- 1Laboratory of Plant Ecology, Department of Ecology, Biology Institute, Federal University of Rio de Janeiro, Rio de Janeiro, Brazil
- 2Laboratory of Forest Ecology and Conservation, Department of Environmental Sciences, Forestry Institute, Federal Rural University of Rio de Janeiro, Seropedica, Brazil
Palms species (Arecaceae) are abundant in tropical forests and influence ecosystems in important ways. Moreover, they are a relevant feature in the Atlantic Forest biodiversity hotspot. In this study, we seek to better understand the distribution of palm richness in Rio de Janeiro state, Brazil, with the aim to support conservation decisions and actions. Maps for 15 palm species were generated through species distribution modeling and then stacked into a palm richness map, which was further combined with current land-use and protected area maps to generate a realistic portrayal of the current situation of Arecaceae in the state. Our results revealed an increasing inland-to-coast pattern of richness that matches the biogeographical subdivision of the Atlantic Forest. Considering the land-use information, the palm species potential distribution is drastically reduced, especially for some species which already have a restricted distribution in the state. We also identified the most relevant protected areas for the conservation of palms in the state and those which might have been overlooked in floristic inventories, thus requiring more detailed investigation. Moreover, we point out those species with few points, for which species distribution models could not be built, and argue that they are the ones more likely to be threatened by habitat loss and should be the focus of specimen collection and recording. Finally, we draw attention to a large medium-richness remnant located between two protected areas which probably functions as a connection between them and should be a priority area for conservation.
Introduction
The Arecaceae family comprises 183 genera and about 2,400 species distributed throughout the tropics (Baker et al., 2011). The family includes 38 genera and 282 species in Brazil, of which approximately 122 species are endemic (Leitman et al., 2012). The largest diversity centers of the family in Brazil are the Amazon Forest (135 spp.), the Cerrado (Brazilian savanna, 92 spp.), and the Atlantic Forest (62 spp.) (Leitman et al., 2012). Despite their abundance and importance in tropical forests (Eiserhardt et al., 2011; Oliveira et al., 2014; Fisch and Gomes, 2015), palms are generally less represented than tree species in inventories and in ecological and botanical studies, especially understory palms that generally have a smaller DBH than the ones adopted by floristic inventories. Apart from high richness, Arecaceae are fundamental organisms in the structure and functioning of tropical ecosystems (Muscarella et al., 2020). Palms produce more than 80% of the fruit biomass in some tropical forests (Genini et al., 2009) and are therefore considered key resources for fauna (Galetti et al., 2013). Moreover, they may represent up to 60% of the total basal area in some Neotropical forests (Muscarella et al., 2020). According to Eiserhardt et al. (2011), species of this family can be considered ecosystem engineers because they increase heterogeneity in environmental factors such as light availability, litter production, and microclimate.
Anthropogenic activities have generated disturbance in nearly all ecosystems around the world (Vitousek et al., 1997; Lindenmayer et al., 2017). Activities such as deforestation for livestock, agricultural fields, and increasing urbanization have led to the reduction of native forests, mainly caused by the increase in human populations and the respective demand for resources (Laurance et al., 2014; Potapov et al., 2017). Habitat loss and fragmentation produce changes in the natural environment (Newbold et al., 2015). Beyond direct forest loss, such processes trigger indirect effects such as changes in abiotic factors (e.g., temperature, humidity, and incidence of light) due to edge effects and canopy openness (Arroyo-Rodríguez et al., 2017). Other indirect effects are changes in biotic factors, such as the loss of species that depend on native forests, invasion by non-native species, disruption of pollination and dispersal processes, and alteration in the dynamics of plant species growth, which can in turn alter succession (Rocha-Santos et al., 2016).
The Atlantic Forest in Brazil is highly susceptible to the harmful effects of deforestation. Currently, only about 32 million ha (28%) of the original vegetation cover remains, of which 25 million ha are small fragments of <50 ha with a high mean distance to the patches around (1,440 m) (Rezende et al., 2018). The Atlantic Forest is the predominant ecosystem in the state of Rio de Janeiro, currently covering 1.35 million ha (31%) of the state's area (Projeto MapBiomas, 2018), and, as in other parts of its distribution, is represented mostly by forest fragments surrounded by a heterogeneous matrix of different land uses. While the state ranks third in nature protection in Brazil, with 629 thousand ha (14.4%) of its area within protected areas (PAs) (IUCN categories I–VI; Jenkins et al., 2010), it also ranks third in population size, estimated at more than 15 million people, or 365 inhabitants/km2 (IBGE, 2020). It ranks fourth in Human Development Index and 24th in size (4,370,000 ha) (IBGE, 2020). The combination of large population and reduced territory leads to high pressure on natural resources. However, such pressure does not affect the state evenly. Rio de Janeiro state has a topographical and geomorphological diversity (Silva, 2002), and regions included on mountainous geomorphological compartments have more standing vegetation. Therefore, identifying which forest remnants are most important for biodiversity conservation should be done considering the geomorphological diversity of the state.
The establishment of PAs is considered one of the most efficient strategies for biodiversity conservation (Bingham et al., 2019). Brazil stands out for its biodiversity as well as for investing in establishing PAs, currently harboring the largest (~ 30 % of its territory) terrestrial PA system in the world (UNEP-WCMC IUCN, 2016). Among the areas under legal protection in the country, 26% are in the Atlantic Forest. The PAs in this biome protect 30% of the remaining forests in the state, 9% of which are in strict protection categories (IUCN categories I–IV) and 21% in sustainable use categories (IUCN categories V–VI; Rezende et al., 2018). The first Brazilian PA, Itatiaia National Park, was established in 1937 in the Atlantic Forest mountains in Rio de Janeiro state. However, the National Protected Area System was only enforced in 1998 (Federal Law no. 9985/1998), setting criteria and standards for the establishment, implementation, and management of PAs in Brazil.
Species distribution modeling (SDM) is helpful for understanding species distributions and for mapping priority areas for conservation and for the establishment of protected areas. This technique is widely used in conservation studies (Guisan et al., 2013). The SDM uses different sets of algorithms to combine georeferenced occurrence data and gridded environmental maps (covariables) to predict the potential distribution of a given species (see Elith and Leathwick, 2009 for further discussion). Stacked distribution maps in turn generate species richness maps (Graham and Hijmans, 2006), which can be used to support conservation decisions (e.g., Zhang et al., 2012).
The main goal of this study was to identify priority areas for the conservation of the Arecaceae in Rio de Janeiro state, considering areas under legal protection or not, with a high potential for the conservation of palm species. To do so, we used the SDM to generate a richness map of palm species in the state of Rio de Janeiro (Fundação SOS Mata Atlântica INPE-Instituto Nacional de Pesquisas Espaciais, 2015). We then combined the output map with information on PA location, current land-use and PA Management Plans to identify PAs with high potential palm species richness, unprotected forest remnants with high potential richness that should be legally protected, and PAs that have been insufficiently studied. We also expect our results to provide information for informed decision making and conservation planning to protect the Arecaceae family in the state of Rio de Janeiro.
Materials and methods
Study area
The state of Rio de Janeiro is in the southeastern of Brazil, with an area of 43,750,425 km2, and the third largest population of the country (IBGE, 2020). Remnants of the Atlantic Forest occupy 18.70% (1.3 million hectares) of the territorial extension of Rio de Janeiro state (Fundação SOS Mata Atlântica and INPE-Instituto Nacional de Pesquisas Espaciais, 2015). Given the history of occupation, these remnants are distributed in a dispersed and fragmented way, especially in three regions located in the more inland portions of the Escarpas and Reversos of Serra do Mar, along the Vale do Paraiba and at the end of the North Fluminense (Fidalgo et al., 2009). Of them, 462,000 hectares is protected in 19 federal and 36 state protected areas (INEA-Instituto Estadual do Ambiente, 2016). The state's relief is formed, especially by mountains, lowlands, and coastal massifs. The inland mountains are in Serra do Mar and Vale do Paraíba do Sul. The culminating point is the peak of Agulhas Negras (2,791 m, Itatiaia National Park). The lowland areas are located between the plateau and the shoreline, and the coastal massifs are elevations that arise inside these areas. The climate of Rio de Janeiro state varies according to the portions of the state and its proximity to the sea (Köppen, 1948). The climate Aw, tropical semi-humid, with summer rains and dry winters, occurs near the massifs and slopes in the western portion of the lowland. The Af climate, humid tropical, with well-distributed rainfall throughout the year, occurs in the lower portions of the Serra do Mar, while the Cfa and Cfb climates occur in the higher portions. The Cwb and Cwa climates occur on the back of the Serra do Mar, on the back of the plateau in areas with less rainfall, with rains concentrated in the summer periods.
The topographical and climatic varieties provide a diversity of vegetation landscapes and, consequently, an expressive variety of habitats and species richness, including several endemic ones. These landscapes, considering the physiognomies, the ecological characteristics, the flora, and the altitude, allow the recognition in the state of different vegetation formations (Supplementary Figure S1). We highlight the Dense Ombrophilous Forest (Lowlands, Submontane, Montana and Upper Montana, or “nebular forests”), the Seasonal Semideciduous Forest (also distinct in Lowlands, Submontane, and Montana), and the ecological refugia, which include the Campos de Altitude, Coastal Plain Forests or Restinga, and mangroves, vegetation with fluviomarine influence (Veloso et al., 1991; IBGE, 2012). About 334 families, 1,821 genera, and 8,203 species are cataloged for the flora of the state of Rio de Janeiro, 1,740 of which are endemic (Baumgratz et al., 2014). The western and central regions of Rio de Janeiro state, followed by Serra dos Órgãos, have high species richness and endemism in the state of Rio de Janeiro (Werneck et al., 2011).
Species selection and cleaning of occurrence data
All species listed in a field guide of the palms of Rio de Janeiro state (Henderson, 2009) were included in the analysis. The only palm species inhabiting the state that the guide fails to register is Syagrus insignis (Devansaye) Becc, but this species was also included in this study. Moreover, this guide was published prior to the most recent revision of Geonoma Willd (Santos et al., 2020). Therefore, while the guide lists nine species of Geonoma, only three are currently recognized (viz., G. elegans Mart., G. pohliana Mart., and G. schottiana Mart.). It must be noticed that G. pohliana Mart. is an extremely variable species and has 11 recognized subspecies (Santos et al., 2020). Of them, G. pohliana subsp. fiscellaria, G. pohliana subsp. gastoniana, G. pohliana subsp. kuhlmannii, G. pohliana subsp. trinervis, G. pohliana subsp. Rodriguesii, and G. pohliana subsp. pohliana inhabit the state. Importantly, the first four subspecies are endemic to the state. Fitting separated SDM to all these species would be valuable, but we decided not to do so for the following reasons. First, the Geonoma genus has a complicated taxonomy, and telling species and subspecies apart is not simple (Santos et al., 2020), hence a considerable part of the occurrence records, especially those of subspecies that are likely to be misidentified. This would require us to visually check the exsiccate associated with each record, which would be very time-consuming. Second, most of Geonoma subspecies have very few occurrence records, precluding the use of SMD at all (see the next paragraph concerning occurrence records prospecting and minimum record number). Therefore, we choose to include only G. pohliana subsp. pohliana, which is the most common subspecies in Rio de Janeiro state, it is less likely to be misidentified, and it has many occurrence records. All the other species' taxonomic status considered here is in consonance with that of the field guide for palms of Rio de Janeiro state (Henderson, 2009). All considered, Rio de Janeiro state has 22 palm species, all of which were included in our study group.
Occurrence data were collected from two online databases: speciesLink (http://www.splink.org.br/) and GBIF (https://www.gbif.org/). The georeferenced occurrence data were cleaned by removing occurrence points that were outside the known native range of the species [obtained from Rio de Janeiro Botanical Garden (http://reflora.jbrj.gov.br/) and Kew Royal Botanic Gardens (http://www.plantsoftheworldonline.org/) sites]. Then, to reduce spatial autocorrelation, the dataset was submitted to geographical filtering using the spThin R package (Aiello-Lammens et al., 2015), removing data points less than 5 km apart of any other data point. After this data cleaning process, only species with more than 10 occurrence points were maintained (Wisz et al., 2008).
Covariable selection
The environmental covariables used in the modeling were selected independently for each species from a common dataset of 22 environmental variables with 2.5 arc minutes of spatial resolution. This dataset was composed by the 19 WorldClim bioclimatic variables (version 2.1) (https://www.worldclim.org/) plus three variables from the ENVIREM database: annual potential evapotranspiration (annualPET), terrain roughness index (tri), and SAGA-GIS topographical wetness index (topoWet) (Title and Bemmels, 2017). The selection was made through a principal component analysis (PCA) with the 22 covariables for each species. The variables that contributed most to the first principal component and were not highly correlated (r <0.70) were selected, respecting the rule of 1 variable for each 10 occurrence points (Wisz et al., 2008). The data used in the PCA were generated by plotting 1,000 random points inside the species range and extracting the value of each covariable from the common dataset. Each species range was defined as the ecoregions (sensu Olson et al., 2001) that contained occurrences of the species.
Model training, evaluation, and ensembling
The models were generated using the R package Model-R (Sánchez-Tapia et al., 2017), currently named modleR (https://github.com/Model-R). The occurrence dataset for training and testing was subdivided using a 3-fold cross-validation technique repeated three times, which generated nine different sets of training and test datasets. Five different algorithms were used: two presence-only environmental distance algorithms (BIOCLIM and DOMAIN), one presence/background algorithm (MaxEnt), and two presence/absence algorithms (GLM and RF). This decision was made to include a diversity of different algorithms selected from the modleR package. This was done based on the assumption that there is no single best algorithm for all cases (Qiao et al., 2015) and that, lacking a priori information of which algorithm should perform better, one should test different algorithms and select those with the best performance. The combination of divided datasets and selected algorithms resulted in 45 models (9 × 5) for each species. For each species, we generated a number of pseudo-absences equivalent to the number of records times 10 and 10,000 background points inside the calibration area. The background points were randomly distributed, as is usually done, because they are generally sampled just to reduce the computational time (Senay et al., 2013). On the contrary, pseudo-absences should ideally be biologically meaningful, and while there are many ways to generate them, approaches based on environmental dissimilarity are generally reported as more accurate and have become somewhat standard (Senay et al., 2013). Hence, the pseudo-absence points were generated randomly inside pixels with 5% or more of environmental dissimilarity compared with those with occurrence records. The environmental dissimilarity was calculated using Euclidean distance in the environmental space defined by the variables selected to a given species' modeling. The calibration area was species specific and defined as the terrestrial ecoregions containing occurrence records of the species plus adjacent ecoregions.
The true skill statistics (TSS) was used to assess model performance (Thuiller et al., 2009). Only those models with a TSS score higher or equal to 0.7 were kept, as values close to 1 reflect a good predictive ability of the model (Allouche et al., 2006). These high-performance models were transformed from continuous to binary using the value that maximized the TSS as threshold (i.e., max TSS threshold). Then, the binary maps were used to create a final binary ensemble model for each species (Araújo and News, 2007), as follows: The binary models were combined, generating a continuous ensemble, and the lowest presence threshold (Pearson et al., 2007) was used to transform the output to binary (as in Santos et al., 2020). Finally, the final binary ensemble models of all species were stacked to create a richness prediction map, which was subsequently clipped to Rio de Janeiro state limits, our area of interest. Predicted richness based on climatic variables can be interpreted as an index of the “abiotic richness” of a site, represented by the number of species it can possibly harbor (Santos et al., 2020).
Landscape analysis with SDM results and bibliographical research
A vector layer containing the PAs in Rio de Janeiro state was obtained from the Instituto Estadual do Ambiente website (http://www.inea.rj.gov.br). Only the protected areas in strict protection categories (IUCN category IA) were considered in this study (Supplementary Figure S2). PAs classified as sustainable use (IUCN categories V–VI) were discarded. By combining the PA layer with the ensemble model raster of each species, we obtained a list of species for each PA. To better understand the actual palm richness distribution in the state and the impact of habitat degradation on each species' potential distributions, the richness map and each species' binary ensemble model were cut using a land-use map. To create this map, a land-cover image was used to assess the natural vegetation remnants in the state of Rio de Janeiro, these being regarded as areas that palm species can inhabit. A 30-m-resolution Landsat image from the year 2018 was obtained from Collection 4.1 of the MapBiomas Project (Projeto MapBiomas, 2018). This image provided by MapBiomas Project already included a classification of categories of land use. We transformed the image into a binary layer (habitat and non-habitat), using as “habitat” the following categories: “Forest formation,” “Savanna formation,” “Mangrove,” and “Other non-forest natural vegetation” (which includes the sandy coastal plain vegetation, i.e., restinga). As the land use and the species' potential distribution had different resolutions, 30 m and 2.5 arc s (~5k m), respectively, we could not do a straightforward cut. Instead, we considered that a given pixel of the species' potential distribution raster was inside the land-use raster if its area was ≥50%covered by the smaller land-use pixels.
A non-metric multidimensional scaling (NMDS) ordination analysis based on the Sørensen dissimilarity was performed to explore the similarity of the prediction of species composition in the protected areas (McCune and Grace, 2002). We used the metaMDS function in the vegan package (Oksanen et al., 2019), setting the “distance” argument to “bray.” This should generate a Bray–Curtis dissimilarity matrix, but as we used presence–absence data instead of abundance data, the Bray–Curtis dissimilarity gets simplified to the Sørensen dissimilarity (Ricotta and Podani, 2017). In addition, environmental variables were used as linear predictors and plotted together with the ordering diagram using the envfit function in the vegan package (Oksanen et al., 2019). The variable selection was made by generating the PCA, with the same 22 variables used for modeling, for random points in PA. The variables that most contributed to the first axis and were less correlated with each other were selected. We also classified the protected areas as inland or coastal using the official classification of the state of Rio de Janeiro that distinguish between coastal and inland municipalities (MMA, 2022). For the classification between plains and mountainous regions, a PA with the mean altitude above 500 m was classified as mountainous, except for Guaxindiba Ecological Station, in the extreme north of the state, which has a different formation from all the others, classified in its management plan as coastal tableland (Table 1).
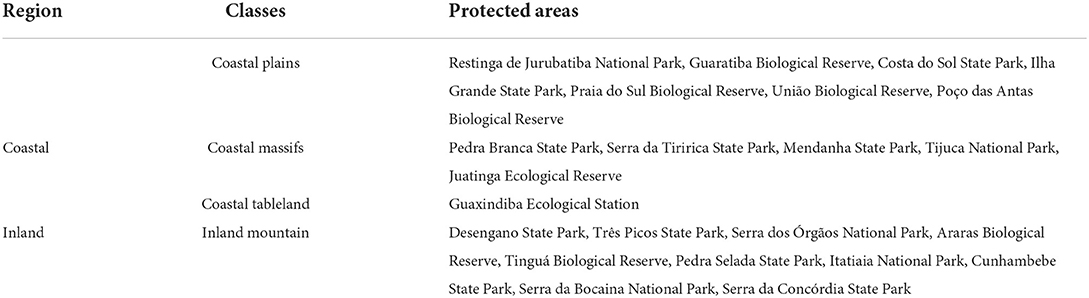
Table 1. Classification of the protected areas of Rio de Janeiro state, Brazil, according to their coastal distance and terrain elevations.
In order to verify which palm species has been registered for each PA in Rio de Janeiro state, we reviewed PA Management Plans (http://www.inea.rj.gov.br/; https://www.icmbio.gov.br/) and occurrence data used to build distribution models. Our search was focused on state (n = 14) and federal (n = 8) strict protection areas. This search was used to compare the known occurrence of species in a PA with the occurrence inferred by species distribution models.
Results
Of the 22 native palm species of the state, only 15 final ensemble models could be built: Allagoptera arenaria (Gomes) Kuntze, A. caudescens (Mart.) Kuntze, Astrocaryum aculeatissimum (Schott) Burret, Attalea dubia (Mart.) Burret, A. humilis Mart. ex Spreng., Bactris caryotifolia Mart., B. setosa Mart., B. vulgaris Barb.Rodr., Euterpe edulis Mart., Geonoma elegans Mart., Geonoma pohliana Mart. (subsp. pohliana), Geonoma schottiana Mart., Syagrus picrophylla Barb.Rodr., Syagrus pseudococos (Raddi) Glassman, and Syagrus romanzoffiana (Cham.) Glassman. These 15 species were the ones that had >10 data points and one or more models with TSS > 0.7 (Supplementary Table S1). Of the seven excluded species, Attalea apoda Burret, Syagrus macrocarpa Barb. Rodr., and Syagrus weddelliana (H. Wendl.) Becc were excluded because they had lass then 10 records, while Acrocomia aculeata (Jacq.) Lodd. ex R.Keith, Desmoncus orthacanthos Mart., Desmoncus polyacanthos Mart., and Syagrus insignis (Devansaye) Becc. were excluded because none of their models had a TSS > 0.7 (Supplementary Table S1). While the two Desmoncus species and A. aculeata have very broad distributions encompassing a large part of the Neotropics, the other four species are much more restricted, and one of them—S. weddelliana—is endemic to the state of Rio de Janeiro (Supplementary Table S1).
The richness map assembled with the final ensemble model of each species predicts a pattern of increasing inland-to-coastal richness for the Arecaceae in Rio de Janeiro state (Figure 1A). The largest area of high richness is represented by the northern coastal lowland forests, while the largest area of low richness is the northern interior part of the state (Figure 1A). When the current land-use map is applied as a filter, these two large areas of high and low richness disappear (Figure 1B). Nevertheless, the loss of area among richness classes is not even, as low-richness classes are more affected (e.g., the areas with richness 1 to 3 disappeared) (Figure 1C). This asymmetry in area loss, with low-richness areas more affected than high-richness ones, results in a difference in the overall mean richness in the state between scenarios considering the whole state (mean richness = 8.66; SD = 2.75) and forest remnants only (i.e., using the land-use map) (mean richness = 9.59; SD = 2.02) (Figure 1C). A Welch two-sample t-test rendered this difference as significant (t = 8.46, p < 0.001). When analyzing natural vegetation remnants only, the largest contiguous area that remains is represented by the forests in the center of the state (Serra dos Órgãos region), with medium-to-high richness (Figure 1B).
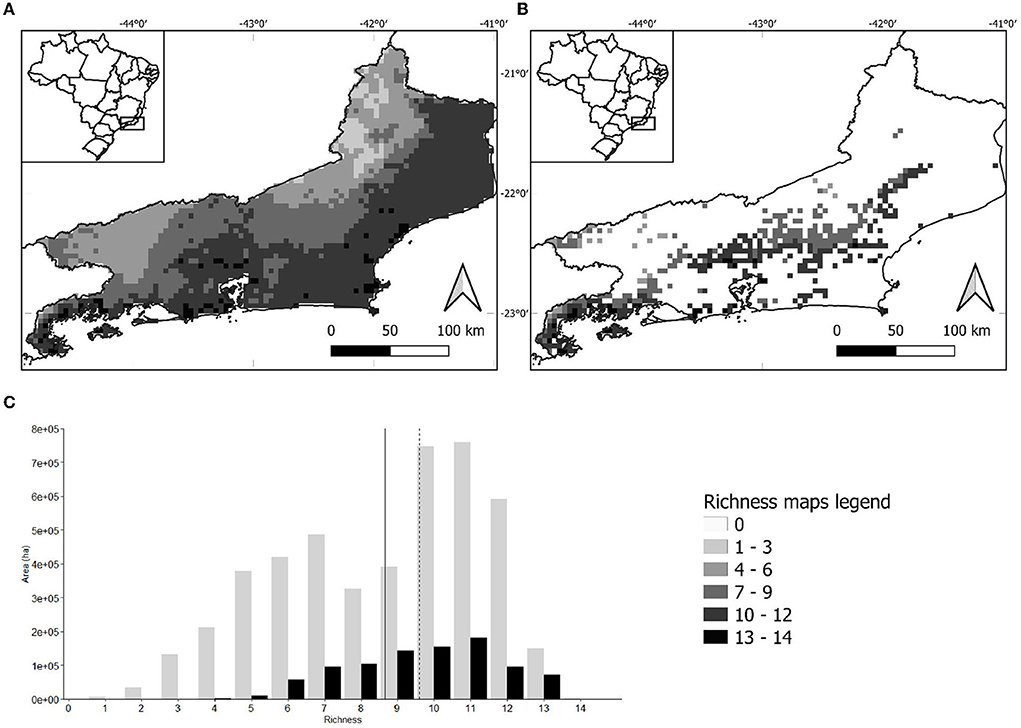
Figure 1. (A) Distribution of palm species richness in Rio de Janeiro state as predicted by stacked species distribution models. (B) Palm species richness in Rio de Janeiro state forest remnants only as predicted by stacked species distribution models. In both maps, light colors represent the areas of low richness and dark colors the high richness. (C) Bar chart showing the area occupied in hectares by each richness class in Rio de Janeiro state as predicted by stacked species distribution models. Gray and black bars represent the palm species distribution considering the entire state of Rio de Janeiro and only forest remnants, respectively. The solid line represents the average of gray bars and the dashed line the average of black bars.
When considered individually, B. setosa, E. edulis, G. elegans, G. schottiana, S. picrophylla, and S. romanzoffiana, the species with the largest potential distributions, have their range reduced to roughly the same percentage (20% of the original range) and area (~900 thousand ha) when the land use is taken into account (Figure 2). On the contrary, the response of the six species with the smallest potential distributions is not uniform. The two species with the smallest distributions, namely, S. pseudococos and A. dubia, have their range reduced to 40 and 50%, respectively, while A. humilis, A. arenaria, B. vulgaris, and A. caudescens had their range reduced to 13, 3, 15, and 11%, respectively (Figure 2). Hence, the species with the highest (A. arenaria) and the lowest proportional loss in potential distribution area (S. pseudococos) both occur in the same group of six species with the smallest potential distributions.
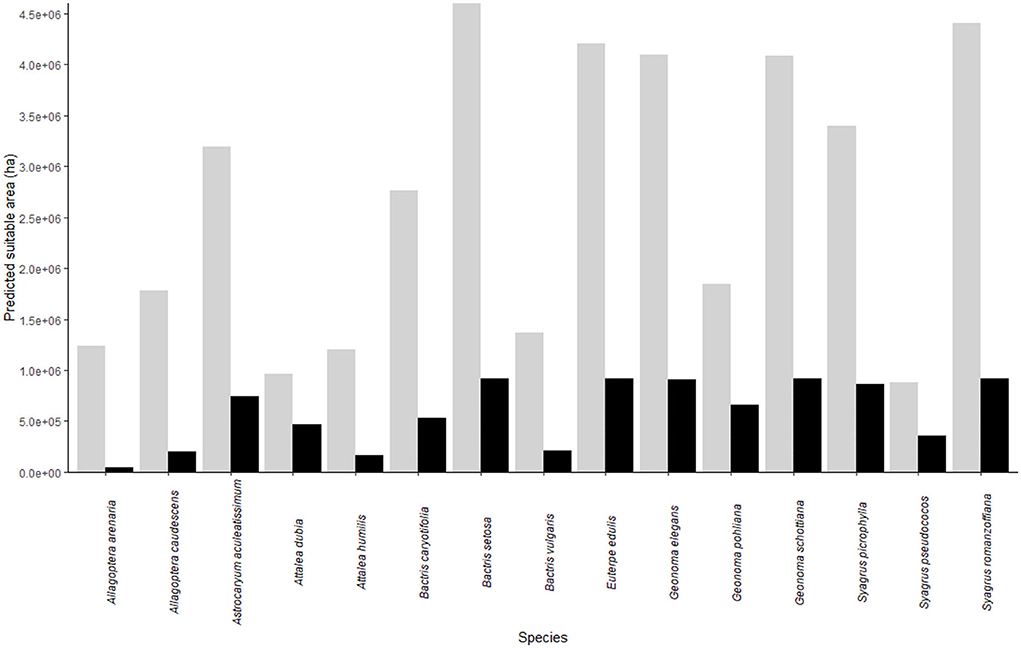
Figure 2. Area in hectares suitable for each of the 15 studied palm species as predicted by species distribution modeling. Gray and black bars represent the area considering the entire Rio de Janeiro state and forest remnants, respectively.
There are no records of palm species in three protected areas: Guaratiba Biological Reserve, Serra da Concordia State Park, and Guaxindiba Ecological Station (Supplementary Figure S2 and Supplementary Table S2). The PAs with the highest number of species are Serra da Tiririca State Park (n = 10 ssp.) and Cunhambebe State Park (n = 9 spp.). Among the most common species found in PAs are Euterpe edulis (n = 18 PAs), Geonoma schottiana (n = 14 PAs), and Astrocaryum aculeatissimum (n = 14 PAs). There are few records of some species such as Syagrus picrophylla (n = 1 PA), Allagoptera caudescens (n = 2 PAs), Bactris vulgaris (n = 2 PAs), Allagoptera arenaria (n = 3 PAs), and Syagrus pseudococos (n = 3 PAs). We also found, for some PAs, a considerable mismatch between predicted and registered richness. Guaratiba Biological Reserve, Serra da Bocaina National Park, and Guaxindiba Ecological Station were predicted as highly rich (> 12 species) and yet had little or no records of palm species occurrence in the literature.
The Pedra Branca State Park, located in the urban area of Rio de Janeiro state (Supplementary Figure S2), is environmentally suitable for all the palm species analyzed (n = 15 spp.). Another seven PAs had almost the same potential species composition, except for the predicted absence of Allagoptera arenaria: Ilha Grande State Park, Praia do Sul Biological Reserve, Cunhambebe State Park, Serra da Bocaina National Park, Tinguá Biological Reserve, União Biological Reserve, and Três Picos State Park. The similarity in potential composition between many PAs is represented by overlapping points in the center of the ordering diagram (Figure 3). The potential composition of species is defined as the composition a PA may have considering the species in the regional pool and the environmental suitability of a PA for each species as determined by the SDM.
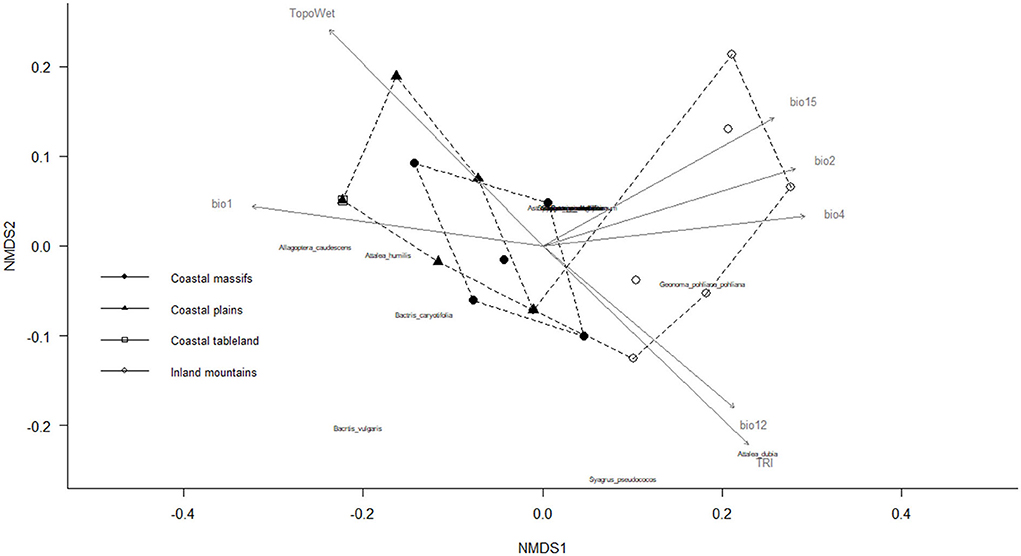
Figure 3. Ordering diagram of non-metric multidimensional scaling (NMDS) based on Bray–Curtis distance (stress: 0.093) for predicting species composition similarity in the protected areas (PAs). Gray arrows indicate the explanation of the sampling units (PA) by the WorldCim and ENVIREM variables: bio1—annual mean temperature (r2 = 0.6545, p = 0.001), bio2—mean diurnal range (r2 = 0.5307, p = 0.001), bio4—temperature seasonality (r2 = 0.5288, p = 0.001), bio12—annual precipitation (r2 = 0.4765, p = 0.002), bio 15—precipitation seasonality (r2 = 0.5318, p = 0.001), TRI—terrain roughness index (r2 = 0.6227, p = 0.001), and TopoWet—topographical wetness index (r2 = 0.7014, p = 0.001).
The environmental variables that were more significantly correlated with potential species composition were as follows: annual mean temperature (R2 = 0.65, p = 0.001), mean diurnal range (R2 = 0.53, p = 0.001), temperature seasonality (R2 = 0.53, p = 0.001), annual precipitation (R2 = 0.48, p = 0.005), precipitation seasonality (R2 = 0.53, p = 0.001), terrain roughness index (R2 = 0.62, p = 0.001), and topographical wetness index (R2 = 0.70, p = 0.001), which means that to some degree they are related to the composition of palm species in a PA in Rio de Janeiro state (Figure 3). The climate in coastal PA region is characterized by higher temperatures and low climatic seasonality and therefore provides greater environmental suitability for Allagoptera species, Bactris vulgaris, Bactris caryotifolia, and Attalea humilis. The climate of the inland mountainous region of the state is, in turn, characterized by lower temperatures and high precipitation and thus provides greater environmental suitability for Attalea dubia, Geonoma pohliana subsp. Pohliana, and Syagrus pseudococos (Supplementary Table S2).
Some parts of Rio de Janeiro state combine the presence of native vegetation remnants with high richness prediction, but are not enclosed in a PA, such as the one on the northern part of the state between Tres Picos State Park and Desengano State Park (Supplementary Figure S2). Richness is high to medium in these forest fragments (7–12 species), which are therefore important for the application of conservation strategies.
Discussion
The potential species richness of palm trees in Rio de Janeiro state was assessed through species distribution modeling to understand richness distribution and inform conservation decisions. We found a pattern of increasing richness from inland to coastal areas. Moreover, our results point to four possible courses of action for the conservation of Arecaceae in the state: (a) highlighting the importance of the richest PA, while ensuring continuous financial support and beneficial public policies; (b) focusing on the four palm species (Allagoptera arenaria, A. caudescens, Attalea humilis, and Bactris vulgaris) most affected by deforestation; (c) need of increasing efforts in collecting palm specimens in the field, especially of those four palm species (A. apoda, S. macrocarpa, S. weddelliana, and S. insignis) with restricted distribution for which the SDM could not be done; and (d) protection of the medium-to-high richness forest remnants between Três Picos and Desengano State Parks. Finally, we highlight some PAs that probably have been overlooked in terms of their composition and would profit from new floristic inventories focused on the family.
The observed pattern of increasing richness toward coastal areas loosely matches the transition between the two Atlantic Forest biogeographical subregions in Rio de Janeiro state (e.g., Silva and Casteleti, 2003). The areas with high richness are concentrated in the subregion mainly represented by coastal Ombrophilous Forests. Conversely, the areas of low richness are concentrated in the subregion mainly represented by inland Deciduous and Semi-Deciduous Forests. A study modeling the richness of fruit-feeding butterflies throughout the Atlantic Forest also found that the richness pattern matched these major subregional divisions (Santos et al., 2020). Furthermore, our study shows that the lowland forest areas are the richest within Rio de Janeiro state, which corroborates the finding of Henderson (2002) that, out of 1,245 palm species, more than 70% occur in lowland moist forests. This richness contrast between dry (i.e., Deciduous and Semi-Deciduous Forests) and wet (i.e., Ombrophilous Forests) biogeographical subregions fits the expectation that climatic variables associated with precipitation are an important driver of Arecaceae diversity in the Neotropics (Eiserhardt et al., 2011).
Analogous composition patterns were observed between PAs with similar geographic formation. They are probably associated with climatic variations resulting from altitudinal variation, as previously observed in the mountainous regions of the Atlantic Forest (Oliveira et al., 2014). Allagoptera arenaria, Allagoptera caudescens, Bactris vulgaris, and Attalea humilis were closely related to PAs in the coastal regions in our analysis, which is in agreement with Reis (2006), who describes these same species as occurring on sandy coastal plain vegetation in the state of Rio de Janeiro. Attalea dubia, Geonoma pohliana, and Syagrus pseudococos characterized the inland mountainous region of the state as well as the mountainous regions in Submontane and Montane formations of the Atlantic Forest in São Paulo state (Oliveira et al., 2014). The analysis of potential species composition in PA, in addition to the NMDS analysis, was important to identify distribution patterns.
Urban ecosystems, although often overlooked, can play an important role in nature conservancy (Soanes and Lentini, 2019). Our study echoes this idea, as Pedra Branca State Park and Tiririca State Park, both urban parks, were the more important in terms of Arecaceae richness. The former is the only environmentally suitable PA for all the palm species in the regional pool, and the latter has the highest recorded richness in the bibliographical survey (Supplementary Table S2). Opportunities for cities to play important roles in the conservation of threatened species abound globally (Soanes and Lentini, 2019). In addition to providing habitat for native species, natural remnants in urban areas also provide ecosystem services such as regulation of microclimate and water availability and pollution reduction (Elmqvist et al., 2015).
When comparing the SDM and bibliographic research results, we observed an overprediction of the models, that is, a larger area of environmental suitability than registered in former studies and management plans (Supplementary Table S2). An overprediction is a widespread shortcoming of the SDM due to many sources of uncertainty. The main source that we were unable to address is the uncertainty generated by factors other than the abiotic environment which also influence species distribution and are not included in the SDM framework (i.e., biotic interactions and dispersal limitations) (Graham and Hijmans, 2006). Previous studies reveal that the net effect of all uncertainty is commonly an overprediction of species range and species richness (Graham and Hijmans, 2006). Nevertheless, some part of the overprediction found here might be due to knowledge gaps regarding protected areas and palm species distribution in Rio de Janeiro state. For example, the richness of Guaratiba Biological Reserve and Guaxindiba Ecological Station was predicted as high or very high, and yet not a single palm species has been recorded in them. The lack of records of any species in our bibliographical research, especially the easily identifiable species such as Euterpe edulis and Syagrus romanzoffiana, predicted for both these PAs, argues in favor of the idea that this overprediction is not related to the model itself, but to the lack of surveys in these areas. This conclusion is supported by the fact that Arecaceae species are often not included in general floristic and phytosociological studies, because of sampling criteria related to plant size (Fisch and Gomes, 2015). We also found, for some PAs, a considerable mismatch between predicted and registered richness. Therefore, in order to gain information about these PAs plant composition and palm species distribution, both valuable information for the conservation of the family in the state, we advise that new floristic inventories, especially focused on the family, should be undertaken in these areas.
The species distribution range is deeply associated with conservation and one of the features used in risk assessments for the IUCN Red List (IUCN, 2012). Species distribution modeling combined with the current land-use maps can provide more realistic predictions of species distributions than traditional methods (e.g., point occurrence to grid and minimum convex polygon) (Solano and Feria, 2007). Using this combined approach, we found that Allagoptera arenaria, A. caudescens, Attalea humilis, and Bactris vulgaris are in the group of six species with the smallest potential area of occurrence and are the ones with the greatest loss of potential habitat when the land-use filter is applied. Hence, these four species should be prioritized in species-level conservation programs, especially A. arenaria, a palm typical of sandy coastal plain vegetation (Henderson, 2002). This ecosystem is under constant threat by real estate speculation despite its important role in coastal protection and inclusion in the National Climate Adaptation Plan (Brasil, 2016). We highlight, however, that these recommendations are based on the situation of these species in Rio de Janeiro state only and that the distribution of none of these four palm species is restricted to this state. Therefore, this regional guideline must be in consonance with a more general policy that ensures the conservation of local populations in Rio de Janeiro state and of the species as a whole. For those species that could not be modeled, the simpler approach of determining the area of extent using minimum convex polygons is the only option. Of those we could not include in the SDM analysis, we call attention to A. apoda, S. macrocarpa, S. weddelliana, and S. insignis. These species have very few occurrence records and also have a restricted distribution. This is especially the case for S. weddelliana, endemic to Rio de Janeiro state, and for S. insignis and S. macrocarpa, endemic to Rio de Janeiro state and one or two neighboring states. Those species are likely to be even more endangered than Allagoptera arenaria, A. caudescens, Attalea humilis, and Bactris vulgaris as those last four ones have a wider distribution, although their potential distribution in Rio de Janeiro state has been quite reduced by deforestation. The first important point toward the conservation of these data deficient species would be the collection of more specimens and their geographical records.
By applying the land-use filter, we showed how forest loss resulted in a drastic reduction in suitable areas for species that depend on this type of habitat. Unfortunately, many forest areas in Rio de Janeiro state have already been converted to anthropic habitats (e.g., agriculture/pasture and urban areas) that are not suitable to most of the palm species studied here. The disparity in forest cover on a landscape scale has a significant effect on the composition of palm species in forest remnants (Benchimol et al., 2017). Landscapes with a high degree of forest cover are inhabited mainly by forest-interior species, while landscapes with reduced forest cover are inhabited by open-area species. The use of land-use filters highlights remnant areas without legal protection with potentially high species richness which are important for conservation efforts and to enable connectivity between forest remnants that are already protected. The unprotected area located between Três Picos and Desengano State Parks, in the central portion of the state, is worthy of special attention, as it encompasses a large area of conserved forest with high suitability for several palm species (Supplementary Figure S2). A study highlights the importance of this region for an endangered species of primate, the southern muriqui (Brachyteles arachnoides) (Honorato, 2020). A forest corridor has been proposed to connect these PAs and thus increase the conservation value of this region (Grelle et al., 2021). Besides that, this region has a huge potential of restoring the Atlantic Forest as rural landowners need to comply with the law (Grelle et al., 2021). We recognize the difficulties in establishing protected areas and restoring native vegetation, but protecting this specific area can highly increase the effectiveness of palm species conservation as well as benefit many other species. This is one of few large forest remnants that may disappear in future due to the lack of protection. Another possible option is to create governmental incentives for the conversion of private properties to private nature reserves in this region.
By using the SDM approach, we were able to identify (a) the PAs that most contribute to the conservation of the Arecaceae in the Atlantic Forest of Rio de Janeiro state (i.e., Pedra Branca State Park and Serra da Tiririca State Park); (b) the PAs that might have been poorly studied and where Arecaceae richness is probably underestimated (e.g., Guaxindiba Ecological Station); (c) need of increasing efforts in collecting palm specimens in the field, especially of those four palm species (A. apoda, S. macrocarpa, S. weddelliana, and S. insignis) with restricted distribution for which the SDM could not be done; (d) species that have lost a great part of their potential distribution range to deforestation and might be endangered (e.g., Allagoptera arenaria); and (e) unprotected forest remnants that might harbor considerable Arecaceae richness. These results demonstrate the usefulness of the SDM in informing conservation decisions. The information presented here, combined with other available data, can contribute to more effective conservation planning and priority setting in terms of species and PAs under a current scenario of rapid loss of biodiversity and limited financial resources.
Data availability statement
The original contributions presented in the study are included in the article/Supplementary material, further inquiries can be directed to the corresponding author.
Author contributions
RP and GO worked together in the bibliographic review. TL and GO worked together on the species distribution models. EM and TL conducted the analyses of landscape ecology. All authors conceived and designed the study, as well as participated in writing the manuscript.
Acknowledgments
We thank Andrea Sánchez Tapia for her important comments and suggestions during the early phase of this project, Ana Cristina Petry for her help with the multivariate analyzes, and the Conselho Nacional de Desenvolvimento Científico e Tecnológico (CNPq) and the Coordenação de Aperfeiçoamento de Pessoal de Nível Superior (CAPES) for the fellowships granted: TL received a PIBIC/CNPq fellowship (137109/2018-0), GO received a CNPq fellowship (88887.476060/2020-00), and EM received a CAPES fellowship (88882.424935/2019-01).
Conflict of interest
The authors declare that the research was conducted in the absence of any commercial or financial relationships that could be construed as a potential conflict of interest.
Publisher's note
All claims expressed in this article are solely those of the authors and do not necessarily represent those of their affiliated organizations, or those of the publisher, the editors and the reviewers. Any product that may be evaluated in this article, or claim that may be made by its manufacturer, is not guaranteed or endorsed by the publisher.
Supplementary material
The Supplementary Material for this article can be found online at: https://www.frontiersin.org/articles/10.3389/ffgc.2022.928446/full#supplementary-material
References
Aiello-Lammens, M. E., Boria, R. A., Radosavljevic, A., Vilela, B., and Anderson, R. P. (2015). spThin: an R package for spatial thinning of species occurrence records for use in ecological niche models. Ecography 38, 541–545. doi: 10.1111/ecog.01132
Allouche, O., Tsoar, A., and Kadmon, R. (2006). Assessing the accuracy of species distribution models: prevalence, kappa and the true skill statistic (TSS). J. Appl. Ecol. 43, 1223–1232. doi: 10.1111/j.1365-2664.2006.01214.x
Araújo, M. B., and News, M. (2007). Ensemble forecasting of species distributions. Trends Ecol. Evol. 22, 42–47. doi: 10.1016/j.tree.2006.09.010
Arroyo-Rodríguez, V., Saldana-Vazquez, R. A., Fahrig, L., and Santos, B. A. (2017). Does forest fragmentation cause an increase in forest temperature? Ecol. Res. 32, 81–88. doi: 10.1007/s11284-016-1411-6
Baker, W., Norup, M. V., Clarkson, J. J., Couvreur, T. L. P., Dowe, J. L., Lewis, C. E., et al. (2011). Phylogenetic relationships among arecoid palms (Arecaceae: Arecoideae). Ann. Bot. 108, 1417–1432. doi: 10.1093/aob/mcr020
Baumgratz, J. F. A., Coelho, M. A. N., Peixoto, A. L., Mynssen, C. M., Bediaga, B. E. H., Costa, D. P., et al. (2014). Catálogo das Espécies de Plantas Vasculares e Briófitas do Estado do Rio de Janeiro. Instituto de Pesquisas Jardim Botânico do Rio de Janeiro. Available online at: http://florariojaneiro.jbrj.gov.br/
Benchimol, M., Talora, D. C., Mariano-Neto, E., Oliveira, T. L., Leal, A., Mielke, M. S., et al. (2017). Losing our palms: the influence of landscape-scale deforestation on Arecaceae diversity in the Atlantic forest. For. Ecol. Manag. 384, 314–322. doi: 10.1016/j.foreco.2016.11.014
Bingham, H. C., Bignoli, D. J., Lewis, E., MacSharry, B., Burgess, N. D., Visconti, P., et al. (2019). Sixty years of tracking conservation progress using the world database on protected areas. Nat. Ecol. Evol. 3, 737–743. doi: 10.1038/s41559-019-0869-3
Brasil (2016). Portaria N° 150, de 10 de maio de 2016. Diário Oficial da União (Seção 1). Available online at: https://www.in.gov.br/web/guest/materia/-/asset_publisher/Kujrw0TZC2Mb/content/id/22804297/do1-2016-05-11-portaria-n-150-de-10-de-maio-de-2016-22804223
Eiserhardt, W. E., Sevenning, J., Kissling, W. D., and Balslev, H. (2011). Geographical ecology of the palms (Arecaceae): determinants of diversity and distribution across spatial scales. Ann Bot. 108, 1391–1416. doi: 10.1093/aob/mcr146
Elith, J., and Leathwick, J. R. (2009). Species distribution models: ecological explanation and prediction across space and time. Ann. Rev. Ecol. Evolut. Systemat. 40, 677–697. doi: 10.1146/annurev.ecolsys.110308.120159
Elmqvist, T., Setala, H., Handel, S. N., van der Ploeg, S., Aronson, J., Blignaut, J. N., et al. (2015). Benefits of restoring ecosystem services in urban areas. Curr. Opin. Environ. Sustain. 14, 101–108. doi: 10.1016/j.cosust.2015.05.001
Fidalgo, E. C. C., Uzêda, M. C., Bergallo, H. G., Costa, T. C. C., and Abreu, M. B. (2009). “Distribuição dos remanescentes vegetais no estado do Rio de Janeiro,” in Estratégias e ações para a conservação da biodiversidade no estado do Rio de Janeiro, eds H. G. Bergallo, E. C. C. Fidalgo, C. F. D. Rocha, M. C. Uzeda, M. B. Costa, M. A. S. Alves, M. Van Sluys, et al. (Rio de Janeiro: Instituto Biomas), 91–99.
Fisch, S. T. V., and Gomes, E. P. C. (2015). “Métodos de amostragem de palmeiras (Arecaceae) e estudo de caso na restinga de Ubatuba, Estado de São Paulo, Brasil,” in the “Fitossociologia no Brasil: Métodos e estudos de caso”, ed V. P. Einsenlohr and J. Felfili (in memoriam), M. M. R. F. Mello, L. A. Andrade, J. A. A. Meira Neto (Viçosa, BR: Editora UFV), 97–118.
Fundação SOS Mata Atlântica INPE-Instituto Nacional de Pesquisas Espaciais (2015). Atlas dos Remanescentes Florestais da Mata Atlântica: Período 2014-2015. São Paulo. Available online at: http://www.sosma.org.br (accessed March 20, 2020).
Galetti, M., Guevara, R., Côrtes, M. C., Fadini, R., Von Matter, S., Leite, A. B., et al. (2013). Functional extinction of bird drives rapid evolutionary changes in seed size. Science 340, 1086–1090. doi: 10.1126/science.1233774
Genini, J., Galetti, M., and Morellato, L. P. C. (2009). Fruiting phenology of palms and trees in an Atlantic rainforest land-bridge island. Flora 204, 131–145. doi: 10.1016/j.flora.2008.01.002
Graham, C. H., and Hijmans, R. J. (2006). A comparison of methods for mapping species ranges and species richness. Glob. Ecol. Biogeogr. 15, 578–587. doi: 10.1111/j.1466-8238.2006.00257.x
Grelle, C. E. V., Niemeyer, J., Viveiros de Castro, E. B., Lanna, A. M., Uzeda, M., and Vieira, M. V. (2021). Sustainability issues in a tropical mega trail. R. Soc. Open Sci. 8, 201840. doi: 10.1098/rsos.201840
Guisan, A., Tingley, R., Baumgartner, J. B., Naujokaitis-Lewis, I., Sutcliffe, P. R., Tulloch, A. I. T., et al. (2013). Predicting species distribution for conservation decisions. Ecol. Lett. 16, 1424–1435. doi: 10.1111/ele.12189
Henderson, A. (2009). Field guide to the palms of Rio de Janeiro State, Brazil. Palms 53, 180–191. doi: 10.2307/j.ctvcszzzd
Honorato, R. S. (2020). Corredores ecológicos na Serra do Mar do Rio de Janeiro entre os Parques Estaduais dos Três Picos e do Desengano. (Master's thesis), Retrieved from Rio de Janeiro Botanical Garden Research Institute - JBRJ, Rio de Janeiro, Brazil. Available online at: http://dspace.jbrj.gov.br/jspui/.
IBGE (2012). Manuais Técnicos em Geociências n° 1: Manual técnico da Vegetação Brasileira, 2ª Ed., revista e ampliada. Rio de Janeiro: IBGE. Available online at: http://www.ibge.gov.br/apps/populacao/projecao (accessed March 20, 2020)
IBGE (2020). Cidades e Estados. Available online at: https://cidades.ibge.gov.br/ brasil/rj/panorama (accessed March 10, 2010).
INEA-Instituto Estadual do Ambiente (2016). Governo do estado do Rio de Janeiro. Unidades de Conservação da Natureza (Uc's). Available online at: http://www.inea.rj.gov.br (accessed March 20, 2020).
IUCN (2012). IUCN Red List Categories and criteria: version 3.1. 2nd Edn. Available online at: https://www.iucnredlist.org/resources/categories-and-criteria (accessed March 10, 2010).
Jenkins, C. N., Alves, M. A. S., and Pimm, S. L. (2010). Avian conservation priorities in a top-ranked biodiversity hotspot. Biol. Conserv. 143, 992–998. doi: 10.1016/j.biocon.2010.01.014
Köppen, W. (1948). Climatologia: Con un Estudio de los Climas De La Tierra. México: Fondo de Cultura Econômica.
Laurance, W. F., Sayer, J., and Cassman, K. G. (2014). Agricultural expansion and its impacts on tropical nature. Trends Ecol. Evol. 29, 107–116. doi: 10.1016/j.tree.2013.12.001
Leitman, P., Henderson, A., Noblick, L., and Martins, R. C. (2012). “Arecaceae,” in Lista de espécies da flora do Brasil. Available online at: http://floradobrasil.jbrj.gov.br/jabot/floradobrasil/FB53 (accessed March 10, 2010).
Lindenmayer, D., Thorn, S., and Banks, S. (2017). Please do not disturb ecosystems further. Nat. Ecol. Evol. 1, 1–3. doi: 10.1038/s41559-016-0031
McCune, B., and Grace, J. B. (2002). Analysis of Ecological Communities. Gleneden Beach, OR: MjM Software Design.
MMA (2022). Ministério do Meio Ambiente, Brasil. Rio de Janeiro: Municípios Costeiros. Available online at: https://antigo.mma.gov.br/informma/item/10599-rio-de-janeiro-munic%C3%ADpios-da-zona-costeira.html (accessed March 20, 2020).
Muscarella, R., Emilio, T., Phillips, O. L., Lewis, S. L., Slik, F., Baker, W. J., et al. (2020). The global abundance of tree palms. Glob. Ecol. Biogeogr. 29, 1–20. doi: 10.1111/geb.13123
Newbold, T., Hudson, L. N., Hill, S. L. L., Contu, S., Lysenko, I., Senior, R. A., et al. (2015). Global effects of land use on local terrestrial biodiversity. Nature 520, 45–50. doi: 10.1038/nature14324
Oksanen, J., Blanchet, F. G., Friendly, M., Kindt, R., Legendre, P., McGlinn, D., et al. (2019). vegan: Community Ecology Package. R package version 2.5.4.
Oliveira, K. F., Fisch, S. T. V., Duarte, J. S., Danelli, M. F., Martins, L. F. S., and Joly, C. A. (2014). Estrutura e distribuição especial de populações de palmeiras em diferentes altitudes na Serra do Mar, Ubatuba, São Paulo, Brasil. Rodriguésia 65, 1043–1055. doi: 10.1590/2175-7860201465414
Olson, D. M., Dinerstein, E., Wikramanayake, E. D., Burgess, N. D., Powell, G. V. N., Underwood, E. C., et al. (2001). Terrestrial ecoregions of the world: a new map of life on Earth. BioScience 51, 933–938. doi: 10.1641/0006-3568(2001)051[0933:TEOTWA]2.0.CO;2
Pearson, R. G., Raxworthy, C. J., Nakamura, M., and Townsend, P. A. (2007). Predicting species distributions from small numbers of occurrence records: a test case using cryptic geckos in Madagascar. J. Biogeogr. 34, 102–117. doi: 10.1111/j.1365-2699.2006.01594.x
Potapov, P., Hansen, M. C., Laestadius, L., Turubanova, S., Yaroshenko, A., Thies, C., et al. (2017). The last frontiers of wilderness: Tracking loss of intact forest landscapes from 2000 to 2013. Sci. Adv. 3, e1600821. doi: 10.1126/sciadv.1600821
Projeto MapBiomas (2018). Coleção 4.1 da Série Anual de Mapas de Cobertura e Uso de Solo do Brasil. Available online at: http://mapbiomas.org (accessed March 10, 2010).
Qiao, H., Soberón, J., and Peterson, A. T. (2015). No silver bullets in correlative ecological niche modelling: insights from testing among many potential algorithms for niche estimation. Methods Ecol. Evol. 6, 1126–1136. doi: 10.1111/2041-210X.12397
Reis, R. C. C. (2006). Palmeiras (Arecaceae) das Restingas do Estado do Rio de Janeiro, Brasil. Acta bot. bras.20, 501–512. doi: 10.1590/S0102-33062006000300001
Rezende, C. L., Scarano, F. R., Assad, E. D., Joly, C. A., Metzger, J. P., Strassburg, B. B. N., et al. (2018). From hotspot to hopespot: an opportunity for the Brazilian Atlantic Forest. Perspect. Ecol. Conserv. 16, 208–214. doi: 10.1016/j.pecon.2018.10.002
Ricotta, C., and Podani, J. (2017). On some properties of the Bray-Curtis dissimilarity and their ecological meaning. Ecol. Complex 31, 201–205. doi: 10.1016/j.ecocom.2017.07.003
Rocha-Santos, L., Pessoa, M. S., Cassano, C. R., Talora, D. C., Orihuela, R. L. L., Mariano-Netos, E., et al. (2016). The shrinkage of a forest: landscape-scale deforestation leading to overall changes in local forest structure. Conserv. Biol. 196, 1–9. doi: 10.1016/j.biocon.2016.01.028
Sánchez-Tapia, A., Siqueira, M. F., Lima, R. O., Barros, F. S. M., Gall, G. M., Gadelha, Jr., et al. (2017). “Model-R: a framework for scalable and reproducible ecological niche modeling. in High Performance Computing CARLA, ed E. Mocskos and S. Nesmachnow (Cham: Communications in Computer and Information Science; Springer), 796.
Santos, J. P., Sobral-Souza, T., Brown Jr, K. S. et al. (2020). Effects of landscape modification on species richness patterns of fruit-feeding butterflies in Brazilian Atlantic Forest. Divers. Distrib. 26, 196–208. doi: 10.1111/ddi.13007
Senay, S. D., Worner, S. P., and Ikeda, T. (2013). Novel three-step pseudo-absence selection technique for improved species distribution modelling. PLoS ONE. 8, e71218. doi: 10.1371/journal.pone.0071218
Silva, J. M. C., and Casteleti, C. H. M. (2003). “Status of the biodiversity of the Atlantic Forest of Brazi,” in The atlantic forest of South America: Biodiversity Status, Threats, and Outlook, ed C. Galindo-Leal and I. G. Câmara (Washington, DC: CABS and Island Press).
Silva, T. M. (2002). A estruturação geomorfológica do Planalto Atlântico no Estado do Rio de Janeiro (Doctoral dissertation). Geosciences Institute, Federal University of Rio de Janeiro, Rio de Janeiro, Brazil.
Soanes, K., and Lentini, P. E. (2019). When cities are the last chance for saving species. Front. Ecol. Environ. 17, 225–231. doi: 10.1002/fee.2032
Solano, E., and Feria, T. P. (2007). Ecological niche modeling and geographic distribution of the genus Polianthes L. (Agavaceae) in Mexico: using niche modeling to improve assessments of risk status. Biodivers Conserv. 16, 1885–1900. doi: 10.1007/s10531-006-9091-0
Thuiller, W., Lafourcade, B., Engler, R., and Araújo, M. B. (2009). BIOMOD - A platform for ensemble forecasting of species distributions. Ecography. 32, 369–373. doi: 10.1111/j.1600-0587.2008.05742.x
Title, P. O., and Bemmels, J. B. (2017). ENVIREM: an expanded set of bioclimatic and topographic variables increases flexibility and improves performance of ecological niche modeling. Ecography 41, 291–307. doi: 10.1111/ecog.02880
UNEP-WCMC IUCN (2016). Protected Planet Report 2016 - How protected areas contribute to achieving global targets for biodiversity. Cambridge: Gland. Amvailable online at: https://wdpa.s3.amazonaws.com/Protected_Planet_Reports/2445%20Global%20Protected%20Planet%202016_WEB.pdf (accessed March 10, 2010).
Veloso, H. P., Rangel Filho, A. L. R., and Lima, J. C. A. (1991). Classificação da Vegetação Brasileira, Adaptada a um Sistema Universal. Rio de Janeiro: IBGE.
Vitousek, P. M., Mooney, H. A., Lubchenco, J., and Melillo, J. M. (1997). Human domination of Earth's ecosystems. Science 277, 494–499. doi: 10.1126/science.277.5325.494
Werneck, M. S., Sobral, M. E. G., Rocha, C. T. V., Landau, E. C., and Stehmann, J. R. (2011). Distribution and endemism of angiosperms in the Atlantic Forest. Natureza Conservação 9, 188–193. doi: 10.4322/natcon.2011.024
Wisz, M. S., Hijmans, R. J., Li, J., Peterson, A. T., Graham, C. H., Guisan, A., et al. (2008). Predicting species distributions working group. Effects of sample size on the performance of species distribution models. Divers. Distrib. 14, 763–773. doi: 10.1111/j.1472-4642.2008.00482.x
Keywords: species distribution modeling, palm inventory, state management, forest fragmentation, endangered species
Citation: de Lima TM, Portela RDCQ, Mendes ETB and Oda GA (2022) Species distribution modeling allied with land-use reveal priority sites and species for palm (Arecaceae) conservation in Rio de Janeiro, Brazil. Front. For. Glob. Change 5:928446. doi: 10.3389/ffgc.2022.928446
Received: 25 April 2022; Accepted: 24 June 2022;
Published: 25 July 2022.
Edited by:
Rakan Zahawi, University of Hawaii at Manoa, United StatesReviewed by:
Miriam San José, Charles Darwin Foundation, EcuadorLaio Zimermann Oliveira, Regional University of Blumenau, Brazil
Copyright © 2022 de Lima, Portela, Mendes and Oda. This is an open-access article distributed under the terms of the Creative Commons Attribution License (CC BY). The use, distribution or reproduction in other forums is permitted, provided the original author(s) and the copyright owner(s) are credited and that the original publication in this journal is cited, in accordance with accepted academic practice. No use, distribution or reproduction is permitted which does not comply with these terms.
*Correspondence: Gabriela Akemi Oda, gabrielaakemioda@gmail.com
†Present Address: Thales Moreira de Lima, Laboratory of Evolutionary Ecology and Plant Genomics, Department of Plant Biology, Biology Institute, State University of Campinas, Campinas, Brazil