- 1Department of Natural Resources and the Environment, University of New Hampshire, Durham, NH, United States
- 2Department of Mathematics and Statistics, University of New Hampshire, Durham, NH, United States
- 3The Nature Conservancy, Arlington, VA, United States
- 4Department of Computer Science, University of New Hampshire, Durham, NH, United States
- 5Gund Institute for Ecological Economics, University of Vermont, Burlington, VT, United States
- 6Spatial Informatics Group, LLC, Pleasanton, CA, United States
Forest disturbances, such as an eastern spruce budworm (Choristoneura fumiferana) outbreak, impact the strength and persistence of forest carbon sinks. Salvage harvests are a typical management response to widespread tree mortality, but the decision to salvage mortality has large implications for the fate of carbon stocks (including forest carbon and harvested wood products) in the near and long terms. In this study, we created decision-support models for salvage harvesting based on carbon after an eastern spruce budworm outbreak. We used lasso regression to determine which stand characteristics (e.g., basal area) are the best predictors of carbon 40 years after an outbreak in both salvage and no salvage scenarios. We modeled carbon at year 40 for different treatment scenarios and discount rates. Treatment scenarios represent residual stand conditions that may be present when an outbreak occurs. Economic discount rates were applied to 40-year carbon values to account for near and long-term carbon storage aspects. We found that the volume and size of eastern spruce budworm host species are significant predictors of salvage preference based on carbon. We found overall that salvaging less volume is recommended to avoid major swings in carbon budgets and that discounting carbon values to apply weight to near or long-term sequestration greatly affects whether salvaging is preferred. Lasso models are constructed for the northeastern US, however, similar concepts may be applied beyond our study area and potentially for other insect outbreaks similar to spruce budworm, such as mountain pine beetle (Dendroctonus ponderosae) or hemlock woolly adelgid (Adelges tsugae). From a policy standpoint widespread salvaging could create a large carbon emissions deficit with the risk of not being fully replenished within a desired timeframe. Since salvaging is often financially driven, especially for private landowners, carbon market payments or incentives for not salvaging is a consideration for future policy.
1. Introduction
An approaching eastern spruce budworm (Choristoneura fumiferana; SBW) outbreak threatens 4.7 million hectares of forest and 94.8 million Mg in carbon stocks of spruce (Picea spp.) and balsam fir (Abies balsamea) in the northeastern US (Dymond et al., 2010; Hennigar et al., 2011; Gunn et al., 2020). Balsam fir is typically more at risk of defoliation compared to spruce species, however, the presence of fir or spruce host species has potential implications for forest carbon budgets (Hennigar et al., 2008; Liu et al., 2019). The previous SBW outbreak that occurred in the 1970–1980s led to increases in salvage harvesting to recover portions of economic loss and resulted in widespread clearcutting in Maine (Irland et al., 1988). By 1989, 45% of Maine’s timber harvests were clearcuts (Wagner et al., 2016). New management restrictions passed in the 1989 Maine Forest Practices Act combined with stricter pesticide regulations and forest composition shifts toward more hardwood species in Maine will likely prevent a response of equal intensity to the coming outbreak (Legaard et al., 2015). However, given Maine and New Hampshire are the two most forested states per capita in the US (USDA Forest Service, 2017) and northern portions of Vermont and New York contain large amounts of SBW host tree species, salvage harvest decision-making has the potential to significantly impact forest carbon budgets in the northeastern US, as well as in other regions, such as eastern Canada that also experience SBW outbreaks.
Previous findings of Gunn et al. (2020) suggest salvaging leads to net carbon emissions in the near-term, but net carbon sequestration over longer time periods. The timing of emissions could be important from a policy context because of an urgent need to reduce greenhouse gas (GHG) emissions (within the next 20 years) to achieve Paris Agreement goals of 1.5°–2°C in global temperature rise and to reduce committed warming (Gasser et al., 2015; Mengel et al., 2018; Zhou et al., 2021). Policies and incentives designed to support the role of forests as a natural climate solution (NCS), such as improved or restorative resource management practices (Griscom et al., 2019), may need to consider the uncertainty of timing and magnitude of the potential carbon benefits of forest management decisions.
The integration of NCS into climate policy is necessary for future climate mitigation (Fargione et al., 2018). NCS are cost-efficient and effective approaches to reducing global GHG emissions with an estimated 37% of global CO2 emissions potentially mitigated by NCS through 2030, a large portion of which is from forest carbon sequestration (Griscom et al., 2017). In a recent report, forestry practices like afforestation, longer rotation periods, setting land aside as no harvest areas, and clear-cutting as a means to increase sequestration rates were assessed as potential NCS strategies in Maine to increase forest carbon sequestration, but salvage logging was not considered (Daigneault et al., 2021). Salvage harvest decisions are likely to become important as SBW populations rise in the northeastern US and parts of Canada over the next several years and more generally, as forest disturbances change in frequency and intensity due to climate change (Anderegg et al., 2020).
Given the economic benefits of salvaging compared to leaving dead trees, when salvaging is the most climate beneficial alternative, further policy support may not be needed. However, if not-to-salvage is climate beneficial, financial, or other incentives might be necessary to change landowner behavior. Since timber management on privately owned land is often financially driven, policies to support no salvage incentives may be more necessary for private compared to publicly owned land. Incentives potentially could be funded through voluntary participation in carbon markets, or through cost-share programs similar to those currently provided by the US Department of Agriculture’s Natural Resource Conservation Service. Voluntary participation in forest-based carbon offset projects has steadily increased since 2018 with record total value and volumes of carbon traded in 2021 (Donofrio et al., 2021). The integration of salvaging as an NCS forestry practice could provide a first step for offering payment to landowners for minimizing carbon loss on private forest land following major disturbances. US Department of Agriculture Natural Resources Conservation Service (NRCS) cost-share is another potential source of carbon project funding for private landowners. Currently, NRCS ranks forestry and agricultural practices based on potential GHG emissions reduction but does not provide financial incentives for carbon-based management.
In this study we assess how forest characteristics could influence post-disturbance forest carbon from salvage logging where salvage decision-making plays an important role in future carbon budgets. We used lasso regression, a machine learning method, to predict a salvage response that maximizes carbon storage 40 years after SBW based on predicted carbon values from Gunn et al. (2020) and a set of stand characteristics with expected relationships to salvage or no salvage carbon outcomes (e.g., basal area of balsam fir). Additionally, given the urgency of climate change and the imperative to reduce emissions in the near-term, the decision to salvage can be further informed by the treatment of emissions over time using discount rates. Below, we apply a range of discount rates (0–5%) to carbon sequestration estimates from life-cycle assessment models to account for fluctuations in carbon storage from salvaging or not salvaging over a 40-year period.
2. Materials and methods
2.1. Simulated carbon outcomes
We used values provided by Gunn et al. (2020) that simulated Mg CO2e/ha of carbon 40 years following a spruce budworm outbreak. Values were simulated at 4,926 USDA Forest Inventory and Analysis (FIA) plots in a portion of the northeastern US, encompassing New York and the six New England states (Figure 1). Simulations encompassed only those plots containing at least some stocking of species preferred by SBW. Gunn et al. (2020) combined Forest Vegetation Simulator-Northeast Variant (FVS-NE) outputs and a full Life-Cycle Assessment (LCA) to track the carbon fate of forests and harvested wood in salvage and no salvage harvesting scenarios for a 40-year period after spruce budworm at each FIA plot. In-forest and post-harvest carbon values were modeled at each FIA plot for six different management regimes that range in harvest intensity from no management to a commercial industrial clearcut. FVS-NE models simulated spruce budworm mortality for 95% of balsam fir and 45% of spruce species in each stand (Solomon et al., 2003) and a 90% removal efficiency in salvaging operations for trees > 10°cm Diameter at Breast Height (DBH).
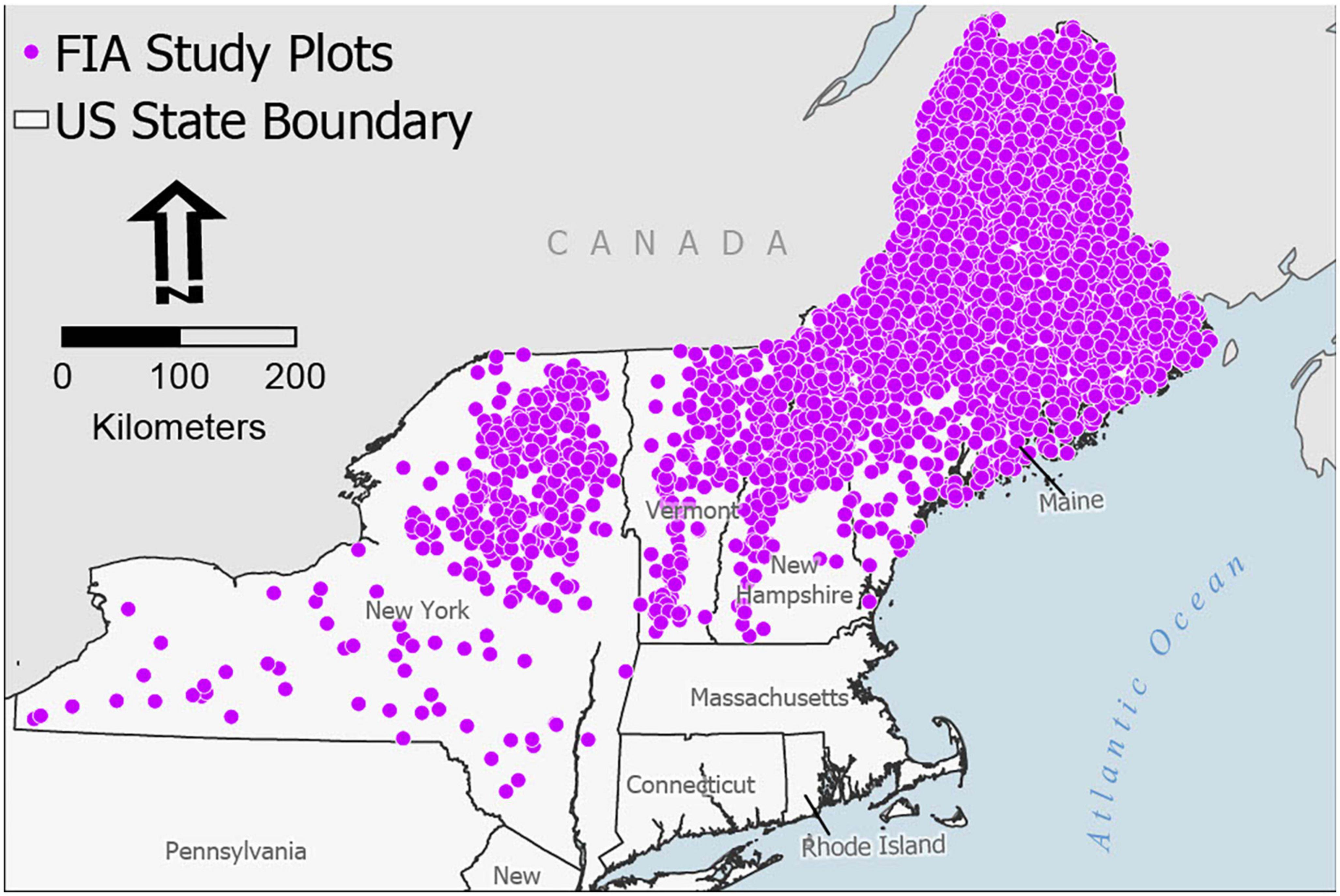
Figure 1. Study area map of Forest Inventory and Analysis (FIA) plots used across the northeastern US (n = 4,926).
At each plot from Gunn et al. (2020), there were two salvage scenarios: (1) a no salvage scenario and (2) a salvage scenario where 90% of tree mortality > 10°cm DBH was removed. For each of these two salvage scenarios, six treatment scenarios (residual stand conditions) were also modeled by FVS (criteria for each treatment scenario is described in detail below). The resulting dataset from Gunn et al. (2020) contained 12 carbon scenarios for each FIA study plot—one for each of the six treatment scenarios with a salvage and no salvage response.
The six treatment scenarios discussed in Gunn et al. (2020) represent a range of residual stand conditions that could be present when an SBW outbreak occurs (Figure 2). Treatment class is determined by the volume of removal and target species and size class. The six pre-outbreak forest treatment scenarios used by Gunn et al. (2020) are listed in decreasing order of intensity: commercial-industrial clearcuts (>80% removal of trees > 20°cm DBH with valuable species preference), heavy (>80% removal of trees > 20.3°cm DBH with no species preference), high grade (30–50% removal for trees > 20°cm DBH with valuable species preference), moderate (45–55% removal with no size class or species preference), light (25–35% removal with no size class or species preference), and no management (no trees harvested). Valuable species targeted in commercial-industrial clearcut and high-grade scenarios include black cherry (Prunus serotina), black walnut (Juglans nigra), paper birch (Betula papyrifera), red oak (Quercus rubra), red spruce (Picea rubens), sugar maple (Acer saccharum), white pine (Pinus strobus), and yellow birch (Betula alleghaniensis).
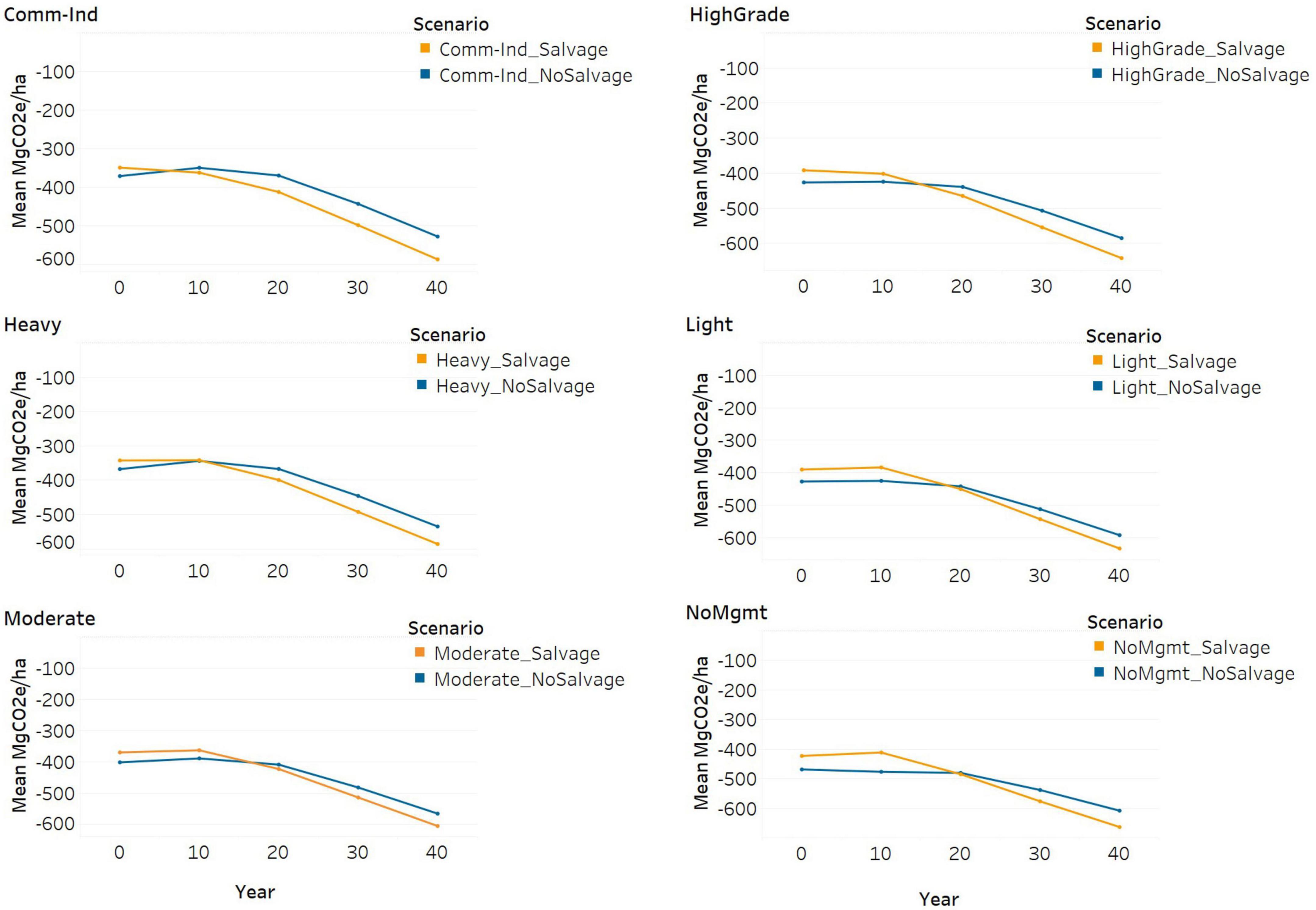
Figure 2. Six treatment scenarios are shown along with expected carbon storage (Mg CO2e/ha) in salvage and no salvage scenarios 40 years following spruce budworm (SBW).
Related to salvage logging post-outbreak, plots previously treated as commercial-industrial clearcuts have the least available volume to salvage, and plots not recently managed have the greatest available volume to salvage. The four remaining treatment scenarios (heavy, moderate, high grade, and light) represent stands with intermediate volumes of timber available for salvage harvesting.
2.2. Discount scenarios
We calculated the present value of future carbon emissions following an SBW outbreak using a range of discount rates. Discounting future carbon emissions captures potential preferences for near- or long-term carbon savings. For example, not salvaging typically stores more carbon within the first 10–20 years following SBW mortality, however, salvaging leads to greater carbon storage over longer time periods due to new regeneration, decay of dead wood, and long-term storage in harvested wood products (Gunn et al., 2020). We applied discount rates to carbon values from Gunn et al. (2020) which used a 40-year modeling period, representative of typical SBW cycles. While salvaging is typically preferred based on carbon 40 years into the future, discounting penalizes the wait period and additional uncertainty from longer periods of regeneration time to replenish forest carbon stocks, giving preference to near-term carbon storage opportunities from not salvaging.
Discount rates applied to carbon emissions can range from 0 to 5%, with most applications using the lower end of this range (Bachmann, 2020). Some studies argue that a decreasing discount rate, calculated using hyperbolic methods, are appropriate for long-term carbon studies (Richards and Green, 2015; Iverson and Karp, 2021). However, given that our study is within a relatively short time period (40 years) and there is little existing guidance for discounting applied to carbon (Lueddeckens et al., 2021), especially within a forestry context, we chose to use a consistent discount rate across the 40-year period. We calculated four scenarios including discount rates of 0, 1, 3, and 5% to provide the extent to which discounting might affect decision-support results. A discount rate of 0% implies the value of one unit of carbon stored in the future is equal to one unit of carbon stored today. As the discount rate increases to 5%, one unit of carbon stored in the future is worth less than one unit of carbon stored today. Within the context of forestry, uncertainties of regeneration, disturbance, and future forest condition that allow stands to recover forest carbon losses from harvesting could make near-term carbon savings from not salvaging more appealing in addition to near-term carbon storage preferences based on emissions-reductions goals.
We discounted carbon values provided by Gunn et al. (2020) over a 40-year time period. Values from Gunn et al. (2020) were generated in 10-year intervals. We calculated the average net emissions at each plot per year and applied a discount rate to annual net emissions before summing values over a 40-year period (Equation 1).
Where e is carbon emissions in Mg CO2e/ha, t is the number of years from the initial SBW event, and r is the discount rate.
2.3. Forest stand and landscape attributes
We used Forest Inventory and Analysis (FIA) data to derive 79 attributes at each FIA plot with expected relationships to carbon outcomes of salvage and no salvage harvesting scenarios (Figure 3). We calculated basal area and stocking for spruce budworm host species and non-host species. Stocking was calculated by summing tree-level stocking values by species provided in FIA “Tree” tables (Arner et al., 2001). We also calculated balsam fir quadratic mean diameter (QMD) since it represents large-diameter trees within a stand and because of SBW preference for more mature balsam fir trees and salvage diameter limits (10°cm DBH) used in FVS models created by Gunn et al. (2020). Non-host species basal area was included as the total non-host basal area (basal area summed for all non-host species) and individual non-host species basal area per plot. There were 67 total non-host species. Individual non-host species measurements were included in addition to total non-host basal area to explore whether certain species may have a stronger influence on whether salvaging is preferred.
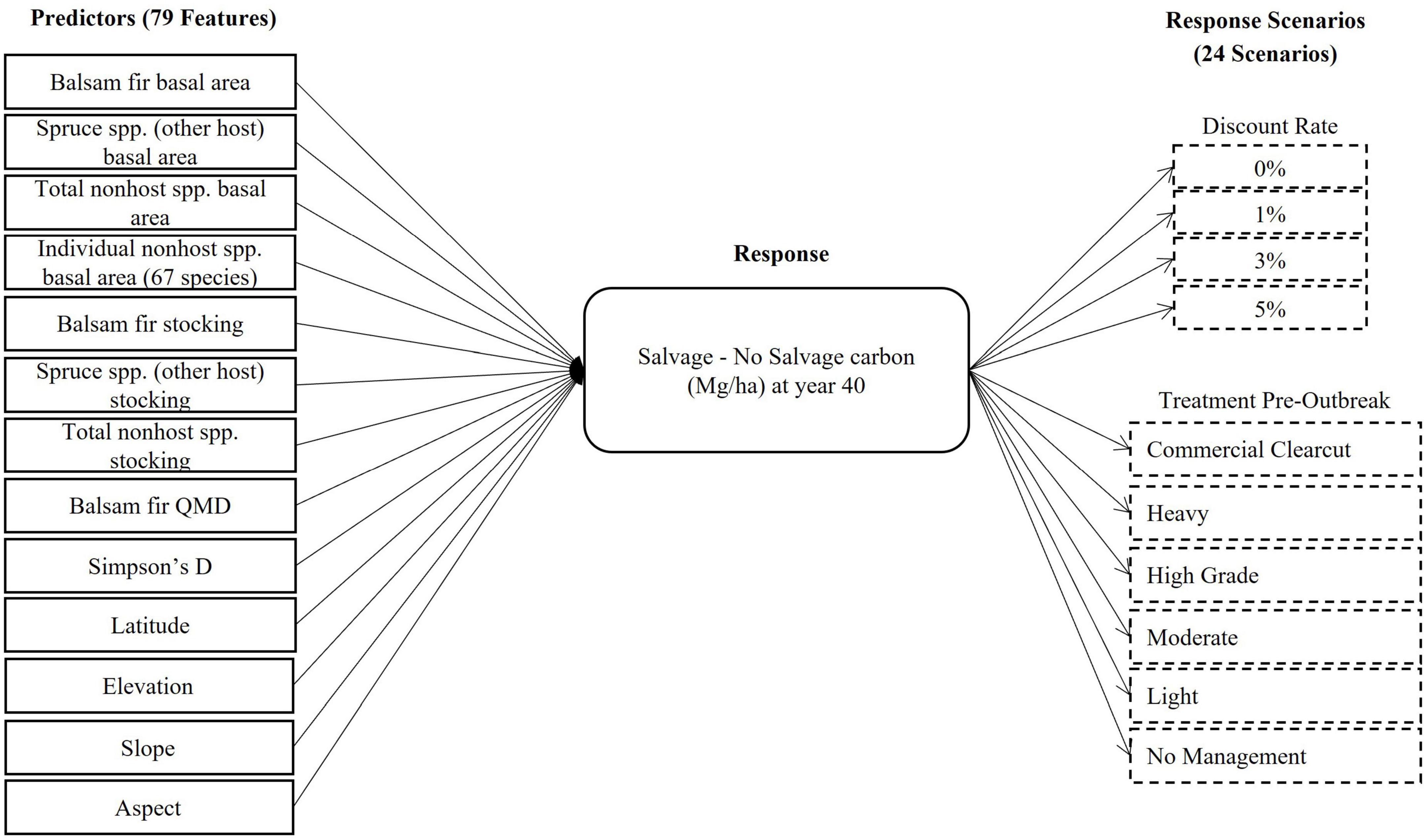
Figure 3. Lasso models use 79 predictive variables (67 of which are individual non-host basal area values) to predict the difference between salvage and no salvage carbon (Mg/ha) for 24 total scenarios (four discount and six treatment scenarios). A negative difference between salvage and no salvage carbon indicates a preference for salvaging (more carbon is stored from salvaging) and a positive value indicates a no salvage preference (more carbon stored from not salvaging).
Geophysical plot characteristics were also included as potential indicators of regeneration response (i.e., whether net carbon emissions will be reduced after salvaging at a given site) and whether site conditions are favorable for spruce budworm host species. Elevation and latitude values were retrieved from FIA “Plot” tables where we expected spruce-fir forest types to occur at higher elevations and higher latitudes and thus, a potentially greater impact of SBW. We derived mean slope and aspect values from FIA “Condition” tables for potential relationships of spruce-fir forests with steeper slopes and a given sunlight aspect. We also included estimates of species abundance and diversity using Simpson’s Diversity Index (Simpson’s D). We chose Simpson’s D, which estimates diversity using relative abundance, or the number of stems of individual species, because this information is potentially relevant for predicting carbon. In some cases, a diverse stand with a high number of fast-growing stems sequesters carbon at a different rate than stands with fewer, larger trees, which could affect the decision to salvage. Simpson’s D provides values ranging from 0 to 1 where values closer to 1 indicate greater diversity (Equation 2).
Where n is the number of individuals in each species and N is the total number of trees in a stand.
2.4. Lasso models
We used lasso regression analysis to generate a predictive model that automatically identifies only the most important stand features for predicting the carbon impact of salvaging (Ranstam and Cook, 2018). We fit individual lasso models for each combination of treatment and discount rate scenario for a total of 24 regression models with the 79 stand and landscape attributes used as predictive features (Figure 3). Lasso is a method of linear regression that identifies linear relationships between response variables (the difference between salvage and no salvage carbon in this case) and predictive variables (our 79 stand and landscape characteristics) (Tibshirani, 1996). The main difference between lasso and simple linear regression is that lasso is an L1-penalized regression method. Meaning that lasso uses a penalty term in its equation to assign coefficient values of zero to non-significant predictive features, effectively removing them from the linear model. We chose to use lasso to only identify the most important predictive features for salvaging response out of our 79 input features. FIA plots were then randomly divided into training and test sets with 70 and 30% of plots, respectively. The training dataset was used to compute lasso models, and we compared root mean square error (RMSE) between predicted and FVS-modeled carbon sequestration using the test sets. We set the regularization penalty lambda to five which we found to offer a balance between prediction quality and model complexity. A lambda value of five provided less than ten significant predictive features for each model, making them more interpretable. Lasso models were fit using the glmnet package in R (Friedman et al., 2010).
To evaluate the accuracy of our lasso models, we compared the predicted carbon sequestration with the FVS output on the test sets for all treatment conditions and discount scenarios. To assess the broader utility of the predictive models, we also compared the carbon savings of lasso-guided salvage strategies with strict strategies which make the same salvage decision for all plots in a specific treatment condition. Lasso strategies prescribe to salvage a specific plot only if our lasso model predicts that salvage generates carbon savings. Another strength of our approach based on lasso is that lasso models identify the most useful predictive stand features for salvage decision-making (Tibshirani, 1996) which we report in the Section “3. Results.”
3. Results
3.1. Proportion of no salvage outcomes
Based on the data provided by Gunn et al. (2020), carbon budgets from salvaging shift from emitting more carbon in the near-term (10–20 years following SBW) to generally storing more carbon over longer time periods (20–40 years following SBW) compared to not salvaging (Figure 4). However, discounting greatly affects the proportion of study plots where salvaging is recommended. The percentage of no salvage plots ranges from 15 to 47% for discount rate scenarios 0–5% respectively (Figure 5). Treatment scenarios representing a range of residual stand conditions also impact salvage response outcomes to a lesser degree compared to discount rates. No salvage proportions range from 17% of plots on average for commercial industrial clearcuts (least additional salvage volume removed) to 40% for no management scenarios where more additional volume is removed from salvaging (Figure 6).
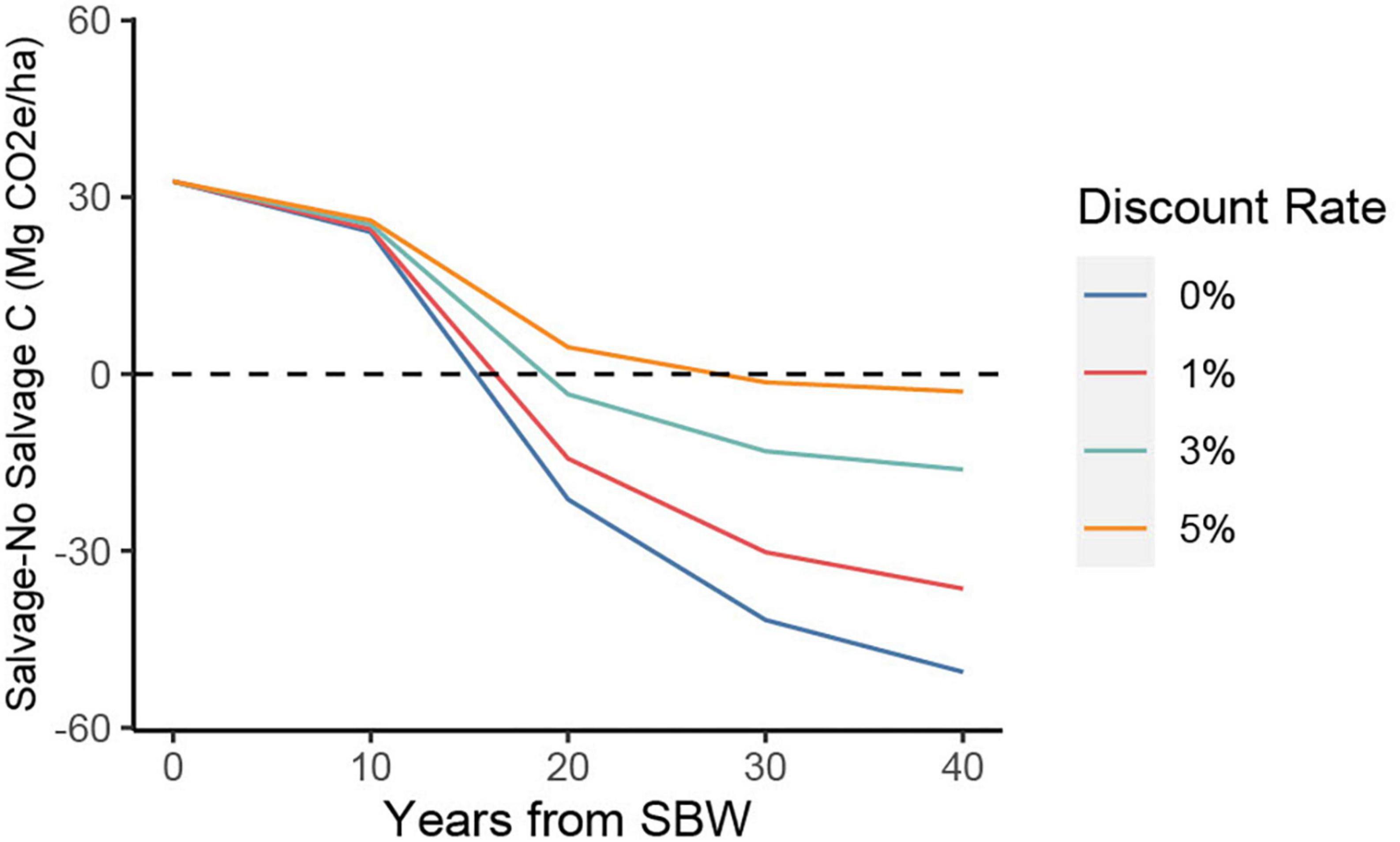
Figure 4. Salvage vs. no salvage carbon comparison over a 40-year period following SBW. Values represent the carbon difference between salvage and no salvage outcomes averaged across all plots and treatment scenarios. Positive values are the amount of additional carbon emitted by salvaging on average compared to not salvaging. Negative values are the additional carbon stored from salvaging compared to not salvaging.
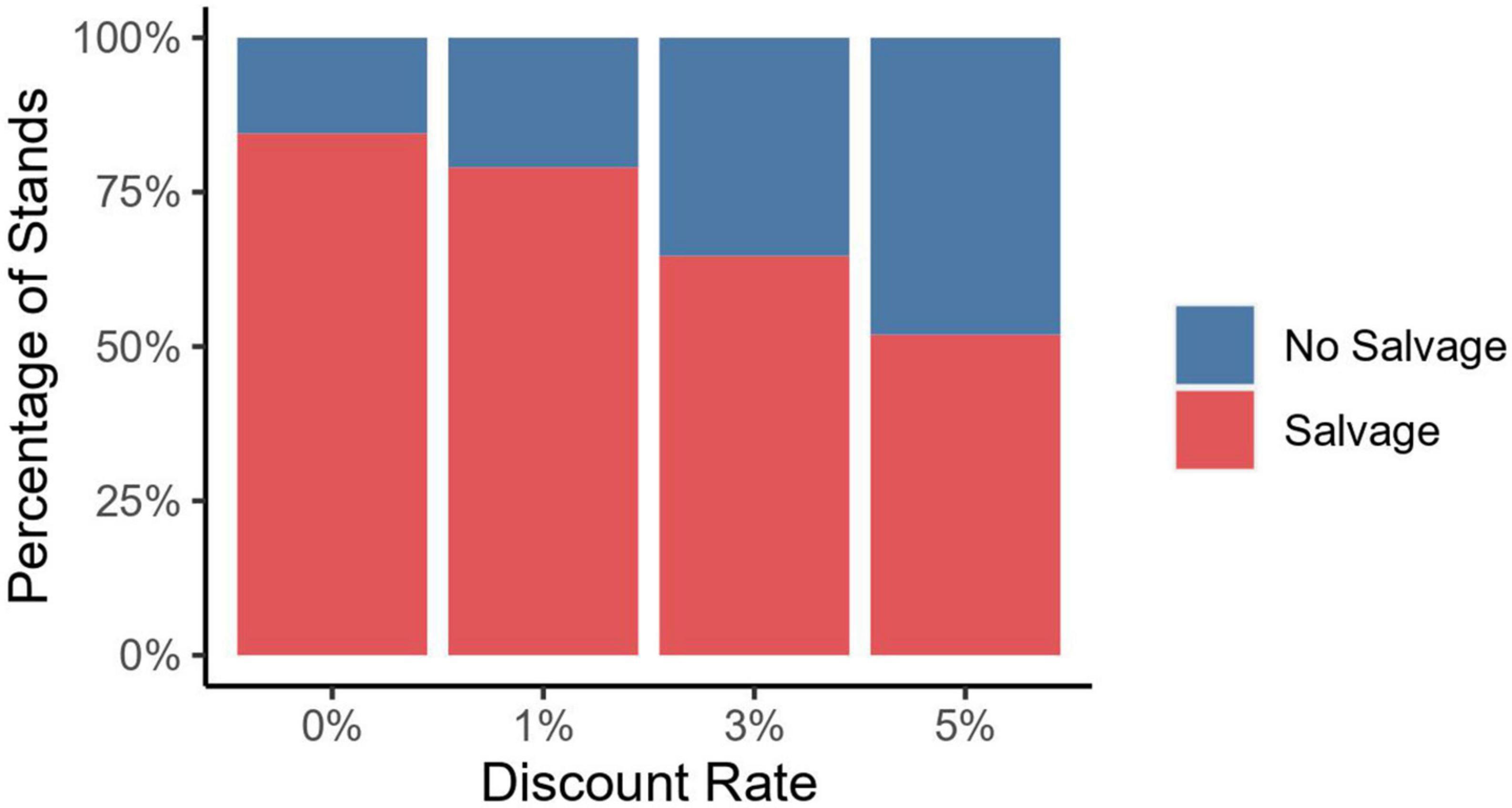
Figure 5. Salvage plot proportions in 0–5% discount rate scenarios averaged across treatment scenarios.
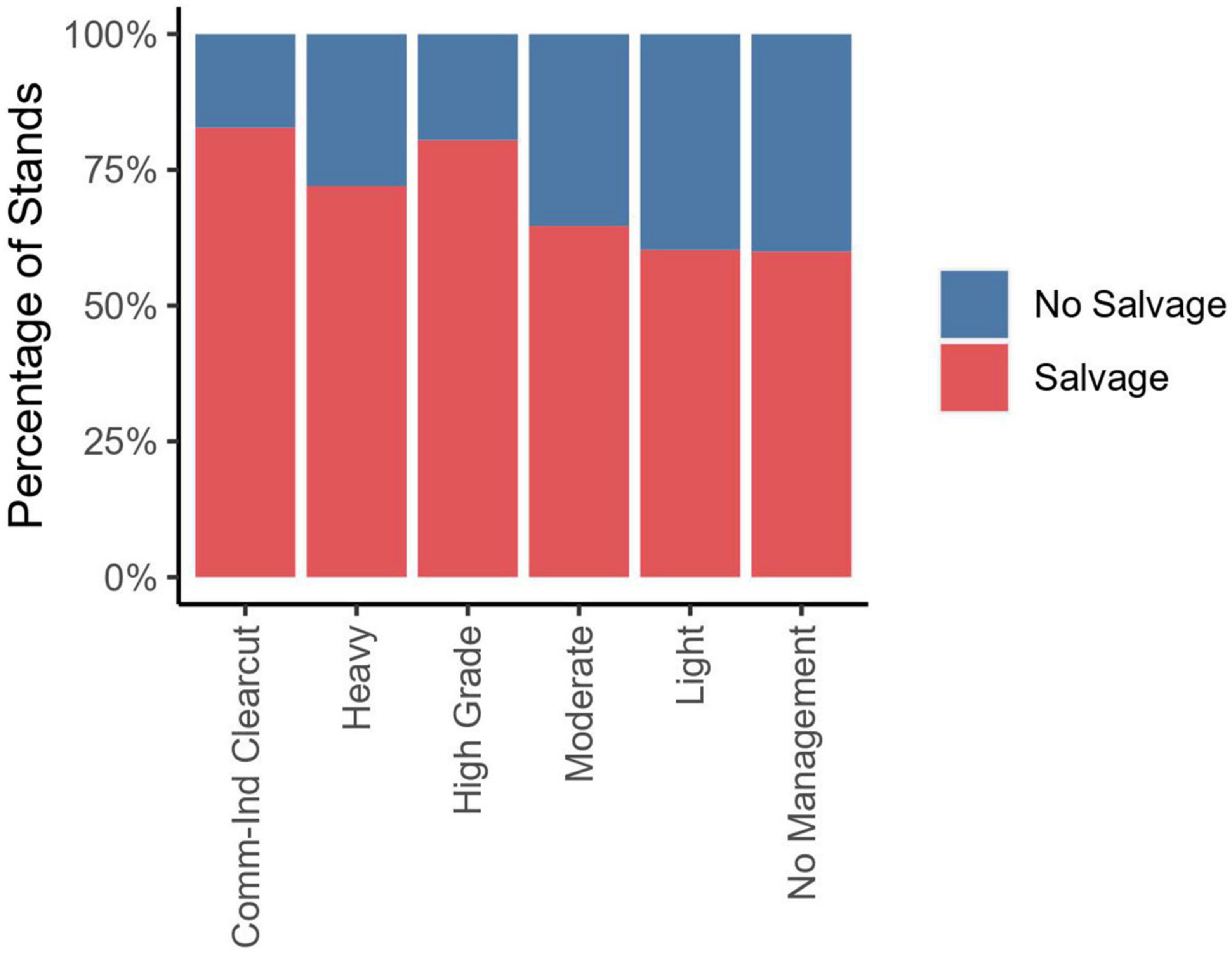
Figure 6. Salvage plot proportions in six treatment scenarios averaged across discount rates shows the degree to which treatment affects salvage results.
3.2. Lasso model performance
Training and test set root mean square error (RMSE) values decrease as discount rate increases, which indicates that lasso models perform better at higher discount rates. At a 0% discount rate, training, and test RMSE values are 60.0 and 59.0 respectively. In 5% discount rate scenarios, training, and test set RMSE values are 35.0 and 38.1 respectively.
While lasso regression fits higher discount rate scenarios better, classification error, or the percentage of plots where salvage response is classified incorrectly by lasso, increases with increasing discount rates. Training and test data classification error ranges from 15 to 32% for 0 to 5% discount rates, respectively. Sensitivity refers to the proportion of actual no salvage plots that are classified correctly by lasso. At 0 and 1% discount rate scenarios, the proportion of no salvage plots is so low that lasso almost always predicts a salvage response with a no salvage sensitivity rate of less than 1%. As the proportion of no salvage plots increases in 3 and 5% discount rate scenarios, sensitivity of no salvage prediction increases to 18 and 52% respectively.
3.3. Lasso carbon savings
Lasso strategies, which are salvage recommendations based on lasso linear equations, generated a net carbon savings (in Mg CO2e/ha) compared to the best strict strategy between always salvaging (salvaging all plots) or never salvaging (salvaging no plots) for 9 out of 24 regression models. Lasso strategies reach parity with the best strict strategy for all commercial-industrial clearcut and heavy treatment scenarios and for all high-grade scenarios except for at a 5% discount. Meaning lasso results recommend salvaging for these treatments except for high discount rate scenarios for high grade treatments. Lasso strategies provide the highest carbon savings at higher discount rates (3 and 5%) and in moderate to no management treatment scenarios where there is more volume removed from salvaging (Figures 7, 8). At a 3% discount rate, lasso strategies generated mean carbon savings of 0.43, 4.41, and 6.55 Mg CO2e/ha of carbon for moderate, light, and no management scenarios, respectively. At a 5% discount rate, lasso strategies stored 5.05, 3.41, 2.14, and 2.48 Mg CO2e/ha of additional carbon for high grade, moderate, light, and no management strategies respectively (Table 1). Lasso strategy decision-making led to a net loss of carbon (−0.03 Mg CO2e/ha) in light treatment scenarios at a 0% discount rate. For reference, the US Environmental Protection Agency’s (2022) carbon equivalency calculator estimates saving 1 Mg CO2e can be compared to saving roughly 2,400 miles driven by an average gas-powered car or 113 gallons of gasoline consumed. When applied at landscape or across larger scales, carbon savings from lasso could have major impacts on regional carbon budgets.
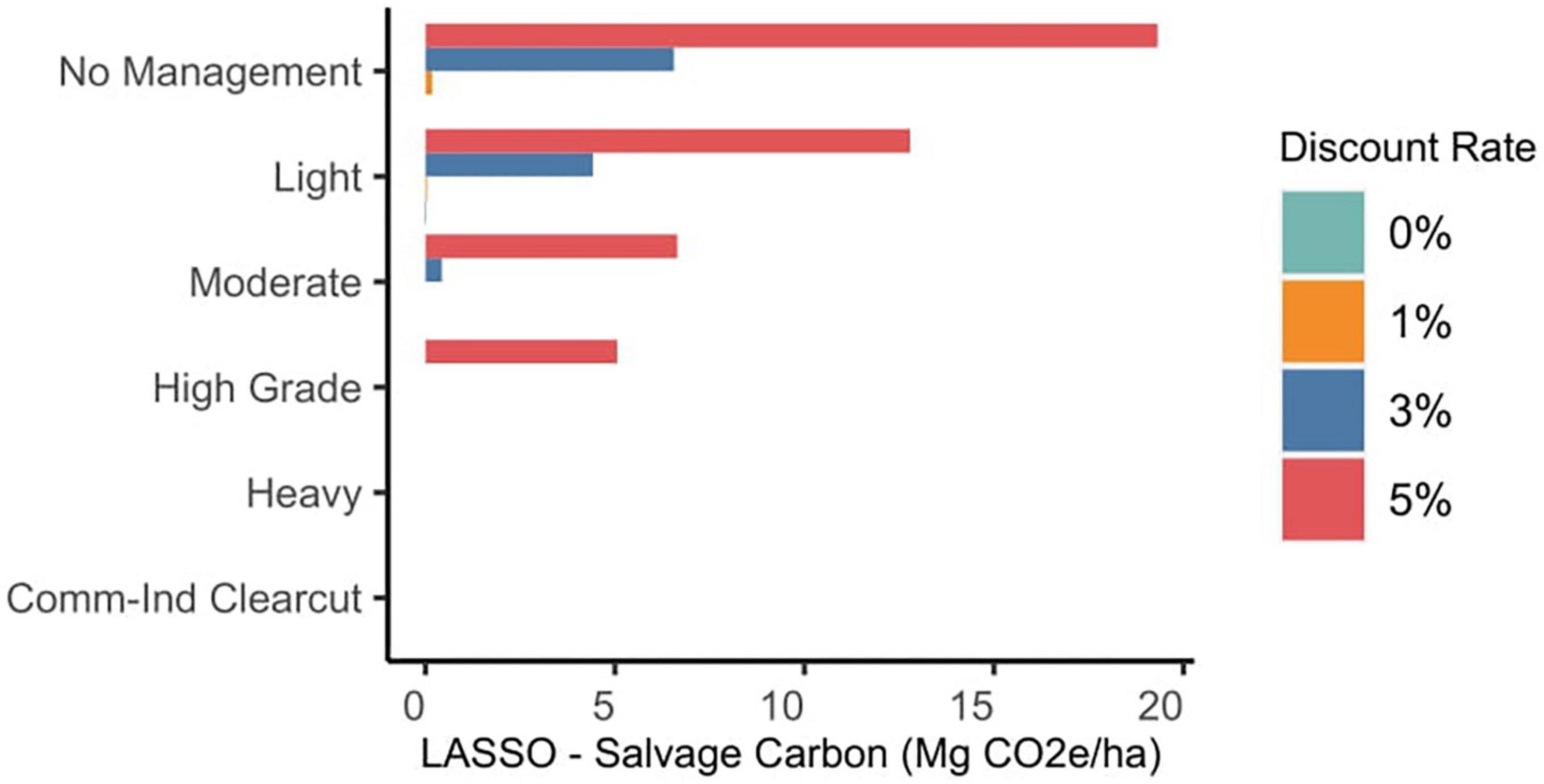
Figure 7. Mean carbon savings of lasso predictions compared to always salvaging. Savings are broken out by 0–5% discount rate scenarios.
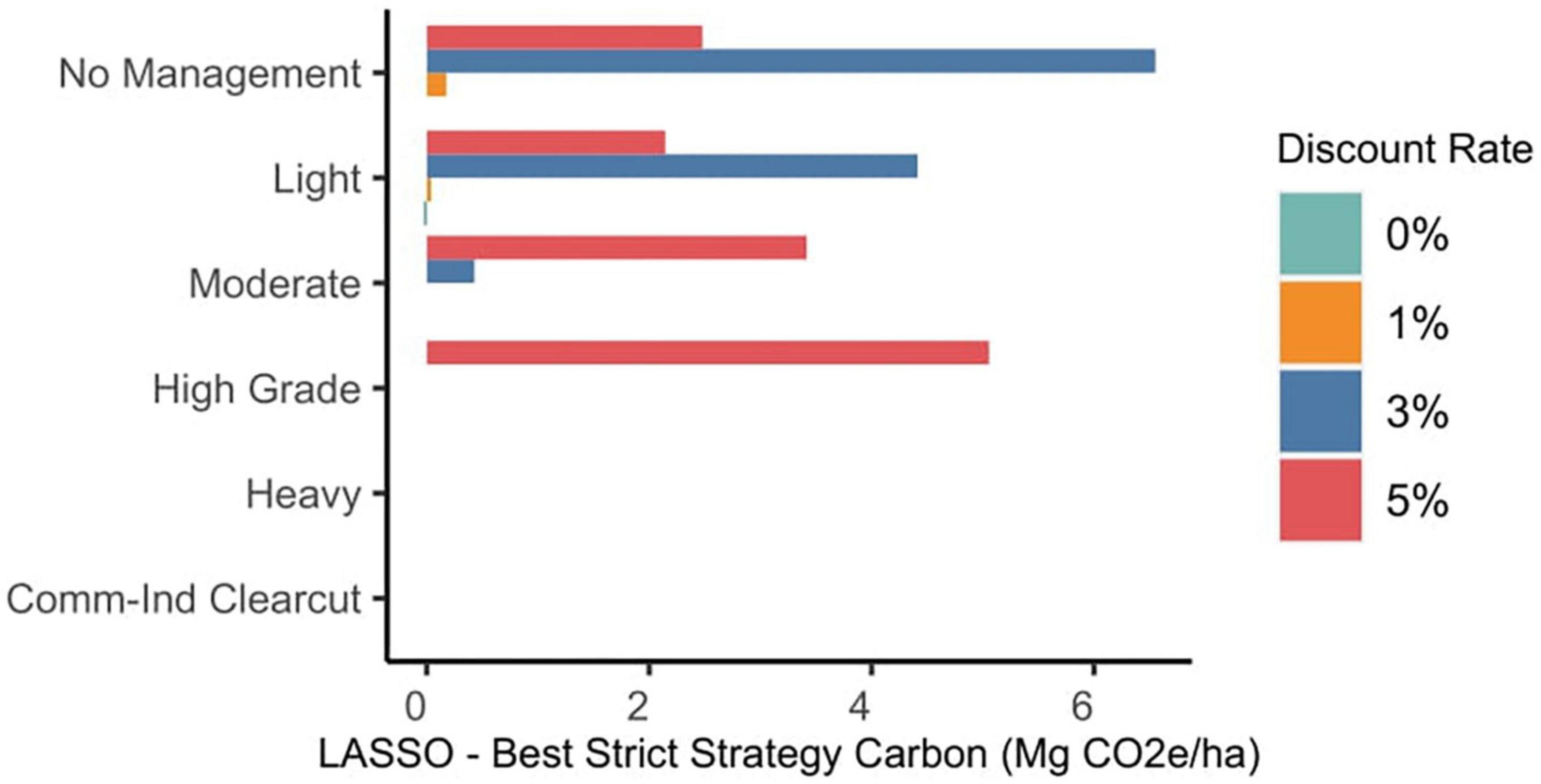
Figure 8. Mean carbon savings of lasso predictions compared to best strict strategy. In most scenarios, always salvaging is the best strict strategy, but a few high discount rate scenarios have never salvaging as the best strict strategy. Savings are broken out by 0–5% discount rate scenarios.
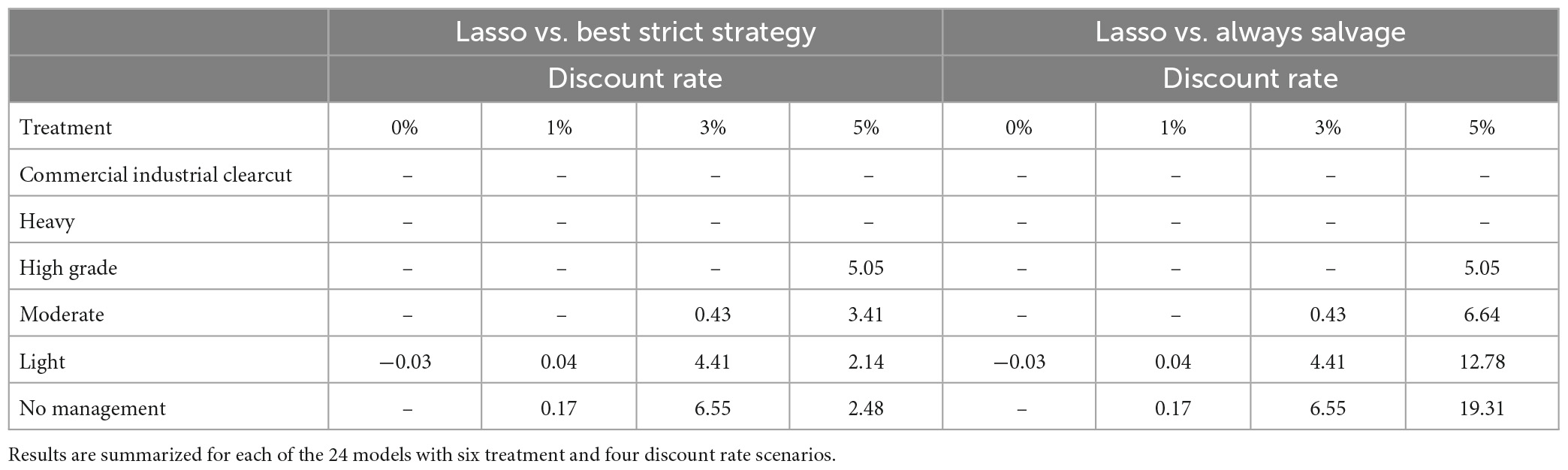
Table 1. Carbon savings (Mg CO2e/ha) generated from lasso strategies are shown in comparison to either using the best strict strategy (always or never salvaging) and always salvaging.
Comparing lasso to always salvaging generates carbon savings in the same scenarios (9 of 24 models) but yields much higher carbon savings in some of the lighter treatment scenarios at higher discount rates. When compared with always salvaging at a 5% discount rate, lasso predictions save an average of 5.05, 6.64, 12.78, and 19.31 Mg CO2e/ha of carbon in high grade, moderate, light, and no management treatment scenarios, respectively.
In most cases, lasso strategies either reach parity or store more carbon compared to always salvaging. The proportion of plots where lasso strategies improve on always salvaging increases at higher discount rates (Figure 9). Lasso strategies store more carbon on a small percentage of plots in 0 and 1% discount rate scenarios (less than 1% of plots in both scenarios). In 3 and 5% discount rate scenarios lasso strategies store more carbon at approximately 7 and 25% of plots, respectively.
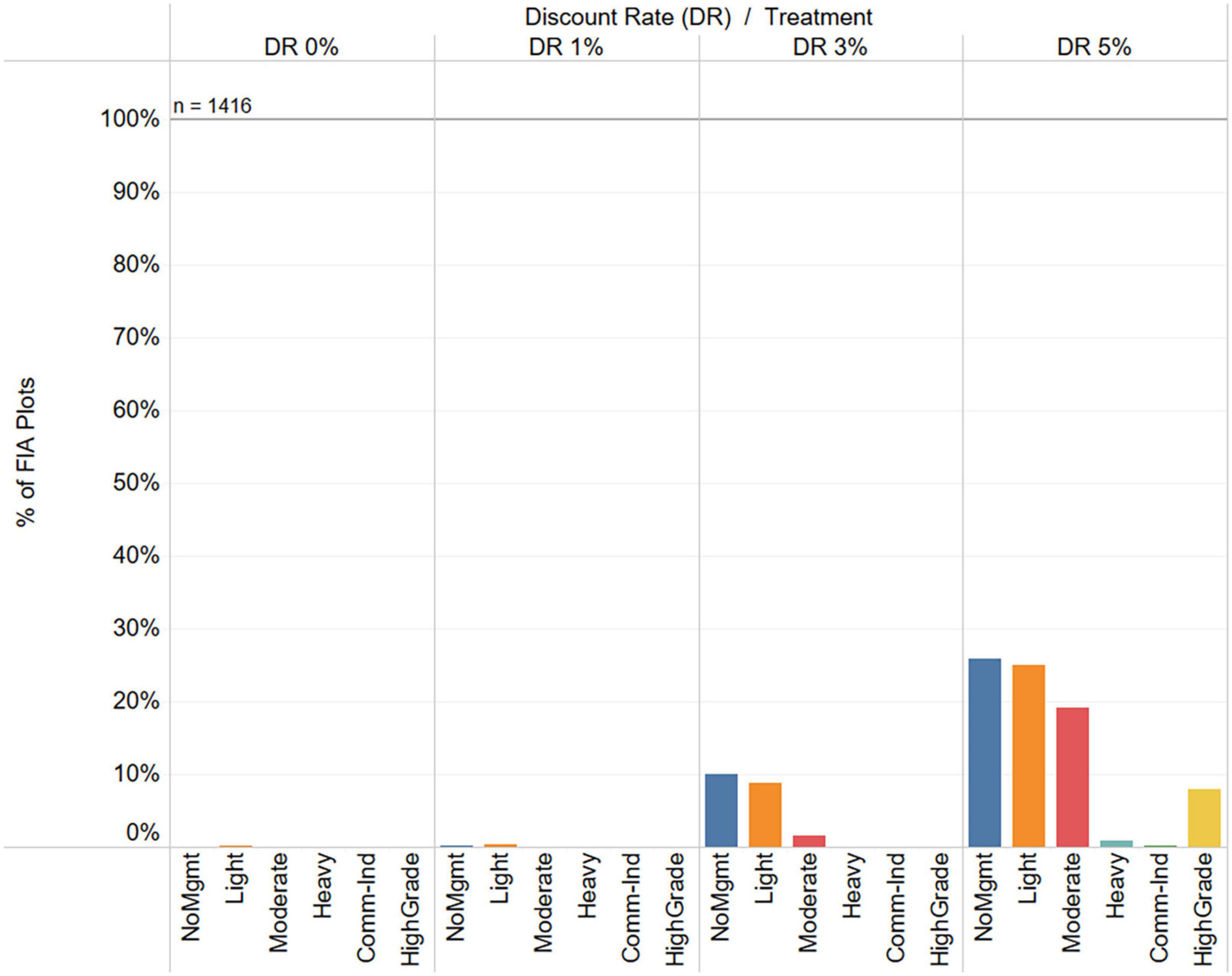
Figure 9. Lasso strategies store more carbon at higher discount rates. Lasso model decision-making stores more carbon compared to always salvaging at approximately 7 and 25% of plots for 3 and 5% discount rate scenarios, respectively, compared to less than 1% of plots for 0 and 1% discount rate scenarios.
3.4. Lasso coefficients
The nine lasso models (in Table 1) which saved more carbon than always salvaging used nine important features as coefficients visualized in Figure 10. Lasso generates linear equations that can be interpreted similarly to other linear regression methods where lasso models learn coefficient values that are then multiplied by feature values and summed to predict expected carbon (e.g., carbon = (β_SDI × SDI)+(β_QMD × QMD)+…). Features with negative lasso coefficients are expected to have lower values on no salvage plots (e.g., a negative Simpson’s D lasso coefficient likely indicates Simpson’s D values are lower on no salvage plots). Simpson’s D, balsam fir stocking, non-host basal area, and quaking aspen basal area all have negative lasso coefficients which implies that they are negatively related to a no salvage response (Table 2). Values for the four features previously listed are lower on average for no salvage plots, however, discount rate scenario affects these results (Figure 10). Balsam fir basal area is the only coefficient that is negative in lower discount scenarios and positive in higher discount rate scenarios. Likely due to average salvage and no salvage plot stand characteristics shown in Figure 10. Balsam fir QMD, other host basal area, other host stocking, and elevation have positive lasso coefficient values and are therefore positively related to not salvaging on average. These positive coefficient values suggest measurements of these four features are higher on no salvage plots compared to salvage plots. The lasso model for a moderate treatment scenario at a 3% discount rate is the only model that has negative coefficient values for both balsam fir QMD and other host basal area.
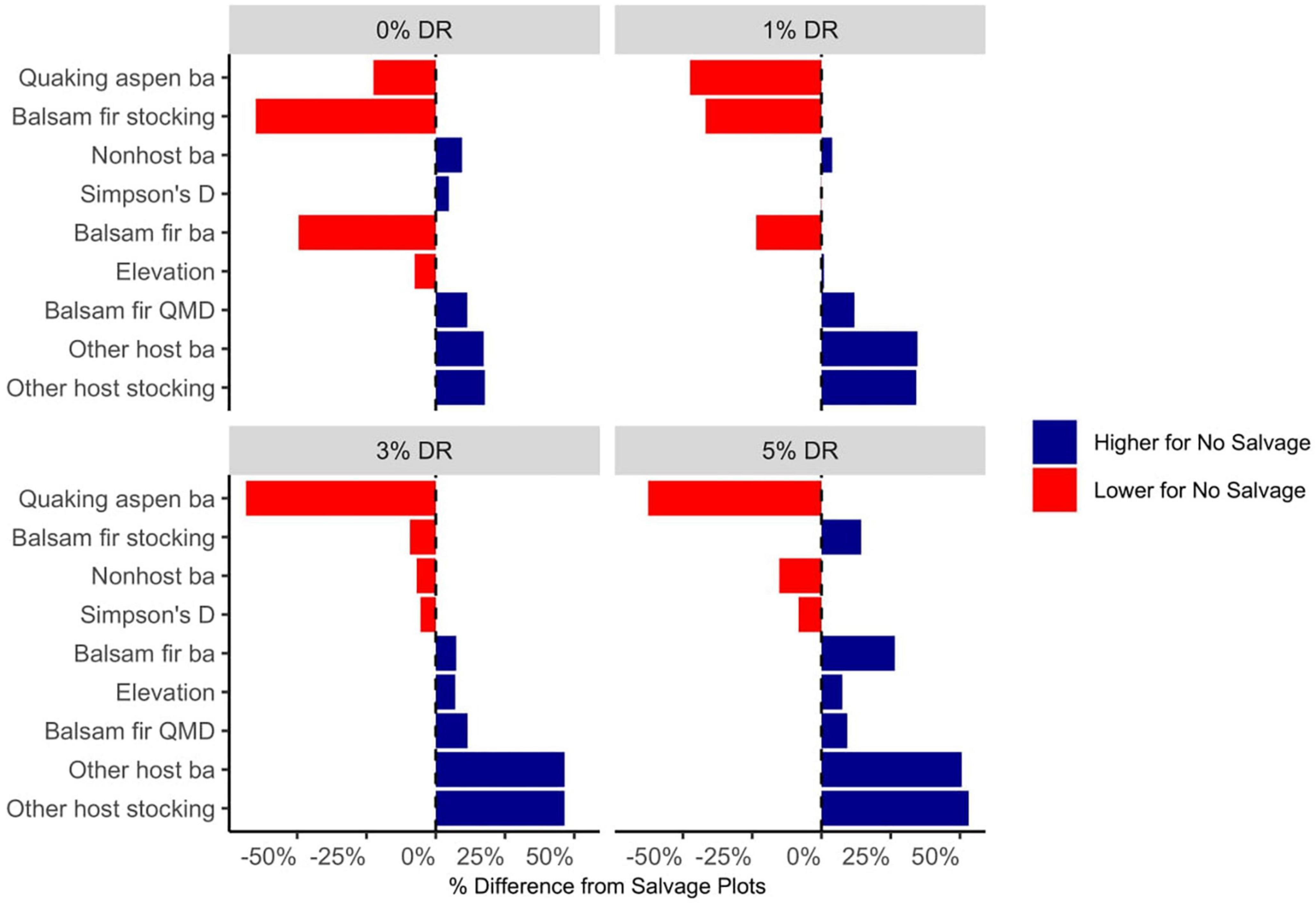
Figure 10. Comparison of stand conditions for salvage and no salvage plots across 0–5% discount rate scenarios. Top 10 lasso model features are shown.
3.5. Stand characteristics
Salvage and no salvage plots generally have different stand characteristics, which are also influenced by discount rate. We looked specifically at mean values for the nine stand characteristics lasso models chose as the best predictors of carbon and observed a range of results based on discount scenario. Other host (spruce species) stocking and basal area and balsam fir QMD are consistently higher on average for no salvage plots across discount rates. Characteristics that support the presence of balsam fir (balsam fir stocking, basal area, and elevation) have mean values that are lower for no salvage plots in low discount scenarios and gradually increase until these characteristics are higher in high discount rate scenarios. Similarly, characteristics that support the presence of spruce budworm non-host species (quaking aspen basal area, non-host basal area, and Simpson’s D) have higher mean values for no salvage plots at lower discount rates and gradually decrease at higher discount rates.
4. Discussion
4.1. Stand characteristics
In the context of spruce budworm (SBW), lasso models recommend salvaging less often on less diverse plots (lower Simpson’s D) and on plots with greater proportions of spruce species and larger balsam fir trees present. A no salvage response on plots with lower Simpson’s D values could be driven by a slower regeneration response after salvaging. In some cases, sites with low diversity are poorer quality or degraded sites that have an increased risk of not recovering forest carbon losses taken on by salvaging. In other cases, Simpson’s D values are low due to large proportions of spruce-fir without many other species present.
The amount of wood that is removed during a salvage operation also plays a role indecision-making based on carbon. Salvaging more volume creates larger swings in carbon budgets and increases the risk of not replenishing carbon stocks removed within the expected 40 years. We also observed this trend based on volume across treatment scenarios. Not salvaging was recommended more often in no management to moderate treatment scenarios, where more volume is available to salvage compared to heavy or commercial industrial clearcut scenarios. Again, this is likely due to more volume removal during salvage operations. Heavy salvaging is more risky and potentially harmful from a carbon standpoint in both the near and long-term.
While it is useful to know that pre-outbreak removal volume is a contributing factor to salvaging outcomes, grouping volume and size class removal into six treatment scenarios reduces the level of detail in our results. For example, light treatments (25–35% basal area removed) recommend salvaging much less often than moderate treatments (45–50% basal area removed), but there is no scenario that captures an in between basal area removal (35–45% basal area removed). The recommendation to salvage could be different based on which treatment scenario is assigned. In this study, we chose ease of interpretation, but more in-depth effects of volume removal could be explored in future studies.
Our finding that the size of balsam fir trees plays a role in salvage preference is supported by other studies that have found larger host species trees to be more susceptible to SBW (Fraver et al., 2007). Younger, smaller balsam fir trees that are beneath larger trees are less vulnerable to SBW-induced mortality (Boulanger and Arseneault, 2004). The presence of larger host species individuals suggests greater mortality due to SBW and more volume to be salvaged, thus having a greater impact on carbon budgets. Cotton-Gagnon et al. (2018) presents additional considerations for the timing of salvage logging. Salvaging during an SBW outbreak rather than post-outbreak potentially increases the mortality of spruce-fir regeneration with greater impact on black spruce. In this study we assumed post-outbreak salvaging, but timing could be explored further in future studies.
Considerations of salvage preference and host species volume and size may also be useful beyond our study region of the northeastern US. Sections of Canada that experience SBW and are shaping carbon policies may also benefit from applying a similar framework that uses stand characteristics to assess carbon outcomes after salvaging. Similar relationships between host tree species and salvaging based on carbon could also be explored for other disturbance regimes similar to SBW, like gypsy moth (Lymantria dispar), mountain pine beetle (Dendroctonus ponderosae), and hemlock woolly adelgid (Adelges tsugae).
4.2. Discounting
The choice of discount rate greatly impacts salvage decision-support results. In general, high discount rates can be applied when maximizing near-term carbon storage is preferred (i.e., achieving climate action plan goals). In cases where the timing of carbon emissions is not a concern, lower discount rate scenarios may be preferred. Higher discount rates increase the proportion of plots where not salvaging is recommended due to a greater preference for near-term carbon savings. Lasso models generally perform better (i.e., lower RMSE values) in higher discount rate scenarios because proportions of salvage and no salvage plots are almost equal. In low discount rate scenarios, where salvaging is preferred at the majority of plots, lasso models almost always predict a salvage response. A majority salvage response in low discount rate scenarios yields lower classification errors, but also low specificity rates (i.e., rate of correct no salvage classification) for predicting no salvage plots. In higher discount rate scenarios, classification error increases because lasso no longer chooses a strict salvage response, however, no salvage plot prediction is greatly improved. This is a consideration for lasso model utility in a management context, where lower discount rate scenario models were not good predictors for no salvage plots compared to higher discount rate scenarios. In these low discount rate scenarios, more general stand characteristics (e.g., less productive site) could be used to inform salvage decision-making.
Discounting also affects average stand characteristics across salvage and no salvage plots. In lower discount rate scenarios, salvaging is preferred at plots with features that support the presence of balsam fir, such as balsam fir stocking and basal area. In these scenarios, we suspect advance regeneration of smaller diameter balsam fir trees play a role in 40-year carbon outcomes, where balsam fir QMD is a better indicator of salvage response compared to volume or stocking. In higher discount rate scenarios, stands with higher volumes SBW host species reflect a preference for near-term sequestration, and recommend salvaging less often.
While discount rates of 0–5% are generally an accepted range for carbon, discounting at a flat rate over longer time periods (e.g., greater than 20 years) can result in pre-determined outcomes where high discount rates encourage activities that increase carbon stocks in the short term while discouraging any long-term activity that yields long-term carbon stock increases (Iverson and Karp, 2021). A hyperbolic discounting approach that decreases discount rate incrementally over time is a possible solution to over-discounting. However, very little guidance is available to apply a hyperbolic discounting approach within a carbon and especially within a forestry context (i.e., when to decrease discount rate and by how much) (Hepburn and Koundouri, 2007). Applying a decreasing hyperbolic discounting approach that would, for example, discount carbon by 5% for the first decade, then discount by only 4% for the next decade and so on, to this study likely yields final carbon results within our provided range of 0–5%. By applying a consistent discount rate at low and high ranges for carbon, we are capturing the full extent to which discounting might impact salvage decision-making results. Caution should be used when applying discount rates at the high end of this range (5%) as this represents heavily discounted values over longer time periods and a much greater preference for near-term carbon storage.
4.3. Lasso model utility
Lasso model equations can be used for salvage decision-making at a stand scale across the northeast. Our findings reflect the utility of using lasso models for decision-support, where models are generally more useful in higher discount rate scenarios in which near-term carbon storage drives salvage response. In these higher discount rate scenarios, lasso recommendations to not salvage lead to greater carbon storage in the near-term compared to always salvaging. The additional Mg CO2e/ha that is stored from choosing not to salvage also varies by stand condition, where more carbon is expected to be stored in lighter treatment scenarios or sites where there is more volume to salvage. When applied at larger regional scales, lasso strategy carbon savings could potentially be significant. These carbon savings have policy implications as described above and could potentially be cause for landowner compensation to not salvage on plots that meet no salvage criteria.
Some potential sources of error in lasso models include the presence of outliers in salvage and no salvage plots. These are the plots where there was a large difference in carbon saved (in some cases up to 700 Mg Co2e/ha difference) between salvaging or not salvaging. There was no obvious driver of outliers in our dataset. Poor predictions of carbon values at these sites could be influenced by non-linear effects not captured in lasso models. Microclimates and other small-scale forest dynamics could also influence carbon values at specific sites and provide a source of error in FVS and lasso models.
4.4. Policy implications
Salvaging is often the preferred management response to large forest disturbances, especially for private landowners who may depend on financial income from private woodlots. As a result, policies to incentivize not salvaging may be more targeted toward private land compared to public forest land. However, it would also be appropriate in contexts where public lands have a mandate to generate revenue. Recent stumpage prices for spruce-fir sawlogs range in value from $75 to $200 per thousand board feet (or $31–$83 per cubic meter) across northern New England states (Evans and NH Department of Revenue Administration, 2021; Maine Forest Service, Forest Policy and Management Division, 2021; Vermont Department of Forests, Parks and Recreation, 2021). Spruce-fir mortality driven by SBW could create economic loss for landowners and the forest products industry. However, a widespread salvage response, such as those that occurred during previous SBW outbreaks, could create major shifts in northeast forest carbon sinks (Chen et al., 2019). A large net emission of carbon in the first 10–20 years following salvaging could prevent states from reaching emissions reduction goals within the near-term. For example, Maine’s 2,045 net-zero carbon initiative that relies heavily on the state’s forest carbon sequestration capacity to balance GHG emissions, is likely to be affected by SBW salvage response. If the majority of landowners choose to salvage spruce-fir affected by SBW, regional carbon sequestration could be greatly reduced in the near-term.
An economic incentive for not salvaging could be necessary to balance salvage response to the coming SBW outbreak, particularly on private lands. Carbon credits, equivalent to one metric ton of carbon dioxide equivalent sequestered, were mostly recently valued at over $7 on average for US-based forestry and land use-based credits (Donofrio et al., 2020). In this context, credits could be rewarded for the additional tons (Mg) of carbon stored from not salvaging. Given the value of spruce-fir sawlogs, carbon credit prices may need to be adjusted to more closely compensate landowners for choosing not to salvage spruce and fir. Adding carbon to the list of projects funded by NRCS cost-share could be beneficial for increasing landowner participation in NCS forestry practices. Within the context of salvage logging, NRCS cost-share payments for wood not salvaged could contribute to managing regional carbon budgets. Additional financial incentives may also need to be considered based on the value of timber foregone by choosing not to salvage. Outside of the US, particularly in regions where the forest is primarily under public management, the approach we present here still has relevance to support decision making when faced with potential widespread tree mortality salvage situations.
5. Conclusion
Salvage harvest decision-making is a necessary addition to local and state climate plans because large scale disturbances, such as SBW outbreaks, have the potential to shift regional carbon budgets. A more balanced salvage and no salvage approach is needed to prevent major regional swings in forest carbon storage. More guidance for sites where not salvaging is preferred is also needed. Based on lasso results across discount and treatment scenarios, stands with greater volumes of host species, especially those stands with larger average diameter host species could generate more dramatic swings in carbon budgets favoring a no salvage response, increasing the risk of timely carbon regeneration. In cases where carbon is an important management objective, not salvaging or partially salvaging is recommended on sites with large diameter host trees or for sites that are poorer quality or where more volume is to be removed due to higher near-term emissions. Salvaging may be more beneficial on sites where a moderate amount of SBW host species and volume available to salvage, and on sites where advance regeneration is present to begin sequestering carbon more quickly post-salvage.
Lasso models provide decision-support for predicting the preferred salvage response based on carbon at a stand scale. The choice of discount rate impacts salvage results from lasso models, where applying a higher discount rate that reflects a greater preference for near-term carbon storage favors salvaging less often. Our findings provide stand-level equations for salvage decision-support based on carbon, and also more broad findings on stand conditions and emissions preferences that influence salvage recommendations. In cases where carbon is an important management objective, salvage preference could be largely dependent on the desired timing of maximizing carbon sinks where many climate action plans cite carbon emissions reduction goals within the next decade, and the condition of the site, where new regeneration is key to regenerating forest carbon stocks. SBW specific results are potentially useful beyond our study area such as in parts of Canada. Broader relationships between host tree species volume and size and salvage preference based on carbon could also be explored for similar disturbances such as mountain pine beetle and hemlock woolly adelgid. Policies that address salvage logging are necessary contributions to future climate action plans seeking effective carbon emissions mitigation outcomes.
Data availability statement
The raw data supporting the conclusions of this article will be made available by the authors, without undue reservation.
Author contributions
JG and MP conceived the study. JG, MP, LS, and SS generated the study and analysis. MD, TB, and EB provided the analytical feedback. LS wrote the first draft of the manuscript. JG, MP, MD, TB, and EB added the additional content and revisions. All authors contributed to the article and approved the submitted version.
Funding
This study was funded by the NSF Award #1920908, an NSF RII Track project, Leveraging Intelligent Informatics and Smart Data for Improved Understanding of Northern Forest Ecosystem Resilience (INSPIRES).
Acknowledgments
We thank the reviewers for their helpful comments to improve this manuscript.
Conflict of interest
TB was employed by Spatial Informatics Group, LLC.
The remaining authors declare that the research was conducted in the absence of any commercial or financial relationships that could be construed as a potential conflict of interest.
Publisher’s note
All claims expressed in this article are solely those of the authors and do not necessarily represent those of their affiliated organizations, or those of the publisher, the editors and the reviewers. Any product that may be evaluated in this article, or claim that may be made by its manufacturer, is not guaranteed or endorsed by the publisher.
References
Anderegg, W. R. L., Trugman, A. T., Badgley, G., Anderson, C. M., Bartuska, A., Ciais, P., et al. (2020). Climate-driven risks to the climate mitigation potential of forests. Science 368:eaaz7005. doi: 10.1126/science.aaz7005
Arner, S. L., Woudenberg, S., Waters, S., Vissage, J., Maclean, C., Thompson, M., et al. (2001). National algorithms for determining stocking class, stand size class, and forest type for forest inventory and analysis plots. Washington, DC: U.S. Department of Agriculture Forest Service.
Bachmann, T. M. (2020). Considering environmental costs of greenhouse gas emissions for setting a CO2 tax: A review. Sci. Total Environ. 720:137524. doi: 10.1016/j.scitotenv.2020.137524
Boulanger, Y., and Arseneault, D. (2004). Spruce budworm outbreaks in eastern Quebec over the last 450 years. Can. J. For. Res. 34, 1035–1043. doi: 10.1139/x03-269
Chen, C., Wei, X., Weiskittel, A., and Hayes, D. J. (2019). Above-ground carbon stock in merchantable trees not reduced between cycles of spruce budworm outbreaks due to changing species composition in spruce-fir forests of Maine, USA. For. Ecol. Manage. 453:117590. doi: 10.1016/j.foreco.2019.117590
Cotton-Gagnon, A., Simard, M., De Grandpré, L., and Kneeshaw, D. (2018). Salvage logging during spruce budworm outbreaks increases defoliation of black spruce regeneration. For. Ecol. Manage. 430, 421–430. doi: 10.1016/j.foreco.2018.08.011
Daigneault, A., Simons-Legaard, E., Birthisel, S., Carroll, J., Fernandez, I., and Weiskittel, A. (2021). Maine Forestry and Agriculture Natural Climate Solutions Mitigation Potential Final Report. Orono, ME: University of Maine.
Donofrio, S., Maguire, P., Myers, K., Associate, S., Daley, C., and Lin, K. (2021). Markets in motion: State of voluntary carbon markets 2021 installment 1. Wageningen: Wageningen University and Research.
Donofrio, S., Maguire, P., Zwick, S., and Merry, W. (2020). Voluntary carbon and the post-pandemic recovery. Washington DC: Ecosystem Marketplace.
Dymond, C. C., Neilson, E. T., Stinson, G., Porter, K., MacLean, D. A., Gray, D. R., et al. (2010). Future spruce budworm outbreak may create a carbon source in Eastern Canadian forests. Ecosystems 13, 917–931. doi: 10.1007/s10021-010-9364-z
Evans, R., and NH Department of Revenue Administration (2021). NH average stumpage value list, April 2021-September 2021. Concord, NH: NH Department of Revenue Administration.
Fargione, J. E., Bassett, S., Boucher, T., Bridgham, S. D., Conant, R. T., Cook-Patton, S. C., et al. (2018). Natural climate solutions for the United States. Sci. Adv. 4:eaat1869.
Fraver, S., Seymour, R. S., Speer, J. H., and White, A. S. (2007). Dendrochronological reconstruction of spruce budworm outbreaks in northern Maine, USA. Can. J. For. Res. 37, 523–529. doi: 10.1139/X06-251
Friedman, J. H., Hastie, T., and Tibshirani, R. (2010). Regularization paths for generalized linear models via coordinate descent. J. Stat. Softw. 33, 1–22. doi: 10.18637/jss.v033.i01
Gasser, T., Guivarch, C., Tachiiri, K., Jones, C. D., and Ciais, P. (2015). Negative emissions physically needed to keep global warming below 2°C. Nat. Commun. 6:8958. doi: 10.1038/ncomms8958
Griscom, B. W., Adams, J., Ellis, P. W., Houghton, R. A., Lomax, G., Miteva, D. A., et al. (2017). Natural climate solutions. Proc. Natl. Acad. Sci. U.S.A. 114, 11645–11650. doi: 10.1073/pnas.1710465114
Griscom, B. W., Lomax, G., Kroeger, T., Fargione, J. E., Adams, J., Almond, L., et al. (2019). We need both natural and energy solutions to stabilize our climate. Glob. Change Biol. 25, 1889–1890.
Gunn, J. S., Ducey, M. J., Buchholz, T., and Belair, E. P. (2020). Forest carbon resilience of eastern spruce budworm (Choristoneura fumiferana) salvage harvesting in the northeastern United States. Front. For. Glob. Change 3:14. doi: 10.3389/ffgc.2020.00014
Hennigar, C. R., Maclean, D. A., Quiring, D. T., and Kershaw, J. A. Jr. (2008). Differences in spruce budworm defoliation among balsam fir and white, red, and black spruce. For. Sci. 54, 158–166. doi: 10.1093/forestscience/54.2.158
Hennigar, C. R., Wilson, J. S., MacLean, D. A., and Wagner, R. G. (2011). Applying a spruce budworm decision support system to maine: Projecting spruce-fir volume impacts under alternative management and outbreak scenarios. J. For. 109, 332–342. doi: 10.1093/jof/109.6.332
Hepburn, C., and Koundouri, P. (2007). Recent advances in discounting: Implications for forest economics. J. For. Econ. 13, 169–189. doi: 10.1016/j.jfe.2007.02.008
Irland, L. C., Dimond, J. B., Stone, J. L., Falk, J., Baum, E., Dimond, J. B., et al. (1988). B819: The spruce budworm outbreak in Maine in the 1970’s-Assessment and directions for the future recommended citation. Orono, ME: University of Maine.
Iverson, T., and Karp, L. (2021). Carbon taxes and climate commitment with non-constant time preference. Rev. Econ. Stud. 88, 764–799. doi: 10.1093/restud/rdaa048
Legaard, K. R., Sader, S. A., and Simons-Legaard, E. M. (2015). Evaluating the impact of abrupt changes in forest policy and management practices on landscape dynamics: Analysis of a lands at image time series in the Atlantic Northern forest. PLoS One 10:e0130428. doi: 10.1371/journal.pone.0130428
Liu, Z., Peng, C., De Grandpré, L., Candau, J., Work, T., Huang, C., et al. (2019). Simulation and analysis of the effect of a spruce budworm outbreak on carbon dynamics in boreal forests of Quebec. Ecosystems 22, 1838–1851. doi: 10.1007/s10021-019-00377-7
Lueddeckens, S., Saling, P., and Guenther, E. (2021). Discounting and life cycle assessment: a distorting measure in assessments, a reasonable instrument for decisions. Int. J. Environ. Sci. Technol. 19, 2961–2972. doi: 10.1007/s13762-021-03426-8
Maine Forest Service, Forest Policy and Management Division (2021). 2019 Stumpage prices by Maine county/unit. Orono, ME: Maine Forest Service.
Mengel, M., Nauels, A., Rogelj, J., and Schleussner, C. F. (2018). Committed sea-level rise under the Paris Agreement and the legacy of delayed mitigation action. Nat. Commun. 9:601. doi: 10.1038/s41467-018-02985-8
Ranstam, J., and Cook, J. A. (2018). LASSO regression. Br. J. Surg. 105:1348. doi: 10.1002/bjs.10895
Richards, T. J., and Green, G. P. (2015). Environmental choices and hyperbolic discounting: An experimental analysis. Environ. Resour. Econ. 62, 83–103. doi: 10.1007/s10640-014-9816-6
Solomon, D. S., Zhang, L. J., Brann, T. B., and Larrick, D. S. (2003). Mortality patterns following spruce budworm infestation in unprotected spruce-fir forests in Maine. North. J. Appl. For. 20, 148–153. doi: 10.1093/njaf/20.4.148
Tibshirani, R. (1996). Regression shrinkage and selection via the lasso. J. R. Stat. Soc. Ser. B 58, 267–288.
US Environmental Protection Agency’s (2022). Greenhouse gas equivalencies calculator. Washington, D.C: US Environmental Protection Agency.
USDA Forest Service (2017). Forest Inventory and Analysis Fiscal Year 2016 Business Report. Washington, DC: United States Department of Agriculture.
Vermont Department of Forests, Parks and Recreation (2021). Vermont stumpage report, 2nd quarter 2021. Montpelier: Vermont Department of Forests, Parks and Recreation.
Wagner, R. G., Strauch, P., Denico, D., Bryant, J., Management, F., Management, A. F., et al. (2016). Coming spruce budworm outbreak: Initial risk assessment and preparation and response recommendations for Maine’s forestry community. Orono, ME: Spruce Budworm Maine.
Keywords: carbon, lasso, eastern spruce budworm, decision-support, discounting
Citation: Scott LN, Smith SM, Gunn JS, Petrik M, Ducey MJ, Buchholz TS and Belair EP (2023) Salvage decision-making based on carbon following an eastern spruce budworm outbreak. Front. For. Glob. Change 6:1062176. doi: 10.3389/ffgc.2023.1062176
Received: 05 October 2022; Accepted: 11 April 2023;
Published: 04 May 2023.
Edited by:
Nicholas Adam Povak, Forest Service (USDA), United StatesReviewed by:
Louis Robert, Department of Natural Resources, CanadaDan Kneeshaw, Université du Québec à Montréal, Canada
Copyright © 2023 Scott, Smith, Gunn, Petrik, Ducey, Buchholz and Belair. This is an open-access article distributed under the terms of the Creative Commons Attribution License (CC BY). The use, distribution or reproduction in other forums is permitted, provided the original author(s) and the copyright owner(s) are credited and that the original publication in this journal is cited, in accordance with accepted academic practice. No use, distribution or reproduction is permitted which does not comply with these terms.
*Correspondence: John S. Gunn, Sm9obi5HdW5uQFROQy5vcmc=