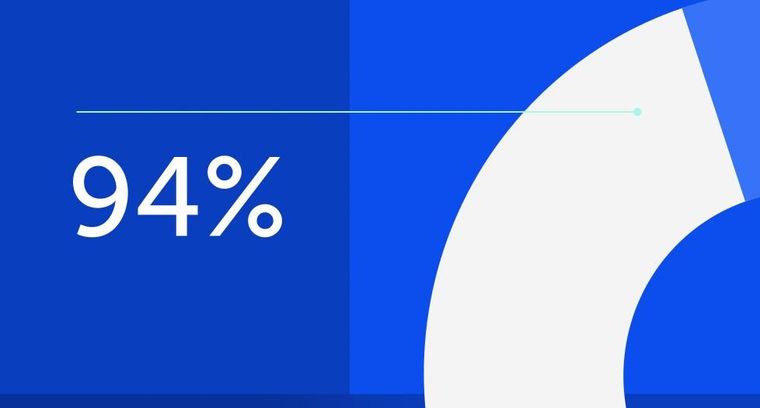
94% of researchers rate our articles as excellent or good
Learn more about the work of our research integrity team to safeguard the quality of each article we publish.
Find out more
ORIGINAL RESEARCH article
Front. For. Glob. Change, 15 March 2023
Sec. Forest Management
Volume 6 - 2023 | https://doi.org/10.3389/ffgc.2023.1144829
This article is part of the Research TopicThe Potential Impacts of Climate Change on the Distribution of Tree SpeciesView all 9 articles
Invasive alien plants pose a serious threat to native ecosystems and their biodiversity. To control their spread and damage, it is critical to clarify the potential distribution patterns of the invaders at the regional scale and their response to climate change. Mimosa bimucronata (DC.) Kuntze, a shrub extremely tolerant of stressful habitat conditions, is used as firewood and hedgerow in the tropics. Since its introduction to China in the 1950s, it has established a sustainable population and become an aggressive invasive species. Due to serious ecological threats in South China, it has been listed as a Key Management Invasive Alien Species. To understand its invasibility in China, two species distribution models (SDMs), BIOCLIM and DOMAIN, were employed to evaluate the main environmental factors regulating its geographical distribution under the current climate and a future climate change scenario with double CO2 concentration. We found an extensive spread of current suitability habitats in South China, concentrated mainly in the tropical-subtropical provinces of Guangdong, Guangxi, Hainan, and Fujian. The complex interplay of local bioclimatic factors shaped the species distribution. Under the current climate, the predicted range was somewhat larger than the current one. Under the climate change scenario, suitable habitats for Mimosa bimucronata would remain similar to the current one. However, its invasiveness in the current biogeographical range would be more serious. The suitability habitats would expand and shift to the Southeastern coastal and Northern parts of South China under future climate change. We suggest adopting early monitoring and eradication measures in potential invasion areas and practical measures to contain its spread. The results could provide a conceptual basis to formulate prevention and control strategies to improve future containment of its invasion.
Biological invasion has become a global issue (Diagne et al., 2021), harming ecology, biodiversity and the national economy (Kumar Rai and Singh, 2020; Feng et al., 2022). In particular, invasive alien plants, characterized by multiple species, wide distribution and rapid reproduction (Vinogradova et al., 2018), have reduced agricultural production, encroached on native-plant habitats, and inflicted long-lasting environmental and economic damages (Diagne et al., 2020). For several decades, the issue of invasive alien plants has remained a major concern of ecologists worldwide (Pyšek et al., 2019).
As a critical element of global change, climate change is indicated mainly by temperature rise, changes in precipitation patterns, and more extreme weather conditions (Zittis et al., 2022). At the regional scale, climatic factors regulate species’ growth, reproduction, phenology, and biogeographic range (Bykova et al., 2012). With increasing greenhouse gas emissions, the future warming trend in China will be further intensified (Mora et al., 2018). Coupled with human disturbances, many ecosystems in China could become fragile and vulnerable to invasion by exotic organisms, especially in the tropics (Ou et al., 2008). The global climate could expand the suitable habitats of invasive alien plants (Kriticos et al., 2003; Lamsal et al., 2018) or shrink them due to climatic conditions exceeding the tolerant range (Panda and Behera, 2019). Therefore, the design of long-term preventive measures requires reliable prediction of the distribution of invasive alien species under future climate change scenarios.
An invasive alien species that can establish or naturalize successfully and build a sustainable population is difficult to eradicate (Allendorf and Lundquist, 2003). Predicting its potential distribution provides critical hints to develop prevention and control strategies to arrest its spread (Gallien et al., 2010). In recent years, species distribution models (SDMs) have been increasingly applied to predict invasive alien species’ potential distribution (Kriticos et al., 2003; Lamsal et al., 2018; Panda and Behera, 2019). SDMs such as BIOCLIM, CLIMEX, DOMAIN, GARP, and MaxEnt, among others, have been commonly used (Xie et al., 2022; Zhang et al., 2022). BIOCLIM was the first SDM package widely adopted to quantify species’ environmental niche, identify areas where a species might be invasive, assist conservation planning, and evaluate the probable impacts of climate change on species distributions (Booth et al., 2014). BIOCLIM and DOMAIN, supported by DIVA-GIS (Hijmans et al., 2001), offer effective methods that have been widely applied in modeling species distribution (Giliba and Yengoh, 2020).
Until recently, woody plants were not widely regarded as significant invasive alien species (Richardson and Rejmánek, 2011). However, thousands of tree and shrub species have been relocated worldwide. Many woody species have spread from planting locations, and some are now among the most common and destructive invasive organisms (Welgama et al., 2022). Mimosa bimucronata (DC.) Kuntze. is a tiny, spiky tree or shrub with many branches and a low, dense crown in Leguminosae. Its native biogeographical range stretches from Bolivia to Brazil and Northeastern Argentina, growing primarily in the seasonally dry tropical biome (Olkoski and Wittmann, 2011). M. bimucronata was introduced to China about seventy years ago by the overseas Chinese of San Francisco and cultivated initially in Zhongshan, Guangdong Province (Xu et al., 1986). The species has adapted well to barren and exposed habitats. It is an excellent firewood and hedgerow species with strong adaptability and regeneration capacity (Lan et al., 2019), and used for soil improvement, soil and water conservation, and nectar plant (Brancalion et al., 2008). Therefore, the species has been widely cultivated recently in China.
However, M. bimucronata has escaped into the wild and spread in Southern China, sometimes establishing dominating or monospecific patches. Its aggressive growing habit can crowd out or even kill native species, significantly suppressing the recipient community’s biodiversity (Cheng et al., 2011). The species has caused serious ecological damage to the distribution area, even threatening livestock and human health (Lan et al., 2019). According to the “Biosecurity Law of the People’s Republic of China,” M. bimucronata has been included in the national “List of Key Management Invasive Alien Species” from January 2023 (Ministry of Agriculture and Rural Affairs of the People’s Republic of China, 2022), stipulating that it should be subject to more stringent biosafety regulation.
The extensive studies of M. bimucronata cover phytochemistry and pharmacology (Schlickmann et al., 2017), reproductive characteristics (Seijo and SolÍS Neffa, 2004; Ferreira, 2018), invasive organism control (Xu, 2014), etc. Its research in China is limited. There is a lack of targeted prevention and control tools, especially at the macro level. Without effective control, M. bimucronata could strengthen its hold in the current distribution area and further expand from the bridgeheads. For this reason, using SDMs to identify its potential spread risk can provide a useful tool for monitoring and early invasion warning. Applying the BIOCLIM and DOMAIN modeling methods, this study’s objectives are: (1) to determine and evaluate the key climatic factors shaping M. bimucronata’s geographical distribution; and (2) to anticipate the probable spread of M. bimucronata’s suitable habitats under the current and future climate scenarios. The findings could offer a useful theoretical framework for monitoring, early warning, and effective prevention and control of M. bimucronata spread in China and elsewhere facing similar biological invasion issues.
A total of 967 known locations with M. bimucronata occurrence were recorded during 2020–2022 from NSII (National Specimen Information Infrastructure),1 GBIF (the Global Biodiversity Information Facility),2 relevant literature and documents (Xu et al., 1986; Cheng et al., 2011; Xu, 2014), and field surveys. After screening to remove ambiguous or repetitive data, 125 occurrence records of M. bimucronata were kept for subsequent analyses (Figure 1).
Figure 1. Locations of 125 selected occurrence records (shown by white circles) of Mimosa bimucronata in China in relation to elevation. Images on the left show the species’ flowers, fruits and a small patch of the species.
The geographical distribution data were imported into DIVA-GIS 7.5 (Hijmans et al., 2001), and 19 current and future bioclimatic factors were extracted as environmental variables. DIVA-GIS is a free computer program for mapping and geographic data analysis, particularly useful for analyzing biodiversity data, such as assessing biodiversity hotspots, predicting species distribution and managing protected areas (Sankar et al., 2015). Current and future climate data were extracted from WorldClim3 (Supplementary Table 1). The current climate data are mainly based on meteorological observations of weather stations worldwide from 1970 to 2000. Global climate data were generated using spatial interpolation (spatial resolution of 2.5’) (Fick and Hijmans, 2017). Future climate (under the double CO2 concentration scenario) was simulated for 2100 (2.5’ spatial resolution) using the National Center for Atmospheric Research Community Climate Model version 3 (CCM3) (Govindasamy et al., 2003). A Pearson correlation analysis of the 19 bioclimatic variables was performed utilizing PAST 4.11 (Hammer et al., 2001) to eliminate multicollinearity influence on the modeling process and select the most fitting variables with strong contributions to the model (Tesfamariam et al., 2022). Ten highly correlated variables with Pearson correlation coefficients (r) more than 0.75 were removed to improve model-simulation accuracy (Padalia et al., 2014). As a result, only nine variables were preserved to model the potential distribution of M. bimucronata under current and future climate scenarios (Supplementary Table 1).
Two SDMs (BIOLIM and DOMAIN) were used to predict the potential distribution of Mimosa bimucronata in China under different climate scenarios. For BIOCLIM, the range of meteorological conditions appropriate for a species’ occurrence can be determined using the environmental data from all known species distribution locations (Booth et al., 2014). The multivariate analysis uses the percentile distribution of each climatic variable inside each grid in the species distribution zone (Nix, 1986). The BIOCLIM can identify a suitable location if the ranges of all climate variables in the grid fall within boundaries appropriate to those appropriate for the species.
For DOMAIN, it uses a Gower distance-based point-to-point similarity measure, which generates a distance matrix from a list of species characteristics. Based on each potential site’s proximity to the location of the most similar positive occurrence, DOMAIN can classify each potential site’s habitat compatibility index (Carpenter et al., 1993). The ecological niche of each species is then determined by selecting a threshold value of appropriateness.
This study divided a set of georeferenced records of M. bimucronata occurrence into two parts: 75% of data were used to drive the model, and 25% were used to verify the modeling results. Based on the default fitness of the two models, the suitable habitats of M. bimucronata were categorized into six classes: excellent, very high, high, medium, low, and not suitable. The area under the curve (AUC) of the receiver operating characteristic (ROC) plot was used to evaluate the model accuracy (Gebrewahid et al., 2020). The AUC ranges from 0 to 1 and measures model performance. The ideal level of discrimination is 1.0 (Abolmaali et al., 2018). The above processes were conducted in DIVA-GIS 7.5 (Hijmans et al., 2001).
The nine screened bioclimatic variables were subjected to principal component analysis (PCA) to explore the key climatic factors affecting the geographic distribution of M. bimucronata. The process was conducted in PAST 4.09 (Hammer et al., 2001). The effect factors of M. bimucronata’s distribution patterns were also assessed by the “most limiting factor model” produced by the two models in DIVA-GIS 7.5 (Hijmans et al., 2001; Zhu et al., 2021).
The 125 occurrence records, the literature and the field surveys found that M. bimucronata has an extensive distribution region covering 11 provinces (including other administrative units) in Southern China. From east to west, they included Taiwan, Fujian, Guangdong, Hong Kong, Macau, Jiangxi, Hainan, Guangxi, Guizhou, Chongqing, and Yunnan (Figure 1). The Southernmost point of the distribution was Sanya (Hainan), the Northernmost point was Chongqing, the Easternmost point was Taipei (Taiwan), and the Westernmost point was Mengwa (Yunnan). The current distribution areas mainly occupy China’s tropical and South subtropical zones.
Although M. bimucronata was distributed widely, most occurrences were concentrated in Guangdong and Guangxi provinces (Figure 1). In Guangdong, the occurrence records were found mainly in the Eastern part and the Pearl River Delta, including Hong Kong and Macau. The earliest introduction site was in the Pearl River Delta. In Guangxi, the occurrences were found in almost all cities, especially in the North and South. In addition to Guangdong and Guangxi, the Southeast part of Fujian, the east part of Yunnan and Hainan also had sizeable populations.
The PCA of independent bioclimatic variables defined an environmental space of reduced dimensionality that allowed displaying of the key factors molding the distribution for M. bimucronata (Figure 2). The first two axes of the PCA explained 67.23% of the total variance in the data. The first principal component explained 43.42% of the variance, mainly variables representing temperature in the warm season. The second principal component explained 23.81% of the variance, primarily variables representing the combination of temperature and precipitation. The results indicated that the precipitation effect was weaker than the temperature. PCA considered the overall conditions of the M. bimucronata distribution area, not covering the impact of each specific area.
Figure 2. Principal component analysis (PCA) of nine bioclimatic variables for the 125 occurrence records of Mimosa bimucronata. The first PCA axis accounts for 43.42% of the total variance, mainly representing the mean temperature of the warmest quarter (Bio10), the maximum temperature of the warmest month (Bio5), and isothermality (Bio3). The second PCA axis accounts for 23.81% of the total variance, mainly representing the minimum temperature of the coldest month (Bio6), the mean temperature of the wettest quarter (Bio8), and the precipitation of the driest month (Bio14).
We established modeled distribution maps with the BIOCLIM and DOMAIN/Most Limiting Factor model to diagnose climatic suitability areas and gain insight into the limiting factors of distribution patterns (Figure 3). Locally, the bioclimatic factors played different roles in shaping the distribution of M. bimucronata. In the BIOCLIM model (Figure 3A), the Eastern region was mainly influenced by Bio6, Bio8, Bio10, and Bio18, covering mainly Guangdong, Hainan, Fujian, and Jiangxi; the Central and Western regions were primarily affected by Bio3, Bio6, and Bio14, covering mainly Guangxi, Guizhou, Yunnan, and Chongqing. In the DOMAIN model (Figure 3B), the influence of Bio8 and Bio14 in each region may be stronger than other bioclimatic factors. However, several bioclimatic factors jointly influenced the distribution in the current major distribution areas, such as Guangdong and Guangxi. The climatic effects on suitable habitats appeared to be complex and synergistic.
Figure 3. Distribution patterns of the limiting factors shaping the distribution areas of Mimosa bimucronata generated by the: (A) BIOCLIM model; and (B) DOMAIN model. The selected nine bioclimatic variables, shown in different colors, had the greatest impact on predicting suitable habitats in the distribution area. The meaning of the bioclimatic variables can be found in Supplementary Table 1.
Applying DIVA-GIS 7.5, the suitable potential areas of M. bimucronata based on the current climatic regime predicted by the BIOCLIM and DOMAIN models are presented in Figure 4. The two models yielded some differences in predicting suitable distribution areas. However, they shared the prediction of Guangxi, Guangdong and Hainan as the main suitable distribution areas. Other provinces with high, very high and excellent suitability levels were present in Taiwan, Southeastern and Northwestern Fujian, Southern Jiangxi, Southern Hunan, Southern Guizhou, Central and Southwestern Yunnan, Eastern Sichuan, and Chongqing (Figures 4A, B).
Figure 4. Potential suitability areas of Mimosa bimucronata under the current climate scenario in China (1970–2000) generated by the: (A) BIOCLIM model; and (B) DOMAIN model. They are divided into six categories based on the calculated habitat suitability index. Red color denotes excellent suitability habitat, orange very high, yellow high, light green medium, dark green low, and gray not suitable.
By habitat suitability, the total areas for excellent, very high, high, medium, low, and not suitable categories are: 3.18 km × 104 km, 14.99 km × 104 km, 13.20 km × 104 km, 15.04 km × 104 km, 41.95 km × 104 km, 873.34 km × 104 km for BIOCLIM, and 0.60 km × 104 km, 13.75 km × 104 km, 24.03 km × 104 km 61.18 km × 104 km, 522.77 km × 104 km, 339.36 km × 104 km for DOMAIN (Table 1). The excellent category is mainly concentrated in South China (Figure 4), especially Guangdong and Guangxi. The suitable habitat categories covered notably different land areas because of the different algorithms defined by the two models. The predicted potential biogeographical range was larger than the current distribution area (Figure 1).
Table 1. Predicted suitable areas (104 km2) for Mimosa bimucronata under the current and future climate scenarios are classified into five suitability categories.
In 2100, the potential suitable habitats for M. bimucronata would change compared to the potential distribution area under the current climate scenario (Table 1 and Figure 5). The results generated by BIOCLIM and DOMAIN models were different. For BIOCLIM, the potential distribution area of the excellent tier increased sharply by 57.56%; meanwhile, the tiers of very high, high and medium all decreased by −0.82% (14.87 km × 104 km), −17.28% (10.92 km × 104 km) and −11.91% (13.25 km × 104 km), respectively. Although the suitable area of excellent, very high, high and medium for the DOMAIN model decreased, the decline was not sharp, −3.49% (0.58 km × 104 km), −2.34% (13.43 km × 104 km), −2.62% (23.40 km × 104 km), and −1.35% (60.36 km × 104 km), respectively. However, low and not suitable increased and decreased by 17.09% (612.12 km × 104 km) and −25.80% (251.81 km × 104 km), respectively.
Figure 5. Potential suitable habitats of Mimosa bimucronata under the climate change scenario (double CO2 concentration) in 2100 generated by the: (A) BIOCLIM model; and (B) DOMAIN model. Red color denotes excellent suitability habitat, orange very high, yellow high, light green medium, dark green low, and gray not suitable.
The performance of the models can be classified using AUC as failing (0.5–0.6), poor (0.6–0.7), fair (0.7–0.8), good (0.8–0.9), or excellent (0.9–1.0) (Wei et al., 2018). The mean AUC in the current and future periods were rated excellent or good: the AUC values were 0.917 ± 0.017 and 0.933 ± 0.021 for BIOCLIM, and 0.858 ± 0.033 and 0.879 ± 0.074 for DOMAIN, respectively. The results implied that the simulations were highly reliable and could be used to study the current climate and climate change impact on M. bimucronata distribution in China.
Many studies have confirmed that sample size can affect the accuracy of SDMs (Hernandez et al., 2006; Wisz et al., 2008; Bean et al., 2012). For BIOCLIM, the simulation results of 75–100 species distribution data could achieve the maximum modeling accuracy, and adding more data did not bring significant improvement (Shao et al., 2009). A similar result was obtained by predicting the distribution patterns for 192 species of woody plants in Israel (Kadmon et al., 2003). Relative to other algorithms, DOMAIN had an intermediate performance at the largest sample size (100) and was among the best performance at the lowest sample size (30) (Wisz et al., 2008). Therefore, this study’s over 100 sample size could meet the modeling accuracy requirements.
BIOCLIM and DOMAIN are typical and widely used SDMs (Navarro-Cerrillo et al., 2011; Natale et al., 2013; Battini et al., 2019). At the initial modeling stage, BIOCLIM generates a climatic profile of a species’ abiotic habitat, known as the climatic envelope. It is defined by the minimum and maximum values of each environmental parameter within a subset of all grid cells where a species occurs, forming a climatic minimum rectangular envelope in three-dimensional space (Walter et al., 2016). The drawback of the climatic envelope approach is treating extreme environmental conditions to maintain population stability, which is unreasonable (Cerdeira et al., 2018). Although species may survive, they cannot sustain the continuity of the population under extreme environmental conditions. Therefore, to reduce the influence of extreme values on model performance and improve predictive ability, the values of each variable of all samples will usually be sorted before defining the boundary of the multidimensional hyperrectangle (Cerdeira et al., 2014). Some extreme samples (e.g., the highest 5%) are selected, and the extreme values of these environmental variables are averaged in demarcating the hyperrectangle boundary, thereby improving the estimation of the species’ potential distribution area (Xu et al., 2015; Walter et al., 2016).
DOMAIN predicts potential distributions using a range-standardized, point-to-point similarity metric. It offers a simple and robust method for predicting plant and animal species’ potential distributions (Carpenter et al., 1993). DOMAIN had an advantage over comparable methods for its ability to operate effectively with only species presence records and a limited set of biophysical attributes (Hernandez et al., 2006). A threshold must be established to exclude unsuitable distribution areas when determining a species’s habitat or biogeographical range. In contrast to the BIOCLIM, the environment hyperrectangle determined by DOMAIN in the environment variable space was not necessarily continuous (Xu et al., 2015). Using a continuous similarity function increases the flexibility of DOMAIN as a heuristic tool, making it suitable for survey design, reserve selection, and potential mapping of rare and common species (Elith and Graham, 2009). Therefore, there was a notable difference in the suitable range area predicted by the two models (Figures 4, 5 and Table 1).
Compared to MaxEnt (Abolmaali et al., 2018; Wei et al., 2018; Gebrewahid et al., 2020; Tesfamariam et al., 2022), one of the most popular SDMs, BIOCLIM and DOMAIN have certain shortcomings. However, both models are useful in estimating a species’ environmental niche, identifying places where a species may be invasive, supporting conservation planning, and predicting the likely implications of climate change on species distribution (Carpenter et al., 1993; Elith and Graham, 2009; Shao et al., 2009; Booth et al., 2014). Hence, the study can provide a helpful basis for managing invasive M. bimucronata.
Most studies, including our study, have used AUC to test model accuracy (Battini et al., 2019; Giliba and Yengoh, 2020). However, overall accuracy measured by AUC is not the only factor in choosing a model. It is also critical to reduce the possibility of false negatives, which is especially essential in the research on invasive alien species (Natale et al., 2013). Based on this judgment, some studies suggested that models with a high proportion of correctly predicted presences (i.e., with high sensitivity) and low omission error should be preferred (low false negatives) (Santika, 2011). In this regard, DOMAIN and BIOCLIM are suitable models for predicting the potential distribution of M. bimucronata in China. Although some studies pointed out that DOMAIN and BIOCLIM did not perform as well as other models (Wisz et al., 2008; Bean et al., 2012), our investigation results verified that the actual invasion range of M. bimucronata was consistent with the simulation results. Therefore, we submit that the species distribution should be studied in relation to actual distribution before selecting the model.
Invasive plants often experience large population explosions in alien lands (Kumar Rai and Singh, 2020). However, their distribution patterns are fundamentally governed by hydrothermal conditions like other plants (Hessburg et al., 2019). The climate is the most significant factor influencing the spread and intensity of alien plant invasions. Studies in China indicated that Ageratina adenophora and Eupatorium odoratum responded similarly to temperature and precipitation factors, although Mikania micrantha was more temperature-sensitive (Fang et al., 2021). For the invasive tropical fern (Cyclosorus afer) in Nigeria, five environmental variables, including annual mean temperature, mean temperature of the driest quarter, precipitation seasonality, precipitation of the driest quarter, and precipitation of the coldest quarter, were the main limiting factors (Akomolafe and Rahmad, 2019). Therefore, the response of different invasive plants to the climatic environment is highly variable. The environmental factors shaping the geographic distribution of M. bimucronata were complicated, as evidenced by the influence at the regional and local scales (Figures 2, 3). However, PCA and SDMs results implied that temperature had a stronger effect than precipitation.
Based on PCA and SDM results (Figures 2, 3), Bio10 (mean temperature of the warmest quarter) and Bio6 (minimum temperature of the coldest month) had a substantial influence on the distribution of M. bimucronata. Seed germination of M. bimucronata can occur in a wide temperature range from 15 to 40°C, but the most suitable range is 25–30°C (Lan et al., 2019). In China, this optimal germination condition can only be attained in the tropical zone. In addition, the actual and predicted potential distribution areas (Figures 1, 4) suggested that M. bimucronata was more concentrated in Southern China or tropical regions, where the annual mean temperature was mostly above 18°C without a cold winter (Li et al., 2010). If they occur at all, the most extreme low temperatures in winter in Southern China are above 0°C and usually last for a short duration in a day. This mild climatic regime indicates that M. bimucronata requires warm conditions for growth and germination and has heat tolerance (Brancalion et al., 2008). The geographical distribution pattern of M. bimucronata signifies an affinity for humidity and heat; hence, it is suitable for tropical and South subtropical (Liu and Liu, 2011) regions. Therefore, many areas in Southern China lie within its possible invasion range.
In addition, M. bimucronata is suitable for relatively infertile soils developed from granite, sandy shale, coastal sediments, etc. (Xu et al., 1986). It does not require rich soil nutrients (Liu and Liu, 2011). In summary, M. bimucronata is highly resistant to various environmental stresses. It can survive under unfavorable environmental conditions, equipping it with a strong competitive edge to invade and establish successfully. Due to anthropogenic introduction, some patches dominated by its aggressive invasiveness can be established quickly, often crowding out native species. Hence, M. bimucronata has developed into a particularly invasive alien species less than 100 years after its introduction into China (Shao et al., 2008).
We conducted a validation survey of the invasive distribution of M. bimucronata in Southern China. The results differed somewhat from model predictions due to the absence in a few areas. Two possible reasons could be proposed for the discrepancy: (1) Insufficient environmental data. We could not obtain detailed environmental data for micro-habitats, and the acquired data were large-scale with limited accuracy and time lag. Consequently, the detailed ecological requirements of M. bimucronata still need to be ascertained by more in-depth environmental data. (2) Limited scope of the models. The models are based on the actual occurrence points of a species and associated environmental variables to simulate its projected ecological demand (ecological niche) and predict its potential distribution. Other factors with a bearing on species distribution, such as intra- and inter-species competition (Franklin, 2010), were not considered in the assessment. However, species distribution could be influenced by various biotic and abiotic factors (King et al., 2021). Adding a wider range of data in future studies should improve simulation results. With more data and better prediction, the simulated potential suitable range of M. bimucronata would better match the real-world distribution area.
Global climate change can impose significant impacts on the distribution patterns of native and exotic species (Morriën et al., 2010; Wei et al., 2018; Xie et al., 2022). For native species, global warming will reduce the habitat suitability of many wild plants in their current natural habitats. The affected species will adapt to the changing climate by migrating to cooler habitats and natural selection (Abrha et al., 2018; Abdelaal et al., 2019). However, future climate change may trigger invasive plant species to fill climatically suitable potential ranges (Adhikari et al., 2019). The niche model simulated the distribution patterns of four invasive plants causing considerable damage in China. Their suitable habitat areas would expand under future climate scenarios (Tu et al., 2021). Three of the most dominant and aggressive invasive plants in the Southeast United States were assessed. The results showed that climate change would bring considerable range expansions (Bradley et al., 2010). Many studies found evidence that climate would enlarge the distribution areas of invasive alien species (Storkey et al., 2014; Adhikari et al., 2019). However, the opposite result has been projected for some species (Padalia et al., 2015; Panda and Behera, 2019).
The two models predicted a large difference in “excellent” suitable areas of M. bimucronata under the climate change scenario (Table 1 and Figure 5). BIOCLIM projected a notable 57.56% increase in excellent habitats, while DOMAIN showed a small −3.49% decrease. The discrepancy can be attributed to different model algorithms and suitability classification criteria (Carpenter et al., 1993; Booth et al., 2014). We reckon that the suitability habitat classes of excellent, very high and high could be considered as a whole. Aggregating the areas of these three classes indicated a reduction of 1.83 and 2.53% in BIOCLIM and DOMAIN (Table 1), respectively. Therefore, doubling CO2 concentration bringing too high temperatures would limit the distribution of M. bimucronata.
Based on the current distribution pattern (Figure 1), M. bimucronata is a warmth-loving plant, and a warming climate should be conducive to its spread. Why would its distribution area contract slightly under the future climate scenario? Low temperatures constitute another limiting factor for its distribution. Low temperatures can damage the mechanical structure of plants to restrict species distribution at high latitudes and altitudes (Körner, 2012). Seasonal temperature changes have a powerful influence on plants’ carbon assimilation and distribution in the tropics (Chen et al., 2022). South China has distinct rainy and dry seasons with a small annual temperature amplitude favorable for the growth and spread of M. bimucronata. With global climate change, the high altitude and high latitude regions should become warmer and wetter in the future (Pepin et al., 2015), and South China coastal areas will also become warmer. In comparison (Figures 4, 5), the suitability habitats of M. bimucronata were predicted to expand in Eastern Fujian and Northwestern Guangxi under the future climate scenario. At the northern edge, the low temperatures would limit its growth. Therefore, the future distribution area of M. bimucronata would be almost the same as the current one. However, managing and preventing M. bimucronata spread in Guangxi, Guangdong, and other coastal areas would become more demanding.
The SDMs can predict an increase in the probability of adding or reducing suitability habitats (Royle et al., 2012). The actual distribution pattern depends on specific responses of the species to the changing environment. Therefore, more care should be taken in applying SDMs. Different models could yield notably different species suitable ranges and actual distribution. Habitat fragmentation, geographic isolation, and species migration rates could profoundly affect the actual species distribution (Schwartz, 1993). The SDMs adopted in the study provided useful predictive tools. The invasive plants have various dispersal modes and notable variations in dispersal distance under different conditions (Allendorf and Lundquist, 2003). The distribution and dispersal of species are also related to their population structure, environment and anthropogenic factors (Chen et al., 2014). For better control of invasive plants, their dispersal patterns should be studied in greater depth, and complex models should generate a higher temporal and spatial accuracy of invasive-species dynamics.
Generally, invasive plants are difficult to monitor when the population size is small. They are often at the stage of population outbreak and are difficult to eradicate when their invasive spread becomes noticeable (Mehta et al., 2007). Invasive species often adapt quickly to new selection pressures (van Boheemen et al., 2019). Hence, risk assessment, early warning and rapid interception are important means to prevent and control biological invasion (Allendorf and Lundquist, 2003). Distinguishing the invasibility levels of different regions and identifying priority control areas offer critical management tools (Welgama et al., 2022). Considering the status of biosafety management in different regions of China, the control of M. bimucronata should be based on its actual distribution and potential distribution trend under different climate scenarios.
We suggest that the actual distribution, potential distribution, and future expansion of M. bimucronata should be assessed at the prefecture level or above so that targeted prevention and control measures can be designed from a macroscopic perspective: (1) Monitoring and interception zones should be assigned at the front edge of its spread, and strengthened interception zones in anthropogenic communities should be established to enhance the resistance of the native plant communities to invasion. (2) The occurrence areas should be listed as key control targets, emphasizing field survey, eradication, alternative control and ecological restoration to compress its distribution gradually. (3) The potential habitats should be designated as key areas for dynamic monitoring, risk assessment and early warning. The plant should be removed as soon as it is found, especially in the habitats of the excellent, very high and high tiers. In addition, future expansion areas should be priority prevention areas to receive emergency defense measures to avoid new invasions induced by climate change.
At present, in China, effective control methods for M. bimucronata are lacking. Manual uprooting, eradication, and other physical control methods are time and labor-intensive, and chemical control may cause pollution (Woyessa, 2022). Combining biological control and integrated utilization presents a promising control approach that should be adopted. Some practical recommendations are proposed to control the invasion better: (1) To understand its distribution and damage, local management can conduct a comprehensive survey of M. bimucronata. Relevant departments can control and eradicate it systematically and curb its spread (Lan et al., 2019). (2) Public education efforts can be augmented to inform the public about its harms, raise awareness of preventing invasion by alien species, protect ecological integrity, and avoid further spread (Fukano and Soga, 2019). (3) Domestic and foreign collaboration should be enhanced to learn from the control experience of other places and develop a more effective defense and control system (Liebhold et al., 2021). The cooperation among relevant departments, including quarantine, agriculture, forestry, conservation and research institutes, can be strengthened. (4) Integrated use and management can be incorporated into the management plan. M. bimucronata can grow rapidly in stressful sites and tolerate waterlogging; such traits can be enlisted as a slope and riverbank protection plant (Xu, 2014). In addition, the species can also be used as charcoal plantations (Xu et al., 1986). Using it to restore degraded habitats or green spaces in tropical areas has been reported in other countries (Brancalion et al., 2008; Rossi et al., 2011). In its management and utilization, priority should be accorded to containing its ecological damage.
From a macroscopic perspective, we predicted the suitable potential range of M. bimucronata under climate change. The results could provide a reference for local authorities to develop control strategies. Nevertheless, some differences between simulated results and potentially suitable areas are expected. Our study assumed that the variables would remain stable, which is not the case in reality. The quality of data used in this study, such as environmental, was not optimal, calling for further refinements in the future. In particular, compound and interacting social, economic, and environmental factors need to be added to improve the simulation. The invasion pathways and modes of M. bimucronata can be traced in greater detail to produce a more comprehensive and reliable prediction of invasion trends.
Developing long-term effective prevention and control measures for an invasive alien species requires knowledge of its distribution under future climate change scenarios. In this study, we used BIOCLIM and DOMAIN modeling tools to simulate the suitable habitats of M. bimucronata in China under the present climate and future climate change scenario with a doubling of CO2 concentration. The prediction results were reliable by objective performance indicators and in good agreement with the actual distribution area of M. bimucronata. Under the current climate scenario, the potential biogeographical range of the warmth-preferring M. bimucronata is mainly found in Southern China’s tropical or subtropical zones. The key environmental factors regulating its growth are the coldest month (Bio6), the mean temperature of the wettest quarter (Bio8), and the mean temperature of the warmest quarter (Bio10). Generally, the temperature is the most critical climate factor limiting the distribution of M. bimucronata, with a stronger influence than precipitation. In the climate change scenario, the area of the future suitability habitat of M. bimucronata is similar to the current range. However, the species’ invasiveness in the Southeast coastal areas and Northern part of South China will be reinforced, requiring local management to be more vigilant. This study presents the suitable distribution and potential invasion areas of M. bimucronata due to climate change, providing the conceptual basis and practical guidance to enhance its future control.
Publicly available datasets were analyzed in this study. This data can be found here: https://doi.org/10.4121/21989216.v1.
CX and CJ conceived the project and designed the methodology. CX and ML analyzed the data and led the writing of the manuscript. ML and DL collected the data. All authors contributed critically to the drafts, read, and approved the final manuscript.
We thank the support from Tropical Biodiversity and Bioresource Utilization Laboratory, Qiongtai Normal University (QTPT21-5). We are also grateful to the reviewers for suggestions to improve our manuscript.
The authors declare that the research was conducted in the absence of any commercial or financial relationships that could be construed as a potential conflict of interest.
All claims expressed in this article are solely those of the authors and do not necessarily represent those of their affiliated organizations, or those of the publisher, the editors and the reviewers. Any product that may be evaluated in this article, or claim that may be made by its manufacturer, is not guaranteed or endorsed by the publisher.
The Supplementary Material for this article can be found online at: https://www.frontiersin.org/articles/10.3389/ffgc.2023.1144829/full#supplementary-material
Abdelaal, M., Fois, M., Fenu, G., and Bacchetta, G. (2019). Using MaxEnt modeling to predict the potential distribution of the endemic plant Rosa arabica Crép. in Egypt. Ecol. Inform. 50, 68–75. doi: 10.1016/j.ecoinf.2019.01.003
Abolmaali, S. M.-R., Tarkesh, M., and Bashari, H. (2018). MaxEnt modeling for predicting suitable habitats and identifying the effects of climate change on a threatened species, Daphne mucronata, in central Iran. Ecol. Inform. 43, 116–123. doi: 10.1016/j.ecoinf.2017.10.002
Abrha, H., Birhane, E., Hagos, H., and Manaye, A. (2018). Predicting suitable habitats of endangered Juniperus procera tree under climate change in Northern Ethiopia. J. Sustain. For. 37, 842–853. doi: 10.1080/10549811.2018.1494000
Adhikari, P., Jeon, J.-Y., Kim, H. W., Shin, M.-S., Adhikari, P., and Seo, C. (2019). Potential impact of climate change on plant invasion in the Republic of Korea. J. Ecol. Environ. 43:36. doi: 10.1186/s41610-019-0134-3
Akomolafe, G. F., and Rahmad, Z. B. (2019). Modelling the distribution of a potential invasive tropical fern, Cyclosorus afer in Nigeria. Afr. J. Ecol. 57, 304–313. doi: 10.1111/aje.12603
Allendorf, F. W., and Lundquist, L. L. (2003). Introduction: Population biology, evolution, and control of invasive species. Conserv. Biol. 17, 24–30.
Battini, N., Farías, N., Giachetti, C. B., Schwindt, E., and Bortolus, A. (2019). Staying ahead of invaders: Using species distribution modeling to predict alien species potential niche shifts. Mar. Ecol. Progr. Ser. 612, 127–140. doi: 10.3354/meps12878
Bean, W. T., Stafford, R., and Brashares, J. S. (2012). The effects of small sample size and sample bias on threshold selection and accuracy assessment of species distribution models. Ecography 35, 250–258. doi: 10.1111/j.1600-0587.2011.06545.x
Booth, T. H., Nix, H. A., Busby, J. R., and Hutchinson, M. F. (2014). Bioclim: The first species distribution modelling package, its early applications and relevance to most current MaxEnt studies. Divers. Distrib. 20, 1–9. doi: 10.1111/ddi.12144
Bradley, B. A., Wilcove, D. S., and Oppenheimer, M. (2010). Climate change increases risk of plant invasion in the Eastern United States. Biol. Invas. 12, 1855–1872. doi: 10.1007/s10530-009-9597-y
Brancalion, P. H. S., Novembre, A. D. L. C., Rodrigues, R. R., and Tay, D. (2008). Priming of Mimosa bimucronata seeds: A tropical tree species from Brazil. Acta Horticul. 782, 163–168.
Bykova, O., Chuine, I., Morin, X., and Higgins, S. I. (2012). Temperature dependence of the reproduction niche and its relevance for plant species distributions. J. Biogeogr. 39, 2191–2200. doi: 10.1111/j.1365-2699.2012.02764.x
Carpenter, G., Gillison, A. N., and Winter, J. (1993). DOMAIN: A flexible modelling procedure for mapping potential distributions of plants and animals. Biodivers. Conserv. 2, 667–680. doi: 10.1007/BF00051966
Cerdeira, J. O., Monteiro-Henriques, T., Martins, M. J., Silva, P. C., Alagador, D., and Franco, A. M. A. (2014). Mathematical contributions to link biota with environment. J. Veg. Sci. 25, 1148–1153. doi: 10.1111/jvs.12188
Cerdeira, J. O., Monteiro-Henriques, T., Martins, M. J., Silva, P. C., Alagador, D., Franco, A. M. A., et al. (2018). Revisiting niche fundamentals with Tukey depth. Methods Ecol. Evol. 9, 2349–2361. doi: 10.1111/2041-210X.13074
Chen, Y., Lu, J., Wu, P., and Mao, L. (2022). Response of invasive area of Ageratina adenophora to future climate change based on climate and species diffusion. J. Beijing For. Univ. 44, 69–76. doi: 10.12171/j.1000-1522.20210063
Chen, Y., Yang, X., Yang, Q., Li, D., Long, W., and Luo, W. (2014). Factors affecting the distribution pattern of wild plants with extremely small populations in Hainan Island, China. PLoS One 9:e97751. doi: 10.1371/journal.pone.0097751
Cheng, J., Ou, J., Chen, X., Tao, W., Wang, H., and Miao, S. (2011). Effect of Mimosa bimucronata leaves water extracts on seeds germination of three cultivated species. J. Anhui Agric. Sci. 39, 18631–18633. doi: 10.13989/j.cnki.0517-6611.2011.30.185
Diagne, C., Catford, J., Essl, F., Nuñez, M. A., and Courchamp, F. (2020). What are the economic costs of biological invasions? A complex topic requiring international and interdisciplinary expertise. NeoBiota 63, 25–37. doi: 10.3897/neobiota.63.55260
Diagne, C., Leroy, B., Vaissière, A.-C., Gozlan, R. E., Roiz, D., Jarić, I., et al. (2021). High and rising economic costs of biological invasions worldwide. Nature 592, 571–576. doi: 10.1038/s41586-021-03405-6
Elith, J., and Graham, C. H. (2009). Do they? How do they? Why do they differ? On finding reasons for differing performances of species distribution models. Ecography 32, 66–77. doi: 10.1111/j.1600-0587.2008.05505.x
Fang, Y., Zhang, X., Wei, H., Wang, D., Chen, R., Wang, L., et al. (2021). Predicting the invasive trend of exotic plants in China based on the ensemble model under climate change: A case for three invasive plants of Asteraceae. Sci. Total Environ. 756:143841. doi: 10.1016/j.scitotenv.2020.143841
Feng, Y., Du, D., and van Kleunen, M. (2022). Global change and biological invasions. J. Plant Ecol. 15, 425–428. doi: 10.1093/jpe/rtac013
Ferreira, V. M. (2018). Biometric characterization and seed germination of giant mimosa [Mimosa bimucronata (DC) O. Kuntze]. Aust. J. Crop Sci. 12, 108–115.
Fick, S. E., and Hijmans, R. J. (2017). WorldClim 2: New 1-km spatial resolution climate surfaces for global land areas. Int. J. Climatol. 37, 4302–4315. doi: 10.1002/joc.5086
Franklin, J. (2010). Moving beyond static species distribution models in support of conservation biogeography. Divers. Distrib. 16, 321–330. doi: 10.1111/j.1472-4642.2010.00641.x
Fukano, Y., and Soga, M. (2019). Spatio-temporal dynamics and drivers of public interest in invasive alien species. Biol. Invas. 21, 3521–3532. doi: 10.1007/s10530-019-02065-y
Gallien, L., Münkemüller, T., Albert, C. H., Boulangeat, I., and Thuiller, W. (2010). Predicting potential distributions of invasive species: Where to go from here? Divers. Distrib. 16, 331–342. doi: 10.1111/j.1472-4642.2010.00652.x
Gebrewahid, Y., Abrehe, S., Meresa, E., Eyasu, G., Abay, K., Gebreab, G., et al. (2020). Current and future predicting potential areas of Oxytenanthera abyssinica (A. Richard) using MaxEnt model under climate change in Northern Ethiopia. Ecol. Proces. 9:6. doi: 10.1186/s13717-019-0210-8
Giliba, R. A., and Yengoh, G. T. (2020). Predicting suitable habitats of the African cherry (Prunus Africana) under climate change in Tanzania. Atmosphere 11:988. doi: 10.3390/atmos11090988
Govindasamy, B., Duffy, P. B., and Coquard, J. (2003). High-resolution simulations of global climate, part 2: Effects of increased greenhouse cases. Clim. Dyn. 21, 391–404. doi: 10.1007/s00382-003-0340-6
Hammer, Ø, Harper, D. A., and Ryan, P. D. (2001). PAST: Paleontological statistics software package for education and data analysis. Palaeontol. Electron. 4:9.
Hernandez, P. A., Graham, C. H., Master, L. L., and Albert, D. L. (2006). The effect of sample size and species characteristics on performance of different species distribution modeling methods. Ecography 29, 773–785. doi: 10.1111/j.0906-7590.2006.04700.x
Hessburg, P. F., Miller, C. L., Parks, S. A., Povak, N. A., Taylor, A. H., Higuera, P. E., et al. (2019). Climate, environment, and disturbance history govern resilience of western North American forests. Front. Ecol. Evol. 7:239. doi: 10.3389/fevo.2019.00239
Hijmans, R. J., Guarino, L., Cruz, M., and Rojas, E. (2001). Computer tools for spatial analysis of plant genetic resources data: 1. DIVA-GIS. Plant Genet. Resour. Newsl. 127, 15–19.
Kadmon, R., Farber, O., and Danin, A. (2003). A systematic analysis of factors affecting the performance of climatic envelope models. Ecol. Appl. 13, 853–867. doi: 10.3390/ijerph191811347
King, T. W., Vynne, C., Miller, D., Fisher, S., Fitkin, S., Rohrer, J., et al. (2021). The influence of spatial and temporal scale on the relative importance of biotic vs. abiotic factors for species distributions. Divers. Distrib. 27, 327–343. doi: 10.1111/ddi.13182
Körner, C. (2012). Alpine treelines: functional ecology of the global high elevation tree limits. Berlin: Springer Science & Business Media.
Kriticos, D. J., Sutherst, R. W., Brown, J. R., Adkins, S. W., and Maywald, G. F. (2003). Climate change and the potential distribution of an invasive alien plant: Acacia nilotica ssp. indica in Australia. J. Appl. Ecol. 40, 111–124. doi: 10.1046/j.1365-2664.2003.00777.x
Kumar Rai, P., and Singh, J. S. (2020). Invasive alien plant species: Their impact on environment, ecosystem services and human health. Ecol. Indic. 111:106020. doi: 10.1016/j.ecolind.2019.106020
Lamsal, P., Kumar, L., Aryal, A., and Atreya, K. (2018). Invasive alien plant species dynamics in the Himalayan region under climate change. Ambio 47, 697–710. doi: 10.1007/s13280-018-1017-z
Lan, L., Ma, T., Zhu, Y., Mao, Y., Dong, R., and Wen, X. (2019). Research progress on the invasive alien plant Mimosa bimucronata. J. Hebei For. Sci. Technol. 1, 47–52. doi: 10.16449/j.cnki.issn1002-3356.2019.01.015
Li, Y., Yang, X., Wang, W., and Liu, Z. (2010). Changes of China agricultural climate resources under the background of climate change.I. Spatiotemporal change characteristics of agricultural climate resources in South China. Chin. J. Appl. Ecol. 21, 2605–2614. doi: 10.13287/j.1001-9332.2010.0362
Liebhold, A. M., Campbell, F. T., Gordon, D. R., Guo, Q., Havill, N., Kinder, B., et al. (2021). “The role of international cooperation in invasive species research,” in Invasive species in forests and rangelands of the United States: A comprehensive science synthesis for the United States Forest Sector, eds T. M. Poland, T. Patel-Weynand, D. M. Finch, C. F. Miniat, D. C. Hayes, and V. M. Lopez (Cham: Springer), 293.
Liu, J., and Liu, Q. (2011). Control and utilization of exotic plant Mimosa bimucronata. China Trop. Agric. 5, 81–84. doi: 10.3969/j.issn.1673-0658.2011.05.030
Mehta, S. V., Haight, R. G., Homans, F. R., Polasky, S., and Venette, R. C. (2007). Optimal detection and control strategies for invasive species management. Ecol. Econ. 61, 237–245. doi: 10.1016/j.ecolecon.2006.10.024
Ministry of Agriculture and Rural Affairs of the People’s Republic of China (2022). List of key management invasive alien species. Beijing: Ministry of Agriculture and Rural Affairs of the People’s Republic of China.
Mora, C., Spirandelli, D., Franklin, E. C., Lynham, J., Kantar, M. B., Miles, W., et al. (2018). Broad threat to humanity from cumulative climate hazards intensified by greenhouse gas emissions. Nat. Clim. Chang. 8, 1062–1071. doi: 10.1038/s41558-018-0315-6
Morriën, E., Engelkes, T., Macel, M., Meisner, A., and Van der Putten, W. H. (2010). Climate change and invasion by intracontinental range-expanding exotic plants: The role of biotic interactions. Ann. Bot. 105, 843–848. doi: 10.1093/aob/mcq064
Natale, E., Zalba, S. M., and Reinoso, H. (2013). Presence - absence versus invasive status data for modelling potential distribution of invasive plants: Saltcedar in Argentina. Écoscience 20, 161–171. doi: 10.2980/20-2-3571
Navarro-Cerrillo, R. M., Hernández-Bermejo, J. E., and Hernández-Clemente, R. (2011). Evaluating models to assess the distribution of Buxus balearica in southern Spain. Appl. Veg. Sci. 14, 256–267. doi: 10.1111/j.1654-109X.2010.01112.x
Nix, H. A. (1986). A biogeographic analysis of Australian elapid snakes. Atlas Elapid Snakes Aust. 7, 4–15.
Olkoski, D., and Wittmann, M. T. S. (2011). Cytogenetics of Mimosa bimucronata (DC.) O. Kuntze (Mimosoideae, Leguminosae): Chromosome number, polysomaty and meiosis. Crop Breed. Appl. Biotechnol. 11, 27–36.
Ou, J., Lu, C., and O’Toole, D. K. (2008). A risk assessment system for alien plant bio-invasion in Xiamen, China. J. Environ. Sci. 20, 989–997. doi: 10.1016/S1001-0742(08)62198-1
Padalia, H., Srivastava, V., and Kushwaha, S. P. S. (2014). Modeling potential invasion range of alien invasive species, Hyptis suaveolens (L.) Poit. in India: Comparison of MaxEnt and GARP. Ecol. Inform. 22, 36–43.
Padalia, H., Srivastava, V., and Kushwaha, S. P. S. (2015). How climate change might influence the potential distribution of weed, bushmint (Hyptis suaveolens)? Environ. Monit. Assess. 187:210. doi: 10.1007/s10661-015-4415-8
Panda, R. M., and Behera, M. D. (2019). Assessing harmony in distribution patterns of plant invasions: A case study of two invasive alien species in India. Biodivers. Conserv. 28, 2245–2258. doi: 10.1007/s10531-018-1640-9
Pepin, N., Bradley, R. S., Diaz, H. F., Baraer, M., Caceres, E. B., Forsythe, N., et al. (2015). Elevation-dependent warming in mountain regions of the world. Nat. Clim. Chang. 5, 424–430. doi: 10.1038/nclimate2563
Pyšek, P., Brundu, G., Brock, J., Child, L., and Wade, M. (2019). Twenty-five years of conferences on the ecology and management of alien plant invasions: The history of EMAPi 1992–2017. Biol. Invas. 21, 725–742. doi: 10.1007/s10530-018-1873-2
Richardson, D. M., and Rejmánek, M. (2011). Trees and shrubs as invasive alien species – a global review. Divers. Distrib. 17, 788–809.
Rossi, M. N., da Silva Rodrigues, L. M., Ishino, M. N., and Kestring, D. (2011). Oviposition pattern and within-season spatial and temporal variation of pre-dispersal seed predation in a population of Mimosa bimucronata trees. Arthropod Plant Interact. 5, 209–217. doi: 10.1007/s11829-011-9130-y
Royle, J. A., Chandler, R. B., Yackulic, C., and Nichols, J. D. (2012). Likelihood analysis of species occurrence probability from presence-only data for modelling species distributions. Methods Ecol. Evol. 3, 545–554.
Sankar, A. U., Anitha, K., Sivaraj, N., Kumari, K., Sunil, N., and Chakrabarty, S. (2015). Screening of horsegram germplasm collected from Andhra Pradesh against anthracnose. Legume Res. Int. J. 38, 753–757. doi: 10.18805/lr.v38i6.6719
Santika, T. (2011). Assessing the effect of prevalence on the predictive performance of species distribution models using simulated data. Glob. Ecol. Biogeogr. 20, 181–192. doi: 10.1111/j.1466-8238.2010.00581.x
Schlickmann, F., de Souza, P., Boeing, T., Mariano, L. N. B., Steimbach, V. M. B., Krueger, C. D. M. A., et al. (2017). Chemical composition and diuretic, natriuretic and kaliuretic effects of extracts of Mimosa bimucronata (DC.) Kuntze leaves and its majority constituent methyl gallate in rats. J. Pharm. Pharmacol. 69, 1615–1624. doi: 10.1111/jphp.12785
Schwartz, M. W. (1993). Modelling effects of habitat fragmentation on the ability of trees to respond to climatic warming. Biodivers. Conserv. 2, 51–61.
Seijo, J. G., and SolÍS Neffa, V. G. (2004). The cytological origin of the polyads and their significance in the reproductive biology of Mimosa bimucronata. Bot. J. Linn. Soc. 144, 343–349. doi: 10.1111/j.1095-8339.2003.00253.x
Shao, H., Tian, J., Guo, K., and Sun, J. (2009). Effects of sample size and species traits on performance of BIOCLIM in predicting geographical distribution of tree species - a case study with 12 deciduous Quercus species indigenous to China. Chin. J. Plant Ecol. 33, 870–877. doi: 10.3773/j.issn.1005-264x.2009.05.005
Shao, Z., Zhao, H., Chen, B., Yang, Y., Qiu, S., and Peng, S. (2008). Intrusion alarm of exotic plant Mimosa bimucronata (DC.) Kuntze. J. Anhui Agric. Sci. 36, 5773–5774. doi: 10.3969/j.issn.0517-6611.2008.14.103
Storkey, J., Stratonovitch, P., Chapman, D. S., Vidotto, F., and Semenov, M. A. (2014). A process-based approach to predicting the effect of climate change on the distribution of an invasive allergenic plant in Europe. PLoS One 9:e88156. doi: 10.1371/journal.pone.0088156
Tesfamariam, B. G., Gessesse, B., and Melgani, F. (2022). MaxEnt-based modeling of suitable habitat for rehabilitation of Podocarpus forest at landscape-scale. Environ. Syst. Res. 11:4. doi: 10.1186/s40068-022-00248-6
Tu, W., Xiong, Q., Qiu, X., and Zhang, Y. (2021). Dynamics of invasive alien plant species in China under climate change scenarios. Ecol. Ind. 129:107919. doi: 10.1016/j.ecolind.2021.107919
van Boheemen, L. A., Atwater, D. Z., and Hodgins, K. A. (2019). Rapid and repeated local adaptation to climate in an invasive plant. New Phytol. 222, 614–627. doi: 10.1111/nph.15564
Vinogradova, Y., Pergl, J., Essl, F., Hejda, M., van Kleunen, M., Pyšek, P., et al. (2018). Invasive alien plants of Russia: Insights from regional inventories. Biol. Invas. 20, 1931–1943. doi: 10.1007/s10530-018-1686-3
Walter, M., Brugger, K., and Rubel, F. (2016). The ecological niche of Dermacentor marginatus in Germany. Parasitol. Res. 115, 2165–2174.
Wei, B., Wang, R., Hou, K., Wang, X., and Wu, W. (2018). Predicting the current and future cultivation regions of Carthamus tinctorius L. using MaxEnt model under climate change in China. Glob. Ecol. Conserv. 16:e00477.
Welgama, A., Florentine, S., and Roberts, J. (2022). A global review of the woody invasive alien species Mimosa pigra (Giant Sensitive Plant): Its biology and management implications. Plants 11:2366. doi: 10.3390/plants11182366
Wisz, M. S., Hijmans, R. J., Li, J., Peterson, A. T., Graham, C. H., Guisan, A., et al. (2008). Effects of sample size on the performance of species distribution models. Divers. Distrib. 14, 763–773. doi: 10.1111/j.1472-4642.2008.00482.x
Xie, C., Tian, E., Jim, C. Y., Liu, D., and Hu, Z. (2022). Effects of climate-change scenarios on the distribution patterns of Castanea henryi. Ecol. Evol. 12:e9597.
Xu, J. (2014). The distribution, prevention and control of the invasive plant, Mimosa bimucronata in China. J. Weed Sci. 32, 41–43.
Xu, Y., Wang, Y., and Huang, Y. (1986). Preliminary study on firewood plantations of Mimosa bimucronata. Trop. For. 3, 9–17.
Xu, Z., Peng, H., and Peng, S. (2015). The development and evaluation of species distribution models. Acta Ecol. Sin. 2, 557–567.
Zhang, S., Liu, W., Cheng, X., Wang, Z., Yuan, F., Wu, W., et al. (2022). Evaluating the productivity of ancient Pu’er tea trees (Camellia sinensis var. assamica): A multivariate modeling approach. Plant Methods 18:95.
Zhu, H., Yi, X., Li, Y., Duan, Y., Wang, X., and Zhang, L. (2021). Limiting climatic factors in shaping the distribution pattern and niche differentiation of Prunus dielsiana in subtropical China. J. For. Res. 32, 1467–1477. doi: 10.1007/s11676-020-01194-8
Keywords: Mimosa bimucronata, invasive species, species distribution model (SDM), BIOCLIM model, DOMAIN model, climate change scenario, bioclimatic factors
Citation: Xie C, Li M, Jim CY and Liu D (2023) Spatio-temporal patterns of an invasive species Mimosa bimucronata (DC.) Kuntze under different climate scenarios in China. Front. For. Glob. Change 6:1144829. doi: 10.3389/ffgc.2023.1144829
Received: 15 January 2023; Accepted: 02 March 2023;
Published: 15 March 2023.
Edited by:
Di Yang, University of Wyoming, United StatesReviewed by:
Gopal Shukla, Uttar Banga Krishi Viswavidyalaya, IndiaCopyright © 2023 Xie, Li, Jim and Liu. This is an open-access article distributed under the terms of the Creative Commons Attribution License (CC BY). The use, distribution or reproduction in other forums is permitted, provided the original author(s) and the copyright owner(s) are credited and that the original publication in this journal is cited, in accordance with accepted academic practice. No use, distribution or reproduction is permitted which does not comply with these terms.
*Correspondence: C. Y. Jim, Y3lqaW1AZWR1aGsuaGs=
Disclaimer: All claims expressed in this article are solely those of the authors and do not necessarily represent those of their affiliated organizations, or those of the publisher, the editors and the reviewers. Any product that may be evaluated in this article or claim that may be made by its manufacturer is not guaranteed or endorsed by the publisher.
Research integrity at Frontiers
Learn more about the work of our research integrity team to safeguard the quality of each article we publish.