' common boundaries. We depict the connectivity between nodes m and n as bidirectional pair of arcs nm, mn and conceptualize the movement of animals as a flow between adjacent connected nodes. The corridor between two refuge areas could be of any configuration but must guarantee that its endpoints are connected to the refuge areas. This was achieved by finding a connected path in the middle of the corridor that connects the two locations. We define three types of nodes in habitat network N: possible locations of the (i) beginning and (ii) end of the path adjacent to the two refuge areas and (iii) the nodes where the path connecting isolated refuge areas could be located (Figure 5A). We introduce an auxiliary start node 1 as a source of the flow through the connected path. Node 1 is connected to all landscape nodes n—possible locations of the path's beginning via arcs 1n. Set Ξ1n, n ∈ N denotes the arcs 1n connecting node 1 with nodes n—possible locations for the path's beginning (Figure 5A). An auxiliary end node, N', is a recipient of the flow from node 1 through the connected path and can receive the flow from landscape nodes m, where the connected path could end, via arcs mN'. Set , m ∈ N denotes the arcs mN' connecting the plausible path's end nodes m to recipient node N' (Figure 5A).
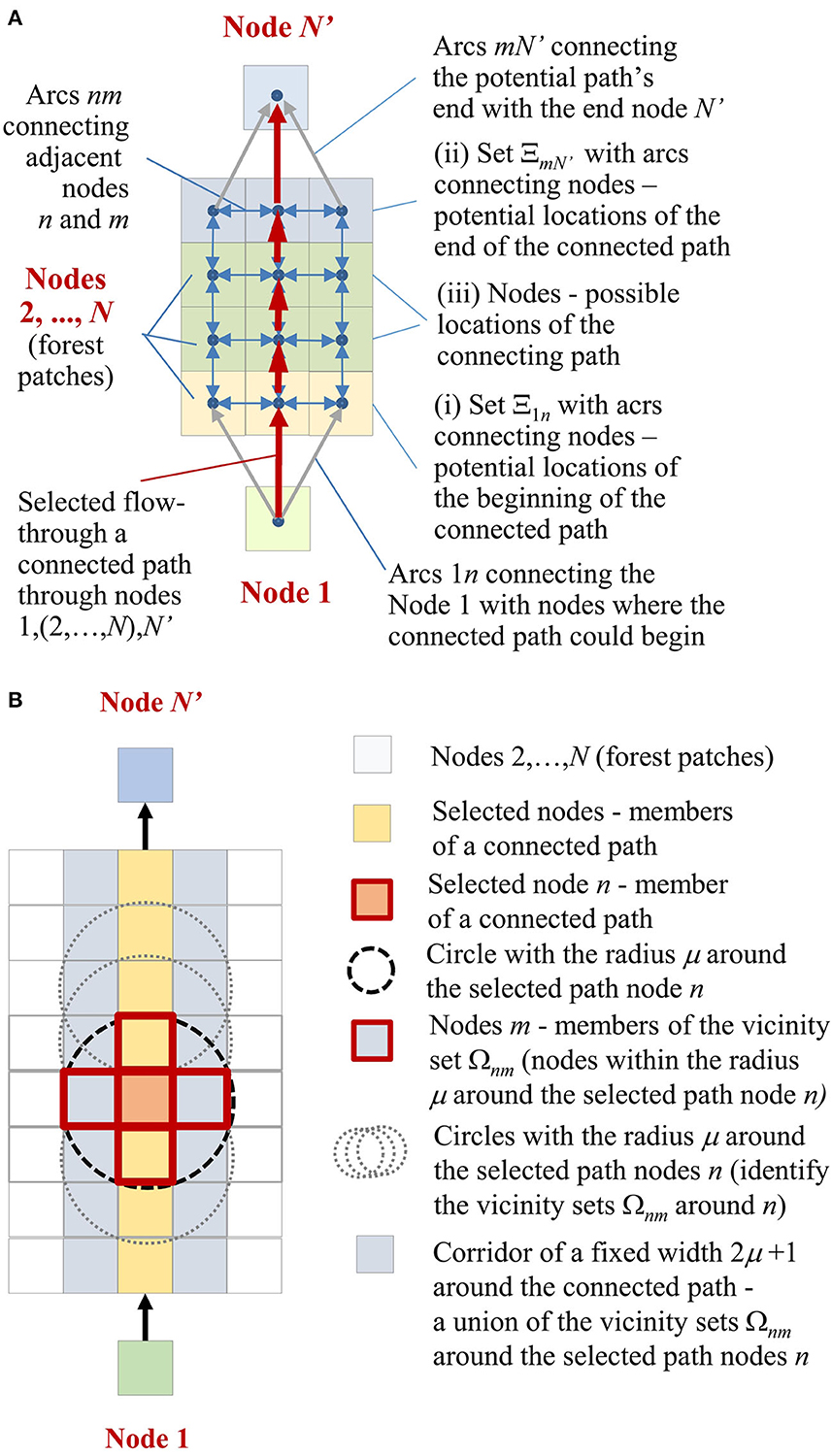
Figure 5. (A) Corridor connectivity sub-network N; (B) the connected path concept: a vicinity set, Ωnm, of nodes m around the selected path node n and a fixed-width corridor connecting two isolated areas (indicated by auxiliary nodes 1 and N').
After selecting a path, we needed to select a corridor space of fixed-width μ nodes from both sides of the connected path. For each node n—a potential location in the connected path, we define a subset Ωnm of surrounding nodes m comprising a circle with radius μ nodes around node n (Figure 5B). For the complete path of connected nodes n, the union of the corresponding subsets Ωnm around each selected path node defines a corridor space of a width 2μ +1.
We define a binary variable, qn, to select nodes n in a path connecting start node 1 and end node N' through nodes 2,…, N (qn= 1 and qn = 0 otherwise). The selection of the connecting path is only allowed within a designated area which ensures the placement of the whole corridor width in area N. A binary parameter Δn, Δn ∈ N, defines the locations of possible path placement in area N (Δn = 1 and Δn = 0 otherwise). To track the path connectivity, we define a binary variable, znm that specifies flow through arc nm between adjacent nodes n and m along the path (znm = 1 and znm = 0 otherwise). A binary variable, wm, selects the nodes m that comprise vicinity subsets Ωnm surrounding each selected path node n (wm = 1 and wm = 0 otherwise). Together, the selected nodes with wm = 1 define the corridor space. In order to maintain the corridor width within the full length of the proposed power line section (9–10 km), we set the corridor cross-section to three nodes, which defines a one-node radius for the subsets, Ωnm around the connecting path nodes n (Figure 5B).
For each node n in a landscape N, we calculate a landscape resistance parameter, bn, that describes how likely animals are to move through that node, as bn = hmax–hn, where symbol hn defines the quality of caribou habitat in site n and hmax sets the maximum possible quality of habitat in a site in landscape N. We assumed that animals preferred to move through sites with comparatively high habitat quality (Cushman et al., 2009, 2013; Rayfield et al., 2010; Parks et al., 2013).
The corridor placement problem [6] minimizes the total resistance of the wildlife corridor connected to isolated refuge areas, i.e.:
subject to:
Constraints [7–11] define the path connecting the two refuge areas and are described in the following lines. Constraint [7] enforces the connectivity of the selected path (i.e., nodes with qn = 1) and implies that the amount of flow coming to node k is equal to the amount of outgoing flow from k. Constraint [8] stipulates that the path can be allocated through a node n only if n belongs to a designated area (defined by the binary parameter value Δn = 1), while constraint [9] enforces agreement between the selection of node m in the connected path and the selection of flow to m along the connected path. Constraints [10] and [11] specify the designated locations for a path's possible beginning and end. Constraint [10] ensures that the flow from auxiliary node 1 can only go to nodes—possible candidates for the beginning of the path—members of set Ξ1n and ensures that the flow only goes through one arc (which guarantees a single connected path). Constraint [11] ensures that the flow from a node m—a plausible candidate for the path's end—member of set comes to node N' (the recipient of the flow) through a single arc.
Constraints [12] and [13] select the corridor space around nodes n in the connected path. Constraint [12] stipulates that all nodes m—members of the subset Ωnm around node n—must be included in the corridor space if n is located in the connected path. Constraint [13] specifies that a node m will not be selected for the corridor if no potential path nodes n that have m in their vicinity subsets Ωnm are included in the connected path.
2.4. Combining the CND and corridor connectivity problems
The habitat network N and fuel connectivity networks G have different spatial resolutions; therefore, we needed to align the nodes in networks G and N in a hierarchical fashion (Figures 4A, B). We introduced a binary parameter γin to denote the spatial overlap between node i in fuel network G and patches n in habitat network N (γin =1 if node i is located in patch n and γin = 0 otherwise). The joint problem [14] minimizes the weighted sum of the wildfire risk reduction and wildlife corridor protection goals, that is, as follows:
subject to: constraints [2–4,7–13] and
The first term of Eq. [14] denotes the wildfire risk reduction objective and the second term denotes the corridor protection objective. Scaling factor ψ defines the relative importance of each objective, while constraint [15] ensures no fuel treatments (i.e., node removal) are placed in the selected corridor space.
2.5. Estimating fire spread likelihoods pij and caribou habitat suitability
We simulated the behavior of forest fires in a 150-km radius around the proposed power line section of the connectivity corridor (Figure 1) and estimated the likelihood of fires spreading from every patch i to every patch j in the area of concern Q, pij. Specifically, the area of concern Q included the power line section in which forest cover was retained to preserve caribou habitat connectivity, along with a 3-km buffer zone around the power line (Figures 5A, B). This buffer size was based on the assessment of likely rates of spread under 95% of plausible fire weather conditions, assuming a fire ignition escapes fire management action (i.e., initial attack) (Dawe et al., 2022).
We used the Burn-P3 model (Parisien et al., 2005) to estimate the fire spread likelihoods pij between node pairs ij in the study area. The model simulates wildfire events on an annual scale. For each fire year, the model generates the stochastic ignition locations and the perimeters of spreading fires (Parisien et al., 2011, 2013) and have been widely used to evaluate fire prevention strategies (Riley et al., 2018; Reimer et al., 2019; Yemshanov et al., 2021a). Burn-P3 implements the fire behavior equations of the Canadian Fire Behavior Prediction (FBP) System (Stocks et al., 1989; Forestry Canada Fire Danger Group, 1992) and applies the Prometheus spatial fire growth model to predict fire spread (Tymstra et al., 2010) (see Supplement S1).
The fire regime in the study area is characterized by high-intensity wildfires, which primarily occur in the summer. Lightning-caused fires account for 87% of the total area burned (Dawe et al., 2022), whereas spring wildfires tend to be human-caused and contribute to a much smaller proportion of wildfire activity (Boulanger et al., 2013). We used Burn-P3 to explore two distinct fire-regime scenarios depicting the current climate conditions and the future climate for 2070 assuming Representative Concentration Pathway (RCP) 8.5 climate forcing projection (van Vuuren et al., 2011) from the global climate model CanESM2, which is characterized by significant increases in both fire spread and fire intensity (Dawe et al., 2022). The CanESM2 product is well adapted to wildfire modeling in Canada and its projections appear plausible compared with those of other general circulation models (Boulanger et al., 2018).
We populated Burn-P3 with the fire-regime assumptions from Dawe et al. (2022) (see Supplement S1). Estimations of future fire regimes, such as the future number of annual ignitions and the duration of fire spread, were based on daily fire weather projections following the methodology of Stralberg et al. (2018) and Wang et al. (2016). Model calibration was performed heuristically by adjusting the model inputs to approximate the output distribution of simulated wildfire sizes to those of the observed historic data. We used the ignition locations and perimeters of simulated fires at a 100-meter resolution on a regular grid to calculate the likelihoods of fire spread, pij, from all locations in our landscape network G to the area of concern Q (see Supplement S2) (Figures 5A, B). The pij values did not incorporate the occurrence of short-range spotting of fires in site j. Although very short-range spotting is incorporated in the spread equations of the FBP System, long range (>300 ha) is not considered in this system nor in the Burn-P3 simulation model. The potential effects of this limitation on the results were muted by the relatively coarse size of spatial units, which were too wide to allow widespread spotting.
The vegetation composition for the future climate scenario was projected using the forest landscape model LANDIS-II (Scheller et al., 2007) following the methodology of Boulanger and Puigdevall (2021) (see Supplement S3). In this model, individual tree age cohorts, which are represented by the intersection of species and age class, are simulated to grow and die according to specific ecology profiles. We have composed the initial tree species composition at a 100-m resolution from provincial forest inventory maps (see Boulanger and Puigdevall, 2021) and projected forest succession using the Biomass Succession extension in LANDIS-II (Scheller, 2013, see Supplement S3). This extension accounts for tree species' cohort age, life-history traits, and species-specific land type responses and simulates changes in cohort aboveground biomass over time. Predictions of vegetation composition changes were run from 2020 to 2070 under the RCP 8.5 climate scenario. We then translated the vegetation composition outputs to fuel types using the FBP System fuel classification rules (Forestry Canada Fire Danger Group, 1992).
For each node n, we estimated boreal caribou habitat suitability, hn using a model developed in Québec by Leblond et al. (2014) and implemented in LANDIS-II by Leblond et al. (2022), (see Supplement S3). This model considers forest composition, age, and human disturbances and accounts for the distance to mines and roads to estimate a habitat suitability index (Leblond et al., 2014) (Figure 5B). Figure 6 depicts the analysis flow: We used individual fire perimeters and ignition points generated with Burn-P3 to calculate the fire spread likelihoods pij to area Q between all pairs of locations. Then, the pij values, habitat suitability hn, and subsets Ξ1n and ΞmN were used as inputs in the CND model to find the optimal corridor position and fuel treatment placement.
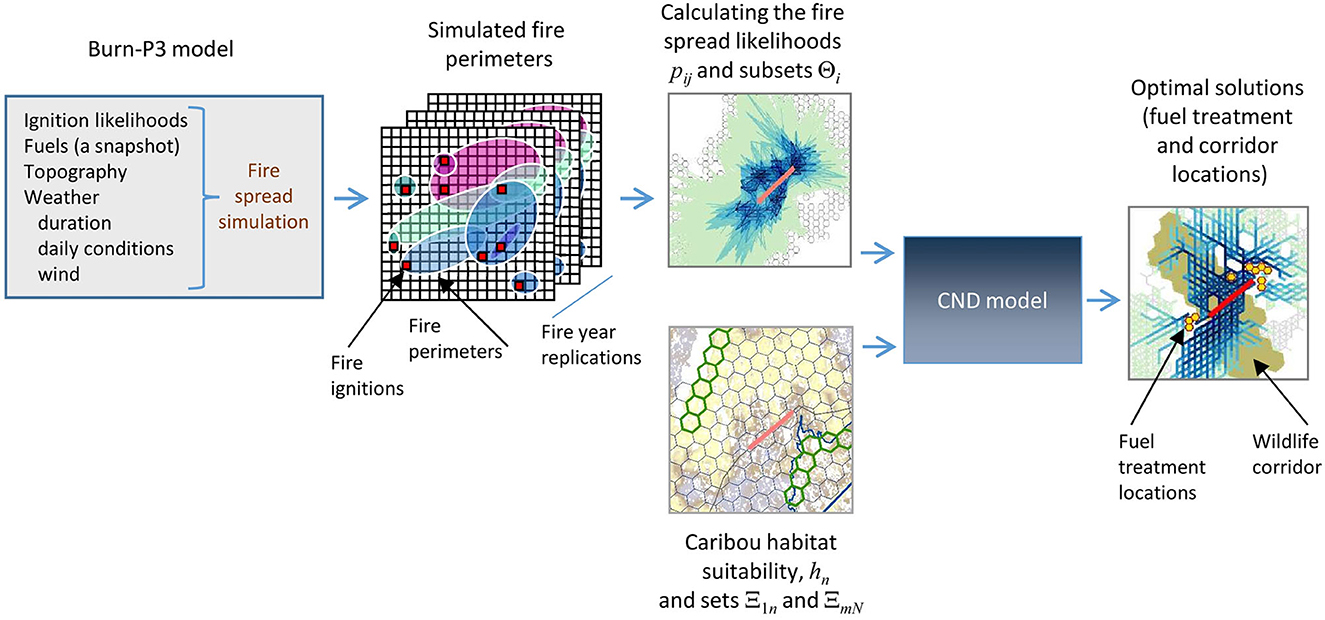
Figure 6. Analysis workflow. The outputs of Burn-P3 model simulations (the ignition locations and the spatial footprints of simulated fires) were used to generate a set of fire spread likelihoods pij between all node pairs i,j in a forest landscape. This set, along with the data depicting the habitat network and the spatial constraints for a caribou corridor placement were used in the CND model to find the fuel treatment placements minimizing the wildfire exposure to the area of concern.
2.6. Model scenarios
Our baseline scenarios intended to explore the fuel treatment placement solutions (i.e., the treatments of forest sites equivalent to fuel node removal) in the study area in the current and future climate conditions and included the sets of CND problem 1 and 2 solutions with and without the placement of the wildlife corridor (Table 2). In the corridor scenarios, no fuel treatments were allowed inside the corridor area. In the “no-corridor” scenarios, the caribou habitat connectivity objective was omitted and fuel treatments could be placed anywhere in the area. For a given treatment budget B, this theoretical scenario indicates the best reduction of wildfire risk to the area of concern Q.
In our model, the wildlife corridor has a fixed width, is relatively short, and always linked to two compact refuge locations. This restricts the possible range of optimal corridor positions, so the corridor placement between the two refuge areas essentially acts as a hard spatial constraint on fuel treatment positioning that reduces the realm of possibilities for fuel treatment decisions. This is why the emphasis in our study is on node removal in network G rather than optimizing the habitat corridor connectivity in network N. We solved the fuel treatment placement problem with the wildlife corridor placement by prioritizing the reduction of wildfire exposure to the area of concern Q and setting the scaling factor value ψ = 0.999 in Eq. [14].
We evaluated a range of fuel treatment budgets, B between 5 and 30 nodes, and compared the treatments' ability to reduce the spread likelihood of the largest fires (CND problem 2 objective [5]) vs. the most frequent fires (CND problem 1 objective [1]) in the area of concern Q. We have also evaluated an alternative problem formulation which selected the site treatment placements according to a node-based wildfire exposure metric ϕi (Figures 4C, D). The alternative problem minimized the sum of node-based exposure metric ϕi across the area, i.e.:
subject to: constraints [2,7–13,15].
We have tested two alternative metrics. The first metric is the likelihood of ignitions in area Q. This metric indicates the occurrence of fire ignitions in patch i, as predicted by the fire simulation model, regardless of the fire size associated with those ignitions. The second metric minimized the expected spread area (ESA) to the area of concern Q. For each node i, we estimated the ESA as a sum of node areas cj in subset Qi, where fires ignited in i were able to spread, times the likelihoods of spread from i to j, pij, i.e.:
We used the ignition locations and fire perimeters simulated in Burn-P3 to identify the subsets of node j in area Q where fires ignited in each node i were able to spread (i.e., subsets Qi in CND problem formulations. The ESA is analogous to the downstream protection value in Pais et al. (2021), which estimates the likely propagation area for fires ignited in node i. Comparatively, the ESA estimated the expected fire propagation area from i from Burn-P3 fire behavior model outputs.
We composed the model in the General Algebraic Modeling System [GAMS (GAMS Development Corporation), 2021] and solved it with the GUROBI linear programming solver [GUROBI (Gurobi Optimization Inc), 2021]. The model was run for 18 h or until reaching an optimality gap of 0.05%, whichever came first. Most solutions reached the optimality gap within 8 h.
3. Results
The patterns of possible fire spread vectors between all node pairs ij, along with the fire spread likelihoods pij to the area of concern Q appeared too dense to interpret (Figures 7A, C); therefore, we traced the spread vectors ij and their corresponding spread likelihoods pij to nearest network edges via a shortest path algorithm and used these maps to display the treatment solutions. We first show the fuel treatment solutions without protecting a caribou corridor (Figures 7B, D; Figure 1 Supplement S4). The treatments in CND problems 1 and 2 aimed to block fire spread paths to the area of concern (Figure 7D callout I). In the solutions with the caribou corridor, the corridor occupied the central portion of the area of concern and fuel removal could only be done in the periphery of the area Q or outside (Figure 8; Figures 2, 3 Supplement S4). For current conditions, the treatments tended to block the arrival of fires from the northeastern part of the area, which contains a large accumulation of fuel (Figures 8A, B).
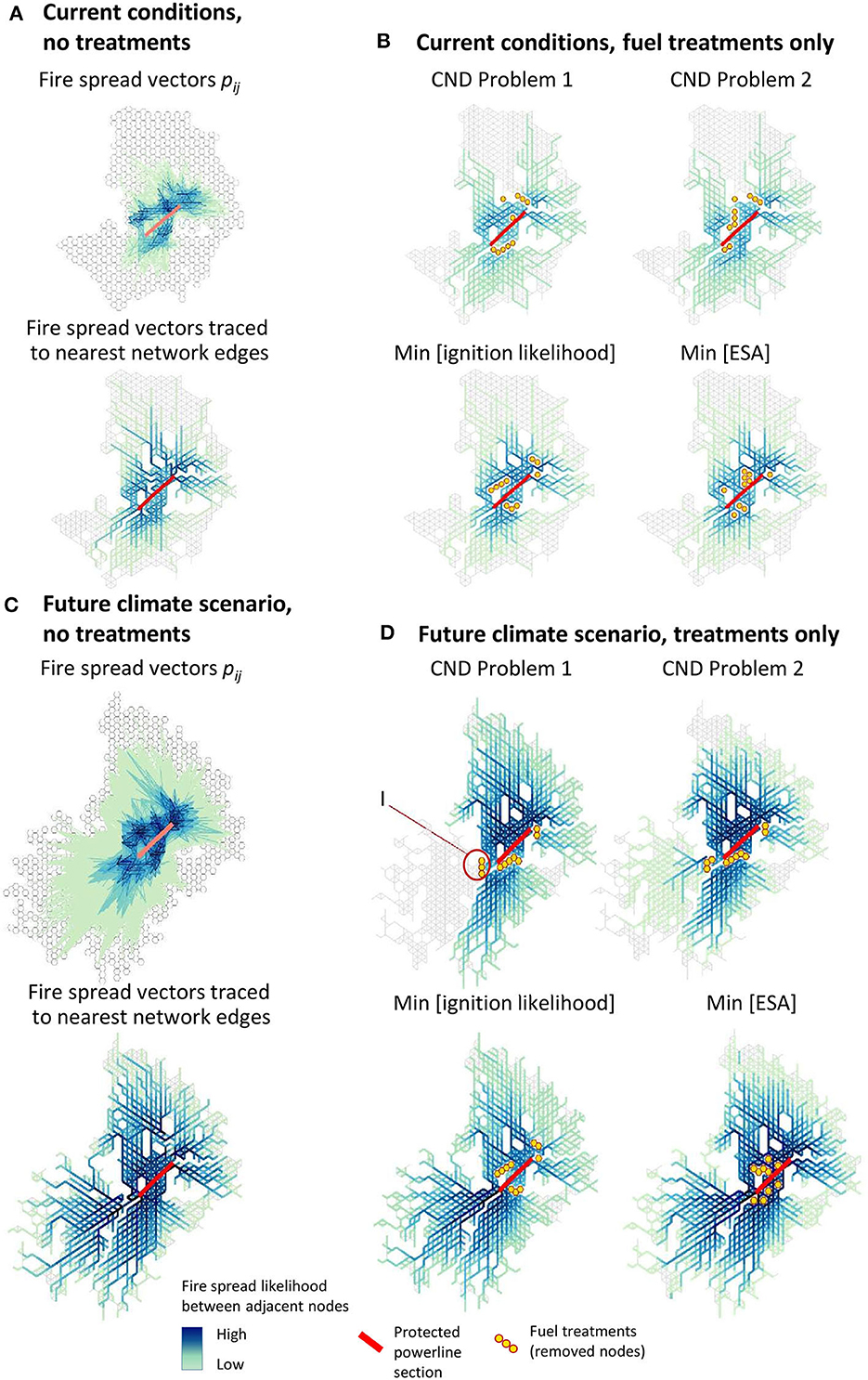
Figure 7. Optimal treatment solutions in no-corridor scenarios: (A) maps of fire spread likelihoods in current climatic conditions without treatments; (B) optimal treatments solutions in current conditions; (C) maps of fire spread likelihoods in future climate scenarios; (D) optimal treatments solutions in future climate scenarios. The optimal solutions are shown for the treatment budget B = 10 nodes. Callout I shows the treatments blocking the potential fire spread paths to the area of concern.
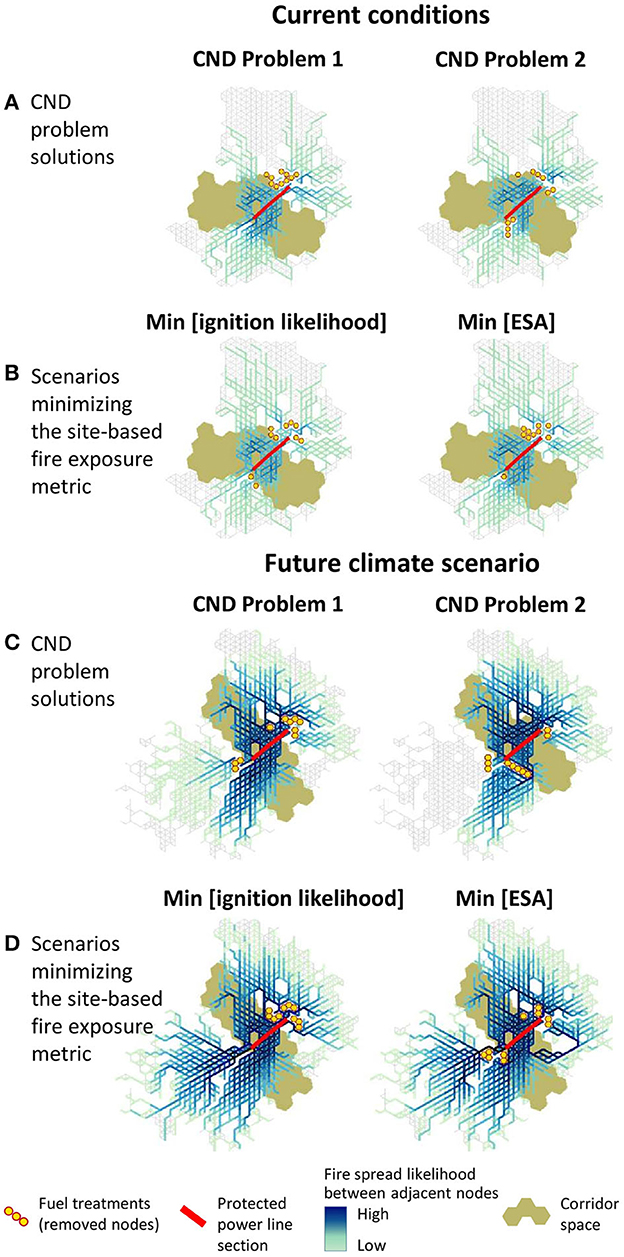
Figure 8. Optimal treatment solutions in wildlife corridor scenarios. Current climate conditions solutions: (A) CND problem 1 and 2 solutions; (B) scenarios minimizing the site-based wildfire exposure metric ϕi. Future climate conditions solutions: (C) CND problem 1 and 2 solutions; (D) scenarios minimizing the site-based wildfire exposure metric ϕi (the ESA and the ignition likelihood). The maps show the solutions for the treatment budget B = 10 nodes.
The climate change scenarios showed more distinctions between the solutions to problems 1 and 2 than the current conditions scenarios (Figures 8C, D). In problem 2, which minimized the spread of the largest fires, fuel treatments tended to block the potential spread of fires from the southwestern part of the area. The landscape in this area is expected to develop from its current early regeneration state into more fire-prone vegetation in the next 50 years. In the problem 1 solution, which minimized the spread of most frequent fires, the treatments were allocated near the power line section on both sides of the corridor.
In small-budget solutions, the treatments yielded a potential reduction of the power line exposure to wildfires of 36–39% in current conditions and of 20–31% in future climate compared with the no-treatment scenarios (Table 3). Comparatively, fuel treatments guided by the site-based fire exposure metric ϕi were less contiguous than the treatments in CND solutions. In the solutions based on the site-based wildfire exposure metric, treatments targeted the nodes with the highest ϕi values without blocking the most threatening fire spread corridors to the area of concern and yielded a lower reduction of wildfire exposure:18–30% in current conditions and 9–15% in future climate vs. the no-treatment scenarios. The CND solutions allocated a sizeable proportion of treatments in clusters aiming to block the most threatening fire spread paths to the area of concern Q from the southwestern and northeastern directions (Figures 7, 8). In some locations, such as the southwestern corner of the area of concern, the fuel treatments acted as firebreaks blocking a narrow segment of forest vegetation that may help the propagation of wildfires arriving from a large region southwest of the power line (Figures 7D, 8C).
We compared the treatment scenarios based on the metric of the potential spread of the largest fires (i.e., objective [5]) and most frequent fires to the area of concern Q (objective [1]) as a function of the treatment budget (Figure 9). The solutions to CND problems 1 and 2 showed the best capacity to reduce the risk of fires spreading to the area of concern in both current and future climate conditions (Table 3). Without the protection of a corridor, the solutions minimizing the likelihood of ignitions showed the worst performance (Figure 9A). This illustrates that spatial information related to fire ignitions is not sufficient to effectively plan preventive measures for wildfire protection because the ignition likelihood is not always a good predictor of patterns of wildfire perimeters. The solutions minimizing the ESA showed better performance in the current conditions, no-corridor scenarios (Figure 9A) compared with solutions minimizing the likelihood of ignitions. In the corridor solutions, increasing the budget above 10 nodes in current condition scenarios and above five nodes in future climate scenarios did not significantly improve the efficacy of treatments (Figure 9B; Table 3). More site treatments are thus likely to be allocated at farther distances from the power line, which reduces their effectiveness (Figure 3 in Supplement S4).
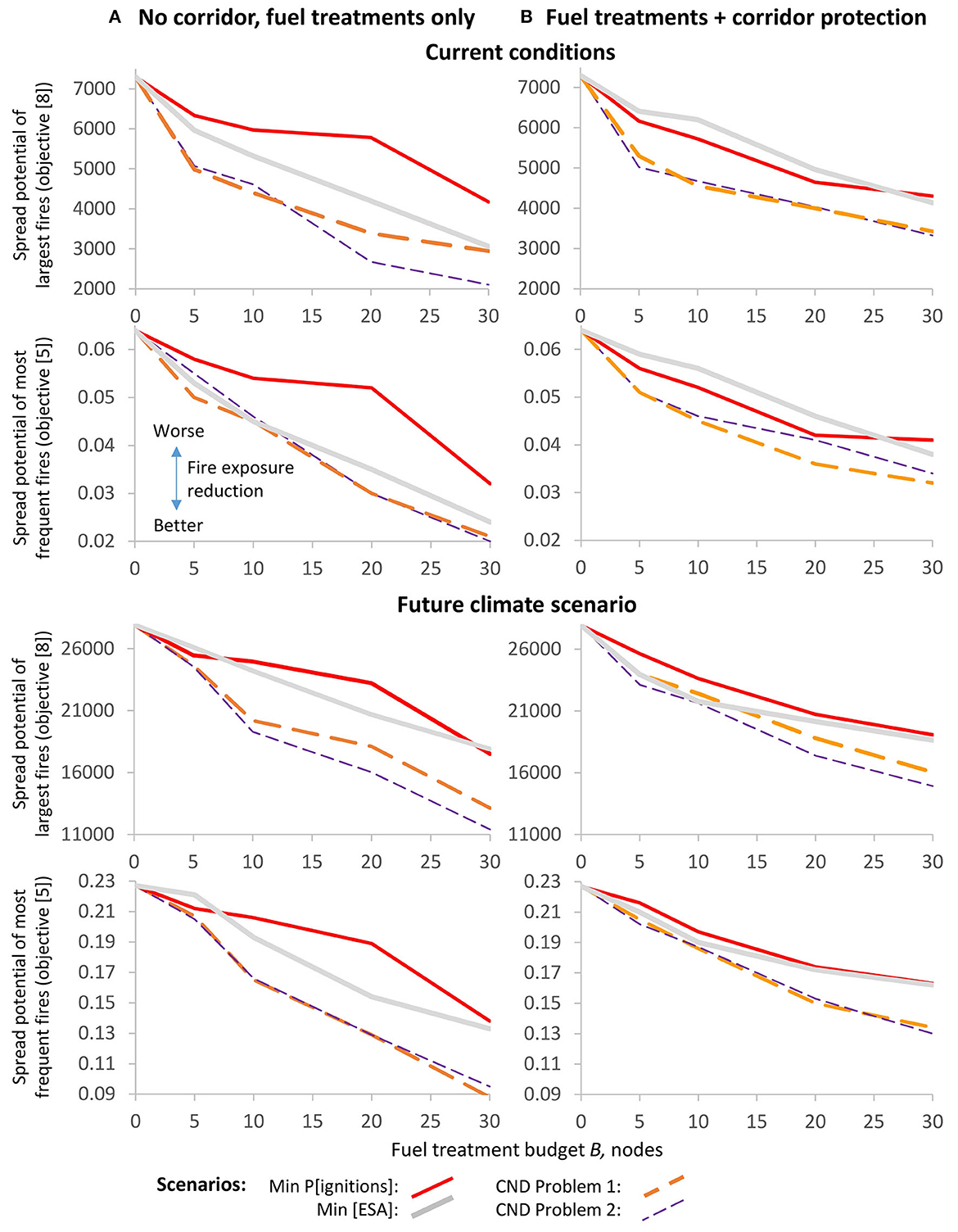
Figure 9. Objective value vs. treatment budget (nodes) in present-day and future climate conditions: (A) no-corridor scenarios; (B) corridor placement + fuel treatment scenarios. X-axis denotes the treatment budget (nodes) and y-axis denotes the fire spread potential (objective functions [5] and [8]). Lower objective values on y-axis show better outcomes.
We also explored the performance of treatments in terms of reducing the spread of the largest fires, as well as reducing the spread of the most frequent fires (Figure 10). The solutions minimizing ESA performed better than the solutions minimizing the likelihood of ignitions in reducing the spread of the most frequent fires, but not as well as CND problem solutions. Adding a wildlife corridor reduced the performance of CND problem solutions except under current conditions, where it had almost no impact on the capacity to reduce the spread of the largest fires (Figure 10A, callout I).
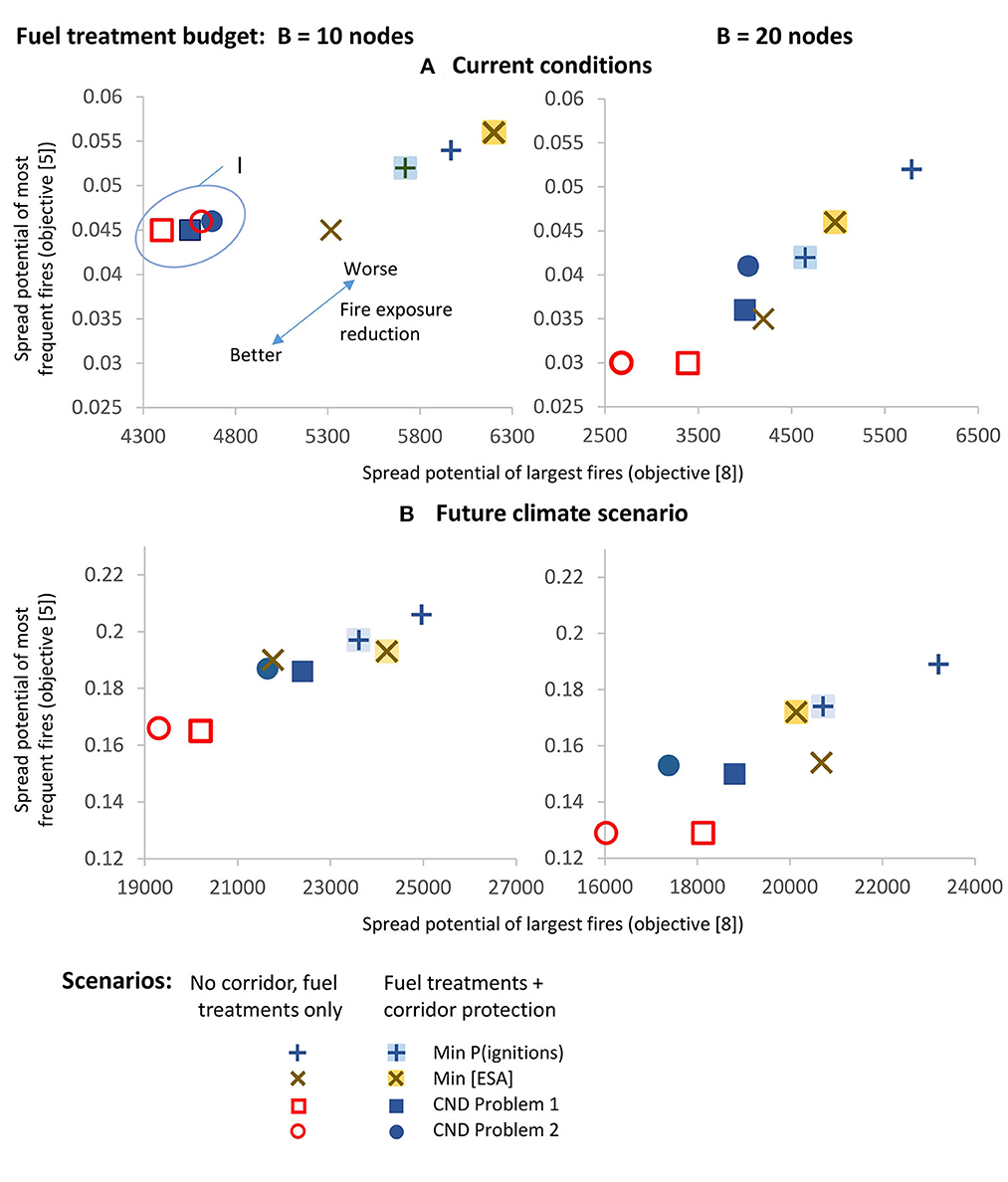
Figure 10. Performance of optimal fuel treatment solutions in dimensions of the risk reduction of the largest fires (X-axis) and the most frequent (small) fires (Y-axis): (A) current conditions scenario; (B) future climate scenario. Callout I shows problem 1 and 2 solutions in no-corridor and corridor scenarios (appear close). X-axis denotes the spread potential of the largest fires (objective function [5]) and y-axis denotes the spread potential of the most frequent fires (objective function [1]).
Fuel treatment placements were less effective in reducing the exposure to wildfires in future climate conditions (Table 3). Climate change assumptions also impacted the placement of the wildlife corridor (Figure 8). In current conditions, the northeastern part of the area had the highest burn probability, which caused the placement of treatments in this area (Figures 8A, B). In the future climate scenario, the forest stands in proximity to the entire corridor were predicted to mature to an older age and become a higher quality habitat for caribou but also more susceptible to fire; hence, the treatment placements in future scenarios aimed to protect both sides of the corridor.
The fuel treatment placements in the solutions minimizing the ESA resembled problem 1 solutions (Figure 8). This is because both models used the fire spread likelihoods between node pairs, pij when calculating the subsets Qi. We also evaluated a scenario that minimized the likelihood of fire ignitions in the area of concern (Figures 8B, D). This strategy consistently removed nodes with the highest likelihoods of ignition; however, as this scenario did not track the size of the ignited fires, the treatment solutions did not reduce the likelihood of fire spread to the area of concern as effectively as in solutions to CND problems (Table 3). Comparatively, the CND models 1–2 checked for the presence of a fire spread path between the pairs of locations and so the treatments tended to block the most threatening fire spread directions.
4. Discussion
Our scenarios investigated the interplay between protecting critical infrastructure from fire damage and maintaining a wildlife connectivity corridor between two isolated refuge areas, for both contemporary and future (2070s) fire environments. The constructed mitigation measure consisting of raising the power line above the forest canopy in order to retain the tree cover should be beneficial to caribou, but it comes at the expense of increased fire risk to the power line. The proposed approach to identify optimal locations of fuel treatments aims to provide managers a tool to inform the mitigation of—but not eliminate—such a risk.
A related study examining this management problem (Dawe et al., 2022) has shown that the current wildfire potential around the power line section of interest is relatively moderate due to a reduced fuel load and flammability in the poorly regenerated 1991 burn. However, Dawe et al. (2022) also showed that wildfire exposure to the corridor section of the power line was likely to increase in the upcoming decades due to vegetation regrowth and more frequent fire-conducive weather (Boulanger et al., 2013). In this context, our results showed that optimal fragmentation of the network of flammable fuels near the area of concern using the CND model was more effective than trying to limit the predicted fire ignitions or mitigate the potential for large, high-intensity wildfires.
Our results indicate that projected future wildfire regimes will not only amplify the vulnerability of caribou habitat and industrial assets to wildfire, but also underscore the need for an adaptive strategy that considers future changes in the wildfire risk. This would render fuel treatments less effective because the fire frequency in future climate conditions is expected to significantly increase (Flannigan et al., 2009; Boulanger et al., 2014; Wang et al., 2020). A future reduction in vegetation flammability associated with warmer and drier climates, namely, through a shift in dominance from conifers to broadleaf, may partially offset more severe fire weather (Terrier et al., 2013). However, projected large-scale vegetation changes are highly uncertain and the treatments may be less effective at limiting wildfire ignitions and spread under more severe fire weather conditions of the study area (Schoennagel et al., 2017; Seidl et al., 2017). Continued—and possibly increasing—investments in wildfire risk reduction measures will likely be required to protect both the caribou habitat and the industrial assets of the study area.
Given that no fuel treatment was allowed in the wildlife corridor area and that the corridor had to be sufficiently wide, attaining both conservation management and reducing wildfire exposure represented a complex tradeoff. This tradeoff is driven by the need to strategically place firebreaks across a landscape to prevent future wildfires while keeping the areas of critical habitat protected (Stevens et al., 2016; Carrasco et al., 2023). In our study area, the corridor blocks a large portion of the area of concern, thus leaving a limited area available for treatments. Without the corridor, we showed that fuel treatment placement strategies were substantially more effective, and their efficacy increased almost linearly with the number of treated nodes. Even though the wildlife corridor prohibited treatment in a large portion of the area of concern, our results showed a notable reduction in fire spread potential, even in the scenarios with limited treatable areas. The drawback of adding large geographical constraints (such as the wildlife corridor or protected areas), however, is that some parts of the area of concern remained exposed to a higher risk of wildfires (Salis et al., 2016), especially when the size of the area set aside for wildlife protection is large (Venier et al., 2022). Furthermore, the treatments, while spatially restricted to the portions of the area of concern that are outside of the caribou corridor, could serve as a fireguard for further tactical prescribed burning in the corridor to reduce the threat of an incoming fire (Agee et al., 2000; Collins et al., 2010).
As a compromise approach for maximizing caribou habitat conservation without significantly reducing the protection of human infrastructure, one could envision conducting less impactful but more spatially expansive fuel treatments within the corridor. These could include a combination of fuel-reduction strategies, such as forest thinning, mechanical treatment (e.g., mulching), conversion to less-flammable vegetation types, or light surface burning (Ager et al., 2010). However, it is still largely unknown how some of these “light” treatments could contribute to the degradation of caribou habitat (Lesmerises et al., 2013b). Such a recommendation may be more applicable in the context of non-endangered species or less critical habitat.
Potentially, the cost of fuel treatments in the area of concern could be mitigated through commercial harvesting practices aimed to reduce the fuel load (Palma et al., 2007). Exploring the interplay between timber harvest and wildlife conservation goals has been previously undertaken using an optimization-based approach (Acuna et al., 2010; Martin et al., 2017; Yemshanov et al., 2020, 2021b). These models could be enhanced to incorporate the wildfire risk estimates and used to reconcile conservation and forest management goals through the mitigation of wildfire risk. Forest harvesting in particular could be appropriate in situations where fire exposure is moderate, such as the current conditions in our study area, so that adequate planning can be deployed across a multi-year horizon. Such an approach could benefit both forest companies and power companies by providing profits to the former and reducing the costs of mitigation to the latter. This would inevitably come at the expense of reduced habitat suitability for caribou (Leblond et al., 2014); hence, an optimization approach such as the one presented in this study would be advised to reconcile these values and optimally separating them in space.
4.1. Methodological aspects
Our network interdiction approach incorporates the uncertainty about possible fire behavior via probabilistic expectations of possible fire spread, pij, and defining the regions of possible fire spread (subsets Θi) around each site i. Subset Θi delineates the possible fire spread from site i, including rare events. Because the problem minimizes all possible fire spread paths ij to the area of concern, the treatment solutions create a more robust fragmentation to block the most threatening fire spread corridors, superior to solutions targeting individual sites with the highest wildfire exposure or likelihood of ignitions. This explains the model's tendency to allocate some treatments in 2–4-node clusters acting as firebreaks (even if we did not consider spatial contiguity constraints for treatments).
Our model used fixed parameter values to depict the habitat suitability, treatment costs, and fire spread likelihoods in each site; however, these parameters may be subject to natural variability. One could consider robust optimization variants of the proposed formulations to define other parameters as random variables and represent them with a large set of scenarios of possible outcomes (Kouvelis and Yu, 1997). These robust models can use the sets of uncertain habitat values and costs and could provide better support for economic decisions to manage forest landscapes.
The CND problem is known to be NP-hard for general graphs (Arulselvan et al., 2009; Veremyev et al., 2015), which restricts the maximum size of the landscape network the problem can be solved for. However, the problem size in our infrastructure protection problem was smaller than the CND formulation for landscape graphs in Yemshanov et al. (2021a) because the fire spread subsets Θi included only the fire spread paths ij to the sites in the area of concern Q. The problem size can be further reduced by setting the threshold pmin for inclusion of fire spread paths slightly above zero and discarding the rarest, longest fire spread paths which otherwise would significantly increase the size of subsets Θi and the problem size.
The solutions to CND problems 1 and 2 depict the end points of the transition between the aspirations to minimize the spread of frequent and small vs. rare and large fires. Potentially, decision-makers can prioritize the likelihood reduction of fires of a particular range of sizes or fire intensities. To accomplish this, the fire spread likelihoods pij in the objective function would need an adjustment by a scaling coefficient based on the distance between i and j or a fire intensity level characterizing a particular fire spread path ij. This would help adapt the approach to manage or change a specific range of fire regimes or changing landscape configurations, for example, reducing the likelihood of fires that are too intense or too large to be extinguished by local fire crews.
The fuel treatment placement solutions generated by the model are based on fire behavior predictions for a specific single period. In order to develop multi-period treatment placement prescriptions, the model could be applied in sequence. First, the optimal treatment placement solution could be found for the current period and used to update the fuel type map, which would then be used as an input to a fire behavior simulation model to generate a new set of fire spread likelihoods pij. The fuel treatment problem would then be solved with the updated pij values to find the optimal treatment solution for the next period and so on.
Data availability statement
The original contributions presented in the study are included in the article/Supplementary material, further inquiries can be directed to the corresponding author.
Author contributions
DY conceived the project, secured the funding, developed the network optimization approach, and wrote the first draft. M-AP and DD developed the wildfire regime scenarios and ran fire behavior model. DY and NL calculated the probabilities of fire spread from fire behavior model outputs. NL prepared the network data. ABa performed optimization model runs and prepared outputs. YB prepared the forest composition datasets. ABe, JB, DA, M-AP, and DD provided the assumptions and modeling decisions related to the fire simulation modeling. ML and YB respectively developed and implemented the caribou habitat suitability model. All authors contributed to writing the manuscript.
Funding
The funding for this study was provided by Natural Resource Canada's Cumulative Effects and Wildfire Risk Management Programs.
Conflict of interest
The authors declare that the research was conducted in the absence of any commercial or financial relationships that could be construed as a potential conflict of interest.
Publisher's note
All claims expressed in this article are solely those of the authors and do not necessarily represent those of their affiliated organizations, or those of the publisher, the editors and the reviewers. Any product that may be evaluated in this article, or claim that may be made by its manufacturer, is not guaranteed or endorsed by the publisher.
Supplementary material
The Supplementary Material for this article can be found online at: https://www.frontiersin.org/articles/10.3389/ffgc.2023.1186616/full#supplementary-material
References
Acuna, M. A., Palma, C. D., Cui, W., Martell, D. L., and Weintraub, A. (2010). Integrated spatial fire and forest management planning. Canad. J. For. Res. 40, 2370–2383. doi: 10.1139/X10-151
Agee, J. K., Bahro, B., Finney, M. A., Omi, P. N., Sapsis, D. B., Skinner, C. N., et al. (2000). The use of shaded fuelbreaks in landscape fire management. For. Ecol. Manage. 127, 55–66. doi: 10.1016/S0378-1127(99)00116-4
Agee, J. K., and Skinner, C. N. (2005). Basic principles of forest fuel reduction treatments. For. Ecol. Manage. 211, 83–96. doi: 10.1016/j.foreco.2005.01.034
Ager, A. A., Vaillant, N. M., and Finney, M. A. (2010). A comparison of landscape fuel treatment strategies to mitigate wildland fire risk in the urban interface and preserve old forest structure. For. Ecol. Manage. 259, 1556–1570. doi: 10.1016/j.foreco.2010.01.032
Alcasena, F. J., Ager, A. A., Salis, M., Day, M. A., and Vega-Garcia, C. (2018). Optimizing prescribed fire allocation for managing fire risk in central Catalonia. Sci. Total Environ. 621, 872–885. doi: 10.1016/j.scitotenv.2017.11.297
Arulselvan, A., Commander, C. W., Elefteriadou, L., and Pardalos, P. M. (2009). Detecting critical nodes in sparse graphs. Comput. Operat. Res. 36, 2193–2200. doi: 10.1016/j.cor.2008.08.016
Barros, A. M., Ager, A. A., Day, M. A., and Palaiologou, P. (2019). Improving long-term fuel treatment effectiveness in the National Forest System through quantitative prioritization. For. Ecol. Manage. 433, 514–527. doi: 10.1016/j.foreco.2018.10.041
Beverly, J. L., Leverkus, S. E., Cameron, H., and Schroeder, D. (2020). Stand-level fuel reduction treatments and fire behaviour in canadian boreal conifer forests. Fire 3, 35. doi: 10.3390/fire3030035
Boulanger, Y., Gauthier, S., and Burton, P. J. (2014). A refinement of models projecting future Canadian fire regimes using homogeneous fire regime zones. Canad. J. For. Res. 44, 365–376. doi: 10.1139/cjfr-2013-0372
Boulanger, Y., Gauthier, S., Gray, D. R., Le Goff, H., Lefort, P., and Morissette, J. (2013). Fire regime zonation under current and future climate over eastern Canada. Ecologic. Applicat. 23, 904–923. doi: 10.1890/12-0698.1
Boulanger, Y., Parisien, M. A., and Wang, X. (2018). Model-specification uncertainty in future area burned by wildfires in Canada. Int. J. Wildland Fire 27, 164–175. doi: 10.1071/WF17123
Boulanger, Y., and Puigdevall, J. P. (2021). Boreal forests will be more severely affected by projected anthropogenic climate forcing than mixedwood and northern hardwood forests in eastern Canada. Landscape Ecol. 36, 1725–1740. doi: 10.1007/s10980-021-01241-7
Campbell, R. J., and Lowry, S. (2012). Weather-related power outages and electric system resiliency. Congressional Research Service, Library of Congress, Washington, DC.
Carrasco, J., Mahaluf, R., Lisón, F., Pais, C., Miranda, A., de la Barra, F., et al. (2023). A firebreak placement model for optimizing biodiversity protection at landscape scale. J. Environ. Manage. 342, 118087. doi: 10.1016/j.jenvman.2023.118087
Collins, B. M., Stephens, S. L., Moghaddas, J. J., and Battles, J. (2010). Challenges and approaches in planning fuel treatments across fire-excluded forested landscapes. J. For. 108, 24–31. Available online at: https://www.fs.usda.gov/psw/publications/4202/psw_2010_collins001.pdf
Conrad, J., Gomes, C. P., van Hoeve, W.-J., Sabharwal, A., and Suter, J. (2012). Wildlife corridors as a connected subgraph problem. J. Environ. Econ. Manage. 63, 1–18. doi: 10.1016/j.jeem.2011.08.001
Cumming, S. G. (2001). A parametric model of the fire-size distribution. Canad. J. For. Res. 31, 1297–1303. doi: 10.1139/x01-032
Cushman, S. A., Landguth, E. L., and Flather, C. H. (2013). Evaluating population connectivity for species of conservation concern in the American Great Plains. Biodiv. Conservat. 22, 2583–2605. doi: 10.1007/s10531-013-0541-1
Cushman, S. A., McKelvey, K. S., and Schwartz, M. K. (2009). Evaluating habitat connectivity and mapping of corridors between Yellowstone National Park and the Canadian border with landscape genetics and least cost path analysis. Conservat. Biol. 23, 368–376. doi: 10.1111/j.1523-1739.2008.01111.x
Dawe, D. A., Parisien, M.-A., Boulanger, Y., Boucher, J., Beauchemin, A., and Arseneault, D. (2022). Short- and long-term wildfire threat when adapting infrastructure for wildlife conservation in the boreal forest. Ecologic. Applicat. 6, e2606. doi: 10.1002/eap.2606
Dilkina, B., and Gomes, C. (2010). “Solving connected subgraph problems in wildlife conservation,” in Integration of AI and OR Techniques in Constraint Programming for Combinatorial Optimization Problems. Lecture Notes in Computer Science, eds Lodi, A., Milano, M., Toth, P. (Springer, Berlin /Heidelberg), 102–116.
Dilkina, B., Houtman, R., Gomes, C., Montgomery, C., McKelvey, K., Kendall, K., et al. (2016). Trade-offs and efficiencies in optimal budget-constrained multispecies corridor networks. Conservat. Biol. 12, 814. doi: 10.1111/cobi.12814
Driscoll, D. A., Armenteras, D., Bennett, A. F., Brotons, L., Clarke, M. F., Doherty, T. S., et al. (2021). How fire interacts with habitat loss and fragmentation. Biol. Rev. 96, 976–998. doi: 10.1111/brv.12687
Dyer, S. J., O'Neill, J. P., Wasel, S. M., and Boutin, S. (2001). Avoidance of industrial development by woodland caribou. J. Wildlife Manage. 65, 531–542. doi: 10.2307/3803106
Dyer, S. J., O'Neill, J. P., Wasel, S. M., and Boutin, S. (2002). Quantifying barrier effects of roads and seismic lines on movements of female woodland caribou in northeastern Alberta. Canad. J. Zool. 80, 839–845. doi: 10.1139/z02-060
Environment Canada (EC) (2011). Scientific assessment to support the identification of critical habitat for woodland caribou Rangifer tarandus caribou), boreal population, in Canada. (Ottawa, ON).
Environment Canada (EC) (2012). Recovery Strategy for the woodland Caribou (Rangifer tarandus caribou), boreal population, in Canada. Species at risk act recovery strategy series. (Ottawa, ON: Environment Canada). Available online at: http://www.registrelep-sararegistry.gc.ca/virtual_sara/files/plans/rs%5Fcaribou%5Fboreal%5Fcaribou%5F0912%5Fe1%2Epdf (accessed January 10, 2020).
Environment and Climate Change Canada (ECCC) (2017). Report on the progress of recovery strategy implementation for the woodland caribou (Rangifer tarandus caribou), Boreal population in Canada for the period 2012-2017. Species at Risk Act Recovery Strategy Series. (Ottawa, ON). Available online at: http://registrelep-sararegistry.gc.ca/virtual_sara/files/Rs%2DReportOnImplementationBorealCaribou%2Dv00%2D2017Oct31%2DEng%2Epdf (accessed March 5, 2020).
Équipe de Rétablissement du Caribou Forestier du Québec (ÉRCFQ) (2013). Plan de rétablissement du caribou forestier (Rangifer tarandus caribou) au Québec−2013-2023. Du Ministère du Développement Durable, de l'Environnement, de la Faune et des Parcs du Québec, Faune Québec. Available online at: https://mffp.gouv.qc.ca/publications/faune/especes/Plan-retablissement2013-2023.pdf (accessed October 20, 2021).
Flannigan, M. D., Krawchuk, M. A., de Groot, W. J., Wotton, B. M., and Gowman, L. M. (2009). Implications of changing climate for global wildland fire. Int. J. Wildland Fire 18, 483–507. doi: 10.1071/WF08187
Forestry Canada Fire Danger Group (1992). Development and structure of the Canadian Forest Fire Behavior Prediction System. Forestry Canada, Headquarters, Fire Danger Group and Science and Sustainable Development Directorate, Information Report ST-X-3 (Ottawa, ON). Available online at: http://cfs.nrcan.gc.ca/pubwarehouse/pdfs/10068.pdf (accessed February 10, 2019).
Fourrier, A., Pothier, D., and Bouchard, M. (2013). A comparative study of long-term stand growth in eastern Canadian boreal forest: fire versus clear-cut. For. Ecol. Manage. 310, 10–18. doi: 10.1016/j.foreco.2013.08.011
GAMS (GAMS Development Corporation) (2021). General Algebraic Modeling System (GAMS) Version 33. Washington, DC, USA. Available online at: http://www.gams.com (accessed December 10, 2021).
Gannon, B. M., Wei, Y., MacDonald, L. H., Kampf, S. K., Jones, K. W., Cannon, J. B., et al. (2019). Prioritising fuels reduction for water supply protection. Int. J. Wildland Fire 28, 785–803. doi: 10.1071/WF18182
GUROBI (Gurobi Optimization Inc) (2021). GUROBI Optimizer Reference Manual. Version 9.1. Available online at: http://www.gurobi.com (accessed May 5, 2021).
Hanes, C. C., Wang, X., Jain, P., Parisien, M.-A., Little, J. M., and Flannigan, M. D. (2019). Fire-regime changes in Canada over the last half century. Canad. J. For. Res. 49, 256–269. doi: 10.1139/cjfr-2018-0293
Houck, D. J., Kim, E., O'Reilly, G. P., Picklesimer, D. D., and Uzunalioglu, H. (2004). A network survivability model for critical national infrastructures. Bell. Labs Technic. J. 8, 153–172. doi: 10.1002/bltj.10093
James, A. R. C., Boutin, S., Hebert, D. M., and Rippin, A. B. (2004). Spatial separation of caribou from moose and its relation to predation by wolves. J. Wildlife Manage. 68, 799–809. doi: 10.2193/0022-541X(2004)068[0799:SSOCFM]2.0.CO;2
Johnston, L. M., and Flannigan, M. D. (2017). Mapping Canadian wildland fire interface areas. Int. J. Wildland Fire 27, 1–14. doi: 10.1071/WF16221
Kalies, E. L., and Kent, L. L. Y. (2016). Tamm Review: Are fuel treatments effective at achieving ecological and social objectives? a systematic review. For. Ecol. Manage. 375, 84–95. doi: 10.1016/j.foreco.2016.05.021
Klinger, C., Landeg, O., and Murray, V. (2014). Power outages, extreme events and health: a systematic review of the literature from 2011-2012. PLoS Currents 6, 673. doi: 10.1371/currents.dis.04eb1dc5e73dd1377e05a10e9edde673
Koen, E. L., Bowman, J., Sadowski, C., and Walpole, A. A. (2014). Landscape connectivity for wildlife: development and validation of multispecies linkage maps. Methods Ecol. Evolut. 5, 626–633. doi: 10.1111/2041-210X.12197
Kouvelis, P., and Yu, G. (1997). Robust Discrete Optimization and its Applications. Norwell, MA: Kluwer Academic Publishers.
Lacerte, R., Leblond, M., and St-Laurent, M.-H. (2021). Determinants of vegetation regeneration on forest roads following restoration treatments: implications for boreal caribou conservation. Restorat. Ecol. 29, e13414. doi: 10.1111/rec.13414
Lacerte, R., Leblond, M., and St-Laurent, M. H. (2022). End of the road: Short-term responses of a large mammal community to forest road decommissioning. J. Nat. Cons. 69, 126256. doi: 10.1016/j.jnc.2022.126256
Lai, K. J., Gomes, C. P., Schwartz, M. K., McKelvey, K. S., Calkin, D. E., and Montgomery, C. A. (2011). The Steiner multigraph problem: wildlife corridor design for multiple species. In ‘Proceedings of the Twenty-Fifth AAAI Conference on Artificial Intelligence (AAAI-11)', August 7-11, 2011. San Francisco, CA, USA.
Leblond, M., Boulanger, Y., Puigdevall, J. P., and St-Laurent, M. H. (2022). There is still time to reconcile forest management with climate-driven declines in habitat suitability for boreal caribou. Global Ecol. Conservat. 39, e02294. doi: 10.1016/j.gecco.2022.e02294
Leblond, M., Dussault, C., and St-Laurent, M.-H. (2014). Development and validation of an expert-based habitat suitability model to support boreal caribou conservation. Biologic. Conservat. 177, 100–108. doi: 10.1016/j.biocon.2014.06.016
León, J., Reijnders, V. M., Hearne, J. W., Ozlen, M., and Reinke, K. J. (2019). A landscape-scale optimisation model to break the hazardous fuel continuum while maintaining habitat quality. Environ. Model. Assess. 24, 369–379. doi: 10.1007/s10666-018-9642-2
Lesmerises, F., Dussault, C., Drapeau, P., and St-Laurent, M.-H. (2013a). Évaluation des impacts des lignes de transport d'énergie sur l'écologie spatiale du caribou forestier au Québec. Rapport scientifique présenté à Hydro-Québec. Rimouski, Québec.
Lesmerises, R., Ouellet, J. P., Dussault, C., and St-Laurent, M. H. (2013b). The influence of landscape matrix on isolated patch use by wide-ranging animals: conservation lessons for woodland caribou. Ecol. Evol. 3, 2880–2891. doi: 10.1002/ece3.695
Malamud, B. D., Millington, J. D. A., and Perry, G. L. W. (2005). Characterizing wild-fire regimes in the United States. Proceed. Nat. Acad. Sci. USA 102, 4694–4699. doi: 10.1073/pnas.0500880102
Martell, D. L., Gunn, E. A., and Weintraub, A. (1998). Forest management challenges for operational researchers. Eur. J. Operat. Res. 104, 1–17. doi: 10.1016/S0377-2217(97)00329-9
Martin, A. B., Ruppert, J. L. W., Gunn, E. A., and Martell, D. L. (2017). A replanning approach for maximizing woodland caribou habitat alongside timber production. Canad. J. For. Res. 47, 901–909. doi: 10.1139/cjfr-2016-0254
Matisziw, T. C., and Murray, A. T. (2009). Modeling s – t path availability to support disaster vulnerability assessment of network infrastructure. Comput. Operat. Res. 36, 16–26. doi: 10.1016/j.cor.2007.09.004
Matsyputa, D., Prokopyev, O. A., and Zahad, A. (2019). Wildfire fuel management: network-based models and optimization of prescribed burning. Euro. J. Operat. Res. 264, 774–796. doi: 10.1016/j.ejor.2017.06.050
Miller, C., and Ager, A. A. (2013). A review of recent advances in risk analysis for wildfire management. Int. J. Wildl. Fire. 22, 1–14. doi: 10.1071/WF11114
Miller, C., Parisien, M. A., Ager, A. A., and Finney, M. A. (2008). “Valuating spatially-explicit burn probabilities for strategic fire management planning,” in ‘WIT Transactions on Ecology and the Environment: Modelling, Monitoring and Management of Forest Fires', eds., J. De las Heras, C.A. Brebbia, D. Viegas, V. Leone. (Boston, MA: WIT Press), 245–252.
Minas, J. P., and Hearne, J. W. (2016). An optimization model for aggregation of prescribed burn units. TOP 24, 180–195. doi: 10.1007/s11750-015-0383-y
Minas, J. P., Hearne, J. W., and Martell, D. L. (2014). A spatial optimisation model for multi-period landscape level fuel management to mitigate wildfire impacts. Euro. J. Operat. Res. 232, 412–422. doi: 10.1016/j.ejor.2013.07.026
Mumma, M. A., Gillingham, M. P., Johnson, C. J., and Parker, K. L. (2017). Understanding predation risk and individual variation in risk avoidance for threatened boreal caribou. Ecol. Evol. 7, 10266–10277. doi: 10.1002/ece3.3563
Pais, C., Carrasco, J., Moudio, P. E., and Shen, Z.-J. M. (2021). Downstream protection value: detecting critical zones for effective fuel-treatment under wildfire risk. Comput. Operat. Res. 10, 5252. doi: 10.1016/j.cor.2021.105252
Palm, E. C., Suitor, M. J., Joly, K., Herriges, J. D., Kelly, A. P., Hervieux, D., et al. (2022). Increasing fire frequency and severity will increase habitat loss for a boreal forest indicator species. Ecologic. Applicat. 20, e2549. doi: 10.1002/eap.2549
Palma, C. D., Cui, W., Martell, D. L., Robak, D., and Weintraub, A. (2007). Assessing the impact of stand-level harvests on the flammability of forest landscapes. Int. J. Wildland Fire 16, 584–592. doi: 10.1071/WF06116
Parisien, M. A., Kafka, V., Hirsch, K. G., Todd, J. B., Lavoie, S. G., and Maczek, P. D. (2005). Mapping Wildfire Susceptibility with the BURN-P3 Simulation Model; Natural Resources Canada, Canadian Forest Service, Northern Forestry Centre, Information Report NOR-X-405, Edmonton, AB.
Parisien, M. A., Parks, S. A., Miller, C., Krawchuk, M. A., Heathcott, M., and Moritz, M. A. (2011). Contributions of ignitions, fuels, and weather to the spatial patterns of burn probability of a boreal landscape. Ecosystems 14, 1141–1155. doi: 10.1007/s10021-011-9474-2
Parisien, M. A., Walker, G. R., Little, J. M., Simpson, B. N., Wang, X., and Perrakis, D. D. B. (2013). Considerations for modeling burn probability across landscapes with steep environmental gradients: an example from the Columbia Mountains, Canada. Nat. Hazards 66, 439–462. doi: 10.1007/s11069-012-0495-8
Parks, S. A., McKelvey, K. S., and Schwartz, M. K. (2013). Effects of weighting schemes on the identification of wildlife corridors generated with least-cost methods. Conservat. Biol. 27, 145–154. doi: 10.1111/j.1523-1739.2012.01929.x
Parks, S. A., Parisien, M. A., and Miller, C. (2011). Multi-scale evaluation of the environmental controls on burn probability in a southern Sierra Nevada landscape. Int. J. Wildland Fire 20, 815–828. doi: 10.1071/WF10051
Rachmawati, R., Ozlen, M., Reinke, K. J., and Hearne, J. W. (2015). A model for solving the prescribed burn planning problem. SpringerPlus 4, 630. doi: 10.1186/s40064-015-1418-4
Rachmawati, R., Ozlen, M., Reinke, K. J., and Hearne, J. W. (2016). An optimisation approach for fuel treatment planning to break the connectivity of high-risk regions. For. Ecol. Manage. 368, 94–104. doi: 10.1016/j.foreco.2016.03.014
Rayfield, B., Fortin, M.-J., and Fall, A. (2010). The sensitivity of least-cost habitat graphs to relative cost surface values. Landscape Ecol. 25, 519–532. doi: 10.1007/s10980-009-9436-7
Reimer, J., Thompson, D. K., and Povak, N. (2019). Measuring initial attack suppression effectiveness through burn probability. Forests 2, 60. doi: 10.3390/fire2040060
Rempel, R. S., and Hornseth, M. L. (2018). Range-specific seasonal resource selection probability functions for 13 caribou ranges in Northern Ontario. Ontario Ministry of Natural Resources and Forestry, Science and Research Branch, Peterborough, ON. Science and Research Internal File Report IFR-01.
Riley, K. L., Thompson, M. P., Scott, J. H., and Gilbertson-Day, J. W. (2018). A model-based framework to evaluate alternative wildfire suppression strategies. Resources 7, 4. doi: 10.3390/resources7010004
Royle, J. A., Chandler, K., Gazenski, K. D., and Graves, T. A. (2013). Spatial capture-recapture models for jointly estimating population density and landscape connectivity. Ecology 94, 287–294. doi: 10.1890/12-0413.1
Rudolph, T. D., Drapeau, P., Imbeau, L., Brodeur, V., Légar,é, S., and St-Laurent, M.-H. (2017). Demographic responses of boreal caribou to cumulative disturbances highlight elasticity of range-specific tolerance thresholds. Biodiv. Conserv. 26, 1179–1198. doi: 10.1007/s10531-017-1292-1
Salis, M., Laconi, M., Ager, A. A., Alcasena, F. J., Arca, B., Lozano, O., et al. (2016). Evaluating alternative fuel treatment strategies to reduce wildfire losses in a Mediterranean area. For. Ecol. Manage. 368, 207–221. doi: 10.1016/j.foreco.2016.03.009
Saucier, J. P., Grondin, P., Robitaille, A., Gosselin, J., Morneau, C., Richard, P. J. H., et al. (2009). “Écologie forestière, Chapter 4. In ‘Manuel de foresterie', 2ème édition, Ouvrage collectif,” in Éditions MultiMondes et Ordre des ingénieurs forestiers du Québec, Québec 165–315.
Scheller, R. M., Domingo, J. B., Sturtevant, B. R., Williams, J. S., Rudy, A., Gustafson, E. J., et al. (2007). Design, development, and application of LANDIS-II, a spatial landscape simulation model with flexible spatial and temporal resolution. Ecologic. Modell. 201, 409–419. doi: 10.1016/j.ecolmodel.2006.10.009
Schoennagel, T., Balch, J. K., Brenkert-Smith, H., Dennison, P. E., Harvey, B. J., Krawchuk, M. A., et al. (2017). Adapt to more wildfire in western North American forests as climate changes. Proceed. Nat. Acad. Sci. 114, 4582–4590. doi: 10.1073/pnas.1617464114
Seidl, R., Thom, D., Kautz, M., Martin-Benito, D., Peltoniemi, M., Vacchiano, G., et al. (2017). Forest disturbances under climate change. Nat. Clim. Change 7, 395–402. doi: 10.1038/nclimate3303
Singleton, P. H., Gaines, W. L., and Lehmkuhl, J. F. (2002). Landscape Permeability for Large Carnivores in Washington: A Geographic Information System Weighted-Distance and Least-Cost Corridor Assessment. Forestry Sciences Res. Pap. PNW-RP-549 USDA Forest Service, Pacific Northwest Research Station. Available online at: http://www.arlis.org/docs/vol1/51864782.pdf (accessed April 11, 2018).
Species at Risk Act (SARA) (2002). Bill C-5, An act respecting the protection of wildlife species at risk in Canada. 25 August 2010. Available online at: http://laws.justice.gc.ca/PDF/Statute/S/S-15.3.pdf (accessed February 10, 2019).
Stevens, J. T., Collins, B. M., Long, J. W., North, M. P., Prichard, S. J., Tarnay, L. W., et al. (2016). Evaluating potential trade-offs among fuel treatment strategies in mixed-conifer forests of the Sierra Nevada. Ecosphere 7, e01445. doi: 10.1002/ecs2.1445
St. John, R., Tóth, S. F., and Zabinsky, Z. (2018). Optimizing the geometry of wildlife corridors in conservation reserve design. Operat. Res. 66, 1758. doi: 10.1287/opre.2018.1758
Stockdale, C., Barber, Q., Saxena, A., and Parisien, M. A. (2019). Examining management scenarios to mitigate wildfire hazard to caribou conservation projects using burn probability modeling. J. Environ. Manage. 233, 238–248. doi: 10.1016/j.jenvman.2018.12.035
Stocks, B. J., Lynham, T. J., Lawson, B. D., Alexander, M. E., Wagner, C. E. V., McAlpine, R. S., et al. (1989). Canadian forest fire danger rating system: an overview. For. Chron. 65, 258–265. doi: 10.5558/tfc65258-4
Stocks, B. J., Mason, J. A., Todd, J. B., Bosch, E. M., Wotton, B. M., Amiro, B. D., et al. (2003). Large forest fires in Canada, 1959-1997. J. Geophysic. Res. D1 8149, 5–12. doi: 10.1029/2001JD000484
Stralberg, D., Wang, X., Parisien, M.-A., Robinne, F.-N., Sólymos, P., Mahon, C. L., et al. (2018). Wildfire-mediated vegetation change in boreal forests of Alberta, Canada. Ecosphere 9, e02156. doi: 10.1002/ecs2.2156
Terrier, A., Girardin, M. P., Péri,é, C., Legendre, P., and Bergeron, Y. (2013). Potential changes in forest composition could reduce impacts of climate change on boreal wildfires. Ecologic. Applicat. 23, 21–35. doi: 10.1890/12-0425.1
Theobald, D. M., and Romme, W. H. (2007). Expansion of the US wildland–urban interface. Landscape Urban Plann. 83, 340–354. doi: 10.1016/j.landurbplan.2007.06.002
Thomas, D. S., Butry, D. T., Gilbert, S. W., Webb, D. H., and Fung, J. F. (2017). The costs and losses of wildfires. Spec. Publ. 17, 1215. doi: 10.6028/NIST.SP.1215
Tymstra, C., Bryce, R. W., Wotton, B. M., Taylor, S. W., and Armitage, O. B. (2010). Development and Structure of Prometheus: The Canadian Wildland Fire Growth Simulation Model; Natural Resources Canada, Canadian Forest Service, Northern Forestry Centre, Information Report NOR-X-417, Edmonton, AB.
van Vuuren, D. P., Edmonds, J., Kainuma, M., Riahi, K., Thompson, A., Hibbard, K., et al. (2011). The representative concentration pathways: an overview. Climatic Change 109, 5–31. doi: 10.1007/s10584-011-0148-z
Venier, L., Pedlar, J., Higgins, K., Lawrence, K., Walton, R., Boulanger, Y., et al. (2022). Insights into minimum intact forest landscape requirements under regional fire regimes and a changing climate. Biologic. Conservat. 276, 109790. doi: 10.1016/j.biocon.2022.109790
Veremyev, A., Boginski, V., and Pasiliao, E. L. (2014b). Exact identification of critical nodes in sparse networks via new compact formulations. Optimizat. Lett. 8, 1245–1259. doi: 10.1007/s11590-013-0666-x
Veremyev, A., Prokopyev, O. A., and Pasiliao, E. L. (2014a). An integer programming framework for critical elements detection in graphs. J. Combinator. Optimizat. 28, 223–273. doi: 10.1007/s10878-014-9730-4
Veremyev, A., Prokopyev, O. A., and Pasiliao, E. L. (2015). Critical nodes for distance-based connectivity and related problems in graphs. Networks 66, 170–195. doi: 10.1002/net.21622
Vistnes, I., and Nellemann, C. (2007). Impacts of human activity on reindeer and caribou: the matter of spatial and temporal scales. Rangifer Report No.12, 47–56. doi: 10.7557/2.27.3.269
Vors, L. S., and Boyce, M. S. (2009). Global declines of caribou and reindeer. Global Change Biol. 15, 2626–2633. doi: 10.1111/j.1365-2486.2009.01974.x
Wade, A. A., McKelvey, K. S., and Schwartz, M. K. (2015). Resistance-surface-based Wildlife Conservation Connectivity Modeling: Summary of Efforts in the United States and Guide for Practitioners. General technical report RMRS-GTR-333, U.S. Department of Agriculture Forest Service, Rocky Mountain Research Station, Fort Collins, CO.
Walteros, J. L., and Pardalos, P. M. (2012). “Selected topics in critical element detection,” in Applications of Mathematics and Informatics in Military Science, eds J. Daras N. (New York, NY: Springer), 9–26.
Wang, X., Parisien, M.-A., Taylor, S. W., Perrakis, D. D., Little, J., and Flannigan, M. D. (2016). Future burn probability in south-central British Columbia. Int. J. Wildland Fire 25, 200–212. doi: 10.1071/WF15091
Wang, X., Studens, K., Parisien, M. A., Taylor, S. W., Candau, J. N., Boulanger, Y., et al. (2020). Projected changes in fire size from daily spread potential in Canada over the 21st century. Environ. Res. Lett. 15, 104048. doi: 10.1088/1748-9326/aba101
Wei, Y. (2012). Optimize landscape fuel treatment locations to create control opportunities for future fires. Canad. J. For. Res. 42, 1002–1014. doi: 10.1139/x2012-051
Wei, Y., Rideout, D., and Kirsch, A. (2008). An optimization model for locating fuel treatments across a landscape to reduce expected fire losses. Canad. J. For. Res. 38, 868–877. doi: 10.1139/X07-162
Williams, R. J., and Bradstock, R. A. (2008). Large fires and their ecological consequences: introduction to the special issue. Int. J. Wildland Fire 17, 685–687. doi: 10.1071/WF07155
Wittmer, H. U., Sinclair, A. R. E., and McLellan, B. N. (2005). The role of predation in the decline and extirpation of woodland caribou. Oecologia 144, 257–267. doi: 10.1007/s00442-005-0055-y
Yemshanov, D., Haight, R. G., Liu, N., Parisien, M.-A., Barber, Q., Koch, F. H., et al. (2020). Assessing the trade-offs between timber supply and wildlife protection goals in boreal landscapes. Canad. J. For. Res. 50, 243–258. doi: 10.1139/cjfr-2019-0234
Yemshanov, D., Haight, R. G., Liu, N., Rempel, R., Koch, F. H., and Rodgers, A. (2022). Exploring the tradeoffs among forest planning, roads and wildlife corridors: a new approach. Optimization Lett. 16, 747–788. doi: 10.1007/s11590-021-01745-w
Yemshanov, D., Haight, R. G., Rempel, R., Liu, N., and Koch, F. H. (2021b). Protecting wildlife habitat in managed forest landscape—how can network connectivity models help? Nat. Resour. Model. 34, e12286. doi: 10.1111/nrm.12286
Yemshanov, D., Liu, N., Thompson, D. K., Parisien, M.-A., Barber, Q. E., Koch, F. H., et al. (2021a). Detecting critical nodes in forest landscape networks to reduce wildfire spread. PLOS One 21, e0258060. doi: 10.1371/journal.pone.0258060
Yemshanov, D., Simpson, M., Koch, F. H., Parisien, M.-A., Barber, Q., Campioni, F., et al. (2021c). Optimal restoration of wildlife habitat in landscapes fragmented by resource extraction—how can network flow models help? Restorat. Ecol. 21, e13580. doi: 10.1111/rec.13580
Keywords: human infrastructure protection, wildfire fuel connectivity, woodland caribou, critical node detection, wildlife corridor, network interdiction
Citation: Yemshanov D, Dawe DA, Bakalarczyk A, Liu N, Boulanger Y, Boucher J, Beauchemin A, Arseneault D, Leblond M and Parisien M-A (2023) Balancing wildlife protection and wildfire threat mitigation using a network optimization approach. Front. For. Glob. Change 6:1186616. doi: 10.3389/ffgc.2023.1186616
Received: 15 March 2023; Accepted: 28 June 2023;
Published: 24 July 2023.
Edited by:
Palaiologos Palaiologou, Agricultural University of Athens, GreeceReviewed by:
Roghayeh Jahdi, University of Mohaghegh Ardabili, IranFermín Alcasena, Universitat de Lleida, Spain
Copyright © 2023 Yemshanov, Dawe, Bakalarczyk, Liu, Boulanger, Boucher, Beauchemin, Arseneault, Leblond and Parisien. This is an open-access article distributed under the terms of the Creative Commons Attribution License (CC BY). The use, distribution or reproduction in other forums is permitted, provided the original author(s) and the copyright owner(s) are credited and that the original publication in this journal is cited, in accordance with accepted academic practice. No use, distribution or reproduction is permitted which does not comply with these terms.
*Correspondence: Denys Yemshanov, ZGVueXMueWVtc2hhbm92QG5yY2FuLXJuY2FuLmdjLmNh
†Present address: Amanda Bakalarczyk, University of Lethbridge, Lethbridge, AB, Canada