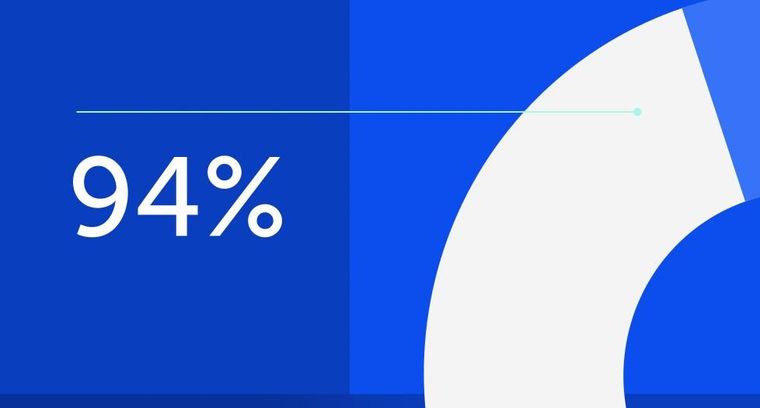
94% of researchers rate our articles as excellent or good
Learn more about the work of our research integrity team to safeguard the quality of each article we publish.
Find out more
ORIGINAL RESEARCH article
Front. For. Glob. Change, 28 March 2022
Sec. Tropical Forests
Volume 5 - 2022 | https://doi.org/10.3389/ffgc.2022.735017
This article is part of the Research TopicTransformation of Tropical Forests Through FireView all 6 articles
More and more, wildfires are raging in large parts of the world due to a warmer climate, more frequent and severe droughts, and continued land-use changes. In Brazil, the weakening of public environmental policies has further aggravated wildfires with widespread impacts across the country. Here, we investigated the determinants of the impact of fire in the Brazilian biomes using a dataset of burned areas between 2001 and 2019 to simulate its future impact under alternative policy and climate scenarios. We began by deriving a fire impact index using a principal component (PC) analysis comprising the variables: 1. fire intensity, 2. fire recurrence, 3. burned area size, 4. mean time interval between successive fires, and 5. predominance of fires in the dry season. We considered as High Impact Fires (HIF) those areas whose values of the first PC were above the 90th percentile. HIF occurred in the Amazon, Cerrado, and Pantanal, but not in the Atlantic Forest, Pampa, and Caatinga biomes. As the main drivers of HIF, our spatial autoregressive models (SAR) (Amazônia R2 = 0.66, Pantanal R2 = 0.86 and Cerrado R2 = 0.79) indicated the climate (Amazon, 25%, Pantanal, 53%, and Cerrado, 56%) together with land-use change (Amazon, 75%, Pantanal, 25%, and Cerrado, 38%). Most HIF occurred in native vegetation remnants (NVR) (55% in the Amazon, 86% in the Pantanal and 94% in the Cerrado), especially in places close to areas deforested over the last two decades. Only in Pantanal fuel loads (dry biomass) play a major role in HIF (22% of explanation). In the Cerrado, it only accounted for 4% of the observed variability and in the Amazon, it was not a significant factor. Over the analyzed period, HIF imposed a loss of 23%, on average, on the NDVI response of the native vegetation in the Amazon, 19% in the Cerrado and 16% in the Pantanal, thus indicating physiological stress. Simulations of future climate and land-use change pointed to a dramatic increase in HIF by 2050. Under the RCP4.5 and strong environmental governance scenario, HIF in the Cerrado would expand from the current 3% of the biome to 15%, from 7 to 8% in the Pantanal and from 0.7 to 1.2% in the Amazon. In addition, the impact of fire would intensify in 95% of the Cerrado, 97% of the Amazon and 74% of the Pantanal. Effective public and private policies will be vital to mitigate the growing threat of HIF. In this sense, our spatially explicit models can help direct prevention and firefighting programs.
The frequency and extent of wildfires are increasing all over the world (Li et al., 2020). Enhanced fire regimes result in more severe events that release a large amount of energy across vast areas in a short time-period, affecting both public and private lands (Pausas and Fernández-Muñoz, 2012; Li et al., 2020; Schmidt and Eloy, 2020). Such fires strongly impact ecosystem services, reducing water and soil quality, impoverishing habitats and biodiversity, and also affecting carbon cycling, the climate (Harper et al., 2018; Pellegrini et al., 2018; Brando et al., 2020) and, consequently, the resilience of terrestrial ecosystems (Pellegrini et al., 2021). Wildfires also cause substantial economic losses by damaging infrastructure, agriculture, and forestry, and compromising water resources and recreation (Mavsar et al., 2013; Carlson et al., 2018; Tedim et al., 2018; Oliveira A.S. et al., 2019). In addition, air pollution from fires poses a serious health hazard (Rappold et al., 2017; Schmidt et al., 2018; Tedim et al., 2018).
Fires, especially those affecting large areas, are in general triggered by human activity under extreme weather conditions. High temperatures and little rainfall, hence low humidity, favor the spread of wildfire fires (Aragão et al., 2008; Matthews et al., 2012; Ganteaume et al., 2013; Chergui et al., 2018; Cullen et al., 2021). The availability of fuel loads (dry biomass) also influences the rate of burning (Matthews et al., 2012; Oliveira U. et al., 2021). While frequently burned areas have lower biomass (i.e., reduced fuel loads), the residual biomass becomes more susceptible to ignition due to lower humidity (Fernandes et al., 2014). Anthropogenic ignition sources are the primary cause of fires (Bowman et al., 2013; Ganteaume and Syphard, 2018), since natural sources, mainly lightning, are less frequent and damaging (Ganteaume and Syphard, 2018). In fact, human action, deliberately or not, accounts for 90% of the ignition sources of large fires (Ganteaume et al., 2013). Fire is also commonly associated with land-use change, given that it is a tool for deforestation and pasture renovation or clearing (Morton et al., 2008). Usually, a fire starts in agricultural or peri-urban areas before spreading to forests and shrublands (Morton et al., 2008). Thus, proximity to agricultural land, roads, villages and urban areas influences forest fire occurrence, especially when managing agricultural areas with fire is a cultural aspect (Carmel et al., 2009; Ganteaume et al., 2013; de Oliveira et al., 2019).
Brazil holds the highest frequency of fire in South America (Li et al., 2020). Among the Brazilian biomes, the Cerrado is the only one whose ecosystems have evolved associated with fire, which plays an important role as an ecological process (Simon and Pennington, 2012; Schmidt and Eloy, 2020). However, large fires have historically devastated vast areas, not only in the Cerrado, but also in the Amazon (Campanharo et al., 2019; Schmidt and Eloy, 2020) and in the Pantanal (Libonati et al., 2020). These three biomes recorded large fires during the dry seasons of 2019 and 2020, although for the Amazon, those dry seasons were not as exceptional as in the droughts of 2005, 2010, and 2015 (Libonati et al., 2020; Schmidt and Eloy, 2020). In 2019, for the first time on record, the smoke from the forest fires in the Amazon reached São Paulo, the largest city in South America, more than 2.7 thousand kilometers to the southeast of the burned regions. And in 2020, one third of the Pantanal biome was burned (Libonati et al., 2020; Schmidt and Eloy, 2020).
In Brazil, large annual fire episodes are historically associated with high rates of deforestation (Schmidt and Eloy, 2020; Silva et al., 2021). Therefore, more important than direct human and environmental factors, these events result from poor or weakened environmental governance. Despite the large fires of 2019 and 2020 associated with higher deforestation rates in the Amazon (Lizundia-Loiola et al., 2020; Schmidt and Eloy, 2020; Silva et al., 2021), the Brazilian government has not instituted any additional public policy to fight forest fires. On the contrary, the 2021 budget proposed for the Ministry of Environment is the lowest on record in the past 21 years. Quite alarming, one of the most substantial cuts went to environmental enforcement and firefighting (Londoño and Casado, 2019), whose federal government funding reduced from BRL 194 million in 2019 to BRL 175 million in 2020 and should be only BRL 127 million in 2021 (Mengassi, 2021). This current logic goes against fire suppression or control policies applied in other countries to reduce the risk of wildfires (Schmidt et al., 2018; Oliveira A.S. et al., 2021). Before the budget cuts, Brazil had shown positive results in reducing burned areas on public and private lands, even with budget expenditures well below those of the Global North countries (Oliveira A.S. et al., 2021). Yet extreme fires are expected to become more prevalent due to climate change (Adams, 2013; Fernandes et al., 2014; Brando et al., 2020). Specifically in Brazil, the weakening of environmental public policies (Abessa et al., 2019), hence rising deforestation rates, tend to further exacerbate wildfire episodes in the next years. In this respect, mapping the history of fire occurrence to understand its driving factors and impacts is essential for guiding regional mitigation programs. For example, environmental factors, such as rain or lack thereof, temperature, fuel loads, wind, and topography, are determinants of fire risk and dynamics (Bowman et al., 2013, 2014). The synergy of these factors with ignition sources from land-use and land practices enables us, as a result, not only to model the risk of fires but also their spatial dynamics (Soares-Filho et al., 2012; Brando et al., 2020).
Here, we investigated the trends in fire events between 2001 and 2019 in the Brazilian biomes, firstly to characterize their impacts, and then to assess the spatial determinants that influence their occurrence and impact. In turn, these results were used as input for modeling the future risk of high impact fires under alternative policy and greenhouse gas emissions scenarios. Our study thus contributes to designing comprehensive fire mitigation strategies for both private and, hopefully, public programs, should the promotion of environmental policies return to the country’s political agenda.
Mapping the occurrence of burned areas helps to determine the historical impact of fires. In general, the most affected areas have a history of (1) more intense and (2) recurrent fires, (3) larger burned areas per event, (4) short time interval between successive fires, and (5) predominance of fires in the dry season (Van Wilgen et al., 2000; Keeley, 2009; Neto et al., 2010; Ryan and Williams, 2010; de Araújo et al., 2012; Pereira et al., 2014; Enright et al., 2015; Prichard et al., 2017; Gomes et al., 2020b). Because these aspects are intrinsically related to the impact of fire, we developed an exploratory analysis using as input a dataset of burned areas between 2001 and 2019 from the Moderate Resolution Imaging Spectroradiometer (MODIS).1 The MODIS monthly composite images, at 500-m spatial resolution, record the area and the date of burning. We used 3,192 images for forming the country mosaic comprising 228 monthly observations. Our methodology consists of the following steps:
1) For each monthly image, we assigned to the pixels of each spatially and temporally contiguous burned area a unique identifier. We then measured each burned area size assigning to all of its pixels the respective area value in hectares. This procedure was repeated for all images to result in a mean area value for each pixel.
2) The previous procedure also enabled us to calculate the frequency, time interval between successive fires, and the season in which the burning occurred.
3) To estimate the mean time interval between successive fires, we assigned to each pixel of a specific burned area, a day value from 1, for first of January 2001, to 6,938 for December 31st of 2019. We then calculated the mean time interval between successive fires for each pixel of burned area.
4) To estimate the prevalence of fires in the dry season, each pixel of a specific burned area received a value for the day of the year (1--366). We then calculated the average day for recurrent fires on the same pixel. To associate the average occurrence day with the dry season, we used the CHELSA monthly average rainfall maps2 (Karger et al., 2020) to establish the driest month and then the middle day of that month (15th day), which was set to 1. As a result, values from 1 to 183 represent the time distance between the driest month’s middle day and the fire occurrence. Thus, the closer the time of burning is to the middle of the dry season, the lower is the value assigned to the pixel.
5) Finally, to estimate fire intensity, we derived the delta Normalized Burn Ratio (dNBR) (Roy et al., 2006) using the MODIS product MOD09A1, version 6. This MODIS product provides an estimate of the surface spectral reflectance corrected for atmospheric conditions, such as gases, aerosols, and Rayleigh scattering. The composite MODIS image at 500 m spatial resolution comprises 8 days of acquisition for cloud removal. Our dataset encompasses 12,222 images covering 873 days of observation. We calculated the dNBR using the image immediately available prior to the date of the burning together with the image just after its occurrence. We then subtracted from values of the former dNBR image the ones of the latter to calculate the burning intensity per unit of burned area.
6) The aforementioned five dimensions were then summarized using a Principal Component (PC) analysis. The first PC accounted for 90% of the data variation, positively correlating with fire intensity, burned area size and number of recurrent events (i.e., frequency), and negatively correlating with mean time interval, and time distance from the dry season. For the analyses described below, we identified the pixels of the first PC with values above the 90th percentile as High Impact Fires (HIF). This limit is arbitrarily set as a way to facilitate the interpretation of results.
Fires can impact native vegetation causing physiological stress (fall of leaves and burnt branches and trunk) or even the death of individuals. To estimate this impact, we derived the Normalized Difference Vegetation Index (NDVI) using our dataset of MODIS product MOD09A1, as described above. NDVI indicates the vegetation’s photosynthetic activity and leaf density (Gamon et al., 1995) as a proxy for its physiological health.
To evaluate whether the native vegetation underwent physiological stress either due to burning in general or HIF, we compared monthly mean NDVI of these areas with those of control areas, in which no fire occurred over the study period (2001–2019) within 50 × 50 km quadrants. This analysis is performed separately for each quadrant to ensure that similar geographic conditions are met and only for native vegetation remnants (NVR), which were extracted from the land-use maps of MapBiomas collection 5 (Souza et al., 2020). We used as samples only the quadrants where all the three conditions were found (never burned, burned at least once and HIF). In each grid cell, we computed the mean NDVI difference by subtracting the monthly mean values of NDVI in burned and HIF areas from those of non-burned areas. Finally, we calculated the monthly means for each biome by averaging the quadrants’ values.
Four major factors determine the impact of fire, i.e., (1) land use and land-use change (LUCC), (2) fuel loads, (3) natural ignition sources, and (4) climate (Bowman et al., 2013). To analyze the influence of these factors in determining the impact of fire, we used the first PC as the dependent variable into a Spatial Autoregressive Model (SAR) (Drukker et al., 2013). SAR includes the effect of the geographic neighborhood in order to remove the spatial dependence between observations, a common problem posed by geographic data. For the SAR method, we used a weighted 8-cell neighborhood matrix as proposed by Tiefelsdorf et al. (1999). To test the relative importance of variables representing the four major factors, we applied a Jackknife test (Efron and Stein, 1981). In order to isolate the effect of individual variables, this test compares the R2 of the full model (with all variables) with those of models with only a subset of variables.
The SAR method uses only continuous variables as input. Therefore, for representing land-use variables, we converted, whenever needed, categorical variables into chorological representation (i.e., a distance map from a cartographic category or discrete feature), selecting the following ones: (1) distance to urban areas, (2) distance to rural areas, (3) distance to deforestation for agricultural use between 2001 and 2019, (4) distance to deforestation for urban use between 2001 and 2019, (5) distance to protected areas, and (6) density of heads of cattle. Variables 1–5 are derived annually from time-series maps of MapBiomas collection 5. Protected areas come from maps.csr.ufmg.br and annual density of heads of cattle is obtained by dividing the number of heads of cattle from Pesquisa Pecuária Municipal (IBGE, 2019) by the respective municipality’s pasture area obtained from MapBiomas.
We built fuel load maps (percentage of dry biomass) using the method by Oliveira U. et al. (2021), which derives the dry biomass content using a combination of infrared and red spectral bands. To do so, we used as input our dataset of MODIS MOD09A1. We then computed all map values for the entire 2001–2019 period to end up with the mean value for each pixel of NVR.
Since the only natural ignition source in Brazil is lightning, as volcanoes are absent and meteors are very rare (Ganteaume and Syphard, 2018), we used a gridded dataset of lighting density from NASA’s Lightning Climatology Collection3 to derive the mean lighting density for the study period.
For representing the climate, we selected within the 2001–2019 study period the mean annual temperature, the maximum annual temperature, the mean annual rainfall and the mean rainfall of the driest month from the CHELSA high-spatial resolution database (see text footnote 2) (Karger et al., 2020).
Among the Brazilian biomes, the Cerrado is the only one in which fire is intrinsic to its ecosystems (Simon and Pennington, 2012). Given that fires caused by natural phenomena, such as lighting, occur mostly in the rainy season, we developed specifically for this biome distinct SAR models for both the rainy and dry season by separating the climate, ignition source and fuel loads variables into their respective seasonal means.
The SAR model allows identifying the influence of each individual factor that determines the impact of fire. However, it is not necessarily the best prediction tool. Other modeling methods, using heuristics or statistical techniques, can yield higher predictive power. On the other hand, some of those methods, namely the ones that use artificial intelligence algorithms, do not explicitly discriminate the contribution of each variable to the model explanation. Thus, together with the SAR, we tested additional four predictive models: Generalized Linear Model (GLM), Generalized Additive Model (GAM), Support Vector Machine (SVM), and Random Forest (RF).
We used the variables indicated as statistically significant by the SAR method (see section “Results”) as input for the four additional methods. Given that the SAR model has detected a strong spatial dependence between observations (see Rho values in section “Results”), we also included as predictive variables the latitude and longitude as a means to solve autocorrelation. As for the dependent variable representing the impact of fire, we randomly sampled 10,000 values within each biome from the map of the first PC.
To test which method would be the best predictive model, we compared the maps from the methods (SAR, GLM, SVM, and RF) with the map of the first PC using Pearson’s correlation. As the RF method yielded the highest correlation with observed data (first PC) (see section “Results”), we used this method to predict the impact of fire under climate change and LUCC scenarios by 2050.
For doing so, we used climate data from the HadGEM2-ES and MIROC5 models under the RCP4.5 and RCP8.5 greenhouse gas emission scenarios, because these projections are closer to the historical trend without more stringent mitigation measures (Chou et al., 2014). We downloaded these data from CHELSA.4 For LUCC scenarios, we used the projections of the Otimizagro model (Soares-Filho et al., 2016; Rochedo et al., 2018) under the Weak Environmental Governance (WEG) and Strong Environmental Governance scenarios (SEG). Projection of heads of cattle comes from Batista et al. (2019). Only fuel loads and lighting density were kept constant. To test the relative influence of the climate and LUCC variables for scenario modeling, we applied the Jackknife test (Efron and Stein, 1981), using as a measure of fitness the correlation between the first PC map and the full model vs. the equivalent correlation values from the models without a particular variable. We developed scenarios modeling for the Amazon, Cerrado and Pantanal only, since the SAR models for the other biomes were not statistically significant. All analyses were performed using the BioDinamica plugin (Oliveira U. et al., 2019) of Dinamica EGO software (Soares-Filho et al., 2013).
By far, the Cerrado is the biome that experienced the largest fire events with areas that burned at least once totaling 72 million ha over the study period. The Amazon comes in second with fire occurring in 38 million ha. In relative terms, the Pantanal biome holds the largest percentage of burned area (45%), followed by the Cerrado (34%), the Amazon (9.0%), Atlantic Forest (8.2%), Caatinga (5.6%), and Pampa (1.8%) (Figure 1). Of these areas, 16.5% were characterized as HIF (first PC values above the 90th percentile) in the Pantanal, 8.8% in the Cerrado, and 6.3% in the Amazon, while HIF did not occur in other biomes (Figure 1). Most of the burned areas were located in NVR in the Pantanal (85%), Cerrado (66%), Caatinga (79%), and almost half in the Amazon (47%). Conversely, only 11% of burned areas occurred in NVR of the Atlantic Forest and 22% of the Pampa (Figure 1). For HIF, most areas were located in NVR, 86% in Pantanal, 94% in Cerrado, and 55% in the Amazon.
Figure 1. Fire impact derived from the first PC. Bar graphs indicate the proportion of burned areas and HIF in native vegetation remnants (NVR) and in relation to the biome’s area.
In the Cerrado, roughly 66% of the land designated as protected area (PA), including conservation units and indigenous territories (Soares-Filho et al., 2010), were burned at least once over the study period, which is equivalent to 6.3% of the burned areas in this biome. Also 29% of HIF occurred within Cerrado’s PAs. We found similar values for the Pantanal, where half of PAs’ expanses burned at least once (4.3% of total burned areas), with 29% of HIF occurring within PAs. Whereas for the Amazon, only 2.5% of PAs’ territory was burned at least once (1.7% of burned areas). HIF was not frequent in the Amazon PAs either, representing only 7.1% of HIF areas. For the Atlantic Forest, Caatinga and Pampa, burned areas within PAs are equivalent to less than 10% of the total burned area (support information S1 and S2). In the Atlantic Forest, 9.7% of the PAs’ territory was burned, in the Caatinga 8.5%, and in the Pampa 9.5%.
The first PC adequately represented the impact of the fire, as it correlated positively with average size of burned areas (r = 0.96), number of fires (r = 0.52), fire intensity (r = 0.49), fires in the dry season (r = 0.29), and negatively with the mean time between successive fires (r = −0.39) (Supplementary Tables 1, 2). Only the first PC was statistically significant, representing 98% of the overall data variation (Figure 1). The highest mean values of the first PC, indicating the impact of the fire, were observed in the Pantanal (479), followed by Cerrado (307), Amazon (198), Caatinga (28), Atlantic Forest (14), and Pampa (6) (Figure 1 and Supplementary Tables 3, 4). As for HIF, pixels with the highest means values are located in the Cerrado (2.638), Amazon (2.594), and Pantanal (2.151). The other biomes did not show HIF (support information S1).
Regular burned areas (moderate or lower impact fire, hereafter named simply burned areas) in the Cerrado showed an average fire recurrence of 2.83 times over the 19 years of the study period, while for HIF, recurrence reached 6.77 times. Mean time between successive fires was 9.93 and 3.29 years for HIF. HIF mostly occurred in September, the end of the dry season. Burned area size averaged 302 ha and 2.6 thousand ha for HIF. In HIF areas, fire intensity was three times greater, on average, than those of burned areas (dNBR = 1.92E-03 and 5.95E-04, respectively).
Similar to the Cerrado, fire recurrence in the Pantanal averaged 2.53 times over the study period, rising to 4.91 times in HIF areas. The mean time between successive fires was 10.82 years in general, reducing to 5.05 years in HIF. Again, HIF mostly occurred in the first half of September. In turn, burned areas size averaged 470 ha and 2.1 thousand ha for HIF. In the HIF areas, fire intensity was twice as high as in burned areas (dNBR = 5.39E-04 and 1.18E-03, respectively). Over the study period, areas that burned at least once totaled 7.2 million ha; only in 2020, wildfires raged across one third of the Pantanal.
For the Amazon, burned areas showed a recurrence of 1.98 and 3.99 years for HIF. Mean time between successive fires was 12.3 years, reducing to 5.3 years in HIF. As for the other former biomes, HIF mostly occurred in the first half of September. Burned area size averaged 193 ha and 2.6 thousand ha for HIF, the largest of all biomes. Fire intensity in HIF was almost three times greater, on average, than those of burned areas (dNBR = 1.04E-03 and 3.73E-04, respectively).
Regarding biomes where HIF did not occur, the Atlantic Forest recorded, on average, 1.69 recurrent fires and 13.5 years as the mean time between successive fires. Burnings also concentrated in the first half of September, averaging in size 9.3 ha, a much smaller value than of those biomes where HIF occurred. In this biome, most burnings concentrated in agricultural areas, mostly pastures, along the ecotone with the Cerrado. In turn, fire intensity (dNBR) reached, on average, 2.26E-04. Over the study period, areas that burned at least once totaled 9.8 million ha in the Atlantic Forest. The Caatinga presented mean of 1.47 years of fire recurrence with mean time interval between successive fires of 14.9 years. Burned areas in this biome consists of patches of 24 ha, on average, with mean fire intensity of 2.03E-04 dNBR, occurring mainly during the first half of October and along the ecotone with Cerrado. Areas that burned at least once in the Caatinga totaled 4.7 million ha over the study period. Finally, the Pampa is the biome with the smallest fire occurrence. In this biome, fires recurred only 1.02 times with mean time interval between successive fires of 18.1 years and burned area size of only 2.3 ha, with dNBR of 4.55E-05, with burnings concentrating in the first half of October. Only about 0.37 million ha of burned areas occurred in this biome over the study period.
NVR in HIF areas, compared with NVR that never burned, showed a striking reduction in photosynthetic activity and leaf density (as measured using the NDVI; Gamon et al., 1995), indicating physiological stress due to recurrent fires (Figure 2). HIF in the Amazon showed the largest reduction (23.2%), followed by HIF areas in Cerrado (18.5%) and Pantanal (16.3%). NDVI reduction in the NVR of the Atlantic Forest reached 14.2%. For typically non-forest vegetation biomes, NDVI in burned NVR in the Pampa presented the largest reduction of 11.3%, while those of Cerrado and Pantanal had reductions of 8.6 and 8.5%, respectively (Figure 2).
Figure 2. Photosynthetic activity (NDVI) in areas that burned at least once. The graphs show the monthly mean NDVI between 2001 and 2019 for nun-burned areas, burned areas and HIF along with the respective reductions from the first.
The SAR model provided a good explanation for the determinants of fire impact in the Pantanal, Cerrado and Amazon biomes. Values of Rho, above 0.78, indicated a strong spatial component, which is consistent with the way fire spreads. Models for the Atlantic Forest, Pampa and Caatinga biomes did not yield statistically significant results, probably due to the smaller and more random occurrence of fires in these biomes.
For the Pantanal, the SAR model (R2 = 0.81 and Rho = 0.91) indicated that climate explained 53% of the model variability, with mean annual temperature and mean annual precipitation accounting for 31 and 22%, respectively. LUCC accounted for 25% of model explanation. Significant variables were distance to PA (12%), distance to NVR (10%), distance to agricultural areas (1%) and density of heads of cattle (1%). In turn, fuel loads accounted for 22% and natural ignition by lightning was not statistically significant (Supplementary Tables 5–7).
SAR model also yielded a good fit for the Cerrado (R2 = 0.72 and Rho = 0.88). Again, climate was the predominant factor accounting for 56% of the model explanation. Mean annual temperature and mean annual precipitation, the only statistically significant variables, accounted for 37 and 19%, respectively. LUCC variables explained the remaining 38%. In the Cerrado, distance to PAs consisted of the main land-use factor driving fire impact, accounting for 13% of model explanation. Conversely to the Amazon, PAs in the Cerrado were strongly associated with fire occurrence. Other statistically significant LUCC variables were density of heads of cattle (7%), distance to deforestation for urban use (7%), distance to deforestation for agricultural use (5%), distance to urban areas (2%), distance to NVR (2%) and distance to agricultural areas (1%). In turn, fuel loads accounted for 4% of model explanation and natural ignition by lightning the remaining 2%.
The two SAR models specifically for the dry and rainy seasons yielded lower fit than that of the year-round model. Whereas the dry season model still presented a satisfactory fit (R2 = 0.50 and Rho = 0.72), including the same variables of those of the year-round model, save natural ignition by lightning, the one for the rainy season was not statistically significant (R2 = 0.07, Rho = 0.31), which may indicate that fires occur randomly in this season (Supplementary Tables 5–7).
For the Amazon, the respective SAR model did not perform so well as those for the previous biomes, but still with a satisfactory fitness (R2 = 0.49 and Rho = 0.78). LUCC accounted for 75% of the model explanation. Again, distance to PA was the primary factor (61%), but conversely to the Cerrado PAs, Amazon PAs showed a strong refractory effect to fire. Density of heads of cattle (13%) and distance to NVR (1%) complemented the LUCC explanation. For climate, only the mean maximum temperature was significant, representing 25% of the model explanation.
The SAR model performed poorly for the Atlantic Forest (R2 = 0.05, Rho = 0.24), Caatinga (R2 = 0.09, Rho = 0.10) and Pampa (R2 = 0.02, Rho = 0.13). As a result, it was not possible to infer the determinants of the impact of fire in these biomes.
The Random Forest (RF) method yielded the highest predictive power for estimating the impact of fire, scoring, on average, 70% higher than the second-best algorithm (Supplementary Table 8). The best forecast was for the Pantanal (R2 = 0.86), and then Cerrado (R2 = 0.79) and Amazon (R2 = 0.66), as measured using the spearman’s correlation with the first PC map. The other biomes were not modeled, given the poor performance of SAR.
The two modeled scenarios differed slightly regarding the impact of fire by 2050. In the RCP4.5-MIROC5 and strong environmental governance scenario, 95% of the Cerrado region would present some increase in the impact of fire. Regions mostly affected would be the west of Cerrado, especially along the ecotones with the Amazon and Pantanal (Figure 3). Although the south of Cerrado would be less affected (Figure 3), there would be an expressive increase in the impact of fire in higher elevations, such as the national parks of Serra do Cipó and Serra da Canastra (Figure 3). HIF in the Cerrado would expand from the current 3% of the biome’s area to 15% by 2050, a fourfold increase (Figure 3). For the Amazon, roughly 97% of the biome would show some increase in the impact of fire. Eastern and southeastern Amazon would be most affected, especially along the ecotone with the Cerrado. In this biome, HIF would expand from 0.7 to 1.2% by 2050, totaling a 67% increase. In the Pantanal, 74% of the biome would present some increase in the impact of fire (Figure 3). Areas that would be most affected concentrate along the ecotone with the Cerrado and in the south portion of the biome (Figure 3). HIF would expand from 7 to 8% by 2050 (Figure 3). Under the RCP8.5-MIROC5 and WEG, fire impact would increase in 97% of the Cerrado, in 98% of the Amazon, and in 93% of the Pantanal. In Cerrado, HIF would expand to 18% of the biome by 2050, a fivefold increase. In the Amazon HIF would expand to 1.6% of the region, and in the Pantanal to virtually 23% of the biome. Results from intermediate scenarios and HadGEM2-ES model are presented in Supplementary Tables 9, 10.
Figure 3. Fire impact from the Random Forest (RF) model for the present, left column and in middle column forecast by 2050 for the RCP4.5-MIROC5 and strong environmental governance. Figures in the third column depict the change in the impact of fire under this scenario. The numbers in red indicate the percentage of the biome with HIF today and in the future, and the numbers in black the percentage of biome that would have an increase in the impact of fire.
In the Cerrado and Pantanal, the climate is the major determinant of fire impact, while anthropogenic factors prevail in the Amazon. Nevertheless, the interplay between both determinants is key in driving the impact of fire. In the former two biomes, most of the burned areas located in NVR, and in the Amazon almost half of them. In addition, in all three biomes, HIF was strongly associated with NVR, which influenced the impact of fire. Historically, fire activity strongly correlates with deforestation rates in the Amazon (Supplementary Figure 1), as the recent spike of 2019/2020 following the highest annual deforestation rates in the decade. Roughly 55% of burned areas in this biome occurred on private properties, thus indicating that fire is a common tool for deforestation (Supplementary Figures 1, 2). In this respect, the use of fire for converting forests into pasturelands or clearing pastures is indirectly indicated by the association with density of heads of cattle in the respective SAR model. The SAR models for the Cerrado and Pantanal also indicated an association of fire impact with deforestation or land-use intensity as pointed out by distances to types of deforestation and agricultural areas, along with density heads of cattle. In fact, gross deforestation rates in the Cerrado are second only to those of the Amazon and are on the rise in three biomes (PRODES, 2020; Souza et al., 2020). In turn, only in the Cerrado natural ignition by lightning was a statistically significant factor, though quite marginal.
Regarding the database of burned areas from MODIS that we used in our analyses, one could argue that they underestimate burned areas in forest biomes, because of understory fires. To verify this claim, we compared our database with the dataset of Morton et al. (2013) for Southern Amazon to find that, on average, MODIS dataset estimated 8% more burned areas than did Morton and colleagues using hybrid image composites of Landsat, AIRS and MODIS.
Today, half of the remaining Amazon Forest is within PAs, yet fire only occurred in 3.4% of PA’s territories, representing 1.7% of the biome’s burned areas. Thus, despite insufficient enforcement (Pelicice and Castello, 2021), Amazon PAs still play a major role in avoiding deforestation and fires as well, even under the country’s current situation of deteriorating public environmental policies. In this respect, it is worth mentioning the role of the ARPA (Amazon Region Protected Areas) Program in supporting many conservation units in the Amazon (Soares-Filho et al., 2010) as well as ICMBio’s (The Chico Mendes Institute for Biodiversity Conservation) brigades (Oliveira A.S. et al., 2021) and the PrevFogo (The National Center to Prevent and Combat Forest fires) program (Ibama, 2020) that prevent and fight fires in conservation units and indigenous lands, respectively.
Conversely, about 60 and 50% of PAs were burned in the Cerrado and Pantanal, respectively, at least once over the study period. Also, almost 60% of HIF occurrences fell within Cerrado’s PAs and 17% within those of Pantanal. There is, therefore, a strong positive association between fire and PAs in both biomes, because either those areas are mostly covered with NVR, and in some cases natural grasslands used for grazing (e.g., Park of Ilha do Bananal), or due to land conflicts. In 2020, large wildfires that burned one third of the Pantanal started on a few specific ranches in the region (Mega, 2020). These fires, accidently or not, then escaped to NVR, affecting as a result some important PAs in the region that burned almost completely, such as Parque Nacional do Pantanal Matogrossense and Terra Indígena Kadiwéu. As for the Cerrado, recurrent wildfires affect several of its conservation units almost every year (Oliveira U. et al., 2021). The source of a large part of those fires may be attributed to retaliation by prior and current inholdings not yet financially compensated for land expropriation, as the recent cases that occurred in the national parks of Chapada dos Veadeiros and Serra da Canastra (Röper, 2000; Rodrigues et al., 2018).
Frequent recurrence of fires can create positive feedback, as areas that burn repeatedly are more prone to new fires (Hoffmann et al., 2020). Furthermore, fires in the Cerrado and Pantanal have a propagation rate much faster than that of fires in forest biomes like the Amazon and Atlantic Forest (Gomes et al., 2020a). In non-forest biomes, fires often associate with fuel loads accumulation (Franke et al., 2018). However, our results show that burned areas present extremely variable fuel loads (SI). This indicates that, if managing fuel loads, as a form of preventive measure, effectively curb fires on a local scale (Hoffmann et al., 2020), it does not suffice to tame severe wildfires, since their primary cause is anthropogenic ignition sources. Thus, local management of fuel loads must be a component of a comprehensive fire mitigation program aimed at preventing fire through better land-use practices, but also through effective law enforcement.
The lower occurrence of fires in the Atlantic Forest may be due to its highly fragmented NVR, and hence large areas already converted into pastures and agricultural lands (Fonseca, 1985). Nevertheless, the Atlantic Forest biome comprises the most developed and urbanized regions of the country, where law enforcement has traditionally been more effective (Lembi et al., 2020). If, in the past, fire was used to deforest and clear pastures, nowadays, this use has virtually vanished from most of this biome’s landscapes. As for the Caatinga and Pampa, agricultural and livestock production in these biomes seldom uses fire for pastures renovation or deforestation, oppositely to what happens in the Amazon, Cerrado and Pantanal (Pivello, 2011).
Fires induce either plant mortality or physiological stress on individuals that manage to sprout after burning (Phillips et al., 2015; Hood et al., 2018). Recurrent and highly intensive fires proportionally affect a wider range of species more severely (Phillips et al., 2015; Hood et al., 2018). Whereas savannas or shrublands (e.g., Cerrado and Pantanal) consist of vegetation more resilient to fire (Simon and Pennington, 2012), the impact of fire on vegetation is greater on forest species (e.g., the Amazon and Atlantic Forest). However, even the Cerrado vegetation, which developed a series of morphophysiological adaptations to fire (Simon and Pennington, 2012), was strongly affected, resulting in a 19% reduction of the NDVI in HIF areas compared to areas that never burned. Also, there was a significant reduction in NDVI in burned areas (Figure 2). This reduction indicates that the vegetation in these locations is not fully recovering after a fire event, i.e., it is not being able to produce leaves and photosynthetic compounds at their maximum potential (Gamon et al., 1995). Lower NDVI might also indicate that some sensitive plants may become scarcer or go extinct as fire regimes intensify (Machida et al., 2021). This calls for research on the impact of fire on biodiversity and associated ecosystem services, such as river and rainfall regulation, soil conservation, carbon stocks, and provision of non-timber and timber products. This is especially important given that fires affect mainly NVR in the Amazon, Cerrado and Pantanal, whose highly biodiverse ecosystems are amongst the most endangered of the world (Reid, 1998; Myers et al., 2000).
Given that climate and LUCC are major determinants of fire impact, our results indicate that wildfires are likely to become more common and intense over the next three decades, with commensurate impacts, especially in the Amazon, Cerrado, and Pantanal biomes. Thus, firefighting will soon become more complex and costly. Current public and private investments in firefighting and prevention in Brazil are at the order of US$3.10 and US$15.89 ha–1yr–1, respectively, which are much smaller than those of the countries of the Global North. Although both investments have proven to pay off (Oliveira A.S. et al., 2021), as climate and deforestation further interact to reduce rainfall (Leite-Filho et al., 2021), much larger investments will be needed. Such an ensued environmental crisis is already unfolding, as indicated by the 2020 wildfires in the Pantanal and in the Amazon (Mega, 2020), the lower rainfall recorded over the agribusiness main production regions (Sena et al., 2012; Jimenez et al., 2018; Leite-Filho et al., 2021), as well as the country’s hydraulic emergency of 2021 (Mano and Samora, 2021). Indeed, the dismantling of environmental public policies tend to further aggravate Brazil’s environmental predicament, reinforcing as a result a vicious cycle of continued deforestation, enhanced and expanded HIF (Aragão et al., 2018), hence more forest degradation and droughts (Nepstad et al., 2008; Soares-Filho et al., 2012), biodiversity loss (Kelly et al., 2020), heath issues (Butt et al., 2020), greenhouse gas emissions, and global climate change (Burton et al., 2021).
While the need for urgent global actions to reduce greenhouse gas emissions remain vital to prevent escalating wildfires, mapping the regional impacts of fire may contribute to design effective policies to tackle this critical environmental issue. In view of the budget cuts in the federal government funding for environmental enforcement and fire mitigation programs, efforts by the private sector and subnational governments that fill this gap will become increasingly pivotal. In this respect, it is worth mentioning two initiatives: the successful fire mitigation program of Aliança da Terra that carries out prevention and firefighting campaigns both on public and private lands (Oliveira A.S., 2021), and the innovative fire-spread risk and monitoring systems of the FIP-Cerrado (Forest Investment Program)5 developed by the Federal University of Minas Gerais and INPE (National Institute for Space Research). Those initiatives demonstrate that the resilience of the Brazilian civil society, together with the prowess of its scientific community, is today the major instrument to counteract the political threats against the country’s rich socioenvironmental heritage (Rajão et al., 2020; Andrade et al., 2021; Caetano, 2021; Vale et al., 2021).
The original contributions presented in the study are included in the article/Supplementary Material, further inquiries can be directed to the corresponding author/s.
UO and BS-F led the development of the research design, analysis, and manuscript writing. UO, BS-F, LG, MB, RR, and JO contributed to the interpretation of results and manuscript writing. All authors revised the draft and gave final approval for publication.
This work was supported by the project “Development of Systems to Prevent Forest Fires and Monitor Vegetation Cover in the Brazilian Cerrado” (World Bank Project no. P143185)–Forest Investment Program (FIP) and the Brazilian National Council for Scientific and Technological Development (CNPq/AWS 032/2019). BS-F and RR also received support from CNPq and Climate and Land Use Alliance (CLUA). MB and LG were supported by CNPq-PREVFOGO (441995/2018-7), INCT-Mudanças Climáticas (CNPq 465501/2014-1), and Rede Clima, and JO were supported by FAPESP 2017/22269-2.
The authors declare that the research was conducted in the absence of any commercial or financial relationships that could be construed as a potential conflict of interest.
All claims expressed in this article are solely those of the authors and do not necessarily represent those of their affiliated organizations, or those of the publisher, the editors and the reviewers. Any product that may be evaluated in this article, or claim that may be made by its manufacturer, is not guaranteed or endorsed by the publisher.
The Supplementary Material for this article can be found online at: https://www.frontiersin.org/articles/10.3389/ffgc.2022.735017/full#supplementary-material
Abessa, D., Famá, A., and Buruaem, L. (2019). The systematic dismantling of Brazilian environmental laws risks losses on all fronts. Nat. Ecol. Evol. 3, 510–511. doi: 10.1038/s41559-019-0855-9
Adams, M. A. (2013). Mega-fires, tipping points and ecosystem services: managing forests and woodlands in an uncertain future. For. Ecol. Manag. 294, 250–261. doi: 10.1016/j.foreco.2012.11.039
Andrade, M. B., Ferrante, L., and Fearnside, P. M. (2021). Brazil’s Highway BR-319 demonstrates a crucial lack of environmental governance in Amazonia. Environ. Conserv. 48, 161–164. doi: 10.1017/S0376892921000084
Aragão, L. E. O. C., Anderson, L. O., Fonseca, M. G., Rosan, T. M., Vedovato, L. B., Wagner, F. H., et al. (2018). 21st Century drought-related fires counteract the decline of Amazon deforestation carbon emissions. Nat. Commun. 9:536. doi: 10.1038/s41467-017-02771-y
Aragão, L. E. O. C., Malhi, Y., Barbier, N., Lima, A., Shimabukuro, Y., Anderson, L., et al. (2008). Interactions between rainfall, deforestation and fires during recent years in the Brazilian Amazonia. Philos. Trans. R. Soc. Lond. B Biol. Sci. 363, 1779–1785. doi: 10.1098/rstb.2007.0026
Batista, E., Soares-Filho, B., Barbosa, F., Merry, F., Davis, J., van der Hoff, R., et al. (2019). Large-scale pasture restoration may not be the best option to reduce greenhouse gas emissions in Brazil. Environ. Res. Lett. 14:125009. doi: 10.1088/1748-9326/ab5139
Bowman, D. M. J. S., Murphy, B. P., Williamson, G. J., and Cochrane, M. A. (2014). Pyrogeographic models, feedbacks and the future of global fire regimes. Glob. Ecol. Biogeogr. 23, 821–824. doi: 10.1111/geb.12180
Bowman, D. M. J. S., O’Brien, J. A., and Goldammer, J. G. (2013). Pyrogeography and the global quest for sustainable fire management. Annu. Rev. Environ. Resour. 38, 57–80. doi: 10.1146/annurev-environ-082212-134049
Brando, P. M., Soares-Filho, B., Rodrigues, L., Assunção, A., Morton, D., Tuchschneider, D., et al. (2020). The gathering firestorm in southern Amazonia. Sci. Adv. 6:eaay1632. doi: 10.1126/sciadv.aay1632
Burton, C., Kelley, D. I., Jones, C. D., Betts, R. A., Cardoso, M., and Anderson, L. (2021). South American fires and their impacts on ecosystems increase with continued emissions. Clim. Resil. Sustain. 15:E8. doi: 10.1002/cli2.8
Butt, E. W., Conibear, L., Reddington, C. L., Darbyshire, E., Morgan, W. T., Coe, H., et al. (2020). Large air quality and human health impacts due to Amazon forest and vegetation fires. Environ. Res. Commun. 2:95001. doi: 10.1088/2515-7620/abb0db
Caetano, M. A. L. (2021). Political activity in social media induces forest fires in the Brazilian Amazon. Technol. Forecast. Soc. Change 167:120676. doi: 10.1016/j.techfore.2021.120676
Campanharo, W., Lopes, A., Anderson, L., da Silva, T., and Aragão, L. (2019). Translating fire impacts in southwestern amazonia into economic costs. Remote Sens. 11:764. doi: 10.3390/rs11070764
Carlson, K. M., Heilmayr, R., Gibbs, H. K., Noojipady, P., Burns, D. N., Morton, D. C., et al. (2018). Effect of oil palm sustainability certification on deforestation and fire in Indonesia. Proc. Natl. Acad. Sci. U.S.A. 115, 121–126. doi: 10.1073/pnas.1704728114
Carmel, Y., Paz, S., Jahashan, F., and Shoshany, M. (2009). Assessing fire risk using Monte Carlo simulations of fire spread. For. Ecol. Manag. 257, 370–377. doi: 10.1016/j.foreco.2008.09.039
Chergui, B., Fahd, S., Santos, X., and Pausas, J. G. (2018). Socioeconomic factors drive fire-regime variability in the mediterranean basin. Ecosystems 21, 619–628. doi: 10.1007/s10021-017-0172-6
Chou, S. C., Lyra, A., Mourão, C., Dereczynski, C., Pilotto, I., Gomes, J., et al. (2014). Assessment of climate change over South America under RCP 4.5 and 8.5 downscaling scenarios. Am. J. Clim. Change 3, 512–527. doi: 10.4236/ajcc.2014.35043
Cullen, A. C., Axe, T., and Podschwit, H. (2021). High-severity wildfire potential – associating meteorology, climate, resource demand and wildfire activity with preparedness levels. Int. J. Wildland Fire 30, 30–41. doi: 10.1071/WF20066
de Araújo, F. M., Ferreira, L. G., and Arantes, A. E. (2012). Distribution patterns of burned areas in the brazilian biomes: an analysis based on satellite data for the 2002-2010 period. Remote Sens. 4, 1929–1946. doi: 10.3390/rs4071929
de Oliveira, A. S., Rajão, R. G., Soares Filho, B. S., Oliveira, U., Santos, L. R. S., Assunção, A. C., et al. (2019). Economic losses to sustainable timber production by fire in the Brazilian Amazon. Geogr. J. 185, 55–67.
Drukker, D. M., Prucha, I. R., and Raciborski, R. (2013). Maximum likelihood and generalized spatial two-stage least-squares estimators for a spatial-autoregressive model with spatial-autoregressive disturbances. Stata J. 13, 221–241. doi: 10.1177/1536867x1301300201
Efron, B., and Stein, C. (1981). The jackknife estimate of variance. Ann. Stat. 9, 1100–1120. doi: 10.1214/aos/1176345462
Enright, N. J., Fontaine, J. B., Bowman, D. M. J. S., Bradstock, R. A., and Williams, R. J. (2015). Interval squeeze: altered fire regimes and demographic responses interact to threaten woody species persistence as climate changes. Front. Ecol. Environ. 13, 265–272. doi: 10.1890/140231
Fernandes, P. M., Loureiro, C., Guiomar, N., Pezzatti, G. B., Manso, F. T., and Lopes, L. (2014). The dynamics and drivers of fuel and fire in the Portuguese public forest. J. Environ. Manage. 146, 373–382. doi: 10.1016/j.jenvman.2014.07.049
Fonseca, G. A. B. (1985). The vanishing Brazilian Atlantic forest. Biol. Conserv. 34, 17–34. doi: 10.1016/0006-3207(85)90055-2
Franke, J., Barradas, A. C. S., Borges, M. A., Menezes Costa, M., Dias, P. A., Hoffmann, A. A., et al. (2018). Fuel load mapping in the Brazilian Cerrado in support of integrated fire management. Remote Sens. Environ. 217, 221–232. doi: 10.1016/j.rse.2018.08.018
Gamon, J. A., Field, C. B., Goulden, M. L., Griffin, K. L., Hartley, A. E., Joel, G., et al. (1995). Relationships between NDVI, canopy structure, and photosynthesis in three californian vegetation types. Ecol. Appl. 5, 28–41. doi: 10.2307/1942049
Ganteaume, A., Camia, A., Jappiot, M., San-Miguel-Ayanz, J., Long-Fournel, M., and Lampin, C. (2013). A review of the main driving factors of forest fire ignition over Europe. Environ. Manage. 51, 651–662. doi: 10.1007/s00267-012-9961-z
Ganteaume, A., and Syphard, A. D. (2018). “Ignition sources,” in Encyclopedia of Wildfires and Wildland-Urban Interface (WUI) Fires, ed. S. L. Manzello (Cham: Springer International Publishing), 1–17. doi: 10.1007/978-3-319-51727-8_43-1
Gomes, L., Miranda, H. S., Silvério, D. V., and Bustamante, M. M. C. (2020a). Effects and behaviour of experimental fires in grasslands, savannas, and forests of the Brazilian Cerrado. For. Ecol. Manage. 458:117804. doi: 10.1016/j.foreco.2019.117804
Gomes, L., Miranda, H. S., Soares-Filho, B., Rodrigues, L., Oliveira, U., and Bustamante, M. M. C. (2020b). Responses of plant biomass in the brazilian savanna to frequent fires. Front. For. Glob. Change 3:507710. doi: 10.3389/ffgc.2020.507710
Harper, A. R., Doerr, S. H., Santin, C., Froyd, C. A., and Sinnadurai, P. (2018). Prescribed fire and its impacts on ecosystem services in the UK. Sci. Total Environ. 624, 691–703. doi: 10.1016/j.scitotenv.2017.12.161
Hoffmann, T. B., Dutra, A. C., Shimabukuro, Y. E., Arai, E., Godinho Cassol, H. L., Girolamo Neto, C. D., et al. (2020). “Fire occurrence in the Brazilian savanna conservation units and their buffer zones,” in Proceedings of the IGARSS 2020 - 2020 IEEE International Geoscience and Remote Sensing Symposium (Waikoloa, HI: IEEE), 4263–4266. doi: 10.1109/IGARSS39084.2020.9324164
Hood, S. M., Varner, J. M., Van Mantgem, P., and Cansler, C. A. (2018). Fire and tree death: understanding and improving modeling of fire-induced tree mortality. Environ. Res. Lett. 13:113004. doi: 10.1088/1748-9326/aae934
Ibama (2020). Prevfogo. Available online at: http://www.ibama.gov.br/prevfogo
IBGE (2019). Instituto Brasileiro de Geografia e Estatística - IBGE. Pesquisa da Pecuária Municipal - PPM. Available Online at: https://sidra.ibge.gov.br/pesquisa/ppm/quadros/brasil/2019 (accessed January 2021).
Jimenez, J. C., Libonati, R., and Peres, L. F. (2018). Droughts over Amazonia in 2005, 2010, and 2015: a cloud cover perspective. Front. Earth Sci. 6:227. doi: 10.3389/feart.2018.00227
Karger, D. N., Schmatz, D., Detttling, D., and Zimmermann, N. E. (2020). High resolution monthly precipitation and temperature timeseries for the period 2006–2100. Sci. Data. 7:248. doi: 10.1038/s41597-020-00587-y
Keeley, J. E. (2009). Fire intensity, fire severity and burn severity: a brief review and suggested usage. Int. J. Wildland Fire 18, 116–126. doi: 10.1071/WF07049
Kelly, L. T., Giljohann, K. M., Duane, A., Aquilué, N., Archibald, S., Batllori, E., et al. (2020). Fire and biodiversity in the anthropocene. Science 370:eabb0355. doi: 10.1126/science.abb0355
Leite-Filho, A. T., Soares-Filho, B. S., Davis, J. L., Abrahão, G. M., and Börner, J. (2021). Deforestation reduces rainfall and agricultural revenues in the Brazilian Amazon. Nat. Commun. 12:2591. doi: 10.1038/s41467-021-22840-7
Lembi, R. C., Cronemberger, C., Picharillo, C., Koffler, S., Sena, P. H. A., Felappi, J. F., et al. (2020). Urban expansion in the Atlantic forest: applying the nature futures framework to develop a conceptual model and future scenarios. Biota Neotrop. 20:e20190904. doi: 10.1590/1676-0611-bn-2019-0904
Li, P., Xiao, C., Feng, Z., Li, W., and Zhang, X. (2020). Occurrence frequencies and regional variations in Visible Infrared Imaging Radiometer Suite (VIIRS) global active fires. Glob. Chang. Biol. 26, 2970–2987. doi: 10.1111/gcb.15034
Libonati, R., DaCamara, C. C., Peres, L. F., Sander de Carvalho, L. A., and Garcia, L. C. (2020). Rescue Brazil’s burning Pantanal wetlands. Nature 588, 217–219. doi: 10.1038/d41586-020-03464-1
Lizundia-Loiola, J., Pettinari, M. L., and Chuvieco, E. (2020). Temporal anomalies in burned area trends: satellite estimations of the amazonian 2019 fire crisis. Remote Sens. 12:151. doi: 10.3390/rs12010151
Londoño, E., and Casado, L. (2019). Amazon Deforestation in Brazil Rose Sharply on Bolsonaro’s Watch. New York, NY: New York Times.
Machida, W. S., Gomes, L., Moser, P., Castro, I. B., Miranda, S. C., da Silva-Júnior, M. C., et al. (2021). Long term post-fire recovery of woody plants in savannas of central Brazil. For. Ecol. Manage. 493:119255. doi: 10.1016/j.foreco.2021.119255
Mano, A., and Samora, R. (2021). Brazil on Drought Alert, Faces Worst Dry Spell in 91 Years. London: Reuters.
Matthews, S., Sullivan, A. L., Watson, P., and Williams, R. J. (2012). Climate change, fuel and fire behaviour in a eucalypt forest. Glob. Chang. Biol. 18, 3212–3223. doi: 10.1111/j.1365-2486.2012.02768.x
Mavsar, R., Japelj, A., and Kovaè, M. (2013). Trade-offs between fire prevention and provision of ecosystem services in Slovenia. For. Policy Econ. 29, 62–69. doi: 10.1016/j.forpol.2012.10.011
Mega, E. R. (2020). “Apocalyptic” fires are ravaging the world’s largest tropical wetland. Nature 586, 20–21. doi: 10.1038/d41586-020-02716-4
Mengassi, D. (2021). Ministério do Meio Ambiente Tem Menor Orçamento das Últimas Duas Décadas. Milwaukie, OR: OECO.
Morton, D. C., Defries, R. S., Randerson, J. T., Giglio, L., Schroeder, W., and Van Der Werf, G. R. (2008). Agricultural intensification increases deforestation fire activity in Amazonia. Glob. Chang. Biol. 14, 2262–2275. doi: 10.1111/j.1365-2486.2008.01652.x
Morton, D. C., Le Page, Y., DeFries, R., Collatz, G. J., and Hurtt, G. C. (2013). Understorey fire frequency and the fate of burned forests in southern Amazonia. Philos. Trans. R. Soc. Lond. B Biol. Sci. 368:20120163. doi: 10.1098/rstb.2012.0163
Myers, N., Mittermeier, R. A., Mittermeier, C. G., Fonseca, G. A. B., and Kent, J. (2000). Biodiversity hotspots for conservation priorities. Nature 403, 853–858.
Nepstad, D. C., Stickler, C. M., Filho, B. S., and Merry, F. (2008). Interactions among Amazon land use, forests and climate: prospects for a near-term forest tipping point. Philos. Trans. R. Soc. Lond. B Biol. Sci. 363, 1737–1746. doi: 10.1098/rstb.2007.0036
Neto, W. N., Sato, M. N., Aires, F. S., Cochrane, M. A., and Miranda, H. S. (2010). Fires in the cerrado, the Brazilian savanna. Trop. Fire Ecol. 2, 427–450. doi: 10.1007/978-3-540-77381-8_15
Oliveira, A. S., Rajão, R. G., Soares Filho, B. S., Oliveira, U., Santos, L. R. S., Assunção, A. C., et al. (2019). Economic losses to sustainable timber production by fire in the Brazilian Amazon. Geogr. J. 185, 55–67. doi: 10.1111/geoj.12276
Oliveira, U., Soares-Filho, B., Leitão, R. F. M., and Rodrigues, H. O. (2019). BioDinamica: a toolkit for analyses of biodiversity and biogeography on the Dinamica-EGO modelling platform. PeerJ 7:e7213. doi: 10.7717/peerj.7213
Oliveira, A. S., Soares-Filho, B. S., Oliveira, U., Van der Hoff, R., Carvalho-Ribeiro, S. M., Oliveira, A. R., et al. (2021). Costs and effectiveness of public and private fire management programs in the Brazilian Amazon and Cerrado. For. Policy Econ. 127:102447. doi: 10.1016/j.forpol.2021.102447
Oliveira, U., Soares-Filho, B., de Souza Costa, W. L., Gomes, L., Bustamante, M., and Miranda, H. (2021). Modeling fuel loads dynamics and fire spread probability in the Brazilian Cerrado. For. Ecol. Manage. 482:118889. doi: 10.1016/j.foreco.2020.118889
Pausas, J. G., and Fernández-Muñoz, S. (2012). Fire regime changes in the Western Mediterranean Basin: from fuel-limited to drought-driven fire regime. Clim. Change 110, 215–226. doi: 10.1007/s10584-011-0060-6
Pelicice, F. M., and Castello, L. (2021). A political tsunami hits Amazon conservation. Aquat. Conserv. Mar. Freshw. Ecosyst. 31, 1221–1229. doi: 10.1002/aqc.3565
Pellegrini, A. F. A., Ahlström, A., Hobbie, S. E., Reich, P. B., Nieradzik, L. P., Staver, A. C., et al. (2018). Fire frequency drives decadal changes in soil carbon and nitrogen and ecosystem productivity. Nature 553, 194–198. doi: 10.1038/nature24668
Pellegrini, A. F. A., Hein, A. M., Cavender-Bares, J., Montgomery, R. A., Staver, A. C., Silla, F., et al. (2021). Disease and fire interact to influence transitions between savanna–forest ecosystems over a multi-decadal experiment. Ecol. Lett. 24, 1007–1017. doi: 10.1111/ele.13719
Pereira, A. C., Oliveira, S. L. J., Pereira, J. M. C., and Turkman, M. A. A. (2014). Modelling fire frequency in a Cerrado savanna protected area. PLoS One 9:e102380. doi: 10.1371/journal.pone.0102380
Phillips, O. L., Silva-Espejo, J. E., Alvarez-Dávila, E., Nuñez Vargas, P., Baccini, A., Brienen, R. J. W., et al. (2015). Ecosystem heterogeneity determines the ecological resilience of the Amazon to climate change. Proc. Natl. Acad. Sci. U.S.A. 113, 793–797. doi: 10.1073/pnas.1511344112
Pivello, V. R. (2011). The use of fire in the cerrado and Amazonian rainforests of Brazil: past and present. Fire Ecol. 7, 24–39. doi: 10.4996/fireecology.0701024
Prichard, S. J., Stevens-rumann, C. S., and Hessburg, P. F. (2017). Forest ecology and management tamm review: shifting global fire regimes: lessons from reburns and research needs. For. Ecol. Manage. 396, 217–233. doi: 10.1016/j.foreco.2017.03.035
PRODES (2020). PRODES. TerraBrasilis—Taxas Anuais de Sesmatamento na Amazônia Legal Brasiliera. Available Online at: http://terrabrasilis.dpi.inpe.br/app/dashboard/deforestation/biomes/legal_amazon/rates (accessed January 2021).
Rajão, R., Soares-Filho, B., Nunes, F., Börner, J., Machado, L., Assis, D., et al. (2020). The rotten apples of Brazil’s agribusiness. Science 369, 246–248. doi: 10.1126/science.aba6646
Rappold, A. G., Reyes, J., Pouliot, G., Cascio, W. E., and Diaz-Sanchez, D. (2017). Community vulnerability to health impacts of wildland fire smoke exposure. Environ. Sci. Technol. 51, 6674–6682. doi: 10.1021/acs.est.6b06200
Reid, W. V. (1998). Biodiversity hotspots. Trends Ecol. Evol. 13, 275–280. doi: 10.1016/S0169-5347(98)01363-9
Rochedo, P. R. R., Soares-Filho, B., Schaeffer, R., Viola, E., Szklo, A., Lucena, A. F. P., et al. (2018). The threat of political bargaining to climate mitigation in Brazil. Nat. Clim. Chang. 8, 695–698. doi: 10.1038/s41558-018-0213-y
Rodrigues, A. A., da Cunha Bustamante, M. M., and Sano, E. E. (2018). As far as the eye can see: scenic view of Cerrado National Parks. Perspect. Ecol. Conserv. 16, 31–37. doi: 10.1016/j.pecon.2017.11.004
Röper, M. (2000). On the way to a better state? The role of NGOs in the planning and implementation of protected areas in Brazil. GeoJournal 52, 61–69. doi: 10.1023/A:1013139912877
Roy, D. P., Boschetti, L., and Trigg, S. N. (2006). Remote sensing of fire severity: assessing the performance of the normalized burn ratio. IEEE Geosci. Remote Sens. Lett. 3, 112–116. doi: 10.1109/LGRS.2005.858485
Ryan, C., and Williams, M. (2010). How does fire intensity and frequency affect miombo woodland tree populations and biomass? Ecol. Appl. 21, 48–60. doi: 10.1890/09-1489
Schmidt, I. B., and Eloy, L. (2020). Fire regime in the Brazilian Savanna: recent changes, policy and management. Flora 268:151613. doi: 10.1016/j.flora.2020.151613
Schmidt, I. B., Moura, L. C., Ferreira, M. C., Eloy, L., Sampaio, A. B., Dias, P. A., et al. (2018). Fire management in the Brazilian savanna: first steps and the way forward. J. Appl. Ecol. 55, 2094–2101. doi: 10.1111/1365-2664.13118
Sena, J. A., Beser de Deus, L. A., Freitas, M. A. V., and Costa, L. (2012). Extreme events of droughts and floods in Amazonia: 2005 and 2009. Water Resour. Manag. 26, 1665–1676. doi: 10.1007/s11269-012-9978-3
Silva, C. A., Santilli, G., Sano, E. E., and Laneve, G. (2021). Fire occurrences and greenhouse gas emissions from deforestation in the Brazilian Amazon. Remote Sens. 13:376. doi: 10.3390/rs13030376
Simon, M. F., and Pennington, T. (2012). Evidence for adaptation to fire regimes in the tropical Savannas of the Brazilian Cerrado. Int. J. Plant Sci. 173, 711–723. doi: 10.1086/665973
Soares-Filho, B., Moutinho, P., Nepstad, D., Anderson, A., Rodrigues, H., Garcia, R., et al. (2010). Role of Brazilian Amazon protected areas in climate change mitigation. Proc. Natl. Acad. Sci. U.S.A. 107, 10821–10826. doi: 10.1073/pnas.0913048107
Soares-Filho, B., Rajão, R., Merry, F., Rodrigues, H., Davis, J., Lima, L., et al. (2016). Brazil’s market for trading forest certificates. PLoS One 11:e0152311. doi: 10.1371/journal.pone.0152311
Soares-Filho, B., Rodrigues, H., and Follador, M. (2013). A hybrid analytical-heuristic method for calibrating land-use change models. Environ. Model. Softw. 43, 80–87. doi: 10.1016/j.envsoft.2013.01.010
Soares-Filho, B., Silvestrini, R., Nepstad, D., Brando, P., Rodrigues, H., Alencar, A., et al. (2012). Forest fragmentation, climate change and understory fire regimes on the Amazonian landscapes of the Xingu headwaters. Landsc. Ecol. 27, 585–598. doi: 10.1007/s10980-012-9723-6
Souza, C. M., Shimbo, J. Z., Rosa, M. R., Parente, L. L., Alencar, A. A., Rudorff, B. F. T., et al. (2020). Reconstructing three decades of land use and land cover changes in brazilian biomes with landsat archive and earth engine. Remote Sens. 12:2735. doi: 10.3390/RS12172735
Tedim, F., Leone, V., Amraoui, M., Bouillon, C., Coughlan, M., Delogu, G., et al. (2018). Defining extreme wildfire events: difficulties, challenges, and impacts. Fire 1:9. doi: 10.3390/fire1010009
Tiefelsdorf, M., Griffith, D. A., and Boots, B. (1999). A variance-stabilizing coding scheme for spatial link matrices. Environ. Plan. A 31, 165–180. doi: 10.1068/a310165
Vale, M. M., Berenguer, E., Argollo de Menezes, M., Viveiros de Castro, E. B., Pugliese de Siqueira, L., and Portela, R. C. Q. (2021). The COVID-19 pandemic as an opportunity to weaken environmental protection in Brazil. Biol. Conserv. 255:108994. doi: 10.1016/j.biocon.2021.108994
Keywords: modeling, deforestation, land use change, protected areas, native vegetation
Citation: Oliveira U, Soares-Filho B, Bustamante M, Gomes L, Ometto JP and Rajão R (2022) Determinants of Fire Impact in the Brazilian Biomes. Front. For. Glob. Change 5:735017. doi: 10.3389/ffgc.2022.735017
Received: 01 July 2021; Accepted: 28 January 2022;
Published: 28 March 2022.
Edited by:
France Gerard, UK Centre for Ecology and Hydrology (UKCEH), United KingdomReviewed by:
Giuliano Maselli Locosselli, University of São Paulo, BrazilCopyright © 2022 Oliveira, Soares-Filho, Bustamante, Gomes, Ometto and Rajão. This is an open-access article distributed under the terms of the Creative Commons Attribution License (CC BY). The use, distribution or reproduction in other forums is permitted, provided the original author(s) and the copyright owner(s) are credited and that the original publication in this journal is cited, in accordance with accepted academic practice. No use, distribution or reproduction is permitted which does not comply with these terms.
*Correspondence: Ubirajara Oliveira, dWJpb2xvZ2lhQHlhaG9vLmNvbS5icg==
Disclaimer: All claims expressed in this article are solely those of the authors and do not necessarily represent those of their affiliated organizations, or those of the publisher, the editors and the reviewers. Any product that may be evaluated in this article or claim that may be made by its manufacturer is not guaranteed or endorsed by the publisher.
Research integrity at Frontiers
Learn more about the work of our research integrity team to safeguard the quality of each article we publish.