- 1National Centre for Earth Observation, University of Edinburgh, Edinburgh, United Kingdom
- 2School of GeoSciences, University of Edinburgh, Edinburgh, United Kingdom
Supporting a transition to net-zero carbon (C) emissions is a key component of international action to avoid dangerous climate change. Science has outlined potential routes to net-zero, which include using nature-based solutions to grow C sinks and diminish sources linked to land use and land use change. However, decision-makers are challenged by ongoing climate change and the complexity of the biosphere, interacting with socio-economic constraints. Decision-makers need science-based, but easy to use, tools to understand the current and potential future states of the terrestrial C-cycle, and its sensitivity to their decisions. These tools must provide clear uncertainty estimates to help take account of risks, must be flexible enough to be updated as new data become available, and simple enough to be deployed broadly. We argue that model-data fusion approaches, combining the systemic ecological theory embedded in intermediate complexity models with an ever-expanding collection of ecosystem observations from field and remote sensing campaigns, provide the scientific means to address each of these challenges and therefore facilitate management decisions as we face an uncertain future.
Introduction
Human interference with the global carbon (C) cycle has already led to dangerous climate change. There are now international efforts to limit the accumulation of atmospheric CO2, focussing primarily on the reduction of C emissions from fossil fuels. However, reducing emissions from the land surface and increasing biological stores of C will also play critical roles on our path to net-zero C emissions. Determining effective policy and management interventions therefore requires an understanding of the terrestrial biosphere’s C balance. In this perspective piece we argue that management of terrestrial ecosystems for net-zero requires tools that address four key challenges; (i) resolving complex behaviours of ecosystems; (ii) maximising information from observations; (iii) characterising model error and uncertainty; and (iv) effective communication of scientific knowledge to decision-makers.
The terrestrial C-cycle is multi-scale, systemic and displays non-linear behaviours. Dynamics arise from ecosystem processes connecting producers (i.e., plants) and consumers (i.e., everything else) in complex food webs. These processes have interactive controls and feedbacks that generate non-linearities. For instance, the interactions between meteorology, photosynthesis, leaf respiration and leaf traits determine the seasonal cycle of production and its geographic variation. C-cycling operates across time scales ranging from seconds (e.g., molecular processes) to millennia (e.g., C accumulation in peat). This complexity is compounded by the heterogeneity of the terrestrial biosphere, linked to topography, hydrology, geology, disturbance history, and land management.
Effective decision-making relies on being grounded in observations and supported by evidence. Earth observation (EO) provides the means to monitor the entire land surface, with increasingly frequent updates. EO can provide insights into the biological and physical states of the land surface, but field-based monitoring is vital for interpreting EO information, quantifying its biases and generating ecological knowledge. All observations contain errors and uncertainties which are often poorly known, particularly in global products. Furthermore, observations provide an incomplete picture of the terrestrial C-cycle as some key states and processes are not currently observable from space.
Process modelling provides a systemic view of the terrestrial C-cycle, with the means to explore scenarios, to compare the outcomes of alternative decisions. Modelling can generate counterfactuals, the “what-ifs” that are vital for decision-making around land management for net-zero. For example, there is active debate about the potential for afforestation, reforestation, and restoration to act as nature-based solutions. Modelling can help test whether decisions are robust against a backdrop of climate change, and project how poorly observable components such as soil C respond to tree planting. However, process models are highly complicated, often with weakly constrained parameters and lacking uncertainty estimates.
Our fourth and final challenge is the effective communication of scientific understanding to support decision-making. Our knowledge of ecosystem function and future trajectories is uncertain. These uncertainties need to be determined, explained and exchanged so that decision-makers can balance risks and opportunities, to take account of low-probability but extreme events. This information needs to be accessible to non-specialists and targetted to user needs. Solutions need to take account of local biodiversity, soils, disturbance history, and people, who must find ways to coexist with ecosystems in a sustainable way. Co-creation between scientists and end-users is vital to meet this challenge.
Recent developments in observing and modelling have enhanced understanding of the terrestrial C-cycle, however, further efforts are required. Both observational and modelling approaches have deficiencies regarding the challenges laid out above which are explored in the following sections. We then argue that robust coupling of models and observations can begin to solve these challenges, and track progress toward better solutions for decision-making. We discuss how engagement with decision-makers can make science accessible and useful. And we conclude with key recommendations for linking science to decisions.
Scaling and Representation Challenges in Observations
Over recent decades, concurrent threads of research have transformed our understanding of the terrestrial carbon cycle. The advent of large international field-based observing networks (e.g., FLUXNET, ICOS, GEM, and RAINFOR; Gwynne, 1982; Pastorello et al., 2020; Malhi et al., 2021) has expanded our understanding of the stocks and fluxes of C through terrestrial ecosystems. Additionally, the creation of collaborative repositories has enabled researchers to pool tens of thousands of field observations, revolutionising our understanding of ecosystem traits and their interrelationships (e.g., TRY and GlobAllomeTree database; Henry et al., 2013; Kattge et al., 2020). At the same time, our capacity to monitor ecosystems at global scales has been revolutionised by the rapid expansion of satellite-based remotely sensed EO. EO can uniquely provide observationally informed estimates of ecosystem status and dynamics with global coverage and increasingly, repeated estimates (Table 1). These data present an opportunity to advance our knowledge of terrestrial ecosystems. However, there remains substantial progress to be made regarding data interpretation and reducing uncertainty and bias in observations. Understanding how best to use these data is critical to providing effective support to decision-making.
Effective use of observations is restricted by measurement error and uncertainty. While field-based observations typically provide the most robust confidence estimates for ecosystem status and function, they tend to represent a small spatial area and/or short time-period. This localisation introduces error and uncertainty when scaling [e.g., via machine learning (ML)] to inform decision-making. Moreover, field-based observing networks are concentrated across temperate latitudes with little spatial and temporal coverage elsewhere, especially tropical ecosystems. For example, the FLUXNET network has just 13 sites across Africa and South America. EO estimates reduce scaling error and uncertainty by providing spatially consistent coverage but require either statistical, ML or process-models to transform the signals observed by satellites to relevant, interpretable ecological metrics. This process introduces a new source of error and uncertainty into estimates. Neither field nor EO data provide a complete picture of ecosystem C dynamics - some processes are not observed (e.g., soil C stocks); some fluxes are net estimates restricting the process knowledge we can infer (e.g., Wang et al., 2020). Constraining the terrestrial C balance therefore requires an integrated view of how stocks and fluxes are connected and correlated to maximise process learning (e.g., Bloom et al., 2020). Furthermore, error and uncertainty associated with observations make it unclear whether estimates of different components of the C-cycle are consistent, e.g., are EO derived estimates of disturbance (e.g., deforestation) consistent with estimates of aboveground biomass (AGB) change generated from a different satellite sensor?
Robust error quantification is critical for understanding the utility of observations for decision-making. However, errors are difficult to quantify, frequently underestimated and vary in space and time (Mitchard et al., 2014; Santoro et al., 2021). Zhao et al. (2020) evaluated three EO leaf area index (LAI) products using field estimates of LAI, demonstrating that the EO uncertainty estimates were a 3–5 fold underestimate of their true error. Similarly, the disagreement between independent approaches has been shown to be substantial, often larger than the uncertainty estimated for individual products, e.g., Global GPP (80–170 PgC year–1; Shao et al., 2013; Joiner et al., 2018; Jung et al., 2020), EO AGB maps (Mitchard et al., 2014; Avitabile et al., 2016) and LAI (Garrigues et al., 2008; Zhao et al., 2020). Disagreement between LAI products tend to be greatest across the tropics (Garrigues et al., 2008) but there is also a persistent overestimation of seasonality of Boreal needleleaf forests in EO LAI (e.g., Heiskanen et al., 2012). In the case of LAI and AGB, strategies for addressing these challenges are being developed, such as transparent methodologies for validation, determination of uncertainty, and the creation of a robust network of validation sites (Duncanson et al., 2019; Fang et al., 2019).
Parameter and Mechanistic Uncertainty in Process Modelling
Terrestrial Biosphere Models (TBMs) simulate the whole ecosystem, bridging the gap between observations, using numerical expressions of hypotheses underpinning ecosystem function. TBMs have varied levels of process-representation complexity, often connecting modules for specific processes such as photosynthesis and respiration. While no TBM has an equivalent complexity of real-world ecosystems, their interconnected representation of ecosystem processes provide a means of addressing complex non-linear responses of terrestrial ecosystems. The hypotheses implemented in TBMs are typically derived from analyses of field observations (e.g., Huntingford et al., 2017) and then calibrated at individual sites (e.g., Blyth et al., 2011) or as the average from trait databases (e.g., Harper et al., 2016). From these calibrations a small number of plant functional types (PFT) are typically defined and then applied globally.
TBMs have been the focus of substantial research and development over decades, providing useful insights into ecosystem function and response to changes in their environment, but have tended to become increasingly complicated to include ever more processes and complex process representations. TBMs are used to assess the response to land use and land cover change, and the associated climate change as defined by the shared socio-economic pathways (SSPs, van Vuuren et al., 2017), forming a key component of information feeding into international frameworks such as the IPCC (2021). However, there remains large divergence between Earth System Models (ESMs, within which TBMs represent the biosphere) and observation-orientated estimates of current terrestrial C stocks (Exbrayat et al., 2019) and between ESM predictions of the trajectory of the terrestrial C-cycle (Eyring et al., 2016; Exbrayat et al., 2018b; Arora et al., 2020).
The current TBM paradigm faces several obstacles toward effectively supporting decision-making toward net-zero. The process representations within TBMs have become progressively more complex (or complicated), with an increasing number of parameters that are often weakly calibrated at a limited number of sites (e.g., Blyth et al., 2011). Furthermore, model uncertainty and error are not well characterised. For example, PFTs neglect the fact that traits vary in space within a given PFT (Butler et al., 2017; Exbrayat et al., 2018a) resulting in parameters which do not represent real-world variability (Reich et al., 2014; Harper et al., 2016). Relatedly, TBMs typically implement land use/cover change by varying the relative amount of co-existing “tiles” of different PFTs simulated in the same location. Additionally, TBM calibrations widely assume that the terrestrial ecosystems are initially in steady state and are only varying in response to climate change. Therefore, these analyses do not typically include direct management impacts such as residue creation from forest felling, or subsequent regrowth dynamics. A coalition of large forest plot databases has started providing uncertainty-bounded observation constraints of post-disturbance C accumulation rates (Cook-Patton et al., 2020). However, simulating the C dynamics of regrowing forests under varied policy-relevant management regimes and future climates, with biologically plausible parameters and model structures, remains an outstanding target (Braakhekke et al., 2019; Pugh et al., 2019; Shiklomanov et al., 2020).
Supporting Decision-Makers Effectively
By 2100 global climate is likely to have changed in ways not seen by life on Earth in hundreds of thousands of years (IPCC, 2021). Furthermore, with the Glasgow Climate Pact (GCP) requiring nations to return with new nationally determined contributions by the end of 2022 and the upcoming global stocktake (UNFCCC, 2021), we expect that there will only be an increasing focus on effective change detection capacity for ecosystem C stocks. The GCP necessitates tools that provide system-level syntheses of C data that can be updated as and when new observations are available (e.g., Quegan et al., 2019). Furthermore, to support adaptation, policy makers and land managers need to understand the impact of management interventions on the resilience of existing and regenerating forests under environmental conditions with no real-world analogues (Hurlbert et al., 2019). TBMs have been and continue to be vital to addressing these challenges, through simulations of the full C-cycle. However, alongside the global and system-level insight provided by TBMs, system-level uncertainties will be essential for managing risk (e.g., low probability, high impact scenarios), and identifying key gaps in observations that can reduce model uncertainty and improve future decision-making (Hurlbert et al., 2019).
Support for decision-makers would be advanced by giving the models to decision-makers to explore; allowing direct access to scenario investigation. Supporting decision-makers to be model users gives them agency over exploring outcomes. But existing models are largely too complicated for non-specialists to use. For effective engagement, models and their user interfaces need to be simple enough to use and allow rapid scenario investigation, exploring for example management decisions and climate interactions under model uncertainty. The degree of translation from model output to user relevant information will also vary (e.g., policy makers verses foresters). Decision-makers will also question models whose initial conditions (e.g., woody C stocks) do not match their experience of reality. Models calibrated and initialised to local conditions have greater relevance and validity for regional decision-makers. Scientists can support decision-makers by co-developing model tools. Collaboration is key to ensuring that the information provided to decision-makers is the best available but also delivered in a format which is understandable and relevant to their specific circumstances.
Model-Data Fusion as a Solution
We argue that Model-Data Fusion (MDF), or data assimilation approaches provide an opportunity to address the challenges we have outlined by exploiting the information available in a diverse range of observations to inform and improve model parameterisations and process representations, while accounting for uncertainty in observational constraints (Figure 1). MDF can be viewed as theoretically informed meta-analyses, capable of synthesising multiple data-streams that constrain disparate aspects of ecosystem through a Bayesian framework. MDF provides uncertainty-bounded calibrated model analyses, which can be used to improve our understanding of ecosystem functioning and make predictions of their likely response to changes in climate, management and disturbance offering invaluable information to policy makers, individual farmers and foresters alike.
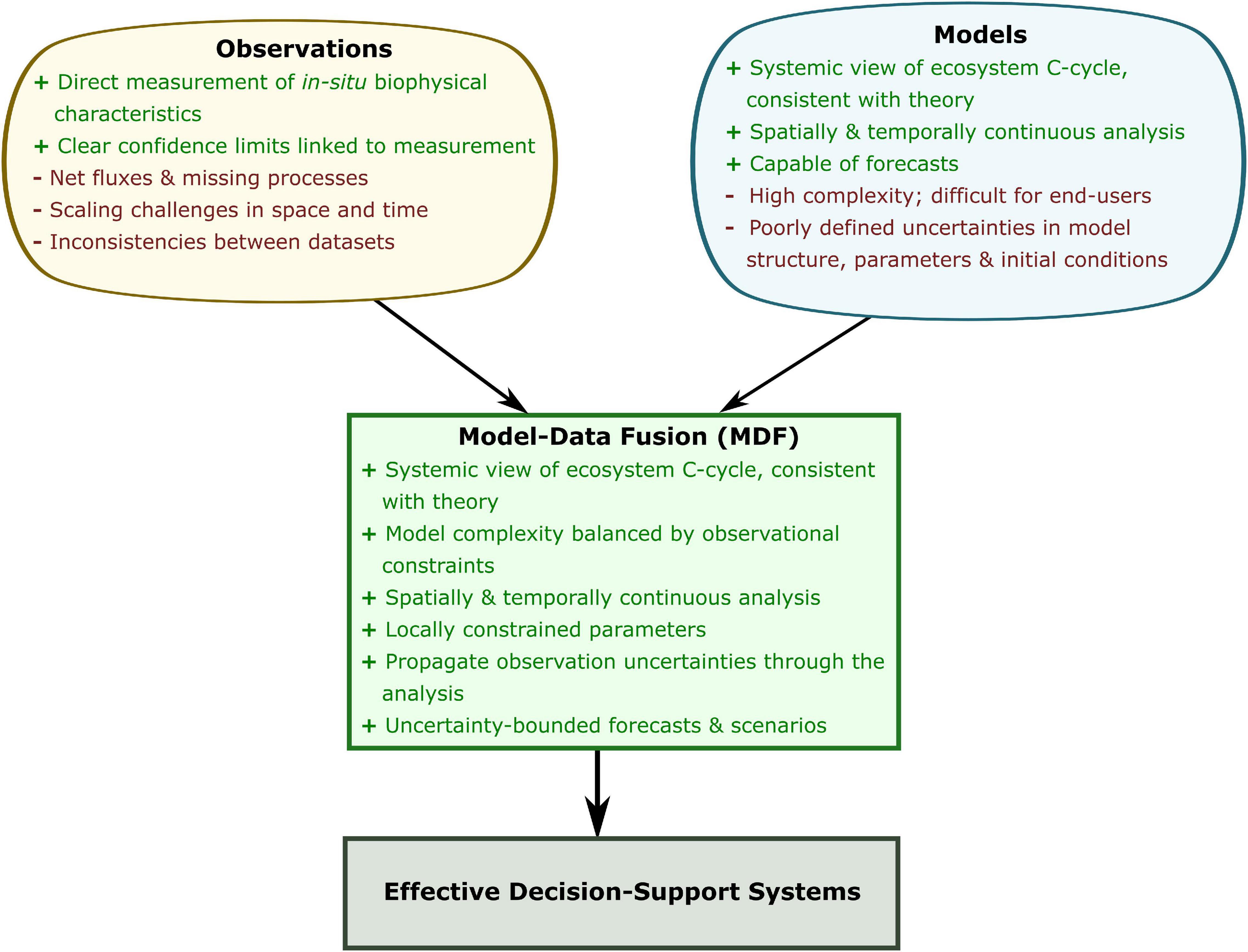
Figure 1. Schematic highlighting the strengths (+) and weaknesses (−) of observations and models and how these can be reduced or accounted for to maximise information content through model-data fusion approaches.
MDF approaches which retrieve parameter information have gained increasing traction over recent years, spanning TBMs of radically different process complexities and exploiting different algorithms (e.g., Williams et al., 2005; Fox et al., 2009; Williams et al., 2009; Kuppel et al., 2012; Keenan et al., 2013; Caen et al., 2021; Famiglietti et al., 2021). These and other studies have identified strategies for maximising the value of MDF. For example, quantifying the information content of different types of observations and how they impact TBM predictive capacity has been widely assessed (Kuppel et al., 2012; Keenan et al., 2013; Smallman et al., 2017; Famiglietti et al., 2021). Predictive skill has been shown to increase with model complexity only when sufficiently informed by observations (Smallman et al., 2017; Famiglietti et al., 2021), i.e., we must match model structure and complexity to the data available for constraint. These studies support using simpler C models supported by available observations. Simpler models are also more accessible and thus meet the challenge for expanding the mode- user community and enhancing co-creation with decision-makers.
The carbon data model framework (CARDAMOM) is an example of a MDF framework which aims to explore and address the challenges we have outlined. CARDAMOM uses a Bayesian approach within an Adaptive Proposal - Markov Chain Monte Carlo (AP-MCMC, Haario et al., 2001) to estimate the likelihood of parameters as a function of observations, observation uncertainty, ecological theory, local conditions (e.g., meteorology) and model structure (Bloom et al., 2016). The resultant CARDAMOM analysis provides pixel-level, i.e., local, ensembles of parameters for the DALEC suite of intermediate complexity terrestrial ecosystem models (Famiglietti et al., 2021) that can be combined to inform national C balance and climate sensitivity (Smallman et al., 2021). Ecological and dynamical constraints ensure that accepted parameter combinations and their resultant C stock ratios and dynamics are consistent with ecological theory (for details see Bloom and Williams, 2015).
By retrieving ensembles of location-specific parameters, CARDAMON can quantify the spatial variability of model parameters (i.e. ecosystem traits). Modelling can then directly estimate how the magnitude and spatial variability of uncertainty associated with model (DALEC) parameters affects predictions of C stocks and fluxes. Using this information, we can determine which parts of the terrestrial ecosystem are the least constrained, identify opportunities for new observations to improve constraint (Smallman et al., 2021), and how uncertainty is propagated into predictions. The DALEC model has an appropriate level of complexity relative to typically available calibration data but is simple enough to use for non-specialists to explore its behaviours and use operationally.
The carbon data model framework is not alone in adopting approaches which aim to maximise the benefits of combining observations with TBMs (e.g., Pinnington et al., 2020; Huang et al., 2021). Each approach has its own advantages and disadvantages which should be considered to ensure that the right analysis framework is used to address a given ecological question. CARDAMOM and similar frameworks are well placed to accelerate progress toward addressing the challenges outlined in the previous sections by building on our existing capacity to provide uncertainty bounded C-cycle analyses using observations of forest regrowth (Smallman et al., 2017), to impose fire (Exbrayat et al., 2018a; Yin et al., 2020) and deforestation/degradation, and simulate ecosystem responses to SSP scenarios within multiple model structures and embedded ecological realism (Smallman et al., 2021).
Recommendations for Researchers to Support Decision-Making
We have identified key challenges in both observations and models of the terrestrial C-cycle which must be overcome to effectively support climate mitigation policies and land management. We have argued that by fusing models and observations within numerical frameworks we can maximise the benefits of both approaches while minimising the limitations. From our discussion above we propose four key guidelines for effectively combining observations and models to support decision-makers, and to accelerate the process of model-data integration:
(1) Use simpler models. Increased model complexity only improves predictive capacity when supported by sufficient calibration data. For much of the C-cycle these data are missing. More complex/complicated models are also harder to explain and understand. Simpler models are quicker to build, evaluate, use or discard.
(2) Improve observational error estimates. Observations are expanding in their spatial and temporal coverages but contain bias and precision error - approaches must be developed to evaluate and then ameliorate these errors.
(3) Calibrate simpler models (i.e., 1) using observations and their errors (i.e., 2) and give decision-makers access to these models. MDF combines models with a diverse array of observations weighted by their uncertainties. Bayesian approaches allow analyses to be updated when new observations become available.
(4) Provide uncertainty estimates on all predictions. Uncertainties allow decision-makers to balance unlikely but high impact outcomes of a changing climate and human interventions. Prediction errors allow decision-makers to weigh the strength of the scientific advice against other factors.
Data Availability Statement
The original contributions presented in the study are included in the article/supplementary material, further inquiries can be directed to the corresponding author.
Author Contributions
All authors contributed equally to the conception, wrote the manuscript, and approved the submitted version.
Funding
TS and MW were supported by the United Kingdom’s National Centre for Earth Observation. DM and MW received funding from the DARE-UK project funded by the United Kingdom’s NERC Research Council (NE/S003819/1). MW and DM were additionally supported by the Newton Fund through the Met Office Climate Science for Service Partnership Brazil (CSSP Brazil).
Conflict of Interest
The authors declare that the research was conducted in the absence of any commercial or financial relationships that could be construed as a potential conflict of interest.
Publisher’s Note
All claims expressed in this article are solely those of the authors and do not necessarily represent those of their affiliated organizations, or those of the publisher, the editors and the reviewers. Any product that may be evaluated in this article, or claim that may be made by its manufacturer, is not guaranteed or endorsed by the publisher.
Acknowledgments
We thank the Global Change Ecology Lab members for useful discussions.
References
Arora, V. K., Katavouta, A., Williams, R. G., Jones, C. D., Brovkin, V., Friedlingstein, P., et al. (2020). Carbon-concentration and carbon-climate feedbacks in CMIP6 models and their comparison to CMIP5 models. Biogeosciences 17, 4173–4222. doi: 10.5194/bg-17-4173-2020
Avitabile, V., Herold, M., Heuvelink, G. B., Lewis, S. L., Phillips, O. L., Asner, G. P., et al. (2016). An integrated pan-tropical biomass map using multiple reference datasets. Glob. Change Biol. 22, 1406–1420. doi: 10.1111/gcb.13139
Bloom, A. A., and Williams, M. (2015). Constraining ecosystem carbon dynamics in a data-limited world: integrating ecological “common sense” in a model–data fusion framework. Biogeosciences 12, 1299–1315. doi: 10.5194/bg-12-1299-2015
Bloom, A. A., Bowman, K. W., Liu, J., Konings, A. G., Worden, J. R., Parazoo, N. C., et al. (2020). Lagged effects regulate the inter-annual variability of the tropical carbon balance. Biogeosciences 17, 6393–6422. doi: 10.5194/bg-17-6393-2020
Bloom, A. A., Exbrayat, J.-F., van der Velde, I. R., Feng, L., and Williams, M. (2016). The decadal state of the terrestrial carbon cycle: global retrievals of terrestrial carbon allocation, pools, and residence times. Proc. Natl. Acad. Sci. U.S.A. 113, 1285–1290. doi: 10.1073/pnas.1515160113
Blyth, E., Clark, D. B., Ellis, R., Huntingford, C., Los, S., Pryor, M., et al. (2011). A comprehensive set of benchmark tests for a land surface model of simultaneous fluxes of water and carbon at both the global and seasonal scale. Geosci. Model Dev. 4, 255–269. doi: 10.5194/gmd-4-255-2011
Braakhekke, M. C., Doelman, J. C., Baas, P., Müller, C., Schaphoff, S., Stehfest, E., et al. (2019). Modeling forest plantations for carbon uptake with the LPJmL dynamic global vegetation model. Earth Syst. Dynam. 10, 617–630. doi: 10.5194/esd-10-617-2019
Butler, E. E., Datta, A., Flores-Moreno, H., Chen, M., Wythers, K. R., Fazayeli, F., et al. (2017). Mapping local and global variability in plant trait distributions. Proc. Natl. Acad. Sci. U.S.A. 114, E10937–E10946. doi: 10.1073/pnas.1708984114
Caen, A., Smallman, T. L., Anderson de Castro, A., Robertson, E., von Randow, C., Cardoso, M., et al. (2021). Evaluating two land surface models for Brazil using a full carbon cycle benchmark with uncertainties. Clim. Resil. Sustain. [Preprint]. doi: 10.1002/cli2.10
Cook-Patton, S. C., Leavitt, S. M., Gibbs, D., Harris, N. L., Lister, K., Anderson-Teixeira, K. J., et al. (2020). Mapping carbon accumulation potential from global natural forest regrowth. Nature 585, 545–550. doi: 10.1038/s41586-020-2686-x
Duncanson, L., Armston, J., Disney, M., Avitabile, V., Barbier, N., and Calders, K. (2019). The importance of consistent global forest aboveground biomass product validation. Surv. Geophys. 40, 979–999. doi: 10.1007/s10712-019-09538-8
Exbrayat, J.-F., Bloom, A., Carvalhais, N., Fischer, R., Huth, A., MacBean, N., et al. (2019). Understanding the land carbon cycle with space data: current status and prospects. Surv. Geophys. 40, 735–745. doi: 10.1007/s10712-019-09506-2
Exbrayat, J.-F., Smallman, T. L., Bloom, A. A., Huntley, L. B., and Williams, M. (2018a). Inverse determination of the influence of fire on vegetation carbon turnover in the pantropics. Glob. Biogeochem. Cycles 32, 1776–1789. doi: 10.1029/2018GB005925
Exbrayat, J.-F., Bloom, A. A., Falloon, P., Ito, A., Smallman, T. L., and Williams, M. (2018b). Reliability ensemble averaging of 21st century projections of terrestrial net primary productivity reduces global and regional uncertainties. Earth Syst. Dynam. 9, 153–165. doi: 10.5194/esd-9-153-2018
Eyring, V., Bony, S., Meehl, G. A., Senior, C. A., Stevens, B., Stouffer, R. J., et al. (2016). Overview of the coupled model intercom-740parison project phase 6 (cmip6) experimental design and organization. Geosci. Model Dev. 9, 1937–1958. doi: 10.5194/gmd-9-1937-2016
Famiglietti, C. A., Smallman, T. L., Levine, P. A., Flack-Prain, S., Quetin, G. R., Meyer, V., et al. (2021). Optimal model complexity for terrestrial carbon cycle prediction. Biogeosciences 18, 2727–2754. doi: 10.5194/bg-18-2727-2021
Fang, H., Baret, F., Plummer, S., and Schaepman-Strub, G. (2019). An overview of global leaf area index (LAI): methods, products, validation, and applications. Rev. Geophys. 57, 739–799. doi: 10.1029/2018RG000608
Fox, A., Williams, M., Richardson, A. D., Cameron, D., Gove, J. H., Quaife, T., et al. (2009). The REFLEX project: comparing different algorithms and implementations for the inversion of a terrestrial ecosystem model against eddy covariance data. Agric. For. Meteorol. 149, 1597–1615. doi: 10.1016/j.agrformet.2009.05.002
Friedlingstein, P., Jones, M. W., O’Sullivan, M., Andrew, R. M., Bakker, D. C. E., Hauck, J., et al. (2021). Global carbon budget 2021. Earth Syst. Sci. doi: 10.5194/essd-2021-386
Garrigues, S., Lacaze, R., Jeffrey, B., Frederic, T. M., Weiss, M., Nickeson, J., et al. (2008). Validation and intercomparison of global leaf area index products derived from remote sensing data. J. Geophys. Res 113:G02028. doi: 10.1029/2007JG000635
Giglio, L., Boschetti, L., Roy, D. P., Humber, M. L., and Justice, C. O. (2018). The collection 6 MODIS burned area mapping algorithm and product. Remote Sens. Environ. 217, 72–85. doi: 10.1016/j.rse.2018.08.005
Gwynne, M. (1982). The global environment monitoring system (GEMS) of UNEP. Environ. Conserv. 9, 35–41. doi: 10.1017/S0376892900019469
Haario, H., Saksman, E., and Tamminen, J. (2001). An adaptive metropolis algorithm. Bernoulli 7, 223–242. doi: 10.2307/3318737
Hansen, M. C., Potapov, P. V., Moore, R., Hancher, M., Turubanova, S. A., Tyukavina, A., et al. (2013). High-Resolution global maps of 21st-century forest cover change. Science 342, 850–853. doi: 10.1126/science.1244693
Harper, A. B., Cox, P. M., Friedlingstein, P., Wiltshire, A. J., Jones, C. D., Sitch, S., et al. (2016). Improved representation of plant functional types and physiology in the Joint UK Land Environment Simulator (JULES v4.2) using plant trait information. Geosci. Model Dev. 9, 2415–2440. doi: 10.5194/gmd-9-2415-2016
Heiskanen, J., Rautiainen, M., Stenberg, P., Mõttus, M., Vesanto, V.-H., Korhonen, L., et al. (2012). Seasonal variation in MODIS LAI for a boreal forest area in Finland. Remote Sens. Environ. 126, 104–115. doi: 10.1016/j.rse.2012.08.001
Henry, M., Bombelli, A., Trotta, C., Alessandrini, A., Birigazzi, L., Sola, G., et al. (2013). GlobAllomeTree: international platform for tree allometric equations to support volume, biomass and carbon assessment. iForest 6, 326–330. doi: 10.3832/ifor0901-006
Huang, X., Lu, D., Ricciuto, D. M., Hanson, P. J., Richardson, A. D., Lu, X., et al. (2021). A model-independent data assimilation (MIDA) module and its applications in ecology. Geosci. Model Dev. 14, 5217–5238. doi: 10.5194/gmd-14-5217-2021
Huntingford, C., Atkin, O. K., Martinez-de la Torre, A., Mercado, L. M., Heskel, M. A., Harper, A. B., et al. (2017). Implications of improved representations of plant respiration in a changing climate. Nat. Commun. 8:1602. doi: 10.1038/s41467-017-01774-z
Hurlbert, M., Krishnaswamy, J., Davin, E., Johnson, F. X., Mena, C. F., Morton, J., et al. (2019). “Risk management and decision making in relation to sustainable development,” in Climate Change and Land: an IPCC Special Report On Climate Change, Desertification, Land Degradation, Sustainable Land Management, Food Security, And Greenhouse Gas Fluxes In Terrestrial Ecosystems, eds P. R. Shukla, J. Skea, E. Calvo Buendia, V. Masson-Delmotte, H.-O. Pörtner, D. C. Roberts, et al. (Geneva: IPCC).
IPCC (2021). Climate Change 2021: The Physical Science Basis. Contribution of Working Group I to the Sixth Assessment Report of the Intergovernmental Panel on Climate Change. Cambridge, MA: Cambridge University Press.
Joiner, J., Yoshida, Y., Zhang, Y., Duveiller, G., Jung, M., Lyapustin, A., et al. (2018). Estimation of terrestrial global gross primary production (gpp) with satellite data-driven models and eddy covariance flux data. Remote Sens. 10:1346. doi: 10.3390/rs10091346
Jung, M., Schwalm, C., Migliavacca, M., Walther, S., Camps-Valls, G., Koirala, S., et al. (2020). Scaling carbon fluxes from eddy covariance sites to globe: synthesis and evaluation of the FLUXCOM approach. Biogeosciences 17, 1343–1365. doi: 10.5194/bg-17-1343-2020
Kattge, J., Bönisch, G., Díaz, S., Lavorel, S., Prentice, I. C., Leadley, P., et al. (2020). TRY plant trait database-enhanced coverage and open access. Glob. Change Biol. 26, 119–188. doi: 10.1111/gcb.14904
Keenan, T. F., Davidson, E. A., Munger, J. W., and Richardson, A. D. (2013). Rate my data: quantifying the value of ecological data for the development of models of the terrestrial carbon cycle. Ecol. Appl. 23, 273–286. doi: 10.1890/12-0747.1
Kuppel, S., Peylin, P., Chevallier, F., Bacour, C., Maignan, F., and Richardson, A. D. (2012). Constraining a global ecosystem model with multi-site eddy-covariance data. Biogeosciences 9, 3757–3776. doi: 10.5194/bg-9-3757-2012
Lacaze, R., Smets, B., Baret, F., Weiss, M., Ramon, D., Montersleet, B., et al. (2015). Operational 333m biophysical products of the copernicus global land service for agriculture monitoring. Int. Arch. Photogramm. Remote Sens Spatial Inf. Sci. 40:53. doi: 10.5194/isprsarchives-xl-7-w3-53-2015
Malhi, Y., Girardin, C., Metcalfe, D. B., Doughty, C. E., Aragão, L. E. O. C., Rifai, S. W., et al. (2021). The global ecosystems monitoring network: monitoring ecosystem productivity and carbon cycling across the tropics. Biol. Conserv. 253:108889. doi: 10.1016/j.biocon.2020.108889
Mitchard, E. T. A., Feldpausch, T. R., Brienen, R. J. W., Lopez-Gonzalez, G., Monteagudo, A., Baker, T. R., et al. (2014). Divergent forest carbon maps from plots & space. Glob. Ecol. Biogeogr. 23, 935–946. doi: 10.1111/geb.12168
Pastorello, G., Trotta, C., Canfora, E., Chu, H., Christianson, D., Cheah, Y. W., et al. (2020). The FLUXNET2015 dataset and the ONEFlux processing pipeline for eddy covariance data. Sci. Data 7:225. doi: 10.1038/s41597-020-0534-3
Pinnington, E., Quaife, T., Lawless, A., Williams, K., Arkebauer, T., and Scoby, D. (2020). The land variational ensemble data assimilation framework: LAVENDAR v1.0.0. Geosci. Model Dev. 13, 55–69. doi: 10.5194/gmd-13-55-2020
Pugh, T. A. M., Lindeskog, M., Smith, B., Poulter, B., Arneth, A., Haverd, V., et al. (2019). Role of forest regrowth in global carbon sink dynamics. Proc. Natl. Sci. U.S.A. 116, 4382–4387. doi: 10.1073/pnas.1810512116
Quegan, S., Le Toan, T., Chave, J., Dall, J., Exbrayat, J., Minh, D. H. T., et al. (2019). The European Space Agency BIOMASS mission: measuring forest above-ground biomass from space. Remote Sens. Environ. 227, 44–60. doi: 10.1016/j.rse.2019.03.032
Reich, P. B., Rich, R. L., Lu, X., Wang, Y.-P., and Oleksyn, J. (2014). Biogeographic variation in evergreen conifer needle longevity and impacts on boreal forest carbon cycle projections. Proc. Natl. Sci. U.S.A. 111, 13703–13708. doi: 10.1073/pnas.1216054110
Rodríguez-Veiga, P., Quegan, S., Carreiras, J., Persson, H. J., Fransson, J. E. S., Hoscilo, A., et al. (2019). Forest biomass retrieval approaches from earth observation in different biomes. Int. J. Appl. Earth Observ. Geoinf. 77, 53–68. doi: 10.1016/j.jag.2018.12.008
Santoro, M., Cartus, O., Carvalhais, N., Rozendaal, D. M. A., Avitabile, V., Araza, A., et al. (2021). The global forest above-ground biomass pool for 2010 estimated from high-resolution satellite observations. Earth Syst. Sci. Data 13, 3927–3950. doi: 10.5194/essd-13-3927-2021
Shao, P., Zeng, X., Sakaguchi, K., Monson, R. K., and Zeng, X. (2013). Terrestrial carbon cycle: climate relations in eight CMIP5 earth system models. J. Climate 26, 8744–8764. doi: 10.1175/JCLI-D-12-00831.1
Shiklomanov, A. N., Bond-Lamberty, B., Atkins, J. W., and Gough, C. M. (2020). Structure and parameter uncertainty in centennial projections of forest community structure and carbon cycling. Glob. Change Biol. 26, 6080–6096. doi: 10.1111/gcb.15164
Smallman, T. L., Exbrayat, J.-F., Mencuccini, M., Bloom, A. A., and Williams, M. (2017). Assimilation of repeated woody biomass observations constrains decadal ecosystem carbon cycle uncertainty in aggrading forests. J. Geophys. Res. Biogeosci. 122, 528–545. doi: 10.1002/2016JG003520
Smallman, T. L., Milodowski, D. T., Neto, E. S., Koren, G., Ometto, J., and Williams, M. (2021). Parameter uncertainty dominates C cycle forecast errors over most of Brazil for the 21st Century. Earth Syst. Dynam. 12, 1191–1237. doi: 10.5194/esd-2021-17
UNFCCC (2021). Glasgow Climate Pact. Report No. FCCC/PA/CMA/2021/L.16. Available online at: https://unfccc.int/sites/default/files/resource/cma2021_L16_adv.pdf (accessed November 15, 2021).
van Vuuren, D. P., Riahi, K., Calvin, K., Dellink, R., Emmerling, J., Fujimori, S., et al. (2017). The shared socio-economic pathways: trajectories for human development and global environmental change. Glob. Environ. Change 42, 148–152. doi: 10.1016/j.gloenvcha.2016.10.009
Wang, J., Feng, L., Palmer, P., Lu, Y., Fang, S., Bösch, H., et al. (2020). Large Chinese land carbon sink estimated from atmospheric carbon dioxide data. Nature 586, 720–723. doi: 10.1038/s41586-020-2849-9
Wang, L., Zhu, H., Lin, A., Zou, L., Qin, W., and Du, Q. (2017). Evaluation of the Latest MODIS GPP Products across multiple biomes using global eddy covariance flux data. Remote Sens. 9:5. doi: 10.3390/rs9050418
Williams, M., Richardson, A. D., Reichstein, M., Stoy, P. C., Peylin, P., Verbeeck, H., et al. (2009). Improving land surface models with FLUXNET data. Biogeosciences 6, 1341–1359. doi: 10.5194/bg-6-1341-2009
Williams, M., Schwarz, P. A., Law, B. E., Irvine, J., and Kurpius, M. R. (2005). An improved analysis of forest carbon dynamics using data assimilation. Glob. Change Biol. 11, 89–105. doi: 10.1111/j.1365-2486.2004.00891.x
Yin, Y., Bloom, A., Worden, J., Saatchi, S., Yang, Y., and Williams, M. (2020). Fire decline in dry tropical ecosystems enhances decadal land carbon sink. Nat. Commun. 11:1900. doi: 10.1038/s41467-020-15852-2020
Keywords: earth observation, terrestrial biosphere models, terrestrial ecosystems, carbon cycle, model-data fusion, decision-making support
Citation: Smallman TL, Milodowski DT and Williams M (2022) From Ecosystem Observation to Environmental Decision-Making: Model-Data Fusion as an Operational Tool. Front. For. Glob. Change 4:818661. doi: 10.3389/ffgc.2021.818661
Received: 19 November 2021; Accepted: 30 December 2021;
Published: 20 January 2022.
Edited by:
Jörg-Peter Schnitzler, Helmholtz Center München, Helmholtz Association of German Research Centres (HZ), GermanyReviewed by:
Steffen M. Noe, Estonian University of Life Sciences, EstoniaCopyright © 2022 Smallman, Milodowski and Williams. This is an open-access article distributed under the terms of the Creative Commons Attribution License (CC BY). The use, distribution or reproduction in other forums is permitted, provided the original author(s) and the copyright owner(s) are credited and that the original publication in this journal is cited, in accordance with accepted academic practice. No use, distribution or reproduction is permitted which does not comply with these terms.
*Correspondence: Thomas Luke Smallman, dC5sLnNtYWxsbWFuQGVkLmFjLnVr
†These authors have contributed equally to this work and share first authorship