- 1Wildlife and Terrestrial Ecosystems, USDA Rocky Mountain Research Station, Missoula, MT, United States
- 2Wildlife and Terrestrial Ecosystems, USDA Rocky Mountain Research Station, Flagstaff, AZ, United States
- 3Forest and Woodland Ecosystems, USDA Rocky Mountain Research Station, Moscow, ID, United States
- 4Air Water and Aquatic Environments, USDA Rocky Mountain Research Station, Boise, ID, United States
- 5Maintaining Resilient Dryland Ecosystems, USDA Rocky Mountain Research Station, Moscow, ID, United States
- 6Wildlife and Terrestrial Ecosystems, USDA Rocky Mountain Research Station, Bozeman, MT, United States
- 7Forest and Woodland Ecosystems, USDA Rocky Mountain Research Station, Fort Collins, CO, United States
- 8Forest and Woodland Ecosystems, USDA Rocky Mountain Research Station, Flagstaff, AZ, United States
- 9Human Dimensions, USDA Rocky Mountain Research Station, Fort Collins, CO, United States
Wildland research, management, and policy in western democracies have long relied on concepts of equilibrium: succession, sustained yield, stable age or species compositions, fire return intervals, and historical range of variability critically depend on equilibrium assumptions. Not surprisingly, these largely static concepts form the basis for societal expectations, dominant management paradigms, and environmental legislation. Knowledge generation has also assumed high levels of stasis, concentrating on correlational patterns with the expectation that these patterns would be reliably transferrable. Changes in climate, the introduction of large numbers of exotic organisms, and anthropogenic land conversion are leading to unprecedented changes in disturbance regimes and landscape composition. Importantly, these changes are largely non-reversable; once introduced exotic species are seldom eradicated, climates will continue to warm for the foreseeable future, and many types of land conversion cannot be easily undone. Due to their effects on extant infrastructure and expectations for ecosystem services, these changes are, and will be, viewed by western societies as overwhelmingly negative. The continued acceleration of change will generate increasingly novel systems for which the transferability of correlational relationships will prove unreliable. Our abilities to predict system trajectories will therefore necessarily decrease. In this environment, top-down, expert dominated approaches to environmental decision making are unlikely to produce results that meet broader societal expectations. To be successful we need to embrace a more inclusive paradigm of collaborative governance and multiple forms of knowledge for adapting to constant change, including indigenous epistemological systems. By increasing public and stakeholder participation, we can encourage collaborative social learning allowing all parties to more fully understand the complexities and tradeoffs associated with wildland management and the technical limits of models that seek to quantify those tradeoffs. System novelty will necessarily make forecasting more dependent on predictive modeling and will require better models. Data collection should therefore be strongly influenced by model input requirements and validation; research will need to focus on fundamental and causal relationships to a much greater degree than is done currently.
The Case for Change—The Challenges
Causes and Magnitude of Change
Wildlands are changing rapidly and many of these changes are irreversible (See Supplementary Table 1 for examples). The primary drivers of these changes include: (1) climate, which is in a state of rapid change that will continue into the indefinite future (Wuebbles et al., 2017), (2) the introduction of invasive organisms that forever change the components of ecosystems, and (3) land uses, which, at the extreme, entirely replace extant ecosystems with novel anthropogenic constructs. In many cases these drivers work in concert to produce altered disturbance regimes, species assemblages, structural characteristics, and ecosystems. Recently, the rate of change in wildlands has greatly accelerated; change agents such as fire, bark beetles, and invasive species over the last three decades have killed/altered vast areas of forests (e.g., Dale et al., 2001; Meddens et al., 2012) and rangelands (Bradley and Mustard, 2008), diminishing the flow of ecosystem goods and services. While these changes are occurring world-wide, we will focus our discussion on landscapes in North America, and on wildlands in the western United States.
Across wildlands in western North America, and around the globe, disturbances are occurring at rates that are unprecedented in modern times (e.g., Allen et al., 2010; Jolly et al., 2015). In North America, changes associated with disturbances are often extensive in their scope, magnitude and duration and ecological change is occurring across both forested and non-forested ecosystems. For example, recent tree mortality across the southern Sierra Nevada in California, USA has been widespread. Nearly half (48.9%) of all trees died between 2014 and 2017 (Fettig et al., 2019) with significantly higher levels of tree mortality occurring in the low elevations compared to the high elevations. These observed changes support model predictions that 18% of trailing-edge forests are at risk for conversion to non-forests by mid-century (Parks et al., 2019). Even in forest types that have produced reliable regeneration in the past may fail to regenerate in the face of multiple year droughts and stand-replacing wildfires (Figure 1). Sagebrush (Artemisia spp.) ecosystems, occupying large portions of the western USA and supporting numerous obligate species, are also in decline. Loss of sagebrush is a result of wildfire, followed by replacement by invasive plants (e.g., cheatgrass, Bromus tectorum; Coates et al., 2016). This vegetative transformation further reinforces high fire frequency return interval because cheatgrass adds dry, fine fuels (Brooks et al., 2004; Bradley et al., 2018).
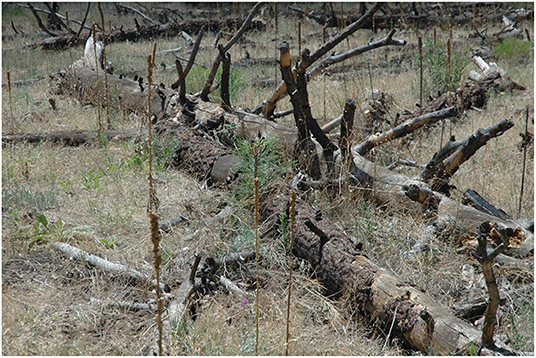
Figure 1. Climate related changes in Southwest forests. Southwestern ponderosa pine (Pinus ponderosa) forests provide an example of the irreversible ecological changes occurring and the interface between these changes and societal expectations. These forests, already stressed by a multi-year drought, are increasingly at risk of uncharacteristic crown fires. Longer, hotter, and dryer summers in recent decades have led to the largest recorded fires in Arizona and New Mexico. Several recent fires have created large (tens to 100's of hectares) patches of high severity fire where 100% of the trees are killed. Following these severe fires, droughty conditions and lack of seed source may preclude ponderosa pine regeneration, especially on some soil types (Puhlick et al., 2012). These post-fire patches may become grassy meadows with low densities of pine seedlings, well below numbers thought to have occurred historically (Owen et al., 2017). Patches of disturbance-adapted non-native species, some of which are highly invasive may become established, replacing native understory plant species (Sabo et al., 2009). Alternatively, more productive areas may develop into dense shrub fields (Savage and Mast, 2005). Evidence increasingly suggests that the combination of high severity fire and subsequent drought can lead to pine regeneration failures (Savage et al., 2013). Public land managers, driven by societal desires to see pine forests return as well as laws and regulations requiring replanting, feel pressure to replant pine seedlings in burned areas. Yet, the reality is that even repeated replanting may fail to restore pine forests in some areas. Replanting success can be increased to some degree by choosing sites where seedlings are more likely to survive, and data from scientific studies and monitoring, as well as modeling can help guide these efforts. However, in some locations and especially after multiple wildfires, the odds of pine seedlings regenerating and surviving are small (e.g., Guiterman et al., 2018) leading to transitions to shrub or grass dominated communities. Acceptance of this type of ecosystem transition may be difficult to accept and only through discussions and monitoring activities can diverse stakeholders become more in tuned to these changes.
These disturbances create widespread and enduring consequences for ecosystems, economies, and communities near and far from wildlands. Local communities bear the brunt of risks to property and people from wildfires and tree mortality (e.g., Camp Wildfire, 2018, California). These communities also feel the effects of dislocated investment in manufacturing infrastructure as wood supply and quality become more variable and uncertain (Alfaro et al., 1982; Gray and MacKinnon, 2006; Loeffler and Anderson, 2018). Wildfire smoke, reduced wildland recreational opportunities and quality, and decreasing flows of goods like wood, fish, wildlife, and clean water from wild areas affect more distant communities and urban centers (e.g., May et al., 2018). The result has been various calls from publics, politicians, professionals, and scientists for “restoration” of wildlands to ecosystem conditions more attuned to the historical range of variability or variation (HRV) (Morgan et al., 1994). These calls for action seek to reset the current conditions to reflect HRV with the hope that the resulting landscapes will be more resilient and resistant to the forces affecting them and return the flow of goods and services in and from the wildlands that were historically received. The question is, can we achieve ecological restoration to historical conditions? Given that changes in climate will not be reversed in the foreseeable future, most exotic species cannot be removed from ecosystems, and landscapes will not return to their pre-Columbian conditions, the answer is no. A more plausible and achievable goal for restoration would be to develop policies and management that shift ecological trajectories to more desirable conditions likely to produce desired ecological goods and services. “How can we achieve these trajectory shifts?” The answer to this question will have varying degrees of nuance depending on the ecosystem and the degree of degradation, but it must be recognized that, while achievable, this paradigm shift requires the restructuring of expectations, laws, wildland governance, data collection approaches, and research goals.
The Societal Expectation of Stasis and Its Effects on Institutions
The idea of fundamentally static ecosystems is baked into many of the societal, cultural, and intellectual beliefs of modern western democracies. We accept ideas such as cyclic phenomena as well as periods of growth and decline, but these periodic events were traditionally seen to occur within a larger stable equilibrium. Droughts might happen, but the rains would come again. Fires might burn, but forests would regrow. In areas pertaining to wildland dynamics, many examples can be found in the traditional paradigms for both ecology and land management that explicitly or implicitly assume static background conditions, or stationarity. The idea of succession, particularly in its original Clemensian incarnation, specifically assumed that a “climax” community was stable (Clements, 1916). Even in its later versions, by scientists such as Daubenmire (1968), the assumption was that you could reliably infer future successional processes by examining current conditions—an idea that implicitly assumed that both the available species pool and abiotic conditions would be constant. Many other ideas common to traditional forestry also carry the implicit idea of stationarity: fire return period, site index, and both maximum sustained yield and the use of linear optimization to assess it (Curtis, 1962). In ecology, fundamental theories such as MacArthur-Wilson's island biogeography (MacArthur and Wilson, 1967) and Levins' (1969, 1970) metapopulation models are dynamic equilibrium models; like Clemensian succession, these models explicitly assume that a stationary equilibrium exists around which the dynamics of disturbance and recovery occur (Pickett and White, 1985; Gunderson and Holling, 2002).
Not only did these static concepts dominate our scientific thinking for decades, but our approaches to wildland management have historically been rooted in a largely static world view, and these static assumptions have been codified in policy and practice through environmental and natural resource management legislation (Craig, 2010). In the United States, the Sustained Yield Forest Management Act (1944; 16 U. S. C. 583) was passed “To promote sustained-yield forest management in order thereby (a) to stabilize communities, forest industries, employment, and taxable forest wealth; (b) to assure a continuous and ample supply of forest products; and (c) to secure the benefits of forests in regulation of water supply and stream flow, prevention of soil erosion, amelioration of climate, and preservation of wildlife.[italics added for emphasis]” In 1960, the Federal Government passed the Multiple Use-Sustained Yield Act (1960; 16 U.S.C. 528; MUSY) which expanded the 1944 Act to include recreation, livestock grazing, and wildlife and fish habitat, but with the same sustainability goals. Both Acts were clearly grounded in a static world view. MUSY planning documents, for example, assumed both sustained forest conditions across periods in excess of 100 years and used constrained linear optimization to estimate sustainable levels of resource extraction (Kent, 1980). While the MUSY was specifically directed toward the Forest Service, all U. S. land management agencies struggled with the concepts of multiple use. To create a more coherent framework for multiple use management, both the Forest Service and Bureau of Land Management—who collectively manage over 1.78 MM km2, primarily in western USA, adopted a new paradigm of “Ecosystem Management” to achieve multiple-use management.
Ecosystem Management brought two important changes. First, it brought explicit recognition of a broad range of values derived from managed lands including intangible services (e.g., spiritual, historical, and symbolic). The second important change involved the practice of resource management (Ludwig, 2001). Instead of top down, rule-based management (Armitage et al., 2012; Koontz et al., 2015), agencies adopted an approach capable of confronting landscape-scale problems with flexibility so that it could be responsive to complex, unpredictable feedbacks between social and ecological components (Chaffin et al., 2014).
While highly flexible and potentially dynamic in concept, Ecosystem Management provided little guidance for how these nebulous concepts should be operationalized. To provide necessary targets, goals, and desired future conditions, HRV was used as a reference forest condition to understand ecosystem dynamics (Morgan et al., 1994). This allowed land managers and scientists to quantify ecosystem changes based on historical norms and identify the range of possible ecosystem conditions that guided management strategies. By framing the nebulous concepts associated with ecosystem management in a way that allowed formulation of measurable and quantifiable management goals, HRV provided land management agencies with a working solution to the vastly expanded list of multiple uses associated with ecosystem management. However, adopting HRV as a management paradigm again rested on the basic static assumption that we can use past patterns (e.g., probability distributions) and processes as direct quantitative guides for current and future patterns and rates of disturbance. Thus, while ecosystem management is not intrinsically static (see Millar et al., 2007), its implementation can clearly trace its roots to the 1944 Sustained-Yield Forest Management Act and arguably was even more strongly static: the number of things that were expected to be sustained increased, and variability in land condition was formally linked to the past.
These patterns or expectations within the scientific and management literature parallel a more general societal desire for and expectation of stasis. Many laws and statutes assume that static conditions can be maintained. In addition to MUSY, mentioned earlier, wildlands are managed under a series of laws, all of which assume that stasis both can be achieved and should be the overarching goal of conservative land management. The Knutson-Vandenberg Act (1930; 16 U.S.C. 576) assumes that areas that were forest can remain forest, the Endangered Species Act (ESA; 1973; 16 U.S.C. 1531, note) assumes that endangered species can be preserved in the areas they currently exist or historically existed, and the National Forest Management Act (1976; 16 U.S.C. 1600, note) assumes that current natural biological diversity can be retained. Doremus (2010) argues that the ESA explicitly embodies static concepts concerning species, their ranges and habitats, and conservation approaches to protect them.
The desire to view the landscape and climate as being predictable and largely static emerges from psychological and economic needs that drive critical decisions throughout society: decisions to build businesses or infrastructure or to strongly associate one's identity with a place carry with them the strong expectation that conditions will remain largely static into the future. Obviously, a fly shop assumes a trout stream, a ski area assumes snow, and a lumber mill assumes trees. But these static assumptions drive far deeper and more prevalent societal norms and decisions: what kind of houses we build and where we build them and what infrastructure we build to sustain critical needs such as drinking and irrigation water all carry with them strong expectations of a reliable and knowable future, generally linked to historical patterns. Policies supporting the protection of wilderness and national parks, for example, have long been guided by a nature preservation mandate; these preserves satisfy a human desire for relative stasis of natural areas when compared to more rapidly shifting human constructs (Cole and Yung, 2010; Purdy, 2015). We look to wildlands as places we can revisit where, perhaps, you can take your grandchild fishing on a lake you fished in your youth–with the expectation that fundamental aspects of those land areas, and therefore the activities that occurred there will be reliably present. Due to the economic and psychological burdens they impose, fundamental, and irreversible changes such as those associated with a shifting climate, large and severe disturbances, or exotic species invasions, will, in western societies, therefore overwhelmingly be viewed as negative.
The call for broad-scale forest restoration must be understood in the context of these generalized societal expectations and legal requirements for both stasis and stability in wildlands. However, for many of the processes that affect land management, rates of change have accelerated to the point where equilibrium concepts and the related guidance provided by historical antecedents or conditions no longer provide reliable examples of probable trajectories or future states. This is not just a challenge for scientists and experts, it forms a critical challenge for social and political systems. For fundamental and unavoidable reasons, we need to critically rethink both what our expectations for wildlands might be, and much of the underlying decision process associated with the management of these lands.
Loss of Predictive Capability and Its Effects on the Status of Expertise
As systems change irreversibly, becoming increasingly chaotic and novel, we need to recognize that there is an accompanying loss of predictability concerning these ecosystems. How ecosystems function, what they can produce, and what their trajectories into the future might be, will become less predictable based on extant knowledge. Much of our ecological knowledge is based on historical observations and correlational relationships. While we have some understanding of general processes in community ecology that apply regardless of the biological players or environmental context (e.g., Hubbell, 2001; Rosindell et al., 2012), specific ecosystem behaviors are primarily based on empirical observations (Real and Levin, 1991). Although often couched in a language of being “process based,” the modeled understandings of system behavior often contain many statistically based descriptions or narratives (e.g., Peel and Blöschl, 2011) that break down when new ecosystem elements are present (e.g., introduced species, Figure 2) or environmental conditions change (e.g., climate, soils, and organismal communities; Figure 3).
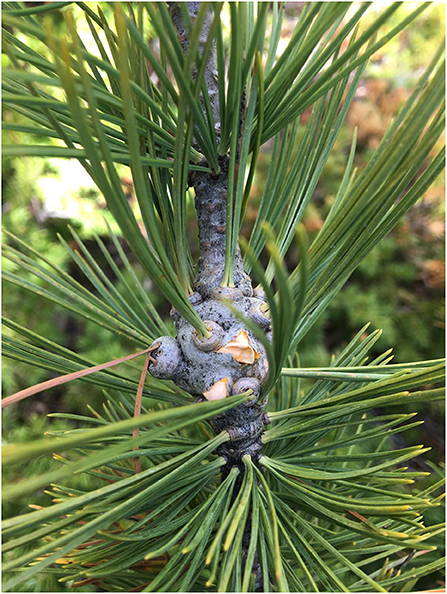
Figure 2. Invasive disease: white pine blister rust. White pine blister rust (WPBR), a lethal forest disease caused by the fungus of Asian origin Cronartium ribicola, has had widespread impacts on North American five-needle pines. Introduced to the Americas in approximately 1900, the disease has led to permanent changes in ecosystems that contain susceptible pine species. These effects range from forest industry collapse to species endangerment to threatening wilderness character (e.g., Tomback et al., 2001; Fins et al., 2002). Management to restore impacted pine populations and proactive interventions to mitigate the development impacts and promote adaptive capacity in threatened ecosystems are underway (e.g., Keane et al., 2012; Schoettle et al., 2019a). Combining statistical and mechanistic relationships into models has integrated field monitoring data with specific biological requirements of the pathogen, host and disease to reduce uncertainty about disease risk, development, and impacts (e.g., Kearns et al., 2014). Sensitivity analyses have further highlighted key factors affecting host population sustainability (Field et al., 2012; Landguth et al., 2017). The arrangement of impacts on the landscape and conditional probabilities of WPBR infection can affect the public's opinion and willingness-to-pay for management and the optimal timing and arrangement of management treatments (e.g., Shanahan et al., 2020). As these models become more complex and applicable at the landscape spatial scale and management's temporal scale, they will be important tools for exploring when, where, and how to conduct management interventions for the greatest likelihood of successfully sustaining the pine populations in the persistent presence of the pathogen and meeting societal expectations for these mountain top ecosystems (Schoettle et al., 2019b).
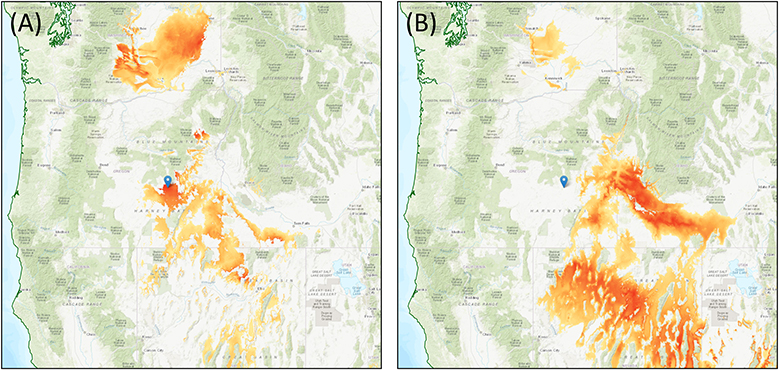
Figure 3. Coupled climate, exotic species, and anthropogenic land change in sagebrush ecosystems. Big sagebrush (Artemisia tridentata) is a widespread and foundational species of the cold deserts in western North America. Sagebrush ecosystems in the warmest regions have been degraded or eliminated due to a combination of increased disturbance from wildfire or human activities, followed by invasion of an exotic annual grass, cheatgrass (Bromus tectorum). Studies have shown that sagebrush in the warmest and driest regions exhibit low resistance and resilience to disturbance and landscapes are frequently transformed to cheatgrass dominated ecosystems (Chambers et al., 2007, 2014). Climatic niche modeling of cheatgrass (Bradley et al., 2016), big sagebrush (Still and Richardson, 2015), and plant community biomes (Rehfeldt et al., 2012), as well as trends vegetative cover (Shi et al., 2018) suggest that the low resilience and resistance of sagebrush in these areas are linked to climate; transformation to cheatgrass in these warmer regions is likely not only caused by increased disturbance, but confounded by a shifting climate. For vulnerable areas, efforts to restore sagebrush could be futile due to abundance of cheatgrass coupled with disturbance regimes and unfavorable climatic regimes for sagebrush establishment. Ultimately, investing in the research that can aid in decision support can pay dividends by focusing restoration efforts where they would be most effective. For example, a decision support tool, the Climate Smart Restoration Tool (CSRT), is being developed that integrate correlative, climatic niche models, and mechanistic trait-based seed transfer models (Richardson and Chaney, 2018). The CSRT maps seed transfer limits based on the genetic adaptation to climates. Managers can develop maps for current and potential future climates using models that reflect differing scenarios of green house gas emissions. Seed transfer limits for a big sagebrush site in southeast Oregon, USA. Map (A) shows seed transfer areas for a contemporary climate (1981–2010) and map (B) shows mid-century climates (2041–2070). The colored gradient, with increasing climatic similarity from yellow to red, shows areas where seed collections would be suitable for the restoration site (blue pin). Note by mid-century local seed sources are no longer suitable and seed must be obtained from warmer, lower elevation sites (B).
With greater uncertainty about the sustainability and trajectories of ecosystems, we can expect less accurate predictions and, therefore, an inability to provide expected stability in the provision of environmental goods and services. Unfortunately, deviations from these expected goals are, and will be, often viewed or framed as failures of management and the science behind it. Because of the loss of information associated with both the unknown and highly stochastic nature of future ecological trajectories, expertise based on past observations will become less valuable because it is less reliable. That is, past observations about how a system behaves may have limited applicability in the presence of a new suite of stressors such as exotic species or severe drought. For projecting future land conditions and therefore current management direction, in a formal sense, the expert has less expertise to offer. Furthermore, when the experts start pointing out the unsustainability of the historical landscapes and traditional outputs that many people so strongly desire, further tension between the managing experts and diverse publics can be expected. Though western culture cultivates a certain ongoing political distrust in institutions (Freemuth, 2018), recent decades show a deepening distrust in expertise and growing anti-institutional sentiment, particularly for scientifically based institutions (Freemuth and Cawley, 1998; Wilkinson, 1998; Fischer, 2000; Sarewitz, 2004; Pittinsky, 2015). Given that land management institutions often operate from an underlying foundation of authority in professional knowledge and expertise (e.g., Hays, 1959; Williams and Matheny, 1995; Fischer, 2000), the loss of knowledge represents a serious erosion of latitude in decision making.
Expecting this trend to continue is reasonable and likely to accelerate due to the increasing novelty of ecosystems and unpredictability of change. As Sarewitz (2004) argues, science is regularly and unavoidably politicized (e.g., climate change, genetically modified foods, and nuclear waste disposal) when addressing environmental problems marked by high levels of complexity and uncertainty. In these situations, science offers different constituencies competing models and facts that take time to adjudicate scientifically. Even with these problems, however, strategies eschewing expertise and scientific principles would leave us societally ill equipped for a world in which irreversible change is both ubiquitous and occurring with extraordinary velocity. Therefore, we need to accept the legitimacy of the current disappointment in scientists' and managers' abilities to effectively address rapid undesirable changes while recognizing that an approach not couched in logic and data is even more likely to fail.
Here we argue that flexibility in how we organize to address problems associated with rapid change could substantially advance our capacity to efficiently achieve more desirable and relatively durable ecosystem services. While the role of expertise, logic, and data must still be critical to management solutions, the role of expertise must change, shifting from determining decisions to a more consultative role, recognizing the contribution of expertise to accelerate learning in a collaborative context. Experts have been historically valued for knowing the answer; we will be relying on them more and more to help society in finding an answer. There will be a transition from a reliance on accrued knowledge and toward the need to learn quickly and reliably and to adapt to new knowledge. The decision process needs to be both more rapid and more complex, with greater reliance on contingent decisions, requiring feedback about outcomes and progress of management activities and the ecosystems they modify. To support this, a high level of expertise is required to design efficient systems for information gathering and analysis. A consequence of this shift is a more integrated partnership between researchers, managers, and stakeholders because the need for learning is a vulnerability that is shared between managers, stakeholders, and the experts that support them.
A key attribute of this collaborative management paradigm involves the frequent updating of questions, a process that is not entirely technical; the choices of which uncertainties are most important often depend on values. Researchers, managers, and stakeholders alike will need to share in the understanding of how information collection and model designs are likely to affect the values most important to them. This may be particularly important with respect to resources and values prioritized by indigenous groups, whose knowledge and system understanding is underrepresented in existing published literature on ecology or changing climate and, consequently, in climate change assessments (Vinyeta and Lynn, 2013; Roesch-McNally et al., 2020). Below, we expand on these two coupled strategies: the democratization of decision processes and a shift in expertise to provide efficient decision support.
Moving Forward
Environmental Governance to Confront Complexity and Uncertainty
The idea that science-based management could be left primarily to a cadre of professionals with minimal input from society never really served us well (Thomas and Burchfield, 1999), as all management options are fraught with uncertainty, have significant potential for failure, and contain value choices (Funtowicz and Ravetz, 1993). Further, as Freemuth and Cawley (1998) pointed out in their criticism of Ecosystem Management, the prioritization of protecting ecosystem process over the choices of ecosystem services to be provided represented an imposition of professional and scientific values.
Lacking status quo options, managing wildlands in a directionally changing world will require even greater need for decisions laden with value preferences concerning future services provided by the landscape (see e.g., Rieman et al., 2010). There is, however, wisdom in structuring decision spaces where (1) citizens, stakeholders, and experts can co-produce a factual accounting of biophysical process and hazards as guidance to publics, and (2) those aspects of land management that require value judgements are democratized: the democratization of management requires everyone to assume the risks associated with uncertain outcomes.
Management of novel and chaotic systems in which predictions are often neither simple nor well-established requires a fundamental rethinking of how science should inform conservation as well as how we think about management itself, including the regulatory environment and who participates and how. Democratic governance is neither particularly nimble nor farsighted in doing the kind of adaptive, collaborative anticipatory governance required to address high levels of complexity and uncertainty. But some version of “anticipatory governance”—a flexible decision framework that uses a wide range of possible futures to prepare for change and to guide current decisions toward maximizing future alternatives or minimizing future threats (Quay, 2010, p. 496)—will need to be developed in relation to the production of laws, practices, and institutions to address the emerging novelty of systems. Collaboration and co-production will not work if they lack support of entities that consider unpredictability as a norm and use of scenarios as a planning tool.
There are two facets to this rethinking. One is to learn how to more astutely acquire and apply science, knowledge, and information about landscape change in ways that are attuned to the political realities and challenges posed by chaotic and novel environments. The other involves the broad challenge of directing these complex social-ecological systems toward socially desirable goals when many facts are uncertain and the values at stake are politically contested. Within the social sciences these situations are called “wicked problems” (Weber et al., 2017) and require more inclusive and collaborative governance approaches and management practices to better accommodate these complexities and uncertainties and not be stymied by them.
A Different Governance Model: Toward Adaptive, Collaborative Governance
The governance paradigm we suggest is distinct from the 20th century management paradigm in how it addresses both facts and values underlying public decision making. In conservation practice, governance models emphasize dialog as a means for advancing social learning (engaging diverse sources of knowledge) and for transforming individual preferences or values (Williams and Matheny, 1995; Williams, 2017). In contrast, the managerial paradigm was built on the view that experts could define and discover the public interest using technical means such as soliciting opinion through polls and other mechanisms. The necessary facts could be discovered using the tools of science and individual preferences and values could be quantified, aggregated and rendered into rational public choices using the tools of economics and decision science. Too often, this approach has led to governance that is short-term and reactionary rather than anticipatory. In this new paradigm, consistent with knowledge co-production and social learning, collaborative governance seeks to transform individual preferences through dialog (e.g., social learning) rather than attempting to simply aggregate the pre-existing preferences of individual stakeholders to decide which policy choice is most valued or beneficial to society. The view of wildland governance as a democratic and dialogic process assumes multi-stakeholder collaboration in decision making. In this paradigm, differences in knowledge among stakeholders are opportunities for shared learning that can improve public decisions rather than being a source of conflict to be adjudicated by experts (Young, 1996).
We emphasize that this collaborative model does not overcome the inherent challenges of making management decisions in a highly uncertain environment. Rather, it advances the idea of creating a learning community that collectively takes ownership of decisions and their consequences in such a way that promotes shared adaptive learning. Perhaps more importantly, the act of involvement, in and of itself, increases the likelihood that errors and management failures are more likely to be viewed as learning opportunities to improve public reasoning. Similarly, by helping stakeholders become more familiar with the value, limitations, and costs associated with generating better information, they are more likely to own and trust that information.
The key to dealing with wicked problems is to use the governance paradigm to broaden the participating stakeholders and organize them into place-based stakeholder networks that create potential learning communities: groups who meet regularly to learn about both the systems they seek to manage and about each other's needs and values (Collins, 2014). Identification and promotion of learning communities within and across multi-scale conservation initiatives helps to facilitate the documentation and integration of different kinds and sources of knowledge, competencies, and values (Jedd and Bixler, 2015). Collaborative, place-based social learning promotes co-production of knowledge among all stakeholders, mitigates power imbalances and access to knowledge, and empowers stakeholders to leverage their various partial understandings of the situation in the service of improved public decision making for adaptively managing complex social-ecological systems (Williams, 2018). Importantly, indigenous and local knowledge systems as well as practitioners' knowledge can provide valid and useful knowledge to enhance our understanding of wildlands governance (Teng et al., 2014). There is, therefore, a great need to develop functioning mechanisms for legitimate, transparent, and constructive ways of creating synergies across knowledge systems (Tengö et al., 2017), thus enabling inclusion of local indigenous knowledge in coproduction and collaboration efforts.
In sum, with the erosion of trust and reliace on expertise based on past observations and institutions for land management (Pittinsky, 2015), society faces a new age in which experts, stakeholders, and everyday citizens alike share the burdens and responsibilities of planning, choosing, and discovery under the complex and uncertain conditions noted above. This understanding has, over recent decades, resulted in the emergence of and experimentation with adaptive, collaborative forms of governance for sustainable conservation (Folke et al., 2005; Ostrom, 2010; Armitage et al., 2012; See also Examples of co-production of science, below). We need to encourage and learn from these experiments.
The Co-production of Science
The democratization of governance extends to the collection and dissemination of information as well as the mechanics of policy decision making. Historically, scientists have generally viewed societal resistance to the acceptance of scientifically generated knowledge as being associated with either problems in communication or in science literacy (e.g., Bickford et al., 2012). However, it is necessary to recognize that science is frequently rejected because it threatens peoples' core beliefs or worldviews and thus makes it unlikely that communication alone can produce greater acceptance of science (Haidt, 2012; Lewandowsky and Oberauer, 2016). In particular, the literature on public understanding of science suggests that increased scientific literacy often increases the polarization of opinions along partisan lines (e.g., Nyhan et al., 2014; Nyhan and Reifler, 2015; but see Guess and Coppock, 2020). To overcome this, democratized governance will require much more stakeholder participation in the production and application of science to inform specific decisions. The focus will need to be on enhancing the consideration of science through engaging more inclusive networks of scientists, stakeholders, and citizens in the co-production of knowledge (Jasanoff, 2004; Wyborn, 2015). Co-production requires a kind of shared, social learning at a systems or collective level where multiple stakeholders work together to understand and ultimately transform their collective knowledge and practice (Collins, 2014, p. 238). Importantly, co-production involves the inclusion of the divergent epistemological contexts associated with indigenous cultures that use separate and more flexible paradigms for knowledge generation through systemic understandings of complex environments, integrating a large number of variables qualitatively over extended periods of time (Mistry and Berardi, 2016).
Changing Priorities for Research in Rapidly Changing Ecosystems
To confront ecological change and to function within the new governance paradigm described above, scientists and subject matter experts have to change how they do science: what they study, how they study it, and how to incorporate diverse kinds and sources of knowledge that defy simple integration into a unifying model (Miller et al., 2008; Mitchell, 2009; Allen and Hoekstra, 2015). Nowhere is this more challenging than how scientists apply models to complex problems. Because ecosystem sciences can only give a partial description of the system using any one model (e.g., Allen and Hoekstra, 2015), “we need many models covering different viewpoints” (Jorgensen and Muller, 2000, p. 12–13). Consequently, both to function within the more participatory governance model, above, and to collect the necessary data effectively, will require changes in how data are collected, organized, and evaluated. Unavoidably and paradoxically, our dependence on predictive models will increase, but they will be used less directly for decisions. Lastly, even with science co-production and high levels of community involvement, building and analyzing models is unavoidably arcane and full of compromises; models require simplifications and choices such as which functional forms to fit. These choices and departures from reality require both understanding and acceptance by those involved in wildlands governance.
Increased Reliance on Predictive Models
There are two broad categories of ecological models: statistical and mechanistic. The application of statistical models in forestry and land management is common and grounded in the expectation of stasis in both the ecological community and the abiotic background conditions, assumptions that can be checked, but only rarely are (e.g., Lute and Luce, 2017). Formally, the statistical inferences are only valid within the sampled population; extrapolation to other times and places is a non-statistical exercise that relies on stasis across space and time. Lacking this stasis, the patterns of the past are not likely to predict the future. Furthermore, variable inclusion and coefficient values in statistical models are chosen by rules of parsimony and information content (Burnham and Anderson, 2004) and may have tenuous relationships to the underlying biological drivers. As such, using covariate relationships in statistical models to focus future research may not lead to improved predictive capability under changing conditions.
In contrast to statistical models, mechanistic models use reasoning based on first principles in biology and geophysics to estimate variation through time in response to weather, disturbance, and management intervention. The ideal mechanistic model requires minimal if any calibration or training through collection of observed data to reliably predict patterns or outcomes because calibration can hide implicit correlations. Ideally, then, parameter values in mechanistic models are determined through independent measurement. Such models are conceptually more reliable in non-stationary conditions. In ecology, however, predictive models are generally complex, typically involving different facets and diverse scientific disciplines. In many cases, only a subset of these complexities has the necessary information available for precise modeling. This, in turn, leads to the inclusion of many untested assumptions and, not surprisingly, dependence on ecological mechanistic models has been fraught with difficulties. In most cases it is not possible to state the degree of confidence in results because modeling assumptions make validation of model components difficult to impossible (IPCC, 2013, Chapter 9, Pfister and Kirchner, 2017). Consequently, mechanistic models are usually used to examine the dynamical dependencies of ecosystems on characteristics or parameters through sensitivity and transient analyses. For example, researchers may search for emergent behaviors such as expectations of community shift or instability under varying climate, after disturbance, or as new species or diseases are introduced (e.g., Ezard et al., 2010; Tavener et al., 2011; Field et al., 2012).
Research on the emergent properties and behaviors of larger integrated ecosystems under the current and future environments will continue to be a primary application of mechanistic models, one that can provide the essential interchange between models, experimental research, environmental monitoring, and adaptive learning, but greater precision and accuracy will be required. Despite the challenges involved, a shift from a correlative to a mechanistic understanding of ecosystem behavior (and therefore delivery of ecosystem services) is essential for reliable prediction under an array of potential futures and environmental scenarios. More robust models of this nature can be a basis for design of adaptive management and learning strategies.
Central to this more mechanistic approach to forecasting is the collection of appropriate data and development of targeted research to inform specific process understandings. Predictive models, such as those discussed above, require data for identification of parameter values and produce forecasts that require assessment. Ideally, predictive models provide frameworks for adaptive learning: model forecasting skill is controlled both by the quality of the mechanistic understandings that form the model structures and the data used to calibrate the models. Each projection therefore provides an opportunity to revisit mechanisms and assess the sensitivity of model results to specific data inputs. This adaptive process logically channels data collection into areas that either improve model calibration or directly test model-based hypotheses, providing a focus for research and data acquisition.
A focus on mechanistic models and targeted experimental studies does not preclude involvement of stakeholders and the public in the learning process. Large participatory modeling efforts have had success (e.g., such as those organized by International Institute for Applied Systems Analysis, IIASA; https://iiasa.ac.at/) and similar principles can be adapted to more narrowly focused models (e.g., Walters, 1997). Stakeholders provide information to frame research priorities and provide potential hypotheses (Roesch-McNally et al., 2020) while research can target experiments to explore and define specific isolated processes as well as assess more integrated ecosystem responses. Both can be executed at multiple spatial (molecules to landscapes and beyond) and temporal (immediate to evolutionary) scales and in collaboration among science-management-stakeholder partnerships (see co-production discussion above).
We need to carefully re-think the mix of local and regional data collected to reflect the accelerated rates of change, the needs for model parameterization and validation, and the necessary involvement of citizen science. Short-term and local data collections are well-suited for testing treatment efficacy, as well as model parameterization and validation. Their role will likely increase; but, because their results cannot directly infer treatment success across space and time, these results need to be formally contextualized within larger and longer-term studies and their results applied to other circumstances through models. Crowd-sourced, long-term data collection efforts are examples of the more participatory approach to knowledge acquisition that we advocate. Related to an increased reliance on citizen scientists is the need to make all resulting datasets freely available. A good example of this approach is the eDNAtlas project that engages minimally trained citizens to collect extensive data that are analyzed and developed into an interactive spatial database of species hosted on a public internet site (Jerde et al., 2011; Carim et al., 2016; Young et al., 2018).
Vulnerability Assessments, Decision Support Tools and Data Syntheses
The above research efforts can feed into the development of vulnerability assessments for specific conservation concerns. For example, models have been useful in (1) developing silvicultural prescriptions to conserve Northern Goshawk (Accipiter gentilis) habitat by enhancing resilience of dry-forest types in the Southwest (Figure 4), (2) identifying suitable nesting areas for woodpecker (Picadae) species of conservation concern, and proposing post-fire treatments for the persistence of these species and their nesting habitats (Figure 5), (3) developing web-based decision support tools for restoration of sagebrush ecosystems (Richardson and Chaney, 2018, Figure 3), and (4) assessing the risk of the establishment of white pine blister rust (caused by the non-native fungal pathogen Cronartium ribicola) in high elevation five-needle pines forests and predicting population trajectories under different ecological conditions and management scenarios (Figure 2). These tools and others evaluate how ecosystem changes to biotic and abiotic components influence the realized species niches (sensu Hutchinson, 1957). Vulnerability assessments can also apply to physical and social systems (Pacifici et al., 2015; Runting et al., 2017).
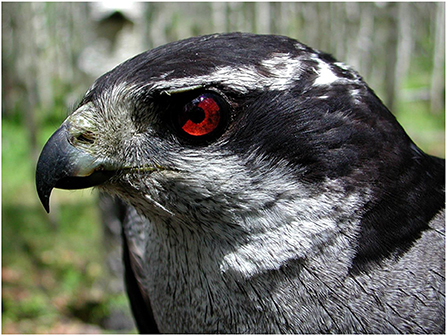
Figure 4. Short term solutions: Managing goshawk habitat through forest resilience. The northern goshawk (Accipiter gentilis) is forest/woodland raptor with a circumpolar distribution (Squires and Kennedy, 2006). Typically associated with older, mature forests, the goshawk is a species of conservation concern throughout its North American range. Over the past century, forest-management practices have resulted in dense, fire-prone forests which lack structure needed by goshawks and are at risk of stand-replacing fire events (Reynolds et al., 1992). Conservation of goshawks requires forest physiognomy and composition to meet the life requisites of the goshawks as well as those of its primary prey species. To ensure that conditions persist through time, forests must be resilient to primary stressors—fire and climate change—that could compromise forest integrity and reduce goshawk habitat. Reynolds et al. (1992) used a synthetic approach to define the habitat needs of the goshawk in the American southwest. They assembled information on habitats of key prey species (birds, squirrels, and rabbits) and the goshawk, as well as knowledge of ponderosa pine (Pinus ponderosa) forest ecology. Forest ecology included composition and structure, tree longevity, disturbance factors, and landscape patterns and scale. As pieces were assembled, they converged on a forest structure that closely resembled that existing prior to European settlement of the southwest. In general terms, the structure was characterized by a matrix of groups and clumps of trees interspersed among grassy openings. Tree groups were comprised of like-sized trees representing various structural stages, from seedlings to large trees >60 cm dbh. Historical fire regimes in these pine forests were typically low-severity surface fires occurring every 2–12 years (Cooper, 1961; White, 1985). Within-group structure and landscape patterns required disturbance (fire primarily) to regulate tree establishment and maintain landscape patterns (Moir et al., 1997). Reynolds et al. (2013) contended that restoration of key elements of these forests—species composition, tree groups, grass-forb-shrub interspaces, snags, logs, and woody debris, and landscape dynamics—would improve the resilience of frequent-fire forests and facilitate ecosystem processes. Restoration would first require combinations of mechanical treatments and prescribed fire to achieve the desired conditions and then a return of natural fire regimes to maintain them through time. A critical assumption of the approach is that historical forests persisted through wide range of environmental conditions, thus they would continue to persist given anticipated changes brought by future climate change. However, as discussed in Figure 1, the recent occurrence of large high severity fires in this region is curtailing pine survival, and sometimes converting pine forests into non-forest types. Photo credit: RMRS goshawk studies.
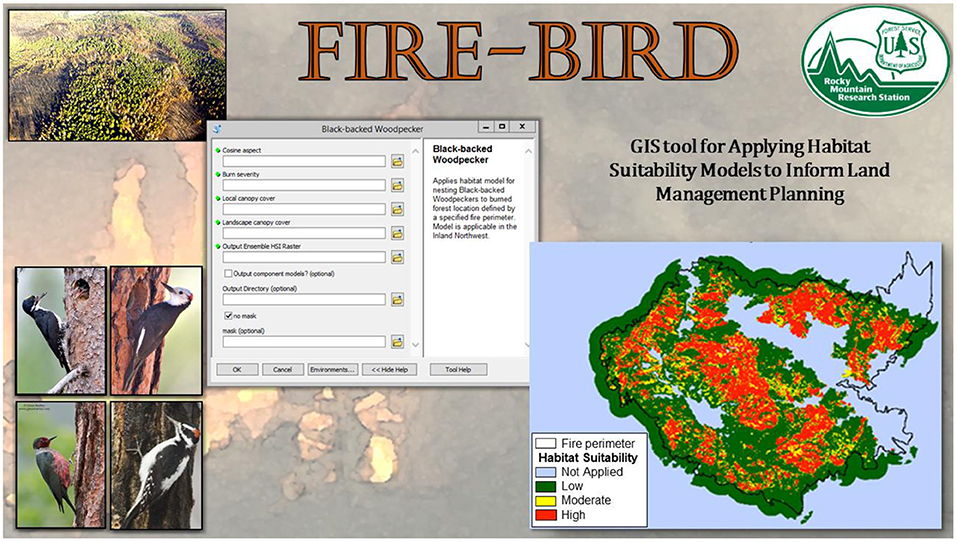
Figure 5. Optimizing ecological services in recently burned forests. Removal of burned or beetle-killed trees through salvage logging can provide economic value to local communities and reduce snag hazards. However, removal of snags following recent wildfire or beetle outbreaks has negative ecological consequences, including reduction of habitat for disturbance-associated wildlife species. Consequently, forest managers are challenged with implementing post-disturbance management projects while concurrently meeting the requirements of existing laws and planning directives to maintain wildlife habitat for disturbance-associated species. Salvage logging is often litigated over concerns regarding negative effects on aquatic and terrestrial ecosystems, and on wildlife associated with recent disturbance (e.g., Lindenmayer and Noss, 2006; Saab et al., 2009; Hutto et al., 2016). Several woodpecker species of conservation concern (SCC; black-backed woodpecker [Picoides arcticus], white-headed woodpecker [Dryobates albolarvatus], Lewis's woodpecker [Melanerpes lewisii]), and American three-toed woodpecker [Picoides dorsalis] are strongly associated with recently burned or beetle-killed forests because forest openings and snags provide critical nesting, perching, and foraging resources. Habitat suitability index (HSI) models for these woodpeckers were developed from nest location and associated environmental variables (Saab et al., 2011, 2019; Latif et al., 2013, 2015, 2020) to map habitat for wildlife. A GIS decision-support tool (FIRE-BIRD) provides habitat suitability maps that inform treatment designs to maximize habitat suitability and minimize negative effects to woodpecker SCC for a single fire or proposed management activity, while accounting for overall habitat in a surrounding national forest (Latif et al., 2018). This information allows maximization of ecosystem services by allowing areas to be harvested with minimal impacts on wildlife.
While the co-production of knowledge, and its dissemination in user-friendly and publicly available spatial databases may help confront the mistrust of scientific processes, the sophistication of current data analyses and the necessary dependence on often complex mechanistic models to project future scenarios leads to a suite of additional challenges that will need to be addressed. As noted above, co-production of science will be key in enhancing science-policy interface because it emphasizes more inclusive networks of scientists, stakeholders, and citizens in the co-production of knowledge (Jasanoff, 2004; Wyborn, 2015). But co-produced data will require analysis, often complex and arcane analyses, and these analyses will be embedded into even more complex and arcane models. Therefore, it is critical that these analyses are not decoupled from the collection of data: those who collect data have to understand why the data they are collecting is important, and at a conceptual level how it will be analyzed and used. Those engaged in analysis need to understand who is collecting the data, what they want to know, and how to effectively communicate both model results and uncertainties. From the start of co-production, the importance of the analysis needs to be emphasized and explained, and at the end of the process, the data collector should be able to locate the data they collected and understand how their data contributed to the analytic conclusions.
Examples of Co-production of Science Approaches
U.S. Congress passed the Forest Landscape Restoration Act of 2009, establishing the Collaborative Forest Landscape Restoration Program (CFLRP; Figure 6). The goals and approaches defined in this act are consistent with many of the governance tenants we outlined above. The Act is intended to “encourage the collaborative, science-based ecosystem restoration of priority forest landscapes through a process that encourages ecological, economic, and social sustainability …” (www.fs.fed.us/restoration/CFLRP). Funds are awarded competitively to large (≥50,000 acres) and long-term (>10 years with 15 years of monitoring) projects. Proposals are developed cooperatively by the Forest Service and stakeholders and conducted primarily on National Forest System lands. The emphasis of CFLRP collaboratives is to develop a shared vision of desired conditions, including social, economic, and ecological attributes of a landscape that management strives to achieve (e.g., reduced risk of uncharacteristically severe fires, improved wildlife habitat, maintenance of large old trees, enhanced use of timber resources). Science legitimacy of the process is gained through science engagement and by involving respected experts to interpret the research, while maintaining independence from the decision-making process (Urgenson et al., 2017). CFLRP projects are required to explain how existing or proposed infrastructure is used to process restoration byproducts in a manner that supports jobs and local economies. The legislation expects that fire suppression costs will decrease over time as a result of CFLRP projects, because of increased efficiency and capacity of both industry and local US Forest Service units (Schultz et al., 2012). Unlike legislation in the past that emphasized fuels reduction (e.g., 2002 Healthy Forests Restoration Act [68 FR 33814]), CFLRP characterizes fire as both a risk to be managed and a natural process to be restored, with a focus on a larger-scale approaches to address fire issues (Schultz et al., 2019).
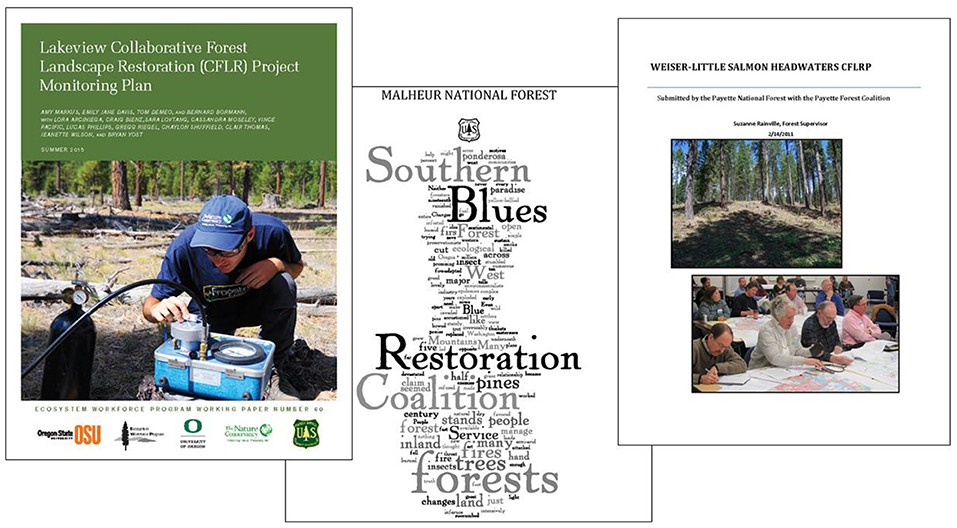
Figure 6. Collaborative wildland governance through CLFRP. The focus of CFLRP collaboratives is developing a shared concept of desired conditions, including social, economic, and ecological attributes of a landscape that management strives to achieve (e.g., reduced risk of severe fires, improved wildlife habitat, maintenance of large old trees). Science legitimacy of the process is attained by engaging scientists and respected experts to interpret the research, while maintaining independence from the decision-making process (Urgenson et al., 2017). Research conducted on woodpecker populations of the Interior Northwestern U.S. is an example of targeted experimental research in conjunction with the required monitoring for CFLRPs. The research has been conducted for the duration (8–10 years) of CFLRPs on three National Forests (Payette, Fremont-Winema, and Malheur National Forests) across two Forest Service Regions (4 and 6) in dry mixed coniferous forests. Collaborative stakeholders comprise local community organizations, state governments, and federal agencies, all with an interest in public land management. Each collaborative invited scientists from state and federal agencies, and universities to participate in developing and implementing monitoring plans for focal plant and animal species within the CFLRP project areas. In the case of these 3 CFLRPs, all chose to include monitoring of woodpecker populations in relation to forest management activities (See Figure 5). The required monitoring allowed rigorous experimental design (Before, After, Control, Impact [BACI]) to determine effectiveness of silvicultural, prescribed fire, and post-wildfire management activities to meet desired conditions and wildlife habitat goals. The research conducted as part of the required monitoring on these CFLRPs, and on projects supported by similar collaboratives, has resulted in a GIS decision-support tool for land management planning (see Figure 5; Latif et al., 2018).
The Adaptive Silviculture for Climate Change (ASCC) program is another example of an application of the science-management partnerships approach (Nagel et al., 2017). The ASCC project is designed to work with resource management realities and national forest decision frameworks while generating critically needed research. The intention of the program is to create a network of installations across the United States using a common experimental design, fully replicated within each site, allowing for both intra- and inter-site comparisons of various adaptive management approaches. The infrastructure opens opportunities for hypothesis-driven experimental research nested within management scenarios and provides for long-term engagement among the partners for continued learning.
These two programs (CFLRP and ASCC) represent a paradigm shift in National Forest policy and in developing successful adaptive learning approaches to help guide management in the future (Schultz et al., 2012; Urgenson et al., 2017). Combined with the modeling and targeted experimental research efforts discussed above they provide further opportunities to explore the co-production of long-term research in conjunction with the required monitoring (e.g., Latif et al., 2015, 2020; Saab et al., 2019). These programs make significant moves toward including diverse participation in (1) identifying and setting the direction of information needs to support decision making as well as (2) involving stakeholders in the execution of the work and syntheses. Although this approach is in its infancy, it is one that integrates the governance and research paradigm shifts that are needed to address the novel challenges we now face.
Actionable Recommendations
We offer both short-term and longer-term actionable recommendations with the goal of pivoting toward addressing the uncertainties associated with rapidly changing ecosystems. Our short-term actionable recommendations in part reiterate those given by others (e.g., Millar et al., 2007):
• Conduct landscape level assessments that identify potential vulnerabilities of key ecosystem components to various disturbances to assess the multiple alternative trajectories that can result following disturbances and how disturbance frequency and severity influence the resiliency of landscapes.
• Enhance adaptive capacity on a landscape scale by keeping as many pieces as possible and maintaining as many ecosystem services as possible. Approaches would include sustaining native species diversity, functional diversity, above- and below-ground food webs, reducing soil loss, and maintaining water quality.
• Emphasize management that creates landscape mosaics of plant composition and structure with a focus on retaining disturbance-resilient and genetically-adapted vegetation.
Beyond these generalized approaches to understanding disturbance effects, creating or maintaining disturbance resistant landscapes, and keeping as many future options open as possible, we recommend:
• Change laws and planning processes to reflect the unknown nature of future landscapes. Continued demands for stasis are unlikely to succeed. Enlarging the social ecological decision space is especially critical but requires more inclusive co-production of knowledge.
• Change governance systems for wildlands to be both more democratic and participatory. This means that working through both knowledge and value disputes in a specific landscape must be accomplished through social learning processes that require from society a sense of common bond or shared fate that allows participants to interact in a civil way (Kemmis, 1990; Haidt, 2012). Social, political, and intellectual diversity are necessary in collaborative processes that aim to produce good public policy.
• Change systems of knowledge acquisition to increase public participation in the cogeneration of knowledge. Quantities of necessary information will demand this, and ownership in the generation of new knowledge is essential to its acceptance. Within this, specifically consider traditional ecological knowledge systems that may shed light on paleoecological process in ways not represented in modern ecological literature (e.g., Mason et al., 2012; Vinyeta and Lynn, 2013).
• Structure knowledge acquisition into a formal adaptive framework with models providing the primary tools for information integration. A large proportion of data collection will be specifically designed to test and parameterize extant models and construct new ones.
• Shift away from largely correlational studies that weakly infer process by studying pattern to direct studies of processes. Correlational patterns are not expected to endure except in very narrow spatiotemporal windows.
Discussion
In a rapidly changing planet, many events will be beyond our control. We recognize that wildlands are on novel trajectories that are largely unknown. For example, we did not anticipate the ecological changes that resulted from excessive tree mortality in the Southern Sierra, Nevada (Fettig et al., 2019), aspen die-off in Colorado (Worrall et al., 2008), or the West Nile Virus (Strausbaugh et al., 2001). Surprises that lead to major ecological shifts such as forests being replaced by shrublands or grasslands will become more common in the future. However, the changes in governance, research focus, data collection, and planning processes advocated here are fully achievable and should help us anticipate, react to, and to some extent modify the consequences of those events that we cannot reliably predict and control.
We have a fundamental societal need to seek approaches for slowing or smoothing environmental changes (e.g., flattening the curve) both to maximize usable ecological services to give society and institutions time to adapt. In the very short term, we can use generic risk spreading approaches such as we advocate in Actionable Recommendations, above. However, we need to recognize that greater changes in how we manage wildlands are necessary and that we need to begin these changes today so that they are operational soon. We recognize that our proposed model involves the expansion of democratic processes beyond existing governmental systems to embrace much broader application of collaboration and adaptive social learning among experts and stakeholders. But we really have no choice. We need to work collectively and democratically to aggregate the necessary information, determine our roles in shaping future landscape trajectories, and define and optimize the flow of desired goods and services from these rapidly changing ecosystems. We are all in this together and our natural resource governance needs to reflect this.
From a governance perspective, the major challenge for implementing our actionable recommendations is that the current social norms and formal planning rules for how society resolves policy and management conflicts are poorly suited to the “social learning” model we have proposed. Social learning is hardly a panacea, requiring stakeholders (managers, experts, and citizens) to transcend their pre-existing ideological inclinations and prepare to learn from one another. This more open mindset requires stakeholders to hold enough of a shared commitment to work and learn together to craft tentative solutions and to be open to new information and learning. Practice and a changed mindset are needed to make successful a more participatory, collaborative, and adaptive approach. There are, however, examples of more collaborative governance. At smaller scales we have examples such as the CFLRP (above; Figure 6). At larger scales examples would include the National Cohesive Wildland Fire Management Strategy (Jewell and Vilsack, 2014) which is designed to work collaboratively among all stakeholders across all landscapes where the vision is to “safely and effectively extinguish fire when needed; use fire where allowable; manage our natural resources; and as a nation, to live with wildland fire.” Fire, and the smoke it creates, transcends ownership and political boundaries and directly affects the lives of everyone living in proximity to fire-prone landscapes. Because everyone has some sort of stake in outcomes, fire management is an ideal opportunity to explore more collaborative governance (Jewell and Vilsack, 2014).
In addition to changes in governance, we need to shift our approaches to generate knowledge. Importantly, we will be more directly dependent on models, and it is therefore more essential that models work. For example, statistical and mechanistic modeling have been used to better understand WPBR disease risk and hazard and to gain knowledge essential for projecting pine population dynamics into the future (Figure 1). Similarly, climate niche modeling of cheatgrass, big sagebrush, and plant communities suggest that climate influences the resilience and resistance of sagebrush, both identifying the problem and leading to a more focused approach of data collection and research (Figure 3). A synthetic approach to define the habitat needs that links disturbance dynamics to forest structure and key food webs (e.g., sustaining habitats of goshawks and dry coniferous forests, Figure 4) provides both short-term guidance and a framework for data collection and knowledge generation to improve future outcomes. Integrating modeled wildlife habitat requirements into spatial timber harvest models allows joint optimization of habitat retention while and timber harvest (Figure 5).
Model-based approaches to wildland management, however, need to be nested within a formal adaptive framework because most ecosystems and societies are changing rapidly. As new challenges arise and societal needs change, processes of synthesis, modeling, and targeted data-collection need to be consistently and continuously applied and updated. This will hypothetically help identify shifts and changes in trajectories and develop new management directions. This, in turn, will require the close coordination between scientists, managers, and the broad public that will engage both in deciding management direction and data collection. Successfully stewarding novel ecosystems requires a paradigmatic change to our most basic approaches to land management. We cannot find that change by looking backward to restore landscapes to their former Holocene condition, but by looking forward to making sense of emergent novelty, complexity, and uncertainty using an adaptive governance paradigm suited to the Anthropocene.
Author Contributions
KM contributed to the general conceptualization of management given irreversible change and took the lead in writing the document. WB provided text and concepts associated with wildlife management. TJ provided concepts and text associated with forested systems, exotic pathogens, historical governance, and extensive editing. CL provided primary text and concepts associated with managing chaotic and novel systems. DP-D provided text and concepts concerning monitoring. BR provided text and concepts associated with the changed role of modeling given novel systems. VS text and concepts associated with changed fire regimes and CFLRP governance. AS provided concepts and text associated with forested ecosystems, exotic pathogens, and modeling. CS provided concepts and text associated with dry forests and extensive editing of the entire document. DW provided primary text and concepts associated with democratized governance of wildlands. All authors contributed both to the paper's conceptualization and text.
Funding
Funding for authors' salaries was provided by the USDA Rocky Mountain Research Station.
Conflict of Interest
The authors declare that the research was conducted in the absence of any commercial or financial relationships that could be construed as a potential conflict of interest.
Acknowledgments
We want to thank Alison Hill for group leadership and logistical support, and Michael Young and Samuel Cushman for their helpful suggestions on previous versions of the manuscript, and the helpful comments from two referees. The findings and conclusions in this publication are those of the authors and should not be construed to represent any official USDA or U.S. Government determination or policy.
Supplementary Material
The Supplementary Material for this article can be found online at: https://www.frontiersin.org/articles/10.3389/ffgc.2021.644696/full#supplementary-material
References
Alfaro, R. I., Sickle, G. V., Thomson, A. J., and Wegwitz, E. (1982). Tree mortality and radial growth losses caused by the western spruce budworm in a Douglas-fir stand in British Columbia. Can. J. Forest Res. 12, 780–787. doi: 10.1139/x82-117
Allen, C. D., Macalady, A. K., Chenchouni, H., Bachelet, D., McDowell, N., Vennetier, M., et al. (2010). A global overview of drought and heat-induced tree mortality reveals emerging climate change risks for forests. For. Ecol. Manage. 259, 660–684. doi: 10.1016/j.foreco.2009.09.001
Allen, T. F. H., and Hoekstra, T. W. (2015). Toward a Unified Ecology (2nd Ed). New York, NY: Columbia University Press. doi: 10.7312/alle06918
Armitage, D., de Loë, R., and Plummer, R. (2012). Environmental governance and its implications for conservation practice. Conserv. Let. 5, 245–255. doi: 10.1111/j.1755-263X.2012.00238.x
Bickford, D., Posa, M. R. C., Qie, L., Campos-Arceiz, A., and Kudavidanage, E. P. (2012). Science communication for biodiversity conservation. Biol. Conserv. 151, 74–76. doi: 10.1016/j.biocon.2011.12.016
Bradley, B. A., Curtis, C. A., and Chambers, J. C. (2016). “Bromus response to climate and projected changes with climate change,” in Exotic Brome-Grasses in Arid and Semiarid Ecosystems of the Western US, eds M. J. Germino, J. C. Chambers, C. S. Brown (New York, NY: Springer), 257–274. doi: 10.1007/978-3-319-24930-8_9
Bradley, B. A., Curtis, C. A., Fusco, E. J., Abatzoglou, J. T., Balch, J. K., Dadashi, S., et al. (2018). Cheatgrass (Bromus tectorum) distribution in the intermountain Western United States and its relationship to fire frequency, seasonality, and ignitions. Biol. Invasions 20, 1493–1506. doi: 10.1007/s10530-017-1641-8
Bradley, B. A., and Mustard, J. F. (2008). Comparison of phenology trends by land cover class: a case study in the Great Basin, USA. Global Change Biol. 14, 334–346. doi: 10.1111/j.1365-2486.2007.01479.x
Brooks, M. L., D'Antonio, C. M., Richardson, D. M., Grace, J. B., Keeley, J. E., DiTomaso, J. M., et al. (2004). Effects of invasive alien plants on fire regimes. BioScience 54, 677–688. doi: 10.1641/0006-3568(2004)054[0677:EOIAPO]2.0.CO;2
Burnham, K. P., and Anderson, D. R. (2004). Multimodel inference: understanding AIC and BIC in model selection. Sociol. Methods Res. 33, 261–304. doi: 10.1177/0049124104268644
Carim, K. J., McKelvey, K. S., Young, M. K., Wilcox, T. M., and Schwartz, M. K. (2016). A Protocol for Collecting Environmental DNA Samples From Streams. US Department of Agriculture, Forest Service Gen. Tech. Rep. RMRS-GTR-355. Fort Collins, CO: Rocky Mountain Research Station. doi: 10.2737/RMRS-GTR-355
Chaffin, B. C., Gosnell, H., and Cosens, B. A. (2014). A decade of adaptive governance scholarship: synthesis and future directions. Ecol. Soc. 19:56. doi: 10.5751/ES-06824-190356
Chambers, J. C., Miller, R. F., Board, D. I., Pyke, D. A., Roundy, B. A., Grace, J. B., et al. (2014). Resilience and resistance of sagebrush ecosystems: implications for state and transition models and management treatments. Rangeland Ecol. Manag. 67, 440–454. doi: 10.2111/REM-D-13-00074.1
Chambers, J. C., Roundy, B. A., Blank, R. R., Meyer, S. E., and Whittaker, A. (2007). What makes Great Basin sagebrush ecosystems invasible by Bromus tectorum? Ecol. Monogr. 77, 117–145. doi: 10.1890/05-1991
Clements, F. E. (1916). Plant Succession: An Analysis of the Development of Vegetation (No. 242). Washington, DC: Carnegie Institution of Washington. doi: 10.5962/bhl.title.56234
Coates, P. S., Ricca, M. A., Prochazka, B. G., Brooks, M. L., Doherty, K. E., Kroger, T., et al. (2016). Wildfire, climate, and invasive grass interactions negatively impact an indicator species by reshaping sagebrush ecosystems. Proc. Natl. Acad. Sci. U.S.A. 113, 12745–12750. doi: 10.1073/pnas.1606898113
Cole, D. N., and Yung, L., (eds) (2010). Beyond Naturalness: Rethinking Park and Wilderness Stewardship in an Era of Rapid Change. Washington, DC: Island Press.
Collins, K. (2014). “Designing social learning systems for integrating social sciences into policy processes: some experiences with water managing,” in Understanding Society and Natural Resources: Forging New Strands of Integration Across the Social Sciences, eds M. J. Manfredo, J. J. Vaske, A. Rechkemmer, and E. A. Duke (Dordrecht: Springer), 229–251. doi: 10.1007/978-94-017-8959-2_11
Craig, R. K. (2010). “Stationarity is dead” – Long live transformation: five principles for climate change adaptation law. Harvard Environ. Law Rev. 34, 9–73.
Curtis, F. H. (1962). Linear programming the management of a forest property. J. Forestry 60, 611–616.
Dale, V. H., Joyce, L. A., McNulty, S., Neilson, R. P., Ayres, M. P., Flannigan, M. D., et al. (2001). Climate change and forest disturbances: climate change can effect forests by altering the frequency, intensity, duration, and timing of fire, drought, introduced species, insect and pathogen outbreaks, hurricanes, windstorms, ice storms, or landslides. BioScience 51, 723–734. doi: 10.1641/0006-3568(2001)051[0723:CCAFD]2.0.CO;2
Daubenmire, R. (1968). Plant Communities. A Textbook of Plant Synecology. New York, NY: Harper & Row.
Doremus, H. (2010). The Endangered species act: static law meets dynamic world. Washihgton Univ. J. Law Policy 32, 175–235.
Ezard, T. H. G., Bullock, J. M., Dalgleish, H. J., Millon, A., Pelletier, F., Ozgul, A., et al. (2010). Matrix models for a changeable world: the importance of transient dynamics in population management. J. Appl. Ecol. 47, 515–523. doi: 10.1111/j.1365-2664.2010.01801.x
Fettig, C. J., Mortenson, L. A., Bulaon, B. M., and Foulk, P. B. (2019). Tree mortality following drought in the central and southern Sierra Nevada, US. For. Ecol. Manage. 432, 164–178. doi: 10.1016/j.foreco.2018.09.006
Field, S. G., Schoettle, A. W., Klutsch, J. G., Tavener, S. J., and Antolin, M. F. (2012). Demographic projection of high-elevation white pines infected with white pine blister rust: a nonlinear disease model. Ecol. Appl. 22, 166–183. doi: 10.1890/11-0470.1
Fins, L., Byler, J., Ferguson, D., Harvey, A., Mahalovich, M. F., McDonald, G., et al. (2002). Return of the Giants: restoring western white pine to the Inland Northwest. J. For. 100, 20–26. doi: 10.1093/jof/100.4.20
Fischer, F. (2000). Citizens, Experts, and the Environment: The Politics of Local Knowledge. Durham, NC: Duke University Press. doi: 10.1215/9780822380283
Folke, C., Hahn, T., Olsson, P., and Norberg, J. (2005). Adaptive governance of social-ecological systems. Ann. Rev. Environ. Res. 30, 441–473. doi: 10.1146/annurev.energy.30.050504.144511
Freemuth, J. (2018). “US public lands and a new administration: New and old issues,” in Environmental Policy and the Pursuit of Sustainability, eds C. Schelly and A. Banerjee (New York: NY: Routledge), 65–78. doi: 10.4324/9781315099996-5
Freemuth, J., and Cawley, R. M. (1998). Science, expertise and the public: the politics of ecosystem management in the Greater Yellowstone ecosystem. Landscape Urban Plann. 40, 211–219. doi: 10.1016/S0169-2046(97)00114-X
Funtowicz, S. O., and Ravetz, J. R. (1993). “The emergence of post-normal science,” in Science, Politics and Morality. Theory and Decision Library (Series A: Philosophy and Methodology of the Social Sciences), Vol. 17, ed T. Von Schomber (Dordrecht, NL: Springer), 85–123. doi: 10.1007/978-94-015-8143-1_6
Gray, D. R., and MacKinnon, W. E. (2006). Outbreak patterns of the spruce budworm and their impacts in Canada. Forestry Chron. 82, 550–561. doi: 10.5558/tfc82550-4
Guess, A., and Coppock, A. (2020). Does counter-attitudinal information cause backlash? Results from three large survey experiments. Br. J. Political Sci. 50, 1497–1515. doi: 10.1017/S0007123418000327
Guiterman, C. H., Margolis, E. Q., Allen, C. D., Falk, D. A., and Swetnam, T. W. (2018). Long-term persistence and fire resilience of oak shrubfields in dry conifer forests of northern New Mexico. Ecosystems 21, 943–959. doi: 10.1007/s10021-017-0192-2
Gunderson, L. H., and Holling, C. S. (eds.), (2002). Panarchy: Understanding Transformations in Human and Natural Systems. Washington, DC: Island Press.
Haidt, J. (2012). The Righteous Mind: Why Good People are Divided by Politics and Religion. New York, NY: Pantheon Books.
Hays, S. P. (1959). Conservation and the Gospel of Efficiency: The Progressive Conservation Movement, 1890-1920. Cambridge, MA: Harvard University Press.
Hubbell, S. P. (2001). The Unified Neutral Theory of Biodiversity and Biogeography. Princeton, NJ: Princeton University Press.
Hutchinson, G. E. (1957). Concluding remarks. Cold Spring Harbor Symp. Quantit. Biol. 22, 415–427. doi: 10.1101/SQB.1957.022.01.039
Hutto, R. L., Keane, R. E., Sherriff, R. L., Rota, C. T., Eby, L. A., and Saab, V. A. (2016). Toward a more ecologically informed view of severe forest fires. Ecosphere 7:e01255. doi: 10.1002/ecs2.1255
IPCC (2013). Climate Change 2013: The Physical Science Basis. Contribution of Working Group I to the Fifth Assessment Report of the Intergovernmental Panel on Climate Change eds T. F. Stocker, D. Qin, G.-K. Plattner, M. Tignor, S. K. Allen, J. Boschung, et al. (New York, NY: Cambridge University Press).
Jasanoff, S. (ed.). (2004). States of Knowledge: The Co-Production of Science and the Social Order. London; New York, NY: Routledge. doi: 10.4324/9780203413845
Jedd, T., and Bixler, R. P. (2015). Accountability in networked governance: Learning from a case of landscape-scale forest conservation. Environ. Policy Govern. 25, 172–187. doi: 10.1002/eet.1670
Jerde, C. L., Mahon, A. R., Chadderton, W. L., and Lodge, D. M. (2011). “Sight-unseen” detection of rare aquatic species using environmental DNA. Conserv. Lett. 4, 150–157. doi: 10.1111/j.1755-263X.2010.00158.x
Jewell, S. and Vilsack, T. J. (2014). The National Strategy: The Final Phase in the Development of the National Cohesive Wildland Fire Management Strategy. Available online at: https://www.forestsandrangelands.gov/documents/strategy/strategy/CSPhaseIIINationalStrategyApr2014.pdf (accessed February 27, 2021).
Jolly, W. M., Cochrane, M. A., Freeborn, P. H., Holden, A. A., Brown, T., Williamson, G. J., et al. (2015). Climate-induced variations in global wildfire danger from 1979-2013. Nat. Commun. 6:7537. doi: 10.1038/ncomms8537
Jorgensen, S. E., and Muller, F. (2000). “Ecosystems as complex systems,” in Handbook of Ecosystem Theories and Management, eds S. E. Jorgensen and F. Muller (Boca Raton, FL: Lewis Publishers), 5–20.
Keane, R. E., Tomback, D. F., Aubry, C. A., Bower, A. D., Campbell, E. M., Cripps, C. L., et al. (2012). A Range-Wide Restoration Strategy for Whitebark Pine (Pinus albicaulis). Gen. Tech. Rep. RMRS-GTR-279. Fort Collins, CO: U.S. Department of Agriculture, Forest Service, Rocky Mountain Research Station, 108. doi: 10.2737/RMRS-GTR-279
Kearns, H. S. J., Jacobi, W. R., Reich, R. M., Flynn, R. L., Burns, K. S., and Geils, B. W. (2014). Risk of white pine blister rust to limber pine in Colorado and Wyoming, USA. For. Path. 44, 21–38. doi: 10.1111/efp.12065
Kent, B. M. (1980). Linear programming in land-management planning on National Forests. J. Forestry 78, 469–471.
Koontz, T. M., Gupta, D., Mudliar, P., and Ranjan, P. (2015). Adaptive institutions in social-ecological systems governance: a synthesis framework. Environ. Sci. Policy 53, 139–151. doi: 10.1016/j.envsci.2015.01.003
Landguth, E. L., Holden, Z. A., Mahalovich, M. F., and Cushman, S. A. (2017). Using landscape genetics simulations for planting blister rust resistant whitebark pine in the US Northern Rocky Mountains. Front. Genet. 8:9. doi: 10.3389/fgene.2017.00009
Latif, Q., Saab, V., and Dudley, J. (2020). Development and evaluation of habitat suitability models for nesting white-headed woodpeckers in burned forests. PLoS ONE 15:e0233043. doi: 10.1371/journal.pone.0233043
Latif, Q., Saab, V., Mellen-McLean, K., and Dudley, J. (2015). Evaluating habitat suitability models for nesting white-headed woodpeckers in unburned forest. J. Wildl. Manage. 79, 263–273. doi: 10.1002/jwmg.842
Latif, Q. S., Saab, V. A., Dudley, J. G., and Hollenbeck, J. P. (2013). Ensemble modeling to predict habitat suitability for a large-scale disturbance specialist. Ecol. Evol. 3, 4348–4364. doi: 10.1002/ece3.790
Latif, Q. S., Saab, V. A., Haas, J. R., and Dudley, J. G. (2018). FIRE-BIRD: A GIS-based Toolset for Applying Habitat Suitability Models to Inform Land Management Planning. Gen. Tech. Rep. RMRS-GTR-391. Fort Collins, CO: US Department of Agriculture, Forest Service, Rocky Mountain Research Station, 74. doi: 10.2737/RMRS-GTR-391
Levins, R. (1969). The effects of random variation of different types on population growth. Proc. Natl. Acad. Sci. U.S.A. 62, 1061–1065. doi: 10.1073/pnas.62.4.1061
Levins, R. (1970). “Extinction,” in Some Mathematical Questions in Biology, ed M. Gerstenhaber (Providence, RI: American Mathematical Society), 77–107.
Lewandowsky, S., and Oberauer, K. (2016). Motivated rejection of science. Psychol. Sci. 25, 217–222. doi: 10.1177/0963721416654436
Lindenmayer, D. B., and Noss, R. F. (2006). Salvage logging, ecosystem processes, and biodiversity conservation. Conserv. Biol. 20, 949–958. doi: 10.1111/j.1523-1739.2006.00497.x
Loeffler, D., and Anderson, N. (2018). Impacts of the mountain pine beetle on sawmill operations, costs, and product values in Montana. Forest Prod. J. 68, 15–24. doi: 10.13073/FPJ-D-17-00041
Ludwig, D. (2001). The era of management is over. Ecosystems 4, 758–764. doi: 10.1007/s10021-001-0044-x
Lute, A. C., and Luce, C. H. (2017). Are model transferability and complexity antithetical? Insights from validation of a variable-complexity empirical snow model in space and time. Water Resour. Res. 53, 8825–8850. doi: 10.1002/2017WR020752
MacArthur, R. H., and Wilson, E. O. (1967). The Theory of Island Biogeography. Monographs in Population Biology I. Princeton, NJ: Princeton University Press.
Mason, L., White, G., Morishima, G., Alvarado, E., Andrew, L., Clark, F., et al. (2012). Listening and learning from traditional knowledge and Western science: A dialogue on contemporary challenges of forest health and wildfire. J. Forestry 110, 187–193. doi: 10.5849/jof.11-006
May, C., Luce, C., Casola, J., Chang, M., Cuhaciyan, J., Dalton, M., et al. (2018). “Northwest [Ch. 24],” in Impacts, Risks, and Adaptation in the United States: Fourth National Climate Assessment, Volume II. U.S. Global Change Research Program, eds D. R. Reidmiller, C. W. Avery, D. R. Easterling, K. E. Kunkel, K. L. M. Lewis, T. K. Maycock, et al. (Washington, DC: U.S. Global Change Research Program), 1036–1100. doi: 10.7930/NCA4.2018.CH24
Meddens, A. J., Hicke, J. A., and Ferguson, C. A. (2012). Spatiotemporal patterns of observed bark beetle-caused tree mortality in British Columbia and the western United States. Ecol. Appl. 22, 1876–1891. doi: 10.1890/11-1785.1
Millar, C. I., Stephenson, N. L., and Stephens, S. L. (2007). Climate change and forests of the future: managing in the face of uncertainty. Ecol. Appl. 17, 2145–2151. doi: 10.1890/06-1715.1
Miller, T. R., Baird, T. D., Littleield, C. M., Kofinas, G., Chapin, F. S. III., and Redman, C. R. (2008). Epistemological pluralism: reorganizing interdisciplinary research. Ecol. Soc. 13:46. doi: 10.5751/ES-02671-130246
Mistry, J., and Berardi, A. (2016). Bridging indigenous and scientific knowledge. Science 352, 1274–1275. doi: 10.1126/science.aaf1160
Mitchell, S. D. (2009). Unsimple Truths: Science, Complexity and Policy. Chicago, IL: University of Chicago. doi: 10.7208/chicago/9780226532653.001.0001
Moir, W. H., Geils, B. W., Benoit, M. A., and Scurlock, D. (1997). “Ecology of southwestern ponderosa pine forests,” in Songbird Ecology in Southwestern Ponderosa Pine Forests: A Literature Review. Eds W. M. Block and D.M. Finch (Fort Collins, CO: U.S. Forest Service, General Technical Report RM-292), 3–27.
Morgan, P., Aplet, G. H., Haufler, J. B., Humphries, H. C., Moore, M. M., and Wilson, W. D. (1994). Historical range of variability: a useful tool for evaluating ecosystem change. J. Sustain. Forestry 2, 87–111. doi: 10.1300/J091v02n01_04
Nagel, L. M., Palik, B. J., Battaglia, M. A., D'Amato, A. W., Guldin, J. M., et al. (2017). Adaptive silviculture for climate change: a national experiment in manager-science partnerships to apply an adaptation framework. J. For. 115, 167–178. doi: 10.5849/jof.16-039
Nyhan, B., and Reifler, J. (2015). Does correcting myths about the flu vaccine work? An experimental evaluation of the effects of corrective information. Vaccine 33, 459–464. doi: 10.1016/j.vaccine.2014.11.017
Nyhan, B., Reifler, J., Richey, S., and Freed, G. L. (2014). Effective messages in vaccine promotion: a randomized trial. Pediatrics 133, 835–e842. doi: 10.1542/peds.2013-2365
Ostrom, E. (2010). Beyond markets and states: polycentric governance of complex economic systems. Am. Econ. Rev. 100, 641–672. doi: 10.1257/aer.100.3.641
Owen, S. M., Sieg, C. H., Meador, A. J. S., Fulé, P. Z., Iniguez, J. M., Baggett, L. S., et al. (2017). Spatial patterns of ponderosa pine regeneration in high-severity burn patches. Forest Ecol. Manage. 405, 134–149. doi: 10.1016/j.foreco.2017.09.005
Pacifici, M., Foden, W. B., Visconti, P., Watson, J. E. M., Butchart, S. H. M., Kovacs, K. M., et al. (2015). Assessing species vulnerability to climate change. Nat. Clim. Change 5, 215–224. doi: 10.1038/nclimate2448
Parks, S. A., Dobrowski, S. Z., Shaw, J. D., and Miller, C. (2019). Living on the edge: trailing edge forests at risk of fire-facilitated conversion to non-forest, Ecosphere 10:e02651. doi: 10.1002/ecs2.2651
Peel, M. C., and Blöschl, G. (2011). Hydrological modelling in a changing world. Prog. Phys. Geogr. 35, 249–261. doi: 10.1177/0309133311402550
Pfister, L., and Kirchner, J. W. (2017). Debates—hypothesis testing in hydrology: theory and practice. Water Resour. Res. 53, 1792–1798. doi: 10.1002/2016WR020116
Pickett, S. T. A., and White, P. S. (1985). “Patch dynamics: a synthesis.” in The Ecology of Natural Disturbance and Patch Dynamics, eds. S. T. A. Pickett and P. S. White (San Diego, CA: Academic Press), 371–384. doi: 10.1016/B978-0-12-554520-4.50026-5
Pittinsky, T. L. (2015). America's crisis of faith in science. Science 349, 511–512 doi: 10.1126/science.348.6234.511-a
Puhlick, J. J., Laughlin, D. C., and Moore, M. M. (2012). Factors influencing ponderosa pine regeneration in the southwestern USA. Forest Ecol. Manage. 264, 10–19. doi: 10.1016/j.foreco.2011.10.002
Purdy, J. (2015). After Nature: A Politics for the Anthropocene. Cambridge, MA: Harvard University Press. doi: 10.4159/9780674915671
Quay, R. (2010). Anticipatory governance: a tool for climate change adaptation. J. Am. Planning Assoc. 76, 496–511. doi: 10.1080/01944363.2010.508428
Real, L. A., and Levin, S. A. (1991). “Theoretical advances: the role of theory in the rise of modern ecology,” in Foundations of Ecology: Classic Papers With Commentaries, eds L. A. Real, and J. H. Brown (Chicago, IL: University of Chicago Press), 177–191.
Rehfeldt, G. E., Crookston, N. L., Saenz-Romero, C., and Campbell, E. M. (2012). North American vegetation model for land-use planning in a changing climate: a solution to large classification problems. Ecol. Appl. 22, 119–141. doi: 10.1890/11-0495.1
Reynolds, R. T., Graham, R. T., Reiser, M. H., Bassett, R. L., Kennedy, P. L., Boyce, D. A. Jr., et al. (1992). Management recommendations for the northern goshawk in the Southwestern United States. Fort Collins, CO: U.S. Forest Service, General Technical Report RM-GTR-217, 90. doi: 10.2737/RM-GTR-217
Reynolds, R. T., Meador, A. J. S., Youtz, J. A., Nicolet, T., Matonis, M. S., Jackson, P. L., et al. (2013). Restoring composition and structure in southwestern frequent-fire forests: a science-based framework for improving ecosystem resiliency. Gen. Tech. Rep. RMRS-GTR-310, 76. doi: 10.2737/RMRS-GTR-310
Richardson, B. A., and Chaney, L. (2018). Climate-based seed transfer of a widespread shrub: population shifts, restoration strategies, and the trailing edge. Ecol. Appl. 44, 367–10. doi: 10.1002/eap.1804
Rieman, B. E., Hessburg, P. F., Luce, C., and Dare, M. R. (2010). Wildfire and management of forests and native fishes: conflict or opportunity for convergent solutions. BioScience 60, 460–468. doi: 10.1525/bio.2010.60.6.10
Roesch-McNally, G., Chang, M., Dalton, M., Lowe, S., Luce, C., May, C., et al. (2020). Beyond climate impacts: knowledge gaps and process-based reflection on preparing a regional chapter for the Fourth National Climate Assessment. Weather Clim. Soc. 12, 337–350. doi: 10.1175/WCAS-D-19-0060.1
Rosindell, J., Hubbell, S. P., He, F., and Harmon, L. J.„ and, Etienne, R. S. (2012). The case for ecological neutral theory. Trends Ecol. Evol. 273, 203–208. doi: 10.1016/j.tree.2012.01.004
Runting, R. K., Bryan, B. A., Dee, L. E., Maseyk, F. J. F., Mandle, L., Hamel, P., et al. (2017). Incorporating climate change into ecosystem services assessments and decisions: a review. Global Change Biol. 23, 28–41. doi: 10.1111/gcb.13457
Saab, V. A., Russell, R. E., and Dudley, J. G. (2009). Nest-site selection by cavity-nesting birds in relation to postfire salvage logging. For. Ecol. Manag. 257, 151–159. doi: 10.1016/j.foreco.2008.08.028
Saab, V. A., Russell, R. E., Rotella, J., and Dudley, J. G. (2011). Modeling nest survival of cavity-nesting birds in relation to postfire salvage logging. J. Wildl. Manag. 75, 794–804. doi: 10.1002/jwmg.111
Saab, V., Latif, Q., Dresser, Q. M., and Dudley, J. (2019). Woodpecker nest survival, density, and a pine beetle outbreak. J. Wildl. Manag. 83, 1387–1400. doi: 10.1002/jwmg.21722
Sabo, K. E., Sieg, C. H., Hart, S. C., and Bailey, J. D. (2009). The role of disturbance severity and canopy closure on standing crop of understory plant species in ponderosa pine stands in northern Arizona, USA. Forest Ecol. Manage. 257, 1656–1662. doi: 10.1016/j.foreco.2009.01.006
Sarewitz, D. (2004). How science makes environmental controversies worse. Environ. Sci. Policy 7, 385–403. doi: 10.1016/j.envsci.2004.06.001
Savage, M., and Mast, J. N. (2005). How resilient are southwestern ponderosa pine forest after crownfire? Can. J. Forest Res. 35, 967–977. doi: 10.1139/x05-028
Savage, M., Mast, J. N., and Feddema, J. J. (2013). Double whammy: high-severity fire and drought in ponderosa pine forests of the Southwest. Can. J. Forest Res. 43, 570–583. doi: 10.1139/cjfr-2012-0404
Schoettle, A. W., Burns, K. S., Cleaver, C. M., and Connor, J. J. (2019a). Proactive Limber Pine Conservation Strategy for the Greater Rocky Mountain National Park Area. Gen. Tech. Rep. RMRS-GTR-379. Fort Collins, CO: US Department of Agriculture, Forest Service, Rocky Mountain Research Station, 81. doi: 10.2737/RMRS-GTR-379
Schoettle, A. W., Jacobi, W. R., Waring, K. M., and Burns, K. S. (2019b). Regeneration for resilience framework to support regeneration decisions for species with populations at risk of extirpation by white pine blister rust. New For. 50, 89–114. doi: 10.1007/s11056-018-9679-8
Schultz, C. A., Jedd, T., and Beam, R. D. (2012). The collaborative forest landscape restoration program: a history and overview of the first projects. J. Forestry 110, 381–391. doi: 10.5849/jof.11-082
Schultz, C. A., Thompson, M. P., and McCaffrey, S. M. (2019). Forest Service fire management and the elusiveness of change. Fire Ecol. 15:13. doi: 10.1186/s42408-019-0028-x
Shanahan, E. A., Raile, E. D., Naughton, H. T., Wallner, M. P., and Houghton, K. A. (2020). Public opinion about management strategies for a low-profile species across multiple jurisdictions: Whitebark pine in the northern Rockies. People Nat. 2, 784–796. doi: 10.1002/pan3.10094
Shi, H., Rigge, M., Homer, C. G., Xian, G., Meyer, D. K., and Bunde, B. (2018). Historical cover trends in a sagebrush steppe ecosystem from 1985 to 2013: links with climate, disturbance, and management. Ecosystems 21, 913–929. doi: 10.1007/s10021-017-0191-3
Squires, J. R., and Kennedy, P. (2006). Northern goshawk ecology: an assessment of current knowledge and information needs for conservation and management. Stud. Avian Biol. 31, 8–62.
Still, S. M., and Richardson, B. A. (2015). Projections of contemporary and future climate niche for wyoming big sagebrush (Artemisia tridentata subsp. wyomingensis): a guide for restoration. Nat. Areas J. 35, 30–43. doi: 10.3375/043.035.0106
Strausbaugh, L. J., Marfin, A. A., and Gubler, D. J. (2001). West Nile encephalitis: an emerging disease in the United States. Clin. Infect. Dis. 33, 1713–1719. doi: 10.1086/322700
Tavener, S., Mikucki, M., Field, S. G., and Antolin, M. F. (2011). Transient sensitivity analysis for nonlinear population models. Methods Ecol. Evol. 2, 560–575. doi: 10.1111/j.2041-210X.2011.00108.x
Teng,ö, M., Brondizio, E. S., Elmqvist, T., Malmer, P., and Spierenburg, M. (2014). Connecting diverse knowledge systems for enhanced ecosystem governance: the multiple evidence base approach. Ambio 43, 579–591. doi: 10.1007/s13280-014-0501-3
Tengö, M., Hill, R., Malmer, P., Raymond, C. M., Spierenburg, M., Danielsen, F., et al. (2017). Weaving knowledge systems in IPBES, CBD and beyond—lessons learned for sustainability. Curr. Opin. Environ. Sustain. 26, 17–25. doi: 10.1016/j.cosust.2016.12.005
Thomas, J. W., and Burchfield, J. (1999). Comments on “The religion of forestry: scientific management”. J. Forestry 97, 10–11.
Tomback, D. F., Arno, S. F., and Keane, R. E., (eds) (2001). Whitebark Pine Communities: Ecology and Restoration. Washington, DC: Island Press, 440.
Urgenson, L. S., Ryan, C. M., Halpern, C. B., Bakker, J. D., Belote, R. T., Franklin, J. F., et al. (2017). Visions of restoration in fire-adapted forest landscapes: lessons from the collaborative forest landscape restoration program. Environ. Manage. 59, 338–353. doi: 10.1007/s00267-016-0791-2
Vinyeta, K., and Lynn, K. (2013). Exploring the Role of Traditional Ecological Knowledge in Climate Change Initiatives. Gen. Tech. Rep. PNW-GTR-879. Portland, OR: US Department of Agriculture, Forest Service, Pacific Northwest Research Station, 37. doi: 10.2737/PNW-GTR-879
Walters, C. J. (1997). Challenges in adaptive management of riparian and coastal ecosystems. Conserv. Ecol. 1:1. doi: 10.5751/ES-00026-010201
Weber, E. P., Lach, D., and Steel, B. S., (eds.) (2017). New Strategies for Wicked Problems: Science and Solutions in the 21st Century. Corvallis, OR: Oregon State University Press.
White, A. S. (1985). Presettlement regeneration patterns in a Southwestern ponderosa pine stand. Ecology 66, 589–594. doi: 10.2307/1940407
Wilkinson, T. (1998). Science Under Siege: The Politicians' War on Nature and Truth. Boulder, CO: Johnson Books.
Williams, B. A., and Matheny, A. R. (1995). Democracy, Dialogue, and Environmental Disputes: The Contested Languages of Social Regulation. CT: Yale University Press.
Williams, D. R. (2017). “Chapter 7: the role of place-based social learning,” in New Strategies for Wicked Problems: Science and Solutions in the 21st century, eds. E. P. Weber, D. Lach, and B. S. Steel (Corvallis, OR: Oregon State University Press), 149–168.
Williams, D. R. (2018). “Spacing conservation practice: place-making, social learning, and adaptive landscape governance in natural resource management,” in The SAGE Handbook of Nature, Vol. 1 ed. T. Marsden (London: Sage Publications), 285–303. doi: 10.4135/9781473983007.n16
Worrall, J. J., Egeland, L., Eager, T., Mask, R. A., Johnson, E. W., Kemp, P. A., et al. (2008). Rapid mortality of Populus tremuloides in southwestern Colorado, USA. Forest Ecol. Manage. 255, 686–696. doi: 10.1016/j.foreco.2007.09.071
Wuebbles, D. J., Fahey, D. W., Hibbard, K. A., Dokken, D. J., Stewart, B. C., and Maycock, T. K., (eds.) (2017). Climate Science Special Report: Fourth National Climate Assessment, Volume I. Washington, DC: U.S. Global Change Research Program. doi: 10.7930/J0J964J6
Wyborn, C. (2015). Co-productive governance: a relational framework for adaptive governance. Glob. Environ. Change 30, 56–67. doi: 10.1016/j.gloenvcha.2014.10.009
Young, I. M. (1996). “Communication and the other: Beyond deliberative democracy,” in Democracy and Difference: Contesting the Boundaries of the Political NJ, ed. S. Benhabib (Princeton, NJ: Princeton University Press), 121–135.
Keywords: governance, forest restoration, novel ecosystems, monitoring, modeling, climate change, wildlands
Citation: McKelvey KS, Block WM, Jain TB, Luce CH, Page-Dumroese DS, Richardson BA, Saab VA, Schoettle AW, Sieg CH and Williams DR (2021) Adapting Research, Management, and Governance to Confront Socioecological Uncertainties in Novel Ecosystems. Front. For. Glob. Change 4:644696. doi: 10.3389/ffgc.2021.644696
Received: 21 December 2020; Accepted: 19 February 2021;
Published: 11 March 2021.
Edited by:
Amy E. Duchelle, Center for International Forestry Research (CIFOR), IndonesiaReviewed by:
Amy Lauren Lovecraft, University of Alaska Fairbanks, United StatesNatalia Vasilievna Lukina, Russian Academy of Sciences (RAS), Russia
Copyright © 2021 McKelvey, Block, Jain, Luce, Page-Dumroese, Richardson, Saab, Schoettle, Sieg and Williams. This is an open-access article distributed under the terms of the Creative Commons Attribution License (CC BY). The use, distribution or reproduction in other forums is permitted, provided the original author(s) and the copyright owner(s) are credited and that the original publication in this journal is cited, in accordance with accepted academic practice. No use, distribution or reproduction is permitted which does not comply with these terms.
*Correspondence: Kevin S. McKelvey, kevin.mckelvey@usda.gov