- 1Institute of Photogrammetry and Remote Sensing, Technische Universität (TU) Dresden, Dresden, Germany
- 2Department of Ecological Modelling, Helmholtz Centre for Environmental Research – UFZ, Leipzig, Germany
- 3German Centre for Integrative Biodiversity Research (iDiv) Halle-Jena-Leipzig, Leipzig, Germany
- 4Alexander von Humboldt Biological Resources Research Institute, Bogotá, Colombia
Analyzing the spatial association pattern among species can help to better understanding the mechanisms that drive forest dynamics and assembly. We applied techniques of spatial point pattern analysis to data from a fully mapped plot of tropical dry forest (TDF) in Colombia to assess the spatial association network among the eight most abundant species and we tested the hypothesis that species traits related to the ability to cope with drought stress could explain the observed spatial association patterns. We conducted three analyses, first we classified the types of spatial association patterns of species pairs against a null model of spatial independence, second, we used a heterogeneous Poisson (HP) null-model to assess competitive and facilitative interactions, and finally, we integrated the spatial association network with a traits space spanned by hydraulic functional traits. Overall, the proportion of significant negative and positive associations were low and we found at smaller spatial scales (5 m) prevalence of positive association patterns (11%) and at intermediate scales (16 m) negative interactions (13%). The dominant, evergreen and bird-dispersed species Trichilia oligofoliata, which followed a hydraulically save strategy, was involved in most positive associations at small scales, whereas the evergreen large statured species Aspidosperma polyneuron, which also follows a conservative resource-use strategy, was involved in most negative interactions. In TDFs where water stress is prevalent, tree community assembly and spatial patterns formation are regulated by environmental heterogeneity (e.g., topography), and both facilitative and competitive processes act simultaneously, but at different spatial scales and involving different species. Our findings highlight the potential importance of the examined association patterns, not only for our understanding of community assembly, but also to provide restoration directions.
1. Introduction
Tropical dry forests (TDFs) that are characterized by marked seasonality with a prolonged period of dry months, account for about 40% of all tropical forests (Miles et al., 2006). This ecosystem plays a key role in regulating the global environment by contributing significantly to the carbon stock (Becknell et al., 2012) and by supporting and regulating several ecosystem services (Djoudi et al., 2015), and TDFs are particularly important for biodiversity conservation, water protection, and soil erosion control. Although the conservation value of TDFs is increasingly recognized (Sánchez-Azofeifa and Powers, 2014), studies on their dynamics and assembly are lacking (Siyum, 2020; Calvo-Rodriguez et al., 2021). However, ecologically effective forest management requires a good understanding of the ecological processes that determine forest dynamics and assembly, especially in Colombia, where only 5% of the TDFs are protected and 90% of their original cover has been lost (Pizano and García, 2014).
The main mechanisms that structure a forest community will depend on the species present in the forest and their adaptations to environmental conditions (Choler et al., 2001). This is especially true in stressful environments such as TDFs, where trees are subject to severe seasonal droughts (Murphy and Lugo, 1986). As a consequence, trees have developed different strategies to cope with seasonal drought, such as drought-tolerance or drought-avoidance (van Ommen Kloeke et al., 2012), which are functional adaptations mainly related to stem hydraulic traits (Poorter et al., 2010). Examples of such adaptations include drought tolerant-evergreen species that typically have high wood density, low storage capacity in stems, and narrow vessels, all of which can limit hydraulic efficiency and increase resistance to drought-induced cavitation (Hacke et al., 2001; Allen et al., 2017). In contrast, species that follow a drought-avoidance strategy are highly deciduous trees with short-lived leaves, low wood density, high sapwood content, and wide vessels, that allow rapid water transport and storage (Poorter et al., 2019). However, they are also less resistant to the risk of xylem cavitation and are more susceptible to hydraulic failure due to drought (Brodribb et al., 2003; Meinzer et al., 2008; Markesteijn et al., 2011; Méndez-Alonzo et al., 2012; Allen et al., 2017). In addition, plant height and hydraulic functioning are known to represent a single coordinated axis of variation, primarily related to habitat water availability, with taller species from moist habitats exhibiting higher xylem efficiency and lower hydraulic safety, wider conduits, lower conduit density, and lower sapwood density (Liu et al., 2019).
The strategy for coping with seasonal drought stress can influence the spatial placement and interactions of pairs of species at the local scale, as different species may respond differently to fine-scale variation in soil moisture (Álvarez-Yépiz et al., 2017; Kupers et al., 2019). For example, we expect that large tree species adapted to sites with higher soil moisture may cluster in wetter areas and tend to outcompete other species in these areas, leading to patterns of negative spatial association. We also expect that positive facilitative interactions, considered particularly important under stressful conditions (Callaway, 1995; Maestre et al., 2009), may influence the spatial placement of pairs of species in TDFs (Espinosa et al., 2016; Álvarez-Yépiz et al., 2017; Gusmán-M et al., 2018). Facilitation is an interaction in which the presence of a given species alters the environment in a way that enhances growth, survival and reproduction of other species (Bronstein, 2009). Drought tolerant species that suffer less from drought deficit may improve soil water infiltration in their closer neighborhood, and plant hydraulic lift may also contribute to moister soils in the shade, leading to positive spatial associations with other species.
Assessing the spatial association pattern among species can therefore help us to better understanding the mechanisms that drive forest dynamics and assembly. One approach to do this is to use spatial point pattern analysis (Diggle, 2003; Illian et al., 2008; Wiegand and Moloney, 2014; Baddeley et al., 2015) to analyze the detailed smaller-scale patterns of trees in fully stem-mapped plots. Spatial patterns may conserve an imprint of past processes, and therefore constitute an ecological archive from which we may recover information about the underlying processes (Wiegand et al., 2003, 2009; Grimm et al., 2005; McIntire and Fajardo, 2009; Perea et al., 2021). Indeed, spatial patterns of tree species have provided valuable information to obtain insights on intra and inter-specific interactions as well as forest community structure (Stoll and Bergius, 2005; Wiegand et al., 2012), and spatial pattern analysis is a focus of current forest ecological research (Wiegand and Moloney, 2014; Velázquez et al., 2016; Ben-Said, 2021; Nguyen et al., 2021; Perea et al., 2021; Ribeiro et al., 2021; Wiegand et al., 2021). Techniques of spatial point pattern analysis have also been used to detect spatial association patterns and signatures of habitat association, facilitation and competition in TDFs (e.g., Espinosa et al., 2016; Álvarez-Yépiz et al., 2017; Gusmán-M et al., 2018). To quantify the spatial association pattern between species, spatial point pattern analysis determines for example the mean density of individuals of a species 2 within distance r of the individuals of a species 1 (Wiegand and Moloney, 2014). This results in three basic types of spatial association patterns, including positive ones if the mean density is larger than expected by the null model of independent placement, negative ones if the mean density is smaller than expected, and independence if it is within the range of stochastic realizations of the null model.
The spatial association patterns between species are generally influenced by habitat filtering, dispersal and species interactions (Vellend, 2010; Keil et al., 2021), where habitat filtering and species interactions can lead to similar spatial association patterns, albeit at different spatial scales (Wiegand et al., 2007a; Wiegand and Moloney, 2014; Ribeiro et al., 2021). Positive or negative spatial association patterns between two species can be caused by shared or opposed habitat requirements, respectively (Harms, 2001; Wiegand et al., 2007b; Allié et al., 2015). In the context of TDFs, a locally patchy distribution of soil moisture, e.g., driven by topography or soil at scales of say >30 m (Kupers et al., 2019), may lead to a sorting of species depending on their drought tolerance (Engelbrecht et al., 2007). A positive association of species to their preferred habitat can also be enhanced by dispersal limitation if seeds are transported mostly to nearby suitable sites (i.e., a positive fitness-density covariance; Chesson, 2009). Positive or negative spatial association pattern between two species can also be caused by positive facilitative interactions (McIntire and Fajardo, 2014; Chacón-Labella et al., 2017) or negative competitive interactions (Bolker and Pacala, 1999; Wiegand et al., 2012; Pescador et al., 2019), respectively. However, in contrast to habitat filtering that operates usually along environmental gradients (e.g., related to topographical features), species interactions operate usually within the immediate neighborhood of individuals (i.e., separation of scales; Wiegand et al., 2007b,2012; Wiegand and Moloney, 2014). Additionally, positive small-scale association, especially among saplings or among saplings and adults, can also be mediated by animal seed dispersal if animals deposit seeds of multiple species preferentially at specific microsites such as nests, roosts, perches or below larger individuals (Arnell et al., 2021; Perea et al., 2021).
Thus, determining the strength, the spatial scales and the direction of departures from the null expectation of independent placement can help to better understand the mechanisms structuring species assemblages in tropical forest (Wiegand et al., 2012), and spatial point pattern analysis (Wiegand and Moloney, 2014; Baddeley et al., 2015) provides a range of techniques for doing this. By taking advantage of separation of scales, point pattern analysis allows also to separate the effects of possible habitat associations and species interactions, given that the spatial range of biotic interactions is usually shorter than the scale of environmental autocorrelation (Diggle, 2003; Wiegand et al., 2007b,2012; Wiegand and Moloney, 2014; Keil et al., 2021). The results of an analysis of pairwise spatial associations among tree species of a forest community defines a weighted and directed network, where the species are the nodes and the strength and direction of the pairwise spatial associations between species defines the weights of the edges (Losapio, 2018; Perea et al., 2021). This allows us to identify key species, which are involved in many spatial associations, and by integrating the network into the trait space (e.g., spanned by hydraulic functional traits) we can gain insight on how species traits influence the observed spatial association patterns.
Our main objective was to characterize the interaction network (i.e., the strength and type of spatial association patterns) among eight dominant tree species in a fully stem-mapped plot of a TDF in Colombia. Our ultimate goal was to understand the observed spatial patterns and the mechanisms that determine the assembly and spatial structure of this forest community. We hypothesize that the abiotic deficit caused by seasonal water limitation leads to an interaction network in which a few “facilitator” species maintain consistent and positive small-scale associations with other species (Gusmán-M et al., 2018). To achieve these goals, we used techniques of point pattern analysis to study different aspects of the spatial placement of all 56 pairs of species. In a first analysis we determined for each species pair the strength and type of departures from the null model of independent placement, which are potentially caused by the effects of species interactions and/or habitat filtering. In a second analysis we determined selectively the strength of small-scale interactions between the 56 species pairs by controlling for medium- and larger-scale effects of the environment. Finally, we integrated the weighted and directed spatial association network of the first analysis into the trait space spanned by hydraulic functional traits to better understand how species traits influence the spatial structure of our study forest.
2. Materials and methods
2.1. Study area
Originally, TDFs covered about 90% of Colombia’s land area; today only 8% remains, of which roughly three quarters are secondary forests (Pizano and García, 2014; González-M et al., 2018). About 20% of the Colombian TDFs are covered by highly fragmented and successional vegetation (Pizano and García, 2014). Our TDF research plot is situated in the upper watershed region of the Magdalena River (5° 05′ 07″ N, 74° 46′ 29″ W, 335 m a.s.l), located in the northern part of the Tolima department, Colombia (see photos from the study site in Supplementary Appendix A). The region corresponds hosts second largest extent of TDFs in Colombia and is covered by a highly fragmented mosaic and successional vegetation (Pizano and García, 2014).
The study site was selected as part of the Colombian TDF socio-ecological monitoring platform (Norden et al., 2020), led by the Instituto Humboldt, Colombia, in conjunction with several other institutions. According to the classification of Espinal (1977), the regional climate characterized by a hot and dry season, which leads to water deficiency (Espinal, 1977). Mean annual temperature is 26.8°C with a maximum monthly mean of 29.8°C from July to September (Santoro, 2002 – Report). The rainfall regime is characterized by a dry and a wet period that receive on average 831 and 2268 mm per annum, respectively (González-M et al., 2021). During the dry season, which lasts from June to September (Santoro, 2002 – Report), more than 50% of all tree species drop their leaves. Extensive pastures for livestock, crop cultivation, and ground fires, which historically dominated the disturbance regime in this region, have reduced the forest extension to small and isolated patches. Most of today’s forest fragments in our study region remain in hilly areas, with an average size of 155 ha (Pizano and García, 2014), and belong mostly to private farms.
2.2. Vegetation sampling
We used a one-hectare (100 m × 100 m) permanent plot established in a mature forest of the Magdalena River valley region. Within the plot, 10 m × 10 m sub-plot were defined to facilitate the plant census. All trees (woody stems ≥2.5 cm diameter at breast height, 1.3 m above ground –dbh) were mapped and measured for height and dbh using a diameter tape. For mapping we recording the distance and azimuth to each tree from the southwest corner of every 10 m × 10 m sub-plot using a hypsometer and sighting compass. The final spatial locations (x-and y-coordinates) of tacked individuals (Figure 1) were determined following a standard field protocol of the Forest Dynamic Plots of the Center for Tropical Forest Science (Condit et al., 2014). Because vegetative propagation by sprouting is common for most species, individual ramets growing from the same base were considered as single individual and their diameters were averaged.
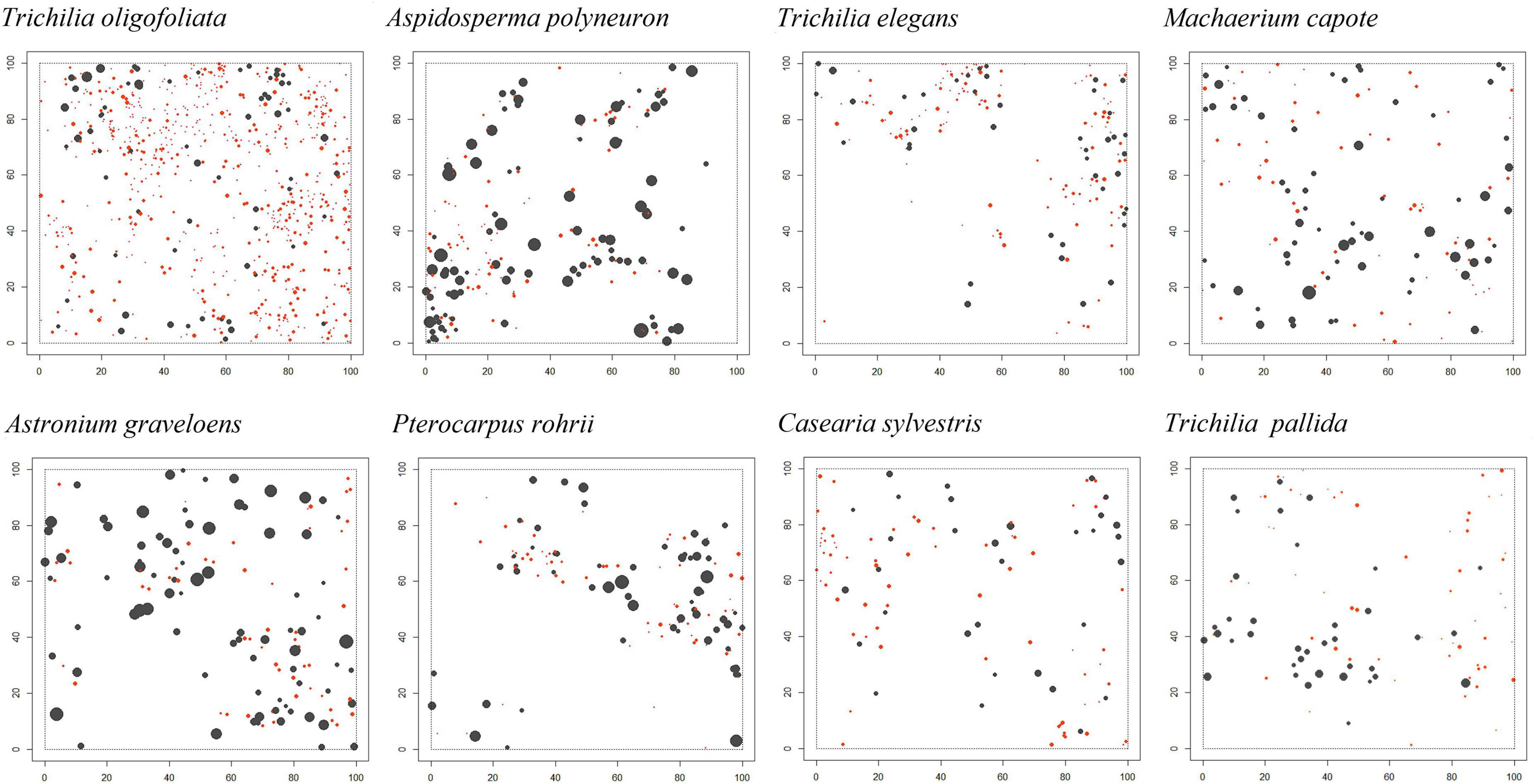
Figure 1. Spatial distribution map of individual trees of the 8 study species in the 1-ha forest plot. Black disks, large trees (≥10 cm dbh; matures); Red disks; small trees (≤10 cm dbh; saplings). The size of the disks is proportional to the dbh of the trees. See Table 1 for population and structural characteristics.
2.3. Study species
In total, 3,543 individuals were located in the study plot (Supplementary Table B1 in Supplementary Appendix B). The plot included 39 plant species that are classified at some threat category in the IUCN red list (Pizano and García, 2014), eight of them are considered Critically Endangered, Endangered or Vulnerable. The region also hosts a large number of endemic plants (Pizano and García, 2014), and 14 endemic species are encountered in our plot. We divided all individual trees into two size classes, large (diameter at breast height, dbh ≥ 10 cm; assumed to be matures) and small (dbh 2.5 to <10 cm; assumed to be saplings). Given that we aim to describe the spatial structure of the dominant species, we used only tree species with more than 35 large sized-individuals (excluding shrubs) for our spatial analysis (Figure 1, Table 1 and Supplementary Table B1 in Supplementary Appendix B). This threshold provides also sufficient statistical power for our analysis and resulted in the inclusion of eight tree species.
2.4. Point-pattern analysis
2.4.1. Summary functions
Our goal was to quantify different aspects of the spatial placement of pairs of species. For this purpose we used summary functions of spatial point pattern analysis (Wiegand and Moloney, 2014) that quantify how individuals of a species 2 are distributed within the neighborhoods (with radius r) of trees of a focal species 1. We used for this purpose three summary functions that measure different aspects of the spatial placement of pairs of species. Ripley’s (2004) K function is based on counting all neighbors of species 2 within distance r of the focal individual of species 1, the pair correlation function is based on counting all neighbors of species 2 within a ring of radius r and width dr of the focal individual of species 1, and the distribution function of the nearest neighbor distances determines for each individual of species 1 the distance to its nearest neighbor of species 2 (Wiegand et al., 2013).
The bivariate K function K12(r) can be estimated as the mean number of trees of species 2 within distance r of the trees of the focal pattern 1, divided by the intensity λ2 of species 2 in the study area (i.e., the number of trees of species 2 divided by area) (Wiegand and Moloney, 2004). If the two species are independently placed we have K12(r) = π r2, if the two species show a positive spatial association we have K12(r) > π r2, and if the two species show a negative spatial association we have K12(r) < π r2.
The bivariate pair correlation function g12(r), which is the non-cumulative version of the K-function, can be estimated as the mean density of individuals of species 2 within distance interval (r−dr, d + dr) of the individuals of species 1, divided by λ2. Positive spatial association occurs for g12(r) > 1 (larger than expected neighborhood density) and negative spatial association for g12(r) < 1 (smaller than expected neighborhood density). Pair correlation functions are especially suitable to isolate effects of specific distances r, for example for studying the effects of species interactions, whereas K-functions are cumulative measures that confounds effects at larger distances with effects at shorter distances (Wiegand and Moloney, 2004).
The bivariate distribution function D12(r) of the nearest neighbor distances complements the K- and g-functions (Wiegand et al., 2012, 2013). It can be estimated as the proportion of trees of the focal species 1 that have their nearest species 2 neighbor within distance r (Diggle, 2003; Illian et al., 2008). While K- or pair correlation functions describe mean neighborhood densities, the D12(r) captures aspects of the variability in the neighborhood densities (Wiegand et al., 2013). For example, D12(r) and K12(r) will show contrasting results if a certain proportion of individuals of species 1 have no neighbor of species 2 within distance r, but others many. However, their results will agree if all individuals of species 1 have more or less the same number of neighbors of species 2.
2.4.2. Null models
2.4.2.1. Overall spatial association network
To determine the strength and direction of the spatial association patterns among our eight study species we compared the summary functions K12(r) and D12(r) of our observed patterns to that of a stochastic realizations of a null model of independent placement. We used for this purpose the toroidal shift null model (Lotwick and Silverman, 1982; Wiegand and Moloney, 2004) that keeps the spatial pattern of each species unchanged, but breaks their potential association. This is accomplished by adding a constant vector (xT, yT) to the coordinates of all individual of species 2 (but randomly drawn for each realization of the null model), and if individuals of species 2 would be displaced to locations outside the study window, they are wrapped following torus geometry. Note that departures from the toroidal shift null model can be caused by species interactions or by habitat filtering.
2.4.2.2. Small-scale interactions
To study the “pure” effects of small-scale species interactions we used a heterogeneous Poisson (HP) process with non-parametric intensity estimate as null model (Wiegand et al., 2007b; Wiegand and Moloney, 2014). This null model takes advantage of separation of scales by assuming that small-scale interactions occur at distances r < R and the environment varies mostly at distances r > R. In practice, this null model displaces each individual of species 2 to a random location within radius R, which should be selected slightly larger than the maximal range of small-scale interactions (Wiegand and Moloney, 2014). This null model therefore keeps potential habitat associations by moving the individuals to nearby locations (showing a similar environment), while removing the signatures of possible small-scale interactions at distances below R. As a result, it will selectively detect departures due to small-scale species interactions (or small-scale edaphic environmental conditions) (Wiegand and Moloney, 2014). We used a maximal displacement distance of R = 30 m.
2.4.3. Assessment of significant departures from the null models
To assess the significance of departures from a null model, we used standardized effect sizes and global envelopes with significance level of α = 0.05 (Velázquez et al., 2016; Wiegand et al., 2016; Chanthorn et al., 2018). The global envelopes indicate for each distance r the range of the summary function f12(r) that is, for a prescribed significance level α, compatible with the null model, and if the observed summary function is outside the global envelopes we have a significant departure with significance level of α. To obtain global envelopes, we calculated in a first step the standardized effect sizes SES(r) as:
where f12(r) stands for the observed g12(r), D12(r), or K12(r). The operators E[.] and SD[.] indicate the expectation and standard deviation of the summary statistics of the null model realizations at distance r, respectively. Standardized effect sizes indicate significant departures from the null model with α = 0.05 (for one summary function and a fixed distance r) if SES(r) < –1.96 or SES(r) > 1.96, which defines a critical value G of by 1.96.
To assess significant departures based on an entire distant interval we need to use global envelopes. This is because we conduct several tests simultaneously (one for each distance bin) (Wiegand et al., 2016). To account for this, global envelopes use a critical value G larger than 1.96 that is adjusted to lead to the convenient property that the null model can be rejected with the prescribed significance level α if the observed SES(r) is for at least one distance r smaller than -G or larger than G (Wiegand et al., 2016). By back-transforming for SES(r) = -G and SES(r) = G we obtain the lower and upper global simulation envelopes E–(r) and E+(r), respectively (Wiegand et al., 2016):
2.4.4. Determining the strength and direction of spatial associations in the network
To obtain a more complete characterization of the spatial association pattern between species (our first analysis), we used the standardized effect sizes of the two summary functions D12(r) and K12(r) (for a given distance r). To account for the multiple testing with two summary functions we used a significance level α≈ 0.025 (instead of α = 0.05 for a single summary function), which results in a critical value of G = 2.33 (instead of 1.96; Getzin et al., 2014).
We allocated each species pair within a two-dimensional classification space spanned by the x-axis P(r) = SES[D12(r)] and the y-axis M(r) = SES[K12(r)] (Wiegand et al., 2012; Wiegand and Moloney, 2014). The scheme allows for a more subtle description of the spatial association patterns than the positive—independent—negative association classification that results from using only one summary function. Apart from independence, where the null model cannot be rejected (the species is located within a box delimited by x- and y-values of −2.33, 2.33), the scheme allows for two types of positive spatial associations and two types of negative spatial associations (see Figure 3). When trees of species 2 occur at consistently lower densities than expected in the neighborhoods of trees of species 1 (i.e., P(r) < 0 and M(r) < 0) we call this negative spatial association “segregation” (the lower left quadrant of the scheme except the independence square). When trees of species 2 occur at consistently higher densities around trees of species 1 (P(r) > 0 and M(r)) > 0, we call this positive spatial association “mixing.” Finally, if spatial association patterns are heterogeneous, we may also observe cases were the mean density of trees of species 2 around trees of species 1 is higher than expected [M(r) > 0], but a notable proportion of trees of species 1 have less than expected nearest neighbors of species 2 [P(r) < 0] (“partial overlap”). A forth type (M(r) < 0; P(r) > 0) does usually not occur (Wiegand and Moloney, 2014). The strength of departures from the null model can be quantified by the length of the vector (P(r), M(r)) in the two-dimensional scheme.
We derived the interaction network for all neighborhood distances r ≤ 50 m, but used two representative distance(s) for further analyses. All analyses were performed using the Programita software package (Wiegand and Moloney, 2014) that can be requested at http://programita.org/.
2.4.5. Species traits and spatial associations
We hypothesized that the response of the species to drought stress would contribute to the spatial association patterns between species (Álvarez-Yépiz et al., 2017). To quantify such potential relationships, we conducted a principal component analysis (PCA) of eight traits, based on the 15 most abundant species (83% of the total abundance) in our plot. We broadened the PCA analysis by including seven additional species to increase the amount of differentiation among species within the plot. If more species are included into the analysis, a clearer PCA pattern should emerge (Jolliffe and Cadima, 2016) through functional dimension between traits, which could improve our inference about the underlying processes. The eight traits we used are related to different strategies to cope with drought stress and include water content (WC), vessel density (VD), vessel area (VA), specific leaf area (SLA), wood density (WD), water content–fiber saturation point (WCfsp), maximum height of tree (MH), and xylem potential hydraulic conductivity (Kh). The first two components of the PCA were used as coordinates to allocate the tree species to interpret their spatial association patterns. The PCA analysis were conducted on the standardized traits applying the prcomp() function of the factoextra package using R. All trait measurements were conducted during a short period in 2017 and were measured from the same forest stand by Instituto Humboldt, following established standard protocols (Carmona et al., 2015).
3. Results
The eight most abundant tree species (i.e., Trichilia oligofoliata, Aspidosperma polyneuron, Trichilia elegans, Machaerium capote, Astronium graveolens, Pterocarpus rohrii, Casearia sylvestris, and Trichilia pallida) accounted for 55% of the total number of tacked individuals in the plot (Table 1), and their abundance ranged between 100 individuals (T. pallida) to 750 individuals (T. oligofoliata). Size distributions based on saplings and mature individuals of M. capote, P. rohrii, C. sylvestris, T. pallida, and A. graveolens showed little skew (i.e., they comprised more individuals of large size), whereas the size distribution of the rest of the species were skewed i.e., they comprised many saplings (see Supplementary Figure B1 in Supplementary Appendix B).
The results from the PCA analyses of functional traits are summarized in the supporting information (see Supplementary Tables C1, C2 in Supplementary Appendix C). The first axis of the PCA of hydraulic functional traits accounted for 44.8% of the variance and was basically related to the water acquisition strategy with the acquisitive strategy “drought avoidance” having negative values and the conservative strategy “drought tolerant” positive values. The second axis accounted for additional 19% of the variance and was related to the stem-leaf spectrum, mostly driven by maximum tree size with larger trees having increasingly larger values.
3.1. Interaction network of spatial association patterns between species
Figure 2 shows how the types of spatial association patterns changed with spatial scale. The most notable result is that the interaction network was dominated by independent placement, accounting for more than 80% of all associations depending on spatial scale r (Figure 2). Apart from the independent type, we found at scales smaller than 13 m mostly spatial associations of the mixing type (a positive association; Figure 3A) and segregation (a negative association) peaked at 14–16 m (Figure 3B), whereas partial overlap and the type IV associations, which characterize heterogeneous spatial associations, could be neglected (Figures 2, 3; see also Supplementary Table D1 in Supplementary Appendix D, for the association type for all distances r). Significant departures almost disappeared at 25 m neighborhoods (Figure 2).
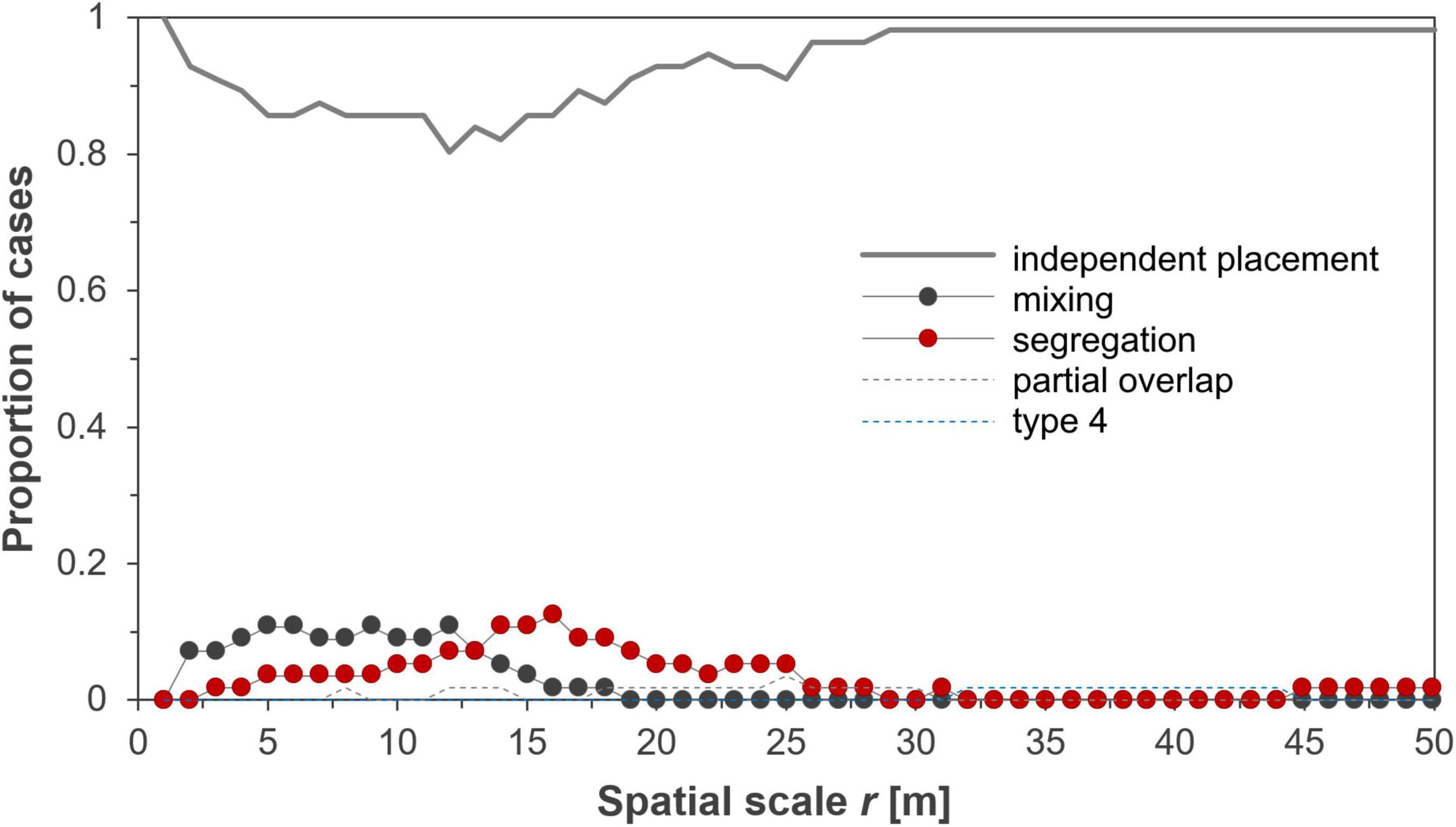
Figure 2. Changes in the network of spatial association patterns among the 8 dominant species in dependence on spatial scale. Shown is the proportion of the 56 species pairs that were categorized into one of the five spatial association types at a given spatial scale r. The gray line indicates the independence type; red solid circles segregation (type 1); black solid circles mixing (type 3); the dashed gray line indicates partial overlap (type 2); and the dashed blue line type 4.
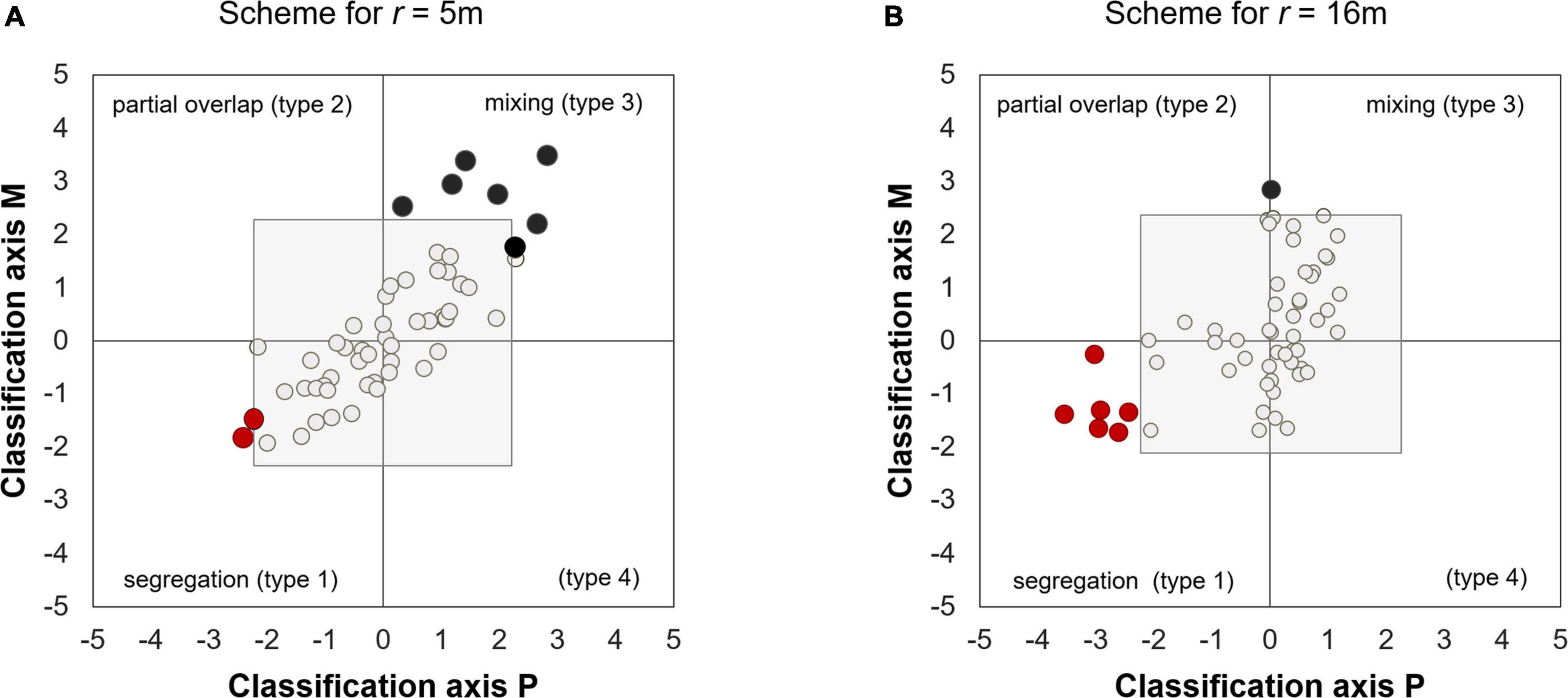
Figure 3. Strength and direction of spatial association patterns among the 8 study species shown by the two dimensional classification scheme. (A,B) Shows the allocation of the 56 species pairs at small (5 m) and medium (16 m), respectively based on the P–M classification axes. Black and red solid circles represents species pairs showing mixing and segregation, respectively. The gray box indicates the area of independent placement. The scheme is spanned by the standardized effect sizes of the nearest neighbor distribution function D12(r) (P-axis) and the K-function K12(r) (M-axis).
Allocating the network of significant spatial association pattern between the 8 study species into the trait space spanned by the first two PCA axes showed that positive spatial association patterns (green arrows in Figure 4) occur mostly among species of similar (small) values of PCA2, but different values of PCA1. The species T. oligofoliata (To), the most extreme species at the PCA1 axis and an intermediate value of PCA2 played a key role in the mixing association at the 5 m scale (Figure 4A). It was involved symmetrically into 6 of the 7 positive associations. Interestingly, negative associations at both scales (red arrows) involved always the species A. polyneuron (Ap), which shows the largest value of PCA2 and an intermediate value of PCA1 (Figure 4). Six of the seven positive associations at the 5 m scale disappeared at the 16 m scale, but 5 of them showed still a non-significant tendency to positive associations (light green arrows in Figure 4B). Our eight study species were all intermediate to larger sized species with positive values of the second axis (Table 1 and Figure 4).
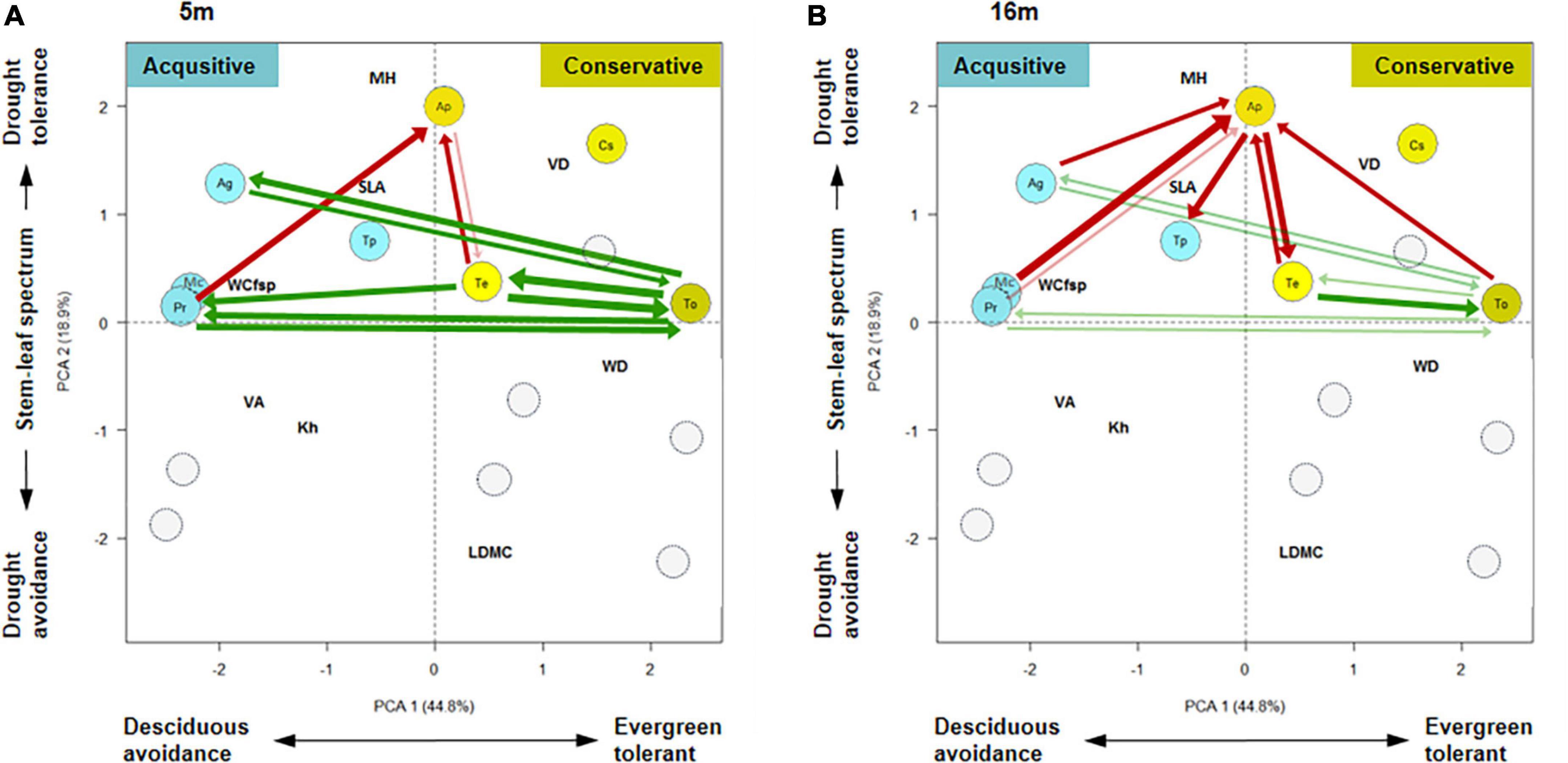
Figure 4. Allocation of significant spatial species associations within the space spanned by the first two axes of the trait space. (A,B) Shows species associations at small (5 m) and medium (16) scales, respectively. The width of the arrows is proportional to the strength of the association. Red arrows indicate significant and negative spatial species associations of the segregation type, and green arrows significant and positive ones of the mixing type. The arrows of the species pairs go from the focal species to the second species, and species pairs with two arrows are symmetric. Transparent arrows (light green or red) are just non-significant with an association strength > 2. Gray transparent circles shows the allocation of other 7 species that were not included in this analysis because they had less than 35 large individuals.
3.2. Small-scale species interactions
The global envelope test of the HP process, which factored out the effect of habitat filtering, detected significant departure for eight species pair combinations (14% of all cases), but only 6 symmetric pairs showed interpretable departures (see Supplementary Table D2 in Supplementary Appendix D, for the results for all species pairs and distances r). The pairs T. oligofoliata vs. T. elegans and T. oligofoliata vs. P. rohrii, showed positive interactions and the pair A. polyneuron vs. P. rohrii negative interactions (Figures 5A–C, respectively). Interestingly, the two species pairs with positive interactions showed also shared habitat associations, as indicated by expected g12(r) functions above the value of 1 (gray lines in Figures 5A, B), and the species pair with weak negative interactions already showed opposed habitat associations as indicated by an expected g12(r) function below the value of 1 (Figure 5C).
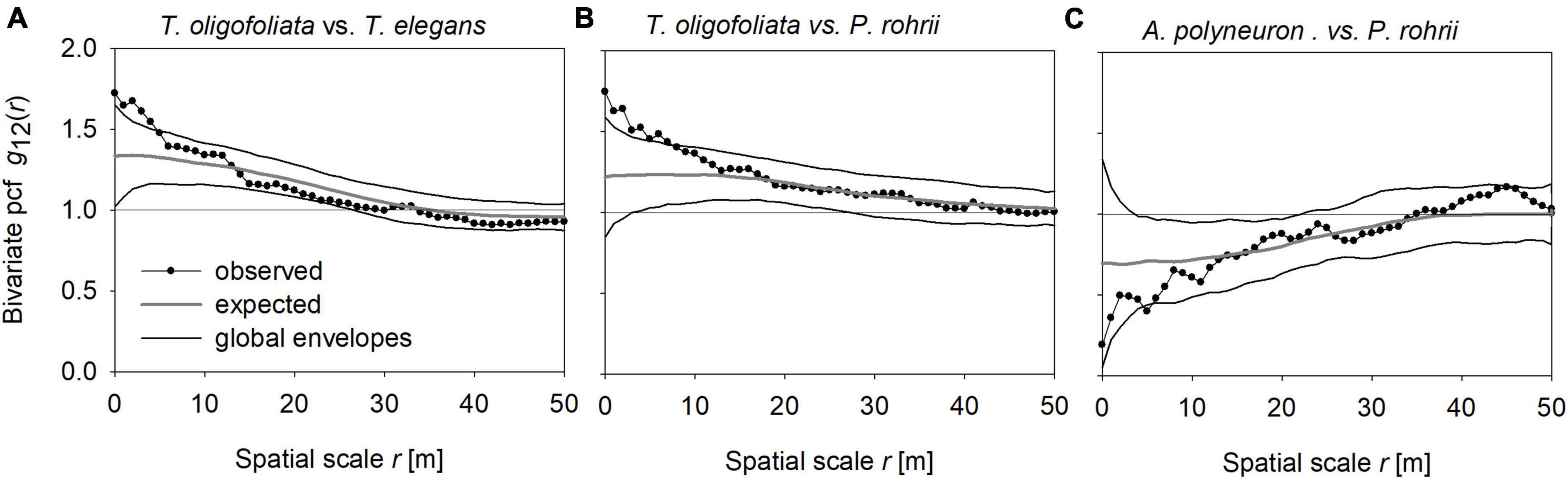
Figure 5. Examples of significant small-scale species interactions: (A) T. oligofoliata vs. T. elegans; (B) T. oligofoliata vs. P. rohrii; (C) A. polyneuron vs. P. rohrii. Shown are the observed pair-correlation function g12(r) in dependence of spatial scale r (dotted line), the global simulation envelopes (solid lines) and the expected g function of the heterogeneous Poisson process null model (gray line; average of the simulation of null-model). The horizontal lines give the expected g12(r) = 1 for no species interactions. The heterogeneous Poisson point process used with a bandwidth R = 30 m, and the ring width dr for the estimation of the pair-correlation function was 3 m.
4. Discussion
In this study, we performed a detailed analysis of the network of spatial association patterns among 8 dominant species in a TDF community in Colombia. More than 80% of the species pairs did not show significant spatial association patterns, and we found prevalence of positive associations at scales smaller than 13 m and prevalence of negative associations at intermediate scales that peaked at 14–16 m. We also identified two key species of the spatial association network: T. oligofoliata was involved in most positive associations at the small scales, and A. polyneuron in negative associations, mostly at intermediate scale. The two key species represent two extreme positions in the hydraulic functional trait space, and positive spatial associations tended to occur among species differing in their hydraulic spectrum, but negative associations among species differing in their stem hydraulic properties (mostly maximal size). An analysis to selectively detect effects of small-scale species interactions (by factoring our potential effects of habitat filtering) revealed that positive interactions were involved in most of the positive spatial association pattern at small scales.
4.1. Interpretations of the network of spatial association patterns
In stressful environments it is frequently assumed that positive interactions would outweigh negative interactions and influence species coexistence (Espinosa et al., 2013, 2016; McIntire and Fajardo, 2014; Gusmán-M et al., 2018). For example, Gusmán-M et al. (2018) found that 11 out of 20 species in a TDF of southern Ecuador showed evidence for facilitation, and in a TDF of northwestern Mexico evergreen species (cycad-palm) were positively associated with each other (Álvarez-Yépiz et al., 2017). Our analysis of spatial data of a dry tropical forest revealed that positive and negative associations occurred only for a relatively small proportion of species pairs, in which the two most abundant species at our plot were involved, thus determining the spatial association structure of the forest to a large extend. However, the majority of our 8 study species showed spatial association to other species (Figure 4).
The species A. polyneuron, a slow-growing, emergent (20–30 m), long-lived, shade-tolerant and evergreen tree with relatively high wood density (Lisi et al., 2008) was the key species for negative species associations at the 16 m scale. Figure 1 and Supplementary Figures B1, B2 in Supplementary Appendix B, shows that A. polyneuron comprises many large trees that are strongly spatially aggregated at distances below 10 m (Supplementary Figures E1–E4 in Supplementary Appendix E). The canopies of large trees of this species form therefore patches that reach typically some 15 m from their stems (Figure 1), which may exert strong negative competitive interactions to other species. The large height of A. polyneuron trees distinguishes them from the other species, both in competitive abilities and in their response to the microenvironment, where the generally taller A. polyneuron trees benefited most. Note that the maximal height drives the second axis of the PCA (i.e., MH in Figures 4A, B and Supplementary Table C1 in Supplementary Appendix C). The strong competitive ability of A. polyneuron at intermediate spatial scales and its ability to partially tolerate drought suggests that it uses its spatial arrangement to exploit relatively resource-rich microenvironments and edaphic factors (e.g., drainage) with high water availability, as suggested in previous studies in TDFs (Méndez-Toribio et al., 2017). The topography of our site is diverse, and ranges from undulating surface to a gently rolling slope (Supplementary Figure F1 in Supplementary Appendix F), covering three sides of the plot. Álvarez-Yépiz et al. (2017) found that evergreen and deciduous species segregated spatially in a TDF of northwestern Mexico, particularly at fine-scales, which reflects their reduced niche overlap due to their different resource-use strategies.
Trichilia oligofoliata, a hydraulically safe evergreen species with costly tissue was the key species for positive small-scale spatial associations. It is an abundant short-stature and mid-story tree species with a dense umbrella crown which can growth up to 20 m in height. Figure 1 and Supplementary Figures B1, B2 in Supplementary Appendix B, shows that T. oligofoliata comprises mostly small trees and only a few medium size trees. Interestingly, the positive small-scale interactions of T. oligofoliata to the two species T. elegans and P. rohrii were driven by the small individuals of T. elegans and P. rohrii (but not by large individuals) (Supplementary Figures G1–G3 in Supplementary Appendix G). In this forest stand, T. oligofoliata is a colonizing species (pers. obs.), explosively dispersed by birds. Thus, T. oligofoliata saplings could act spatially as a nuclei, favoring the colonization and establishment of T. elegans and P. rohrii individuals through the enhancement of microhabitat conditions leading to higher soil wetness (Soliveres et al., 2011; Espinosa et al., 2013). The shared animal seed dispersal mode with T. oligofoliata and T. elegans may additionally promote the joined placement of seeds (Pausas et al., 2006). In contrast, a nurse effect of T. oligofoliata trees may provide a moderately favorable microhabitat for the establishment of the wind-dispersed tree P. rohrii, thereby contributing to its spatial distribution structure. Finally, the lack of positive associations of saplings of T. oligofoliata with individuals of A. graveolens, the third species with strong positive spatial associations (Supplementary Figure G2 in Supplementary Appendix G), suggests that these two species show a shared medium to larger-scale habitat associations.
Besides of existence of the two key species that were involved into most spatial species associations, the predominant association type in this forest was independent placement that occurred in the large majority of the species pairs at all spatial scales. This finding is in agreement with spatial analyses in species rich forests and shrublands (e.g., Wiegand et al., 2007a,b; Perry et al., 2014; Punchi-Manage et al., 2015; Wang et al., 2016; Chacón-Labella et al., 2017), an analysis in a TDF (Espinosa et al., 2016), and the assumption of independent placement of species in many biodiversity theories (McGill, 2010). Independence may also occur due to statistical reasons that hinder detection of potential associations because individuals of the two rare species will occur rarely close to each other (Wiegand et al., 2007b). Additionally, the placement of two species is conditioned by other species which are not accounted for in pairwise analyses, and high stochasticity in the species composition in the neighborhood of individuals of a given species may blur patterns generated by facilitative or competitive interactions and may not allow for emergence of directed and detectable pairwise species associations (Wang et al., 2016).
Nevertheless, our results should be considered as a first approximation, because we do not have replicates (located in close proximity) to test these assertions. Similarly, because of the limited number of species (8) analyzed here, albeit the most abundant ones, we caution its representativeness in shaping the assembly of the full community.
5. Conclusion
In this study we analyzed the spatial association patterns between eight dominant species in a TDF stand. We found that two key species structure the species associations, a small-stature drought-tolerant pioneer was positively associated at the small scale with several other species, whereas an emergent (20–30 m) long-lived shade-tolerant species was mainly involved in negative associations. These two key species followed the same extreme strategy of drought tolerance, as shown by the visualization of the spatial species associations in the trait space (Figure 4). This is an interesting pattern not unexpected for a forest community subject to seasonal drought stress, which requires further investigations, especially with respect to interactions with other pattern generating processes such as vegetative propagation, animal seed dispersal and microhabitat heterogeneity. However, the majority of all species pairs were characterized by patterns of independent placement. In light of these characteristics, our results suggest the need of future experiments designed to examine the possibility that other environmental factors (e.g., topographic attributes) may exert an effect on tree community assembly.
Data availability statement
The original contributions presented in this study are included in the article/Supplementary material, further inquiries can be directed to the corresponding author.
Author contributions
MS: conceptualization, methodology, software, validation, formal analysis, investigation, resources, organization of data collection, writing—original draft, visualization, and funding acquisition. TW: conceptualization, software, validation, writing—review and editing, and supervision. RG-M: validation, investigation, and writing—original draft. SR-B: writing—original draft. MQ: reviewing and writing. EC: conceptualization, investigation, and supervision. All authors contributed to the article and approved the submitted version.
Funding
This publication was funded by the joint publication fund of the TU Dresden, the Medical Faculty Carl Gustav Carus, and the SLUB Dresden.
Acknowledgments
We would like to express our gratitude to the Alexander von Humboldt Biological Resources Research Institute for the forest field data collection-provision and to Colciencias for funding the Ph.D. of MS to the research study at TU Dresden. We highly appreciate the constructive comments of the two reviewers and the handling editor for useful comments and suggestions, which very much improved the manuscript.
Conflict of interest
The authors declare that the research was conducted in the absence of any commercial or financial relationships that could be construed as a potential conflict of interest.
Publisher’s note
All claims expressed in this article are solely those of the authors and do not necessarily represent those of their affiliated organizations, or those of the publisher, the editors and the reviewers. Any product that may be evaluated in this article, or claim that may be made by its manufacturer, is not guaranteed or endorsed by the publisher.
Supplementary material
The Supplementary Material for this article can be found online at: https://www.frontiersin.org/articles/10.3389/ffgc.2023.1028515/full#supplementary-material
References
Allen, K., Dupuy, J. M., Gei, M. G., Hulshof, C., Medvigy, D., and Pizano, C. (2017). Will seasonally dry tropical forests be sensitive or resistant to future changes in rainfall regimes? Environ. Res. Lett. 12:23001. doi: 10.1088/1748-9326/aa5968
Allié, E., Pélissier, R., Engel, J., Petronelli, P., Freycon, V., and Deblauwe, V. (2015). Pervasive local-scale tree-soil habitat association in a tropical forest community. PLoS One 10:e0141488. doi: 10.1371/journal.pone.0141488
Álvarez-Yépiz, J. C., Búrquez, A., Martínez-Yrízar, A., Teece, M., Yépez, E. A., and Dovciak, M. (2017). Resource partitioning by evergreen and deciduous species in a tropical dry forest. Oecologia 183, 607–618. doi: 10.1007/s00442-016-3790-3
Arnell, M., Ehrlén, J., and Eriksson, O. (2021). Local distribution patterns of fleshy-fruited woody plants – testing the orchard hypothesis. Ecography 44, 481–492. doi: 10.1111/ecog.05359
Baddeley, A., Rubak, E., and Turner, R. (2015). Spatial Point Patterns: Methodology and Applications With R. Boca Raton, FL: Chapman and Hall/CRC. doi: 10.1201/b19708
Becknell, J. M., Kucek, L. K., and Powers, J. S. (2012). Aboveground biomass in mature and secondary seasonally dry tropical forests. A literature review and global synthesis. For. Ecol. Manag. 276, 88–95. doi: 10.1016/j.foreco.2012.03.033
Ben-Said, M. (2021). Spatial point-pattern analysis as a powerful tool in identifying pattern-process relationships in plant ecology: an updated review. Ecol. Process. 10:56. doi: 10.1186/s13717-021-00314-4
Bolker, B. M., and Pacala, S. W. (1999). Spatial moment equations for plant competition. understanding spatial strategies and the advantages of short dispersal. Am. Nat. 153, 575–602. doi: 10.1086/303199
Brodribb, T. J., Holbrook, N. M., Edwards, E. J., and Gutiérrez, M. V. (2003). Relations between stomatal closure, leaf turgor and xylem vulnerability in eight tropical dry forest trees. Plant Cell Environ. 26, 443–450. doi: 10.1046/j.1365-3040.2003.00975.x
Bronstein, J. L. (2009). The evolution of facilitation and mutualism. J. Ecol. 97, 1160–1170. doi: 10.1111/j.1365-2745.2009.01566.x
Callaway, R. M. (1995). Positive interactions among plants. Bot. Rev. 61, 306–349. doi: 10.1007/BF02912621
Calvo-Rodriguez, S., Sanchez-Azofeifa, G. A., Durán, S. M., Do, M. M., and Nunes, Y. R. F. (2021). Dynamics of carbon accumulation in tropical dry forests under climate change extremes. Forests 12:106. doi: 10.3390/f12010106
Carmona, C. P., Rota, C., Azcárate, F. M., and Peco, B. (2015). More for less: sampling strategies of plant functional traits across local environmental gradients. Funct. Ecol. 29, 579–588. doi: 10.1111/1365-2435.12366
Chacón-Labella, J., de la Cruz, M., and de la Cruz, A. (2017). Evidence for a stochastic geometry of biodiversity. The effects of species abundance, richness and intraspecific clustering. J. Ecol. 105, 382–390. doi: 10.1111/1365-2745.12710
Chanthorn, W., Wiegand, T., Getzin, S., Brockelman, W. Y., and Nathalang, A. (2018). Spatial patterns of local species richness reveal importance of frugivores for tropical forest diversity. J. Ecol. 106, 925–935. doi: 10.1111/1365-2745.12886
Chesson, P. (2009). Scale transition theory with special reference to species coexistence in a variable environment. J. Biol. Dyn. 3, 149–163. doi: 10.1080/17513750802585491
Choler, P., Michalet, R., and Callaway, R. M. (2001). Facilitation and competition on gradients in alpine plant communities. Ecology 82, 3295–3308. doi: 10.1890/0012-9658(2001)082[3295:FACOGI]2.0.CO;2
Condit, R., Lao, S., Singh, A., Esufali, S., and Dolins, S. (2014). Data and database standards for permanent forest plots in a global network. For. Ecol. Manag. 316, 21–31. doi: 10.1016/j.foreco.2013.09.011
Djoudi, H., Vergles, E., Blackie, R., Koffi Koame, C., and Gautier, D. (2015). Dry forests, livelihoods and poverty alleviation. Understanding current trends. Int. For. Rev. 17, 54–69. doi: 10.1505/146554815815834868
Engelbrecht, B. M. J., Comita, L. S., Condit, R., Kursar, T. A., Tyree, M. T., and Turner, B. L. (2007). Drought sensitivity shapes species distribution patterns in tropical forests. Nature 447, 80–82. doi: 10.1038/nature05747
Espinal, S. (1977). Zonas de Vida o Formaciones Vegetales de Colombia. Memoria Explicativa sobre el Mapa Ecologico. Vol. XIII, no. 11. (+ maps, scale 1. 500,000). Instituto Geografico “Agustin Codazzi”.
Espinosa, C. I., de la Cruz, M., Jara-Guerrero, A., Gusmán, E., and Escudero, A. (2016). The effects of individual tree species on species diversity in a tropical dry forest change throughout ontogeny. Ecography 39, 329–337. doi: 10.1111/ecog.01328
Espinosa, C. I., Luzuriaga, A. L., de la Cruz, M., Montero, M., and Escudero, A. (2013). Co-occurring grazing and climate stressors have different effects on the total seed bank when compared to the persistent seed bank. J. Veg. Sci. 24, 1098–1107. doi: 10.1111/jvs.12043
Fernández-Méndez, F. (2014). “Status of knowledge, conservation, and management of tropical dry forest in the magdalena river valley, Colombia,” in Tropical Dry Forests in the Americas: Ecology, Conservation, and Management, eds F. Sánchez-Azofeifa and J. S. Powers (Boca Raton, FL: CRC Press Taylor & Francis Group), 35–54.
Getzin, S., Wiegand, T., and Hubbell, S. P. (2014). Stochastically driven adult-recruit associations of tree species on Barro Colorado Island. Proc. R. Soc. B 281:20140922. doi: 10.1098/rspb.2014.0922
González-M, R., García, H., Isaacs, P., Cuadros, H., López-Camacho, R., and Rodríguez, N. (2018). Disentangling the environmental heterogeneity, floristic distinctiveness and current threats of tropical dry forests in Colombia. Environ. Res. Lett. 13:45007. doi: 10.1088/1748-9326/aaad74
González-M, R., Posada, M. J., Carmona, C. P., Garzón, P., Salinas, V., Idárraga-Piedrahita, A., et al. (2021). Diverging functional strategies but high sensitivity to an extreme drought 601 in tropical dry forests. Ecol. Lett. 24, 451–463.
Grimm, V., Revilla, E., Berger, U., Jeltsch, F., Mooij, W. M., Railsback, S. F., et al. (2005). Pattern-oriented modeling of agent-based complex systems. Lessons from ecology. Science 310, 987–991. doi: 10.1126/science.1116681
Gusmán-M, E., de la Cruz, M., Espinosa, C. I., and Escudero, A. (2018). Focusing on individual species reveals the specific nature of assembly mechanisms in a tropical dry-forest. Perspect. Plant Ecol. Evol. Syst. 34, 94–101. doi: 10.1016/j.ppees.2018.07.004
Hacke, U. G., Sperry, J. S., Pockman, W. T., Davis, S. D., and McCulloh, K. A. (2001). Trends in wood density and structure are linked to prevention of xylem implosion by negative pressure. Oecologia 126, 457–461. doi: 10.1007/s004420100628
Harms, K. E. (2001). Habitat associations of trees and shrubs in a 50- ha neotropical forest plot. J. Ecol. 89, 947–959. doi: 10.1111/j.1365-2745.2001.00615.x
Illian, J., Penntinen, A., Stoyan, H., and Stoyan, D. (2008). Statistical Analysis and Modelling of Spatial Point Patterns. New York, NY: John Wiley. doi: 10.1002/9780470725160
Jolliffe, I. T., and Cadima, J. (2016). Principal component analysis. A review and recent developments. Philos. Trans. R. Soc. A 374:20150202. doi: 10.1098/rsta.2015.0202
Keil, P., Wiegand, T., Tóth, A. B., McGlinn, D. J., and Chase, J. M. (2021). Measurement and analysis of interspecific spatial associations as a facet of biodiversity. Ecol. Monogr. 91:e01452. doi: 10.1002/ecm.1452
Kupers, S. J., Wirth, C., Engelbrecht, B. M. J., and Rüger, N. (2019). Dry season soil water potential maps of a 50 hectare tropical forest plot on Barro Colorado Island. Panama. Sci. Data 6:63. doi: 10.1038/s41597-019-0072-z
Lisi, C. S., Filho, M. T., Botosso, P., Roig, F. A., Maria, V. R. B., and Ferreira-Fedele, L. (2008). Tree-ring formation, radial increment periodicity, and phenology of tree species from a seasonal semi-deciduous forest in southeast Brazil. IAWA J. 29, 189–207. doi: 10.1163/22941932-90000179
Liu, H., Gleason, S. M., Hao, G., Hua, L., He, P., Goldstein, G., et al. (2019). Hydraulic traits are coordinated with maximum plant height at the global scale. Sci. Adv. 5:eaav1332. doi: 10.1126/sciadv.aav1332
Losapio, G. (2018). The assembly of a plant network in alpine vegetation. J. Veg. Sci. 29, 999–1006. doi: 10.1111/jvs.12681
Lotwick, H. W., and Silverman, B. W. (1982). Methods for analysing spatial processes of several types of points. J. R. Stat. Soc. 44, 406–413. doi: 10.1111/j.2517-6161.1982.tb01221.x
Maestre, F. T., Callaway, R. M., Valladares, F., and Lortie, C. J. (2009). Refining the stress-gradient hypothesis for competition and facilitation in plant communities. J. Ecol. 97, 199–205. doi: 10.1111/j.1365-2745.2008.01476.x
Markesteijn, L., Poorter, L., Paz, H., Sack, L., and Bongers, F. (2011). Ecological differentiation in xylem cavitation resistance is associated with stem and leaf structural traits. Plant Cell Environ. 34, 137–148. doi: 10.1111/j.1365-3040.2010.02231.x
McGill, B. J. (2010). Towards a unification of unified theories of biodiversity. Ecol. Lett. 13, 627–642. doi: 10.1111/j.1461-0248.2010.01449.x
McIntire, E. J. B., and Fajardo, A. (2009). Beyond description. The active and effective way to infer processes from spatial patterns. Ecology 90, 46–56. doi: 10.1890/07-2096.1
McIntire, E. J. B., and Fajardo, A. (2014). Facilitation as a ubiquitous driver of biodiversity. N. Phytol. 201, 403–416. doi: 10.1111/nph.12478
Meinzer, F. C., Woodruff, D. R., Domec, J. C., Goldstein, G., Campanello, P. I., Gatti, M. G., et al. (2008). Coordination of leaf and stem water transport properties in tropical forest trees. Oecologia 156, 31–41. doi: 10.1007/s00442-008-0974-5
Méndez-Alonzo, R., Paz, H., Cruz Zuluaga, R., Rosell, J. A., and Olson, M. E. (2012). Coordinated evolution of leaf and stem economics in tropical dry forest trees. Ecology 93, 2397–2406. doi: 10.1890/11-1213.1
Méndez-Toribio, M., Ibarra-Manríquez, G., Navarrete-Segueda, A., and Paz, H. (2017). Topographic position, but not slope aspect, drives the dominance of functional strategies of tropical dry forest trees. Environ. Res. Lett. 12:85002. doi: 10.1088/1748-9326/aa717b
Miles, L., Newton, A. C., DeFries, R. S., Ravilious, C., May, I., and Blyth, S. (2006). A global overview of the conservation status of tropical dry forests. J. Biogeogr. 33, 491–505. doi: 10.1111/j.1365-2699.2005.01424.x
Murphy, P. G., and Lugo, A. E. (1986). Ecology of tropical dry forest. Annu. Rev. Ecol. Syst. 17, 67–88. doi: 10.1146/annurev.es.17.110186.000435
Nguyen, H. H., Erfanifard, Y., Bui, V. B., Mai, T. H., Petritan, A. M., and Petritan, I. C. (2021). Topographic effects on the spatial species associations in diverse heterogeneous tropical evergreen forests. Sustainability 13:2468. doi: 10.3390/su13052468
Norden, N., González-M, R., González-M, A., Negret, B. S., Caicedo, C. A., and Rodriguez-Buritica, S. (2020). Building a socio-ecological monitoring platform for the comprehensive management of tropical dry forests. Plants People Planet 2, 228–248. doi: 10.1002/ppp3.10113
Pausas, J. G., Bonet, A., Maestre, F. T., and Climent, A. (2006). The role of the perch effect on the nucleation process in Mediterranean semi-arid oldfields. Acta Oecol. 29, 346–352. doi: 10.1016/j.actao.2005.12.004
Perea, A. J., Wiegand, T., Garrido, J. L., Rey, J. L., and Alcántara, J. M. (2021). Legacy effects of seed dispersal mechanisms shape the spatial interaction network of plant species in Mediterranean forests. J. Ecol. 109, 3670–3684. doi: 10.1111/1365-2745.13744
Perry, G. L. W., Miller, B. P., Enright, N. J., and Lamont, B. B. (2014). Stochastic geometry best explains spatial associations among species pairs and plant functional types in species-rich shrublands. Oikos 123, 99–110. doi: 10.1111/j.1600-0706.2013.00400.x
Pescador, D. S., de la Cruz, M., Chacón-Labella, J., and Escudero, J. (2019). The shape is more important than we ever thought: Plant to plant interactions in a high mountain community. Methods Ecol. Evol. 10, 1584–1593. doi: 10.1111/2041-210X.13248
Pizano, C., and García, H. (2014). El Bosque Seco Tropical en Colombia. Bogotá: Instituto de Investigación de Recursos Biológicos Alexander von Humboldt.
Poorter, L., McDonald, I., Alarcón, A., Fichtler, E., Licona, J. C., Peña-Claros, M., et al. (2010). The importance of wood traits and hydraulic conductance for the performance and life history strategies of 42 rainforest tree species. N. Phytol. 185, 481–492. doi: 10.1111/j.1469-8137.2009.03092.x
Poorter, L., Rozendaal, D., Sanaphre, L., Bongers, F., Almeida-Cortez, J. S., and Andrade, J. L. (2019). Wet and dry tropical forests show opposite successional pathways in wood density but converge over time. Nat. Ecol. Evol. 3, 928–934. doi: 10.1038/s41559-019-0882-6
Punchi-Manage, R., Wiegand, T., Wiegand, K., Getzin, S., Huth, A., Gunatilleke, C. V., et al. (2015). Neighborhood diversity of large trees shows independent species patterns in a mixed dipterocarp forest in Sri Lanka. Ecology 96, 1823–1834. doi: 10.1890/14-1477.1
Ribeiro, K. F. O., Martins, V. F., Wiegand, T., and Santos, F. A. M. (2021). Habitat filtering drives the local distribution of congeneric species in a Brazilian white-sand flooded tropical forest. Ecol. Evol. 11, 1797–1813. doi: 10.1002/ece3.7169
Sánchez-Azofeifa, G.-A., and Powers, J. S. (eds) (2014). Tropical Dry Forests in the Americas: Ecology, Conservation, and Management. Abingdon-on-Thames: Taylor & Francis. doi: 10.1201/b15417
Santoro, H. (2002). Estudios de Caracterización Biofísica y Socioeconómica de la Ecorregión Estratégica del Valle del Alto Magdalena. Ibagué: Universidad Surcolombiana. Report.
Siyum, Z. G. (2020). Tropical dry forest dynamics in the context of climate change. Syntheses of drivers, gaps, and management perspectives. Ecol. Process 9:25. doi: 10.1186/s13717-020-00229-6
Soliveres, S., Eldridge, D. J., Maestre, F. T., Bowker, M. A., Tighe, M., and Escudero, A. (2011). Microhabitat amelioration and reduced competition among understorey plants as drivers of facilitation across environmental gradients. Towards a unifying framework. Perspect. Plant Ecol. Evol. Syst. 13, 247–258. doi: 10.1016/j.ppees.2011.06.001
Stoll, P., and Bergius, E. (2005). Pattern and process. Competition causes regular spacing of individuals within plant populations. J. Ecol. 93, 395–403. doi: 10.1111/j.0022-0477.2005.00989.x
van Ommen Kloeke, A. E. E., Douma, J. C., Ordoñez, J. C., Reich, P. B., and Van Bodegom, P. M. (2012). Global quantification of contrasting leaf life span strategies for deciduous and evergreen species in response to environmental conditions. Glob. Ecol. Biogeogr. 21, 224–235. doi: 10.1111/j.1466-8238.2011.00667.x
Velázquez, E., Martínez, I., Getzin, S., Moloney, K. A., and Wiegand, T. (2016). An evaluation of the state of spatial point pattern analysis in ecology. Ecography 39, 1042–1055. doi: 10.1111/ecog.01579
Vellend, M. (2010). Conceptual synthesis in community ecology. Q. Rev. Biol. 85, 183–206. doi: 10.1086/652373
Wang, X., Wiegand, T., Kraft, N. J., Swenson, N. G., Davies, S. J., Hao, Z., et al. (2016). Stochastic dilution effects weaken deterministic effects of niche-based processes in species rich forests. Ecology 97, 347–360. doi: 10.1890/14-2357.1
Wiegand, T., Grabarnik, P., and Stoyan, D. (2016). Envelope tests for spatial point patterns with and without simulation. Ecosphere 7:e01365. doi: 10.1002/ecs2.1365
Wiegand, T., He, F., and Hubbell, S. P. (2013). A systematic comparison of summary characteristics for quantifying point patterns in ecology. Ecography 36, 92–103. doi: 10.1111/j.1600-0587.2012.07361.x
Wiegand, T., Huth, A., Getzin, S., Wang, X., Hao, Z., Gunatilleke, C. V., et al. (2012). Testing the independent species’ arrangement assertion made by theories of stochastic geometry of biodiversity. Proc. R. Soc. B 279, 3312–3320. doi: 10.1098/rspb.2012.0376
Wiegand, T., Jeltsch, T., Jeltsch, F., Hanski, I., and Grimm, G. (2003). Using pattern-oriented modeling for revealing hidden information. A key for reconciling ecological theory and application. Oikos 100, 209–222. doi: 10.1034/j.1600-0706.2003.12027.x
Wiegand, T., Martínez, I., and Huth, A. (2009). Recruitment in tropical tree species. Revealing complex spatial patterns. Am. Nat. 174, E106–E140. doi: 10.1086/605368
Wiegand, T., and Moloney, K. A. (2004). Rings, circles, and null-models for point pattern analysis in ecology. Oikos 104, 209–229. doi: 10.1111/j.0030-1299.2004.12497.x
Wiegand, T., and Moloney, K. A. (2014). Handbook of Spatial Point-Pattern Analysis in Ecology. Boca Raton, FL: CRC Press Taylor & Francis Group. doi: 10.1201/b16195
Wiegand, T., Savitri Gunatilleke, V. C., Nimal Gunatilleke, I. A. U., and Huth, A. (2007a). How individual species structure diversity in tropical forests. Proc. Natl. Acad. Sci. U.S.A. 104, 19029–19033. doi: 10.1073/pnas.0705621104
Wiegand, T., Gunatilleke, S., and Gunatilleke, N. (2007b). Species associations in a heterogeneous Sri Lankan dipterocarp forest. Am. Nat. 170, E77–E95. doi: 10.1086/521240
Keywords: tropical dry forest, spatial point patterns, facilitation, competition, neutral effects, ecological process
Citation: Salazar Villegas MH, Wiegand T, González-M R, Rodriguez-Buritica S, Qasim M and Csaplovics E (2023) Spatial facilitation and competition regulate tree species assembly in a tropical dry forest. Front. For. Glob. Change 6:1028515. doi: 10.3389/ffgc.2023.1028515
Received: 26 August 2022; Accepted: 16 February 2023;
Published: 14 March 2023.
Edited by:
Leonel Lopez-Toledo, Universidad Michoacana de San Nicolás de Hidalgo, MexicoReviewed by:
Andrea Jara-Guerrero, Universidad Técnica Particular de Loja, EcuadorG. N. Tanjina Hasnat, University of Chittagong, Bangladesh
Copyright © 2023 Salazar Villegas, Wiegand, González-M, Rodriguez-Buritica, Qasim and Csaplovics. This is an open-access article distributed under the terms of the Creative Commons Attribution License (CC BY). The use, distribution or reproduction in other forums is permitted, provided the original author(s) and the copyright owner(s) are credited and that the original publication in this journal is cited, in accordance with accepted academic practice. No use, distribution or reproduction is permitted which does not comply with these terms.
*Correspondence: Mike H. Salazar Villegas, mhvillegas@gmail.com