- 1Programa Nacional de Pós-Doutorado (PNPD), Programa de Pós-Graduação em Ecologia, State University of Campinas (UNICAMP), Campinas, Brazil
- 2Programa de Pós-Graduação em Botânica, Instituto Nacional de Pesquisas da Amazonia (INPA), Manaus, Brazil
- 3Coordenação de Pesquisas em Biodiversidade, Instituto Nacional de Pesquisas da Amazonia (INPA), Manaus, Brazil
The study of plant species and trait distributions can provide answers to many of the ecological challenges of our times, from climate change to the biodiversity crisis. Although traits are classically measured at the species level, understanding intraspecific variation is necessary to determine the type of response species will have to climate change. Here we measured and analyzed seven leaf traits (leaf area—LA, specific leaf area—SLA, leaf thickness—LT, leaf dry mass content—LDMC, venation density—VD, stomata length—SL, and stomata density—SD) across 14 locally dominant palm species (10 individuals/species) distributed along hydro-topographic gradients (1.4–37 m of terrain height above nearest drainage) of a central Amazonian forest to disentangle the role of species identity, relatedness, and local hydrology on trait variation and covariation. Our results show that trait variation is not always larger between species than within species as expected. Intraspecific variation accounted for 23–74% of trait variation depending on the trait. Most of the variation happened at species level for SL, LA, LT, and SD but not for SLA, VD, and LDMC. For a third of the traits (LDMC, SLA, and SD), we found some evidence of phylogenetic inertia. This lack of independency among traits is confirmed by the maintenance of strong correlation among some of those traits after controlling for local environmental conditions. Intraspecific variation, however, was not related to height above nearest drainage for any of the traits. Most of the trait–environment relationships were species-specific. Therefore, the change in palm trait composition detected along topography, from higher community means of SLA and LA, lower LT, LDMC, SL, and SD in the wet valleys to opposite traits in drier plateaus, is mostly due to the turnover in species composition and relative abundance variation. We conclude these palm species have well-defined hydrological niches, but their large intraspecific variation in leaf traits does not contribute to the adjustment of individuals to the local hydrological conditions in this Amazonian forest.
Introduction
In recent years, there has been increased interest in documenting and understanding patterns of trait intraspecific variation, and an emphasis in the importance of individual level traits to understand several ecological processes (Messier et al., 2010; Siefert et al., 2015). This is natural, since functional traits per definition are individual-level properties (Violle et al., 2007) and the individuals are the direct units of response to any environmental pressure, even if these later combine into higher levels. If traits vary considerably among individuals and, especially, if this variation is coupled to important environmental drivers, it needs to be incorporated into analyses and models to predict the responses of populations, communities, and ecosystems to environmental change (Albert et al., 2011). It has been frequently assumed that within-species variation is smaller than among species. This is exemplified by the large number of studies based on species means (e.g., Liu et al., 2021; Kelly et al., 2021) regardless of both theoretical background (Bolnick et al., 2011; Violle et al., 2012) and empirical evidence for intraspecific variation (e.g., Albert et al., 2011; Marenco et al., 2017; Osnas et al., 2018; Dong et al., 2020), pointing out that we still know little at which scales, life-forms, taxa and across which environmental gradients intraspecific variation is relevant to ecological processes. Moreover, there is a virtual lack of information on these patterns of intra to interspecific trait variation in palms, which have been suggested to be particularly susceptible to droughts (Eiserhardt et al., 2011; Esquivel-Muelbert et al., 2019; but see Emilio et al., 2019). Thus, understanding how much palm intraspecific trait variation is linked to hydrological gradients will help determine if species have the potential to adjust to new climatic conditions or will need to migrate to follow their climatic niches.
Climate seasonality is widely recognized as a driver of palm species distribution (Blach-Overgaard et al., 2010; Eiserhardt et al., 2011) and functional trait composition (Göldel et al., 2015) in large scales, but local hydrology is also strongly associated to floristic patterns in the local scale (review in Svenning, 2001; Vormisto et al., 2004; Costa et al., 2009). Local hydrology is mostly determined by topography. In the upper parts of topographic profiles the soil surface is vertically far from the water table, and plants depend more on precipitation for water supply; in the lower portions of the profile roots may be in direct contact with the water table seasonally or year-round (Fan et al., 2017). Filtering of functional traits by these varying hydrological conditions may be the basis of species composition changes along topographic gradients, as described for trees (Cosme et al., 2017; Oliveira et al., 2019). There is so far no study of this local hydrological filtering effect on palms, regardless of the many studies pointing to their clear turnover along topographic gradients. Understanding how local hydrology affects trait selection under the same macroclimate is critical to model and forecast the effects of climate change, since the interaction of plant traits and local hydrology modulates plant performance under extreme weather conditions (Estebán et al., 2021).
Local hydrology is an important ecological force driving community assembly (Silvertown et al., 1999, 2015) and is related with palm floristic variation (Costa et al., 2009; Schietti et al., 2014). Although local hydrological gradients may drive species selection, many species are generalists (Ruokolainen and Vormisto, 2000), or at least have wide niche breadths, raising the question of how they adjust to the varying soil moisture conditions. The basic expectation would be that palms have large plasticity or genetic variation (Melo et al., 2018), leading to large intraspecific variation coupled to the local hydrological variation. This has been much less examined so far, with studies showing either large intraspecific trait variation well coupled to hydro-topographic gradients (Schmitt et al., 2020) or small contribution of intraspecific variation (Cornwell and Ackerly, 2009). Again, there are no studies of intraspecific variation on palms regardless of the evidence for genetic variation from local to broad scales. Genotypic negative frequency-dependent selection has been shown to increase genetic diversity at local scales (Browne and Karubian, 2018). At large scales, genetic variation across species ranges has been detected in widespread palms (Gomes et al., 2011; Melo et al., 2018), suggesting that widespread palms may have enough genetic variation to adjust to varying environments.
In the other hand, palms could be expected to have lower plasticity in leaf traits than trees, given their lower architectural flexibility, as compared to dicotyledonous trees (Tomlinson, 1990, 2006). An example of a very conserved trait of palms is the evergreen stem-leaf apical continuum, which may limit the options to adjust to hydrological variation, as palms are not able to shed leaves in response to drought. Phylogenetic inertia and/or strong selective pressures on certain traits could also limit the capacity of intraspecific adjustment to environmental variation. For example, the well-known discrepancy in palm species richness between moist and wet habitats is more related to palms retaining traits of the ancestral biome than to differences in diversification rates between them (Cássia-Silva et al., 2019). Those potential constraints have not prevented palms from occupying a wide range of environments, from deserts to tropical forests (Dransfield et al., 2008), suggesting an ample adaptation capacity. At the same time, morphological (LMA), chemical (N and P contents), and physiological (assimilation and respiration rates) leaf traits were shown not to be conserved along the palm phylogeny, but to converge to similar values in different lineages as an adaptation to shaded conditions of forest understories (Ma et al., 2015). Thus, it is not yet clear what is the potential of palms for intraspecific adjustments to environmental variation, given their phylogenetic constraints.
Importantly, our limited understanding of trait variation is not restricted to the palm family. Although several studies have looked at intraspecific variation (e.g., Messier et al., 2010; Fajardo and Siefert, 2018; Fyllas et al., 2020), we are still unable to generalize from those results. Intrinsic and extrinsic processes operating from ecological to evolutionary timescales make understanding trait variation a complex task. As a consequence, the variance and shifts in trait–environment relationships among different evolutionary lineages may result simply from the broad phylogenetic contexts in which most studies are done, and thus obscure the understanding of the drivers of intraspecific variation (Cavender-Bares et al., 2020). This can be particularly problematic given that adaptations to new environments normally involve a combination of traits responding together to a range of selection pressures—not single traits and pressures (Reznick and Ghalambor, 2001).
Traits are unlikely to vary independently, and the set of traits (as phenotypes) may respond collectively to genetic and environmental factors (Armbruster and Schwaegerle, 1996). Traits can also show covariation that is not driven by the current environment but by the selection of optimal adaptative responses along their evolutionary histories. For example, stomata morphology may have evolved in angiosperms as response to a trade-off to maximize gas exchange while minimizing the fraction of epidermis covered by stomata (de Boer et al., 2016) and while we can hypothesize about the adaptative value of both traits separately, it is unlikely that they were selected independently. Different lineages may also have evolved alternative traits and trait combinations to respond to the same pressures (Marks and Lechowicz, 2006); thus, understanding drivers of intraspecific variation on traits requires understanding of how traits are phylogenetically constrained within lineages and the trait combinations that might be expressed in response to environmental variation. For example, in a tropical forest, most species are more likely to occur where their traits are closely related to local community weighted mean (CWM) values (Muscarella and Uriarte, 2016), but constraints to local functional variation act more strongly on multivariate phenotypes than on univariate traits. Hence, it is necessary to account for at least some of those sources of variation if we want to increase our ability to predict plastic plant responses to climate change (Nicotra et al., 2010).
To understand which traits are likely to show variation in palms, we first analyzed the sources of variation and covariation on leaf traits of co-occurring species. Then, we related trait variation at individual and community levels to local hydrological gradients. Specifically, we asked:
(1) How much of leaf trait variation is due to (a) intraspecific variation and (b) phylogenetic conservatism? We expect trait variation between species to be larger than trait variation within species. Models predict that individual traits whose values have a low dependence on the values of other traits will be more related to phylogeny than the integrative traits, which are more likely to be related to the environment (Marks, 2007) and therefore, show wider intraspecific variation. Here we evaluate this prediction by testing the phylogenetic signal for seven leaf traits and its relationship with intraspecific variation. If trait variation is phylogenetically constrained, we expect to observe lower intraspecific variation among lineages where close relatives are more similar in traits than expected by chance.
(2) What is the role of trait covariation on intraspecific variation? Traits that are related to each other can shift together as result of genetic constraints or due to intrinsic coordination in response to environmental pressures, with impact on intraspecific variation. If correlation among traits results from genetic constraints, trait covariation will be retained after controlling for trait–environment variation. In this case, intraspecific variation is expected to be low as trait combinations should be fixed. Alternatively, traits can be ecologically coordinated while structurally (or functionally) independent (Givnish, 1987). In this case, trait covariation is not intrinsic but the result of both traits being co-selected in each environment. Here, correlations among traits will disappear after controlling for the environmental effect and intraspecific variation is expected to be high if traits show plastic responses to the environment.
(3) How does environment (local hydrology) affect (a) intraspecific and (b) community level variation on leaf traits? If intraspecific variation is adaptative in relation to local hydrology, we expect trait variation to relate to the local hydrological gradient after controlling for species and other environmental gradients (hereafter local conditions). Alternatively, if intraspecific variation emerges independently from hydrological gradients—for example, as a result of trait covariation (hypothesis question 2), this relationship will not persist after controlling for species or other environmental effects. At the community level, we expect average community traits to be related to local hydrological gradients even when trait variation within species is decoupled from local hydrology variation, if selection is operating over multivariate phenotypes (hypothesis question 2) and those traits are not strongly phylogenetically constrained (hypothesis question 1). In this case, average community weighted traits will be the result of species turnover and abundance variation instead of trait responses to hydrological gradients within species.
Materials and Methods
Study Site
The study was conducted at the Ducke Forest Reserve, a Long-Term Ecological Research (PELD) site with an area of 10,000 ha, located 26 km north of Manaus, Brazil (2° 55′ S, 59° 59′ W). Climate is moist tropical (“Am” tropical according to Köppen–Geiger classification), with a relative humidity of 75–86%, mean annual rainfall of 2,500 mm (over the last 53 y), and 1–2 dry months with <100 mm precipitation (Costa et al., 2020). The predominant vegetation is dense lowland forest, locally known as terra-firme forest. Topography is undulating with a dense drainage network, resulting in plateaus that are dissected by small streams and valleys, with an average height difference of about 30–40 m between valleys and plateaus (Chauvel et al., 1987). In the valleys, the water table is around 0–2 m deep year-round (Hodnett et al., 1997), while in plateaus and hillslopes, the water table is below 30 m (Cuartas et al., 2012). Clay content is higher in the plateaus and sand content is higher in the valleys (Luizão et al., 2004). The soils are acidic and poor in phosphorus, calcium, magnesium, sodium, and potassium, and in general the aluminum content is high (Chauvel et al., 1987). We refer to the combination of all those environmental characteristics and their consequences (e.g., forest dynamics) as local conditions.
A grid of trails covering 8 × 8 km gives access to 72 permanent plots 1 km apart from each other (Magnusson et al., 2005). Plots are 250 m long, with this long axis aligned with the elevation contour to minimize variation on soil properties and hydrology. We selected 14 plots covering the hydrological gradient for the collection of data on functional characteristics (cf. Supplementary Figure 1). Species abundance data came from 72–250 × 4 m plots sampled by Jean Louis Guillaumet (see Costa et al., 2009) and made available in the data repository of the Brazilian Biodiversity Research Program (PPBio, please see section “Data Availability Statement”).
Collection of Leaf Trait Data
We collected data on the leaf traits of 10 individuals per species, for the 14 most abundant species in the area (Costa et al., 2009; Supplementary Table 1). Individuals were selected along the plots to cover the full range of hydrological conditions occupied by each species (Supplementary Figure 1). For understory palms, we selected only adult individuals, who were recognized by the presence of reproductive structures. For the canopy species, the selection was made based on individuals 3–12 m height. Canopy height in those forests is around 25–30 m height; sampling was performed avoiding forest gaps and other light patches. Thus, all individuals (regardless been from canopy or understory species) were sampled under the same average understory light conditions. We collected from each individual showing evidence of healthy growth, i.e., growing apical meristem and at least three green intact leaves, one healthy leaf (without or with minimum visually detectable fungi, lichens, herbivory marks, or deformities), to represent the optimal state of the organ. Whenever possible, we standardized out sampling to collect the second or third leaf of the plant, which represents a fully expanded mature dark green leaf, in order to minimize the effect of leaf age on the measured traits. A summary of collected samples, including plant height, is available (Supplementary Table 1).
In the laboratory, we separated six leaflets (two from the base, two from the middle, and two from the apex of the leaf), three of which were used for anatomical measurements and three for morpho-anatomical measurements. The values of each anatomical and morpho-anatomical characteristics of each individual were then calculated as the average of the three leaflets representing the base, middle, and apex of the leaves. Individuals of Bactris hirta and Geonoma aspidiifolia had entire or semi-entire leaf blades, so the entire blade was weighed and scanned, and we made only one measurement of thickness and one anatomical cut per leaf of those species. On these leaflets, we measured the thickness with a micrometer (between the central rib and the margin) and the area (scanned and quantified with the ImageJ software, Rasband (1997–2008), available at: http://rsb.info.nih.gov/ij) according to the protocol of Perez-Harguindeguy et al. (2013). After these measurements, leaflets were dried at 65°C for 72 h, and weighed with an accuracy of 0.0001 g. All the leaflets of each leaf were weighed to obtain fresh weight.
We cut a square centimeter between the border and the central rib of the three other leaflets to quantify the anatomical structures. These pieces were fixed for 72 h in FAA solution (formaldehyde, glacial acetic acid, and alcohol). After this period, one of the pieces was prepared for visualization of veins (with diaphanization in 2% sodium hypochlorite) and another immersed in Franklin’s solution 1:1 (glacial acetic acid and hydrogen peroxide) for dissociation of the epidermis and visualization of stomata. Subsequently, both samples were clarified in sodium hypochlorite 5%, dehydrated in an alcoholic series (25–95%), and stained with safranin (Kraus and Arduin, 1997), and the slides were mounted for measurements.
The dry matter content (LDMC, mg g–1) was calculated by the ratio of the sum of the fresh weights to the sum of the dry weights of the three leaflets per individual. Specific leaf area (SLA, cm2 g–1) was calculated by the sum of the areas of the three leaflets divided by the sum of their dry weight. To estimate the total light-absorbing surface of the leaves, we measured and weighted a single leaflet and then scaled up by converting the summed weight of all leaflets to area (Renninger and Phillips, 2011).
The vein density (mm2) was measured in three images per leaflet, with 40× magnification, using the Leaf Gui software (Price, 2012, available at: http://www.leafgui.org/). Stomata density was measured in three images per leaflet, at 20× magnification. The size of the stomata, estimated here by the length of the guard cells (μm), was also calculated from the average of three images from each leaflet, recorded at a 40× magnification. The images were analyzed using the ImageJ software.
Environmental Data
The local hydrology was represented by the vertical distance of the terrain surface to the nearest drainage (HAND, Height Above Nearest Drainage; Rennó et al., 2008) at the plot level. HAND (m) is an estimate of the soil water drainage capacity, with low values indicating terrain close to the water table, and high values are terrains with greater vertical distance to the water table. The HAND values were obtained from Schietti et al. (2014). Local hydrology, determined by changes in topography and highly associated to soil texture, is the major gradient structuring community composition in this area (Costa et al., 2009, 2015; Schietti et al., 2014).
Data Analysis
Variation Partitioning
To understand how much of the leaf trait variation is due to intraspecific variation (Question 1a), we performed a variance component analysis using linear mixed models (LMM), in which we partitioned trait variance between species and local conditions. To estimate the components of trait variance, we fitted an intercept-only linear mixed model, where random differences from group to group are modeled as a group dependent intercept (Snijders and Bosker, 2011) as in the equation below:
where each trait (T) was measured from an individual that belongs to a species(s) found in a plot (p) with specific local conditions. Here we implemented the model with plots representing the spatial variation on local conditions given by the environment, e.g., topography, soil, and hydrology (Nakagawa and Schielzeth, 2010; Harrison et al., 2018). Then, we estimate the among-species and among-plot variance in our trait of interest from the comparison of the observed variance within and between species and plots. This model also allows the estimation of the residual variance term (R, here the amount of variation that is not explained by species or plots) which includes both natural within-species variability not related to plot variation, and any measurement error. All parameters were estimated by the residual maximum likelihood (REML) method with the lme4 library (Bates et al., 2015).
Total explained variance was calculated by pooling among-species (s), among-plots(p), and residual (R) variance together. Variance was then partitioned among the three components in relation to the total with s% representing the amount of variation explained by species, p% representing the amount of variation explained by plot, and R% representing the unexplained variation. Intraspecific variation was calculated from pooling together p% and R%. Using mixed models for variance partitioning is advantageous when considering numerous species or sites because of increased estimation accuracy, as it does not use as many degrees of freedom as ANOVA (Harrison et al., 2018).
Phylogenetic Signal
To understand how much of leaf trait variation is due to phylogenetic conservatism (Question 1b), the presence of a phylogenetic signal (i.e., is the tendency of related species to resemble one another) was tested for each trait using two most commonly used indexes, Pagel’s lambda (Pagel, 1999) and Bloomberg’s K (Blomberg et al., 2003). Pagel’s lambda (λ) is a scaling parameter for the correlations of traits between species relative to the expected under a Brownian motion model of evolution. The value of λ approximates (i) zero when the relationship between species traits departs from the expected under Brownian motion (i.e., traits are changing along evolution independently of phylogeny) and (ii) one when the relationship between species traits approaches the expected under Brownian motion (i.e., tend toward phylogenetic conservation). Bloomberg’s K is a scaled ratio of the variance among species traits over the contrast’s variance. The value of Bloomberg’s K approximates (i) zero when related species have traits more similar than expected from their phylogenetic relationships, (ii) one when the relationship between species traits approaches the expected under Brownian motion, and (iii) exceed one when related species traits are less similar than expected by their phylogenetic relationships. Analysis of simulated data shows that Pagel’s lambda performs better when traits follow the Brownian motion model of evolution and Bloomberg’s K when there are changes in evolutionary rates along time (Münkemüller et al., 2012); thus, their combined use should cover both possibilities. Phylogenetic data were obtained from a published species level palm phylogeny (Faurby et al., 2016). Simple mean trait values per species were used for phylogenetic signal tests over 1,000 posterior distribution equally probable phylogenetic trees from Faurby et al. (2016).
Trait Covariation
The role of trait covariation on intraspecific variation (Question 2) was examined using correlation matrices among traits. Specifically, we tested the hypothesis of trait coordination constraints on intraspecific variation by partitioning the coordinated response of multiple traits to local conditions (ecological coordination) from trait covariation that is decoupled from local conditions (intrinsic coordination). The rational for this approach is the expectation that when we control for the local conditions on trait variation, the trait covariations resulting from local conditions co-selection will disappear while trait covariation that is independent from local conditions will persist (Figure 1). We implemented this by comparing the correlations based on mean trait values per species calculated from the original sampled (raw) data to the correlation calculated from species random intercepts, which here represents differences among species excluding the environmental conditions given by the plot-effect (see section “Variation Partitioning”). Significant associations among traits were graphically represented using trait covariation networks for models based on raw data and environmentally controlled data given by the R package qgraph (Epskamp et al., 2012).
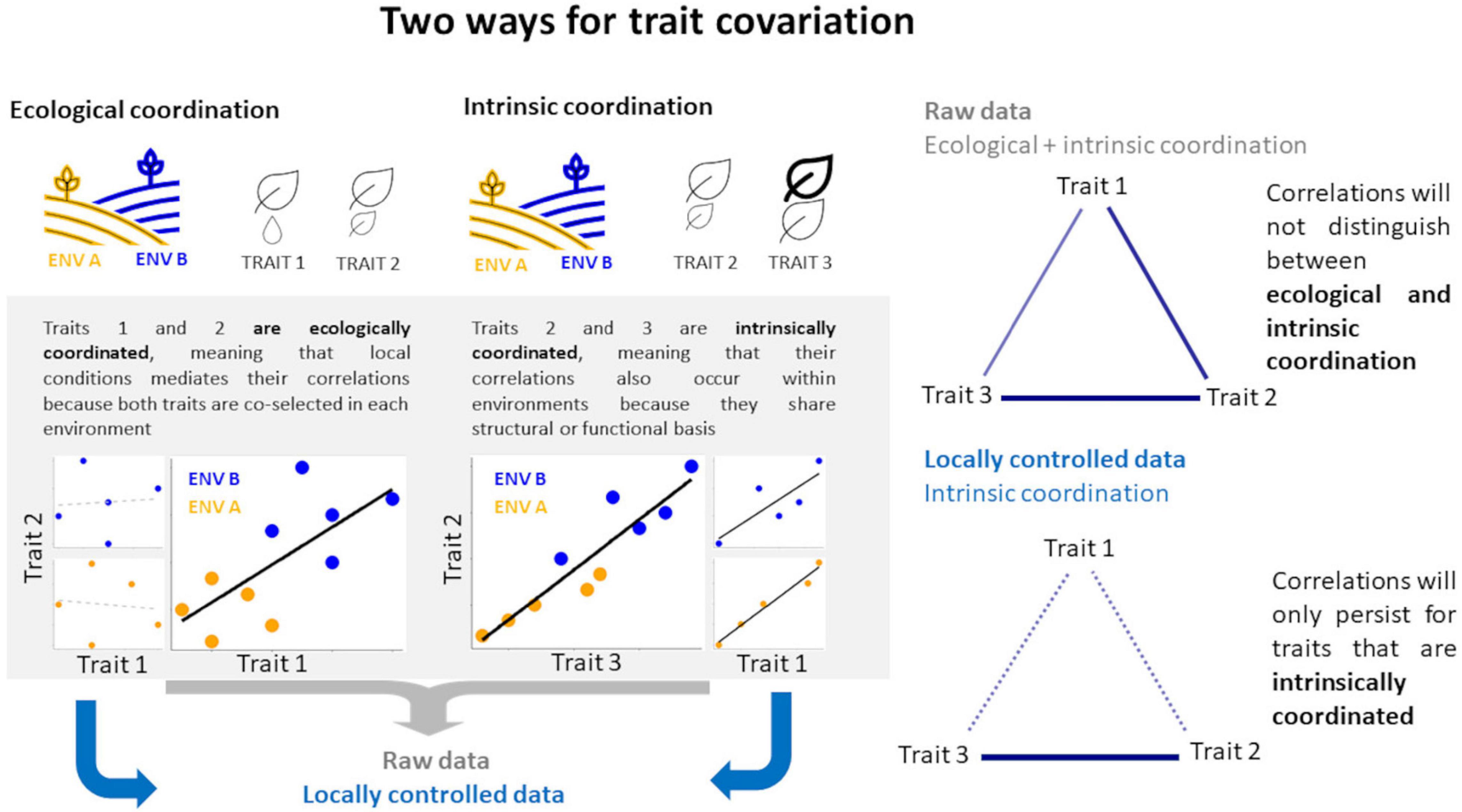
Figure 1. Diagram showing two ways for trait covariation. In this hypothetical example, a single species is distributed along two local environmental conditions (ENV A = orange, ENV B = blue) and three traits are measured (Trait 1, Trait 2, and Trait 3) along the gradients in ENV A and ENV B. Correlations among traits occur for Trait 1 vs. Trait 2 and for Trait 2 vs. Trait 3 when data from both environmental conditions are pooled together (large plots, left-hand side). However, correlations may be stronger, weaker, or non-existent when the effect of environment is controlled (small plots, left-hand side). Therefore, we will only be able to discriminate between ecological and intrinsic coordination when comparing correlations based on raw data with correlations based on locally controlled data.
Trait–Environment Relationships
To evaluate the role of local hydrology on intraspecific variation on leaf traits (Question 3a), we tested the effect of local hydrology (HAND) on the intraspecific trait variation by expanding the LMM model used before to include HAND as a fixed factor, and species and site as random intercepts. The site effect considers any spatial or unmeasured environmental effects on trait variation. Then, to determine the effect of HAND in the community level trait values (Question 3b), we regressed plot CWM against HAND. Community weighted means of the 72 plots for which we have relative abundances were calculated with the formula:
where w = relative abundance; j = species; k = plot; and T = mean species trait.
Results
How Much of Leaf Trait Variation Is Due to Intraspecific Variation? Within vs. Between Species Trait Variation
Within-species leaf trait variation was not always smaller than between-species variation (Supplementary Table 2). SL, LA, SD, and LT were the traits with larger proportion (>50%) of variation explained by species identity than by intraspecific variation. VD and LDMC had less than 50% of variation explained by species identity, and thus a larger contribution of within-species variation. Species identity explained between 26 and 77% of the variance on leaf traits. Intraspecific variation was between 22 and 73% with local environmental conditions explaining between 1 and 11% and unexplained (residual) variation accounting for 19–71%. The decreasing in the proportion of variance explained by species identity was mirrored by an increase in the residuals, and not by an increased amount of variation explained by local conditions (Figure 2).
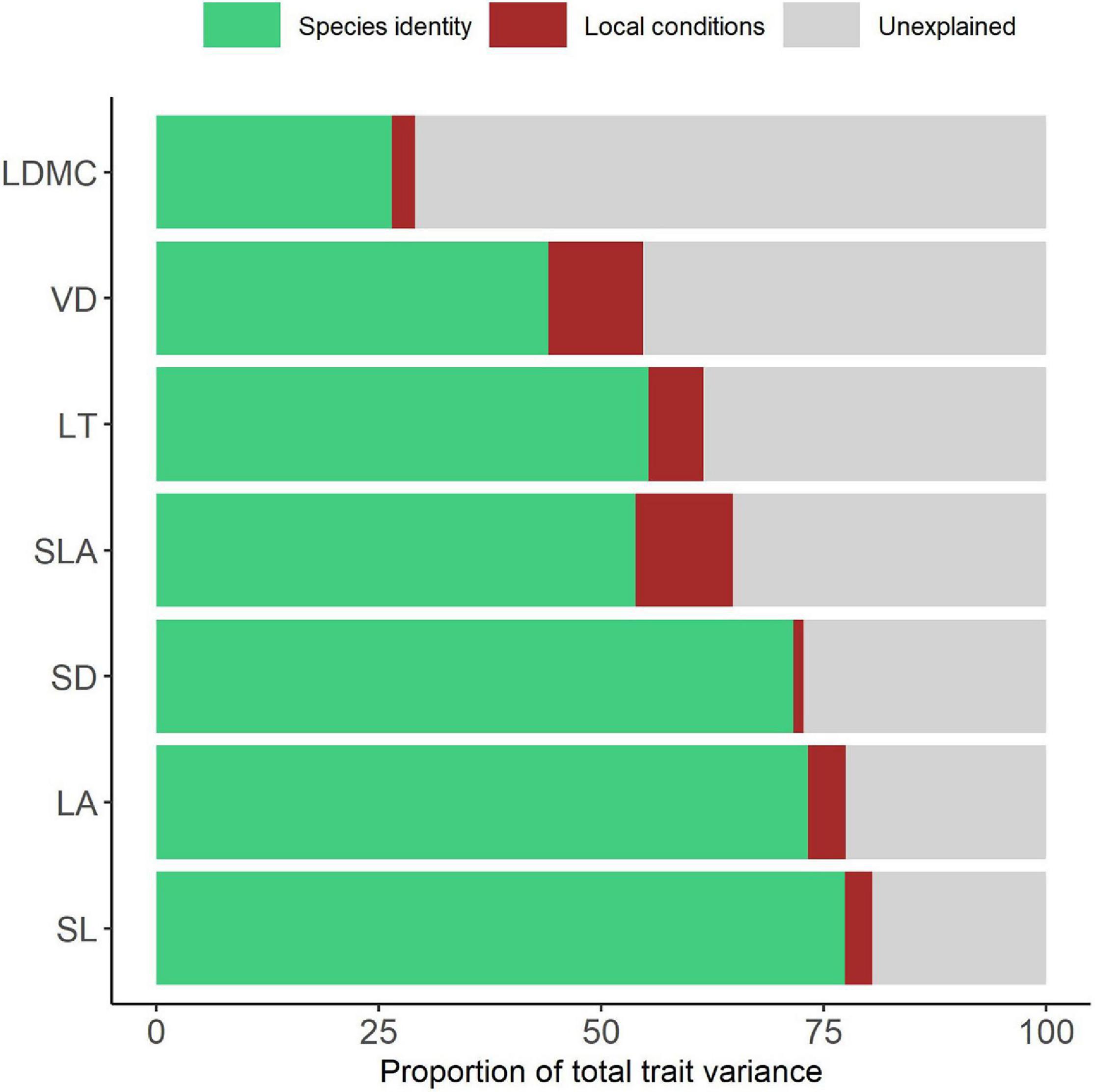
Figure 2. Partitioning of variance for each leaf trait into local conditions (plot), species identity and unexplained (residual). Leaf traits are sorted from low to high unexplained variation. LDMC, leaf dry mass content; VD, venation density; LT, leaf thickness; SLA, specific leaf area; SD, stomata density; LA, leaf area; SL, stomata length.
How Much of Leaf Trait Variation Is Due to Phylogenetic Conservatism? Phylogenetic Variation
Our results show evidence of phylogenetic conservatism in the leaf traits of co-occurring palm species for only a third of traits (Table 1). In most of the phylogenetic trees, leaf trait values varied as much within close related species than among distant ones (Supplementary Figure 2). Pagels’s lambda was above the threshold of statistical significance (p < 0.05) for all phylogenetic trees while Bloomberg’s K was above this threshold for most of them. We found evidence of trait conservatism in 74% of the phylogenetic trees for SD, 27% for SL, and 22% for LDMC. For VD and LT, we found phylogenetic signal for less than 5% of the 1,000 equally probable phylogenetic trees, while for LA and SLA, we found for none. Phylogenetic signal was not correlated to trait intraspecific variation (r = 0.06, p = 0.895, Supplementary Figure 3).
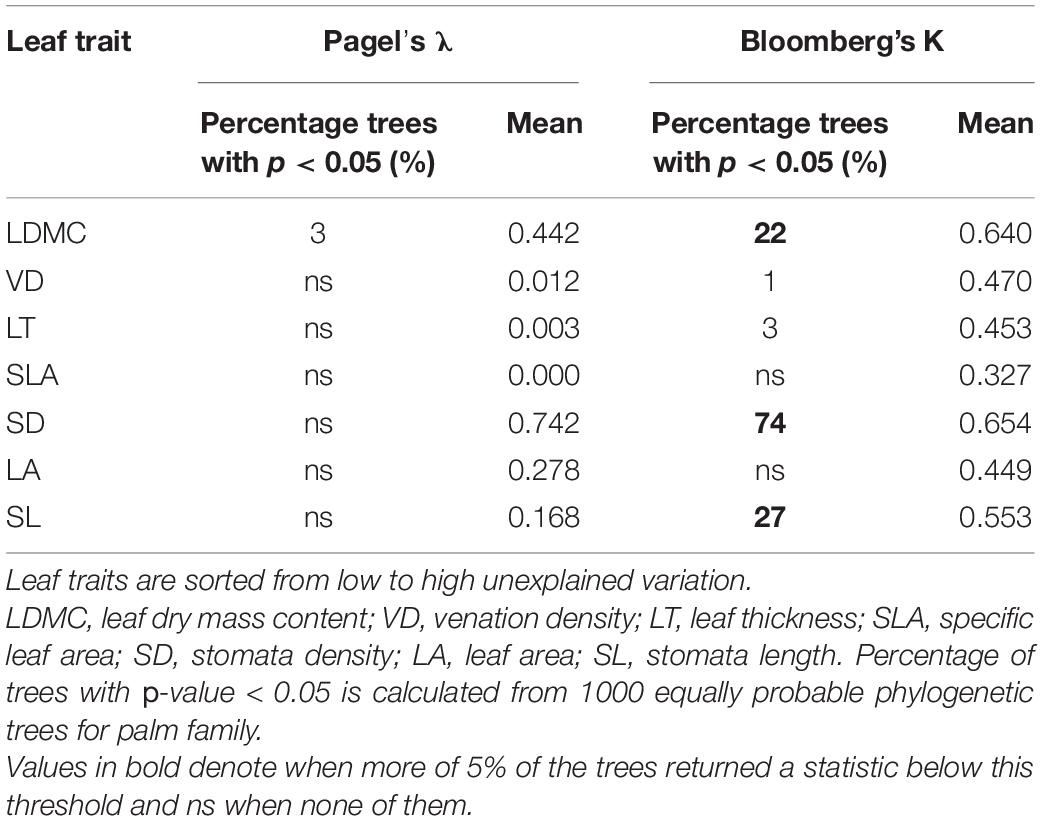
Table 1. Phylogenetic signal of the palm leaf functional traits, across the phylogeny of the local community.
What Is the Role of Trait Covariation on Intraspecific Variation? Among Traits Relationships
The simple correlations between traits were between 0.19 and 0.57 among all comparisons (Supplementary Figure 4). LA, SD, VD, and SLA were positively correlated to each other and negatively correlated to LT. SL was negatively correlated to all other traits, but LT. Trait covariation relationships changed when we control for trait–environment relationships (Figure 3). The only correlations that remained significant were among SLA, VD, and LT in one cluster, and among SL and LA in another. Correlations between VD and other traits nearly doubled after controlling for environmental effects, as well between SLA and LT. Correlations between LA and SD changed little.
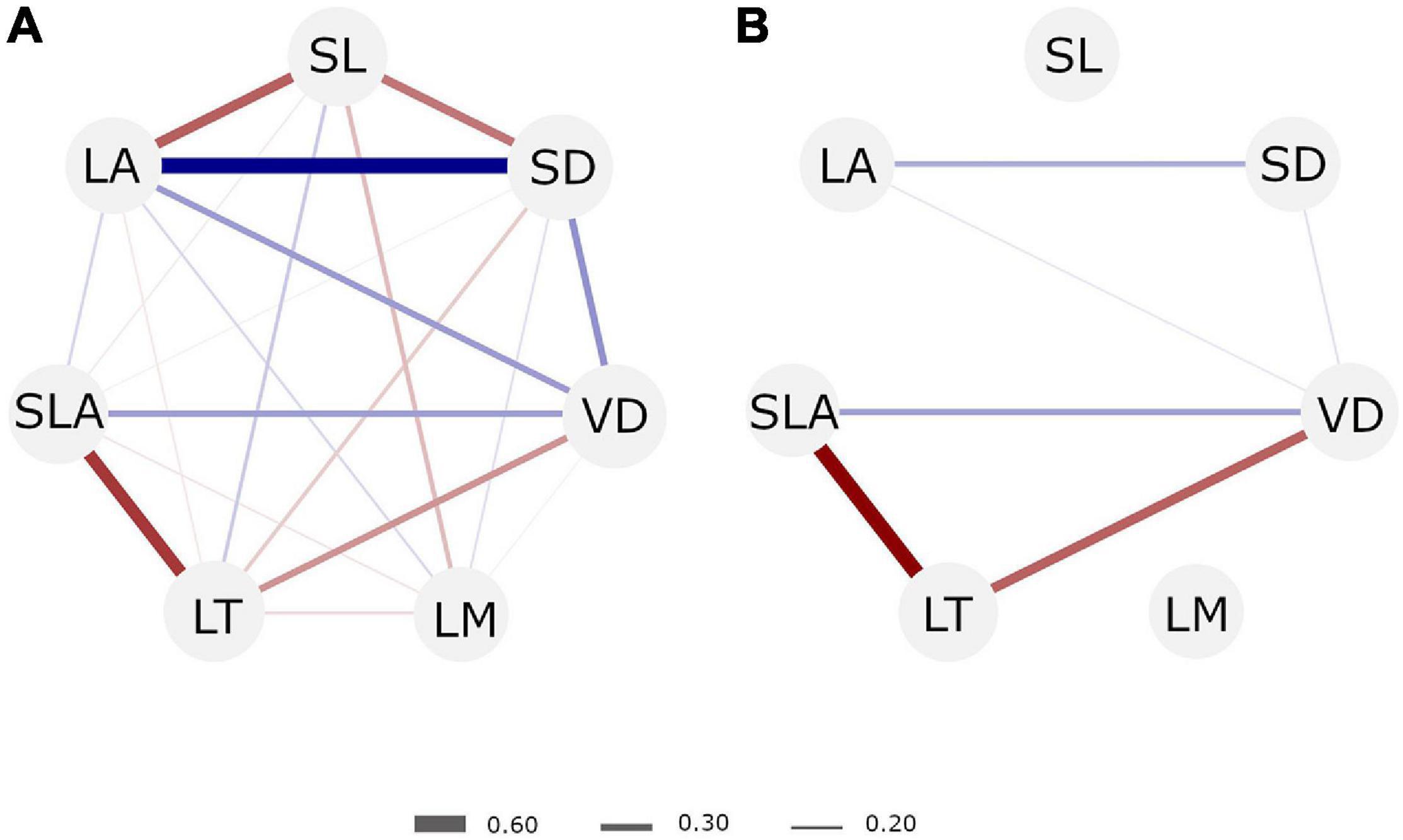
Figure 3. Correlation between leaf traits (A) based on raw trait data and (B) based on the proportion of phenotypic variation attributed to the species effect (s%) that excludes the plot(environmental)-effect (see Figure 1 and section “Materials and Methods” for details). Positive correlations are denoted in blue tones and negative correlations in red tones. Correlation strengths are represented by line thickness. Only correlations with p-value below the threshold of 0.05 are shown. SL, stomata length; SD, stomata density; VD, venation density; LM, leaf dry mass content; LT, leaf thickness; SLA, specific leaf area; LA, leaf area.
How Does Environment (Local Hydrology) Affect Intraspecific and Community Level Variation of Leaf Traits? Trait–Environment Relationships
Height above nearest drainage explained almost no intraspecific variation on palm leaf traits on the models controlling for species and local conditions (Table 2), meaning that, on average, the observed intraspecific variation was not associated with the variation in the hydrological condition. Some species, however, did show a trend of intraspecific variation of some traits (e.g., VD and SL for Bactris hirta) aligned to the environmental variation, although most of these trends tend to be weak (Figure 4). The lack of a concerted response of intraspecific variation across all species to the environment is given not only by weak within-species effects but also by opposed trends across species, e.g., leaf thickness of Astrocaryum tucuma increased with HAND while that of Bactris hirta decreased (Figure 4). At the community level, most traits’ CWM changed along HAND (Table 3). SLA and LA decreased, while LT, SD, and VD increased with HAND (Figure 4).
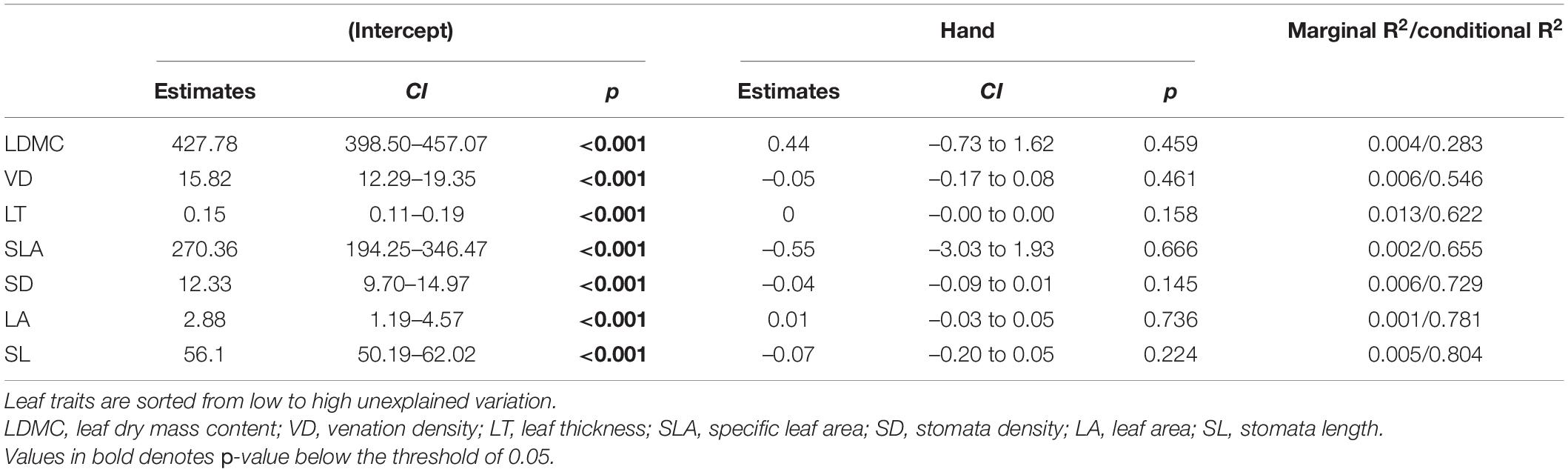
Table 2. Results of linear mixed models including height above nearest drainage (HAND) as fixed factor and species and local conditions (plot) as random intercepts.
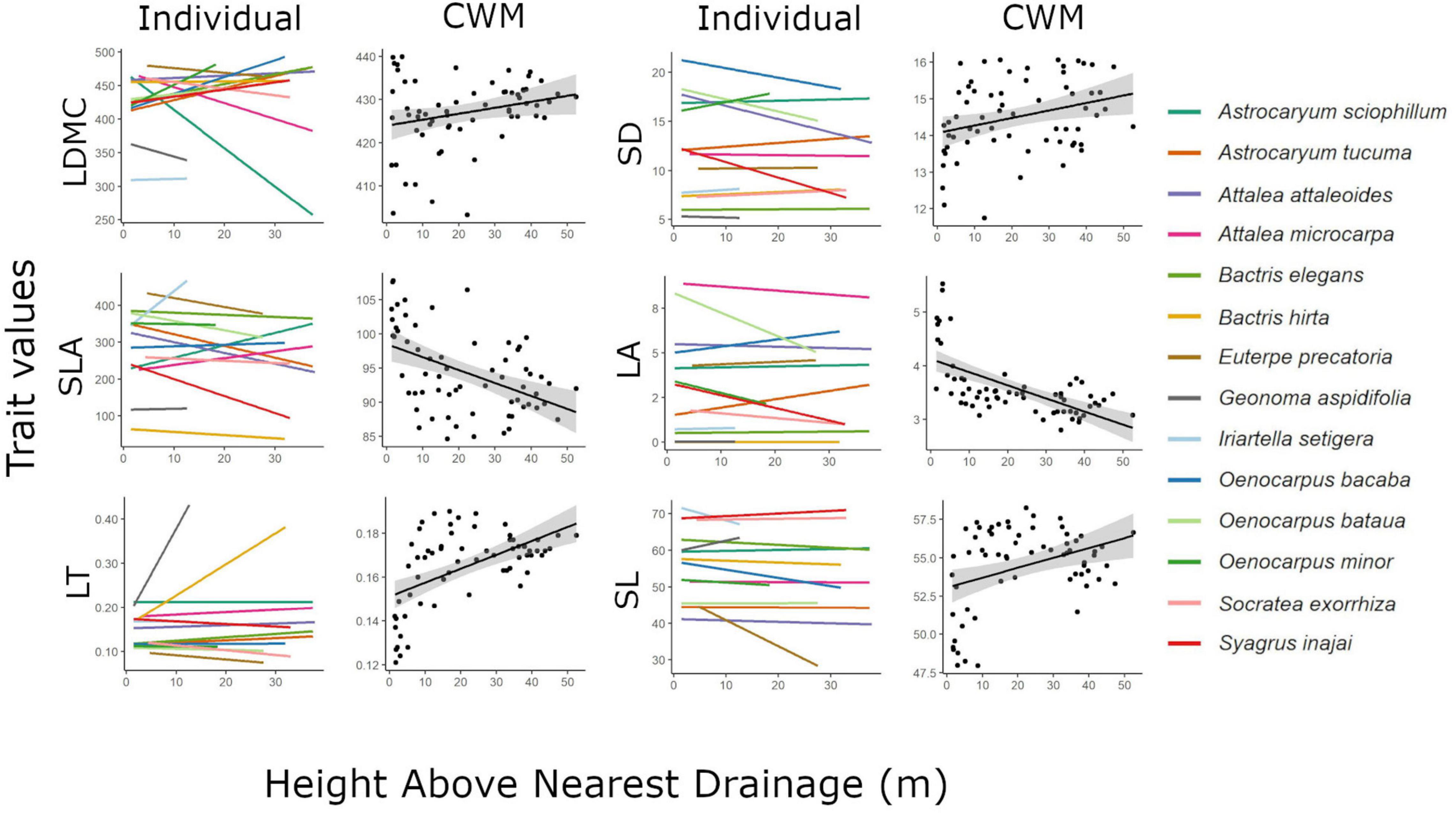
Figure 4. Individual and community weighted mean (CWM) trait variation along height above nearest drainage gradient. Only relations with p-value below the threshold of 0.05 for CWM models (Table 3) are shown. LDMC, leaf dry mass content; VD, venation density; LT, leaf thickness; SLA, specific leaf area; SD, stomata density; LA, leaf area; SL, stomata length.
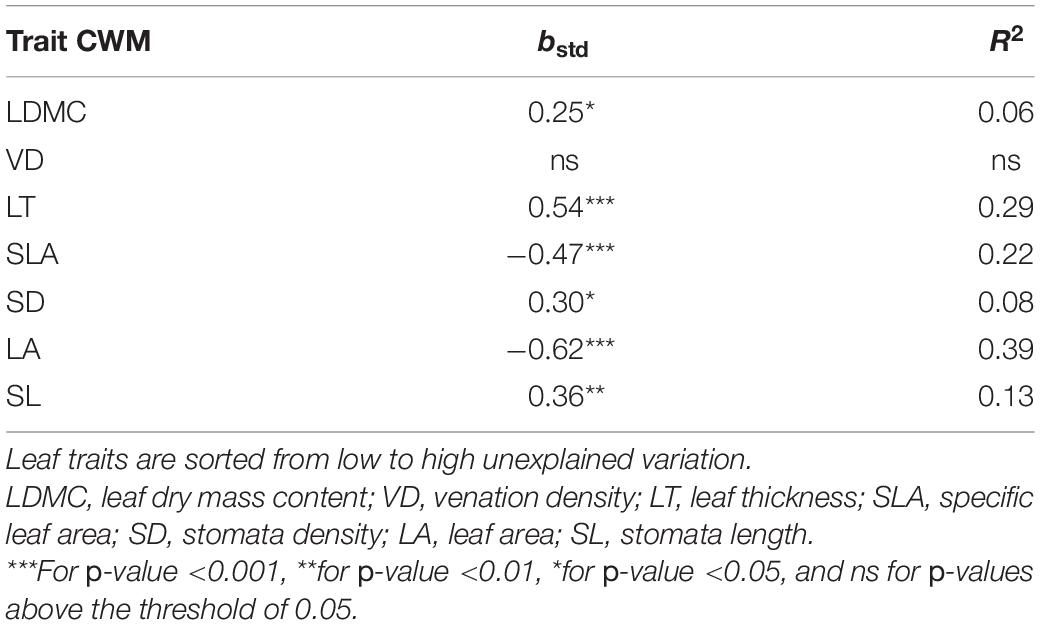
Table 3. Standardized beta coefficients for relationships between the community weighted mean of seven leaf traits (Trait CWM) and the local hydrology, represented by height above nearest drainage (HAND).
Discussion
In this study, we addressed the patterns of intra to interspecific variation in leaf traits of locally dominant palm species, and its relationships to local hydrology, in a moist forest of central Amazonia. We found that: (1) traits vary widely within species; (2) most traits do not have phylogenetic signal at the studied mesoscale (10 × 10 km); (3) correlations among traits were mostly due to intrinsic species constraints, i.e., few of the evaluated traits are free to respond to the environment independently from others; (4) intraspecific trait variation was not associated to the hydrological gradient in a coherent form across all species; and (5) even though species showed low intraspecific adjustment to hydrology, community-averaged traits closely tracked hydrological changes.
Our results show that, even in a local scale, the palm leaf traits have large amounts of intraspecific variation. Within-species variation was mostly not related to the variation in local hydrological conditions across species. Although some combinations of species and traits did show trends of variation aligned to the environment, we did not find the trait variation along the main environmental gradient driving community composition changes to be consistent. Studies comparing contributions of intra vs. interspecific variation to the changes of trait values along environmental gradients have shown consistently higher contributions of interspecific than the intraspecific component, on either trees or herbs, and across different vegetation types and spatial scales (Cornwell and Ackerly, 2009; Jung et al., 2010; Kichenin et al., 2013; Siefert et al., 2014; Luo et al., 2016). Here we suggest that the same is true for the palm life-form, even though the exceptionally low contribution of the intraspecific component recorded in this study is new.
We did not detect strong phylogenetic conservatism in the examined traits at this local scale that could suggest limited capacity of change in trait values. This may result from the combination between our focus on the local scale—where genetic distinction among populations is less likely to be relevant—and a single monophyletic lineage, therefore restricting the number of alternative traits and combinations that might have evolved in response to the same pressures. These results are in agreement with the lack of phylogenetic conservatism of leaf traits in 80 species widely distributed among the major palm clades (Ma et al., 2015). Most of the evolutionary divergences in traits of our studied palms occurred within genus or family levels, and there is strong segregation of congeneric or confamilial species along the hydrological gradient (Supplementary Figure 1). At the same time, we observed substantial intraspecific variation in the examined leaf traits, indicating that indeed these species have the potential to express variation in traits. Therefore, it is not phylogenetic inertia that limits the intraspecific adjustment of trait values to the hydrological gradient.
It is not clear if palms have an intrinsically lower capacity for environmental adjustment of the anatomical and morphological leaf traits such as those studied here, in comparison with other life-forms, or other factors may be responsible for the observed patterns. One possibility is that the low seasonality (∼2 dry months) of the studied forest could decrease the plant-perceived local hydrological differences, even if they are striking to us (e.g., Hodnett et al., 1997), and not demand intraspecific adjustments. This, however, would be at odds with the strong palm species turnover along this gradient (Costa et al., 2009) and the strong adjustment to similar gradients observed in Guiana trees subjected to the same seasonality (Schmitt et al., 2020). Intraspecific adjustments may, otherwise, be occurring in physiological traits, below-ground traits, or even at the whole organism level (e.g., above- to belowground ratios, stem to leaf area ratios, fine root to leaf area ratios, etc.). Seasonal hypoxic or anoxic soil conditions in the valleys should demand adjustments in the metabolism and usually require special structures such as aerenchyma, lenticels, or physiological adjustments (Parent et al., 2008). These adjustments may not be linked to the traits examined here, and thus we cannot conclude that no intraspecific adjustments to the hydrological gradient are occurring, but only that not in the leaf morpho-anatomical traits we studied.
Intraspecific variation, however, was not associated with the hydrological gradient in our study area in a coherent form across all species. Although it is possible that intraspecific variation in palm leaves results from unmeasured environmental variables, the relatively small amount of variation explained by plots within-species suggests that conditions that vary at plot scale (e.g., soil characteristics and canopy openness) are unlikely to be related to the observed variation. It is interesting to note that in our study area, the hydrological conditions covary with soil fertility, with a tendency for lower fertility toward bottomlands. Although this correlation may be a confounding factor, the direction of trait selection we observed at the community level is more compatible with selection by the hydrological than by the fertility gradient. For example, we observed higher values of SLA and lower values of LDMC where soils are very wet but poorer, which is the opposite of the expected based on fertility. Therefore, the patterns of trait variation observed here are more likely to be linked to changes in local hydrological conditions than the other known dominant gradients. Examining how contrasting selection forces (as exemplified here) could also be contributing to increase intraspecific variation may be an interesting line of investigation for next studies.
Differences in leaf age and microenvironment conditions could explain some of the intraspecific variation observed. Although we have controlled in our sampling for leaf age and forest stratum, the longevity of palm leaves in forest understory varies between 2 and 9 years (Henderson, 2002). During this time, the microenvironmental conditions in forest understory (especially light conditions) may change adding an uncontrolled source of variation at the individual level. Other microenvironmental conditions of relevance for palms are topography, soil, and litter distribution (Svenning, 2001). In this study, we controlled by soil and topographic variation sampling in plots that follow topographic contour in order to minimize local soil variation (Magnusson et al., 2005). Litter variation was not controlled in this study. Litter depth shows temporal and spatial variation in our study area (Rodrigues and Costa, 2012) but is unlikely to affect any of the traits measured here, at least not directly. We believe the care taken during the sampling was compatible with the microenvironment variation observed in the field and we do not expect it to have an important impact on our conclusions. However, microenvironmental variation experienced in scales of few meters or along the leaf lifespan should account for some of the intraspecific variation observed here.
Another reason why intraspecific variation of single traits may not be linked to environmental variation is the existence of structural constraints to variation, when one trait is not free to vary because it is structurally bounded to other traits. A single axis of trade-offs between leaf traits has been the dominant idea regarding trait coordination (Wright et al., 2004). More recently, one study has shown that the same traits can be either coupled or decoupled in the same species, depending on the environmental conditions (Ramırez-Valiente et al., 2020). Much of the controversy about trait covariation arises from the confusion between trait coordination or trait co-selection. Trait coordination arises from inevitable trade-offs between one trait and others. Trait co-selection, on the other hand, will be the result of two traits being selected simultaneously by environmental conditions. In our study, we show that a large part of trait covariation in palm leaves happens independently of the environmental variation, suggesting a major role of intrinsic trait coordination in trait variation patterns, but very little co-selection by environment.
A previous study (Muscarella and Uriarte, 2016) has provided empirical evidence that species are more likely to occur where some of their traits have values closer to the CWM, but other traits diverge from that; reinforcing the idea that selection may be operating over a multivariate trait space. We observed a community-level change in trait values along the hydrological gradient that was given mostly by the turnover of species, with little contribution of intraspecific changes. Several of the community-wide functional changes along the topo-hydrological gradient reflect the same pattern of acquisitive strategies associated to the wetter valleys switching to more conservative strategies toward the plateaus observed for trees in this same forest (Cosme et al., 2017), other Amazonian forests (Fontes et al., 2020; Schmitt et al., 2020), and other biomes (Cornwell and Ackerly, 2009). Together, our findings that even within a monophyletic lineage, shifts in trait–environment relationships are common suggest that trait–environment relationships at the community level are the result mainly of shifts in community composition and relative species abundances. This means that while CWM can be informative of the functional strategies in place for some traits, this is more likely to reflect the co-selection of traits along a common environmental gradient than single trait–environment relationships. Our results and proposition echo the findings of previous studies showing the decoupling between functional traits in tropical rain forests (e.g., Baraloto et al., 2010). Therefore, we need to be cautious when inferring ecological strategies from single traits without accounting for covariation of traits. Future work should evaluate phenotype performance along other gradients and traits.
Together our findings expand the current knowledge on trait variation to show that leaf traits can be widely variable within species, tightly coordinated to each other and at the same time, this variation can be independent from local environmental conditions. An additional practical implication of this finding is that sampling along hydrological gradients for those traits may be simplified as, at least in this situation, intraspecific variation is not driven by local hydrological gradients. Our study contributes to disentangle different sources of leaf variation by combining the study of trait variation and covariation in an environmentally and phylogenetically controlled framework. Although we found some evidence of phylogenetic conservatism in leaf traits, this is unlikely to be the main constraint to trait variation since phylogenetic signal is not related to the amount of intraspecific variance in this study. Alternatively, we observed large amounts (56–81%) of trait covariation that could be either generating or constraining interspecific variation on palm leaf traits, which requires further investigation. Finally, we conclude that although within-species variation in the examined traits is large, it does not contribute to the adjustment of individuals to the local hydrological conditions in this Amazonian forest. Therefore, species may need to migrate to follow their hydrological niches as climate changes. Species can also use other routes of adjustment and new traits, especially drought resistance traits, should be investigated.
Data Availability Statement
The original contributions presented in the study are included in the article/Supplementary Material, further inquiries can be directed to the corresponding author/s.
Author Contributions
TE and FC conceptualized the study. TE and HP collected the data under the supervision of FC. TE, FC, and HP analyzed the data. TE and FC drafted the manuscript with contributions from HP. All authors contributed to the article and approved the submitted version.
Funding
This study was supported by the CAPES Science Without Borders Project (078/2013) and CNPq Grants (441282/2016-4, 403764/2012-2, and 558244/2009-2) that funded the Central Amazon Long-Term Ecological Project, whose plots were used for this study. This study was financed in part by the Coordenação de Aperfeiçoamento de Pessoal de Nível Superior – Brasil (CAPES)—Finance Code 001 to TE.
Conflict of Interest
The authors declare that the research was conducted in the absence of any commercial or financial relationships that could be construed as a potential conflict of interest.
Publisher’s Note
All claims expressed in this article are solely those of the authors and do not necessarily represent those of their affiliated organizations, or those of the publisher, the editors and the reviewers. Any product that may be evaluated in this article, or claim that may be made by its manufacturer, is not guaranteed or endorsed by the publisher.
Acknowledgments
We thank Elisângela Rocha, Jackeline Milagros, Magno Plinck, and Talitha Ferreira for assistance during fieldwork and the Agroforestry Botany Laboratory of the Federal University of Amazonas where part of the sample processing was carried out. We are grateful to Maria Gracimar Pacheco for lab support and guidance on anatomical measurements. TE thanks Demetrius Martins for the valuable discussion on the analysis of trait covariation and Fabricio Baccaro for his comments on the early version of this manuscript.
Supplementary Material
The Supplementary Material for this article can be found online at: https://www.frontiersin.org/articles/10.3389/ffgc.2021.715266/full#supplementary-material
References
Albert, C. H., Grassein, F., Schurr, F. M., Vieilledent, G., and Violle, C. (2011). When and how should intraspecific variability be considered in trait-based plant ecology? Perspect. Plant Ecol. Evol. Syst. 13, 217–225. doi: 10.1016/j.ppees.2011.04.003
Armbruster, W. S., and Schwaegerle, K. E. (1996). Causes of covariation of phenotypic traits among populations. J. Evol. Biol. 9, 261–276. doi: 10.1046/j.1420-9101.1996.9030261.x
Baraloto, C., Timothy Paine, C. E., Poorter, L., Beauchene, J., Bonal, D., Domenach, A.-M., et al. (2010). Decoupled leaf and stem economics in rain forest trees. Ecol. Lett. 13, 1338–1347. doi: 10.1111/j.1461-0248.2010.01517.x
Bates, D., Mäechler, M., Bolker, B., and Walker, S. (2015). Fitting linear mixed-effects models using lme4. J. Statist. Softw. 67, 1–48. doi: 10.18637/jss.v067.i01
Blach-Overgaard, A., Svenning, J. C., Dransfield, J., Greve, M., and Balslev, H. (2010). Determinants of palm species distributions across Africa: the relative roles of climate, non-climatic environmental factors, and spatial constraints. Ecography 33, 380–391. doi: 10.1111/j.1600-0587.2010.06273.x
Blomberg, S. P., Garland, T., and Ives, A. R. (2003). Testing for phylogenetic signal in comparative data: behavioral traits are more labile. Evolution 57, 717–745. doi: 10.1111/j.0014-3820.2003.tb00285.x
Bolnick, D. I., Amarasekare, P., Araújo, M. S., Bürger, R., Levine, J. M., Novak, M., et al. (2011). Why intraspecific trait variation matters in community ecology. Trends Ecol. Evol. 26, 183–192. doi: 10.1016/j.tree.2011.01.009
Browne, L., and Karubian, J. (2018). Rare genotype advantage promotes survival and genetic diversity of a tropical palm. New Phytol. 218, 1658–1667. doi: 10.1111/nph.15107
Cássia-Silva, C., Freitas, C. G., Alves, D. M. C. C., Bacon, C. D., and Collevatti, R. G. (2019). Niche conservatism drives a global discrepancy in palm species richness between seasonally dry and moist habitats. Glob. Ecol. Biogeogr. 28, 814–825. doi: 10.1111/geb.12895
Cavender-Bares, J., Fontes, C. G., and Pinto-Ledezma, J. (2020). Open questions in understanding the adaptive significance of plant functional trait variation within a single lineage. New Phytol. 227, 659–663. doi: 10.1111/nph.16652
Chauvel, A., Lucas, Y., and Boulet, R. (1987). On the genesis of the soil mantle of the region of Manaus, Central Amazonia, Brazil. Experientia 43, 234–241. doi: 10.1007/BF01945546
Cornwell, W. K., and Ackerly, D. D. (2009). Community assembly and shifts in plant trait distributions across an environmental gradient in coastal California. Ecol. Monogr. 79, 109–126. doi: 10.1890/07-1134.1
Cosme, L. H. M., Schietti, J., Costa, F. R. C., and Oliveira, R. S. (2017). The importance of hydraulic architecture to the distribution patterns of trees in a central Amazonian forest. New Phytol. 215, 113–125. doi: 10.1111/nph.14508
Costa, F. V., Costa, F. R., Magnusson, W. E., Franklin, E., Zuanon, J., Cintra, R., et al. (2015). Synthesis of the first 10 years of long-term ecological research in Amazonian Forest ecosystem-implications for conservation and management. Natur. Conserv. 13, 3–14. doi: 10.1016/j.ncon.2015.03.002
Costa, F. R. C., Guillaumet, J.-L., Lima, A. P., and Pereira, O. S. (2009). Gradients within gradients: the mesoscale distribution patterns of palms in a central amazonian forest. J. Veg. Sci. 20, 69–78. doi: 10.1111/j.1654-1103.2009.05314.x
Costa, F. R. C., Zuanon, J. A. S., Baccaro, F. B., de Almeida, J. S., Menger, J., Souza, J. L. P., et al. (2020). Effects of climate change on central amazonian forests: a two decades synthesis of monitoring tropical biodiversity. Oecologia Aust. 24, 317–335. doi: 10.4257/oeco.2020.2402.07
Cuartas, L. A., Tomasella, J., Nobre, A. D., Nobre, C. A., Hodnett, M. G., Waterloo, M. J., et al. (2012). Distributed hydrological modeling of a micro-scale rainforest watershed in Amazonia: Model evaluation and advances in calibration using the new HAND terrain model. J. Hydrol. 46, 15–27. doi: 10.1016/j.jhydrol.2011.12.047
de Boer, H. J., Price, C. A., Wagner-Cremer, F., Dekker, S. C., Franks, P. J., and Veneklaas, E. J. (2016). Optimal allocation of leaf epidermal area for gas exchange. New Phytol. 210, 1219–1228. doi: 10.1111/nph.13929
Dong, N., Prentice, I. C., Wright, I. J., Evans, B. J., Togashi, H. F., Caddy-Retalic, S., et al. (2020). Components of leaf-trait variation along environmental gradients. New Phytol. 228, 82–94. doi: 10.1111/nph.16558Dransfield
Dransfield, J., Uhl, N. W., Asmussen, C. B., Baker, W. J., Harley, M. M., and Lewis, C. E. (2008). GENERA PALMARUM: The Evolution and Classification of Palms. Kew: Kew Publishing, 732.
Eiserhardt, W. L., Svenning, J. C., Kissling, W. D., and Balslev, H. (2011). Geographical ecology of the palms (Arecaceae): determinants of diversity and distributions across spatial scales. Ann. Bot. 108, 1391–1416. doi: 10.1093/aob/mcr146
Emilio, T., Lamarque, L. J., Torres-Ruiz, J. M., King, A., Charrier, G., Burlett, R., et al. (2019). Embolism resistance in petioles and leaflets of palms. Ann. Bot. 124, 1173–1184. doi: 10.1093/aob/mcz104
Epskamp, S., Schmittmann, V. D., and Borsboom, D. (2012). qgraph: network visualizations of relationships in psychometric data. J. Stat. Softw. 48, 1–18.
Esquivel-Muelbert, A., Brienen, R. J. W., Baker, T. R., Dexter, K. G., Lewis, S. L., Feldpausch, T. R., et al. (2019). Compositional response of Amazon forests to climate change. Glob. Chang. Biol. 25, 39–56. doi: 10.1111/gcb.14413
Estebán, E. J. L. V., Castilho, C., Melgaço, K. L., and Costa, F. R. C. (2021). The other side of droughts: wet extremes and topography as buffers of drought negative effects in an Amazonian forest. New Phytol. 229, 1995–2006. doi: 10.1111/nph.17005
Fajardo, A., and Siefert, A. (2018). Intraspecific trait variation and the leaf economics spectrum across resource gradients and levels of organization. Ecology 99, 1024–1030. doi: 10.1002/ecy.2194
Fan, Y., Miguez-Macho, G., Jobbágy, E. G., Jackson, R. B., and Otero-Casal, C. (2017). Hydrologic regulation of plant rooting depth. Proc. Natl. Acad. Sci. U.S.A. 114, 10572–10577. doi: 10.1073/pnas.1712381114
Faurby, S., Eiserhardt, W. L., Baker, W. J., and Svenning, J. (2016). Molecular phylogenetics and evolution an all-evidence species-level supertree for the palms (Arecaceae). Mol. Phylogenet. Evol. 100, 57–69. doi: 10.1016/j.ympev.2016.03.002
Fontes, C. G., Fine, P. V. A., Wittmann, F., Bittencourt, P. R. L., Piedade, M. T. F., Higuchi, N., et al. (2020). Convergent evolution of tree hydraulic traits in Amazonian habitats: implications for community assemblage and vulnerability to drought. New Phytol. 228, 106–120. doi: 10.1111/nph.16675
Fyllas, N. M., Michelaki, C., Galanidis, A., Evangelou, E., Zaragoza-castells, J., Dimitrakopoulos, P. G., et al. (2020). Functional trait variation among and within species and plant functional types in mountainous Mediterranean forests. Front. Plant Sci. 11:212. doi: 10.3389/fpls.2020.00212
Givnish, T. J. (1987). Comparative studies of leaf form: assessing the relative roles of selective pressures and phylogenetic constraints. New Phytol. 106, 131–160.
Göldel, B., Kissling, W. D., and Svenning, J. C. (2015). Geographical variation and environmental correlates of functional trait distributions in palms (Arecaceae) across the New World. Bot. J. Linn. Soc. 179, 602–617. doi: 10.1111/boj.12349
Gomes, L. R. P., Lopes, M. T. G., Bentes, J. L., da, S., Barros, W. S., Costa Neto, P., et al. (2011). Genetic diversity in natural populations of Buriti (Mauritia flexuosa L. f.). Crop Breed. Appl. Biotechnol. 11, 216–223. doi: 10.1590/s1984-70332011000300003
Harrison, X. A., Donaldson, L., Correa-Cano, M. E., Evans, J., Fisher, D. N., Goodwin, C. E. D., et al. (2018). A brief introduction to mixed effects modelling and multi-model inference in ecology. PeerJ 6:e4794. doi: 10.7717/peerj.4794
Hodnett, M. G., Vendrame, I., de, O., Marques Filho, A., Oyama, M. D., and Tomasella, J. (1997). Soil water storage and groundwater behaviour in a catenary sequence beneath forest in central Amazonia: I. Comparisons between plateau, slope and valley floor. Hydrol. Earth Syst. Sci. 1, 265–277. doi: 10.5194/hess-1-265-1997
Jung, V., Violle, C., Mondy, C., Hoffmann, L., and Muller, S. (2010). Intraspecific variability and trait-based community assembly. J. Ecol. 98, 1134–1140. doi: 10.1111/j.1365-2745.2010.01687.x
Kelly, R., Healy, K., Anand, M., Baudraz, M. E., Bahn, M., Cerabolini, B. E., et al. (2021). Climatic and evolutionary contexts are required to infer plant life history strategies from functional traits at a global scale. Ecol. Lett. 24, 970–983. doi: 10.1111/ele.13704
Kichenin, E., Wardle, D. A., Peltzer, D. A., Morse, C. W., and Freschet, G. T. (2013). Contrasting effects of plant inter- and intraspecific variation on community-level trait measures along an environmental gradient. Funct. Ecol. 27, 1254–1261. doi: 10.1111/1365-2435.12116
Kraus, J. E., and Arduin, M. (1997). Manual Básico de Métodos em Morfologia Vegetal, Vol. 1. Rio de Janeiro: Edur, 198.
Liu, Q., Sterck, F. J., Zhang, J. L., Scheire, A., Konings, E., Cao, M., et al. (2021). Traits, strategies, and niches of liana species in a tropical seasonal rainforest. Oecologia 196, 499–514. doi: 10.1007/s00442-021-04937-4
Luizão, R. C. C., Luizão, F. J., Paiva, R. Q., Monteiro, T. F., Sousa, L. S., and Kruijt, B. (2004). Variation of carbon and nitrogen cycling processes along a topographic gradient in a central Amazonian forest. Glob. Chang. Biol. 10, 592–600. doi: 10.1111/j.1529-8817.2003.00757.x
Luo, Y. H., Liu, J., Tan, S. L., Cadotte, M. W., Wang, Y. H., Xu, K., et al. (2016). Trait-based community assembly along an elevational gradient in subalpine forests: quantifying the roles of environmental factors in inter- and intraspecific variability. PLoS One 11:e0155749. doi: 10.1371/journal.pone.0155749
Ma, R.-Y., Zhang, J. L., Cavaleri, M. A., Sterck, F., Strijk, J. S., and Cao, K. F. (2015). Convergent evolution towards high net carbon gain efficiency contributes to the shade tolerance of palms (Arecaceae). PLoS One 10:e0140384. doi: 10.1371/journal.pone.0140384
Magnusson, W. E., Lima, A. P., Luizao, R., Costa, F. R. C., de Castilho, C. V., and Kinupp, V. F. (2005). RAPELD: a modification of the Gentry method for biodiversity surveys in long-term ecological research. Biota Neotrop. 5:bn01005022005.
Marenco, R. A., Camargo, M. A. B., Antezana-Vera, S. A., and Oliveira, M. F. (2017). Leaf trait plasticity in six forest tree species of central Amazonia. Photosynthetica 55, 679–688. doi: 10.1007/s11099-017-0703-6
Marks, C. O. (2007). The causes of variation in tree seedling traits: The roles of environmental selection versus chance. Evolution 61, 455–469. doi: 10.1111/j.1742-4658.2007.00021.x
Marks, C. O., and Lechowicz, M. J. (2006). Alternative designs and the evolution of functional diversity. Am. Nat. 167, 55–66. doi: 10.1086/498276
Melo, W. A., Freitas, C. G., and Bacon, C. D. (2018). The road to evolutionary success: insights from the demographic history of an Amazonian palm. Heredity 121, 183–195. doi: 10.1038/s41437-018-0074-1
Messier, J., McGill, B. J., and Lechowicz, M. J. (2010). How do traits vary across ecological scales? A case for trait-based ecology. Ecol. Lett. 13, 838–848. doi: 10.1111/j.1461-0248.2010.01476.x
Münkemüller, T., Lavergne, S., Bzeznik, B., Dray, S., Jombart, T., Schiffers, K., et al. (2012). How to measure and test phylogenetic signal. Methods Ecol. Evol. 3, 743–756. doi: 10.1111/j.2041-210X.2012.00196.x
Muscarella, R., and Uriarte, M. (2016). Do community-weighted mean functional traits reflect optimal strategies? Proc. R. Soc. B 283:20152434. doi: 10.1098/rspb.2015.2434
Nakagawa, S., and Schielzeth, H. (2010). Repeatability for Gaussian and non-Gaussian data: A practical guide for biologists. Biol. Rev. 85, 935–956. doi: 10.1111/j.1469-185X.2010.00141.x
Nicotra, A. B., Atkin, O. K., Bonser, S. P., Davidson, A. M., Finnegan, E. J., Mathesius, U., et al. (2010). Plant phenotypic plasticity in a changing climate. Trends Plant Sci. 15, 684–692. doi: 10.1016/j.tplants.2010.09.008
Oliveira, R. S., Costa, F. R. C., Van Baalen, E., de Jonge, A., Bittencourt, P. R., Almanza, Y., et al. (2019). Embolism resistance drives the distribution of Amazonian rainforest tree species along hydro-topographic gradients. New Phytol. 221, 1457–1465. doi: 10.1111/nph.15463
Osnas, J. L. D., Katabuchi, M., Kitajima, K., Wright, S. J., Reich, P. B., Van Bael, S. A., et al. (2018). Divergent drivers of leaf trait variation within species, among species, and among functional groups. Proc. Natl. Acad. Sci. U.S.A. 115, 5480–5485. doi: 10.1073/pnas.180398911
Parent, C., Capelli, N., Berger, A., Crèvecoeur, M., and Dat, J. F. (2008). An overview of plant responses to soil waterlogging. Plant Stress 2, 20–27.
Perez-Harguindeguy, N., Díaz, S., Garnier, E., Lavorel, S., Poorter, H., Jaureguiberry, P., et al. (2013). New Handbook for standardized measurment of plant functional traits worldwide. Aust. J. Bot. 61, 167–234. doi: 10.1071/BT12225
Price, C. A. (2012). “LEAF GUI: Analyzing the geometry of veins and areoles using image segmentation algorithms,” in High-Troughput Phenotyping in Plants. Methods in Molecular Biology (Methods and Protocols), Vol. 918, ed. J. Normanly (Totowa, NJ: Humana Press).
Ramırez-Valiente, J. A., López, R., Hipp, A. L., and Aranda, I. (2020). Correlated evolution of morphology, gas exchange, growth rates and hydraulics as a response to precipitation and temperature regimes in oaks (Quercus). New Phytol. 227, 794–809. doi: 10.1111/nph.16320
Rasband, W. S. (1997–2008). ImageJ. National Institutes of Health, Bethesda, Maryland, MA, United States. Available online at: https://imagej.nih.gov/ij/
Renninger, H. J., and Phillips, N. (2011). Hydraulic properties of fronds from palms of varying height and habitat. Oecologia 167, 925–935. doi: 10.1007/s00442-011-2038-5
Rennó, C. D., Nobre, A. D., Cuartas, L. A., Soares, J. V., Hodnett, M. G., Tomasella, J., et al. (2008). HAND, a new terrain descriptor using SRTM-DEM: mapping terra-firme rainforest environments in Amazonia. Remote Sens. Environ. 112, 3469–3481. doi: 10.1016/j.rse.2008.03.018
Reznick, D. N., and Ghalambor, C. K. (2001). “The population ecology of contemporary adaptations: what empirical studies reveal about the conditions that promote adaptive evolution,” in Microevolution Rate, Pattern, Process. Contemporary Issues in Genetics and Evolution, Vol. 8, eds A. P. Hendry and M. T. Kinnison (Dordrecht: Springer). doi: 10.1007/978-94-010-0585-2_12
Rodrigues, F. R. O., and Costa, F. R. C. (2012). Litter as a filter of emergence for herbaceous seedlings and sporophytes in central Amazonia. J. Trop. Ecol. 28, 445–452. doi: 10.1017/S0266467412000491
Ruokolainen, K., and Vormisto, J. (2000). The most widespread Amazonian palms tend to be tall and habitat generalists. Basic Appl. Ecol. 1, 97–108. doi: 10.1078/1439-1791-00020
Schietti, J., Emilio, T., Rennó, C. D., Drucker, D. P., Costa, F. R. C., Nogueira, A., et al. (2014). Vertical distance from drainage drives floristic composition changes in an Amazonian rainforest. Plant Ecol. Divers 7, 241–253. doi: 10.1080/17550874.2013.783642
Schmitt, S., Hérault, B., Ducouret, É, Baranger, A., Tysklind, N., Heuertz, M., et al. (2020). Topography consistently drives intra- and inter-specific leaf trait variation within tree species complexes in a Neotropical forest. Oikos 129, 1521–1530. doi: 10.1111/oik.07488
Siefert, A., Fridley, J. D., and Ritchie, M. E. (2014). Community functional responses to soil and climate at multiple spatial scales: when does intraspecific variation matter? PLoS One 9:e111189. doi: 10.1371/journal.pone.0111189
Siefert, A., Violle, C., Chalmandrier, L., Albert, C. H., Taudiere, A., Fajardo, A., et al. (2015). A global meta-analysis of the relative extent of intraspecific trait variation in plant communities. Ecol. Lett. 18, 1406–1419. doi: 10.1111/ele.12508
Silvertown, J., Araya, Y., and Gowing, D. (2015). Hydrological niches in terrestrial plant communities: a review. J. Ecol. 103, 93–108. doi: 10.1111/1365-2745.12332
Silvertown, J., Dodd, M. E., Gowing, D. J. G., and Mountford, J. O. (1999). Hydrologically defined niches reveal a basis for species richness in plant communities. Nature 400, 61–63.
Snijders, T. A., and Bosker, R. J. (2011). Multilevel Analysis: An Introduction to Basic and Advanced Multilevel Modeling. London: Sage, 354.
Svenning, J.-C. (2001). On the role of microenvironmental heterogeneity in the ecology and diversification of neotropical rain-forest palms (Arecaceae). Bot. Rev. 67, 1–53.
Tomlinson, P. B. (2006). The uniqueness of palms. Botan. J. Linn. Soc. 151, 5–14. doi: 10.1111/j.1095-8339.2006.00520.x
Violle, C., Enquist, B. J., McGill, B. J., Jiang, L., Albert, C. H., Hulshof, C., et al. (2012). The return of the variance: intraspecific variability in community ecology. Trends Ecol. Evol. 27, 244–252. doi: 10.1016/j.tree.2011.11.014
Violle, C., Navas, M. L., Vile, D., Kazakou, E., Fortunel, C., Hummel, I., et al. (2007). Let the concept of trait be functional! Oikos 116, 882–892. doi: 10.1111/j.2007.0030-1299.15559.x
Vormisto, J., Tuomisto, H., and Oksanen, J. (2004). Palm distribution patterns in Amazonian rainforests: What is the role of topographic variation? J. Veg. Sci. 15:485.
Keywords: Amazon forest, climate change, hydrological niche, trait covariation, phylogenetic conservatism, community weighted mean, Arecaceae
Citation: Emilio T, Pereira H Jr and Costa FRC (2021) Intraspecific Variation on Palm Leaf Traits of Co-occurring Species—Does Local Hydrology Play a Role? Front. For. Glob. Change 4:715266. doi: 10.3389/ffgc.2021.715266
Received: 26 May 2021; Accepted: 14 July 2021;
Published: 01 September 2021.
Edited by:
Nick Smith, Texas Tech University, United StatesReviewed by:
Kelsey R. Carter, Los Alamos National Laboratory, United StatesEric J. Ward, United States Geological Survey (USGS), United States
Copyright © 2021 Emilio, Pereira and Costa. This is an open-access article distributed under the terms of the Creative Commons Attribution License (CC BY). The use, distribution or reproduction in other forums is permitted, provided the original author(s) and the copyright owner(s) are credited and that the original publication in this journal is cited, in accordance with accepted academic practice. No use, distribution or reproduction is permitted which does not comply with these terms.
*Correspondence: Thaise Emilio, dGhhaXNlLmVtaWxpb0BnbWFpbC5jb20=; Flávia R. C. Costa, ZmxhdmlhY29zdGEwMDFAZ21haWwuY29t