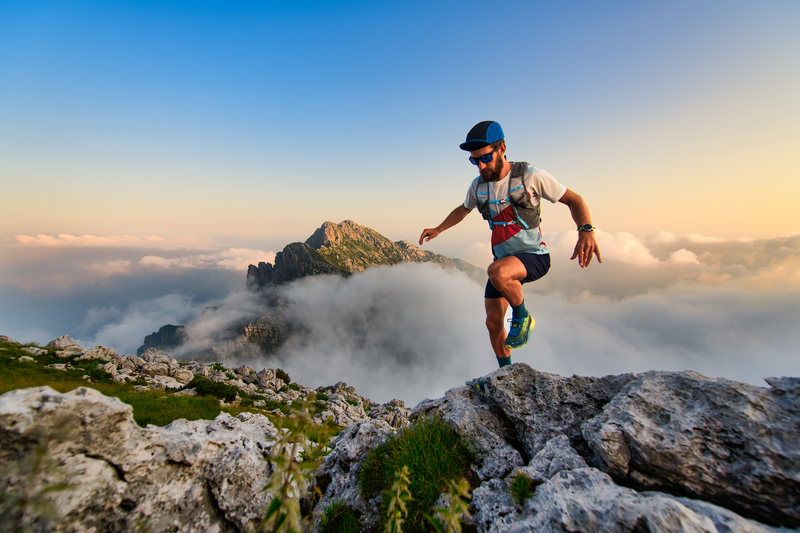
94% of researchers rate our articles as excellent or good
Learn more about the work of our research integrity team to safeguard the quality of each article we publish.
Find out more
ORIGINAL RESEARCH article
Front. For. Glob. Change , 20 June 2022
Sec. Pests, Pathogens and Invasions
Volume 5 - 2022 | https://doi.org/10.3389/ffgc.2022.872584
This article is part of the Research Topic Forest Pathology in Changing Climate View all 10 articles
Climate change (CC) conditions projected for many temperate areas of the world, expressed by way of excessive temperatures and low water availability, will impact forest health directly by means of abiotic stress but also by predisposing trees to pathogenic attack. However, we do not yet know how such environmental conditions alter the physiology and metabolism of trees to render them more susceptible to pathogens. To explore these mechanisms, we conditioned 3-year-old Austrian pine saplings to a simulated CC environment (combined drought and elevated temperatures), followed by pathogenic inoculation with two sister fungal species characterized by contrasting aggressiveness, Diplodia sapinea (aggressive) and D. scrobiculata (less aggressive). Lesion lengths resulting from infection were measured after 3 weeks to determine phenotypes, while dual transcriptomics analysis was conducted on tissues collected from the margins of developing lesions on separate branches 72 h post inoculation. As expected, climate change conditions enhanced host susceptibility to the less aggressive pathogen, D. scrobiculata, to a level that was not statistically different from the more aggressive D. sapinea. Under controlled climate conditions, D. sapinea induced suppression of critical pathways associated with host nitrogen and carbon metabolism, while enhancing its own carbon assimilation. This was accompanied by suppression of host defense-associated pathways. In contrast, D. scrobiculata infection induced host nitrogen and fatty acid metabolism as well as host defense response. The CC treatment, on the other hand, was associated with suppression of critical host carbon and nitrogen metabolic pathways, alongside defense associated pathways, in response to either pathogen. We propose a new working model integrating concurrent host and pathogen responses, connecting the weakened host phenotype under CC treatment with specific metabolic compartments. Our results contribute to a richer understanding of the mechanisms underlying the oft-observed increased susceptibility to fungal infection in trees under conditions of low water availability and open new areas of investigation to further integrate our knowledge in this critical aspect of tree physiology and ecology.
The elevated temperatures predicted under climate change scenarios can directly impact plant physiology. The effects are expressed mostly through exacerbation of water limitation due to elevated vapor pressure deficits, which impose extra demands on the water relation capabilities of trees (Adams et al., 2009; Williams et al., 2013). Under sub-lethal drought conditions, physiological processes other than carbon starvation or interruption of water conduction can render droughted plants more susceptible to mortality from pathogen attack. For example, plants often respond by reducing photosynthesis and growth, accumulating compatible solutes (osmoprotectants) such as the amino acid proline (Pro), producing reactive oxygen species (ROS), and altering specialized metabolism, among other processes (Chaves et al., 2003; Bhargava and Sawant, 2013).
One system-level question emerges from this conceptual framework: “How does climate change-associated stress affect the internal environment of a tree to predispose it to fungal infection?” This is currently one of the top 10 unanswered questions in plant-pathogen interactions (Harris et al., 2020).
Tree pathosystems, such as those involving Diplodia spp. and other Botryosphaeriaceae, represent a large class of emerging diseases caused by opportunistic fungi that mostly ‘sit-and-wait’ as asymptomatic endophytes, becoming highly destructive necrotrophs only under certain host and environmental conditions (Herre et al., 2007; Slippers and Wingfield, 2007), such as low water availability, that predispose the tree to infection (Blodgett et al., 1997a,b). However, the molecular and metabolic mechanisms underlying stress-induced tree susceptibility to pathogens remain poorly understood. This inhibits predictions of how tree pathosystems will behave and evolve under projected climate change scenarios. System-level studies of the main players will address this deficiency (Bostock et al., 2014). Our work to date using the Austrian pine (Pinus nigra) – D. sapinea pathosystem has addressed basic mechanisms underlying host susceptibility under normal growth (Blodgett and Bonello, 2003; Luchi et al., 2005; Wang et al., 2006; Eyles et al., 2007; Barto et al., 2008; Wallis et al., 2008, 2011; Sherwood and Bonello, 2013, 2016) as well as under drought conditions. Under relatively severe drought, we have observed alterations in (1) levels of some free phenolics, lignin, and terpenoids; (2) Pro metabolism; (3) ROS homeostasis; and (4) possible alteration in programmed cell death (PCD), and fungal capacity to neutralize/take advantage of host responses (Sherwood et al., 2015).
Our aim in this study was to investigate how climate change affects the interactions between host and opportunistic pathogens like Diplodia spp. To do so, we subjected 3-year-old Austrian pine saplings to simulated climate change conditions of combined reduced water availability and elevated temperatures. After a period of conditioning, we inoculated the saplings and monitored infection processes using two closely related species of contrasting aggressiveness: D. sapinea (aggressive) and D. scrobiculata (non-aggressive) (Blodgett and Bonello, 2003; De Wet et al., 2003). To gain insight into system-level processes, we conducted transcriptomic analyses of simultaneous host and pathogen responses. We hypothesized that any shifts in aggressiveness (or, conversely, host susceptibility) due to climate change conditions would be more evident with D. scrobiculata and be explained by differential dual (host and pathogen) gene expression in the preliminary stages of infection.
Open pollinated, 3-year-old potted Austrian pine saplings were received from Willoway Nurseries (Madison, OH, United States). Trees were maintained in an ornamental nursery yard in the Dept. of Horticulture and Crop Sciences at The Ohio State University (Columbus, OH, United States). After repotting trees into a potting mix consisting of equal parts of Com-Til compost (provided by Department of Public Utilities, city of Columbus, OH, United States), pine bark, and organic matter (the latter primarily comprised of composted yard waste) trees were transferred to the greenhouse for several months of growth.
Three weeks before inoculation, trees were transferred to two growth chambers for acclimation. We imposed the following conditions (more details in Supplementary Information SI1):
Control treatment (CT): daily minimum of 15°C and maximum of 28°C (corresponding to a 16/8 h light/dark cycle), and RH of 60% throughout, to generate a VPD of 0.7 and 1.5 kPa1 during dark and light, respectively.
CC treatment (CCT): daily minimum of 20°C and maximum of 33°C (corresponding to a 16/8 h light/dark cycle), and RH of 60% throughout, to generate a VPD of 0.9 and 2.0 kPa during dark and light, respectively. Pre-dawn needle water potentials were measured with a Scholander pressure bomb (PMS Instrument Co., Corvallis, OR, United States).
Our experimental design was constrained by logistical limitations imposed by the availability of growth chambers: one for the CT and one for the CCT. To account for variation between growth compartments (Potvin, 2000), we used a split-plot design with combined heat/drought treatment (the CCT) as the main plot factor (represented by individual growth chambers) and inoculation state (i.e., mock-inoculated and plants inoculated with D. sapinea or D. scrobiculata) and sampling time (i.e., 12 and 72 h, and 3 weeks post-inoculation) as the subplot factors (Potvin, 2000). Each inoculation treatment was replicated six times, for a total of 18 trees in each chamber.
On February 17, 2017, trees were inoculated with D. sapinea or D. scrobiculata, or mock inoculated. Isolates of D. sapinea (Sherwood and Bonello, 2013) and D. scrobiculata (Santamaria et al., 2011) were obtained from Dr. Glenn Stanosz of University of Wisconsin Madison and grown and maintained on potato dextrose agar (PDA) in the dark at room temperature. Each treated shoot was lightly wounded by removing a small (<5 mm × 5 mm) area of epidermis, using a razor blade, 3–4 cm distal to the transition point between previous and current year growth. Inoculum (or just PDA growth medium in the case of mocks) was placed on the wound, mycelium side down, and the inoculation court was then wrapped with parafilm. Each tree was randomly assigned to a single inoculation treatment (mock, D. sapinea or D. scrobiculata) and randomly treated on six different branches (two branches per time point). The whole inoculation process was conducted over the course of 5 h. No uninoculated controls were used due to growth chamber space availability imposing limitations on numbers of usable plants.
Shoots were sampled at 12, 72 h (3 days), and 3 weeks for transcriptomics and dissected at 3 weeks for determination of relative susceptibility (phenotype) based on lesion lengths. All mock inoculations resulted in no lesions and were excluded from determination of relative susceptibility. Lesions from D. sapinea and D. scrobiculata-infected branches on each tree were averaged and the means used as a single data point for that tree. Differences in lesion length between D. sapinea and D. scrobiculata were analyzed separately for CT and CCT trees using two-tailed t-tests assuming unequal variances, as the number of available biological replicates was n = 3–6.
We did not have the resources to conduct RNA-seq analysis at all time points, so we decided to analyze host and fungal responses at 72 h, which we deemed the best compromise to uncover significant genes in the interactions. Based on all our work for the past 18 years on this system, we did not expect any detectable systemic effects of multiple, concurrent inoculations on different shoots of the same tree over the local host responses expressed in each shoot.
At sampling time, shoots were removed with pruners, placed individually into paper coin envelopes, and immediately flash frozen in liquid N before transfer to −80°C until processing. Subsequently, tissues were harvested to contain either the whole lesion on CT saplings, or the margin of the much longer lesions on CCT saplings, by collecting shavings obtained with a liquid N-chilled razor blade and liquid N-chilled tweezers. Shavings were first dropped into a 50 ml Falcon tube containing liquid N, and then transferred to pre-weighed 2-ml screw-capped Eppendorf tubes, which were then dropped into liquid N. The tubes were transferred to cardboard freezer boxes and stored at −80°C.
Tissue collected 72 h post-inoculation was ground in liquid nitrogen using a mortar and pestle that were pre-chilled in liquid N. Ground tissue was returned to the sampling tube and weight determined before storage at −80°C. RNA extraction was carried out following a standard protocol (Chang et al., 1993) modified for pine samples (500 ul extraction buffer preheated at 65°C, sample in buffer incubated at 65°C for an hour), from approximately three biological replicates of each treatment combination. RNA extracts were then treated with DNaseI, amplification grade per the manufacturer’s instructions (Invitrogen, Thermo Fisher Scientific, Waltham, MA, United States) and cleaned-up with the RNeasy Plant Mini Kit per manufacturer’s instructions (Qiagen, Germantown, MD, United States). Samples were quantified spectrophotometrically using a NanoDrop (Thermo Fisher Scientific, Wilmington, DE, United States) and quality and concentration were assessed using TapeStation (Agilent Technologies, Inc., Santa Clara, CA, United States). Samples with 260/280 nm and 260/230 nm ratios between 1.8–2.2 and 1.6–2.2, respectively, were considered of sufficient purity. RNA library preparation for dual transcriptome analyses of host and pathogen was carried out and paired-end sequences were generated via Illumina NextSeq500 platform at the Molecular and Cellular Imaging Center, The Ohio State University, Wooster, OH, United States.
The workflow used here is shown in Supplementary Figure 2 (Supplementary Information SI1). Briefly, all samples were multiplexed and sequenced across four lanes. To exclude lane biases, sequenced reads were mapped to the Pinus tecunumanii transcriptome (Visser et al., 2018) using kallisto v0.44.0 (Bray et al., 2016) and expression data were clustered. Subsequently, reads from all lanes were concatenated for each sample. Concatenated reads were trimmed for adaptors and low-quality bases with Trimmomatic v0.36 (Bolger et al., 2014) and those with a trimmed length >40 were retained. Concatenated and filtered read quality was assessed using FastQC2. To generate a P. nigra reference transcriptome, a series of preliminary transcriptomes was assembled with transABYSS v2.0.1 (Robertson et al., 2010), using trimmed read data with a k-mer range of 21 to 51 with a step of 2 and 55 to 75 with a step of 4, and Trinity v2.4.0 (Grabherr et al., 2011), with a k-mer range of 21 to 31 with a step of 2 using trimmed data as well as in silico normalized trimmed reads both de novo and genome guided against the P. taeda v2.01 genome assembly3. The preliminary assemblies were combined, and the resulting superset of transcripts was run through the Evidential genes pipeline (Gilbert, 2016) to reduce redundancy and select for optimally assembled transcripts, producing a set of putative host unigenes.
As an unannotated D. sapinea reference genome was available, pathogen unigenes were predicted using AUGUSTUS v3.3.3 (Stanke et al., 2008) on the Diplodia sapinea genome (Van Der Nest et al., 2014) using the Aspergillus fumigatus training annotation as well as Diplodia corticola cDNA (ENA accession PRJNA325745) to improve the prediction. Both pine and fungal unigenes were annotated using EnTAP v0.8.2 (Hart et al., 2020) with GeneMarkS-T v5.1 (Tang et al., 2015), diamond v0.8.31 (Buchfink et al., 2014) and eggnog v0.12.7 (Huerta-Cepas et al., 2016). Annotation parameters used were minimum query coverage = 80, minimum target coverage = 60, and minimum e-value = 1.0e–05. BLASTp similarity search alignments were performed against the RefSeq complete protein (release 87), UniProt/SwissProt-KB (2018-04) and NCBI non-redundant (nr, 2018-04) databases for both species, as well as the TAIR10 proteome for pine. For the host, the final reference unigenes were produced by removing sequences with best BLASTp hits from the following taxa as contaminants: Fungi, Bacteria, Insecta, Opisthokonta, Archaea, Viruses and Alveolata. The pine and Diplodia proteomes were further annotated using GhostKOALA (Kanehisa et al., 2016) to add KEGG orthology (KO) numbers and Mercator (Lohse et al., 2014) was used to annotate the Austrian pine proteome for analysis with MapMan (Thimm et al., 2004).
Host (from the final reference transcriptome assembly) and pathogen (predicted from the D. sapinea reference genome) unigenes were concatenated to produce a combined reference transcriptome. Kallisto v0.44.0 (Bray et al., 2016) was used to map the concatenated sequenced reads and quantify expression against the combined reference for each sample. Kallisto output was imported into R v4.0.4 (R Core Team, 2021) using tximport v1.22.0 (Soneson et al., 2015) to separate host and pathogen mapped read sets and perform expression analysis. Transcripts that were only represented in mock samples were removed and only those transcripts, which had at least 20 reads in 3 or more samples in each dataset, were retained.
Host expression was analyzed using DESeq2 v1.34.0 (Love et al., 2014). Filtered count data were read into a differential expression (DE) object and library sizes were estimated by treatment type. A Poisson distance matrix was generated for the normalized host reads to determine possible clustering of samples by treatment type. A multi-dimensional scaling (MDS) plot was generated to investigate possible correlation by treatment or condition. Analyses of differentially expressed genes (DEGs) were then performed for the following pairwise comparisons of interest: (1) host responses to D. sapinea vs. mock inoculation under CT, (2) host responses to D. scrobiculata vs. mock inoculation under CT, (3) dual responses of host and pathogen following D. sapinea attack vs. D. scrobiculata attack under CT, (4) dual responses of host and pathogen following D. scrobiculata attack under CCT vs. CT (Figure 1).
Figure 1. Pairwise comparisons carried out in this study for estimating differential gene expression. Black arrows indicate comparisons for host only; white arrows indicate both host and pathogen comparisons. CT, control treatment; CCT, climate change treatment; Dsap, inoculated with Diplodia sapinea; Dscr, inoculated with D. scrobiculata; Mock, inoculated with PDA only.
Both DESeq2 v1.34.0 (Love et al., 2014) and edgeR v3.36.0 (Robinson et al., 2010) were used to identify differentially expressed genes for the pathogen comparisons. Filtered counts were read into a DE object and effective library sizes were estimated for each treatment type. An MDS plot was generated to visualize the profile differences between different treatment types (climate conditions and inoculation type) and a mean-variance plot was generated to determine the overall fitness of the model. Pairwise comparisons for DEGs were established as follows: (3) D. sapinea vs. D. scrobiculata during infection of pines under CT, (4) D. scrobiculata during infection of CCT pines vs. CT pines (Figure 1).
To perform GO functional enrichment analysis of DEGs we used a false discovery rate (FDR) p < 0.05 and a threshold of log2 fold change ratio of ±0.5 (∼1× fold change in expression). These filtered DEGs were sorted by EGGNOG annotations and KEGG pathways reconstructed to visualize what genes were affected in global metabolic pathways. However, to investigate biological relevance of the most significant and highly expressed genes for a given comparison we applied a more stringent threshold of log2 fold change ratio of ±2.3 (∼5× fold change in expression).
On February 14, March 1, March 3, and March 8, 2017, needle water potentials averaged −0.1 and −1.3 kPa, −0.3 and −2.0 kPa, −0.3 and −2.0 kPa, and −0.1 and −2.4 kPa, (N = 6 for each mean), for the pines in the CT and CCT chambers, respectively. Differences in water potential were reflected in needle appearance (Figure 2A).
Figure 2. (A) Chlorotic and partly desiccated appearance of pine needles subjected to climate change treatment (left) vs. deep green and turgid needles in the control treatment (right). (B) Lesion lengths (mm) measured 3 weeks post inoculation. X-axis: Dsap, inoculation with D. sapinea; Dscr, inoculation with D. scrobiculata. Blue bars represent the control climate treatment (CT), red bars the climate change treatment (CCT). Error bars: standard error of the mean. Different letters represent significant differences within climate treatment (lower case letters for CT, upper case letters for CCT). CCT: t6 = 3.078, p = 0.015; CT: t8 = 1.189, p = 0.279.
Three weeks post-inoculation, all pines in the CT chamber were alive and had developed lesions in all pathogen inoculated pines. On the other hand, trees in the CCT chamber appeared close to dying with at least half (greater than 50%) of them having at least 1/3 of the crown still green but chlorotic (Figure 2A). In all cases, lesions on CCT saplings were significantly longer than those on CT plants (Figure 2B). Notably, lesions produced by D. scrobiculata in the CCT chamber (Figure 1 in Supplementary Information SI1) were as long as those produced by D. sapinea, i.e., D. scrobiculata became as aggressive as D. sapinea or, conversely, the host became equally susceptible to both pathogens (Figure 2B).
FastQC was used to obtain an overview of general read quality (Bray et al., 2016). Trimmed and filtered reads generated using Trimmomatic (Bolger et al., 2014) retained 539,379,336 of the original 857,867,066 reads (∼63%), ranging between 40 and 150 nucleotides in length. Preliminary k-mer assemblies were produced de novo (n = 33) and genome guided against the P. taeda v2.01 genome (n = 6), followed by concatenation to produce a superset containing 5,246,838 transcripts (Table 1). This superset was further reduced, using the Evidential genes pipeline, to 315,357 transcripts for 30,632 Austrian pine unigenes, while 13,863 D. sapinea unigenes were extracted from the D. sapinea genome using the Augustus gene prediction pipeline (Table 1).
The Austrian pine and D. sapinea unigenes were annotated using EnTAP (Hart et al., 2020). Non-pine sequences as well as unannotated sequences were discarded (Table 1). Annotation resulted in P. nigra v1.0 assembly (Pini_v1.0) containing 19,882 unigenes (8,196 with KEGG Orthology – KO – numbers) and 10,612 D. sapinea unigenes (4,021 with KO numbers). [All raw sequence reads are publicly available in NCBI, and accession information is provided in Supplementary Information SI4.]
Mapping and filtering produced a subset of 17,443 expressed genes for Austrian pine and 2,303 expressed genes for Diplodia spp. The similarity of the host reads was assessed using a Poisson matrix (Figure 3, Supplementary Information SI1); identical treatment types generally showed the closest relationships. Library size ranges were 15–20 million and 0.004–0.3 million reads per sample for the host and pathogen datasets, respectively (Figure 3). The mean-variance plot indicated that the Poisson model was likely inappropriate for the fungi due to higher-than-expected dispersion over the mean (Figure 4, Supplementary Information SI1). Therefore, we generated respective MDS plots for host RNA-seq data and filtered pathogen RNA-seq data, to evaluate clustering based on treatment/species types (Figure 5, Supplementary Information SI1).
Figure 3. Average library sizes retrieved for host and pathogen for all treatment types. Host average library size range: 15–20 million reads; pathogen average library size range: 0.004–0.3 million reads. CT, control treatment; CCT, climate change treatment; Dsap, inoculated with Diplodia sapinea; Dscr, inoculated with D. scrobiculata.
A series of comparisons enabled us to dissect the changes in gene expression patterns associated with key metabolic responses in the host and pathogens under the two climate conditions (Figure 1, Supplementary Information SI2). Specifically, comparisons 1 and 2 in Figure 1 focus on host gene expression resulting from pathogen inoculation under CT, comparison 3 focuses on dual responses of host and pathogen following D. sapinea vs. D. scrobiculata inoculation under CT, while comparison 4 centers on dual responses of host and pathogen following D. scrobiculata inoculation under CCT vs. CT. The volcano plots in Figure 4 provide an overview of the numbers of highly significant DEGs for the four comparisons, which were then investigated by their EGGNOG annotations (Huerta-Cepas et al., 2016), followed by mapping to KEGG (Kanehisa and Sato, 2020) pathways and biological processes through Gene Ontology (GO).
Figure 4. Volcano plots of DEGs for individual host and pathogen comparisons. Upregulated and downregulated genes marked in green and red, respectively, based on actual fold-change >5 (upregulated) or <–5 (downregulated) [i.e., log2 (fold-change) >2.3 (up) or <–2.3 (down)] and p < 0.05. Labels: (A) host comparison 1, (B) host comparison 2, (C) host comparison 3, (D) host comparison 4, (E) pathogen comparison 3, (F) pathogen comparison 4 (Figure 1). CT, control treatment; CCT, climate change treatment; Dsap, Diplodia sapinea; Dscr, D. scrobiculata; Mock, mock inoculated.
We also looked at gene expression overlaps between different host and pathogen comparisons, respectively. We found 2,526 (31%) overlapping DEGs between comparisons 1 and 2, while 405 (5%) DEGs were exclusive to CTDsap vs. CTMock and 2,910 (35%) DEGs were exclusive to CTDscr vs. CTMock (Figure 4). At the same time, 437 (5%) DEGs overlapped between comparisons 2 and 4, with 1,099 (13%) DEGs exclusive to CCTDscr vs. CTDscr (Figure 5). Similarly for pathogen comparisons, we found 12 (6%) DEGs overlapping between comparisons 3 and 4. Comparison 3 produced 40 (21%) DEGs and 135 (72%) DEGs were mapped to CCTDscr vs. CTDscr, respectively (Figure 5). The complete sets of DEGs and their corresponding GO: Biological processes, for individual comparisons of host and pathogen were tested for enrichment using the TopGO package (Alexa and Rahnenfuhrer, 2021). The classic Fisher method was selected for running the enrichment analysis based on quality comparisons with the elimKS method (Figure 6, Supplementary Information SI1). Enriched GO terms are listed in Supplementary Information SI3.
Figure 5. Venn diagram showing unique and overlapping sets of DEGs for host (top) and pathogen (bottom). CT, control treatment; CCT, climate change treatment; Dsap, Diplodia sapinea; Dscr, D. scrobiculata; Mock, mock inoculated.
In this comparison, a total of 3,509 genes were differentially expressed (adj. p-value < 0.05). 2,661 of these were assigned KEGG orthologs and were mapped to various general pathways, while 1,221 were annotated to various GO terms. Out of all 3,509 DEGs, 781 were annotated via all three platforms (i.e., EGGNOG, KEGG, and GO) and were assigned to various metabolic functions/pathways. DEGs were annotated to 32 different GO terms, including response to stimulus (646 DEGs), response to stress (381 DEGs), response to oxygen containing compounds (425 DEGs), response to endogenous stimulus (236 DEGs), response to biotic stimulus (292 DEGs), and defense response (298 DEGs), among other responses (Figure 6A). Our stringent filtering threshold (actual fold-change ±5) produced a list of 512 DEGs, with 308 upregulated genes and 204 downregulated genes that had KEGG and GO annotations (spreadsheet Host-comparison1 in Supplementary Information SI2).
Figure 6. (A) Significant host gene functions annotated using Blast2GO. X-axis: number of differentially expressed genes; y-axis: ontology associated functions. Left: comparison 1; right: comparison 2 (Figure 1). (B) Significant host gene functions annotated using Blast2GO. X-axis: number of differentially expressed genes; y-axis: ontology associated functions. Left: comparison 3; right: comparison 4 (Figure 1). (C) Significant pathogen gene functions annotated using Blast2GO. X-axis: number of differentially expressed genes; y-axis: ontology associated functions. Left: comparison 3; right: comparison 4 (Figure 1). CT, control treatment; CCT, climate change treatment; Dsap, Diplodia sapinea; Dscr, D. scrobiculata; Mock, mock inoculated.
Among 28 DEGs with photosynthetic GO terms, only two were assigned to both KEGG and GO (Figure 7A). Six different transcripts were mapped to fatty acid biosynthesis on KEGG and assigned to fatty acid or lipid metabolism GO terms (Figure 7A).
Figure 7. (A) Most significant DEGs by actual fold-change (Y-axis) >5 (up) or <–5 (down) and p < 0.05, in host metabolic pathways (T = transcript): T1 – ATP synthase delta chain, T2 – photosystem II, T11–T14 – 3-oxoacyl-[acyl-carrier-protein] reductase (fabG), T15 – fatty acid synthase (FAS1), T16 – FAS2, T17–T18 – Stearoyl-[acyl-carrier-protein] 9-desaturase 7, chloroplastic (FAB2), T21 – carbonic anhydrase, T24 – Glutamate dehydrogenase A (gdhA) (Spreadsheet Host-comparison2 in Supplementary Information SI2). (B) T26–T29-calmodulin-like proteins (CML), T31-calmodulin (CALM), T33–T35- pathogenesis related 1 (PR1), T36-WRKY, T37–39-enhanced disease susceptibility (EDS1), T40-elongation factor (efTu), T43–T45-jasmonate domain protein (JAZ), T46–T47-auxin responsive protein, T49-Gibberellin Insensitive 1 (GID1), T50–T52 protein phosphatase 2C (PP2C), T53-coronatine insensitive protein (COI1), T54-age-related resistance-A (ARR-A) protein, T55-serine threonine protein kinase, T56–T57-xyloglucan endotransglycosylase (TCH4), T59-cyclin D3, T61–T62-cinnamoyl alcohol dehydrogenase (CAD), T64–T67-peroxidase, T69-caffeoyl CoA o-methyltransferase, T70–T71-beta glucosidase, T74–T77-4-coumarate CoA ligase, T78-cinnamoyl CoA reductase, T79-putative lysophospholipase, T82–T87-chalcone synthase, T88–T89-flavonoid 3′-monoxygenase, T90-anthocyanidin reductase, T91-acetyl CoA-acetyltransferase, T92-farnesyl pyrophosphate synthetase (Spreadsheet Host-comparison2 in Supplementary Information SI2). (C) T3-a/bccP, T4-ferredoxin, T5–T7-chlorophyll a-b binding protein, T8-fructose-1,6-bisphosphatase, T9-glyceraldehyde-3-phosphate dehydrogenase (GAPD), T12-3-oxoacyl-[acyl-carrier-protein] reductase (fabG), T15-fatty acid synthase (FAS1), T16-FAS2, T17-adaptor protein (fadD), T19–T20-myristoyl-acyl carrier protein thioesterase, T22, T23-carbonic anhydrase, T28, T32-CML, T38-WRKY, T40-efTu, T72, T73-beta-glucosidase, T110, T111-isocitrate dehydrogenase, T112-dihydrolipoyl dehydrogenase, T113-citrate synthase, T114-ATP-citrate synthase subunit, T115-fumarate hydratase, T116-aconitase hydratase, T118-gdhA, T119-alanine aminotransferase, T120-PyrABCN, T123-alcohol dehydrogenase. (D) T41-ROS burst protein, T46-auxin responsive protein, T50-PP2C, T58-TCH4, T68-peroxidase, T69-caffeoyl-CoA o-methyl transferase, T72, T73-beta glucosidase, T80, T81-reductase, T90-anthocyanidin reductase, T93, T94-geranylgeranyl pyrophosphate synthase (GGPPS), T95-Cu transporting ATPase, T96-chitinase, T97-1-aminocyclopropane-1-carboxylate synthase, T98-isocitrate dehydrogenase, T99-T106-glutathione reductase, T107-glutathione peroxidase, T108-Ribonucleotide Reductase Catalytic Subunit M1 (RRM1), T109-ribonucleotide diphosphate (RNDP) reductase, T123-alcohol dehydrogenase (Spreadsheet Host-comparison4 in Supplementary Information SI2). Numeric bar labels indicate actual fold-change of respective transcripts.
Gene ontology terms associated with defense responses, such as oxygen containing compounds (646 DEGs), defense response to fungus (73 DEGs), response to chitin (40 DEGs), cell wall thickening (4 DEGs), and callose deposition (8 DEGs) were identified (Figure 6A). 12 DEGs were mapped to the KEGG pathway for plant-pathogen interactions. Two calcium binding (CML – calmodulin like) proteins associated with cell morphogenesis involved in differentiation and 18 DEGs assigned to GO: calcium-mediated signaling were identified (Figure 6A). Three transcripts were annotated to pathogenesis-related protein 1 (PR1) on KEGG and EGGNOG and assigned GO: defense response, along with a WRKY transcription factor and another transcript annotated to disease resistance (RPS2) (Figure 7B) (Spreadsheet Host-comparison1 in Supplementary Information SI2).
A total of 15 transcripts were mapped to the plant hormone transduction pathway on KEGG, including significant responses for jasmonate ZIM domain-containing (JAZ) proteins, gibberellin receptor (GID1), and auxin responsive GH3 gene (Figure 7B).
The biosynthesis and metabolism of terpenoids were represented in various GO terms such as terpenoid metabolic process (17 DEGs), tetraterpenoid biosynthetic process (5 DEGs), sesquiterpenoid metabolic process (6 DEGs), and diterpenoid metabolic process (9 DEGs) (Figure 6A). We found 7 DEGs mapped to terpenoid backbone biosynthesis on KEGG, 29 DEGs associated with GO: phenylpropanoid metabolic process (Figure 6A), and 11 DEGs mapped to phenylpropanoid biosynthesis pathway on KEGG. These included beta-glucosidase, cinnamyl alcohol dehydrogenase, peroxidase, 4-coumarate-CoA ligase, cinnamoyl-CoA reductase, chalcone synthase, and flavonoid 3′-monooxygenase (Figure 7B) (Spreadsheet Host-comparison1 in Supplementary Information SI2).
The enrichment analysis produced a list of 10 most significant GO terms with respect to both classic Fisher and Kolmogorov-Smirnov elimination tests (Spreadsheet Host-comparison1 in Supplementary Information SI3). The most enriched terms included obsolete oxidation-reduction process, carbohydrate metabolic process, cellular amino acid metabolic process, fatty acid metabolic process, and response to chitin (Figure 7 in Supplementary Figures 7–10).
In this comparison, a total of 6,628 genes were differentially expressed. 2,935 of these were assigned KEGG orthologs and were mapped to various general pathways, while 2,384 were annotated to various GO terms. Out of all 6,628 DEGs, 1,576 were filtered via annotation on all three platforms and mapped to 32 different GO terms including response to stimulus (1,788 DEGs), response to biotic stimulus (286 DEGs), defense response (373 DEGs), carbohydrate metabolic process (352 DEGs), among others (Figure 6A). Our 5-fold threshold produced a filtered list of 2,434 DEGs, with 1,537 upregulated genes and 897 downregulated genes (Spreadsheet Host-comparison2 in Supplementary Information SI2).
A total of 90 DEGs were represented by photosynthesis through GO annotation (Figure 6A), and further identified on KEGG, including ATP synthase (ATPF1D), photosystem II (psb27) protein, and a chlorophyll a-b binding (LHCB7) protein (Spreadsheet Host-comparison2 in Supplementary Information SI2) (Figure 7A). 21 DEGs were mapped to fatty acid biosynthesis process on KEGG, including fatty acid synthase genes, short-chain type dehydrogenase (fabG), and acyl-CoA-synthetase (Spreadsheet Host-comparison2 in Supplementary Information SI2). Under nitrogen metabolism, carbonic anhydrase (CAH) protein and another glutamate dehydrogenase (gdhA) transcript were higher in D. scrobiculata infected pines as compared to mock infected pines (Figure 7A).
A total of 495 DEGs were mapped to various GO terms associated with defense responses by the host, including defense response (373 DEGs), defense response to fungus (54 DEGs), defense response by cell wall thickening (12 DEGs), and callose deposition (14 DEGs) (Figure 6A). 25 DEGs were assigned to the plant-pathogen interaction pathway (Figure 6A), including calcium binding (CML) protein, calmodulin (CALM) protein, elongation factor Tu, heat shock (HSP90A) protein, and WRKY proteins (Figure 7B).
A total of 415 DEGs were assigned to hormone related GO terms, including GO: response to hormone (322 DEGs) and hormone mediated signaling pathway (93 DEGs) and 43 DEGs were mapped to the plant hormone signal transduction pathway (Figure 6A). Most significant DEGs included JAZ proteins, auxin responsive GH3 gene, xyloglucan endotransglucosylase hydrolase (TCH4) protein, gibberellin receptor (GID1) protein, coronatine-insensitive (COI-1) protein, ubiquitin dependent protein catabolic process, two-component response regulator (ARR-A) protein, protein phosphatase 2C, cyclin D3 (CYCD3) protein (Figure 7B).
A total of 32 DEGs were mapped to terpenoid backbone biosynthesis pathway on KEGG (Figure 6A). Most significant DEGs included, acetyl-CoA acetyltransferase and farnesyl pyrophosphate synthetase (FPPS) protein (Figure 7B). We also found 22 transcripts mapped to the phenylpropanoid biosynthesis pathway on KEGG, including cinnamyl alcohol dehydrogenase, peroxidase, reductase, and 4-coumarate-CoA ligase (4CL) (Figure 7B). Further, there were 11 transcripts mapped to flavonoid biosynthesis pathway on KEGG, including chalcone synthase (CHS), flavonoid 3′-monooxygenase (CYP75B1) (Figure 7B).
The enrichment analysis produced a list of 19 most significant GO terms with respect to both classic Fisher and Kolmogorov-Smirnov elimination tests (Spreadsheet Host-comparison2 in Supplementary Information SI3). The most enriched terms included ribosome biogenesis, rRNA metabolic process, response to chitin, and cytosolic transport (Figure 8 in Supplementary Figures 7–10).
In this comparison, a total of 434 genes were differentially expressed. All 434 DEGs were assigned KEGG orthologs and were mapped to various general pathways, but only 172 were annotated to various GO terms. 130 host DEGs were annotated to different metabolic pathways on all three platforms, with higher number of annotations for pathways like response to stimulus (50 DEGs), lipid metabolic process (30 DEGs), nitrogen compound metabolic process (44 DEGs), and carbohydrate metabolic process (38 DEGs) (Figure 6B). Our 5-fold threshold produced a filtered list of 233 genes that were less expressed in hosts infected by D. sapinea relative to D. scrobiculata infected hosts (Spreadsheet Host-comparison3 in Supplementary Information SI2).
Under the citric acid cycle pathway on KEGG and assigned GO: carbohydrate metabolic process, significant DEGs were reported for isocitrate dehydrogenase genes, citrate synthase genes, dihydrolipoyl dehydrogenase, fumarate hydratase, aconitate hydratase (Figure 7C). Under nitrogen metabolism, significant DEGs were annotated as alanine aminotransferase and glutamate dehydrogenase (Figure 7C). Four transcripts mapped to fatty acid biosynthesis pathway on KEGG were also downregulated, including fatty acid synthase, acyl-CoA-synthase, and short chain dehydrogenase (Figure 7C).
Three genes mapped to the plant-pathogen interactions and MAPK signaling pathway on KEGG and assigned GO: receptor mediated endocytosis, nucleotide metabolic process, mitochondrial translation, were downregulated. Of them, calmodulin, serine-threonine protein kinase, elongation factor efTu protein were the most significant DEGs (Figure 7D).
Under plant hormone signal transduction pathway on KEGG, significant DE included xyloglucan endotransglucosylase hydrolase protein (TCH4) and assigned GO: response to external stimulus, plant-type cell wall organization (Figure 7D). Additionally, 9 DEGs were mapped to the glutathione metabolism pathway on KEGG and assigned GO: response to oxidative stress, glutamate metabolic process, NADP metabolic process, including glutathione S-transferase and glutathione reductase (Figure 7D). Others cAMP signaling pathway associated genes such as cell division control protein RAC1, RAS homolog gene family protein RHOA, and a serine threonine protein phosphatase were lower (Figure 7D).
The cinnamoyl alcohol dehydrogenase, mapped to phenylpropanoid and lignin biosynthesis pathways was also lower in D. sapinea infected hosts relative to D. scrobiculata infected hosts (Figure 7D).
The enrichment analysis produced a list of 10 most significant GO terms with respect to both classic Fisher and Kolmogorov-Smirnov elimination tests (Spreadsheet Host-comparison3 in Supplementary Information SI3). The most enriched terms included DNA metabolic process, DNA methylation, DNA duplex unwinding, leaf vascular tissue pattern formation, and phloem or xylem histogenesis (Figure 9 in Supplementary Figures 7–10).
In this comparison, a total of 2,048 genes were differentially expressed. 732 of these were mapped to various KEGG pathways and 679 DEGs assigned to various GO terms and the most represented GO functions included response to stress (561 DEGs), response to nitrogen metabolic process (256 DEGs), carbohydrate metabolic process (209 DEGs), response to oxygen-containing compound (145 DEGs), response to abiotic stimulus (158 DEGs), and defense response (51 DEGs) (Figure 6B). Our 5-fold threshold produced a filtered list of 936 DEGs, with 162 upregulated genes and 674 downregulated genes (Spreadsheet Host-comparison4 in Supplementary Information SI2).
We found that 7 transcripts mapped to KEGG pathways associated with photosynthesis and carbon fixation, including chlorophyll A-B binding protein, ferredoxin protein, phosphoenolpyruvate carboxykinase, fructose-1,6-bisphosphatase, and glyceraldehyde-3-dehydrogenase (Figure 7C). We also found two beta-glucosidase genes downregulated 23-fold and 6-fold, respectively in pines infected with D. scrobiculata under CCT as compared to CT (Figure 7C). We also found 60 genes mapped to GO terms associated with lipid metabolic process, including myristoyl-acyl carrier protein thioesterase, and fatty acyl-CoA reductase (Figure 7C). An alcohol dehydrogenase gene assigned to GO: response to hypoxia, response to abiotic stimulus, was also upregulated (Figure 7C).
Among the key GO terms related to defense, 51 DEGs were assigned to defense response, 101 DEGs assigned to cellular response to stimulus, 158 DEGs for response to abiotic stress, 25 DEGs for defense response to fungus, 70 DEGs for response to biotic stress (Figure 6B). Other important defense associated GO functions included respiratory burst involved in defense, resistance gene-related defense response signaling pathway, defense response by cell wall thickening, and defense response by callose deposition (Figure 6B). We further found 9 DEGs mapped to the plant-pathogen interactions pathway on KEGG. Significant DEGs included 3-ketoacyl-coa synthase, respiratory burst oxidase, calcium binding protein, and WRKY transcription factor (Figures 7C,D).
We also found 117 DEGs assigned GO: response to hormone, 56 DEGs assigned GO: response to abscisic acid (Figure 6B), and 9 DEGs mapped to the plant hormone signal transduction process on KEGG. Significant DEGs included auxin-responsive protein, phosphatase 2C, xyloglucan endotransglucosylase hydrolase, copper-transporting ATPase protein (Figure 7D). Other DEGs mapped under the host mitogen associated protein kinase (MAPK) signaling included respiratory burst oxidase, chitinase and 1-aminocyclopropane -1-carboxylate synthase (ACS6) (Figure 7D). Interestingly, we also found 14 DEGs mapped to the glutathione metabolism pathway, besides 145 DEGs assigned GO: response to oxygen-containing compound and 2 DEGs assigned GO: respiratory burst involved in defense (Figure 6B). Significant DEGs included glutathione S-transferase, and glutathione peroxidase (Figure 7D) (Spreadsheet Host-comparison4 in Supplementary Information SI2).
We also observed 47 DEGs assigned to GO: terpenoid metabolic process (Figure 6B), and 7 transcripts that were mapped to the terpenoid backbone biosynthesis pathway, including geranylgeranyl pyrophosphate synthase (Figure 7C). 30 DEGs were assigned to phenylpropanoid metabolic process (Figure 6B), and 7 DEGs were mapped to the phenylpropanoid and flavonoid biosynthesis pathway on KEGG, including reductase, caffeoyl-CoA o-methyltransferase, peroxidase, and flavonoid 3′-monooxygenase (Figure 7D) (Spreadsheet Host-comparison4 in Supplementary Information SI2).
The enrichment analysis produced a list of 13 most significant GO terms with respect to both classic Fisher and Kolmogorov-Smirnov elimination tests (Spreadsheet Host-comparison4 in Supplementary Information SI3). The most enriched terms included cotyledon vascular tissue pattern formation, alpha-amino acid biosynthetic process, mRNA metabolic process, fatty acid beta-oxidation, and establishment of localization in cell (Figure 10 in Supplementary Figures 7–10).
In this comparison, a total of 211 genes were differentially expressed. Out of these, 156 DEGs were assigned KEGG orthologs and were mapped to various general pathways, and 172 DEGs were annotated to various GO terms. Our 5-fold threshold produced a filtered list of 58 DEGs, with 27 genes more highly expressed, and 21 genes expressed lower in D. sapinea relative to D. scrobiculata (Spreadsheet Pathogen-comparison3 in Supplementary Information SI2). We also found 30 DEGs that were assigned to various GO terms and the most represented GO functions included response to stimulus (50 DEGs), response to nitrogen metabolic process (44 DEGs), carbohydrate metabolic process (38 DEGs), and lipid metabolic process (30 DEGs) (Figure 6C).
We found two transcripts mapped to KEGG pathways for carbon metabolism and biosynthesis of amino acids. Significant DEGs included glyceraldehde-3-phosphate dehydrogenase, and triose phosphate isomerase under various carbon-associated pathways (Table 2). Additionally, a thiamine biosynthesis protein (NMT1) that was assigned GO: nitrogen metabolic process, aromatic compound metabolic process, was also higher (Table 2). Under various lipid associated pathways, a sterol 24-c-methyltransferase transcript, and RING finger transcript were also reported (Table 2).
Table 2. Significant DE genes under various metabolic pathways for pathogen comparison 3 (Figure 1): CTDsap vs. CTDscr.
We also found a gamma-glutamyl transpeptidase transcript annotated to glutathione metabolism pathway on KEGG and assigned GO: response to nitrogen starvation, and elongation factor 2 transcript to be lower in D. sapinea as compared to D. scrobiculata under CT (Table 2).
The enrichment analysis produced a list of 8 most significant GO terms with respect to both classic Fisher and Kolmogorov-Smirnov elimination tests (Spreadsheet Pathogen-comparison3 in Supplementary Information SI3). The most enriched terms included nuclear chromosome segregation, ascospore-type prospore assembly, protein deubiquitination, polyol metabolic process, and response to stress (Figure 11 in Supplementary Figures 11–12).
We documented a total of 147 DEGs significantly affected by the climate treatment. Out of these 147 DEGs, 56 were assigned KEGG orthologs and were mapped to various general pathways, and all were annotated with GO terms. Thereafter, our 5-fold threshold produced a filtered list of 30 DEGs, with 6 upregulated and 24 downregulated by climate change conditions (Spreadsheet Pathogen-comparison4 in Supplementary Information SI2). The same 56 DEGs that had KEGG orthologs were also assigned various GO terms, including nitrogen compound metabolic process (51 DEGs), carbohydrate and its derivative metabolic process (42 DEGs), response to stress (26 DEGs), response to heat (12 DEGs) (Figure 6C).
Various carbon metabolizing enzyme genes such as triose phosphate isomerase, beta-glucosidase, L-iditol 2-dehydrogenase, and cardiolipin synthase were among the most significant (Table 3). We also found 21 DEGs assigned GO: amino acid metabolic process (Figure 6C), including thiamine biosynthesis protein (NMT1), cyanide hydratase, aspartyl-tRNA-synthetase, heat shock protein (HSPA1), large subunit of ribosomal protein L7Ae and small subunit of the ribosomal protein (Table 3). The large subunit was assigned GO: cytoplasmic translation, obsolete mycelium development and the small subunit was assigned GO: RNA export from nucleus, cytoplasmic translation (Table 3), although both subunits are constituents of the EF-Tu protein domain.
Table 3. Significant DE genes under various metabolic pathways for pathogen comparison 4 (Figure 1): CCTDscr vs. CTDscr.
The enrichment analysis produced a list of 11 most significant GO terms with respect to both classic Fisher and Kolmogorov-Smirnov elimination tests (Spreadsheet Pathogen-comparison4 in Supplementary Information SI3). The most enriched terms included ATP metabolic process, cellular amino acid catabolic process, alpha-amino acid catabolic process, electron transport chain, and respiratory electron transport chain (Figure 12 in Supplementary Figures 11–12).
Climate change poses major physiological challenges that can shift the dynamics of tree-pathogen interactions (Desprez-Loustau et al., 2006). The CCT used in this study mimicked possible climate change scenarios that allowed the investigation of how host and pathogens may be impacted by such adverse conditions. While we found clear indications of abiotic stress responses in both host and pathogen, we acknowledge that the responses we documented are on a noticeably short time scale, one that does not consider longer term adaptive responses, both physiological/ecological (habituation) and evolutionary. Even so, we found that a simulated CCT shifted the outcome of the interactions between Austrian pine and the normally non-aggressive fungus D. scrobiculata, resulting in lesions on par with D. sapinea. We speculate either enhanced aggressiveness of the pathogen, increased susceptibility of the host, or both, support the phenotypic findings of past studies (reviewed in Desprez-Loustau et al., 2006).
We dissected the underlying molecular features of this phenomenon via a dual transcriptomics approach. One challenge with this approach is that read recovery rates vary widely between host and pathogen due to substantially lower absolute amounts of pathogen RNA relative to host RNA in infected tissues (Naidoo et al., 2018). We mitigated this challenge in our recovery method for host and pathogen reads by filtering out transcripts that were only represented in mock samples but had no reads in infected samples. We further only retained transcripts that were represented at least 20 times in at least three sample types irrespective of treatment combination (Visser et al., 2019; Hernandez-Escribano et al., 2020).
Within these limits we asked several key questions through different comparisons, considering the host response to the two pathogens separately, the host response under climate change conditions and the pathogen responses under both climate change and control climate. The most significant DEGs were then analyzed under two major categories, primary and specialized metabolism, the latter associated with defense. This eventually resulted in the cellular models presented in Figure 8, one for the host and one for the pathogens. We infer the following conclusions from the main comparisons in the study.
Figure 8. Working models of host (top) and pathogen (bottom) cellular networks of various primary and specialized metabolic pathways. Abbreviations represent GO function and colored arrows indicate respective host and pathogen comparisons. Arrow widths represent arbitrary relative magnitude of changes in significantly expressed DEGs mapped under each pathway for a given host/pathogen comparison. Enriched pathways are marked with asterisks.
Host photosynthetic pathways were suppressed, specifically in D. sapinea infection vs. mock inoculation under CT (comparison 1). The ATP synthase delta chain protein and photosystem II proteins are localized in the chloroplast and are involved in ATP proton pump assembly and PSII (photosystem II) light harvesting complex, respectively. Meanwhile, carbonic anhydrase (CAH) is involved in important cellular functions such as mesophyll carbon dioxide conductance, oxidative stress protection, lipid biosynthesis, and phytohormone mediated signaling (Polishchuk, 2021) but, perhaps more importantly, with ABA-independent stomatal closure (Kolbe et al., 2018). Taken together, this evidence suggests that attack by D. sapinea suppresses photosynthesis, leading to host carbon starvation.
Under CT, and compared directly to D. scrobiculata infection, critical defense responses were suppressed by D. sapinea infection, including calmodulin-like (CML) protein genes that have been associated with HR expression (Chiasson et al., 2005), enhanced resistance to insect pests (Ma et al., 2008), and biotrophic and hemi-biotrophic lifestyles (Leba et al., 2012). Similarly, PR proteins, an inducible and diverse group that accumulate in response to stress and have been demonstrated to be significantly associated with necrotrophic pathogens (Boccardo et al., 2019), were suppressed, as were WRKY transcription factors, which reportedly mediate the cross-talk between salicylic acid and jasmonic mediated defense signaling (Li et al., 2004; Zheng et al., 2006). Finally, pathways associated with biosynthesis of specialized metabolites like phenolics and flavonoids, which have been associated with resistance in this system (Sherwood and Bonello, 2013), were also suppressed. Thus, compared to D. scrobiculata infection, the elicitation of host defense responses by D. sapinea is severely impaired under CT, which explains, at least in part, the baseline difference in aggressiveness between the two pathogens.
Plant defense responses are regulated by the crosstalk between phytohormone pathways. Under CT and upon D. sapinea infection, Austrian pine responded by ramping up crosstalk of various phytohormones, leading primarily to jasmonate-mediated defense signaling, consistent with the basic necrotrophic aspects of this association. Such response was concomitant with a downregulation of auxin and gibberellic acid-responsive genes and an upregulation of PP2C type proteins. Jasmonate ZIM-domain (JAZ) containing proteins have been associated with regulation of jasmonate accumulation via suppression of MYC2 proteins (Chini et al. 2007), while auxin responsive genes are linked to reductions of host defense by suppression of the salicylic acid pathway (Djami-Tchatchou et al., 2020). Gibberellin receptors such as GID1 are crucial for GA signal transduction and have been associated with enhanced susceptibility in host plants via antagonistic crosstalk with jasmonate pathways (Song et al., 2014). Finally, PP2C type proteins have been linked to modulation of defense responses by possible suppression of various target PR proteins and ROS-scavenging enzymes (Zhu et al., 2018).
Our data suggest that D. scrobiculata infection increases metabolism and transport of amino acids and lipids but does not affect photosynthesis, unlike D. sapinea under CT. However, one glutamate dehydrogenase (GDH) transcript was upregulated, suggesting that infection results in enhanced reversible transamination of 2-oxoglutarate to form glutamate (Labboun et al., 2009), which contributes to leaf glutamate homeostasis along with the NADH-GOGAT cycle. The fatty acid biosynthesis pathway was also overall highly induced. Among induced fatty acid enzymes, acyl-CoA dehydrogenase and various short chain fatty acid dehydrogenases catalyze the initial step in respective cycles of fatty acid beta oxidation. Similarly, fatty acid synthases (FAS) catalyze the biosynthesis of various long chain fatty acids from respective precursor acyl-CoAs. Furthermore, xyloglucan endotransglucosylase hydrolase (XTH) activity was enhanced. XTH induces cell growth and extension (Miedes et al., 2014) via increased short chain xyloglucan synthesis (Niraula et al., 2021). Taken together, this evidence suggests that Austrian pine enhances homeostasis and growth processes in response to D. scrobiculata infection under CT, contributing to a positive outcome for the host against this less aggressive pathogen.
Under CT, D. scrobiculata infection elicited a much stronger host defense response compared to D. sapinea infection and the mock treatment via enhancement of PR protein biosynthesis, as well as calcium and jasmonate-mediated defense signaling. This was especially evident by enhanced biosynthesis of coronatine insensitive1 (COI1) proteins, which are critical in almost every step of jasmonate signaling (Katsir et al., 2008). There are further indications of phytohormone cross-talk from enhanced ARR-A proteins that are involved in age-related defense response in coordination with phytohormones such as salicylates, jasmonates, and ethylene (Shah and Zeier, 2013). At the same time, enhanced CALM proteins, along with CMLs, a calcium dependent protein kinase, and calcineurin B-like proteins suggests active regulation of the calcium-calmodulin signaling pathway (Cheval et al., 2013), which has been associated with responses to both biotic and abiotic stress.
Diplodia scrobiculata also induced host defense-associated phenylpropanoid pathways, demonstrated by several significant DEGs. Cinnamoyl alcohol dehydrogenases (CAD) are involved in lignin biosynthesis and have been associated with defense-induced phenylpropanoid metabolism (Logemann et al., 1997; Tronchet et al., 2010). Furthermore, the peroxidase-generated apoplastic oxidative burst contributes to damage associated molecular pattern (DAMP)-elicited immunity (Survila et al., 2016). We also documented an induction in flavonoid and terpenoid biosynthesis. Strong induction of host defenses is likely a key contributor to the less aggressive baseline phenotype exhibited by D. scrobiculata infection under CT.
Diplodia sapinea had more profound effects than D. scrobiculata on host primary metabolism. What appears to be a rapid depletion of carbon, nitrogen, and lipid resources might explain why the host is less able to counter a D. sapinea infection than a D. scrobiculata infection under CT. We observed some overlap in DEGs between biological comparisons 1 and 2, where we investigated host responses to D. sapinea vs. mock and host responses to D. scrobiculata vs. mock inoculation, respectively, under CT. We then used comparison 3 to further dissect differences in dual host and pathogen responses following D. sapinea and D. scrobiculata attacks under CT. While we observed no significant differences in host photosynthetic and carbon fixation pathways, the carbohydrate metabolic process was heavily suppressed in D. sapinea infected hosts compared to D. scrobiculata infected hosts. Specifically, key genes of the Krebs cycle, such as citrate synthase, aconitase hydratase (which catalyzes isomerization of citrate to isocitrate), isocitrate dehydrogenase (which catalyzes conversion of isocitrate to alpha-ketoglutarate and release of carbon dioxide), and fumarate hydratase (which catalyzes conversion of fumarate into malate) were significantly suppressed. Dihydrolipoyl dehydrogenase, which is part of the pyruvate dehydrogenase multienzyme complex that connects cytosolic glycolysis with mitochondrial citrate cycle and acts as an ROS neutralizer (Babady et al., 2007), was also suppressed.
Fatty acid biosynthesis and nitrogen metabolism were also inhibited in Austrian pine inoculated with D. sapinea, compared to D. scrobiculata. Suppressed activity of critical enzymes such as gdhA, alanine aminotransferase, and glutamine aminotransferase (pyrABCN) indicate reduced nitrogen assimilation, possibly as a result of cellular hypoxia (Diab and Limami, 2016). Such processes may be the consequence of stomatal closure induced by D. sapinea and subsequent reduction in photosynthesis and nitrogen assimilation, which ultimately leads to depletion of carbon and nitrogen, and respiratory oxygen.
Finally, we observed reduced host defense responses, as indicated by suppression of CML, efTu, and glutathione reductase. Thus, it appears that reduced assimilation/metabolism of carbon, nitrogen, and fatty acids in Austrian pine, coupled with various suppressed defense responses, contribute to the accelerated baseline pathogenesis observed with D. sapinea vs. D. scrobiculata under CT.
In line with suppression of host carbon and nitrogen assimilation, fungal nitrogen assimilation and carbon metabolism were higher in D. sapinea compared to D. scrobiculata under CT. Specifically, glycolytic enzyme genes such as glyceraldehyde 3-phosphate dehydrogenase (GAPD) and another triose phosphate isomerase were enhanced in D. sapinea compared to D. scrobiculata under CT. At the same time, enhanced nitrogen metabolism (Nmt1 and elongation factor 2) is also indicative of higher activity of D. sapinea compared to D. scrobiculata under CT. In addition, we found enhanced environmental information processing in D. sapinea, as indicated by signaling pathways involved in necrotopsis, AMPK, and plant-pathogen interactions. For instance, a gamma-glutamyltranspeptidase (GGT) is involved in glutathione metabolism and is associated with enhanced transport of amino acids and detoxification of free oxygen radicals (Mehdi et al., 2001). Heat shock proteins also aid in fungal morphogenesis and environmental processing, including hyphal formation and pathogenicity (Tiwari et al., 2015).
Host trees subjected to CCT and further challenged with D. scrobiculata displayed depletion of carbon resources, an outcome (if not a process) similar to the situation with D. sapinea under CT. This was evident from suppression of pigments involved in photoexcitation of chlorophyll, to enzymatic genes involved in the Calvin cycle, as well as starch and sucrose metabolism. Additionally, we also documented suppressed carbonic anhydrase activity, similar to D. sapinea infected hosts in comparison 3. Furthermore, enhanced upstream enzymes of host fatty acid metabolism, such as biotin carboxyl carrier protein (a/bccp) and an alcohol dehydrogenase, indicated synthesis of short chain fatty acids; however, fatty acid elongation and branching was affected as indicated by suppressed myristoyl-acyl carrier protein thioesterases. Suppressed fatty acid biosynthesis suggests impairment of membrane integrity and lipid transport, which contribute to stress responses (Michaud and Jouhet, 2019). Thus, climate change conditions, as implemented here, cause suppression of carbon fixation and metabolism leading to carbon starvation, compounding the effects of D. scrobiculata infection and resulting in enhanced pathogenesis.
In D. scrobiculata infected hosts under CCT, reduced defense responses were indicated by suppression of CML and WRKY, in addition to enzymes in the phenylpropanoid biosynthesis pathway, such as caffeoyl-CoA-o-methyl transferase and beta glucosidases, and geranylgeranyl pyrophosphate synthases (GGPS) in the terpenoid biosynthesis pathway. Additionally, we report enhanced MAPK signaling and oxidative burst, as implied by reduced glutathione metabolism, all indicative of host responses to abiotic stress. Similarly to D. sapinea infected hosts under CT, the PP2C family proteins were also induced in D. scrobiculata infected hosts under CCT, indicating direct host defense suppression. Reduced glutathione metabolism, by means of suppressed glutathione and ribonucleotide diphosphate reductase (RNDP) activities, further indicates reduced ROS detoxification and lowered nitrogen transport in Austrian pine under CCT. Thus, climate change conditions, as implemented here, result in suppression of host defense responses.
Comparison 4, in which we investigated dual responses of host and pathogen following D. scrobiculata attack under CCT vs. CT, also revealed important patterns in gene expression in D. scrobiculata itself. For example, we found evidence of enhanced carbon metabolism by way of triose phosphate isomerase and enolase gene upregulation. Amino acid metabolism was enhanced as indicated by upregulation of a glutamine synthetase gene, in addition to the triose phosphate isomerase gene. Interestingly, the glutamine synthetase transcript was also mapped to the necroptosis pathway and has been reported to induce susceptibility via nitrogen competition between host and pathogen (Huang et al., 2017). We also documented enhanced protein processing and stress responses, as indicated by upregulation of heat shock (HSP) proteins and an aminoacyl-tRNA synthase gene. Taken together, this evidence suggests that the focus of pathogen metabolism is to acquire carbon and nitrogen, while lowered lipid metabolism could be a response to the climate change conditions as implemented in this study.
All in all, our work highlights some major themes that facilitate a deeper understanding of pine-pathogen interactions under variable climate. We synthesize our results in two cellular models, one for the host and one for the two pathogens (Figure 8). The comparisons arranged in panels of Figures 6A–C, 7A–D highlight host responses to the two pathogens vs. the mock and, more importantly, two different scenarios of host susceptibility, one including the baseline response to D. sapinea infection under CT, the other host responses to D. scrobiculata infection under CCT. The first observation is that maintaining primary metabolism homeostasis is key for survival of both the host and the pathogens. Infection by D. sapinea induces suppression of host carbon fixation and metabolism, fatty acid biosynthesis, and nitrogen metabolism, thereby leading to primary nutrient starvation (Figure 8). This is further supported by enhanced carbon and nitrogen metabolism in D. sapinea itself (Figure 8), which likely further contributes to host starvation. Moreover, suppressed host fatty acid metabolic pathways likely affect membrane integrity and vesicular trafficking, thereby influencing host response to stress (Michaud and Jouhet, 2019). On the other hand, suppressed lipid metabolism and steroid biosynthesis in D. sapinea perhaps suggests reduced activity of lipid transporters as well as reduced membrane trafficking (Rizzo et al., 2019). In contrast, infection by the less aggressive D. scrobiculata did not alter host carbon fixation, while nitrogen metabolism and fatty acid biosynthesis were enhanced (Figure 8) along with indications of active growth and homeostasis in hosts. Thus, the impaired state of host carbon fixation and metabolism is one of the primary explanations for the higher aggressiveness of D. sapinea, while primary metabolism of carbon, nitrogen, and fatty acids either remain unaffected or are enhanced.
Suppressed primary metabolism in P. nigra also likely contributes to the limitation of carbon-based defenses (phenolics, terpenoids), as well as ROS signaling. Indeed, host defense activation against D. sapinea was on a much lesser scale, relative to D. scrobiculata, under the baseline conditions of CT (Figure 8). For example, phenylpropanoid biosynthesis, which is at the core of defense-associated phenolics and flavonoids (Sherwood and Bonello, 2013), was much more pronounced in hosts under attack by D. scrobiculata than D. sapinea (Figure 8). Furthermore, terpenoid biosynthesis in D. scrobiculata-infected hosts was enhanced, whereas D. sapinea infection did not induce any changes (Figure 8). Interestingly, on the pathogen side, the plant pathogen interactions pathway was more enhanced and GO enriched in D. sapinea than in D. scrobiculata (Figure 8). Thus, the state of specialized metabolism on both sides of the interaction supports a view in which D. sapinea elicits global impairment of host metabolism affecting assimilation, growth and defense response via carbon and nitrogen starvation, explaining the occurrence of longer lesions under CT.
The picture changed dramatically under the climate change regimen for D. scrobiculata-infected hosts, which clearly experienced suppression of carbon fixation, starch and sucrose metabolism, nitrogen metabolism, and fatty acid biosynthesis, in a manner similar to that of hosts attacked by D. sapinea under control climate. These pathways were also GO enriched, further highlighting how CCT led to depletion of host resources and increased D. scrobiculata aggressiveness (Figure 8). Likewise, CCT induced suppression of phenylpropanoid biosynthesis, terpenoid biosynthesis, and defense response pathways in the host (Figure 8). This was accompanied by suppression of ROS and hormone signaling pathways. On the pathogen side, while carbon metabolism and nitrogen metabolism were both GO enriched and enhanced, activity of phenylpropanoid metabolism was lower under CCT (Figure 8), possibly due to feedback from low production of phenylpropanoids in the starving host. Concurrently, we recorded a glutamine synthetase transcript mapped to the necroptosis pathway in D. scrobiculata under CCT, like D. sapinea infected hosts under CT. Also, CCT appears to affect D. scrobiculata via reduced MAPK signaling and post-translational protein processing, suggesting some impairment of host immune signaling (Figure 8). This suggests that CCT causes genome-wide suppression of various critical host secondary metabolic pathways either directly or via suppression of primary metabolism, thereby predisposing hosts to pathogenic infections.
Taken together, our evidence shows how critical carbon and nitrogen are for sustenance of cellular integrity and operation in plant pathogen interactions, no matter what the environmental conditions. Nitrogen and carbon mobilization and transport are highly responsive to both biotic and abiotic stress, and the cumulative stress from the climate change regime and pathogenic infection further aggravates host resource depletion and thus the ability to fight off infection. This appears to ultimately explain the altered lesion phenotypes under CCT. While informative, a study like ours points to metabolic pathways being affected; however, direct measurements of metabolites are necessary to determine how CC-associated stress affects the internal environment of the tree host at a system level to predispose trees to fungal infection.
The data presented in this study are deposited in the NCBI repository with Bioproject accession number PRJNA825834.
PB and JS ideated, designed, and planned the project. PB secured the funding. AC, BK, and VV contributed to experimental execution. SG, EV, SN, and MS conducted the gene expression analyses. SG and PB wrote the first drafts. AC, JS, EV, SN, and MS contributed to the editing, revisions, and final draft. All authors contributed to the article and approved the submitted version.
This work was funded by Ohio Agricultural Research Development Center SEED grant No. 2016-007 to PB and by other state and federal funds appropriated to The Ohio State University, College of Food, Agricultural, and Environmental Sciences, Ohio Agricultural Research and Development Center. This work was supported in part by the United States Department of Agriculture Forest Service. JS was supported by the National Science Foundation DEB-1638999.
The use of trade names is for the information and convenience of the reader and does not imply official endorsement or approval by the USDA or the Forest Service of any product to the exclusion of others that may be suitable.
The authors declare that the research was conducted in the absence of any commercial or financial relationships that could be construed as a potential conflict of interest.
All claims expressed in this article are solely those of the authors and do not necessarily represent those of their affiliated organizations, or those of the publisher, the editors and the reviewers. Any product that may be evaluated in this article, or claim that may be made by its manufacturer, is not guaranteed or endorsed by the publisher.
The authors wish to thank Caterina Villari for help with initial set up, Michael Kelly for providing the in-chamber CO2 system, and Katie D’Amico-Willman and Carrie Fearer for their assistance with RNA extraction.
The Supplementary Material for this article can be found online at: https://www.frontiersin.org/articles/10.3389/ffgc.2022.872584/full#supplementary-material
Adams, H. D., Guardiola-Claramonte, M., Barron-Gafford, G. A., Villegas, J. C., Breshears, D. D., Zou, C. B., et al. (2009). Temperature sensitivity of drought-induced tree mortality portends increased regional die-off under global-change-type drought. Proc. Natl. Acad. Sci. U S A 106, 7063–7066. doi: 10.1073/pnas.0901438106
Alexa, A., and Rahnenfuhrer, J. (2021). topGO: Enrichment Analysis for Gene Ontology. R Package Version 2.46.0. Available online at: https://bioconductor.org/packages/release/bioc/html/topGO.html
Babady, N. E., Pang, Y.-P., Elpeleg, O., and Isaya, G. (2007). Cryptic proteolytic activity of dihydrolipoamide dehydrogenase. Proc. Natl. Acad. Sci. 104, 6158–6163. doi: 10.1073/pnas.0610618104
Barto, E. K., Enright, S., Eyles, A., Wallis, C. M., Chorbadjian, R., Hansen, R., et al. (2008). Effects of soil fertility on systemic protein defense responses of Austrian pine to attack by a fungal pathogen and an insect defoliator. J. Chem. Ecol. 34, 1392–1400.
Bhargava, S., and Sawant, K. (2013). Drought stress adaptation: metabolic adjustment and regulation of gene expression. Plant Breed. 132, 21–32.
Blodgett, J. T., and Bonello, P. (2003). The aggressiveness of Sphaeropsis sapinea on Austrian pine varies with isolate group and site of infection. Forest Pathol. 33, 15–19.
Blodgett, J. T., Kruger, E. L., and Stanosz, G. R. (1997a). Effects of moderate water stress on disease development by Sphaeropsis sapinea on red pine. Phytopathology 87, 422–428. doi: 10.1094/PHYTO.1997.87.4.422
Blodgett, J. T., Kruger, E. L., and Stanosz, G. R. (1997b). Sphaeropsis sapinea and water stress in a red pine plantation in central Wisconsin. Phytopathology 87, 429–434. doi: 10.1094/PHYTO.1997.87.4.429
Boccardo, N. A., Segretin, M. E., Hernandez, I., Mirkin, F. G., Chacón, O., Lopez, Y., et al. (2019). Expression of pathogenesis-related proteins in transplastomic tobacco plants confers resistance to filamentous pathogens under field trials. Sci. Rep. 9:2791.
Bolger, A. M., Lohse, M., and Usadel, B. (2014). Trimmomatic: a flexible trimmer for Illumina sequence data. Bioinformatics 30, 2114–2120. doi: 10.1093/bioinformatics/btu170
Bostock, R. M., Pye, M. F., and Roubtsova, T. V. (2014). Predisposition in plant disease: exploiting the nexus in abiotic and biotic stress perception and response. Ann. Rev. Phytopathol. 52, 517–549. doi: 10.1146/annurev-phyto-081211-172902
Bray, N. L., Pimentel, H., Melsted, P., and Pachter, L. (2016). Near-optimal probabilistic RNA-seq quantification. Nat. Biotechnol. 34, 525–527.
Buchfink, B., Xie, C., and Huson, D. H. (2014). Fast and sensitive protein alignment using DIAMOND. Nat. Methods 12, 59–60. doi: 10.1038/nmeth.3176
Chang, S., Puryear, J., and Cairney, J. (1993). A simple and efficient method for isolating RNA from pine trees. Plant Mol. Biol. Reporter 11, 113–116. doi: 10.1385/MB:19:2:201
Chaves, M. M., Maroco, J. P., and Pereira, J. S. (2003). Understanding plant responses to drought - from genes to the whole plant. Funct. Plant Biol. 30, 239–264. doi: 10.1071/FP02076
Cheval, C., Aldon, D., Galaud, J.-P., and Ranty, B. (2013). Calcium/calmodulin-mediated regulation of plant immunity. Mol. Cell Res. 1833, 1766–1771. doi: 10.1016/j.bbamcr.2013.01.031
Chiasson, D., Ekengren, S. K., Martin, G. B., Dobney, S. L., and Snedden, W. A. (2005). Calmodulin-like proteins from Arabidopsis and tomato are involved in host defense against Pseudomonas syringae pv. tomato. Plant Mol. Biol. 58, 887–897. doi: 10.1007/s11103-005-8395-x
Clifford, M. J., Royer, P. D., Cobb, N. S., Breshears, D. D., and Ford, P. L. (2013). Precipitation thresholds and drought-induced tree die-off: insights from patterns of Pinus edulis mortality along an environmental stress gradient. N. Phytol. 200, 413–421. doi: 10.1111/nph.12362
De Wet, J., Burgess, T., Slippers, B., Preisig, O., Wingfield, B. D., and Wingfield, M. J. (2003). Multiple gene genealogies and microsatellite markers reflect relationships between morphotypes of Sphaeropsis sapinea and distinguish a new species of Diplodia. Mycol. Res. 107, 557–566. doi: 10.1017/s0953756203007706
Desprez-Loustau, M. L., Marcais, B., Nageleisen, L. M., Piou, D., and Vannini, A. (2006). Interactive effects of drought and pathogens in forest trees. Ann. Forest Sci. 63, 597–612.
Diab, H., and Limami, A. M. (2016). Reconfiguration of N Metabolism upon Hypoxia Stress and Recovery: roles of Alanine Aminotransferase (AlaAT) and Glutamate Dehydrogenase (GDH). Plants 5, 2–25. doi: 10.3390/plants5020025
Djami-Tchatchou, A. T., Harrison, G. A., Harper, C. P., Wang, R., Prigge, M. J., Estelle, M., et al. (2020). Dual role of auxin in regulating plant defense and bacterial virulence gene expression during Pseudomonas syringae PtoDC3000 pathogenesis. Mol. Plant-Microb. Interact. 33, 1059–1071. doi: 10.1094/MPMI-02-20-0047-R
Eyles, A., Chorbadjian, R., Wallis, C. M., Hansen, R. C., Cipollini, D. F., Herms, D. A., et al. (2007). Cross-induction of systemic induced resistance between an insect and a fungal pathogen in Austrian pine over a fertility gradient. Oecologia 153, 365–374. doi: 10.1007/s00442-007-0741-z
Grabherr, M. G., Haas, B. J., Yassour, M., Levin, J. Z., Thompson, D. A., and Amit, I. (2011). Full-length transcriptome assembly from RNA-Seq data without a reference genome. Nat. Biotechnol. 29, 644–652. doi: 10.1038/nbt.1883
Harris, J. M., Balint-Kurti, P., Bede, J. C., Day, B., Gold, S., Goss, E. M., et al. (2020). What are the top 10 unanswered questions in molecular plant-microbe interactions? Mol. Plant-Microb. Interact. 33, 1354–1365. doi: 10.1094/MPMI-08-20-0229-CR
Hart, A. J., Ginzburg, S., Xu, M., Fisher, C. R., Rahmatpour, N., Mitton, J. B., et al. (2020). EnTAP: bringing faster and smarter functional annotation to non-model eukaryotic transcriptomes. Mol. Ecol. Resour. 20, 591–604. doi: 10.1111/1755-0998.13106
Hernandez-Escribano, L., Visser, E. A., Iturritxa, E., Raposo, R., and Naidoo, S. (2020). The transcriptome of Pinus pinaster under Fusarium circinatum challenge. BMC Genom. 21:28. doi: 10.1186/s12864-019-6444-0
Herre, E. A., Mejia, L. C., Kyllo, D. A., Rojas, E., Maynard, Z., Butler, A., et al. (2007). Ecological implications of anti-pathogen effects of tropical fungal endophytes and mycorrhizae. Ecology 88, 550–558. doi: 10.1890/05-1606
Huang, H., Nguyen Thi, Thu, T., He, X., Gravot, A., Bernillon, S., et al. (2017). Increase of fungal pathogenicity and role of plant glutamine in nitrogen-induced susceptibility (NIS) to rice blast. Front. Plant Sci. 8:265. doi: 10.3389/fpls.2017.00265
Huerta-Cepas, J., Szklarczyk, D., Forslund, K., Cook, H., Heller, D., Walter, M. C., et al. (2016). EGGNOG 4.5: a hierarchical orthology framework with improved functional annotations for eukaryotic, prokaryotic and viral sequences. Nucleic Acids Res. 44, D286–D293. doi: 10.1093/nar/gkv1248
Kanehisa, M., and Sato, Y. (2020). KEGG Mapper for inferring cellular functions from protein sequences. Protein Sci. 29, 28–35. doi: 10.1002/pro.3711
Kanehisa, M., Sato, Y., and Morishima, K. (2016). BlastKOALA and GhostKOALA: KEGG tools for functional characterization of genome and metagenome sequences. J. Mol. Biol. 428, 726–731. doi: 10.1016/j.jmb.2015.11.006
Katsir, L., Schilmiller, A. L., Staswick, P. E., He, S. Y., and Howe, G. A. (2008). COI1 is a critical component of a receptor for jasmonate and the bacterial virulence factor coronatine. Proc. Natl. Acad. Sci. 105, 7100–7105. doi: 10.1073/pnas.0802332105
Kolbe, A. R., Brutnell, T. P., Cousins, A. B., and Studer, A. J. (2018). Carbonic anhydrase mutants in Zea mays have altered stomatal responses to environmental signals. Plant Physiol. 177, 980–989. doi: 10.1104/pp.18.00176
Labboun, S., Tercé-Laforgue, T., Roscher, A., Bedu, M., Restivo, F. M., Velanis, C. N., et al. (2009). Resolving the role of plant glutamate dehydrogenase. I. In vivo real time nuclear magnetic resonance spectroscopy experiments. Plant Cell Physiol. 50, 1761–1773. doi: 10.1093/pcp/pcp118
Leba, L.-J., Cheval, C., Ortiz-Martín, I., Ranty, B., Beuzón, C. R., Galaud, J.-P., et al. (2012). CML9, an Arabidopsis calmodulin-like protein, contributes to plant innate immunity through a flagellin-dependent signalling pathway. Plant J. 71, 976–989. doi: 10.1111/j.1365-313X.2012.05045.x
Li, J., Brader, G. N., and Palva, E. T. (2004). The WRKY70 transcription factor: a node of convergence for jasmonate-mediated and salicylate-mediated signals in plant defense. Plant Cell 16, 319–331. doi: 10.1105/tpc.016980
Logemann, E., Reinold, S., Somssich, I. E., and Hahlbrock, K. (1997). A novel type of pathogen defense-related cinnamyl alcohol dehydrogenase. Biol. Chem. 378, 909–914. doi: 10.1515/bchm.1997.378.8.909
Lohse, M., Nagel, A., Herter, T., May, P., Schroda, M., Zrenner, R., et al. (2014). Mercator: a fast and simple web server for genome scale functional annotation of plant sequence data. Plant Cell Environ. 37, 1250–1258. doi: 10.1111/pce.12231
Love, M. I., Huber, W., and Anders, S. (2014). Moderated estimation of fold change and dispersion for RNA-seq data with DESeq2. Genom. Biol. 15:550. doi: 10.1186/s13059-014-0550-8
Luchi, N., Ma, R., Capretti, P., and Bonello, P. (2005). Systemic induction of traumatic resin ducts and resin flow in Austrian pine by wounding and inoculation with Sphaeropsis sapinea and Diplodia scrobiculata. Planta 221, 75–84. doi: 10.1007/s00425-004-1414-3
Ma, W., Smigel, A., Tsai, Y.-C., Braam, J., and Berkowitz, G. A. (2008). Innate immunity signaling: cytosolic Ca2+ elevation is linked to downstream nitric oxide generation through the action of calmodulin or a calmodulin-like protein. Plant Physiol. 148, 818–828. doi: 10.1104/pp.108.125104
Mehdi, K., Thierie, J., and Penninckx, M. J. (2001). γ-Glutamyl transpeptidase in the yeast Saccharomyces cerevisiae and its role in the vacuolar transport and metabolism of glutathione. Biochem. J. 359, 631–637. doi: 10.1042/0264-6021:3590631
Michaud, M., and Jouhet, J. (2019). Lipid trafficking at membrane contact sites during plant development and stress response. Front. Plant Sci. 10:2. doi: 10.3389/fpls.2019.00002
Miedes, E., Vanholme, R., Boerjan, W., and Molina, A. (2014). The role of the secondary cell wall in plant resistance to pathogens. Front. Plant Sci. 5:358. doi: 10.3389/fpls.2014.00358
Naidoo, S., Visser, E. A., Zwart, L., Toit, Y. D., Bhadauria, V., and Shuey, L. S. (2018). Dual RNA-sequencing to elucidate the plant-pathogen duel. Curr. Iss. Mol. Biol. 27, 127–142. doi: 10.21775/cimb.027.127
Niraula, P. M., Zhang, X., Jeremic, D., Lawrence, K. S., and Klink, V. P. (2021). Xyloglucan endotransglycosylase/hydrolase increases tightly-bound xyloglucan and chain number but decreases chain length contributing to the defense response that Glycine max has to Heterodera glycines. PLoS One 16:e0244305. doi: 10.1371/journal.pone.0244305
Polishchuk, O. V. (2021). Stress-related changes in the expression and activity of plant carbonic anhydrases. Planta 253:58.
Potvin, C. (2000). ““ANOVA: Experimental Layout and Analysis,”,” in Design and analysis of ecological experiments, 2nd Edn, eds S. M. Scheiner and J. Gurevitch (New York: Oxford University Press), 415.
R Core Team (2021). R: A Language and Environment for Statistical Computing. Vienna, Austria: R Foundation for Statistical Computing.
Rizzo, J., Stanchev, L. D., Da Silva, V. K. A., Nimrichter, L., Pomorski, T. G., and Rodrigues, M. L. (2019). Role of lipid transporters in fungal physiology and pathogenicity. Comput. Struct. Biotechnol. J. 17, 1278–1289. doi: 10.1016/j.csbj.2019.09.001
Robertson, G., Schein, J., Chiu, R., Corbett, R., Field, M., Jackman, S. D., et al. (2010). De novo assembly and analysis of RNA-seq data. Nat. Methods 7, 909–912. doi: 10.1038/nmeth.1517
Robinson, M. D., Mccarthy, D. J., and Smyth, G. K. (2010). edgeR: a Bioconductor package for differential expression analysis of digital gene expression data. Bioinformatics 6, 139–140. doi: 10.1093/bioinformatics/btp616
Santamaria, O., Smith, D. R., and Stanosz, G. R. (2011). Interaction between Diplodia pinea and D. scrobiculata in red and jack pine seedlings. Phytopathology 101, 334–339. doi: 10.1094/PHYTO-07-10-0180
Shah, J., and Zeier, J. (2013). Long-distance communication and signal amplification in systemic acquired resistance. Front. Plant Sci. 4:30. doi: 10.3389/fpls.2013.00030
Sherwood, P., and Bonello, P. (2013). Austrian pine phenolics are likely contributors to systemic induced resistance against Diplodia pinea. Tree Physiol. 33, 845–854. doi: 10.1093/treephys/tpt063
Sherwood, P., and Bonello, P. (2016). Testing the systemic induced resistance hypothesis with Austrian pine and Diplodia sapinea. Physiol. Mol. Plant Pathol. 94, 118–125.
Sherwood, P., Villari, C., Capretti, P., and Bonello, P. (2015). Mechanisms of induced susceptibility to Diplodia tip blight in drought-stressed Austrian pine. Tree Physiol. 35, 549–562. doi: 10.1093/treephys/tpv026
Slippers, B., and Wingfield, M. J. (2007). Botryosphaeriaceae as endophytes and latent pathogens of woody plants: diversity, ecology and impact. Fungal Biol. Rev. 21, 90–106.
Soneson, C., Love, M. I., and Robinson, M. D. (2015). Differential analyses for RNA-seq: transcript-level estimates improve gene-level inferences. F1000Research 4:1521. doi: 10.12688/f1000research.7563.2
Stanke, M., Diekhans, M., Baertsch, R., and Haussler, D. (2008). Using native and syntenically mapped cDNA alignments to improve de novo gene finding. Bioinformatics 24, 637–644. doi: 10.1093/bioinformatics/btn013
Survila, M., Davidsson, P. R., Pennanen, V., Kariola, T., Broberg, M., Sipari, N., et al. (2016). Peroxidase-generated apoplastic ROS impair cuticle integrity and contribute to DAMP-elicited defenses. Front. Plant Sci. 7:1945. doi: 10.3389/fpls.2016.01945
Tang, S., Lomsadze, A., and Borodovsky, M. (2015). Identification of protein coding regions in RNA transcripts. Nucleic Acids Res. 43, 1–10. doi: 10.1093/nar/gkv227
Thimm, O., Bläsing, O., Gibon, Y., Nagel, A., Meyer, S., Krüger, P., et al. (2004). MAPMAN: a user-driven tool to display genomics data sets onto diagrams of metabolic pathways and other biological processes. Plant J. 37, 914–939. doi: 10.1111/j.1365-313x.2004.02016.x
Tiwari, S., Thakur, R., and Shankar, J. (2015). Role of Heat-Shock Proteins in Cellular Function and in the Biology of Fungi. Biotechnol. Res. Int. 2015, 1–11. doi: 10.1155/2015/132635
Tronchet, M., Balagué, C., Kroj, T., Jouanin, L., and Roby, D. (2010). Cinnamyl alcohol dehydrogenases-C and D, key enzymes in lignin biosynthesis, play an essential role in disease resistance in Arabidopsis. Mol. Plant Pathol. 11, 83–92. doi: 10.1111/j.1364-3703.2009.00578.x
Van Der Nest, M. A., Bihon, W., De Vos, L., Naidoo, K., Roodt, D., Rubagotti, E., et al. (2014). Draft genome sequences of Diplodia sapinea, Ceratocystis manginecans, and Ceratocystis moniliformis. IMA Fungus 5, 135–140. doi: 10.5598/imafungus.2014.05.01.13
Visser, E. A., Wegrzyn, J. L., Myburg, A. A., and Naidoo, S. (2018). Defence transcriptome assembly and pathogenesis related gene family analysis in Pinus tecunumanii (low elevation). BMC Genom. 19:1–13. doi: 10.1186/s12864-018-5015-0
Visser, E. A., Wegrzyn, J. L., Steenkamp, E. T., Myburg, A. A., and Naidoo, S. (2019). Dual RNA-seq analysis of the pine-Fusarium circinatum interaction in resistant (Pinus tecunumanii) and susceptible (Pinus patula) hosts. Microorganisms 7:315. doi: 10.3390/microorganisms7090315
Wallis, C., Eyles, A., Chorbadjian, R. A., Riedl, K., Schwartz, S., Hansen, R., et al. (2011). Differential effects of nutrient availability on the secondary metabolism of Austrian pine (Pinus nigra) phloem and resistance to Diplodia pinea. Forest Pathol. 41, 52–58.
Wallis, C. M., Eyles, A., Chorbadjian, R., Mcspadden-Gardner, B. B., Hansen, R., Cipollini, D. F., et al. (2008). Systemic induction of phloem secondary metabolism and its relationship to resistance to a canker pathogen in Austrian pine. N Phytol. 177, 767–778. doi: 10.1111/j.1469-8137.2007.02307.x
Wang, D., Eyles, A., Mandich, D., and Bonello, P. (2006). Systemic aspects of host-pathogen interactions in Austrian pine (Pinus nigra): a proteomics approach. Physiol. Mol. Plant Pathol. 68, 149–157.
Williams, A. P., Allen, C. D., Macalady, A. K., Griffin, D., Woodhouse, C. A., Meko, D. M., et al. (2013). Temperature as a potent driver of regional forest drought stress and tree mortality. Nat. Clim. Change 3, 292–297.
Zheng, Z., Qamar, S. A., Chen, Z., and Mengiste, T. (2006). Arabidopsis WRKY33 transcription factor is required for resistance to necrotrophic fungal pathogens. Plant J. 48, 592–605. doi: 10.1111/j.1365-313X.2006.02901.x
Keywords: climate change, Pinus nigra, tree defense, host metabolism, necrotrophic, Diplodia sapinea, Diplodia scrobiculata, fungal metabolism
Citation: Ghosh SK, Slot JC, Visser EA, Naidoo S, Sovic MG, Conrad AO, Kyre B, Vijayakumar V and Bonello P (2022) Mechanisms of Pine Disease Susceptibility Under Experimental Climate Change. Front. For. Glob. Change 5:872584. doi: 10.3389/ffgc.2022.872584
Received: 09 February 2022; Accepted: 16 May 2022;
Published: 20 June 2022.
Edited by:
Jane E. Stewart, Colorado State University, United StatesReviewed by:
Eeva Terhonen, Natural Resources Institute Finland (Luke), FinlandCopyright © 2022 Ghosh, Slot, Visser, Naidoo, Sovic, Conrad, Kyre, Vijayakumar and Bonello. This is an open-access article distributed under the terms of the Creative Commons Attribution License (CC BY). The use, distribution or reproduction in other forums is permitted, provided the original author(s) and the copyright owner(s) are credited and that the original publication in this journal is cited, in accordance with accepted academic practice. No use, distribution or reproduction is permitted which does not comply with these terms.
*Correspondence: Soumya K. Ghosh, Z2hvc2guMTg4QG9zdS5lZHU=
†Present addresses: Anna O. Conrad, USDA Forest Service, Northern Research Station, Hardwood, Tree Improvement and Regeneration Center, West Lafayette, IN, United States; Bethany Kyre, Department of Entomology, University of Kentucky, Lexington, KY, United States; Vinod Vijayakumar, Charles River Laboratories, Columbus, OH, United States
Disclaimer: All claims expressed in this article are solely those of the authors and do not necessarily represent those of their affiliated organizations, or those of the publisher, the editors and the reviewers. Any product that may be evaluated in this article or claim that may be made by its manufacturer is not guaranteed or endorsed by the publisher.
Research integrity at Frontiers
Learn more about the work of our research integrity team to safeguard the quality of each article we publish.