- 1Institute of Crop Science and Resource Conservation (INRES), University of Bonn, Bonn, Germany
- 2Department of Agronomy, MNS-University of Agriculture, Multan, Pakistan
- 3Department of Agricultural Sciences, University of Helsinki, Helsinki, Finland
Limited modeling studies are available for the process-based simulation of ecosystem services (ESS) and biodiversity (BD) in agroforestry systems (AFS). To date, limited field scale AFs models are available to simulate all possible ESS and BD together. We conducted an extensive systematic review of available agroforestry (AF), BD, and soil erosion models for the simulation potential of seven most desirable ESS in AFS. Simple to complex AF models have an inherent limitation of being objective-specific. A few complex and dynamic AF models did not meet the recent interest and demands for the simulation of ESS under AFS. Further, many ESS modules especially soil erosion, GHGs emission, groundwater recharge, onsite water retention, nutrients and pesticide leaching, and BD are often missing in available AF models, while some existing soil erosion models can be used in combination with AF models. Likewise mechanistic and process-based BD diversity models are lacking or found limited simulation potential for ESS under AFS. However, further efforts of model development and improvement (integration and coupling) are needed for the better simulation of complex interactive processes belonging to ESS under AFS. There are different possibilities but a proficient modeling approach for better reliability, flexibility, and durability is to integrate and couple them into a process-based dynamic modular structure. Findings of the study further suggested that crop modeling frameworks (MFW) like SIMPLACE and APSIM could be potential ones for the integration and coupling of different suitable modeling approaches (AF, soil protection, GHGs emission, flood prevention, carbon sequestration, onsite water retention, ground recharge, nutrient leaching, and BD modules) in one platform for dynamic process based ESS estimation on daily basis at the field scale.
1. Introduction
1.1. Typology of agroforestry system and ecosystem services
Current crop and forest production systems face new challenges as they must now also account for climate change and utilize sustainable production methods to meet the demands of an ever-increasing population. In contrast to conventional (monoculture) cropping systems, agroforestry (AF) promotes several ecosystem services (ESS) and biodiversity (BD) without compromising productivity or economic returns (Nguyen et al., 2013). Agroforestry systems (AFS), consequently, have the potential to support sustainable, climate-resilient production systems by considering both pillars of climate change, i.e., adaptation and mitigation, and by preserving ESS, ecosystem resilience, and BD (Palma et al., 2007a; FAO, 2015; Crous-Duran et al., 2019a; Kay et al., 2019). Furthermore, varied and innovative approaches toward AF could help to sustain food production in different environments (Nguyen et al., 2013; Burgess and Rosati, 2018). AFS soil-related ESS has numerous advantages, like improving soil health, water infiltration and regulation, groundwater recharge, microclimate development, reduced soil erosion, improved soil fertility, enhanced carbon sequestration, reduce GHG emissions, and reduced nutrient leaching. AFS also promotes BD in terms of genetics, species, and crop diversity, as well as by improving microhabitats (above and below ground) and promoting wild, native, and non-native species which are crucial for the promotion of ESS and environmental functions (Jose, 2012; Sistla et al., 2016; Sagastuy and Krause, 2019). The benefits of BD are attributed to plant/vegetation heterogeneity, soil conditions, organic carbon, the microclimate, and improved ESS. Furthermore, AFS provides short and long-term financial support for farmers (Bayala et al., 2014). The contributions of AF toward ESS provisions and sustainability depend on the system components of the site-specific responses and may take time to become established (Coe et al., 2014; Luedeling et al., 2014).
Estimating ESS provisions in AFS is crucial to understand the interactions among different components of the system and the sustainability of the ecosystem. Innovative tools and techniques, like modeling approaches, can be used to estimate the provisions of ESS and the potential impacts on the environment (Palma et al., 2007a). Estimations of the positive effects can be assessed with the help of process-based dynamics modeling approaches, as they provide insight into the interactions and competition for resources (light, water, and nutrients). While attempts have been made to model AFS and estimate ESS (Bayala et al., 2015; Luedeling et al., 2016), there are many challenges and issues still to be overcome, as the available process-based dynamic crop models are not well developed. It is crucial, however, that we model ESS for sustainability and BD assessments (Coe et al., 2014). Seven ESS, including soil protection and conservation, water retention for flood prevention, carbon sequestration, soil biodiversity, reducing GHG emissions, increasing groundwater recharge, reduction in N leaching, and BD conservation are desirable for current and future scenarios, but dynamic field scale modeling of these important aspects is limited. ESS to achieve sustainable development goals are provided by AFS and require modeling approaches for dynamic process-based simulations (Table 1). The objective of the present study is to highlight potential and key challenges in AFS modeling and its internal processes for ESS and BD and the dynamic processes of the tree–crop interactions. We present a way forward in the development of dynamic processed-based AFS modeling for the simulation of ESS and BD at the field scale, by considering the interactions of tree and crop species. We will do so by reviewing the existing AF, soil erosion, and BD modeling approaches and by evaluating their potential and key challenges and suggesting possible options to integrate and develop a modeling framework that can reliably predict a range of ESS and provide sustainability assessments.
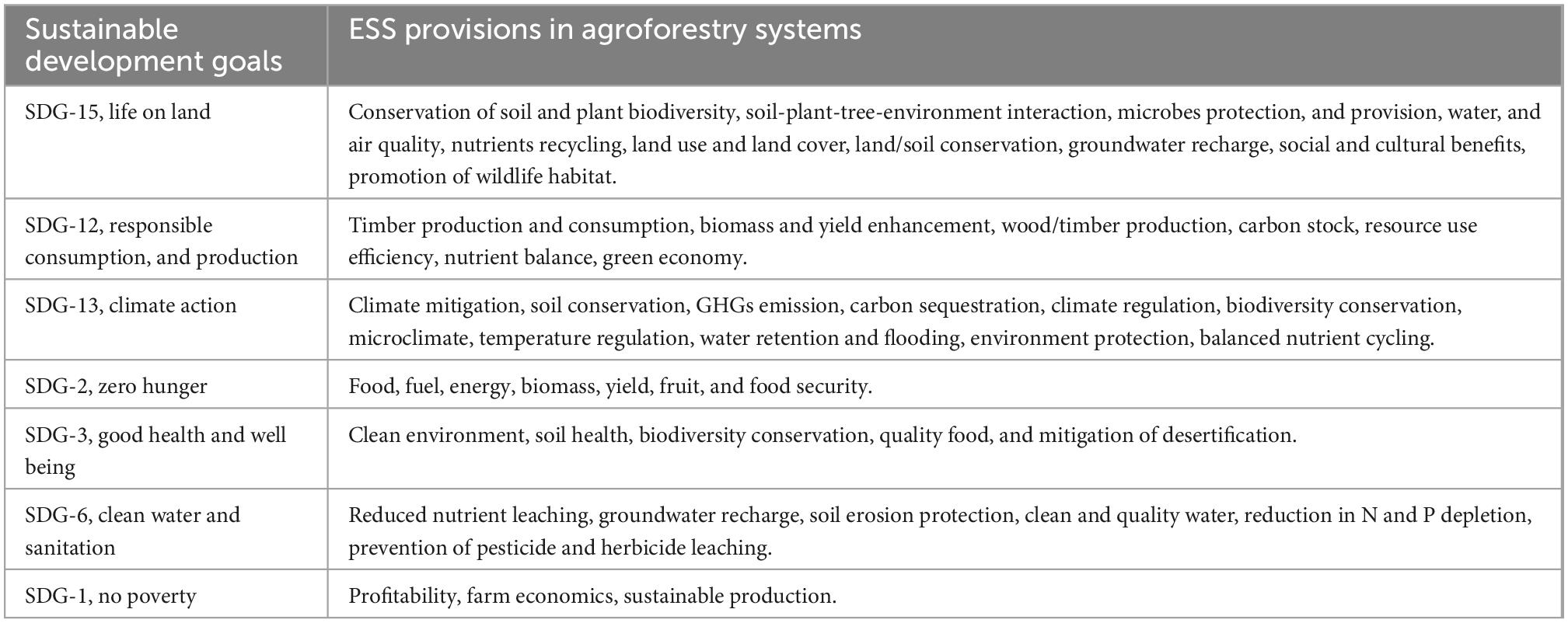
Table 1. Ecosystem services (ESS) need to be simulated using optimized agroforestry system (AFS) models and modeling frameworks according to the sustainable development goals of the United Nations.
2. Materials and methods
The systematic review was performed to identify the current and future promising modeling approaches for the simulation of ESS and BD under the agroforestry system. The study focused on the identification, screening and shortlisting of the articles focusing on the model structure, output, and classification. A thorough screening of literature was done for available models to simulate the ESS under agroforestry and particularly focused on the simulation of seven more demanded ESS under AFS (soil erosion, GHGs emission, groundwater recharge, onsite water retention, nutrients and pesticide leaching, and BD). Generally, this study focused on a global review of available models having potential for the simulation of AF system, while further, the study focused on Europe for the simulation of ESS in agroforestry system. Total articles identified and screened through database search (1,771) using the designed keywords in specific combinations (agroforestry models, simple to complex models (1D–3D), soil erosion models, ESS, GHGs emission, carbon sequestration, groundwater recharge, soil conservation, onsite water retention, flood prevention, nutrients and pesticide leaching, BD conservation). The following most popular search engine for scientific knowledge was assessed and the number of the article identified were as; Google Scholar (n = 667), Science Direct (n = 309), Scopus (n = 145), Research Gate (n = 391), Refseek (n = 24), Web of Science (n = 81), Semanticscholar (n = 18), Wiley Online Library (n = 27), PubMed Central (n = 31), CiteSeerX (n = 12), Europe PMC (n = 66). Further, the 1,210 articles were excluded based on title, and abstract (less focus on the keywords and research questions). Furthermore, the 561 articles were assessed and retrieved from the above-mentioned search engine for further eligibility and focus on the model classification. This review paper is structured in the following way. A brief explanation and overview of agroforestry, soil erosion, and BD models for ESS simulation and potential are in section “2 Modeling for agroforestry, soil erosion and biodiversity for ESS simulation.” The shortlisted models are reviewed in terms of their objective, model structure, model components, as well as their application, ease of model calibration, and parameter requirements. While further the existing models and MFW of AF and BD were discussed in section “3 Existing models and modeling frameworks of AF and biodiversity for ESS simulation.” Limitations and key challenges of current AFS models for the simulation of ESS and BD (soil protection, onsite water retention, carbon sequestration and soil biodiversity, GHG emissions, groundwater recharge, nitrogen, pesticides, and herbicides leaching, BD conservation and assessment in AFS) were discussed in section “4 Limitations and key challenges of current AFS models for the simulation of ecosystem services and biodiversity.” In section “5 Key challenges for the dynamic simulation of process-based models in AFS,” the key challenges for the dynamic simulation of process-based models in AFS were deliberated. Section “6 Ways forward and options for the integration of agroforestry, ESS modules, and biodiversity models in dynamic processed-based modeling frameworks for the simulation of ESS and biodiversity in AFS” focused on the way forward and options for the integration of agroforestry, ESS modules, and BD models in dynamic processed-based MFW for the simulation of ESS and BD in AFS. The last part consists of a summary and conclusion of the study.
3. Results
3.1. Modeling agroforestry, soil erosion and biodiversity for ESS simulation
3.1.1. Competition of resources and the need for process-based dynamic AFS models for ESS and biodiversity
AFS are characterized by the competition of trees and crops for resources like soil, light, water, nutrients, and space (Keating and Carberry, 1993). Negative competition occurs for light, water, and nutrients while positive impacts of the ESS regulate water relationships and promote microclimates (Van Noordwijk and Lusiana, 1998; Bayala et al., 2015; Luedeling et al., 2016; Smethurst et al., 2018). Modeling the competition for light, water, and nutrients is complex as a large number of interactions need to be considered, and this is especially true for AFS in contrast to the crop models for mono-cropping systems (Dupraz, 2002; Smethurst et al., 2017). Current intercropping crop modules may not provide accurate predictions for tree and crop interactions, as they are not designed to handle the complex interactions and competition that occurs in AFS; for example, they do not consider critical resource competition or the facilitative interactions between trees and crops (Powlson et al., 2011; Pinto et al., 2019). Modeling the competition for resources with dynamic (i.e., time-dependent) approaches is critical to understand the nature, degree, and extent of competition and interactions (Van Noordwijk and Lusiana, 1998; Jose et al., 2004; Hussain et al., 2016). Several simple, empirical, and mechanistic tree models have been developed for different scales, and these have the potential to simulate tree features reasonably well. However, the underlying principles and their structure are quite different when coupled with crop modeling approaches and their success rate is low (Bugmann, 2001; Dupraz, 2002; Charbonnier et al., 2013; Smethurst et al., 2017). Complex processes and AFS management have previously been modeled for tree mixtures, but these models have limited scope for AFS simulations as they require large data sets (López-Serrano et al., 2015; Hussain et al., 2016). Similarly, simple to complex process-based dynamic crop simulation models have been developed for individual crops and later attempts have been made to extend these models for intercropping (mixed cropping) systems (Baumann et al., 2002; Tsubo et al., 2005; Gou et al., 2017). The coupling of tree and crop models with different structures and complexities is challenging.
Existing soil erosion models can be empirical, physical, or process-based for the assessment of soil loss and runoff and are generally at larger scales, although some have been successfully applied at field scale. However, their applications at the field and subfield scale for AFS is limited (Bhuyan et al., 2002; Avwunudiogba and Hudson, 2014; Igwe et al., 2017; Raza et al., 2021). Similarly, the existing models for other ESS and BD range from simple (1D) to complex approaches and each model has its inherent limitations, and a few are very simple, while others are too complex (Araújo et al., 2019; Chopin et al., 2019; van Oijen et al., 2020). The developed AFS models are also useful for the simulation of some ESS (carbon sequestration and vegetation, water infiltration and retention, biomass production, and yield), but they lack accurate and reliable estimations for all ESS (Van Noordwijk and Lusiana, 1998; Luedeling et al., 2016; Holzworth et al., 2018; Dupraz et al., 2019). New research efforts to couple or integrate these capabilities into a state-of-the-art process-based dynamic modeling framework are required for the simultaneous assessment of ESS, erosion, and BD at the field scale in AFS.
3.1.2. Agroforestry modeling, overview, and potentials for the simulation of ESS
The modeling of tree and crop interactions is comparatively new when compared to crop modeling in the early nineties, as there was no model available to simulate the AFS-related processes (Mead and Willey, 1980). This may be due to the low importance of AFS in modern agriculture and to the complexity of the processes, like the above and below-ground interactions between tree and crop components. There are different types of AF models available with varying complexities and dimensions. For the tree components, there are simple empirical relationships (allometric models) expressing the association of the tree dry matter to the height, diameter, and volume, and focusing on the tree components only (Khaine and Woo, 2018). These also included simple functional tree branch analysis models to determine the biomass from the diameter. Other AFS models have been designed to simulate the impacts on the environment, but they have only small or no capability to simulate the dynamic growth of the tree and crop components (biomass and yield). The “Soil Changes under AgroForestry” (SCUAF) model (Young et al., 1998) is a prominent example of this, as it uses both crop and tree growth observations as input data and then simulates the effect of AFS on soil properties like nutrients, soil organic matter, and soil erosion but cannot simulate the above-ground components.
Likewise, the CO2FIX model is used to estimate the ESS related to soil and biomass yearly (Panwar et al., 2017). Most of the cited and used AF models are dynamic as they predict the effects of tree and crop interactions over time. Furthermore, most AF models also include a “mechanistic” or “explanatory” component to attempt to describe the behavior at one level by using an understanding of what occurs at a sub-level. An important feature of AFS is the interaction that occurs over time between trees, crops, and the atmospheric and soil environments (Palma et al., 2007a). Yield-SAFE is the simplest mechanistic AF model but it is one-dimensional while other models are two and three-dimensional (complex). It includes simple light capture algorithms and was originally designed to describe an alley cropping silvo-arable system (van der Werf et al., 2007). With the addition of new algorithms to the Yield-SAFE and Farm-SAFE models, they can now simulate ESS (García de Jalón et al., 2018b) but still lack assessments of the dynamic processes. As the time step for the model is a day, it is assumed that each part of the alley cropping field will receive the same shade from the trees when this is not the case (Luedeling et al., 2016).
Water, Nutrient, and Light Capture in Agroforestry Systems (WaNuLCAS) is a more complex 2 D (Figure 1). AF model (Van Noordwijk and Lusiana, 1998). It includes a vertical four-layer soil profile with four horizontal spatial zones and it has the potential to simulate the interactions of trees and crops for water, nitrogen balance, and uptake, either by crop or tree. While the WaNuLCAS model is used to assess the effects of trees on crops by considering the distance between the two, it assumes that the light interception is the same in both directions, either West-East or North-South. In reality, this is not the case for row orientation, and a three-dimensional model is required to truly simulate the light and shade effects, and especially the microclimates. The Hi-sAFe AF model (Dupraz et al., 2005) is the most recent example of a three-dimensional model (Figure 1), as it was developed using a combination of the crop (PARCH model) and tree models (Hybrid). Hi-sAFe can simulate different conditions like isolated tree systems, tree hedges, alley cropping, and crop and forest mono-cropping (Artru et al., 2017). It also has a well-developed system for root competition and has many other tree and crop management options. It can consider the impacts of a fluctuating seasonal water table on tree–crop competition (Lecomte et al., 2016).
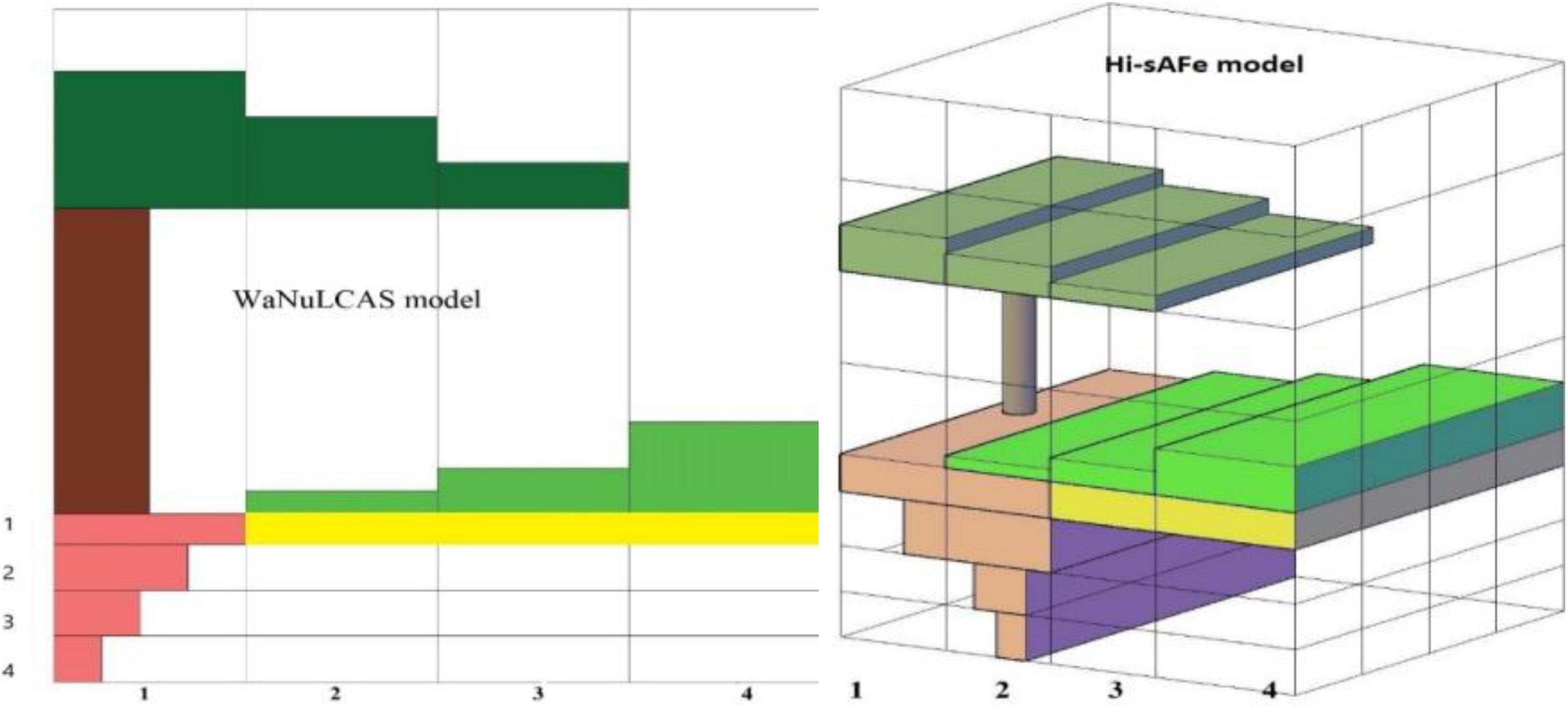
Figure 1. The WaNuLCAS described by Van Noordwijk and Lusiana (1998) comprises four root zone depths (vertical zones) and four distances from the tree (horizontal zones) and the same four layers are included above ground for light. Hi-SAFE (3D) model zones represent the canopy (dark green color), distance from a tree (light green) and below ground, soil, and root (dark purple) (Dupraz et al., 2005).
The Agricultural Production Systems Simulator (APSIM) framework is flexible and can be used for AFS modeling (Holzworth et al., 2018), and is currently used for intercropping and tree and crop interactions. Smethurst et al. (2017) described the use of the APSIM Next Generation framework to provide a 2D representation of Gliricidia-maize intercropping, and it was found that it could accurately describe many ESS related to soil, crops, and trees. However, a static proxy of the Gliricidia trees was that it lacked a process-based simulation. There are many types of AF models available for large-scale assessments, such as for farm-scale management, architecture models, landscape models, economics, and financial models but we have focused on field scale AF models to identify the opportunities and challenges of integrating them into developed crop MFW to develop a comprehensive system for accurate simulations of tree and crop interactions in AFS. Detailed descriptions of the different types of models and the simulations of different ESS and processes under AFS are mentioned in Table 2. Although there have been efforts by the modeling community previously to develop a dynamic, process-based modeling system for AFS, there are still many limitations and challenges, and in particular for the simulation of ESS and BD.
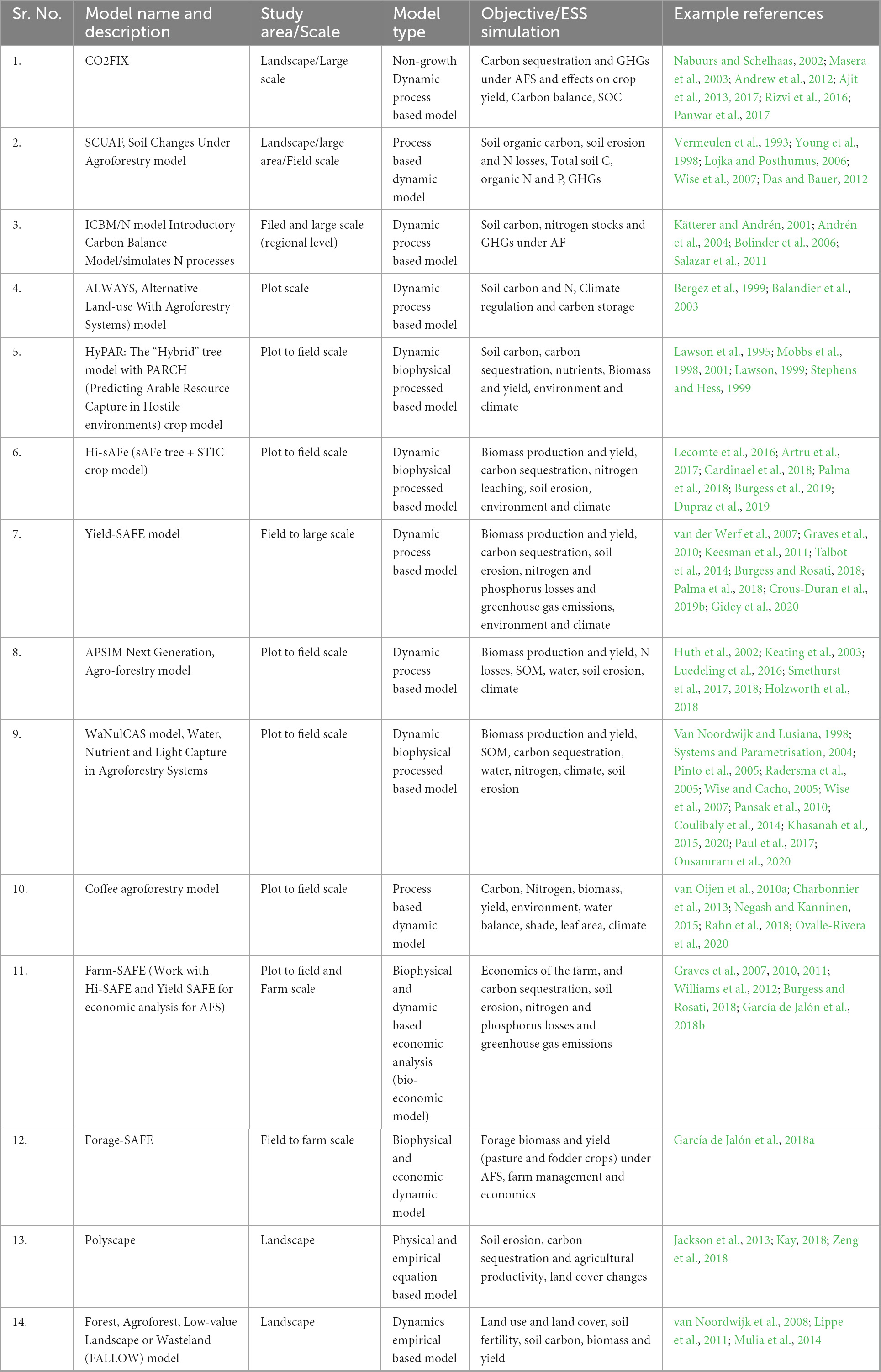
Table 2. Ecosystem services (ESS) simulations using available modeling approaches for agroforestry systems (AFSs).
3.1.3. Biodiversity modeling, overview, and potential for ESS estimations
AF is regarded as a potential solution for the provision of ESS and the conservation and improvement of BD (Jose, 2009). Modern agriculture and innovation practices have affected BD (Zhang and Liu, 2005; Blanco-Canqui and Lal, 2010). Plant diversity, however, enhances soil quality, reduces erosion, supports agricultural productivity, and promotes BD (Sistla et al., 2016; Udawatta et al., 2019). AF significantly promotes BD through the provision of improved interactions between the soil, plants, water, and microclimate. BD is thus crucial for ecosystem maintenance and especially for the promotion of ESS and environmental functions (Wise et al., 2007; Jose, 2012; Sistla et al., 2016). Different types of models are used to study BD in forestry and AF practices, and they range from simple to complex and dynamics-based models and each has both potential and limitations. Although, some models are appropriate for the simulation of ESS and can simulate the new management strategies to enhance BD and ESS, including environmental and production benefits. Studies have shown a strong relationship between BD and ESS and stressed that BD conservation is required for the promotion of ESS. ESS provided by BD can help to maintain the productivity of cropping systems and reduce the application of the inputs responsible for environmental complications and hazards (Chopin et al., 2019). Different types of approaches are used to simulate the relationship of ESS with BD under AFS. Generally, most studies related to the assessment of the impacts of BD and its relationship with ESS have been at a large scale (landscape) and limited studies are available for field scale assessments, and especially for infield heterogeneity or subfield assessments. The main reason given for not changing to an AFS is the lack of AF models and knowledge, especially for the provision of ESS (Sagastuy and Krause, 2019). Details about modeling approaches for BD are mentioned in details in sections 4.6 and 5.7.
3.1.4. Soil erosion models, overview, and potentials for simulation under AFS
Soil erosion (wind and water) is a serious environmental problem in current agricultural production systems and specifically, in regions with different elevations and slopes, as it threatens their sustainability (Magcale-macandog, 2002; Rodrigo-Comino et al., 2020). It has serious implications for agricultural production levels, due to the loss of ESS and BD (Bakker et al., 2007; Panagos et al., 2018). Approximately two billion hectares of land, which is one-third of the world’s agricultural soils, are already under threat of degradation, and the major causal factor (approximately 1.1 billion hectares) is water erosion (Lal, 2001; Panagos et al., 2015b). Soil erosion reduces the soil quality and quantity, replenishment of nutrients, and ultimately negatively affects ESS and the loss of BD (Powlson et al., 2011; Palm et al., 2014). Soil erosion models and modeling frameworks (MFW) were developed for large-scale simulations but have also been applied at smaller scales (Avwunudiogba and Hudson, 2014; Evans and Boardman, 2016; Igwe et al., 2017; Bagarello et al., 2018). Most soil erosion models are simple, physical, and empirical equation and process-based and have limited applications for field scale predictions, especially in AFS (Avwunudiogba and Hudson, 2014; Igwe et al., 2017). Although some have made reasonably good predictions, there are still limitations, issues and challenges for field scale assessments in AFS. Each soil erosion model is specific for a certain management type and widely applied at large and watershed levels. Detail limitations, challenges, and issues of each previously developed soil erosion model are described in section 4.
Although processed-based models have been developed for field scale assessments, they require large data sets. Based on the findings for soil erosion modeling under AFS at the field and subfield levels, the limitations of the existing soil erosion models and their applications were identified. Innovative modeling approaches and initiatives are required in the future to meet the current demands for ESS simulation with improved accuracy and reliability. Previously, models were developed but improvement, maintenance, and testing were not conducted. Similarly, the main issues were the large data set requirements, complexities in the structure, complex computing environments, detailed basic knowledge requirements, and the gradual shift to other models, are among the top factors limiting their applicability. Each model and modeling framework for AF, BD, and soil erosion have several limitations in their current form for use with ESS and BD simulations at field scale. A modeling framework that can simulate ESS and BD in AFS with reasonable accuracy and reliability is required to improve management decisions.
4. Existing models and modeling frameworks of AF and biodiversity for ESS simulation
There have been several attempts to model tree–crop interactions at various levels of detail, including HyPAR (Mobbs et al., 1998), WaNuLCAS (Van Noordwijk and Lusiana, 1999), and the SAFE family of models [Hi-SAFE (Talbot and Dupraz, 2012) and Yield-SAFE (van der Werf et al., 2007) that form the basis for plot-SAFE and Farm-SAFE (Graves et al., 2011)]. There are also models for the interactions of windbreaks and crops in the Sahel (Mayus et al., 1998) and AFS based on coffee trees (van Oijen et al., 2010b). A basic functionality exists for model trees within the APSIM crop modeling framework, but there are limits regarding tree–crop interactions (Huth et al., 2002). These tree–crop interaction models, capture interactions to variable degrees, but often fall short of accurately predicting attainable yields for tree and crop components simultaneously and over a range of conditions (Walker et al., 2007; Bazié et al., 2012). Furthermore, they are dependent on the model and the number of ESS simulated. In this investigation, we considered the simulation potential of these AF models for above (light) and below-ground (soil, water, carbon, and erosion) interactions, dynamic processes, and model structures. The working interactions and simulations of the ESS at the field scale were also recently applied and tested for their prediction of ESS and flexibility.
4.1. Yield-SAFE and Hi-sAFe AF models
Yield-SAFE (Yield estimator for long-term design of Silvo-arable Agroforestry in Europe) is a simple 1 D biophysical mechanistic AF model (van der Werf et al., 2007). Initially, the model was developed with only a few equations and parameters. It has been used for the prediction of farm-scale growth, productivity, yield, and economics in Europe (García de Jalón et al., 2018b). Although it is a simple model that consists of seven equations, it has the potential to simulate biomass and leaf area, the number of shoots/branches of a tree, soil water content, and daily total heat (Graves et al., 2010). It includes simple light capture algorithms and was originally designed to describe an alley cropping silvo-arable system (van der Werf et al., 2007). The outputs of Yield-SAFE are used in Farm-SAFE as inputs and it is also used to calculate economics and profitability (Burgess and Rosati, 2018). By adding new algorithms to the Yield-SAFE and Farm-SAFE models, they can be used to simulate ESS like carbon sequestration, soil erosion, nitrogen and phosphorus losses, and greenhouse gas (GHGs) emissions (Graves et al., 2010; García de Jalón et al., 2018b; Palma et al., 2018; Crous-Duran et al., 2019a). A recently developed 3D AF model is the process-based dynamic Hi-SAFE model for the estimation of ESS in AFS (Dupraz et al., 2005, 2019; Luedeling et al., 2016). It has a unique structure, as it is integrated with a well-developed crop model STIC (Brisson et al., 1998) that has a general tree model with the interaction of submodules that simulate light, water, nitrogen, and competition reasonably well for both tree and crop components (Brisson et al., 1998; Graves et al., 2010; Artru et al., 2017; Dupraz et al., 2019).
The model simulates a rectangular scene, which can be replicated in all directions via periodic boundary conditions. For a better representation of the competition dynamics, the scene is divided into “cells,” where each cell grows either a crop or a tree (Dupraz et al., 2019). Alley cropping (cropped area) and uncropped area (bare soil) closer to the trees are defined based on the tree’s position. Furthermore, the cells are subdivided into separate “voxels” for a better prediction of the below-ground processes including root densities and water and nitrogen pools and fluxes (Dupraz et al., 2019).
A new dynamic approach for the modeling of tree root systems was also developed using the voxel concept (3D architecture), to control root growth and development while the carbon allocation to each root segment in each rooted voxel depends on the water uptake in the voxel during the previous day, as compared with the overall supply and demand at the plant level (Mulia et al., 2010). In Hi-SAFE, root competition is addressed using a 3D opportunistic tree root growth module while soil water extraction and competition between plant components are managed at the voxel scale, allowing for dynamic water extraction in 3D heterogeneous soil. The new approach considers competition for the extraction of soil water between the plant components as well as the effect of the groundwater table (Lecomte et al., 2016). Most recently, Dupraz et al. (2019) revealed the potential of the model for the evaluation of AF designs, tree and crop management, and the simulation of the ESS related to soil, fertility, and responses to climate change, but this analysis requires a detailed understanding of the complex processes and interactions. The Yield-SAFE model is only one-dimensional and thus only considers some of the horizontal extents of the arable crop.
4.2. Soil changes under agroforestry
Soil changes under agroforestry (SCUAF) is a process-response model that simulates the effect of specific land-use systems and soil conditions on crops, forests, and AF (Young et al., 1998). It simulates the effects of trees on soil carbon and land conservation and predicts soil loss and productivity (Nelson et al., 1997). It can predict the differences in soil properties with crops, forestry, and AF (Vermeulen et al., 1993; Das and Bauer, 2012). It is a simple model that is used to assess the changes in crop yield (annual or perennial) due to changes in the soil carbon, nitrogen, and phosphorus content with different land uses. Furthermore, it can also simulate soil loss, the recycling of plant material, and mineral uptake by plants in a specified system under specific environments (Young et al., 1998; Ellis and Schoeneberger, 2004). Soil loss and erosion are predicted using a simplified version of RUSLE, which is based on climatic conditions, soil erodibility, slope characteristics, and crop cover factors (Lojka and Posthumus, 2006; Das and Bauer, 2012). SCUAF is not a plant growth model like the other well-developed models (Mobbs et al., 1998; Ellis and Schoeneberger, 2004). It is user-friendly and has a physical environment under different land-use systems (climate, soil, slope, and initial condition). It can simulate soil processes reasonably well but lacks above-ground simulations, especially for light competition in AFS. The model also has several limitations in terms of light competition between trees and crops, nutrients and water, and even growth rates. As the model works annually, it is unable to predict crop growth processes at a temporal scale (Young et al., 1998; Lojka and Posthumus, 2006; Luedeling et al., 2016).
4.3. HyPAR model for agroforestry
HyPAR is a limited “Hybrid” forest model and “PARCH” (Predicting Arable Resource Capture in Hostile environments) crop model that was recently used for the evaluation of different management options under AFS (Mobbs et al., 1998, 2001). Previously it has been used to predict AF productivity (Muthuri et al., 2004). Although, it is a biophysical process-based model and has the potential to simulate light, water, nutrient competition, and daily carbon allocation, its use is limited due to complex challenges and poor responses to interaction processes (Stephens and Hess, 1999; Holzworth et al., 2015). It works on a daily time step when assessing physiological and other processes (photosynthesis, respiration, nitrogen uptake, and transpiration). While canopy light interception is computed by adopting a disaggregated approach for tree and crop plants. The 3D approach is also being used for water and nutrient competition depending on the root length density (Lawson et al., 1995; Stephens and Hess, 1999; Holzworth et al., 2018). The crop developmental phases depend on thermal time and drought but have limitations when simulating microclimates and the interactions of trees and crops (Mobbs et al., 1998, 2001). The tree model simulates competition based on physiology (photosynthesis, stomatal conductance, and transpiration) and canopy height, while nutrient fluxes are determined at different soil depths. Physiology and light competition are computed using a “big leaf” model, which simulates the leaf canopy in different layers and then considers the average horizontally across a large plot (Mobbs et al., 1998; Luedeling et al., 2016).
4.4. Water, nutrient, and light capture in AFS
WaNuLCAS is the most advanced dynamic, process-based simulation model for AFS. It is an example of a complex 2-D model at the field and plot scale (Van Noordwijk and Lusiana, 1999). It is the only specialized AFS model that has been consistently updated, refined, and regularly used over many years in a range of geographic settings (Khasanah et al., 2015). It is capable of simulating a wide range of geometric arrangements and different temporal patterns but was primarily designed for tropical regions i.e., tree and crop species. It was last updated in 2011 but is still under active development and has been widely used by researchers (Hussain et al., 2016; Boote, 2019; Khasanah et al., 2020). Its high level of complexity, however, makes it less user-friendly. The large array of spatial arrangements and processes that are simulated by the model requires a substantial understanding of the underlying processes. For the spatial arrangement of crops and trees, the user can define zones and subdivide them vertically and horizontally in layers (Figure 2). The user can also prescribe spatial patterns for tree and crop interactions (Van Noordwijk and Lusiana, 1998). It has specific modules for soil water, nitrogen, and phosphorus dynamics, as well as crop and tree growth and development processes. The soil in each zone, defined by its distance from the tree, is subdivided into four vertical soil layers (Figures 1, 2). It requires specific sets of input data like soil conditions, tree and crop properties, as well as information about crop and tree management. The flexible structure allows the configuration of various combinations of trees and crops for different production systems like mono-cropping, mix cropping, fallow system, and alley cropping. Tress can be planted in both zone one and four (which form the outer zones) (Figure 2), while zone widths and layers can be changed according to the experimental treatment structure. The below or above-ground competition can be monitored in each zone and distance from the tree to crop plants (Van Noordwijk and Lusiana, 1998; Coulibaly et al., 2014). Details about the core and submodules are presented in Table 3.
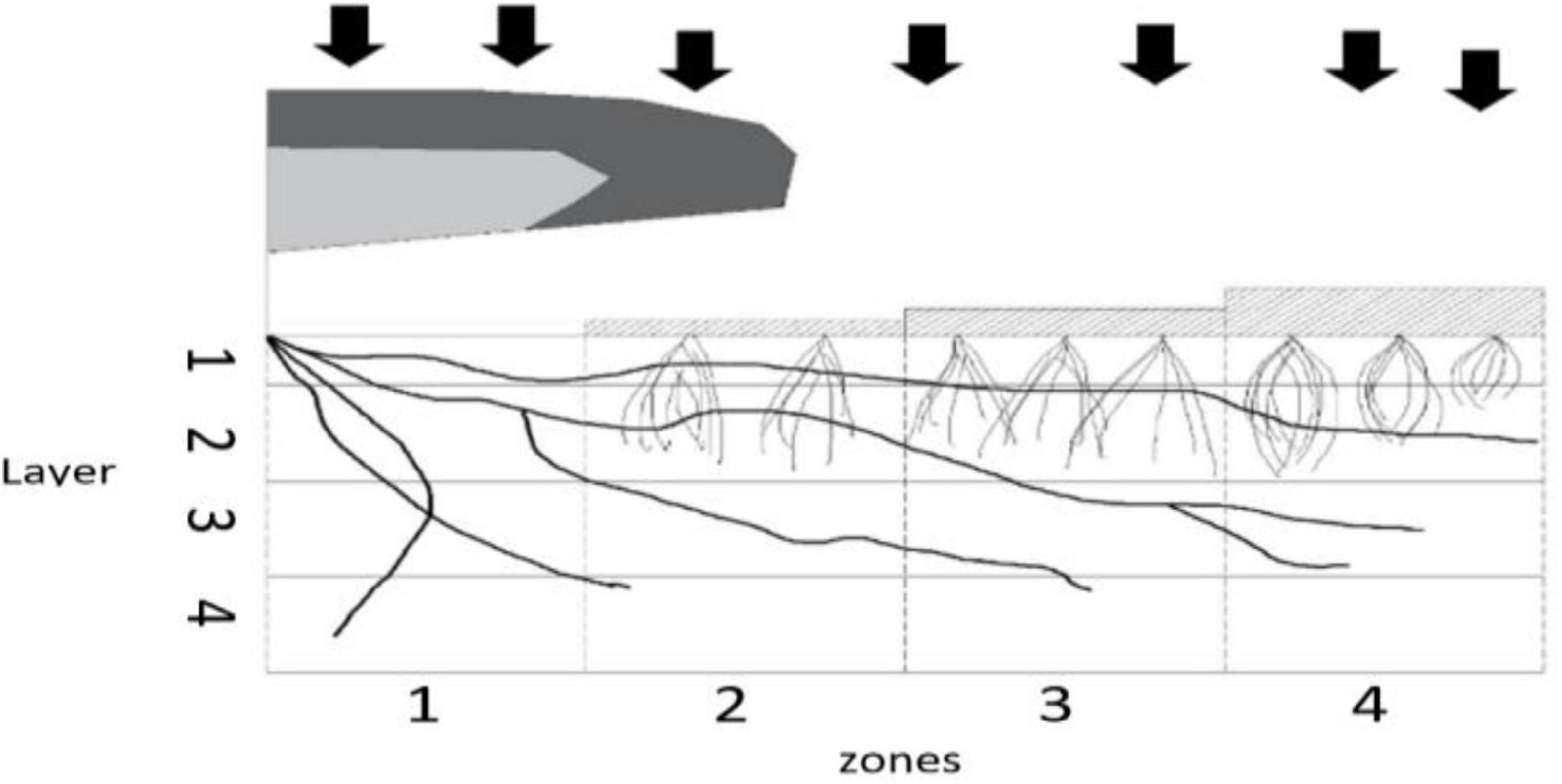
Figure 2. WaNuLCAS 2D spatial discretization with four soil depths (vertical layers) and four distance zones from a tree (horizontal layers), and the same is applied to the above ground canopy for light competition.
4.5. APSIM-a next generation-AF model
APSIM is a crop model that is widely used in research and for decision management (Keating et al., 2003). Recently, APSIM next generation was launched and it includes an AF module that can simulate crop and pasture production as well as several important interactions between trees and crops (Keating et al., 2003; Huth et al., 2010, 2014). Although it is currently under development, promising results have been reported for the simulation of ESS under AFS in studies of nitrogen fixation and how it is affected by trees and legumes crops, how carbon and nitrogen are influenced by tree litter on soil, the competition of water and nitrogen between trees and crops, and the lower crop planting densities near trees (Huth et al., 2002; Dilla et al., 2018; Holzworth et al., 2018). The model simulates crop production based on the distance from a tree by considering user-defined zones. While a simple tree sub-model is added which facilitates the addition of input data about the tree and further enables the simulation of interactions. Currently, a complete dynamic tree model is being developed to simulate wood production, which will be more dynamic and improve our understanding of tree and crop interactions. The currently available APSIM-AF model uses a tree proxy in the recently released APSIM version. It is a significant step for AF modeling as, it can simulate tree production (Gliricidia, Faidherbia, Acacia, and Eucalyptus) by using tree-defined proxies for a single tree and tree belt examples. While an active tree model is also being developed for the accurate simulation of tree and crop interactions. It computes interactions based on zones in the model and further includes smaller zones (trees) to simulate the soil and plant processes. It uses the information about the tree and especially the structure of the tree-like the height and canopy dimensions, from a smaller zone (tree model) to calculate effects and interactions. The effects of windbreaks are also simulated in the current version by computing the wind speed in the cover of windbreaks as a distance function. Generally, the height of multiple trees is used to determine the optical porosity of the windbreaks (Huth et al., 2002; Keating et al., 2003; Smethurst et al., 2017, 2018). Although, APSIM is not a basic modeling framework for AFS it has submodules that can facilitate processes related to trees and the interactions of trees and crops (Huth et al., 2010). While the AF module showed good results, it requires improvement in many submodules for the reliable and accurate simulation of a tree and crop interaction, and for the simulation of ESS under AFS (Mokany and Ferrier, 2011; DeAngelis and Grimm, 2014; Smethurst et al., 2017).
4.6. Biodiversity models and modeling frameworks for the simulation of ESS under AFS
Generally, different modeling approaches are used to assess the impacts of BD on ESS i.e., agent-based models (vegetation models), mechanistic models, statistical models, and landscape indicators (Botkin et al., 2009; Guisan and Rahbek, 2011; Chopin et al., 2019). Agent-based dynamic models are used to assess the spatial and temporal changes in BD (Kirby and Potvin, 2007). JABOWA (acronym derived from the last names of the developers, Janak, Botkin, Wallis) is an example of a forest gap model (Smith et al., 2016) that simulates the effect of shading concerning tree height in a small geographical area and at field scale. There are many other examples of forest gap models that were previously developed for specific objectives under certain conditions (Bugmann, 2001). These previously developed models, however, did not meet the recent modeling requirements, in terms of combined ESS and BD simulations, and especially for AFS. While there is a more robust ESS simulation for tree growth, dynamics are also considered in recent modern gap models (Chopin et al., 2019). The dynamic vegetation model (DVM), is an example of an indigenous small-scale forest dynamic gap model, with improved simulation when compared with Dynamic Global Vegetation Models (DGVMs), which involve functional plant compositions from mechanistic models at large scales. Recently, a “Spatially Explicit Species Assemblage Modeling” (SESAM) framework (Reed et al., 2013) and Dynamic Framework for Occurrence Allocation in Meta communities “Dynamic FOAM” (Guisan and Rahbek, 2011) were proposed by the modeling community, as they consider the interactions and diversity patterns more explicitly than other models (Botkin et al., 2009). Different statistical approaches and equations are also being used to model the simple relationship for independent and explanatory variables for BD impact assessments in AFS at a spatial scale for the distribution of patches (Guillem et al., 2015; Sistla et al., 2016; Chopin et al., 2019). Different statistical packages in “R” and simple models are being used for predictions and to assess relationships to make diversity and BD estimations.
Process-based mechanistic models assess changes in BD using a range of equations. These models link the variation among different variables for BD, generally at a smaller scale, and are helpful to understand the relationship between species and populations in different communities, such as the occurrence of sage-grouse with cropland (Grimm et al., 2017). Such models are process-based and there are many types, like a biophysical, prototype, and resource use models that depend on the nature of the study and can be used to assess BD and its impacts at the field scale (163). Large data sets at a temporal scale are required for these models based on multiple continuous field observations, as this allows for daily simulations (Arroyo-Rodríguez et al., 2017). The simulation of process-based mechanistic and vegetation-based models is more appropriate as they have the potential to account for the processes and underlying facts (Wang et al., 2016; Smith et al., 2019).
Landscape indicators can also be used to identify the state of BD using simple equations without any explicit model structure. Other indicators used to assess the state of BD are habitat suitability, crop, and land cover in a region, as higher habitats showed increased BD, but these are simple assessments and not true modeling. In studies related to modeling BD, the processes are simplified to better adopt the modeling techniques and assess the BD (Botkin et al., 2009; Chopin et al., 2019). Bayesian hierarchical modeling is also used for the assessment of BD and the relationship of ESS with communities in the ecosystem, it is a flexible approach used to integrate and incorporate different components at temporal and spatial scales (Grimm et al., 2017; Ovalle-Rivera et al., 2020).
5. Limitations and key challenges of current AFS models for the simulation of ecosystem services and biodiversity
5.1. Soil protection against erosion at the field scale
5.1.1. AFS models for soil protection and conservation
Currently, available AF models have been developed for certain ESS simulations but are lacking for soil erosion assessments and soil conservation under AFSAFS (Van Noordwijk and Lusiana, 1998; Luedeling et al., 2016; Dupraz et al., 2019; van Oijen et al., 2020). Only a few AF models (Hi-SAFE and WaNuLCAS) are widely applied for ESS estimations and others (SCUAF, HyPAR, and HyCAS) have rarely been tested in recent years. While a few AFS models have been developed and applied (Mobbs et al., 1998; Van Noordwijk and Lusiana, 1998; Dupraz et al., 2019), but were not tested dynamically for soil protection and conservation at the field scale (García de Jalón et al., 2018b; Onsamrarn et al., 2020). Models such as HyPAR consequently have limitations regarding basic soil processes, as its simulations of water, runoff, soil water storage capacity, drainage, and water holding capacity are poor, which means it is unsuitable for soil erosion and conservation estimations. Simple empirical and physical relationships are used for runoff simulations at different rainfall intensities and depend on the function of the slope, surface roughness, vegetation cover, and saturation of the soil but have limitations in dynamic simulations at the plot-to-field scale under AFS (Mobbs et al., 1998, 2001). Limited AF models (Hi-SAFE, APSIM-AF, and WaNuLCAS) have functional modules for soil erosion but to the best of our knowledge, these have not been widely tested, and most of these models lack proper simulations for AFS, especially at the field scale. The SCUAF model estimates the effects of hedgerow intercropping and reduced tillage for the control of soil erosion. It supports farm management decisions regarding the adoption of hedgerow intercropping and reduces the erosion effect (Das and Bauer, 2012). It has also been used for soil erosion prediction under different vegetation covers (shrubs and perennial grass) (Lojka and Posthumus, 2006), and has been applied to model the changes in erosion within various AFS like maize and Miombo woodlands. The Hi-SAFE model also has limited applications for soil erosion and is limited regarding the dynamic processes at the plot-to-field scale. Although it can be modified and utilized for soil erosion under AFS, this has not yet been widely reported (Luedeling et al., 2016; Dupraz et al., 2019).
Recent advancements in the APSIM-AF module are being assessed, but they have rarely been applied and tested in soil protection and conservation studies (Huth et al., 2002; Smethurst et al., 2017). Soil erosion processes are simulated with the aid of the Freebairn erosion module, which is a modified form of the USLE equation (Hagen, 2004) and the rose equation for sediment concentration function (Hagen et al., 2009). Erosion is simulated by considering the surface cover and runoff and uses slope-length, erodibility, and supporting practice factors. The surface cover value is derived from the surface organic matter module and accounts for combined crop and residue covers on the soil surface. Currently, the soil erosion module does not explicitly include a crop management factor but the supporting practice factor is used for cover crops. While soil erosion simulations have not been tested in AFS to evaluate the effects of trees and hedges on soil erosion and their ability to act as a soil barrier to prevent soil loss at different slopes at the field scale (Nerger et al., 2017; Smethurst et al., 2017).
WaNuLCAS also did not meet the recent interest and was not developed, especially for the simulation of soil protection and conservation. Complexities in the model lead to lower adoptability and wider application. Although it has been widely used for the simulation of ESS under AFS at the field scale and it has a sub-module for water-induced soil erosion and different possible management options, it can be simulated to see the effect of trees and crop combinations on runoff and soil losses (Pansak et al., 2010). The physically based rose equation for the prediction of runoff and soil loss is used but still, but this module has not yet been well tested. Although it can simulate temporal dynamics of the soil structure, other aspects related to runoff and especially soil loss have only been satisfactorily predicted on a limited scale in a hillside cropping system (Pansak et al., 2010). Although its application for the simulation of ESS is still limited, there have been studies on the promotion of ESS and soil conservation, as these sub-modules are not fully developed. Furthermore, the model structure and data requirement-related complexity make it less user-friendly (Fryrcar et al., 2001; Paul et al., 2017).
Most AF models and developed frameworks have adopted dynamic processed-based approaches to simulate soil erosion-related processes, and they range from simple 1D to complex 3D models (Holzworth et al., 2018; Dupraz et al., 2019). Most available soil erosion models are simple, physical, and empirical, and were developed for large-scale use and consequently, are rarely used for field scale or AFS level assessments of soil erosion and conservation. Process-based models are also being applied at both large and small scales with different management strategies (Huth et al., 2002; García de Jalón et al., 2018b; Jiang et al., 2019). The complex interaction processes in AFS and the unavailability of long series of dynamic data are possible reasons, however, for the limited development of innovative dynamic processed-based models for soil processes, soil erosion, and the interaction of hedgerows and crop plants. The function for wind erosion and to simulate the effects of windbreaks (reduced wind speed and soil loss by wind) are limited in the previously discussed AF models (Mayus et al., 1998; Huth et al., 2002; Dupraz et al., 2019), but there are simple empirical and physical-based models available like SWEEP (Hagen, 2004; Hagen et al., 2009; Nerger et al., 2017), RWEQ (Meyer et al., 2001; Pi et al., 2017; Jiang et al., 2019) and WEPS (Hagen, 2004; Laflen and Flanagan, 2013; Li et al., 2014).
5.1.2. Soil erosion models and modeling frameworks for soil protection and conservation simulations at field scale
Most soil erosion models that have been developed for soil loss assessments are physical and empirical-based, while some use a process-based equation (Avwunudiogba and Hudson, 2014; Igwe et al., 2017). Universal and revised universal soil loss equations are currently lacking sediment deposition processes (Wischmeier et al., 1978; Wischmeier and Smith, 1978), while process-based physical models like the European Soil Erosion Model (Morgan et al., 1998) are lacking simulations for saturation overland flow and erosion by the ephemeral gullies. RUSLE is an empirical model, and consequently, it does not consider the detachment of sediments or take runoff into account (Igwe et al., 2017; Alewell et al., 2019). This model instead focuses on soil erosion estimations at the landscape level with clear land (without vegetation), and it was not originally designed for forest and AFS. Its application is limited to the stream bank and gully erosion and hence its applications for AFS at field scale are limited (Panagos et al., 2015a,2018; Alewell et al., 2019), however, it can be used with modifications to the AFS equation (Panagos et al., 2015a,2018; Alewell et al., 2019).
The Watershed Erosion Prediction Project (WEPP) is a process-based physical distributed complex model that showed good results for small catchments and at the field scale levels for soil loss and erosion estimations, but it requires a large and detailed data set for accurate prediction. It is useful for the prediction of soil erosion impacts with different levels of vegetation for forest management, it shows that organic matter and nutrient loss are lower under tree systems (Zhang and Liu, 2005). It requires modifications to assess spatial soil and vegetative cover variability, and only a limited number of studies have assessed AF at large and field scales (Chandramohan et al., 2015; De Mello et al., 2016). Griffith University Erosion System Template (GUEST) is a physical model that works at the plot scale and field level, it simulates runoff and sediment concentrations but has only been tested for AF at field and subfield levels (Igwe et al., 2017; Hajigholizadeh et al., 2018). The rose equation is used for erosion and deposition processes (Brisson et al., 2003). Productivity, Erosion, and Runoff Functions to Evaluate Conservation Techniques is a physical-based model, and while it was developed for field scale use, it could potentially be used to assess the effects of crops while sediment yield is simulated using MUSLE. It considers the water balance and runoff and could potentially simulate crop growth and yield. It incorporates the planting sequence and harvesting, can manage the stubbles during fallow, and be used for AFS with proper modifications (Alewell et al., 2019). Although EPIC is a cropping system, it can simulate soil erosion (Williams et al., 1983, 1989). Consequently, it has been widely applied to assess soil erosion when different management practices for forests and trees are used, but studies are limited regarding the accurate predictions in AFS at the field scale. It uses RUSLE, but is still flexible and can be used with modifications in AFS to consider the interactions of trees and crops (Stolpe, 2005; Williams and Renard, 2015; Maharjan et al., 2018). EPIC has been widely applied to assess soil erosion under different management practices for forests and trees, but studies regarding the accurate prediction of soil erosion under AFS have been limited.
Many empirical and physical equation-based models are widely used for soil erosion estimations at a large scale but are limited for field-scale measurements. While processed-based models are also being applied at both spatial and small scales with different management strategies but have had limited developed for the assessment of soil erosion at field and subfield levels (Huth et al., 2002; Jiang et al., 2019). Each model is thus specific for certain management strategies and widely applied at large and watershed levels. Although processed-based models have been developed and applied for the field scale level they require larger data sets. Soil erosion modeling for AFS at both the field and subfield levels has shown that there are limitations in the existing soil erosion models and their applications. Furthermore, research and development for specific soil erosion dynamic process-based modules is required for accurate and reliable predictions, the management of AFS and for the provisions of ESS and BD conservation for sustainability.
5.2. Onsite water retention to prevent flooding
AFS could potentially conserve soil through the prevention of floodings, such as reduced runoff, increased infiltration by hedgerows, and the addition of liter and organic matter (185, 186). However, AF models have limitations in terms of their water retention estimations and flood measurements due to their complex structures. Efforts have been made in a few AF models, but there are still limitations as these models lack accurate estimations for these effects under AFS (Wösten et al., 1998; Pansak et al., 2010; Dufour et al., 2013; Onsamrarn et al., 2020). Among the AF models, HyPAR is rarely used, in part because it has poor simulations for hydrological properties (infiltration, water storage capacity, drainage, and water holding capacity) and lacks accurate function estimations for water retention and flooding. Simple empirical and physical relationships are used for the simulation of runoff but they are limited for accurate dynamic process-based simulations (Mobbs et al., 1998, 2001; Luedeling et al., 2016). Soil organic carbon correlates with hydrological properties, but models are generally poor at making accurate estimations for carbon allocation to different components as it follows simple algometry rules. Tree and crop root competition for water is poor and the plasticity of tree rotting systems is not considered in the majority of below-ground resource allocation and sharing for AFS (Das and Bauer, 2012; Holzworth et al., 2015).
Hi-sAFe also has limitations for soil water competition and interactions, and especially for horizontal soil water which is not modeled when integrating STICS into a 3D heterogeneous environment (Brisson et al., 1998; van der Werf et al., 2007). As both model structures are different, it makes the model structure complex, and especially the communication between the two different models and algorithms used to aggregate and disaggregate between the 1 cm thick mini layers of STICS and the ∼20 cm thick voxels of Hi−sAFe (Brisson et al., 1998; Kay et al., 2019). The model has difficulty making accurate simulations for flooding, water infiltration, water holding, and runoff estimations at different rainfall intensities, as it assumes a homogeneous soil depth D (m) and volumetric water content (Lecomte et al., 2016; Dupraz et al., 2019). The APSIM-AF model could potentially simulate ESS in AFS, but has limitations in terms of waterlogging, water flux, infiltration, runoff, and water flooding especially due to pre-defined crop zones. As below-ground interactions between trees and crops are calculated by the APSIM Soil Arbitrator module, which is concerned with the competition among zones, it is assumed that there are no fluxes of water among the zones (Smethurst et al., 2017). Water demands are determined by the tree leaf area using APSIM standard algorithms, which may not result in accurate simulations (DeAngelis and Grimm, 2014). The modules used in the APSIM next-generation model have limited abilities to assess soil hydrological properties like runoff, drainage, and infiltration. The model appears to work well for water stress and nitrogen but needs further development to assess nutrients and a boarder range of trees and crops. Furthermore, extensive assessments of its application in contrasting environments to estimate water retention and prevent flooding in AFS are required (Smethurst et al., 2017; Holzworth et al., 2018). Submodules in WaNuLCAS calculate the infiltration and runoff based on rainfall intensity, time for rain interception, surface cover, and soil slope, and the rose equation is used for flooding and soil loss (Brisson et al., 2003; Heinlein et al., 2008; Francaviglia et al., 2012). Several factors control the soil loss, while runoff is only affected by the infiltration rate. Pedo-transfer functions based on soil texture, soil structure, soil organic matter, and bulk density are used to estimate the saturated hydraulic conductivity (Cardinael et al., 2015). It can simulate the temporal dynamics of soil structures but other aspects like runoff and flooding have only had limited satisfactory predictions on a hillside (Pansak et al., 2010).
5.3. Carbon sequestration and soil biodiversity
AF improves soil health through the provision of microorganisms by adding leaves and liter, which ultimately enhances SOC, nitrogen availability, and soil biodiversity (Wise et al., 2007; Powlson et al., 2011). Several models have reportedly been used for carbon sequestration and soil health in cropping systems. Studies are limited though for AFS, as models showed limited potential for the simulation of long-term carbon sequestration, soil organic carbon, the amount of liter (leaves), biomass, and the yield of trees and crops at the field scale (Lojka and Posthumus, 2006; Wise et al., 2007; Nabuurs et al., 2008; Malézieux et al., 2009; Cardinael et al., 2018; Burgess et al., 2019). However, SCUAF has been used for the last 20 years to predict different management scenarios for hedgerow intercropping and AFS but limitations were also identified in terms of the reliability of the carbon sequestration predictions. Although, several models have been suitable in simulating the interactions of trees and crops they are not suitable for SOC dynamics in deeper soil layers and SOC storage in AFS (Palma et al., 2007b; Burke and Poncé-Hernandez, 2014; Dilla et al., 2019; Abbas et al., 2020). The CENTURY model has the potential to simulate SOM and soil biodiversity under AFS (Cardinael et al., 2018) but has not been recently tested. Previous studies of SOC predictions for carbon stocks in tropical and temperate AFS, used a single-layer modeling approach (0–20 cm) but did not assess the deep soil layers (Muthuri et al., 2004; Cardinael et al., 2018). Simulations of vertically discretized soils are important to consider in modeling, especially in AFS for soil organic carbon stocks, but to the best of our knowledge, vertically spatialized SOC models have not yet been tested.
Other soil and biodiversity-related models have also had limited testing, especially at the field scale (Botkin et al., 2009; Chopin et al., 2019). Similarly, the uncertainties and challenges for the accurate predictions of CO2FIX were also previously reported and require improvements for carbon stock calculations (Mäkipää et al., 2012). Carbon allocation is also not satisfactory in the HyPAR model as it follows simple allometry rules for carbon allocation to different components as tree height is deduced from the tree diameter in the tree model. Tree and crop root competition for carbon is poor and the plasticity of tree rotting systems is not considered in the model. While the most below-ground resource allocation and sharing is satisfactory and a mixed continuous and disaggregated approach is used in the model which is not satisfactory for AFS (Mobbs et al., 1998; Das and Bauer, 2012; Holzworth et al., 2015). Several modeling studies of BD assessments showed that AF promoted soil biodiversity which improved environmental performance in Europe. The effects of the environment are simulated by the Hi-SAFE model by modifying the existing modules and algorithms. Carbon sequestration, SOC, SOM, nitrogen leaching, and landscape diversity indicators were compared with arable systems. While carbon sequestration and landscape diversity were promoted, overall, there was a positive impact on ESS and BD (Amadi et al., 2016). Positive relationships between ecosystem functions and plant diversity, such as for carbon sequestration, have been well known in various ecosystem models, but the nature of the processes is complex (Sistla et al., 2016). There are limitations in the currently available AF models, especially for the true soil processes and the competition between trees and crops under AFS. The AF model in APSIM was used to simulate the soil carbon interactions for maize crops with gliricidia, under different management options, such as different proportions of trees and crops. The results were satisfactory, but the authors recommended further testing under a wide range of environmental conditions and management options (Smethurst et al., 2017).
5.4. Reducing GHG emissions
GHGs emissions are increasing, especially in intensive agricultural systems, and this leads to global warming and climate change (Xing et al., 2011; Moore et al., 2014). AF practices have the potential to reduce GHGs emissions by sequestering carbon into the soil and wood and by enhancing nutrient cycling, as tree roots better utilize nutrients in deeper layers (Williams et al., 2010; Burke and Poncé-Hernandez, 2014; Ajit et al., 2017; Khasanah et al., 2020). GHG emissions are directly related to the SOC levels in the soil for the biological, physical, and chemical processes, especially in tropical and temperate regions, and AFS regulates and normalizes the soil processes and reduces GHG emissions (Young et al., 1998; Parton et al., 2015). Several physical and process-based models (FullCAM, DayCent, DNDC, APSIM, WNMM, and AgMod) were developed and applied to estimate GHG emissions in cropping systems, but these models have uncertainties and limitations for carbon fluxes, carbon sequestration, and CH4 emissions at a large scale (Holzworth et al., 2014). While dynamic process-based biophysical and biogeochemical models have had limited development, but are applied in AFS at plot-to-field scales. While the currently available and used AF models (SCUAF, HyPAR, Hi-SAFE/Yield-SAFE, ICBM, WaNuLCAS, and APSIM next generation AF) have basic limitations and challenges for GHGs emission simulations, and so, are rarely used for this purpose (Parton et al., 2015; Luedeling et al., 2016).
While simple statistical and empirical-based models have been developed and used for GHG emission estimations, such as the Holos model which takes into account CO2, N2O, and CH4 emissions, as well as C sequestration from tree plantings and changes in land use and management (Williams et al., 2010). While dynamic process-based biophysical and biogeochemical models have had limited development and application in AFS at the field scale. AF models also have limited functions for the simulation of GHG emissions; for example, the Yield-SAFE model uses the LCA equation to derive carbon dioxide emission equivalents (CO2e) that are associated with geochemical processes, but does not consider N2O emissions and has not been extensively tested (García de Jalón et al., 2018b; Crous-Duran et al., 2019a). The CENTURY and RothC (Rothamsted) models were developed and applied to assess soil carbon, SOM, and GHG emissions, but they do not provide accurate predictions for the carbon cycle under tropical AFS (Bolinder et al., 2006; Ilstedt et al., 2007; Palma et al., 2007b). SCUAF also considers gas losses but has not been extensively tested in AFS for fertilizer management and GHG emissions (Connolly et al., 2002; Nabuurs et al., 2008). The ICBM model also has limitations in terms of the residue decay rate and C inputs for the reliable prediction of C stocks and the limited application of GHG emissions in AFS in contrasting environmental/weather conditions (Kätterer and Andrén, 2001; Andrén et al., 2004; Bolinder et al., 2006). Furthermore, advanced and developed models like APSIM-AF have not been tested in various types of AFS. Soil processes like immobilization and mineralization are determined based on the C: N ratio and the N2 to N2O emissions are computed by denitrification, but they require modification (APSIM-SoilN module) to accurately consider the competition, including SOC, water, and nutrients, between tree roots and crops (Young et al., 1998; DeAngelis and Grimm, 2014; Holzworth et al., 2014). Similarly, non-functional modules for WaNuLCAS are available for GHG emission estimations but need to be further developed and consider applications in contrasting environments (Van Noordwijk and Lusiana, 1998; Khasanah et al., 2020; Tenreiro et al., 2020). Efforts are required for the development of reliable and accurate modeling platforms that also have the potential to consider soil processes properly, especially those related to SOC and GHG emissions under AFS.
5.5. Increasing groundwater recharge
Groundwater recharge can be determined using a variety of factors such as geology, topography, vegetation cover, soil type, and management. This information is crucial for water availability estimations concerning the aquifer recharge in water-scarce regions. AF affects the infiltration capacity and groundwater recharge more than mono-cropping systems or grasslands (De Souza et al., 2019; Tenreiro et al., 2020). AFS can be effective for reducing and halting deeper drainage by enhancing the uptake of superficial water, even from water-logged zones through deeper roots. AF has positive environmental effects that reduce soil salinization but modeling of these aspects is currently limited. Modeling the below-ground competition for water is still a challenge under AFS for accurate estimation of water balance, infiltration, and drainage in deeper layers. Limited studies available where WaNuLCAS was used for soil water balance, drainage, and evapotranspiration simulation, results showed poor estimation for groundwater recharge, and improvements are required for better results to consider the competition between trees and crops (Boote, 2019; Onsamrarn et al., 2020). Although infiltration and water recharge capacity is being simulated but has limited tests (García de Jalón et al., 2018b; Onsamrarn et al., 2020).
HyPAR also has limitations for the dynamic simulation of basic processes related to water, infiltration, and groundwater recharge especially when considering the interactive competition for water between crops and trees (Mobbs et al., 1998, 2001; Luedeling et al., 2016). Previously, only a small number of studies reported on the simulation of groundwater recharge in AFS by applying the HyPAR model. Hydrological processes (infiltration, groundwater recharge, and runoff) in AF models are poorly estimated as the water module is partially working in SCUAF, which is based on soil carbon and defines the water holding capacity and runoff (Young et al., 1998; Lojka and Posthumus, 2006). Functions were developed for the Hi-SAFE and Yield-SAFE models to determine a soil water balance but they lack validation and broad-range spectrum testing under contrasting environments with different scenarios (Lecomte et al., 2016; Dupraz et al., 2019). APSIM with SWIM modules can simulate the hydrological properties like an infiltration, runoff, and water balance, and deep drainage under cropping systems (Zhang et al., 1999), but did not truly test for ground recharge and had limited capabilities for waterlogging (Zhang et al., 1999; Holzworth et al., 2018). Capillary rise function and infiltration effect estimations are important modeling approaches for the accurate estimation of water balance and drainage, especially in shallow water table regions, but both approaches are poorly considered in AF models and especially in the APSIM tree proxy model (Smethurst et al., 2017; Isaac and Borden, 2019).
All currently available AF models have inherent limitations in their structures as they have been developed for limited and specific ESS. Furthermore, the complexities of the tree–crop interactions, especially below-ground competition for resources such as water, are not yet fully understood. These limitations create difficulties for ESS simulations and provisions. The BALSEQ model (acronym of SEQuential BALance) has the potential to estimate groundwater recharge but depends on the differences between soil water content and the water stored in layers. However, this model has not yet been tested in AFS and has limitations, as it did not work properly when the water table or capillary effects were in contact with the tree roots, which affect evapotranspiration (Kay et al., 2018). Previously, the TOPOG_IRM model (Palma et al., 2016) was also tested for groundwater recharge but the vertical soil moisture dynamics that were assumed to happen in isothermal, non-hysteretic, and isotropic ways, and it also had limitations and was not tested in AFS. In short, all the models currently available face limitations and challenges when estimating groundwater recharge in AFS. Serious effort is required in the future to develop reliable modules for groundwater estimations in AFS.
5.6. Reduction of N leaching, pesticides, and herbicides
The estimation of N leaching is crucial to understand water quality, and as AF practices claim to reduce environmental impacts and groundwater pollution by reducing nutrient losses and herbicides and pesticides leaching into the groundwater (Suprayogo et al., 2002). Nitrate leaching in the deeper layers of the groundwater strongly depends on the soil water balance. Different types of simple to complex empirical, physical, and process-based models have recently been used for nutrient leaching estimations, but each has specific limitations. Generally, the available models consider the C:N ratio for modeling the mineralization and immobilization of nitrogen in a one-dimensional soil plant atmosphere continuum. Nitrogen modeling for all components of the system, including leaching, is more complex and especially in AFS, as some models focus on specific fluxes and ignore others (Young et al., 1998; Ilstedt et al., 2007; Holzworth et al., 2014). The modeling of each flux is important as these are interdependent, like the amount of N released from the residue decompositions under AFS and the way of modeling nitrogen losses and immobilization. Nutrient retention and leaching (N and P) can be assessed with the simple empirical model MODIFFUS in AF but the does not account for dynamic processes and interactive competition between the tree and crop (Van Noordwijk and Cadisch, 2002; Kay, 2018).
Yield-SAFE estimated that 28% of N leaching can be reduced in high-risk areas but this also depends on the tree density (Palma et al., 2007a), as N leaching is modeled using the equation proposed by Feldwisch, in which the soil water exchange factor and leaching are estimated from excess nitrogen. Yield-SAFE was found to predict lower N leaching levels based on the competition in the tree–crop interactions, using a model of the downstream approach that did not consider N dynamics, i.e., the relationship with yield, but further tests are required in different AF systems and under contrasting environments (Meier et al., 2020). Similarly, Hi-SAFE has the potential to simulate N leaching in AFS (Burke and Poncé-Hernandez, 2014; Cardinael et al., 2018). Horizontal soil water and nutrient fluxes are not considered in the model, which may limit its application for AFS on hillslopes (Brisson et al., 1998; Kay et al., 2019). WaNuLCAS has also been used for the prediction of mineral nitrogen leaching, the effect of nutrient limitation on tree and crop production under different combination patterns and ecosystems (Radersma et al., 2005; Walker et al., 2007; Shrestha and Datta, 2015; Pardon et al., 2017; Paul et al., 2017). Although it was previously used in cropping systems, there are challenges regarding the accurate simulation of the N process and leaching in AFS. Furthermore, the adsorption coefficient and retardation factors in the N-module for NO3 and NH4 to improve the N leaching, especially for hedgerow intercropping, require further refinement. Improving water retention will reduce N leaching, especially during higher rainfall intensities. Increased tree root length densities are required to improve predictions for leaching and complete N uptake (Van Noordwijk and Lusiana, 1998).
While APSIM simulates the N fluxes, it needs to maintain N concentrations related to the potential growth of organs to improve the N threshold (Holzworth et al., 2018). The harvested organs are removed from the system, but the residues with their specific C: N ratios typically remain in the field and the residues are mineralized depending on various factors in the field where immobilization is not accurately simulated and this leads to uncertainty in N leaching (Hagen, 2004; Khasanah and Mulia, 2012; Rival, 2018). Efforts are required to improve the capability and reliability of models for improved simulations of complex environmental issues, like nitrogen losses and leaching (Holzworth et al., 2018). Limited functions and capabilities are available in AFS models for the estimation of pesticide and herbicide leaching to groundwater, for example (Noordwijk et al., 2015), the leaching of metribuzin herbicide through the soil profile was estimated using the RZWQM and PESTFADE models under field conditions but the results were unsatisfactory. Currently, pesticide transport models are not well developed and are unavailable for AFS (Dupraz et al., 2018). The application of AFS models for N, pesticide, and herbicide leaching are limited due to the restricted capabilities for complex interactions, inadequate flexibility, data requirements, and parameterization challenges.
5.7. Biodiversity conservation and assessment in AFS
Assessing BD in AF is a complex process and developing a process-based dynamic model that has the potential to consider all processes contributing to BD is challenging. Previously, simple to complex process-based models was developed and used at a large scale for forestry and on a limited scale for agroforestry but they had limitations in their simulations of BD. Different submodules are currently being developed to address this, but they still require vigorous testing (Udawatta et al., 2019). Although several models have been developed that were shown to make reasonable estimations, there has been a limited focus on BD at the field scale and a lack of dynamic processed-based simulations for its aspects in AFS (Malézieux et al., 2009; Mokany and Ferrier, 2011; Smith et al., 2016; Araújo et al., 2019). Simple empirical to complex process-based BD models are also being used to assess BD and ESS in forestry and AF, such as agent-based vegetation models, DVM, DGVMs, SESAM, dynamic FOAM, forest gap models, mechanistic models, statistical models, and landscape indicators (Botkin et al., 2009; Malézieux et al., 2009; Guisan and Rahbek, 2011; Chopin et al., 2019). Prediction is good in some cases as there is a more robust simulation of ESS that is related to tree growth and dynamics have also been considered in recent modern gap models. Some AF models are appropriate, however, and have the potential to simulate the new management strategies for the enhancement of BD and ESS, including environmental and production benefits. APSIM and Hi-SAFE are the examples recently used for the provision of BD under AF but each model does have some limitations, while different submodules are being developed, they have not been tested recently (Udawatta et al., 2019). A substantial effort, including new modeling approaches, will be required in the future to develop a more focuses approach to this research and should consider integrations with dynamic processed-based models to improve the simulation of BD.
6. Key challenges for the dynamic simulation of process-based models in AFS
6.1. Complexity in practices, management, and resource competition
AFS is more complex than mono-cropping or intercropping as it deals with more interactions and ESS at the same time. There is complex competition at the spatial and temporal scale for interactions and nutrients, light, water and other resources between trees and crops in AFS (Cardinael et al., 2015; Khasanah et al., 2015; Noordwijk et al., 2015). Tree and crop species, however, have different needs and strategies when competing for resources at both the spatial and temporal scales. Furthermore, AF provides provisions for BD, making the system more complex and the required models are challenging to develop. Different approaches and strategies have been used to model ESS and the competition at different scales but comprehensive and detailed modeling formworks are still required to simulate these interactions accurately, even at the field scale (Palma et al., 2007a; Hussain et al., 2016; Luedeling et al., 2016; Smethurst et al., 2017).
6.2. Spatial and temporal domains and competition for water and nutrients under AF
Modeling the interactions in AF at both temporal and spatial scales is interesting, and this interaction leads to above and below-ground competition, which ultimately affects resource utilization efficiencies. Simple hypothetical horizontal and vertical zones drawing the spatial distributions of trees and crops in different proportions, which ultimately affect light, water, and other resource competition, are presented in Figure 3. It is a simple replica of reality and simple models should also follow this concept, and this is seen with WaNuLCAS. AF models should be capable of simulating all resources and competition with reliable predictions when considering both vertical and horizontal competition within different strata, as it is quite crucial for above and below-ground predictions (Artru et al., 2017; Palma et al., 2018). Flexible modeling approaches are required to consider the spatial arrangements of trees in AFS in combination with annual crops. Competition for resources like light, water, and nutrients requires more attention, as these are crucial, and there are complex interactions to consider, such as the shadow of a tree if it is taller than the crops. Trees are generally deeply rooted (>10 m) when compared with crops (<10 m) and there is more competition in the upper soil layer and comparison with the lower subsoil layer but modeling of these parameters requires further consideration as many developed AF models lack reliable simulations of these parameters/ESS (Van Noordwijk and Lusiana, 1998; Cardinael et al., 2018; Dupraz et al., 2019). Studies have also revealed that tree roots prefer the water from surface zones, especially when the fine roots of the tree compete for water and nutrients in the upper layer and interactions become more complex, but this is varied due to the influence of many factors, such as rainfall and nutrient availability in the soil, which are challenging to model in AFS (Walker et al., 2007; Hussain et al., 2016; Dupraz et al., 2019). The modeling of water competition in AF systems for spatial dimensions is also challenging, as many factors contribute and affect the life cycle, phenology, roots, and nature of plants (Mulia et al., 2010; Dupraz et al., 2018).
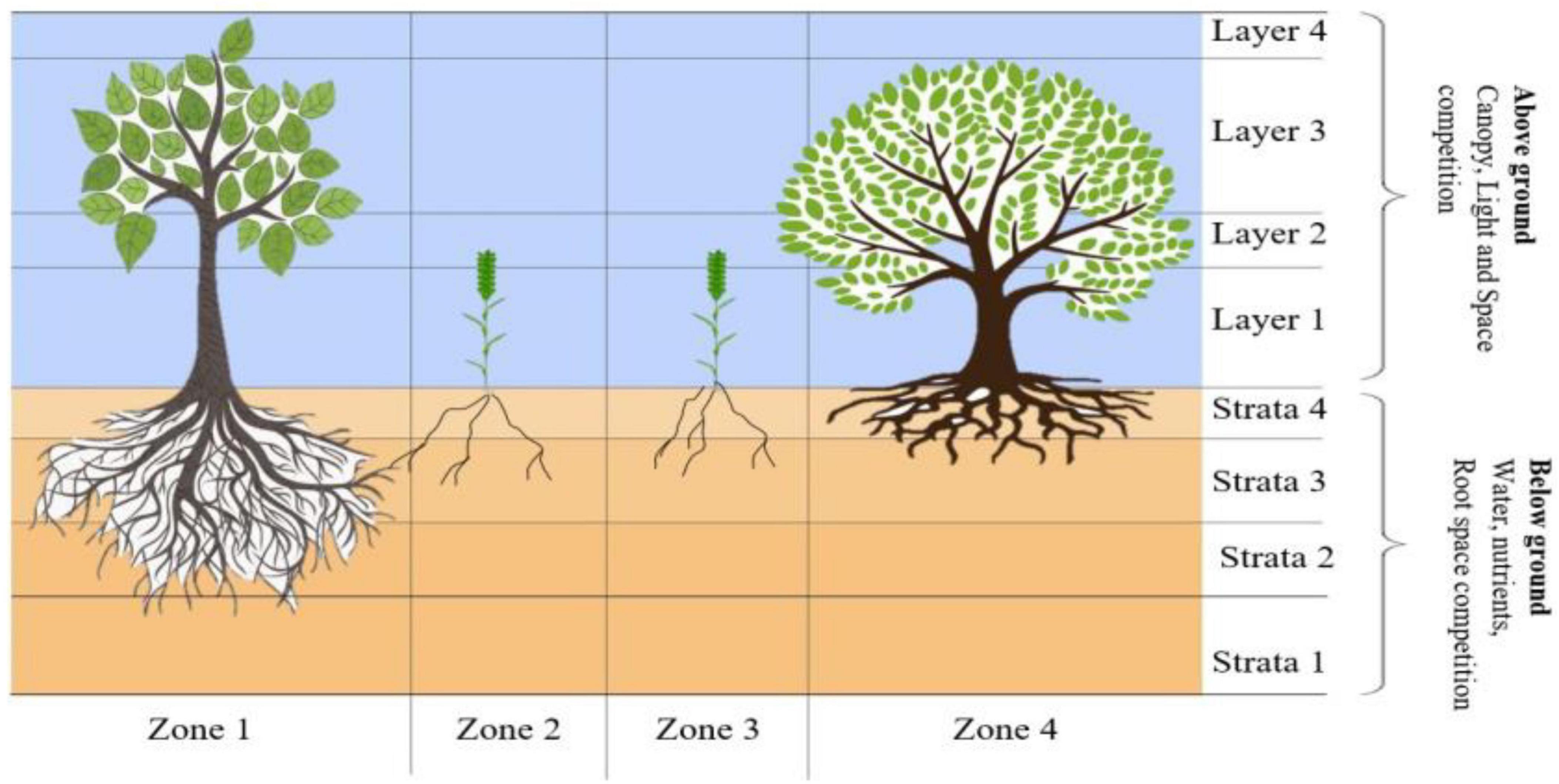
Figure 3. Sketch of the spatial hypothetical distribution of soil, water, and light in different zones, strata, and layers and the competition that exists between trees and crops in agroforestry systems and a simple and complex model of AF.
6.3. Light and radiation spatial and temporal distribution and competition under AF
Similarly, the competition for light is also a key challenge (Lecomte et al., 2016; Artru et al., 2017; Dupraz et al., 2019) when developing models for reliable results and decision management in AFS. Trees affect crops and soil by shading and reducing the amount of light that reaches the ground, but many factors affect the amount of radiation, like tree canopy, height, tree row direction, leading area, the spread of the tree canopy, and pruning management in different seasons (Wiles and Wilkerson, 1991; Friend et al., 1997; Parveaud et al., 2008; Cardinael et al., 2015). Assessing the qualitative effects of shading and the interactive effects within a microclimate and their impacts on crop growth is also quite a complex process. While negative and positive effects are present at the same time, for example, shading reduces the radiation and photosynthesis in crop plants while at the same time reducing the air temperature and increasing humidity in the trees which protects crop plants from hot climates, especially in arid and semi-arid environmental conditions. Previous studies found that trees significantly reduced sunlight, radiation, and light interception effects on crop growth and yield, especially cereals crops in the AFS. However, this depends on the nature of the tree species, canopy, and crop plants grown under the trees (Bazié et al., 2012; Artru et al., 2017).
Many tree models and AF models consider the effects of light and interception on crops (227), while recently researchers focused on more sophisticated models for the simulation of light. The light module is well developed in some AF models like Hi-SAFE, HyPAR, and WaNuLCAS and has the potential to simulate interactions and competition (Burgess and Rosati, 2018; Moreno et al., 2018; Plexida et al., 2018; Dupraz et al., 2019; Santos et al., 2019; Khasanah et al., 2020), while it is partially developed and the results are not as reliable in other models like SCUAF (Young et al., 1998; Luedeling et al., 2016). Light competition between trees and crops is simple, a proportion of solar radiation intercepted by the trees is only defined based on the LAI of a tree and the light extinction coefficient in Hi-SAFE (van der Werf et al., 2007). While subsequent work has revealed that it is essential to change the value of the light extinction coefficient with the development of the canopy (Keesman et al., 2011). The schematic diagram for light competition between trees and crops, and for a simple hypothetical model as above-ground part i.e., the canopy of tree and crop is divided into different vertical zones/layers to find the light competition as followed in WaNuLCAS model is presented in Figure 4, it may adopt to develop the simple to complex model for light completion to consider the tree and crop interaction for light and radiation.
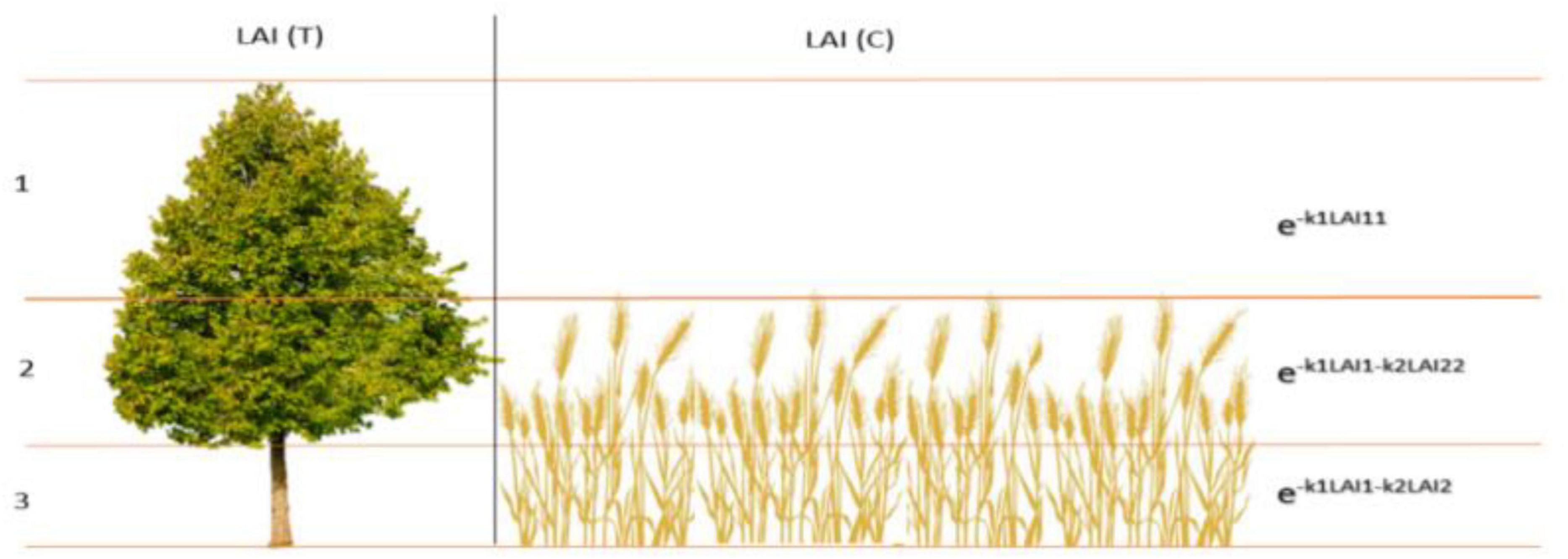
Figure 4. Schematic diagram of light competition between trees and crops for a simple hypothetical model including the above-ground part and canopy of the tree and the crop is divided into different vertical zones/layers to identify the light competition (a similar pattern is used in the WaNuLCAS model).
7. Ways forward and options for the integration of agroforestry, ESS modules, and biodiversity models in dynamic processed-based modeling frameworks for the simulation of ESS and biodiversity in AFS
7.1. Challenges for the coupling and integration of models
The results of this extensive review have revealed that models either belong to agroforestry, BD, or soil erosion categories and they each have constraints and inherent issues for dynamic process-based predictions of ESS and BD at a field scale. Future modeling approaches are required to focus on ESS simulations and decision-making, by developing dynamic process-based field scale models for AFS to achieve SDGs (Table 1). Modelers and scientists may consider different approaches like the coupling modeling approaches (low to high level), integrating models (soil erosion, BD, and AF) at the source code level, or adopting the modeling framework coupling approach where models are coupled using a coupler to produce dynamic simulations at the field scale for ESS and BD estimations (Siad et al., 2019). Furthermore, it has been proposed that predictions would be improved with a combination of different suitable modeling approaches and dynamic processed-based models or frameworks (crop modeling framework) to integrate/combine at a different level to get accurate and reliable estimates of ESS at the field scale for improved better decision support and sustainability of AFS. While the modeling integration approaches/techniques should also simulate the basic mechanisms and processes of different crop and tree management strategies for soil erosion, ESS, and BD in AFS, from the field to regional scales. Combining these modeling approaches or coupling them in a modeling framework to develop a more processed-based modeling platform that is also simple, flexible, and easily understandable for the stakeholders like researchers and decision-makers is a challenging process. There are many interactions and competition between trees and crops, as has been mentioned in the previous section, that is still challenging to consider and model.
7.2. A dynamic process-based crop modeling framework
Crop models and MFW have already been developed and recent advancements have been considered as dynamic simulations (a process-based mechanism) (Jones et al., 2017). Crop models are flexible as new crops can be added, and hence these models and frameworks can be used in combination with AF models without major modification. The required modeling approaches for ESS and BD can be taken from their respective models and coupled within this framework for the simulation of ESS in AFS at a field scale. But it is challenging, as crop models are only specific for growth simulations when considering temperature and solar radiation (Jones et al., 2003; Keating et al., 2003; Wajid et al., 2013; Awais et al., 2017; Rahman et al., 2018, 2019; Sezen et al., 2021). Still, many changes are also required for the above and below-ground competition between trees and crops, as conditions are different in AFS. The microclimate is different in the trees and competition for light, water, and nutrients become more complex. It is critical that all these processes are considered, and even challenged in process-based dynamic models, as major physiological processes will be affected due to the presence of trees when compared to mono-cropping (Lawson et al., 1995; Lott et al., 2009). However, substantial efforts and modeling research work is required, as recent efforts have been made and progress is ongoing, such as for the APSIM tree proxy model for AF (tree + crop models), but simulations for all process and ESS are still required (Smethurst et al., 2017, 2018; Holzworth et al., 2018). The microclimate, shading effects, and light competition are also critical as they can lead to temperature variations and divert physiological processes, even within a day at specific hours, and consequently, efforts are still required to test all these effects and validate the developing models.
There are many other crops MFW that are available and being used for integration and coupling at different scales to simulate the effects of a particular factor. The Hi-SAFE model was developed by integrating a tree and crop model and it has been applied and tested (Dupraz et al., 2019). Similarly, the other MFW, especially those that are simple, flexible, user friendly, and have features of quality and longevity, can also be used for integration models to develop more processed-based dynamic models by considering suitable AF models. While crop models simulate basic daily processes, the majority lack tree growth estimations, which are required to develop tree sub-models or import from developed tree models (Wise and Cacho, 2005; Parveaud et al., 2008; Holzworth et al., 2018). While there is also a need to make compatible crop growth models, although tree models are available, they do not operate on a daily time step like crop models, but the tree submodules available in WaNuLCAS, APSIM, and Hi-SAFE are the exceptions. Substantial efforts will be required to add the tree model to the crop growth model, especially for the process that is absent in annual crops and competitions like roots, water, and nutrients between the trees and crops, while phenology and physiological process are also different, as growth habits are different.
7.3. Options for coupling and integration under the modeling framework
There are different possible options to consider for the combination and coupling (integration) of models to develop reliable and accurate ESS simulations.
• Either use already available potential AF models (dynamic and process-based and consider all possible competition between the tree and crop) with proper modifications for the estimation of ESS and BD.
• AF models that lack accurate simulations for basic processes and competition should be developed using a new model with the source codes of a particular model in any other framework and then integrated into specific EES and BD modules.
• Possible options for coupling and integration are objective-specific and vary in different situations and circumstances.
• Ensuring accuracy and reliability depends on the testing of available models and coupling according to studied objectives. For example, the integration of soil erosion and BD models or any other submodule related to specific ESS for AFS with crop modeling framework.
• It is difficult to ensure accuracy and reliability when there are a large number of ESS simulations at the same time. There are two possible approaches, either better accuracy with a minimum number of variable simulations or vice-versa. Extensive knowledge and skills in modeling are required to adopt these scenarios of modeling integration and coupling.
Three different possible approaches are:
○ Sensitivity analysis, to test the nature of the relationship and significance of that particular factor/process in the model.
○ To draw the conceptual model for categorizing the priorities in processes and mechanisms (adopt, skip, or low preference) in the simulation process.
○ Multivariate analysis to understand the grading and variability among factors/processes.
To tackle this challenging situation, there may be different possible options adopted according to the objective and nature of the study. A MFW is one of the best options in this scenario to adopt, as it is flexible and can add and remove different models by coupling in a modular structure. While different submodules can also be developed and linked. A MFW thus has the potential to be developed and to test different submodules with reasonably good accuracy and reliability. There are several process-based dynamic crop models available that are widely used, and adding a tree model from any well-adopted AF model to one of these crop models, with proper modifications, is a strategy to develop a new model. Here different submodules for each tree, soil erosion, BD, and other ESS can be developed under the MFW and coupled for the simulation of a specific ecosystem service.
7.4. Features of a modeling framework for the integration of BD and AF models for the simulation of ESS in AFS
The results showed that major attempts to improve models, especially for AF, were not continuously focused on model improvement, which is the basic reason for limited use and applicability, and validation at a large scale. Previously AF models were outdated and lacked state-of-the-art developments for innovation and the requirements of the developing AFS. A good MFW should be simple, flexible, and have longevity, and should be updated when required. It should also effectively interact among different submodules and remain active in the modeling community to participate in different modeling comparison tests. As AF is a complex system when compared with other agricultural systems, its modeling is also quite complex. Basic knowledge and understanding of competition and processes is crucial and details about the processes and interaction should be provided for the users. Above and below-ground competition and interactions are quite complex and require an understanding of details such as water and nutrient competition processes and mechanisms to calibrate and parameterize the model as a black box, and these details will consequently affect model accuracy and results. Models should also have both simple and complex versions and different structure details for general users and developers. Furthermore, their codes should be easy to modify with other’s detailed knowledge, as this could otherwise limit their use and applicability.
7.5. Scientific impact assessment and modeling platform for advanced crop and ecosystem management (SIMPLACE) modeling framework
There are different MFW available, such as Scientific Impact assessment and Modeling Platform for Advanced Crop and Ecosystem Management (SIMPLACE), which is a crop modeling framework that is flexible in terms of customization. It can adopt standard procedures and protocols, and its multi-threaded high-performance architecture enables calibration and simulations at different spatial scales, with good accuracy and reliability (Enders et al., 2010). It also provides different ways to interact, depending on the need. This is like the SIMPLACE spatial decision support systems (SDSS) engine that can be used for basic understanding and general use, but researchers and scientists can also apply advanced levels of approach and model development, which can also be used and tested for coupling and integration. It has the potential to simulate more than twenty crops and further new crops can easily be adopted, while it is also flexible in its structure and can be coupled and integrated with different AF, soil erosion, and BD models with proper modifications. However, the development of a processed-based model to consider the tree and crop interactions and which has the potential to simulate all ESS mentioned in section “3 Existing models and modeling frameworks of AF and biodiversity for ESS simulation” is challenging.
In comparison with other MFW, coupling and integration offer improved and more flexible options. These frameworks have already been used for SDSS in different studies, and serve as a user interface on which to run hydrological (SWAT, UHP) and agro-ecosystem (EPIC, SAHYSMOD, CROPWAT) models and support land-use classification and modeling, which has shown its potential for coupling and integration. It has also recently been used in many global studies like the agricultural model inter-comparison and improvement project (AgMIP), and it has performed reasonably well when compared with other world-leading models and molding frameworks. Since most process-based crop simulation models have been optimized to simulate conventional cropping systems, the integration of AFS as well as the simulation of major ESS provided by such systems, such as erosion control, enhanced soil fertility, carbon sequestration, reduced fertilizer losses especially nitrogen leaching and wind and water regulations, is also challenging. The simulations of ESS is rarely available in current modeling systems, while the integration of ESS into crop system models is also a challenge, but as mentioned above, there are possible ways and opportunities for future models to integrate soil erosion, AF, and BD models into a crop MFW after the development of individual submodules for each component and couple.
Crop MFW like SIMPLACE (Enders et al., 2010) have the potential to simulate the dynamics of the interactions between critical components of agricultural systems (light, water, and nutrients) at the field scale. A potential possible option is to develop soil erosion sim-components and other ESS-related sim-components under the SIMPLACE modeling framework for AFS at the field level, which could then be upscaled to a larger area. There will be two- and three-dimensional interactions and competition above and below ground that needs to be considered, while trees can be added as crop types in the modeling framework. There will be many complex steps, especially in the development of below-ground sim-components, which must be considered when assessing the competition for water and nutrients between tree and crop roots at both temporal and spatial scales.
Although there may be many possible options that could be adopted for soil erosion modules like RUSLE and RUWLE, they require proper modifications to simulate soil erosion losses by water and wind, respectively. Simple physical process-based models for soil erosion models are available, such as WEPP, EPIC, GUEST, and ESEM. Each of these models has limitations but with proper modifications, like for the crop growth models EPIC and APSIM, the basic physical equating could also be adopted for the development of soil erosion submodules in the SIMPLACE platform. While output like runoff, sedimentation, N leaching, and soil loss can be used as inputs in the SIMPLACE framework. While further modifications will be required in other sim-components like water and nutrients to assess the competition of trees and crops by considering root architecture. Soil erosion sim-components will be linked with other components, but there will be further development and testing of the components required, especially for light. The current light sim- components in SIMPLACE are for intercropping during development and may be further modified by considering all possible competition sources for light between the tree and crop canopy. While there may be other options to add the light module that is already available in AF models (Wanulcas and Hi-safe) also requires modification in canopy parameters, like light use efficiency, and radiation interception, when considering AFS. Similarly, a submodule for BD should also be developed following previous models like the DVM, DGVMs, and SESAM and dynamic FOAM for the prediction and assessment of BD in AFS at the field scale. However, modeling these processes and the interactions of both above and below-ground competition is challenging for the reliable prediction of ESS in AFS at the field scale.
8. Conclusion and way forward
AF has the potential to increase and sustain production. Furthermore, it provides provisions for ESS and environmental sustainability to tackle climate change. Modeling AF by considering above and below-ground competition for the simulation of ESS and justifying sustainable development goals is challenging. Previously, efforts were made to model the ESS provided by AFS but the momentum was not maintained as AFS are much more complex than mono or intercropping systems. The current study provides a clear description of the models used for BD, soil erosion, and AF, to identify those that are suitable for dynamics-based simulations of ESS from a field to regional scale. This investigation focused on the simulation of 7 ESS which is produced by modern AFS, such as soil protection and conservation, water retention for flood prevention, carbon sequestration and soil biodiversity, reducing GHG emissions, increasing groundwater recharge, reductions in N leaching, and BD conservation. Simple (1D) to complex (3D) > 16 models related to AF were reviewed and the findings showed that none of them were capable of simulating dynamic ESS when considering the above and below-ground competition at a field-to-regional scale. Only a few AF models (Hi-SAFE and WaNuLCAS) were widely applied in the past and used for the general simulation of AFS, while others (SCUAF, HyPAR, and HyCAS) have rarely been tested or applied and have not been continuously updated and maintained. Although Hi-SAFE and WaNuLCAS have been widely used in the recent past and continuously updated, they did not satisfactorily simulate all the ESS and BD that are currently of interest in AFS. Recently, the APSIM tree proxy model has progressed in development with the combination of tree and crop modules but many aspects still need to be tested and validated in contrasting environments, while the majority of the ESS has not been considered for dynamic simulations.
Similarly, many of the most suitable and relevant empirical and physical process-based soil erosion models are related to forestry and AFS were considered in their development. Most of the models have inherent limitations, as they were designed for specific objectives under certain conditions. Furthermore, only a few were continuously updated and applied at small and field levels, like RUSLE, RUWLE, WEPP, GUEST, ESEM, and EPIC but these recently updated models were limited when applied to AFS at the field scale for the assessment of soil loss and runoff, especially about the effects of hedgerows for soil protection and conservation. These models are still applied in a variety of conditions at both small and large scales and can be used with proper modifications for coupling and integration with different models and frameworks for the estimation of soil protection and conservation. Similarly, current BD models are being used for the assessment of BD but are limited and rarely applied for BD provisions in modern AFS. A few mechanistic and process-based models (DVM, SESAM, and dynamic FOAM) can be used with proper modifications under any modeling framework for the prediction and assessment of BD under AFS at the field scale. The findings revealed that models for AF, BD, or soil erosion were developed for a specific purpose and tested under specific conditions, but have had limited development in terms of their ESS provisions in the current AFS and have limits in terms of their predictions for tree and crop interaction which is also a major limitation in AF models.
Furthermore, research and development are required for dynamic process-based models for ESS and BD assessments at the field scale for the accurate and reliable prediction of tree and crop interactions in AFS. Future modeling work should be used to develop a platform for process-based dynamic simulations for more desirable ESS with reliable estimates. There are a few promising modules available in each group that can be coupled and integrated under a well-developed modeling framework. To meet the objectives a flexible, easy-to-understand, and longevity-based modular modeling strategy is required, which should accommodate different combinations of models to achieve innovative system configurations and simulate key processes and interactions for certain requirement conditions. The crop modeling framework SIMPLACE has been found to have the potential in simulating the dynamics of the interactions between critical components (light, water, and nutrients) at the field scale. The suitable modeling approaches that are available could be integrated and coupled by extending the framework to incorporate, AF, soil erosion, BD, and other related ESS sub-modules to model the basic processes and interactions on a dynamic basis in AFS at a field-to-regional scale. Integrating all of these approaches in a crop modeling framework for dynamic simulations of AFS is quite complex, and it is simultaneously challenging to have many modules interacting for each component. This can be achieved by following a simple strategy in the beginning and then moving toward complexity so that processes and accuracy can be tested at the individual module level to build the capacity for AF modeling. These pathways are quite important for modelers and are widely used for the decision management and simulation of ESS in modern AFS. Developed modeling approaches/frameworks should be able to simulate a wide range of complex spatial and temporal management practices under contrasting environmental conditions to compete for both national and global levels of decision support and management of the AFS for the provision of ESS and moves toward sustainability.
Author contributions
MHR, TG, and HEA: conceptualization and methodology. MHR, TG, HEA, and AR: resource, data curation, and writing—review and editing. MHR: writing—original draft preparation. TG: project administration. TG and HEA: funding acquisition. All authors have read and agreed to the published version of the manuscript.
Funding
This research was funded by the German Federal Ministry of Education and Research (BMBF) through the Digital Agriculture Knowledge and Information System (DAKIS) Project (grant no. 031B0729E) and by the Deutsche Forschungsgemeinschaft (DFG, German Research Foundation) under Germany’s Excellence Strategy-EXC 2070-390732324 (PhenoRob). While APC was funded by the Crop Science Group, University of Bonn, Germany and University of Helsinki, Department of Agricultural Sciences, Finland.
Conflict of interest
The authors declare that the research was conducted in the absence of any commercial or financial relationships that could be construed as a potential conflict of interest.
Publisher’s note
All claims expressed in this article are solely those of the authors and do not necessarily represent those of their affiliated organizations, or those of the publisher, the editors and the reviewers. Any product that may be evaluated in this article, or claim that may be made by its manufacturer, is not guaranteed or endorsed by the publisher.
References
Abbas, F., Hammad, H., Ishaq, W., Farooque, A., Bakhat, H., Zia, Z., et al. (2020). A review of soil carbon dynamics resulting from agricultural practices. J. Environ. Manage. 268:110319. doi: 10.1016/j.jenvman.2020.110319
Ajit, Dhyani, S., Handa, A., Newaj, R., Chavan, S. B., Alam, B., et al. (2017). Estimating carbon sequestration potential of existing agroforestry systems in India. Agroforestry Syst. 91, 1101–1118. doi: 10.1007/s10457-016-9986-z
Ajit, Dhyani, S., Ramnewaj, Handa, A. K., Prasad, R., Alam, B., et al. (2013). Modeling analysis of potential carbon sequestration under existing agroforestry systems in three districts of Indo-gangetic plains in India. Agroforestry Syst. 87, 1129–1146. doi: 10.1007/s10457-013-9625-x
Alewell, C., Borrelli, P., Meusburger, K., and Panagos, P. (2019). Using the USLE: Chances, challenges and limitations of soil erosion modelling. Int. Soil Water Conserv. Res. 7, 203–225. doi: 10.1016/j.iswcr.2019.05.004
Amadi, C., Van Rees, K., and Farrell, R. (2016). Greenhouse gas mitigation potential of shelterbelts: Estimating farm-scale emission reductions using the holos model. Can. J. Soil Sci. 97, 353–367. doi: 10.1139/cjss-2016-0017
Andrén, O., Kätterer, T., and Karlsson, T. (2004). ICBM regional model for estimations of dynamics of agricultural soil carbon pools. Nutr. Cycl. Agroecosyst. 70, 231–239.
Andrew, E. E., Pascal, T., Beatrice, A., and Eneke, B. (2012). Simulation of the impacts of three management regimes on carbon sinks in rubber and oil palm plantation ecosystems of South- Western Cameroon. J. Ecol. Nat. Environ. 4, 154–162. doi: 10.5897/jene11.146
Araújo, M., Anderson, R., Barbosa, A., Beale, C., Dormann, C., Early, R., et al. (2019). Standards for distribution models in biodiversity assessments. Sci. Adv. 5:eaat4858. doi: 10.1126/sciadv.aat4858
Arroyo-Rodrí,guez, V., Moreno, C., and Galán-Acedo, C. (2017). Applied hierarchical modeling in ecology: Analysis of distribution, abundance and species richness in R and BUGS. Rev. Mex. Biodivers.
Artru, S., Garré, S., Dupraz, C., Hiel, M.-P., Blitz-Frayret, C., Lassois, L., et al. (2017). Impact of spatio-temporal shade dynamics on wheat growth and yield, perspectives for temperate agroforestry. Eur. J. Agron. 82, 60–70. doi: 10.1016/j.eja.2016.10.004
Avwunudiogba, A., and Hudson, P. (2014). A review of soil erosion models with special reference to the needs of humid tropical mountainous environments. Eur. J. Sustain. Dev. 3, 299–310. doi: 10.14207/ejsd.2014.v3n4p299
Awais, M., Wajid, A., Nasim, W., Ahmad, A., Saleem, M. F., Raza, M. A. S., et al. (2017). Modeling the water and nitrogen productivity of sunflower using OILCROP-SUN model in Pakistan. Field Crop Res. 205, 67–77. doi: 10.1016/j.fcr.2017.01.013
Bagarello, V., Ferro, V., and Flanagan, D. (2018). Predicting plot soil loss by empirical and process-oriented approaches. A review. J. Agric. Eng. 49, 1–18. doi: 10.4081/jae.2018.710
Bakker, M., Govers, G., Jones, R., and Rounsevell, M. (2007). The effect of soil erosion on Europe’s crop yields. Ecosystems 10, 1209–1219. doi: 10.1007/s10021-007-9090-3
Balandier, P., Bergez, J., and Etienne, M. (2003). Use of the management-oriented silvopastoral model ALWAYS: Calibration and evaluation. Agroforestry Syst. 57, 159–171. doi: 10.1023/A:1024863408559
Baumann, D., Bastiaans, L., Goudriaan, J., van Laar, H. H., and Kropfl, M. J. (2002). Analysing crop yield and plant quality in an intercropping system using an eco-physiological model for interplant competition. Agric. Syst. 73, 173–203. doi: 10.1016/S0308-521X(01)00084-1
Bayala, J., Sanou, J., Teklehaimanot, Z., Kalinganire, A., and Ouédraogo, S. J. (2014). Parklands for buffering climate risk and sustaining agricultural production in the Sahel of West Africa. Curr. Opin. Environ. Sustain. 6, 28–34. doi: 10.1016/j.cosust.2013.10.004
Bayala, J., Sanou, J., Teklehaimanot, Z., Ouedraogo, S. J., Kalinganire, A., Coe, R., et al. (2015). Advances in knowledge of processes in soil-tree-crop interactions in parkland systems in the West African Sahel: A review. Agric. Ecosyst. Environ. 205, 25–35. doi: 10.1016/j.agee.2015.02.018
Bazié, H., Bayala, J., Zombré, G., Sanou, J., and Ilstedt, U. (2012). Separating competition-related factors limiting crop performance in an agroforestry parkland system in Burkina Faso. Agroforestry Syst. 84, 377–388. doi: 10.1007/s10457-012-9483-y
Bergez, J., Etienne, M., and Balandier, P. (1999). ALWAYS: A plot-based silvopastoral system model. Ecol. Model. 115, 1–17. doi: 10.1016/S0304-3800(98)00153-7
Bhuyan, S., Kalita, P., Janssen, K., and Barnes, P. (2002). Soil loss predictions with three erosion simulation models. Environ. Model. Softw. 17, 135–144. doi: 10.1016/s1364-8152(01)00046-9
Blanco-Canqui, H., and Lal, R. (2010). Principles of soil conservation and management. Berlin: Springer.
Bolinder, M., Vandenbygaart, A., Gregorich, E., Angers, D. A., Janzen, H. H., et al. (2006). Modelling soil organic carbon stock change for estimating whole-farm greenhouse gas emissions. Can. J. Soil Sci. 86, 419–429.
Boote, K. (2019). Advances in crop modelling for a sustainable agriculture. Cambridge: Burleigh Dodds Science Publishing. doi: 10.1201/9780429266591
Botkin, D., Janak, J., and Wallis, J. (2009). Some ecological consequences of a computer model of forest growth. J. Ecol. 60, 849–872.
Brisson, N., Gary, C., Justes, E., Roche, R., Mary, B., Ripoche, D., et al. (2003). An overview of the crop model STICS. Eur. J. Agron. 18, 309–332.
Brisson, N., Mary, B., Ripoche, D., Hélène Jeuffroy, M., Ruget, F., Nicoullaud, B., et al. (1998). STICS: A generic model for the simulation of crops and their water and nitrogen balances. I. Theory and parameterization applied to wheat and corn. Agronomie 18, 311–346. doi: 10.1051/agro:19980501
Burgess, P., Graves, A., de Jalón, S., Palma, J., Dupraz, C., and van Noordwijk, M. (2019). “Modelling agroforestry systems,” in Agroforestry for sustainable agriculture, eds M. R. Mosquera-Losada and R. Prabhu Burleigh (Cambridge: Burleigh Dodds Science Publishing Limited), 209–238.
Burgess, P., and Rosati, A. (2018). Advances in European agroforestry: Results from the AGFORWARD project. Agroforestry Syst. 92, 801–810. doi: 10.1007/s10457-018-0261-3
Burke, S., and Poncé-Hernandez, R. (2014). Connecting the water and carbon cycles for the generation of food security and ecosystem services. Future Food 2, 8–20.
Cardinael, R., Chevallier, T., Barthès, B., Saby, N. P. A., Parent, T., Dupraz, C., et al. (2015). Impact of alley cropping agroforestry on stocks, forms and spatial distribution of soil organic carbon - A case study in a Mediterranean context. Geoderma 259-260, 288–299. doi: 10.1016/j.geoderma.2015.06.015
Cardinael, R., Guenet, B., Chevallier, T., Dupraz, C., Cozzi, T., Chenu, C., et al. (2018). High organic inputs explain shallow and deep SOC storage in a long-term agroforestry system - Combining experimental and modeling approaches. Biogeosciences 15, 297–317. doi: 10.5194/bg-15-297-2018
Chandramohan, T., Venkatesh, B., and Balchand, A. (2015). Evaluation of three soil erosion models for small watersheds. Aquat. Procedia 4, 1227–1234. doi: 10.1016/j.aqpro.2015.02.156
Charbonnier, F., le Maire, G., Dreyer, E., Casanoves, F., Christina, M., Dauzat, J., et al. (2013). Competition for light in heterogeneous canopies: Application of MAESTRA to a coffee (Coffea arabica L.) agroforestry system. Agric. For. Meteorol. 181, 152–169. doi: 10.1016/j.agrformet.2013.07.010
Chopin, P., Bergkvist, G., and Hossard, L. (2019). Modelling biodiversity change in agricultural landscape scenarios - A review and prospects for future research. Biol. Conserv. 235, 1–17.
Coe, R., Sinclair, F., and Barrios, E. (2014). Scaling up agroforestry requires research “in” rather than “for” development. Curr. Opin. Environ. Sustain. 6, 73–77. doi: 10.1016/j.cosust.2013.10.013
Connolly, R., Bell, M., Huth, N., Freebairn, D. M., and Thomas, G. (2002). Simulating infiltration and the water balance in cropping systems with APSIM-SWIM. Aust. J. Soil Res. 40, 221–242. doi: 10.1071/SR01007
Coulibaly, Y., Mulia, R., Sanou, J., Zombré, G., Bayala, J., Kalinganire, A., et al. (2014). Crop production under different rainfall and management conditions in agroforestry parkland systems in Burkina Faso: Observations and simulation with WaNuLCAS model. Agroforestry Syst. 88, 13–28. doi: 10.1007/s10457-013-9651-8
Crous-Duran, J., Graves, A., Garcia-De-Jalón, S., Paulo, J. A., Tomé, M., and Palma, J. H. N. (2019a). Assessing food sustainable intensification potential of agroforestry using a carbon balance method. IForest 12, 85–91. doi: 10.3832/ifor2578-011
Crous-Duran, J., Graves, A., Paulo, J., Mirck, J., Oliveira, T. S., Kay, S., et al. (2019b). Modelling tree density effects on provisioning ecosystem services in Europe. Agroforestry Syst. 93, 1985–2007. doi: 10.1007/s10457-018-0297-4
Das, R., and Bauer, S. (2012). Bio-economic analysis of soil conservation technologies in the mid-hill region of Nepal. Soil Tillage Res. 121, 38–48. doi: 10.1016/j.still.2012.01.016
De Mello, C., Norton, L., Pinto, L., Beskow, S., and Curi, N. (2016). Agricultural watershed modeling: A review for hydrology and soil erosion processes. Cien. Agrotecnol. 40, 7–25. doi: 10.1590/S1413-70542016000100001
De Souza, E., Pontes, L., Fernandes Filho, E., Gonçalves Reynaud Schaefer, C. E., and dos Santos, E. E. (2019). Spatial and temporal potential groundwater recharge: The case of the doce river basin, Brazil. Rev. Bras. Cien. Solo 43, 1–27. doi: 10.1590/18069657rbcs20180010
DeAngelis, D., and Grimm, V. (2014). Individual-based models in ecology after four decades. F1000Prime Rep. 6:39. doi: 10.12703/P6-39
Dilla, A., Smethurst, P., Barry, K., Parsons, D., and Denboba, M. A. (2019). Tree pruning, zone and fertiliser interactions determine maize productivity in the Faidherbia albida (Delile) A. Chev parkland agroforestry system of Ethiopia. Agroforestry Syst. 93, 1897–1907. doi: 10.1007/s10457-018-0304-9
Dilla, A., Smethurst, P., Barry, K., Parsons, D., and Denboba, M. (2018). Potential of the APSIM model to simulate impacts of shading on maize productivity. Agroforestry Syst. 92, 1699–1709. doi: 10.1007/s10457-017-0119-0
Dufour, L., Metay, A., Talbot, G., and Dupraz, C. (2013). Assessing light competition for cereal production in temperate agroforestry systems using experimentation and crop modelling. J. Agron. Crop Sci. 199, 217–227. doi: 10.1111/jac.12008
Dupraz, C. (2002). Tree-crops interaction models State of the Art Report Silvoarable Agroforestry For Europe (SAFE) Tree-crops interaction models Captions for the cover pictures. Available online at: https://www1.montpellier.inra.fr/safe/english/results/annual_report_3/SAFE%20third%20Year%20Annual%20Report%20Volume%202.pdf (accessed September 21, 2021).
Dupraz, C., Blitz-Frayret, C., Lecomte, I., Molto, Q., Reyes, F., and Gosme, M. (2018). Influence of latitude on the light availability for intercrops in an agroforestry alley-cropping system. Agroforestry Syst. 92, 1019–1033. doi: 10.1007/s10457-018-0214-x
Dupraz, C., Burgess, P., and Graves, A. (2005). Synthesis of the silvoarable agroforestry for Europe project. Montpellier: INRA.
Dupraz, C., Wolz, K., Lecomte, I., Talbot, G., Vincent, G., Mulia, R., et al. (2019). Hi-sAFe: A 3D agroforestry model for integrating dynamic tree-crop interactions. Sustainability 11:2293. doi: 10.3390/su11082293
Ellis, E., and Schoeneberger, M. (2004). Computer-based tools for decision support in agroforestry: Current state and future needs. Agroforestry Syst. 61-62, 401–421. doi: 10.1023/B:AGFO.0000029015.64463.65
Enders, A., Diekkrüger, B., Laudien, R., Gaiser, T., and Bareth, G. (2010). “The IMPETUS spatial decision support systems,” in Impacts of global change on the hydrological cycle in west and northwest africa, eds P. Speth, M. Christoph, and B. Diekkrüger (Berlin; Heidelberg: Springer), 360–393.
Evans, R., and Boardman, J. (2016). The new assessment of soil loss by water erosion in Europe. Panagos P. et al, 2015 Environmental Science & Policy 54, 438-447-A response. Environ. Sci. Policy 58, 11–15. doi: 10.1016/j.envsci.2015.12.013
Francaviglia, R., Coleman, K., Whitmore, A., Doro, L., Urracci, G., Rubino, M., et al. (2012). Changes in soil organic carbon and climate change - Application of the RothC model in agro-silvo-pastoral Mediterranean systems. Agric. Syst. 112, 48–54. doi: 10.1016/j.agsy.2012.07.001
Friend, A., Stevens, A., Knox, R., and Cannell, M. (1997). A process-based, terrestrial biosphere model of ecosystem dynamics (Hybrid v3.0). Ecol. Model. 95, 249–287. doi: 10.1016/S0304-3800(96)00034-8
Fryrcar, D., Chen, W., and Lester, C. (2001). Revised wind erosion equation. Ann. Arid Zone 40, 265–279.
García de Jalón, S., Graves, A., Palma, J., Williams, A., Upson, M., Burgess, P. J., et al. (2018b). Modelling and valuing the environmental impacts of arable, forestry and agroforestry systems: A case study. Agroforestry Syst. 92, 1059–1073. doi: 10.1007/s10457-017-0128-z
García de Jalón, S., Graves, A., Moreno, G., Palma, J. H. N., Crous-Durán, J., Kay, S., et al. (2018a). Forage-SAFE: A model for assessing the impact of tree cover on wood pasture profitability. Ecol. Model. 372, 24–32. doi: 10.1016/j.ecolmodel.2018.01.017
Gidey, T., Oliveira, T., Crous-Duran, J., and Palma, J. (2020). Using the yield-SAFE model to assess the impacts of climate change on yield of coffee (Coffea arabica L.) under agroforestry and monoculture systems. Agroforestry Syst. 94, 57–70. doi: 10.1007/s10457-019-00369-5
Gou, F., van Ittersum, M., Simon, E., Leffelaar, P. A., Putten, P. V., Zhang, L., et al. (2017). Intercropping wheat and maize increases total radiation interception and wheat RUE but lowers maize RUE. Eur. J. Agron. 84, 125–139. doi: 10.1016/j.eja.2016.10.014
Graves, A., Burgess, P., Liagre, F., Terreaux, J.-P., Borrel, T., Dupraz, C., et al. (2011). Farm-SAFE: The process of developing a plot- and farm-scale model of arable, forestry, and silvoarable economics. Agroforestry Syst. 81, 93–108. doi: 10.1007/s10457-010-9363-2
Graves, A., Burgess, P., Palma, J., Herzog, F., Moreno, G., Bertomeu, M., et al. (2007). Development and application of bio-economic modelling to compare silvoarable, arable, and forestry systems in three European countries. Ecol. Eng. 29, 434–449. doi: 10.1016/j.ecoleng.2006.09.018
Graves, A., Burgess, P., Palma, J., Keesman, K. J., van der Werf, W., Dupraz, C., et al. (2010). Implementation and calibration of the parameter-sparse Yield-SAFE model to predict production and land equivalent ratio in mixed tree and crop systems under two contrasting production situations in Europe. Ecol. Model. 221, 1744–1756. doi: 10.1016/j.ecolmodel.2010.03.008
Grimm, V., Ayllón, D., and Railsback, S. (2017). Next-generation individual-based models integrate biodiversity and ecosystems: Yes we can, and yes we must. Ecosystems 20, 229–236. doi: 10.1007/s10021-016-0071-2
Guillem, E., Murray-Rust, D., Robinson, D., Barnes, A., and Rounsevell, M. (2015). Modelling farmer decision-making to anticipate tradeoffs between provisioning ecosystem services and biodiversity. Agric. Syst. 137, 12–23. doi: 10.1016/j.agsy.2015.03.006
Guisan, A., and Rahbek, C. (2011). SESAM - a new framework integrating macroecological and species distribution models for predicting spatio-temporal patterns of species assemblages. J. Biogeogr. 38, 1433–1444. doi: 10.1111/j.1365-2699.2011.025
Hagen, L. (2004). Evaluation of the Wind Erosion Prediction System (WEPS) erosion submodel on cropland fields. Environ. Model. Softw. 19, 171–176. doi: 10.1016/S1364-8152(03)00119-1
Hagen, L., Schroeder, P., and Thai, L. (2009). Estimated particulate emissions by wind erosion from the Indiana Harbor confined disposal facility. Pract. Period. Hazard. Toxic Radioact. Waste Manag. 13:1. doi: 10.1061/(ASCE)1090-025X2009
Hajigholizadeh, M., Melesse, A., and Fuentes, H. (2018). Erosion and sediment transport modelling in shallowwaters: A review on approaches, models and applications. Int. J. Environ. Res. Public Health 15:518. doi: 10.3390/ijerph15030518
Heinlein, F., Goebel, L., and Priesack, E. (2008). “Development of Agro-Forestry Models to assess productivity and environmental protection capacity,” in Proceedings of the 20th conference EGU General Assembly, EGU2018, Vienna.
Holzworth, D., Huth, N., deVoil, P., Zurcher, E. J., Herrmann, N. I., McLean, G., et al. (2014). APSIM - Evolution towards a new generation of agricultural systems simulation. Environ. Model. Softw. 62, 327–350. doi: 10.1016/j.envsoft.2014.07.009
Holzworth, D., Huth, N., Fainges, J., Brown, H. E., Zurcher, E. J., Cichota, R., et al. (2018). APSIM Next Generation: Overcoming challenges in modernising a farming systems model. Environ. Model. Softw. 103, 43–51. doi: 10.1016/j.envsoft.2018.02.002
Holzworth, D., Huth, N., Fainges, J., Hermann, N. I., Zurcher, E., Brown, H., et al. (2015). “APSIM next generation: The final frontier?,” in Proceedings of the 21st International congress on modelling and simulation, MODSIM 2015, Canberra.
Hussain, K., Wongleecharoen, C., Hilger, T., Ahmad, A., Kongkaew, T., Cadisch, G., et al. (2016). Modelling resource competition and its mitigation at the crop-soil-hedge interface using WaNuLCAS. Agroforestry Syst. 90, 1025–1044. doi: 10.1007/s10457-015-9881-z
Huth, N., Banabas, M., Nelson, P., and Webb, M. (2014). Development of an oil palm cropping systems model: Lessons learned and future directions. Environ. Model. Softw. 62, 411–419. doi: 10.1016/j.envsoft.2014.06.021
Huth, N., Carberry, P., Poulton, P., Brennan, L. E., and Keating, B. A. (2002). A framework for simulating agroforestry options for the low rainfall areas of Australia using APSIM. Eur. J. Agron. 18, 171–185. doi: 10.1016/S1161-0301(02)00103-X
Huth, N., Robertson, M., and Poulton, P. (2010). Regional differences in tree-crop competition due to soil, climate and management. Crop Pasture Sci. 61, 763–770. doi: 10.1071/CP09254
Igwe, P. U., Onuigbo, A. A., Chinedu, O. C., Ezeaku, I. I., Muoneke, M. M., et al. (2017). Soil erosion: A review of models and applications. Int. J. Adv. Eng. Res. Sci. 4, 138–150. doi: 10.22161/ijaers.4.12.22
Ilstedt, U., Malmer, A., Verbeeten, E., and Murdiyarso, D. (2007). The effect of afforestation on water infiltration in the tropics: A systematic review and meta-analysis. For. Ecol. Manage. 251, 45–51. doi: 10.1016/j.foreco.2007.06.014
Isaac, M., and Borden, K. (2019). Nutrient acquisition strategies in agroforestry systems. Plant Soil 444, 1–19.
Jackson, B., Pagella, T., Sinclair, F., Orellana, B., Henshaw, A. J., Reynolds, B., et al. (2013). Polyscape: A GIS mapping framework providing efficient and spatially explicit landscape-scale valuation of multiple ecosystem services. Landsc. Urban Plan. 112, 74–88. doi: 10.1016/j.landurbplan.2012.12.014
Jiang, C., Zhang, H., Zhang, Z., and Wang, D. (2019). Model-based assessment soil loss by wind and water erosion in China’s Loess Plateau: Dynamic change, conservation effectiveness, and strategies for sustainable restoration. Glob. Planetary Change 172, 396–413. doi: 10.1016/j.gloplacha.2018.11.002
Jones, J. W., Antle, J. M., Basso, B., Boote, K. J., Conant, R. T., Foster, I., et al. (2017). Brief history of agricultural systems modeling. Agric. Syst. 155, 240–254. doi: 10.1016/j.agsy.2016.05.014
Jones, J., Hoogenboom, G., Porter, C., Boote, K. J., Batchelor, W. D., Hunt, L. A., et al. (2003). The DSSAT cropping system model. Eur. J. Agron. 18, 235–265.
Jose, S. (2009). Agroforestry for ecosystem services and environmental benefits: An overview. Agroforestry Syst. 76, 1–10.
Jose, S. (2012). Agroforestry for conserving and enhancing biodiversity. Agroforestry Syst. 85, 1–8. doi: 10.1007/s10457-012-9517-5
Jose, S., Gillespie, A., and Pallardy, S. (2004). Interspecific interactions in temperate agroforestry. Agroforestry Syst. 61, 237–255.
Kätterer, T., and Andrén, O. (2001). The ICBM family of analytically solved models of soil carbon, nitrogen and microbial biomass dynamics - Descriptions and application examples. Ecol. Model. 136, 191–207. doi: 10.1016/S0304-3800(00)00420-8
Kay, S. (2018). Assessment of Ecosystem Services provided by Agroforestry Systems at the Landscape Scale. Ph.D. dissertation. Zürich: Universität Zürich.
Kay, S., Crous-Duran, J., Ferreiro-Domínguez, N., Moreno, M. R., García de Jalón, S., Graves, A., et al. (2018). Spatial similarities between European agroforestry systems and ecosystem services at the landscape scale. Agroforestry Syst. 92, 1075–1089. doi: 10.1007/s10457-017-0132-3
Kay, S., Rega, C., Moreno, G., den Herder, M., and Palma, J. (2019). Agroforestry creates carbon sinks whilst enhancing the environment in agricultural landscapes in Europe. Land Use Policy 83, 581–593. doi: 10.1016/j.landusepol.2019.02.025
Keating, B., and Carberry, P. (1993). Resource capture and use in intercropping: Solar radiation. Field Crops Res. 34, 273–301. doi: 10.3389/fpls.2022.848893
Keating, B., Carberry, P., Hammer, G., Probert, M. E., Robertson, M. J., Holzworth, D., et al. (2003). An overview of APSIM, a model designed for farming systems simulation. Eur. J. Agron. 18, 267–288.
Keesman, K., Graves, A., van der Werf, W., Burgess, P., Palma, J., Dupraz, C., et al. (2011). A system identification approach for developing and parameterising an agroforestry system model under constrained availability of data. Environ. Model. Softw. 26, 1540–1553. doi: 10.1016/j.envsoft.2011.07.020
Khaine, I., and Woo, S. (2018). Exploration of the aboveground carbon sequestration and the growth estimation models of four species in agroforestry system of semi-arid region, Myanmar. Agroforestry Syst. 92, 183–194. doi: 10.1007/s10457-016-0024-y
Khasanah, N., and Mulia, R. (2012). Exploration of tree management options to manipulate tree and crop interaction trade-off. Available online at: http://apps.worldagroforestry.org/downloads/WaNuLCAS/Example%20of%20Model%20Application.pdf (accessed September 21, 2021).
Khasanah, N., Perdana, A., Rahmanullah, A., Manurung, G., Roshetko, J. M., and van Noordwijk, M. (2015). Intercropping teak (Tectona grandis) and maize (Zea mays): Bioeconomic trade-off analysis of agroforestry management practices in Gunungkidul, West Java. Agroforestry Syst. 89, 1019–1033. doi: 10.1007/s10457-015-9832-8
Khasanah, N., van Noordwijk, M., Slingerland, M., Sofiyudin, M., Stomph, D., Migeon, A., et al. (2020). Oil palm agroforestry can achieve economic and environmental gains as indicated by multifunctional land equivalent ratios. Front. Sustain. Food Syst. 3:122. doi: 10.3389/fsufs.2019.00122
Kirby, K., and Potvin, C. (2007). Variation in carbon storage among tree species: Implications for the management of a small-scale carbon sink project. For. Ecol. Manage. 246, 208–221. doi: 10.1016/j.foreco.2007.03.072
Laflen, J., and Flanagan, D. (2013). The development of U. S. soil erosion prediction and modeling. Int. Soil Water Conserv. Res. 1, 1–11. doi: 10.1016/S2095-6339(15)30034-4
Lawson, G. (1999). HyPAR model for agroforestry systems technical manual. Available online at: https://agris.fao.org/agris-search/search.do?recordID=GB2012107731 (accessed September 21, 2021).
Lawson, G., Crout, N., Levy, P., Mobbs, D., Wallace, J., Cannell, M., et al. (1995). The tree-crop interface: Representation by coupling of forest and crop process-models. Agroforestry Syst. 30, 199–221. doi: 10.1007/BF00708921
Lecomte, I., Dupraz, C., and Gosme, M. (2016). Improvement of the Hi-sAFe Model. Available online at: https://www.agforward.eu/documents/MS30_Hi-sAFe_Improvements.pdf (accessed September 21, 2021).
Li, J., Okin, G., Tatarko, J., Webb, N., and Herrick, J. (2014). Consistency of wind erosion assessments across land use and land cover types: A critical analysis. Aeolian Res. 15, 253–260. doi: 10.1016/j.aeolia.2014.04.007
Lippe, M., Thai Minh, T., Neef, A., Hilger, T., Hoffmann, V., Lam, N., et al. (2011). Building on qualitative datasets and participatory processes to simulate land use change in a mountain watershed of Northwest Vietnam. Environ. Model. Softw. 26, 1454–1466. doi: 10.1016/j.envsoft.2011.07.009
Lojka, B., and Posthumus, H. (2006). Prediction of soil loss and crop yields with different management scenarios in barrier system using the SCUAF model in semi-arid Burkina Faso. Agric. Trop. Subtrop. 39, 158–164.
López-Serrano, F., Martínez-García, E., Dadi, T., RubioF, E., García-MoroteM, A., Lucas-Borja, E., et al. (2015). Biomass growth simulations in a natural mixed forest stand under different thinning intensities by 3-PG process-based model. Eur. J. For. Res. 134, 167–185. doi: 10.1007/s10342-014-0841-3
Lott, J., Ong, C., and Black, C. (2009). Understorey microclimate and crop performance in a Grevillea robusta-based agroforestry system in semi-arid Kenya. Agric. For. Meteorol. 149, 1140–1151. doi: 10.1016/j.agrformet.2009.02.002
Luedeling, E., Kindt, R., Huth, N., and Koenig, K. (2014). Agroforestry systems in a changing climate-challenges in projecting future performance. Curr. Opin. Environ. Sustain. 6, 1–7.
Luedeling, E., Smethurst, P., Baudron, F., Bayala, J., Huth, N. I., and van Noordwijk, M. (2016). Field-scale modeling of tree-crop interactions: Challenges and development needs. Agric. Syst. 142, 51–69.
Magcale-macandog, D. (2002). Soil erosion and sustainability of different land uses of smallholder Imperata Grasslands in Sea. Available online at: https://www.tucson.ars.ag.gov/isco/isco12/VolumeII/SoilErosionandSustainability.pdf (accessed September 21, 2021).
Maharjan, G., Prescher, A., Nendel, C., Ewert, F., Mboh, C., and Gaiser, T. (2018). Approaches to model the impact of tillage implements on soil physical and nutrient properties in different agro-ecosystem models. Soil Tillage Res. 180, 210–221.
Mäkipää, R., Liski, J., Guendehou, S., Malimbwi, R., and Kaaya, A. (2012). Soil carbon monitoring using surveys and modelling: General description and application in the United Republic of Tanzania. Rome: Food and Agriculture Organization of The United Nations.
Malézieux, E., Crozat, Y., Dupraz, C., Laurans, M., Makowski, D., Ozier-Lafontaine, H., et al. (2009). Mixing plant species in cropping systems: Concepts, tools and models. A review. Agron. Sustain. Dev. 29, 43–62.
Masera, O., Garza-Caligaris, J., Kanninen, M., Karjalainen, T., Liski, J., Nabuurs, G. J., et al. (2003). Modeling carbon sequestration in afforestation, agroforestry and forest management projects: The CO2FIX V.2 approach. Ecol. Model. 164, 177–199. doi: 10.1016/S0304-3800(02)00419-2
Mayus, M., Van Keulen, H., and Stroosnijder, L. (1998). A model of tree-crop competition for windbreak systems in the Sahel: Description and evaluation. Agroforestry Syst. 43, 183–201. doi: 10.1023/a:1026444414803
Mead, R., and Willey, R. (1980). The concept of a ‘land equivalent ratio’ and advantages in yields from intercropping. Exp. Agric. 16, 217–228. doi: 10.1017/S0014479700010978
Meier, E., Thorburn, P., Bell, L., Harrison, M., and Biggs, J. (2020). Greenhouse gas emissions from cropping and grazed pastures are similar: A simulation analysis in Australia. Front. Sustain. Food Syst. 3, 1–18. doi: 10.3389/fsufs.2019.00121
Meyer, C. R., Wagner, L. E., Yoder, D. C., and Flanagan, D. C. (2001). The Modular Soil Erosion System (MOSES). St. Joseph, MI: American Society of Agricultural and Biological Engineers. doi: 10.13031/2013.3280
Mobbs, D., Cannell, M., Crout, N., Lawson, G. J., and Friend, A. D. (1998). Complementarity of light and water use in tropical agroforests I. Theoretical model outline, performance and sensitivity. For. Ecol. Manage. 102, 259–274. doi: 10.1016/S0378-1127(97)00167-9
Mobbs, D., Lawson, G., and Brown, T. (2001). Model for agroforestry systems user guide. Wallingford: Centre for Ecology and Hydrology.
Mokany, K., and Ferrier, S. (2011). Predicting impacts of climate change on biodiversity: A role for semi-mechanistic community-level modelling. Divers. Distrib. 17, 374–380. doi: 10.1111/j.1472-4642.2010.00735.x
Moore, A., Eckard, R., Thorburn, P., Grace, P., Wang, E., and Chen, D. (2014). Mathematical modeling for improved greenhouse gas balances, agro-ecosystems, and policy development: Lessons from the Australian experience. Wiley Interdiscip. Rev. Clim. Change 5, 735–752. doi: 10.1002/wcc.304
Moreno, G., Aviron, S., Berg, S., Crous-Duran, J., Franca, A., and de Jalón, S. G. (2018). Agroforestry systems of high nature and cultural value in Europe: Provision of commercial goods and other ecosystem services. Agroforestry Syst. 92, 877–891. doi: 10.1007/s10457-017-0126-1
Morgan, R., Quinton, J., Smith, R., Govers, G., Poesen, J. W. A., and Auerswald, K. (1998). The European soil erosion model (EUROSEM): A dynamic approach for predicting sediment transport from fields and small catchments. Earth Surf. Process. Landforms 23, 527–544.
Mulia, R., Dupraz, C., and van Noordwijk, M. (2010). Reconciling root plasticity and architectural ground rules in tree root growth models with voxel automata. Plant Soil 337, 77–92. doi: 10.1007/s11104-010-0502-3
Mulia, R., Widayati, A., Suyanto, Agung, P., and Zulkarnain, M. (2014). Low carbon emission development strategies for Jambi, Indonesia: Simulation and trade-off analysis using the FALLOW model. Mitig. Adapt. Strateg. Glob. Chang. 19, 773–788. doi: 10.1007/s11027-013-9485-8
Muthuri, C., Ong, C., Black, C., Mati, B. M., Ngumi, V. W., and van Noordwijk, M. (2004). Modelling the effects of leafing phenology on growth and water use by selected agroforestry tree species in semi-arid Kenya. Land Use Water Resour. Res. 4, 1–11.
Nabuurs, G., and Schelhaas, M. (2002). Carbon profiles of typical forest types across Europe assessed with CO2FIX. Ecol. Indic. 1, 213–223. doi: 10.1016/s1470-160x(02)00007-9
Nabuurs, G., van Putten, B., Knippers, T., and Mohren, G. (2008). Comparison of uncertainties in carbon sequestration estimates for a tropical and a temperate forest. For. Ecol. Manage. 256, 237–245. doi: 10.1016/j.foreco.2008.04.010
Negash, M., and Kanninen, M. (2015). Modeling biomass and soil carbon sequestration of indigenous agroforestry systems using CO2FIX approach. Agric. Ecosyst. Environ. 203, 147–155. doi: 10.1016/j.agee.2015.02.004
Nelson, R., Grist, R., Menz, K., Cramb, R., Paningbatan, E., and Mamicpic, M. (1997). A cost-benefit analysis of hedgerow intercropping in the Philippine uplands using the SCUAF model. Agroforestry Syst. 35, 203–220.
Nerger, R., Funk, R., Cordsen, E., and Fohrer, N. (2017). Application of a modeling approach to designate soil and soil organic carbon loss to wind erosion on long-term monitoring sites (BDF) in Northern Germany. Aeolian Res. 25, 135–147. doi: 10.1016/j.aeolia.2017.03.006
Nguyen, Q., Hoang, M., Öborn, I., and van Noordwijk, M. (2013). Multipurpose agroforestry as a climate change resiliency option for farmers: An example of local adaptation in Vietnam. Clim. Change 117, 241–257. doi: 10.1007/s10584-012-0550-1
Noordwijk, M., van, Lawson, G., Hairiah, K., and Wilson, J. (2015). “Root distribution of trees and crops: Competition and/or complementarity,” in Tree-crop interactions: Agroforestry in a changing climate, eds C. K Ong, C. R Black, and J Wilson (Wallingford: CAB International), 221–257. doi: 10.1079/9781780645117.0221
Onsamrarn, W., Chittamart, N., and Tawornpruek, S. (2020). Performances of the WEPP and WaNuLCAS models on soil erosion simulation in a tropical hillslope, Thailand. PLoS One 15:e0241689. doi: 10.1371/journal.pone.0241689
Ovalle-Rivera, O., Van Oijen, M., Läderach, P., Ovalle-Rivera, O., Van Oijen, M., Laderach, P., et al. (2020). Assessing the accuracy and robustness of a process-based model for coffee agroforestry systems in Central America. Agroforestry Syst. 94, 2033–2051. doi: 10.1007/s10457-020-00521-6
Palm, C., Blanco-Canqui, H., DeClerck, F., Gatere, L., and Grace, P. (2014). Conservation agriculture and ecosystem services: An overview. Agric. Ecosyst. Environ. 187, 87–105. doi: 10.1016/j.agee.2013.10.010
Palma, J., Crous-Duran, J., Graves, A., Garcia de Jalon, S., Upson, M., Oliveira, T. S., et al. (2018). Integrating belowground carbon dynamics into Yield-SAFE, a parameter sparse agroforestry model. Agroforestry Syst. 92, 1047–1057. doi: 10.1007/s10457-017-0123-4
Palma, J., Graves, A., Bunce, R., Burgess, P. J., de Filippi, R., Keesman, K. J., et al. (2007a). Modeling environmental benefits of silvoarable agroforestry in Europe. Agric. Ecosyst. Environ. 119, 320–334. doi: 10.1016/j.agee.2006.07.021
Palma, J., Graves, A., Burgess, P., Keesman, K., van Keulen, H., Mayus, M., et al. (2007b). Methodological approach for the assessment of environmental effects of agroforestry at the landscape scale. Ecol. Eng. 29, 450–462. doi: 10.1016/j.ecoleng.2006.09.016
Palma, J., Graves, A., Crous-Duran, J., Upson, M., Paulo, J. A., Oliveira, T. S., et al. (2016). Yield-SAFE model improvements. Available online at: https://www.agforward.eu/documents/MS29_Yield-SAFE_Improvements.pdf (accessed September 21, 2021).
Panagos, P., Borrelli, P., Poesen, J., Ballabioa, C., Lugatoa, E., Meusburger, K., et al. (2015b). The new assessment of soil loss by water erosion in Europe. Environ. Sci. Policy 54, 438–447. doi: 10.1016/j.envsci.2015.08.012
Panagos, P., Borrelli, P., Meusburger, K., Alewell, C., Lugato, E., and Montanarella, L. (2015a). Estimating the soil erosion cover-management factor at the European scale. Land Use Policy 48, 38–50. doi: 10.1016/j.landusepol.2015.05.021
Panagos, P., Standardi, G., Borrelli, P., Lugato, E., Montanarella, L., and Bosello, F. (2018). Cost of agricultural productivity loss due to soil erosion in the European Union: From direct cost evaluation approaches to the use of macroeconomic models. Land Degrad. Dev. 29, 471–484. doi: 10.1002/ldr.2879
Pansak, W., Hilger, T., Lusiana, B., Kongkaew, T., Marohn, C., Cadisch, G., et al. (2010). Assessing soil conservation strategies for upland cropping in Northeast Thailand with the WaNuLCAS model. Agroforestry Syst. 79, 123–144. doi: 10.1007/s10457-010-9290-2
Panwar, P., Chauhan, S., Kaushal, R., Das, D., Ajit, Arora, G., et al. (2017). Carbon sequestration potential of poplar-based agroforestry using the CO2FIX model in the Indo-Gangetic Region of India. Trop. Ecol. 58, 1–9.
Pardon, L., Ian Huth, N., Netelenbos Nelson, P., Banabas, M., Gabrielle, B., Bessou, C., et al. (2017). Yield and nitrogen losses in oil palm plantations: Main drivers and management trade-offs determined using simulation. Field Crop Res. 210, 20–32. doi: 10.1016/j.fcr.2017.05.016
Parton, W., Ojima, D., Cole, C., and Schimel, D. (2015). “A general model for soil organic matter dynamics: Sensitivity to litter chemistry, texture and management,” in Proceedings of the Quantitative modeling of soil forming processes, Minneapolis, MN, 147–167.
Parveaud, C., Chopard, J., Dauzat, J., Courbaud, B., and Auclair, D. (2008). Modelling foliage characteristics in 3D tree crowns: Influence on light interception and leaf irradiance. Trees 22, 87–104. doi: 10.1007/s00468-007-0172-9
Paul, C., Weber, M., and Knoke, T. (2017). Agroforestry versus farm mosaic systems – Comparing land-use efficiency, economic returns and risks under climate change effects. Sci. Total Environ. 587-588, 22–35. doi: 10.1016/j.scitotenv.2017.02.037
Pi, H., Sharratt, B., Feng, G., and Lei, J. (2017). Evaluation of two empirical wind erosion models in arid and semi-arid regions of China and the USA. Environ. Model. Softw. 91, 28–46. doi: 10.1016/j.envsoft.2017.01.013
Pinto, L., Bernardes, M., Van Noordwijk, M., Pereirad, A. R., Lusianac, B., Muliac, R., et al. (2005). Simulation of agroforestry systems with sugarcane in Piracicaba, Brazil. Agric. Syst. 86, 275–292. doi: 10.1016/j.agsy.2004.09.009
Pinto, V., van Dam, J., de Jong van Lier, Q., and Reichardt, K. (2019). Intercropping simulation using the SWAP model: Development of a 2x1D algorithm. Agriculture 9:126. doi: 10.3390/agriculture9060126
Plexida, S., Solomou, A., Poirazidis, K., and Sfougaris, A. (2018). Factors affecting biodiversity in agrosylvopastoral ecosystems with in the Mediterranean Basin: A systematic review. J. Arid Environ. 151, 125–133. doi: 10.1016/j.jaridenv.2017.11.017
Powlson, D., Gregory, P., Whalley, W., Quinton, J. N., Hopkins, D. W., Whitmore, A. P., et al. (2011). Soil management in relation to sustainable agriculture and ecosystem services. Food Policy 36, (Suppl. 1), 72. doi: 10.1016/j.foodpol.2010.11.025
Radersma, S., Lusiana, B., and Van Noordwijk, M. (2005). Simulation of soil drying induced phosphorus deficiency and phosphorus mobilization as determinants of maize growth near tree lines on a Ferralsol. Field Crop Res. 91, 171–184. doi: 10.1016/j.fcr.2004.06.005
Rahman, M., Ahmad, A., Wajid, A., Hussain, M., Rasul, F., Ishaque, W., et al. (2019). Application of CSM-CROPGRO-Cotton model for cultivars and optimum planting dates: Evaluation in changing semi-arid climate. Field Crop Res. 238, 139–152. doi: 10.1016/j.fcr.2017.07.007
Rahman, M., Ahmad, A., Wang, X., Wajid, A., Nasim, W., Hussain, M., et al. (2018). Multi-model projections of future climate and climate change impacts uncertainty assessment for cotton production in Pakistan. Agric. For. Meteorol. 253-254, 94–113. doi: 10.1016/j.agrformet.2018.02.008
Rahn, E., Vaast, P., Läderach, P., Van Asten, P. J. A., Jassogne, L., and Ghazoul, J. (2018). Exploring adaptation strategies of coffee production to climate change using a process-based model. Ecol. Modell. 371, 76–89. doi: 10.1016/j.ecolmodel.2018.01.009
Raza, A., Ahrends, H., Habib-Ur-rahman, M., and Gaiser, T. (2021). Modeling approaches to assess soil erosion by water at the field scale with special emphasis on heterogeneity of soils and crops. Land 10:422. doi: 10.3390/land10040422
Reed, M., Hubacek, K., Bonn, A., Burt, T. P., Holden, J., Stringer, L. C., et al. (2013). Anticipating and managing future trade-offs and complementarities between ecosystem services. Ecol. Soc. 18:5. doi: 10.5751/ES-04924-180105
Rival, A. (2018). Achieving sustainable cultivation of oil palm volume 1: Introduction, breeding and cultivation techniques. Cambridge: Burleigh Dodds Science Publishing. doi: 10.4324/9781351114387
Rizvi, R., Newaj, R., Prasad, R., Handa, A. K., Alam, B., Chavan, S., et al. (2016). Assessment of carbon storage potential and area under agroforestry systems in Gujarat Plains by CO2FIX model and remote sensing techniques. Curr. Sci. 110, 41–55. doi: 10.18520/cs/v110/i10/2005-2011
Rodrigo-Comino, J., Senciales-González, J., and Ruiz-Sinoga, J. (2020). The effect of hydrology on soil erosion. Water 12, 10–13. doi: 10.3390/w12030839
Sagastuy, M., and Krause, T. (2019). Agroforestry as a biodiversity conservation tool in the atlantic forest? Motivations and limitations for small-scale farmers to implement agroforestry systems in North-Eastern Brazil. Sustainability 11, 1–24. doi: 10.3390/su11246932
Salazar, O., Casanova, M., and Kätterer, T. (2011). The impact of agroforestry combined with water harvesting on soil carbon and nitrogen stocks in central Chile evaluated using the ICBM/N model. Agric. Ecosyst. Environ. 140, 123–136. doi: 10.1016/j.agee.2010.11.019
Santos, P., Crouzeilles, R., and Sansevero, J. (2019). Can agroforestry systems enhance biodiversity and ecosystem service provision in agricultural landscapes? A meta-analysis for the Brazilian Atlantic Forest. For. Ecol. Manage. 433, 140–145. doi: 10.1016/j.foreco.2018.10.064
Sezen, S., Ahmad, I., Habib-ur-Rahman, M., Amiri, E., Tekin, S., Oz, K., et al. (2021). Growth and productivity assessments of peanut under different irrigation water management practices using CSM-CROPGRO-Peanut model in Eastern Mediterranean of Turkey. Environ. Sci. Pollut. Res. 29, 26936–26949. doi: 10.1007/s11356-021-17722-w
Shrestha, S., and Datta, A. (2015). Field measurements for evaluating the RZWQM and PESTFADE models for the tropical zone of Thailand. J. Environ. Manage. 147, 286–296. doi: 10.1016/j.jenvman.2014.09.017
Siad, S., Iacobellis, V., Zdruli, P., Gioia, A., Stavi, I., and Hoogenboom, G. (2019). A review of coupled hydrologic and crop growth models. Agric. Water Manag. 224:105746. doi: 10.1098/rstb.2005.1739
Sistla, S. A., Roddy, A. B., Williams, N. E., Kramer, D. B., Stevens, K., and Allison, S. D. (2016). Agroforestry practices promote biodiversity and natural resource diversity in atlantic Nicaragua. PLoS One 11: e0162529. doi: 10.1371/journal.pone.0162529
Smethurst, P., Huth, N., and Dilla, A. (2018). Plot-Scale biophysical modelling of tree-crop interactions using APSIM. Montpellier: World Congress on Agroforestry.
Smethurst, P., Huth, N., Masikati, P., Sileshi, G. W., Akinnifesi, F. K., Wilson, J., et al. (2017). Accurate crop yield predictions from modelling tree-crop interactions in gliricidia-maize agroforestry. Agric Syst 155, 70–77. doi: 10.1016/j.agsy.2017.04.008
Smith, C., MacDonald, B., Xing, H., Denmead, O. T., Wang, E., McLachlan, G., et al. (2019). Measurements and APSIM modelling of soil C and N dynamics. Soil Res. 58, 41–61. doi: 10.1071/SR19021
Smith, J., Evans, J., Martin, B., Baruch-Mordo, S., Kiesecker, J., and Naugle, D. (2016). Reducing cultivation risk for at-risk species: Predicting outcomes of conservation easements for sage-grouse. Biol. Conserv. 201, 10–19. doi: 10.1016/j.biocon.2016.06.006
Stephens, W., and Hess, T. (1999). Modelling the benefits of soil water conservation using the PARCH model - A case study from a semi-arid region of Kenya. J. Arid Environ. 41, 335–344. doi: 10.1006/jare.1998.0486
Stolpe, N. (2005). A comparison of the RUSLE, EPIC and WEPP erosion models as calibrated to climate and soil of south-central Chile. Acta Agric. Scand. Sect. B Soil Plant Sci. 55, 2–8. doi: 10.1080/09064710510008568
Suprayogo, D., Van Noordwijk, M., Hairiah, K., and Cadisch, G. (2002). The inherent “safety-net” of an Acrisol: Measuring and modelling retarded leaching of mineral nitrogen. Eur. J. Soil Sci. 53, 185–194. doi: 10.1046/j.1365-2389.2002.00447.x
Systems, A., and Parametrisation, T. (2004). 6 Modelling growth and carbon sequestration of agroforestry systems in Leyte. Agroforestry Syst. 159–188.
Talbot, G., and Dupraz, C. (2012). Simple models for light competition within agroforestry discontinuous tree stands: Are leaf clumpiness and light interception by woody parts relevant factors? Agroforestry Syst. 84, 101–116. doi: 10.1007/s10457-011-9418-z
Talbot, G., Roux, S., Graves, A., Dupraz, C., and Marrou, H. (2014). Relative yield decomposition: A method for understanding the behaviour of complex crop models. Environ. Model. Softw. 51, 136–148. doi: 10.1016/j.envsoft.2013.09.017
Tenreiro, T., García-Vila, M., Gómez, J., Jimenez-Berni, J., and Fereres, E. (2020). Water modelling approaches and opportunities to simulate spatial water variations at crop field level. Agric. Water Manag. 240:106254. doi: 10.1016/j.agwat.2020.106254
Tsubo, M., Walker, S., and Ogindo, H. (2005). A simulation model of cereal-legume intercropping systems for semi-arid regions: I. Model development. Field Crop Res. 93, 10–22. doi: 10.1016/j.fcr.2004.09.002
Udawatta, R., Rankoth, L., and Jose, S. (2019). Agroforestry and biodiversity. Sustainability 11:2879. doi: 10.3390/su11102879
van der Werf, W., Keesman, K., Burgess, P., Graves, A. R., Pilbeam, D. J., Incoll, L. D., et al. (2007). Yield-SAFE: A parameter-sparse, process-based dynamic model for predicting resource capture, growth, and production in agroforestry systems. Ecol. Eng. 29, 419–433. doi: 10.1016/j.ecoleng.2006.09.017
Van Noordwijk, M., and Cadisch, G. (2002). Access and excess problems in plant nutrition. Plant Soil 247, 25–40. doi: 10.1023/A:1021194628358
Van Noordwijk, M., and Lusiana, B. (1998). WaNulCAS, a model of water, nutrient and light capture in agroforestry systems. Agroforestry Syst. 43, 217–242.
Van Noordwijk, M., and Lusiana, B. (1999). “WaNuLCAS, a model of water, nutrient and light capture in agroforestry systems,” in Agroforestry for sustainable land-use fundamental research and modelling with emphasis on temperate and mediterranean applications. forestry sciences, Vol. 60, eds D. Auclair and C. Dupraz (Dordrecht: Springer).
van Noordwijk, M., Suyamto, D., Lusiana, B., Ekadinata, A., and Hairiah, K. (2008). Facilitating agroforestation of landscapes for sustainable benefits: Tradeoffs between carbon stocks and local development benefits in Indonesia according to the FALLOW model. Agric. Ecosyst. Environ. 126, 98–112. doi: 10.1016/j.agee.2008.01.016
van Oijen, M., Barcza, Z., Confalonieri, R., Korhonen, P., Kröel-Dulay, G., Lellei-Kovács, E., et al. (2020). Incorporating biodiversity into biogeochemistry models to improve prediction of ecosystem services in temperate grasslands: Review and roadmap. Agronomy 10:259.
van Oijen, M., Dauzat, J., Harmand, J., Lawson, G., and Vaast, P. (2010a). Coffee agroforestry systems in Central America: I. A review of quantitative information on physiological and ecological processes. Agroforestry Syst. 80, 341–359. doi: 10.1007/s10457-010-9294-y
van Oijen, M., Dauzat, J., Harmand, J.-M., Lawson, G., and Vaast, P. (2010b). Coffee agroforestry systems in Central America: II. Development of a simple process-based model and preliminary results. Agroforestry Syst. 80, 361–378. doi: 10.1007/s10457-010-9291-1
Vermeulen, S., Woomer, P., Campbell, B., Kamukondiwa, W., Swift, M. J., Frost, P. G. H., et al. (1993). Use of the SCUAF model to simulate natural miombo woodland and maize monoculture ecosystems in Zimbabwe. Agroforestry Syst. 22, 259–271.
Wajid, A., Rahman, M., Ahmad, A., Khaliq, T., Mahmood, N., Rasul, F., et al. (2013). Simulating the Interactive Impact of Nitrogen and Promising Cultivars on Yield of Lentil (Lens culinaris) Using CROPGRO-legume Model. Int. J. Agric. Biol. 15, 1331–1336.
Walker, A., Mutuo, P., Van Noordwijk, M., Albrecht, A., and Cadisch, G. (2007). Modelling of planted legume fallows in Western Kenya using WaNuLCAS. (I) Model calibration and validation. Agroforestry Syst. 70, 197–209. doi: 10.1007/s10457-007-9049-6
Wang, B., Zheng, F., and Guan, Y. (2016). Improved USLE-K factor prediction: A case study on water erosion areas in China. Int. Soil Water Conserv. Res. 4, 168–176. doi: 10.1016/j.iswcr.2016.08.003
Wiles, L., and Wilkerson, G. (1991). Modeling competition for light between soybean and broadleaf weeds. Agric. Syst. 35, 37–51.
Williams, A., Audsley, E., and Sandars, D. (2010). Environmental burdens of producing bread wheat, oilseed rape and potatoes in England and Wales using simulation and system modelling. Int. J. Life Cycle Assess. 15, 855–868. doi: 10.1007/s11367-010-0212-3
Williams, J., Dyke, P., and Jones, C. (1983). Epic - a model for assessing the effects of erosion on soil productivity. Dev. Environ. Model. 5, 553–572.
Williams, J., Jones, C., Kiniry, J., and Spanel, D. (1989). EPIC crop growth model. Trans. Am. Soc. Agric. Eng. 32, 497–511. doi: 10.13031/2013.31032
Williams, J., and Renard, K. (2015). “Assessments of soil erosion and crop productivity with process models (EPIC),” in Soil erosion and crop productivity, eds R F. Follett and B.A. Stewart (Madison, WI: American Society of Agronomy), 67–103. doi: 10.2134/1985.soilerosionandcrop.c5
Williams, J., Zaurralde, R. C., and Steglich, E. M. (2012). Agricultural policy/environmental eXtender Model: Theoretical Documentation Version 0806. Temple, TX: Texas A&M AgriLife Blackland Research & Extension Center.
Wischmeier, H., Dwight, D., and Smith, D. (1978). Predicting rainfall erosion losses: A Guide to Conservation Planning - Walter H. Wischmeier, Dwight David Smith - Google Libros. Hyattsville, MD: USDA.
Wischmeier, W. H., and Smith, D. (1978). Predicting rainfall erosion lossesa guide to conservation planning with the universal soil loss equation (USLE). Agriculture Handbook No. 537. Springfield, IL: US Department of Agriculture.
Wise, R., and Cacho, O. (2005). Tree-crop interactions and their environmental and economic implications in the presence of carbon-sequestration payments. Environ. Model. Softw. 20, 1139–1148. doi: 10.1016/j.envsoft.2004.08.001
Wise, R., Cacho, O., and Hean, R. (2007). Fertilizer effects on the sustainability and profitability of agroforestry in the presence of carbon payments. Environ. Model. Softw. 22, 1372-1381. doi: 10.1016/j.envsoft.2006.10.002
Wö,sten, J., Lilly, A., Nemes, A., and Le Bas, C. (1998). Using existing soil data to derive hydraulica parameters for simulation models in environmental studies and in land use planning. Final report on the European Union funded project, 1998. (Report; No. 156). SC-DLO. Wageningen: Winand Staring Centre for integrated Land, soil, and water Research.
Xing, H., Wang, E., Smith, C., Rolston, D. E., and Yu, Q. (2011). Modelling nitrous oxide and carbon dioxide emission from soil in an incubation experiment. Geoderma 167-168, 328–339. doi: 10.1016/j.geoderma.2011.07.003
Young, A., Menz, K., Muraya, P., and Smith, C. (1998). SCUAF Version 4: A model to estimate soil changes under agriculture, agroforestry and forestry. ACIAR Tech Reports Ser No 41. Canberra: ACIAR.
Zeng, X., Li, T., Chen, C., Si, Z., Huang, G., Guo, P., et al. (2018). A hybrid land-water-environment model for identification of ecological effect and risk under uncertain meteorological precipitation in an agroforestry ecosystem. Sci. Total Environ. 633, 1613–1628. doi: 10.1016/j.scitotenv.2018.03.224
Zhang, L., Dawes, W., Hatton, T., Reece, P. H., Beale, G. T. H., and Packer, I. (1999). Estimation of soil moisture and groundwater recharge using the TOPOG IRM model. Water Resour. Res. 35, 149–161. doi: 10.1029/98WR01616
Keywords: carbon sequestration, soil conservation (SC), groundwater recharge, water retention, flooding, GHGs emission, leaching, BD conservation
Citation: Rahman MHu, Ahrends HE, Raza A and Gaiser T (2023) Current approaches for modeling ecosystem services and biodiversity in agroforestry systems: Challenges and ways forward. Front. For. Glob. Change 5:1032442. doi: 10.3389/ffgc.2022.1032442
Received: 30 August 2022; Accepted: 20 December 2022;
Published: 18 January 2023.
Edited by:
Huilin Gao, Shenyang Agricultural University, ChinaReviewed by:
Nyong Princely Awazi, The University of Bamenda, CameroonJaqueline Sgarbossa, Federal University of Santa Maria, Brazil
Sharda Gupta, Kurukshetra University, India
Copyright © 2023 Rahman, Ahrends, Raza and Gaiser. This is an open-access article distributed under the terms of the Creative Commons Attribution License (CC BY). The use, distribution or reproduction in other forums is permitted, provided the original author(s) and the copyright owner(s) are credited and that the original publication in this journal is cited, in accordance with accepted academic practice. No use, distribution or reproduction is permitted which does not comply with these terms.
*Correspondence: Muhammed Habib ur Rahman, bWhhYmlidXJAdW5pLWJvbm4uZGU=;
aGFiaWJhZ3JpQGhvdG1haWwuY29t