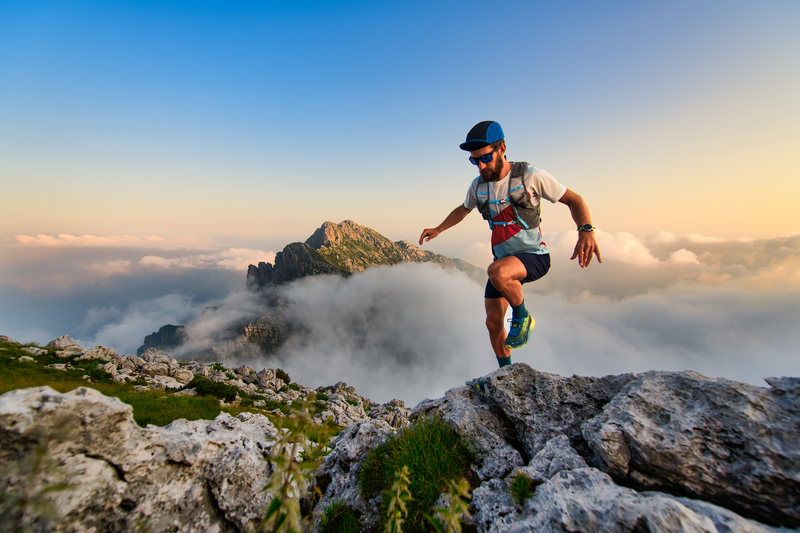
94% of researchers rate our articles as excellent or good
Learn more about the work of our research integrity team to safeguard the quality of each article we publish.
Find out more
ORIGINAL RESEARCH article
Front. For. Glob. Change , 06 October 2020
Sec. Forests and the Atmosphere
Volume 3 - 2020 | https://doi.org/10.3389/ffgc.2020.562044
The pressure to increase forest and land carbon stocks simultaneously with increasing forest based biomass harvest for energy and materials emphasizes the need for dedicated analyses of impacts and possible trade-offs between these different mitigation options including also forest related biophysical factors, surface albedo and the formation of biogenic Secondary Organic Aerosols (SOA). We analyzed the change in global radiative forcing (RF) due to changes in these climatic agents as affected by the change in state of Finnish forests under increased or decreased harvest scenarios from a baseline. We also included avoided emissions due to wood material and energy substitution. Increasing harvests from baseline (65% of Current Annual Increment) decreased the total carbon sink (carbon in trees, soil and harvested wood products) at least for 50 years. When we coupled this change in carbon with other biosphere responses, surface albedo and aerosols, decreasing harvests from the baseline produced the largest cooling effect during 50 years. Accounting also for the avoided emissions due to increased wood use, the RF responses of the two lowest harvest scenarios were within uncertainty range. Our results show that the effects of forest management on SOA formation should be included in the analyses trying to deduce the net climate impact of forest use. The inclusion of the rarely considered SOA effects enforces the view that the lower the harvest, the more climatic cooling boreal forests provide. These results should act as a caution mark for policy makers who are emphasizing the increased utilization of forest biomass for short-living products and bioenergy as an efficient measure to mitigate climate change.
IPCC special report ‘Climate Change and Land’ (IPCC, 2019) emphasizes the urgent need of mitigation actions in the land sector. Globally the report gives the highest maximum technical mitigation potential in forest-related actions, e.g., reforestation, reducing deforestation, and bioenergy combined with carbon capture and storage (BECCS). The report also indicates increased forest and land carbon stocks as one of the most cost-efficient and feasible carbon dioxide removal measures to generate the negative emissions required to restrict global warming under 2°C. The report puts clear constrains on the many higher-end bioenergy-based scenarios generated by Integrated Assessment Models striving for the objectives of Paris Agreement in the special report of 1.5°C global warming (IPCC, 2018). The pressure to increase forest and land carbon stocks simultaneously with increasing forest based biomass harvest for energy and materials emphasizes the need for dedicated analyses of impacts and possible trade-offs between these different mitigation options (Smith et al., 2016; Griscom et al., 2017; Erb et al., 2018) in terms of radiative forcing (RF). So far, the pathways limiting warming to under 2°C do not evaluate other than carbon changes in land use sector and these studies call for more research on these effects (Roe et al., 2019).
Finland is one of the most forested countries in the world, with 86% of land cover being forests. The carbon stock of Finnish forests has increased during the last 50 years (Korhonen et al., 2017). Forest-based bioeconomy and increased use of wood-based materials have been seen as an effective climate mitigation strategy in governmental decision making (e.g., The National Forest Strategy 2025, 2019). The net atmospheric effect of increased harvests has not been analyzed, however, in Finland nor in any other country which uses its forest resources intensively.
Since wood products can be used for substituting more carbon intensive non-wood alternatives, the use of forests provides a potentially cost effective tool for mitigation of greenhouse gas emissions (Sathre and O’Connor, 2010; Soimakallio et al., 2016; Pingoud et al., 2018; Seppälä et al., 2019). The current harvest level of European forests is substantially lower than their wood increment (Forest Europe, 2015), which could be seen as an opportunity to increase biomass utilization to replace fossil fuels. However, wood harvesting immediately reduces forest carbon sink and the net effect thus may not result in the required emission reductions (Schulze et al., 2012; Soimakallio et al., 2016; Seppälä et al., 2019). Different forest management strategies (e.g., variations in harvest intensity, selection of regenerated species, and length of rotation period) can have significant impacts on carbon sink and storage (Pihlainen et al., 2014; Heinonen et al., 2017). The sink reduction due to increased wood use for bioenergy or short-living wood products like pulp and paper is not easily compensated (Ter-Mikaelian et al., 2015; Seppälä et al., 2019). Especially in boreal forests where trees grow slowly and soil carbon stock plays a major role, the time span of climate benefits from increased traditional wood use has been found to be too long (Mitchell et al., 2012; Lemprière et al., 2013; Chen et al., 2018; Dugan et al., 2018) to cope with the very limited carbon budget determined by the objectives of Paris Agreement (IPCC, 2018). Studies analyzing climatic impact of wood use show that the result depends heavily on the assumed portfolio of wood products and therefore the obtained substitution benefits (Seppälä et al., 2019). Moreover, rapidly developing renewable energy solutions, e.g., rapid development of solar and wind energy use, further decreases the relative pace of gained substitution benefits.
In addition to the carbon impact of forest use, the forest ecosystem also produces so-called biophysical non-carbon climate impacts, i.e., albedo (Bright et al., 2014; Kuusinen et al., 2014; Matthies and Valsta, 2016; Naudts et al., 2016; Arvesen et al., 2018; Luyssaert et al., 2018) and secondary organic aerosols (SOA) (e.g., Tunved et al., 2006; Spracklen et al., 2008; Paasonen et al., 2013; Kulmala et al., 2014; Unger, 2014; Roldin et al., 2019). In comparison of well-mixed and long-living greenhouse gas (GHG) like CO2, these biophysical effects have shorter time scale and regional and local impacts may be more important than impacts on global climate.
Some of the studies including surface albedo changes have reached the conclusion that increased forest cover in high latitudes, especially in the boreal region, would result in warming instead of cooling, due to decreased surface albedo (e.g., Betts, 2000; Unger, 2014; Popkin, 2019). None of the studies have so far simultaneously accounted for both of these biophysical effects, albedo and aerosols, in combination with different forest use scenarios.
Atmospheric aerosol particles influence the Earth’s radiative budget directly by reflecting and absorbing radiation (direct effect, Charlson et al., 1992) and indirectly, by acting as cloud condensation nuclei (CCN, Kerminen et al., 2012; Paasonen et al., 2013), and thereby changing the formation, optical properties and longevity of clouds (indirect effect, Twomey, 1977; Albrecht, 1989). Aerosols are connected to land cover changes and forests through the production of Biogenic Volatile Organic Compounds (BVOC) acting as precursors for SOA. It is well-known that aerosol particles are responsible for the largest uncertainties in climate change predictions (IPCC, 2013). For example, the understanding of how BVOCs contribute and affect the formation of new particles (NPF) is not complete. Even more uncertain than the direct SOA effect is formation of clouds due to changes in SOA and thus the indirect cloud albedo effect (Unger, 2014; Unger et al., 2017). Particularly, the growth of newly formed particles to CCN sizes, i.e., from less than 2 nm to over 50–100 nm in diameter, is not well-known (Paasonen et al., 2018; Roldin et al., 2019). Although NPF has been observed to occur almost everywhere (Nieminen et al., 2018), factors like measurement time span, campaign length, continuous vs. discontinuous measurements, also differ between studies and increase the uncertainties in the relative importance of aerosols.
However, analyses including also these most uncertain elements are needed to enhance the use of these factors in models, such as Earth system models used in projecting land sector impacts of climate change for IPCC reports. Accounting for the non-carbon effects deepens our understanding of system dynamics and may emphasize regional differences in the optimal mitigation solutions.
The main motivation of our study is to address the RF impacts of potential forest management scenarios at the scale of one country, Finland, where sufficient data exists for such an analysis. In this paper, we predicted size dependent aerosol particle concentrations in different forest ecosystems by the SOSAA model (Boy et al., 2011; Zhou et al., 2014). The direct reflective effect of the particles on RF we calculated based on the method described in the Supplementary Material of Lihavainen et al. (2009) and Paasonen et al. (2013). For the estimated impacts on cloud albedo effects, we rely on the seminal work by Kurtén et al. (2003). They reached the conclusion that BVOC-induced cloud formation could increase cloud albedo in terms of RF from −1.0 to −31.7 W m–2 as an annual average of forest in southern Finland. According to our knowledge, for the geographical region of Finland, no formal uncertainty boundaries of SOA radiative forcing effect could be generated. Thus, we rely on the range presented by Kurtén et al. (2003).
Our objective is to analyze the implications on RF of an increase or decrease of harvests from a given baseline, rather than to compare forest management vs. no management. In our analysis, we account for (1) carbon stock changes (in trees and soil, i.e., forest ecosystems, and harvested wood products, HWP), (2) surface albedo of forest area, (3) forest originating secondary aerosols (SOA), and (4) avoided CO2-emissions due to wood energy and material substitution. We calculate the net impact of these effects on global RF during 50 years due to the changes of Finnish forests.
We estimated the effect of regional forest harvest scenarios on the global RF development (Figure 1). Forest harvest scenarios were defined as the ratio of harvested stem wood volume (m3 year–1) to the current annual stem wood increment (CAI, year 2013 as a reference level) in Finland. These scenarios (harvest levels) were 50, 65, 80, 100, and 130% of CAI. During 2009–2018, harvest removals have been ca. 62% of increment in Finland (Luke statistics database), and thus, we defined 65% of CAI harvest scenario as a baseline (Supplementary Figures S1–S6). The development of forests under the different harvesting scenarios was based on stand level projections (see Supplementary Material). For simplifying the forested land cover, we described the forests as single species stands of three dominant boreal tree species Supplementary Table S1, Scots pine (Pinus sylvestris), Norway spruce (Picea abies), and silver birch (Betula pendula), separately in three different site fertility classes (herb-rich, mesic, sub-xeric, Cajander, 1949) under the current climate. This is justified because over one third of the Finnish forests are rather homogeneous stands where single species represents > 75% of the basal area and most of them are managed using the rotation forestry scheme1. Also, carbon storage, albedo and emissions of volatile compounds can be assumed to be additive. This means mixed stands can be represented by an average of single stands.
Figure 1. Schematic presentation of the study. We simulated the stand development [changes of carbon storage in trees, soil, and harvested wood products (HWP)] of most common Finnish tree species at representative forest types (sub-xeric Scots pine, mesic Norway spruce, herb-rich Norway spruce, and herb rich Silver birch) at the current climate and compiled an expression of Finnish forests using recent National Forest Inventory data (NFI). Based on literature values we estimated the potential of avoided emissions obtained with wood use. Using this information, we calculated how a change in state of forests in Finland influenced global radiative forcing (RF) during 50 years relative to the baseline assuming different policy options for harvesting [annual harvest 50–130% of current annual forest growth (CAI)].
We simulated the stand level development with the MOTTI Stand Simulator (Salminen et al., 2005). From these single species stands we compiled an initial state of Finnish forests using 11th Finnish national forest inventory (NFI, Korhonen et al., 2017). This compilation covered all forest land available for wood supply (ca. 18.2 million hectares). We used this setup containing dynamic age-class matrices (age classes 0–10, 10–20…101–) of surface-area distributions of the three studied species and three fertility classes to simulate forest development in Finland. After setting the initial state we calculated the annual change of age structure and division of growing stock in the different age classes in different harvest scenarios. We simulated the harvests with a prescribed proportion of volume (and surface area) in each age class and moved 10% of the remaining volume (and surface area) to the next older 10-year-wide age class. Forest management followed the recommendations for private forest owners in Finland (Äijälä et al., 2014, see Supplementary Material), which means that annually harvested volume consisted of stem wood from irecommended thinnings and final harvests. If the pursued national harvest level was not reached after thinnings, final harvests of stands were performed until the preset harvest level was reached. In practice, this means that rotation lengths of single stands were either increased or decreased to fulfill the national level harvesting scenario. However, shorter stand rotations than the recommended minimum final felling age were not used in the simulations.
We needed this simplified approach in order to be able to analyze simultaneously the change in global RF due to changes in different climatic agents, f(CO2, SOA, albedo) as affected by the change in state of Finnish forests under different harvest scenarios.
Forests on peatland soils were assumed to follow the same growth dynamics and albedo and aerosol response as forests on mineral soils, and soil carbon (see below) was always calculated assuming mineral soil type. Exclusion of peatland forests simplified this complex analysis in many ways, e.g., we did not need to consider dynamics of other greenhouse gases (GHG) than CO2 or dynamics of accumulating and decomposing peat. Also we are lacking information of BVOC and SOA dynamics in peatland forests. Similarly, we did not consider forests in conservation areas (following the classification in the national forest inventory), or other tree species than those mentioned above.
We did not perform sensitivity analysis of forest carbon simulations because we used well-established models. Instead, we used the total uncertainty of modeled forest carbon dynamics of 15% in our analysis. This value consists of parametric uncertainty of MOTTI (Salminen et al., 2005) and Yasso (Tuomi et al., 2011) models, and errors in biomass expansion factors (BEF) between different stand age classes. The prediction error of MOTTI has been found to vary from 1.2 to 10.3% depending on the predicted variable within 20 years simulation period (Mäkinen et al., 2008). Total uncertainty of Yasso simulations varied from 12 to 15% (Liski et al., 2005). However, the value we used quite probably underestimates the uncertainty since in GHG-inventory of Finland, uncertainty of forest land carbon sink is 31% when soil carbon is included (National Inventory Report (NIR), 2019).
We assumed all the forest to be in mineral soils with mineral soil C dynamics (see section “Estimation of Annual Carbon Balance and the Radiative Forcing Effect of Carbon Stock Change”). In reality, 25% is in organic soils, where the C and N dynamics differ a lot from those on mineral soils. This causes a significant bias in the results, but will not change our conclusions because we compare only the difference between scenarios and this error due the omission of peatlands is same in all scenarios. We provide our interpretation of the effect of excluding peatland forests in the discussion section.
We estimated the annual change of forest area and biomass in each age class of each forest type to derive a country-wise carbon stock change estimate (based on state transfer between age classes, see above). We calculated the change of aboveground biomass by converting the species and age class specific stem volume to biomass through BEF presented by Lehtonen et al. (2004). We assumed 50% carbon concentration in dry-weight woody biomass.
We also considered soil carbon pool development in the scenarios. Litter input into soil in different forest types was calculated by multiplying simulated biomass compartments and turnover rates in MOTTI with the BEFs used in the Finnish greenhouse gas inventory. The soil decomposition model YASSO07 (Tuomi et al., 2011) was used to simulate soil carbon dynamics with the obtained litter inputs. We multiplied YASSO outputs with the share of surface area of each age class in each site fertility class for getting soil carbon dynamics in the whole forest area available for wood production in Finland (18.2 million ha in this analysis, i.e., conservation areas not included).
The carbon stores and dynamics of HWPs (Supplementary Figure S6) were computed from the timber harvest assortment information provided by MOTTI, and species-specific product distributions and life cycle information (Table 1). For sawlogs, the allocation of roundwood to industrial products was based on Karjalainen et al. (1994) because that source enabled computations by species and was the most detailed source available. Pulpwood-sized wood was added to fiber raw material from sawlog residues and was consumed for paper and packaging products. Storage of carbon in products was based on four lifecycle categories with exponential decay, given in Karjalainen et al. (1994, Supplementary Figure S9).
We estimated the initial carbon storage of wood products by simulating HWP dynamics with initial forest structure, pulp-log ratio, and baseline harvests until equilibrium in the HWP carbon storage was reached. Then we simulated the effect of the actual harvest scenarios on the dynamics of HWPs by using the equilibrium HWP storage as initial value (Supplementary Figure S6). In reality, the HWP pools are far from equilibrium and adding more long lived products to these pools will yield a higher mitigation benefit than assumed here because global HWP stocks are still increasing.
We analyzed the annual stock change of the total carbon stored in the system (above- and belowground biomass, soil carbon, and HWP). The contribution of annual carbon stock changes ΔC(ti) between timest0 and tn to RF is:
where RFi is radiative forcing at time ti, f(t) is lifetime function of CO2 in the atmosphere describing the removal of CO2 in the atmosphere (IPCC, 2007):
where t is time, aj,j = 1, 2, 3 are weights, and τj are time constants (IPCC, 2013, cf. Supplementary Table S4 for parameter values).
We assumed the changes of management of the whole production forestry area of Finland to have only marginal change for the RF of the Earth. Thus, we converted CO2 to RF as the product between calculated CO2 response curve and constant radiative efficiency of CO2 defined as the radiative forcing per kg increase in atmospheric burden of the gas (Frolking and Roulet, 2007; Arvesen et al., 2018). This way we assumed in the calculation constant atmospheric background conditions and time-invariant radiative efficiency.
The RF values are then expressed relative to the initial state of the forest, i.e., RF = RFi – RF0, where i is simulation year running from 1 to tn = end year of simulation. Finally, baseline RF is subtracted from the RF values of the scenarios
where and is cumulative radiative forcing in scenario s and baseline, respectively.
We computed the avoided CO2 emissions related to wood utilization separately for harvested sawlogs and pulpwood substitution in a Consequential Life Cycle Analysis framework (CLCA, Helin et al., 2013). For sawnwood, the displacement factors (DF) of individual studies listed in Sathre and O’Connor (2010) were used as data points to compute the average values for avoided carbon emissions with the restriction that only those studies that dealt with whole buildings or the construction sector were included. Data values that referred to individual products were discarded as they were considered to be case dependent (see Supplementary Material). Then, the arithmetic average of DFs was 2.1. To arrive to factors applicable to raw material carbon amounts, this was multiplied by the recovery rates of mechanical wood products (sawnwood and plywood) (Table 1, Karjalainen et al., 1994). This yielded average DF values of 0.913, 0.905, and 0.819, for sawnwood of Scots pine, Norway spruce and silver birch, respectively. For determining the uncertainty range of the values, standard errors of 0.566, 0.560, and 0.507 were used for each species, respectively, calculated from the individual studies reported in Sathre and O’Connor (2010). For avoided emissions due to pulpwood use we used a common value of 0.695 across species (Pingoud et al., 2010). This value includes the energy substitution in processes of forest industry because of using side products like black liquor. Another fossil alternative to pulpwood could also be plastic but this was not applied due to the lack of information about substitutability at large scale (Pingoud et al., 2018), although some analysis of recycled wood potential for substituting plastics, e.g., in package sector has been done (e.g., Sommerhuber et al., 2015). A notable source of uncertainty in this substitution analysis is that these substitution computations rely on the comparison of forest management alternatives that lead to changes in wood material produced. An associated assumption in these CLCA is that the societal need for materials/energy is fixed and the additional production is used for substituting fossil materials/energy. This assumption also means that when less wood is harvested, more fossil energy/products are required and emissions in other sectors will increase.
We calculated the RF change due to avoided emissions using the same methodology as for forest carbon sequestration (Eqs 1 and 2, see section “Estimation of Annual Carbon Balance and the Radiative Forcing Effect of Carbon Stock Change”).
Forest albedo were estimated for an area located in central Finland based on MODIS MCD43A3 blue-sky albedos (Schaaf et al., 2002) and forest resource data produced by the Natural Resources Institute Finland (Tomppo et al., 2008). A total of 2180 MODIS pixels located completely on forest land were used. Regression models were used to estimate tree species specific forest albedos for different volume thresholds utilizing information on the fractional covers of different forest types within the MODIS pixels (Kuusinen et al., 2013, 2014). The land cover data were divided into five components: clear cut (growing stock ≤ 5 m3 ha–1), young stand (pine or spruce forest with growing stock > 5 but < 60 m3 ha–1), pine forest (growing stock ≥ 60 m3 ha–1), spruce forest (growing stock ≥ 60 m3 ha–1) and deciduous broadleaved forest (growing stock > 5 m3 ha–1). Monthly means of component albedos (Figure 2) were calculated for February–September, but due to low solar zenith angles during autumn and winter, there were no good quality albedo retrievals available from October to January. For December and January, the same albedo values were used as for February; October was treated as equivalent to September and November albedo was estimated through linear interpolation between the values of October (September) and December (February).
Figure 2. Monthly mean albedo estimated from multi-source national forest inventory data (MS-NFI) and MODIS satellite images.
Species-specific albedos for Scots pine and Norway spruce were assumed to follow a stepwise function during the forest rotation. Albedo was the highest in open clear cuts and linearly decreased in Scots pine and Norway spruce stands until the growing stock reached 60 m3 ha–1. Deciduous broadleaved species (mainly birches) albedo was noted to be insensitive to changes in growing stock, so it was estimated as one value for the total rotation (from stand volume > 5 m3 ha–1). A more detailed description of the albedo estimation can be found from the Supplementary Material of Matthies et al. (2016).
The resulting albedo values were translated into net shortwave radiation at the top of the atmosphere using ECHAM5 radiative transfer model (Roeckner et al., 2003) as explained in Matthies et al. (2016) and the stand level results were scaled up in the same age class and site fertility platform as in the carbon stock change analysis.
The uncertainty of the albedo impact on RF was estimated to be 25%. This albedo uncertainty includes the differences between vegetation types and in radiative transfer. The uncertainty estimate for albedo was derived based on the relative differences of the uncertainty estimates of albedos of different forest classes to those of clear cut (used as a reference here). This assumes that changes in RF are directly proportional to changes in albedo. The analysis is based on published values of albedo and their errors (Table 1 in Kuusinen et al., 2013).
We modeled the impacts of BVOC emissions on SOA concentrations using the SOSAA model (Boy et al., 2011; Zhou et al., 2014). From the modeled size distributions, the radiative forcing due to aerosol-radiation interactions (scattering of solar radiation, see details in Supplementary Material) and due to aerosol-cloud interactions (the cloud albedo effect, Kurtén et al., 2003, see details in Supplementary Material) were calculated.
SOSAA is a one-dimensional chemical-transport model (Boy et al., 2011; Zhou et al., 2014). The most important supporting equations are provided in Boy et al. (2011), Zhou et al. (2014) and updates and validations are presented in Mogensen et al. (2015). In brief, the meteorological transport in the model is based on the coupled plant-atmosphere boundary layer model SCADIS (Sogachev et al., 2002, 2012; Sogachev and Panferov, 2006), while the aerosol dynamics module is based on the University of Helsinki Multicomponent Aerosol model (UHMA, Korhonen et al., 2004). The chemistry is calculated using the Kinetic PreProcessor (KPP) (Damian et al., 2002) with chemical reaction equations from the Master Chemical Mechanism2. SOSAA includes a modified version of MEGAN 2.04 (Guenther et al., 2006; Smolander et al., 2014) which calculates the emissions of organic vapors from the forest canopy. The estimated emissions are very dependent on meteorological factors (in particular temperature and light), foliage mass and leaf area, and the potential to emit individual organic compounds is highly specific for individual tree species. For our simulations, we utilized continuous chamber measurements of BVOC emission rates obtained from the SMEAR II site together with literature values of BVOC emission potentials for different species (Hakola et al., 1998, 2001, 2003, 2006, 2017; Bäck et al., 2012). Information on BVOC emissions from Norway spruce was much lower than that from Scots pine, and therefore simulations of spruce include more uncertainty than those of pine. Laboratory measurements of BVOC emissions from birch are rare (e.g., Yli-Pirilä et al., 2016; Hakola et al., 2017) and field measurements are even more rare (Hakola et al., 1998, 2001). Very recent continuous field measurements (manuscript in preparation) obtained after completion of the SOSAA simulations and radiative forcing calculations, suggest that the canopy scale emissions of monoterpenes from silver birch based on Hakola et al. (2001) could be highly overestimated. Thus, we reduced the number and size of aerosol particles from birch stands (see details in Supplementary Material).
The SOA effects were calculated for three different ages of pine, spruce, and birch forest stands (Table 2 and Supplementary Figure S10) and the values over the stand development were interpolated from those stages. The foliage biomass of each stand age class was used as a parameter in BVOC modeling and the foliage mass throughout the season changed based on leaf are index (LAI) observations at SMEAR II. The changes in direct RF from aerosols were calculated between stand ages of each forest type and species combination (Table 2). The method used in calculation is based on the Supplementary Material of Paasonen et al. (2013) and on the article by Lihavainen et al. (2009).
Table 2. The characteristics of stands used in modeling the emissions of organic vapors (BVOC) by MEGAN2.04 and the formation of secondary organic aerosols (SOA) by SOSAA.
The indirect effect dominates the aerosol radiative effects (>95% of total aerosol effect in our analysis). We calculate the indirect effect using the method of Kurtén et al. (2003). From the reanalyzed low cloud cover fraction (Uppala et al., 2005; Dee et al., 2011) of from the European Centre for Medium-Range Weather Forecast (ECMWF3) we calculated the monthly mean cloud cover fraction. The changes in indirect RF from aerosols were calculated similarly as for direct aerosol (reflection) effect between stand ages of each forest type and species combination (Table 2, see section “Stand Level Radiative Forcing Dynamics of CO2, SOA, Albedo and Substitution” in Supplementary Material). The results in both direct and indirect forcing calculations were averaged for annual values in W m–2, which represents the total amount of the radiative effect for 1 square meter of forest. Stand level aerosol RF was scaled up for Finnish forests in the same age and site fertility platform as carbon stock changes and albedo.
The uncertainty range of SOA RF was adopted from the study by Spracklen et al. (2008). They found a RF range from −1.6 to −6.7 Wm–2 for the SOA effect for the difference between no forest and closed canopy. Our simulations gave an average value for the difference between open area and forest canopy of −3.8 Wm–2 when the current species distribution in Finland was assumed. This yielded an average uncertainty range of ± 70% for the SOA effect. Although the whole uncertainty range of aerosols is probably much larger, we deemed that showing this range with an explicit logic behind it still provides a better indication of the limitations of our current understanding than just omitting the uncertainty estimation because we are unable to quantify the possible error sources.
Increasing harvests from baseline (65% of CAI) decreased total carbon sink (change in carbon in trees, soil and HWPs) at least for 50 years in our analysis (Figure 3).
Figure 3. The annual difference of the carbon sink (CO2, Mtn year– 1) between each scenario and the baseline (65% of CAI), i.e., , where = sink of scenario S at year i and = sink of baseline scenario at year i. In the sink, we included CO2 stored in different tree compartments, soil carbon, and harvested wood products (HWP). Negative values denote larger carbon sink than in baseline.
When the change in carbon sink was coupled with other biosphere responses, surface albedo and aerosols, decreasing harvests from the baseline produced the largest cooling effect during 50 years (Figure 4A). Also continuing with baseline harvest level produced a clear cooling effect, due to harvest level being lower than CAI, but cumulative RF saturated before the end of the 50 year period in baseline scenario.
Figure 4. The annual difference of the global radiative forcing (RF) between each scenario and the baseline (65% of CAI), in the current climate (cf. Eq. 3) (A) excluding avoided emissions from substitution and (B) including avoided emissions (the total impact). Different colors represent varying harvest levels given as a percentage of present CAI in Finland and the shaded area represents the estimated min-max range (see text).
When the avoided emissions gained from the use of wood products and energy instead of fossil based energy and materials were also considered the differences in the RF impact between harvest scenarios were reduced greatly. The cooling impact of substitution increased along the larger harvests but depended greatly on the DFs (Figure 4B).
Accounting for all the factors, the RF responses of the two lowest harvest scenarios were within uncertainty range, although in terms of mean response the 50% harvest scenario produced largest cooling. Harvest scenarios with removals higher than 80% of CAI produced warming effect compared with baseline scenario, while lower scenarios than that had uncertainty ranges which covered baseline. The large uncertainty range highlights the effect of the selected DFs. The higher substitution and albedo associated with a higher harvest rate largely compensated for the lower direct cooling effect of SOA and CO2 so that the net difference between these harvest levels (50–80%) was small within considered time span (Figure 4B).
Importantly, the differences between harvesting scenarios were almost entirely due to the different carbon impacts, because the surface albedo and SOA effects counterbalanced each other at country level (Figure 5).
Figure 5. Contribution of different factors to the average change of global radiative forcing (RF) in 50 years due to changes in Finnish forests in different harvest scenarios, expressed as difference from the baseline (65% CAI). The analysis was done at current climate. CO2 includes carbon sequestered in trees, soil and wood products, ‘Albedo’ refers to the change in surface albedo, ‘SOA’ equals both direct and indirect effects of secondary organic aerosols, ‘Subst’ equals avoided emissions due to substitution of product materials and energy. Positive values indicate warming, and negative values cooling effect. The error bars indicate the estimated min-max range.
Stemwood increment decreased clearly in the low harvest scenario (Supplementary Figure S1) as harvests were much lower than CAI, leading to aging of forests (Supplementary Figure S2). Within the 50-year period, the growing stock volume clearly increased in all harvest scenarios with harvests lower than CAI, and in contrast, declined in the two highest harvest scenarios (Supplementary Figure S3).
In the low harvest scenario, the relative share of clear-cut areas and forests at sapling stage decreased (Supplementary Figure S2). As BVOC production and thus the SOA effect was lower in these stands than in older forest, accounting for the SOA effect enhanced the differences in RF between harvesting scenarios. The RF of two highest harvest scenarios approach each other toward the end of the simulation period because harvests larger than the annual increment (130%) could only be maintained for a restricted time. After that the actual harvest level first dips down to 80% of CAI, then starts to increase again (Supplementary Figure S4).
At the stand level, the combination of all effects resulted in the large negative RF over the rotation. Both the 50-year average RF (Table 3) and average of one rotation (Figure 6, different species have different rotation length), were increasingly negative with increasing site fertility. In each conifer forest type case, the cooling SOA effect slightly surpassed the warming albedo effect (Figure 6). In silver birch growing on fertile herb-rich site, both albedo and SOA effects had a cooling effect over stand rotation.
Table 3. The 50-year average local radiative forcing (RFa = RFT/100, W m–2) in the current climate of the three most common forest site types and species combinations in Finland by separate influences CO2 sequestration in forest stands and wood products (CO2), surface albedo (Albedo), aerosols (SOA) and avoided emissions from the use of wood products (Substitution) and in decreasing order of site productivity.
Figure 6. Accumulated radiative forcing over one rotation (RFT, T = i…t in Eq. 1) in (A) Norway spruce fertile mesic stand, (B) Scots pine infertile sub-xeric stand, and (C) silver birch herb-rich stand. The RF is defined here as a local change in the radiative energy balance (W m– 2) at the tropopause per one square meter in response to the change of the state of 1 m2 of forest area, negative values denote the cooling effect and positive values the warming effect.
In this study, we combined for the first time the forest harvest level effect on carbon sequestration in forests and wood products, the surface albedo of forests, the direct and indirect forcing of secondary organic aerosols and the avoidance of fossil emissions by product substitution. We did not analyze the climatic impact of forest management (i.e., no comparison to ‘no management’ as a baseline) but rather the difference between alternative harvest scenarios. However, our results show that the effects of forest management on SOA formation should be included in the analyses trying to deduce the net climate impact of forest use. Naudts et al. (2016) concluded that forest management favoring conifers has contributed to climate warming since the 1750s, particularly in the European temperate forest. Their conclusion was based on carbon sequestration together with albedo and evapotranspiration impacts, but did not include the SOA effects. Some studies have concluded that in boreal zone surface albedo effect may counterbalance the decrease of carbon stocks due to increased forest utilization (e.g., Unger, 2014). In our analysis, SOA and albedo largely counterbalanced each other. If compared with the studies where only carbon and albedo effects have been considered, the inclusion of the rarely considered SOA effects enforces the view that the lower the harvest, the more climatic cooling boreal forests provide.
In the light of our results, the combination of larger harvests than baseline (65% of CAI) and an increase in wood use to products with low DFs, such as pulp or bioenergy, is not beneficial from a climate change mitigation viewpoint in Finland at least during a time span of 50 years. The differences between the lowest and highest harvest scenarios (50 vs. 130% of CAI) led to a net global RF difference of ca 0.004 W m–2 over 50 years without accounting for the avoided emissions (Figure 4A). This difference was reduced to ca. 0.002 W m–2 when we included the avoided emissions (Figure 4B). The DF value of all harvested wood should be ∼1.7 tC tC–1 for other harvest scenarios to reach climate neutrality within 50 years (ΔRF = 0) in comparison with the baseline scenario (Figure 7). This is somewhat lower value than found by Seppälä et al. (2019) (2.0 -2.4 tC tC-1) but also clearly higher than their estimation of the current average DF value, < 1.1 tC tC–1, of Finnish forest industry. This result highlights the importance of striving for long-living wood products for ensuring higher DFs and more efficient mitigation. Also the possibility to combine short-living wood products, especially forest based bioenergy, with carbon capture and storage technology (CCS) would change the role of substitution substantially in analyses like this study and greatly enhance forest based climate mitigation.
Figure 7. The annual difference of the global radiative forcing (RF) between baseline (65% of CAI) and each scenario. Negative RF values indicate cooling impact compared with the baseline. Analysis was done in the current climate with the assumption that all harvested wood has a displacement factor (DF) value of 1.8 or 2.0 tC tC– 1. Climate neutrality (in terms of RF) of each scenario compared with the baseline reached at the year they cross the x-axis.
The results of this study demonstrate that deciding on a forest harvest scheme for producing climate mitigation requires a holistic consideration of all associated effects, and that appropriate management of boreal forests can have an important climate change mitigation role. Our results, in support of previous similar findings (e.g., EASAC, 2017; Dugan et al., 2018), should caution policy makers who are emphasizing the increased utilization of forest biomass for short-living products and bioenergy as a measure to mitigate climate change. Within the analyzed 50 years timeframe, the largest mitigation effects emerged in the scenarios where carbon stocks increased because of longer rotations. In these scenarios, also the commodities portfolio shifted slightly to longer-lived products, which was indicated by a higher log:pulpwood ratio of the growing stock (Supplementary Figure S5) and lower pulpwood:sawnwood ratio of HWP and therefore higher DFs. The 50 years average ratio, however, varied only from 26% in the 50% harvest scenario to 30% in the 130% scenario (Supplementary Figure S7), and thus, had only marginal effect on our results. Please see further analysis of commodities portfolio effect on RF in Supplementary Figure S8.
The resulting warming effect due to increasing harvests from baseline can be viewed as a climate debt that results from forest utilization (Mitchell et al., 2012; Schulze et al., 2012; Seppälä et al., 2019). It may be counterintuitive that such a debt emerges, because the harvested forests do regenerate and start to sequester carbon again. However, the recovery of carbon stocks after regeneration has a significant delay and therefore, also at the landscape level, forest carbon stocks and SOA formation decrease due to increased harvesting, as do their associated RF effects.
To estimate the net differences between forest harvest scenarios, we must also consider how the forest products as a whole influence the climate, in relation to alternative products and production chains. When we considered the avoided emissions from product and energy substitution, both the modest increase (80% scenario) and modest decrease (50%) were within the used uncertainty range for the baseline scenario. At the time of harvest, carbon stored in the forest ecosystem is partly lost to the atmosphere and partly transferred to HWPs and, assuming a given demand of products and full substitutability (Helin et al., 2013), fossil fuel emissions are reduced as wood products act as substitutes. Numerous studies agree that in the long run the avoided emissions of substitution inevitably lead to climate cooling as they accumulate over time (Sathre and O’Connor, 2010). A coefficient of variation ± 62% was used here for sawn timber based on the reported values of Sathre and O’Connor (2010). With high values of DFs (i.e., replacement of high emission products with wood) the uncertainty ranges of 50 and 80% scenarios overlapped. Low substitution values required low harvest scenarios for maximum cooling. Extremely intensified forest harvests (100 and 130% of CAI), with an associated decrease in harvested wood dimensions led to clear reductions in the associated cooling effect (Figure 4). This is mainly due to reduced forest carbon sink and also slightly increased proportion of pulp and energy wood having smaller DFs relative to those of long-lived timber products. Analyses including avoided emissions heavily depend on the prevailing production technologies and are highly sensitive to the DFs (Hurmekoski et al., 2018). While the direct impacts of forest on atmosphere depend on biosphere processes, the substitution effect can lower quite drastically with technological innovations in products to be substituted for, without any changes in the forest sector. Within the time span of our analysis, a tightening climate policy should lead to a lower C intensity of the energy system, leading to lower substitution benefits of biomass (Pingoud et al., 2012). Also, the so-called rebound effect may reduce the amount of the avoided emissions, e.g., the increased use of bioenergy lowers the fossil crude oil price which increases the use of fossil oil (Smeets et al., 2014).
A full-blown CLCA includes associated effects on industrial activity and product markets. In the case of Finnish forestry, the coverage should be global as Finland accounts in the main product groups for 6–8% of the global exports and 15–20% of European exports (Natural Resources Finland, 2019; UNECE, 2019). A market-level forest industry and trade analysis is beyond the scope of the present study. Some existing studies provide guidance to the magnitude of market-level adjustments to changes in the production of one region (in our case Finland). According to Kallio et al. (2018), a reduction in European roundwood production would be compensated for 79% by increasing roundwood production elsewhere in the world. Similar levels of leakage rates have been reported by Nepal et al. (2013) and Hu et al. (2014). The leakage rates would naturally depend on the availabilities of roundwood and production capacities of different products as well as the geographical locations of production and markets. Overall, the studies indicate that the leakage effects can be significant.
Our analysis could be improved by accounting for forest disturbances, which have been projected to increase already during the next few decades in boreal forests (Seidl et al., 2017). This effect may limit the possibilities of increasing forest carbon storages as a mitigation strategy. In many boreal regions, large outbreaks of insects and increases in wildfire emissions have already been seen while in Finland natural disturbance rate has been low in recent decades. In this sense, our results are very Finland specific. These risks should be acknowledged and properly accounted for in improved forest management aiming for increased ecosystem carbon stocks (e.g., Griscom et al., 2017; Nabuurs et al., 2017; Erb et al., 2018). We also did not include changes in tree species distributions, share of mixed forests or forest structural changes in our forest management scenarios. Studies have indicated these changes to improve resilience and adaptation of forests (Brang et al., 2014; Matthies and Valsta, 2016). Also our approach in the description of state change (see section “Estimation of the Forest Development”) of Finnish forests due to harvest scenarios was simplified and thus creates uncertainty of how robust the dynamics of forests in different scenarios are. Especially the increment of forests in high harvest scenarios seem to deviate from some other model projections showing decreasing increment in harvest levels approaching CAI (Kalliokoski et al., 2019, Supplementary Figure S1). The observed increment dynamics may be a consequence of not including all site fertility classes. However, adapting our results of forest increment toward results of other models would increase the difference between harvest scenarios of this study even further and thus, we regard our results to be conservative.
This same challenge also concerns peatlands which we were not able to include because so far peatland BVOC measurements are only available from ground vegetation, not from trees. In addition to CO2, methane (CH4) and nitrous oxide (N2O) are strong greenhouse gases, emitted in large quantities mainly from organic soils. Land-use change by drainage increases the emissions of N2O and decreases that of CH4 from organic soils. We assumed that all the production forests in Finland lie on mineral soil. In reality, 4.6 million ha, i.e., 25% of the 18.2 million ha of production forests are on peatlands, and the great majority of these, 4.1 million ha, are on drained peatlands (Luke statistics database, 2019). The fertile drained sites, which correspond to herb-rich and mesic site types in mineral soils, lose about 200 g CO2 m–2 in peat decomposition annually (Ojanen et al., 2014). At country level the loss was reported to be 4.3 Mt/year CO2 in 2017. This is in a big contrast to the mineral soils, where C accumulates at the rate of 10 Mt/year. In our analysis, soil C dynamics were modeled assuming mineral soils, which means that soil CO2 balance is very significantly biased for 25% of the forest area. The emissions of all greenhouse gases are dependent on soil fertility and water table depth so that higher fertility and deeper water table increase the emissions overall (Ojanen et al., 2014). Water table depth is in turn affected by tree stand volume. Thus the different harvesting levels affect the GHG emissions in complicated ways, which were not included in our analyses. The tree stand growth rates in drained peat and mineral soils are similar in corresponding site types, which means that the modeling of tree stand C stocks is likely less biased than that of the soil C stocks. However, at the moment peatlands are not harvested as much as the mineral soil forests are, because of higher logging costs and lower profitability. If harvesting levels were to be increased in Finland, it would shift the balance of forest harvests from mineral soils toward peat soils. Increased use of peatlands would mean increased need for forest operations, including remedial drainage, soil preparation and fertilizations, which are all prone to increase C loss from soils due to increased decomposition rates (Minkkinen et al., 2008; Ojanen and Minkkinen, 2019). This would have a large effect on the overall impact of forestry – higher levels of harvests would mean even higher RF compared to the lower levels, if peatlands were included in the analysis. In other words, it is beneficial for the climate change mitigation to keep harvests at a level that will not increase fellings in drained peatlands.
Our results suggest a weaker albedo impact than indicated previously (Betts, 2000). However, estimates of the albedo effects of deforestation or disturbance of boreal forests vary widely in the literature (Betts, 2000; O’Halloran et al., 2012; Bright et al., 2014; Alkama and Cescatti, 2016). Inaccurate estimates of forest structure have been shown to cause large spread in snow-covered time albedos in climate models (Wang et al., 2016). Likewise, different results from empirical studies may reflect differences in forest structure and climatic conditions, and uncertainties in, e.g., inventory data. For example, O’Halloran et al. (2012) derived albedo values from MODIS data for a time series of coniferous stands following fire and obtained winter albedo values that were lower for mature forests and larger for open stands than those used in our study. Several sources cause uncertainty to the albedo estimates used in this study. These include errors in the source data, i.e., forest resource estimates and MODIS albedos, and in the estimation of albedos for different types of forests using these data. It has been suggested that the real differences between albedos of different land cover or forest types are larger than those estimated using similar approach than here (Kuusinen et al., 2013, 2014).
Our results strongly suggest that the radiative effects of SOA need to be included in a full assessment of the climate impacts of forest management, however, the conclusions are conditional onto our current understanding of SOA formation. Numerous atmospheric processes and feedback mechanisms influence the RF of aerosols and, e.g., the effect of cloud altitude was not accounted for in our simulations. In our results, the average RF values due to SOA impacts are in the middle range of previously estimated values (Spracklen et al., 2008), which is reassuring and gives confidence to the estimates. Our method has the clear advantage over the GCMs in many aspects related to the aerosols. First of all, while the dynamics of the aerosol particle population (coagulation, self-coagulation, growth, and scavenging) are very roughly considered in GCMs, in SOSAA their treatment is robust (Zhou et al., 2014). Secondly, in SOSAA the background aerosol concentrations and size distributions are taken from the ambient measurements for each modeled night, and thus the modeled changes in CCN concentrations are compared to a realistic baseline. The current state-of-the art GCMs are typically not capable of producing similar background aerosol concentrations as observed (e.g., Bergman et al., 2012; Xausa et al., 2018), and thus their sensitivity toward changes in concentrations of condensable vapors and subsequent changes in CCN concentration can be questioned. And finally, the multiple reasons for the variation of aerosol concentrations causes a lot of noise for the signal. Thus, applying a coupled vegetation-climate GCM for a relatively small area such as Finland would require various long model runs in order to be able to distinguish the signal, as an average of all the runs, from the noise.
The main disadvantage of our method in comparison to GCM runs is the three-dimensional nature of the aerosol-cloud interactions. Even if the formation of particles large enough to act as cloud condensation nuclei is caused by the growth of aerosol particles due to BVOC emissions from Finnish forests, the activation of the CCN, i.e., the cloud formation can only take place when the air mass containing these CCN is uplifted high enough (in a low-pressure system or for some other reasons). The true impact on radiative forcing will be very different depending on where and above which kind of Earth surfaces the cloud droplets will be transported. Additionally, our calculation cannot take into account the feedbacks, such as the altered BVOC emissions due to the increased cloud albedo.
When comparing the aerosol forcing to the other forcing mechanisms, it is important to acknowledge that the forcing from aerosol-cloud interactions does not include interactions other than the cloud albedo effect, typically neither in analytical calculations nor in GCM simulations. This naturally causes a lot of uncertainties, as demonstrated in IPCC AR5 for all anthropogenic activities. Also in aerosol-radiation effects there are significant uncertainties, e.g., in terms of radiative impacts of SOA formation onto absorbing black carbon aerosol. Nevertheless, we find it is necessary to use an order of magnitude estimate of the forcing in order to draw an image of the relative importance of different contributors to the total RF impacts.
We performed our analysis in current climate. All uncertainties would increase notably if on-going environmental and climate changes were accounted for. For illustrating possible effects we made an analysis where temperature increased 2,1°C, precipitation increased 17% and atmospheric CO2 concentration reached 680 ppm at the end of simulation (see details in Supplementary Material) Due to the changed climatic conditions, the relative importance of carbon sequestration decreased due to more rapid carbon turnover, whereas the BVOC emissions and SOA formation simultaneously gained importance (Supplementary Figure S13). In particular, the BVOC emissions are exponentially increasing with temperature (Guenther et al., 1993) and would likely lead to significantly higher new particle formation rate than in current climate (Paasonen et al., 2018).
The approximate difference between the simulated lowest and highest harvest scenarios for Finnish forests without the substitution effect up to year 2050 reached 0.17% of the global anthropogenic RF in 2011 (0.004 out of 2.29 Wm–2, IPCC, 2013). While this number is low, it is important to note that Finland covers approximately 2% of the total boreal forest area. Although most boreal forests are currently not managed at the intensity found in the Nordic region, more than half of the biome is commercially utilized. Accordingly, the influence of the selected management could be around 3% (about −0.08 Wm–2) of the current anthropogenic RF in 50 years in the current climate, and potentially even higher with climatic warming. During last years the harvest removals in Finland have increased clearly from the baseline we used here (record high removals 78 million m3 in 2018) and wood product portfolio has shifted toward more short-living products, mainly pulp. This indicates that current land use sector policies do not support enhanced forest carbon sinks but boost forest biomass use for short-living products and bioenergy. Policies also do not account for biophysical effects. The results of this study can support strategic planning and decision-making in forest policy. If carbon retention time in wood products could be notably increased then the faster carbon turnover due to increased growth combined with high harvest levels could produce climate mitigation within a relevant time span. Current situation where only < 20% of harvest removals in Finland are used for wood products of long lifetime emphasizes the role of forest carbon, i.e., biosphere dynamics. In this situation, the inclusion of SOA further emphasizes the result that the lower the harvests, the more climatic cooling boreal forests provide. Innovative new long-living wood products should form the main share of pulpwood removals if effective climate mitigation with increased wood use is pursued.
The raw data supporting the conclusions of this article will be made available by authors on request, without undue reseravation.
TK had the responsibility for the writing of the article and created platform for the calculation of country level results, combined all effects in terms of radiative forcing, calculated all final results and produced figures and tables. TK, AM, FM, and MP produced the carbon dynamics of trees, soil and harvested wood products. DT, AV, and JB produced BVOC estimates. PZ, LZ, MB, and MK produced aerosol effect on basis of BVOC estimates. FB and NK produced the albedo effect. LV produced the decay function of wood products and their substitution factors. KM coordinated the work and participated all calculations. All authors participated in planning the study and commenting the manuscript.
The leader of the project Professor Eero Nikinmaa passed away before this study was finalized. We dedicate this work to his memory.
The data in this work was collected and analyses were done mostly in the Research Project “Optimizing forest management and conservation to account for multiple interactions with the climate” (HENVI FOREST), funded by the University of Helsinki. We acknowledge the support and data received to this work from Helsinki University Centre for Environment, Natural Resources Institute Finland, Finnish Meteorological Institute, Academy of Finland FCoE “The Centre of Excellence in Atmospheric Science – From Molecular and Biological processes to The Global Climate,” Integrated Carbon Observation System (ICOS), Nordic Centers of Excellence CRAICC and Defrost, EU Life C (LIFE09 ENV/FI/000571), EU I3 ExpeER, Finnish Academy project #140781, ACTRIS (no. 328616), Academy professorship (no. 302958) and Jane and Aatos Erkko Foundation (project title “Quantifying carbon sink, CarbonSink C and their interaction with air quality”). DT was additionally supported by the Academy of Finland (no. 307957).
The authors declare that the research was conducted in the absence of any commercial or financial relationships that could be construed as a potential conflict of interest.
The earlier version of this manuscript has been released as a preprint at Biogeosciences Discussions (doi: 10.5194/bg-2017-141; Nikinmaa et al., 2017).
The Supplementary Material for this article can be found online at: https://www.frontiersin.org/articles/10.3389/ffgc.2020.562044/full#supplementary-material
Albrecht, B. A. (1989). Aerosols, cloud microphysics, and fractional cloudiness. Science 245, 1227–1230. doi: 10.1126/science.245.4923.1227
Alkama, R., and Cescatti, A. (2016). Biophysical climate impacts of recent changes in global forest cover. Science 351, 600–604. doi: 10.1126/science.aac8083
Arvesen, A., Cherubini, F., del Alamo Serrano, G., Astrup, R., Becidan, M., Belbo, H., et al. (2018). Cooling aerosols and changes in albedo counteract warming from CO2 and black carbon from forest bioenergy in Norway. Sci. Rep. 8:3299.
Bäck, J., Aalto, J., Henriksson, M., Hakola, H., He, Q., and Boy, M. (2012). Chemodiversity of a Scots pine stand and implications for terpene air concentrations. Biogeosciences 9, 689–702. doi: 10.5194/bg-9-689-2012
Bergman, T., Kerminen, V.-M., Korhonen, H., Lehtinen, K. J., Makkonen, R., Arola, A., et al. (2012). Evaluation of the sectional aerosol microphysics module SALSA implementation in ECHAM5-HAM aerosol-climate model. Geosci. Model Dev. 5, 845–868. doi: 10.5194/gmd-5-845-2012
Betts, R. A. (2000). Offset of the potential carbon sink from boreal forestation by decreases in surface albedo. Nature 408, 187–190. doi: 10.1038/35041545
Boy, M., Sogachev, A., Lauros, J., Zhou, L., Guenther, A., and Smolander, S. (2011). SOSAA – a new model to simulate the concentrations of organic vapours and sulphuric acid inside the ABL- Part 1: model description and initial evaluation. Atmos. Chem. Phys. 11, 43–51. doi: 10.5194/acp-11-43-2011
Brang, P., Spathelf, P., Larsen, J. B., Bauhus, J., Bončìna, A., Chauvin, C., et al. (2014). Suitability of close-to-nature silviculture for adapting temperate European forests to climate change. Forestry 87, 492–503. doi: 10.1093/forestry/cpu018
Bright, R. M., Antón-Fernández, C., Astrup, R., Cherubini, F., Kvalevåg, M., and Strømman, A. H. (2014). Climate change implications of shifting forest management strategy in a boreal forest ecosystem of Norway. Glob. Change Biol. 20, 607–621. doi: 10.1111/gcb.12451
Charlson, R. J., Schwartz, S. E., Hales, J. M., Cess, R. D., Coakley, J. A. Jr., Hansen, J. E., et al. (1992). Climate forcing by anthropogenic aerosols. Science 255, 423–430. doi: 10.1126/science.255.5043.423
Chen, J., Ter-Mikaelian, M. T., Yang, H., and Colombo, S. J. (2018). Assessing the greenhouse gas effects of harvested wood products manufactured from managed forests in Canada. Forestry 91, 193–205. doi: 10.1093/forestry/cpx056
Damian, V., Sandu, A., Damian, M., Potra, F., and Carmichael, G. R. (2002). The kinetic preprocessor KPP – a software environment for solving chemical kinetics. Comput. Chem. Eng. 26, 1567–1579. doi: 10.1016/s0098-1354(02)00128-x
Dee, D. P., Uppala, S. M., Simmons, A. J., Berrisford, P., Poli, P., Kobayashi, S., et al. (2011). The ERA-interim reanalysis: configuration and performance of the data assimilation system. Q. J. R. Meteorol. Soc. 137, 553–597.
Dugan, A. J., Birdsey, R., Mascorro, V. S., Magnan, M., Smyth, C. E., Olguin, M., et al. (2018). A systems approach to asses climate change mitigation options in landscapes of the United States forest sector. Carbon Balance Manage. 13:13.
EASAC (2017). Multi-Functionality and Sustainability in the European Union’s Forests. (Brussels: EASAC), 43.
Erb, K., Kastner, T., Plutzar, C., Bais, A. L. S., Carvalhais, N., Fetzel, T., et al. (2018). Unexpectedly large impact of forest management and grazing on global vegetation biomass. Nature 553, 73–76. doi: 10.1038/nature25138
Forest Europe (2015). State of Europe’s Forests 2015. Available online at: https://foresteurope.org/wp-content/uploads/2016/08/summary-policy-makers.pdf (accessed January 28, 2019).
Frolking, S., and Roulet, N. T. (2007). Holocene radiative forcing impact of northern peatland carbon accumulation and methane emissions. Glob. Change Biol. 13, 1079–1088. doi: 10.1111/j.1365-2486.2007.01339.x
Griscom, B. W., Adams, J., Ellis, P. W., Houghton, R. A., Lomaxa, G., Mitevad, D. A., et al. (2017). Natural climate solutions. Proc. Natl. Acad. Sci. U.S.A. 114, 11645–11650.
Guenther, A., Karl, T., Wiedinmyer, C., Palmer, P. I., and Geron, C. (2006). Estimates of global terrestrial isoprene emissions using MEGAN (Model of Emissions of Gases and Aerosols from Nature). Atmos. Chem. Phys. 6, 3181–3210. doi: 10.5194/acp-6-3181-2006
Guenther, A., Zimmermann, P., and Wildermuth, M. (1993). Natural volatile organic compound emission rate estimates for U.S. Woodland landscapes. Atmos. Environ. 28, 1197–1210. doi: 10.1016/1352-2310(94)90297-6
Hakola, H., Laurila, T., Lindfors, V., and Rinne, J. (2001). Variation of the VOC emission rates of birch species during the growing season. Boreal Environ. Res. 6, 237–249.
Hakola, H., Rinne, J., and Laurila, T. (1998). The hydrocarbon emission rates of tea-leafed willow (Salix phylicifolia), silver birch (Betula pendula) and European aspen (Populus tremula). Atmos. Environ. 32, 1825–1833. doi: 10.1016/s1352-2310(97)00482-2
Hakola, H., Tarvainen, V., Bäck, J., Ranta, H., Bonn, B., Rinne, J., et al. (2006). Seasonal variation of mono- and sesquiterpene emission rates of Scots pine. Biogeosciences 3, 93–101. doi: 10.5194/bg-3-93-2006
Hakola, H., Tarvainen, V., Laurila, T., Hiltunen, V., Hellén, H., and Keronen, P. (2003). Seasonal variation of VOC concentrations above a boreal coniferous forest. Atmos. Environ. 37, 1623–1634. doi: 10.1016/s1352-2310(03)00014-1
Hakola, H., Tarvainen, V., Praplan, A. P., Jaars, K., Hemmilä, M., Kulmala, M., et al. (2017). Terpenoid and carbonyl emissions from Norway spruce in Finland during the growing season. Atmos. Chem. Phys. 17, 3357–3370. doi: 10.5194/acp-17-3357-2017
Heinonen, T., Pukkala, T., Mehtätalo, L., Asikainen, A., Kangas, J., and Peltola, H. (2017). Scenario analyses for the effects of harvesting intensity on development of forest resources, timber supply, carbon balance and biodiversity of Finnish forestry. For. Policy Econ. 80, 80–98. doi: 10.1016/j.forpol.2017.03.011
Helin, T., Sokka, L., Soimakallio, S., Pingoud, K., and Pajula, T. (2013). Approaches for inclusion of forest carbon cycle in life cycle assessment – a review. GCB Bioenergy 5, 475–486. doi: 10.1111/gcbb.12016
Hu, X., Shi, G., and Hodges, D. G. (2014). International market leakage from China’s forestry policies. Forests 2014, 2613–2625. doi: 10.3390/f5112613
Hurmekoski, E., Jonsson, R., Korhonen, J., Jänis, J., Mäkinen, M., Leskinen, P., et al. (2018). Diversification of the forest industries: role of new wood-based products. Can. J. For. Res. 48, 1417–1432. doi: 10.1139/cjfr-2018-0116
IPCC (2007). Climate Change 2007: The Physical Science Basis. Contribution of Working Group I to the Fourth Assessment Report of the Intergovernmental Panel on Climate Change, eds S. Solomon et al. (Cambridge: Cambridge University Press), 996.
IPCC (2013). “Climate Change 2013: the physical science basis,” in Contribution of Working Group I to the Fifth Assessment Report of the Intergovernmental Panel on Climate Change, eds F. T. Stocker et al. (Cambridge: Cambridge University Press), 1535.
IPCC (2018). Global Warming of 1.5°C. An IPCC Special Report on the Impacts of Global Warming of 1.5°C Above Pre-Industrial Levels and Related Global Greenhouse Gas Emission Pathways, in the Context of Strengthening the Global Response to the Threat of Climate Change, Sustainable Development, and Efforts to Eradicate Poverty, eds V. Masson-Delmotte, P. Zhai, H. O. Pörtner, D. Roberts, J. Skea, P. R. Shukla, et al. (Geneva: IPCC).
IPCC (2019). Climate Change and Land: An IPCC Special Report on Climate Change, Desertification, Land Degradation, Sustainable Land Management, Food Security, and Greenhouse Gas Fluxes in Terrestrial Ecosystems, eds P. R. Shukla, J. Skea, E. Calvo Buendia, V. Masson-Delmotte, H.-O. Pörtner, D. C. Roberts, et al. (Geneva: IPCC).
Kallio, M. A. I., Solberg, B., Käär, L., and Päivinen, R. (2018). Economic impacts of setting reference levels for the forest carbon sinks in the EU on the European forest sector. For. Policy Econ. 92, 193–201. doi: 10.1016/j.forpol.2018.04.010
Kalliokoski, T., Heinonen, T., Holder, J., Lehtonen, A., Mäkelä, A., Minunno, F., et al. (2019). Skenaarioanalyysi metsien Kehitystä Kuvaavien Mallien Ennusteiden Yhtäläisyyksistä ja Eroista. Finnish Climate Panel Report 2/2019, 88. (Suomen ilmastopaneeli). Available online at: https://www.ilmastopaneeli.fi/wp-content/uploads/2019/02/Ilmastopaneeli_mets%C3%A4mallit_raportti_180219.pdf (accessed February 15, 2020).
Kalliokoski, T., Mäkinen, H., Linkosalo, T., and Mäkelä, A. (2017). Evaluation of stand level hybrid PipeQual-model with permanent sample plot data of Norway spruce. Can. J. Forest Res. 47, 234–245. doi: 10.1139/cjfr-2016-0236
Karjalainen, T., Kellomäki, S., and Pussinen, A. (1994). Role of wood-based products in absorbing atmospheric carbon. Silva Fenn. 28, 67–80.
Kerminen, V.-M., Paramonov, M., Anttila, T., Riipinen, I., Fountoukis, C., Korhonen, H., et al. (2012). Cloud condensation nuclei production associated with atmospheric nucleation: a synthesis based on existing literature and new results. Atmos. Chem. Phys. 12, 12037–12059. doi: 10.5194/acp-12-12037-2012
Korhonen, H., Lehtinen, K. E. J., and Kulmala, M. (2004). Multicomponent aerosol dynamics model UHMA: model development and validation. Atmos. Chem. Phys. 4, 757–771. doi: 10.5194/acp-4-757-2004
Korhonen, K. T., Ihalainen, A., Ahola, A., Heikkinen, J., Henttonen, H. M., Hotanen, J., et al. (2017). Suomen metsät 2009–2013 ja Niiden Kehitys 1921–2013. Available online at: http://urn.fi/URN:ISBN:978-952-326-467-0 (accessed June 20, 2019).
Kulmala, M., Nieminen, T., Nikandrova, A., Lehtipalo, K., Manninen, H. E., Kajos, M. K., et al. (2014). CO2-induced terrestrial climate feedback mechanism: from carbon sink to aerosol source and back. BER 19, 122–122.
Kurtén, T., Kulmala, M., Dal Maso, M., Suni, T., Reissell, A., Vehkamäki, H., et al. (2003). Estimation of different forest-related contributions to the radiative balance using observations in southern Finland. Boreal Environ. Res. 8, 275–286.
Kuusinen, N., Tomppo, E., and Berninger, F. (2013). Linear unmixing of MODIS albedo composites to infer subpixel land cover type albedos. Int. J. Appl. Earth Obs. Geoinf. 23, 324–333. doi: 10.1016/j.jag.2012.10.005
Kuusinen, N., Tomppo, E., Shuai, Y., and Berninger, F. (2014). Effects of forest age on albedo in boreal forests estimated from MODIS and Landsat albedo retrievals. Remote Sens. Environ. 145, 145–153. doi: 10.1016/j.rse.2014.02.005
Lehtonen, A., Mäkipää, R., Heikkinen, J., Sievänen, R., and Liski, J. (2004). Biomass expansion factors (BEFs) for Scots pine, Norway spruce and birch according to stand age for boreal forests. For. Ecol. Manage. 188, 211–224. doi: 10.1016/j.foreco.2003.07.008
Lemprière, T. C., Kurz, W. A., Hogg, E. H., Schmoll, C., Rampley, G. J., Yemshanov, D., et al. (2013). Canadian boreal forests and climate change mitigation. Environ. Rev. 21, 293–321.
Lihavainen, H., Kerminen, V.-M., Tunved, P., Aaltonen, V., Arola, A., Hatakka, J., et al. (2009). Observational signature of the direct radiative effect by natural boreal forest aerosols and its relation to the corresponding first indirect effect. J. Geophys. Res. 114:D20206.
Liski, J., Palosuo, T., Peltoniemi, M., and Sievänen, R. (2005). Carbon and decomposition model Yasso for forest soils. Ecol. Model. 189, 168–182. doi: 10.1016/j.ecolmodel.2005.03.005
Luke statistics database (2019). Luke Statistics Database. http://statdb.luke.fi/PXWeb/pxweb/fi/LUKE/LUKE__04%20Metsa__06%20Metsavarat/?rxid=dc711a9e-de6d-454b-82c2-74ff79a3a5e0
Luyssaert, S., Marie, G., Valade, A., Chen, Y.-Y., Djomo, S. N., Ryder, J., et al. (2018). Trade-offs in using European forests to meet climate objectives. Nature 562, 259–262. doi: 10.1038/s41586-018-0577-1
Mäkelä, A. (2002). Derivation of stem taper from the pipe theory in a carbon balance framework. Tree Physiol. 22, 891–905. doi: 10.1093/treephys/22.13.891
Mäkinen, A., Kangas, A. S., and Kalliovirta, J. (2008). Comparison of treewise and standwise forest simulators by means of quantile regression. For. Ecol. Manage. 255, 2709–2717. doi: 10.1016/j.foreco.2008.01.048
Matthies, B. D., Kalliokoski, T., Eyvindson, K., Honkela, N., Hukkinen, J. I., Kuusinen, N. J., et al. (2016). Nudging service providers and assessing service trade-offs to reduce social inefficiencies of payments for ecosystem services. Environ. Sci. Policy 55, 228–237. doi: 10.1016/j.envsci.2015.10.009
Matthies, B. D., and Valsta, L. T. (2016). Optimal forest species mixture with carbon storage and albedo effect for climate change mitigation. Ecol. Econ. 123, 95–105. doi: 10.1016/j.ecolecon.2016.01.004
Minkkinen, K., Byrne, K. A., and Trettin, C. (2008). “Climate impacts of peatland forestry,” in Peatlands and Climate Change, ed. M. Strack (Jyväskylä: International Peat Society), 98–122.
Mitchell, S. R., Harmon, M. E., and O’Connell, K. E. B. (2012). Carbon debt and carbon sequestration parity in forest bioenergy production. GCB Bioenergy 4, 818–827. doi: 10.1111/j.1757-1707.2012.01173.x
Mogensen, D., Gierens, R., Crowley, J. N., Kerone, P., Smolander, S., Sogachev, A., et al. (2015). Simulations of atmospheric OH, O3 and NO3 reactivities within and above the boreal forest. Atmos. Chem. Phys. 15, 3909–3932. doi: 10.5194/acp-15-3909-2015
Nabuurs, G. J., Delacote, P., Ellison, D., Hanewinkel, M., Hetemäki, L., Lindner, M., et al. (2017). By 2050 the mitigation effects of EU forests could nearly double through climate smart forestry. Forests 8, 1–14. doi: 10.3390/f8120484
National Inventory Report (NIR) (2019). Greenhouse Gas Emissions in Finland 1990–2018. Available online at: https://www.stat.fi/static/media/uploads/tup/khkinv/fi_nir_un_2018_2020_04_09.pdf
Natural Resources Finland (2019). Metsäsektorin Suhdannekatsaus 2019-2020. Available online at: www.luke.fi/wp-content/uploads/2019/10/Metsasektorin-suhdannenakymat-ja-Suomen-asema-globaalissa-ymparistossa.pdf (accessed December 5, 2019).
Naudts, K., Chen, Y., McGrath, M. J., Ryder, J., Valade, A., Otto, J., et al. (2016). Europe’s forest management did not mitigate climate warming. Science 351, 597–600. doi: 10.1126/science.aad7270
Nepal, P., Ince, P. J., Skog, K. E., and Chang, S. J. (2013). Projected US timber and primary forest product market impacts of climate change mitigation through timber set-asides. Can. J. For. Res. 43, 245–255. doi: 10.1139/cjfr-2012-0331
Nieminen, T., Kerminen, V.-M., Petäjä, T., Aalto, P. P., Arshinov, M., Asmi, E., et al. (2018). Global analysis of continental boundary layer new particle formation based on long-term measurements. Atmos. Chem. Phys. 18, 14737–14756.
Nikinmaa, E., Kalliokoski, T., Minkkinen, K., Bäck, J., Boy, M., Gao, Y., et al. (2017). Accounting for multiple forcing factors and product substitution enforces the cooling effect of boreal forests. Biogeosci. Discuss. 1–28.
O’Halloran, T. L., Law, B. E., Goulden, M. L., Wang, Z., Barr, J. G., Schaaf, C., et al. (2012). Radiative forcing of natural forest disturbances. Glob. Change Biol. 18, 555–565. doi: 10.1111/j.1365-2486.2011.02577.x
Ojanen, P., Lehtonen, A., Heikkinen, J., Penttilä, T., and Minkkinen, K. (2014). Soil CO2 balance and its uncertainty in forestry-drained peatlands in Finland. For. Ecol. Manage. 325, 60–73. doi: 10.1016/j.foreco.2014.03.049
Ojanen, P., and Minkkinen, K. (2019). The dependence of net soil CO2 emissions on water table depth in boreal peatlands drained for forestry. Mires Peat 24, 1–8. doi: 10.19189/MaP.2019.OMB.StA.1751
Paasonen, P., Asmi, A., Petäjä, T., Kajos, M. K., Äijälä, M., Junninen, H., et al. (2013). Warming-induced increase in aerosol number concentration likely to moderate climate change. Nat. Geosci. 6, 438–442. doi: 10.1038/ngeo1800
Paasonen, P., Peltola, M., Kontkanen, J., Junninen, H., Kerminen, V.-M., and Kulmala, M. (2018). Comprehensive analysis of particle growth rates from nucleation mode to cloud condensation nuclei in boreal forest. Atmos. Chem. Phys. 18, 12085–12103. doi: 10.5194/acp-18-12085-2018
Pihlainen, S., Tahvonen, O., and Niinimäki, S. (2014). The economics of timber and bioenergy production and carbon storage in Scots pine stands. Can. J. For. Res. 44, 1091–1102. doi: 10.1139/cjfr-2013-0475
Pingoud, K., Ekholm, T., and Savolainen, I. (2012). Global warming potential factors and warming payback time as climate indicators of forest biomass use. Mitig. Adapt. Glob. Change 17, 369–386. doi: 10.1007/s11027-011-9331-9
Pingoud, K., Ekholm, T., Sievänen, T., Huuskonen, S., and Hynynen, J. (2018). Trade-offs between forest carbon stocks and harvests in a steady state - A multi-criteria analysis. J. Environ. Manage. 210, 96–103. doi: 10.1016/j.jenvman.2017.12.076
Pingoud, K., Pohjola, J., and Valsta, L. (2010). Assessing the integrated climatic impacts of forestry and wood products. Silva Fenn. 44, 155–175.
Popkin, G. (2019). How much can forests fight climate change? Nature 565, 280–282. doi: 10.1038/d41586-019-00122-z
Roe, S., Streck, C., Obersteiner, M., Frank, S., Griscom, B., Drouet, L., et al. (2019). Contribution of the land sector to a 1.5 °C world. Nat. Clim. Change 9, 817–828.
Roeckner, E., Bäuml, G., Bonaventura, L., Brokopf, R., Esch, M., Giorgetta, M., et al. (2003). The Atmospheric General Circulation Model ECHAM5, part 1 Model Description. Hamburg: Max-Planck-Institut für Meteorologie.
Roldin, P., Ehn, M., Kurtén, T., Olenius, T., Rissanen, M. P., Sarnela, N., et al. (2019). The role of highly oxygenated organic molecules in the Boreal aerosol-cloud-climate system. Nat. Commun. 10:4370.
Salminen, H., Lehtonen, M., and Hynynen, J. (2005). Reusing legacy FORTRAN in the MOTTI growth and yield simulator. Comput. Electron. Agric. 49, 103–113. doi: 10.1016/j.compag.2005.02.005
Sathre, R., and O’Connor, J. (2010). Meta-analysis of greenhouse gas displacement factors of wood product substitution. Environ. Sci. Policy 13, 104–114. doi: 10.1016/j.envsci.2009.12.005
Schaaf, C. B., Gao, F., Strahler, A. H., Lucht, W., Li, X., Tsang, T., et al. (2002). First operational BRDF, albedo nadir reflectance products from MODIS. Remote Sens. Environ. 83, 135–148. doi: 10.1016/s0034-4257(02)00091-3
Schulze, E.-D., Körner, C., Law, B. E., Haberl, H., and Luyssaert, S. (2012). Large-scale bioenergy from additional harvest of forest biomass is neither sustainable nor greenhouse gas neutral. GCB Bioenergy 4, 611–616. doi: 10.1111/j.1757-1707.2012.01169.x
Seidl, R., Thom, D., Kautz, M., Martin-Benito, D., Peltoniemi, M., Vacchiano, G., et al. (2017). Forest disturbances under climate change. Nat. Clim. Change 7, 395–402. doi: 10.1038/nclimate3303
Seppälä, J., Heinonen, T., Pukkala, T., Kilpeläinen, A., Mattila, T., Myllyviita, T., et al. (2019). Effect of increased wood harvesting and utilization on required greenhouse gas displacement factors of wood-based products and fuels. J. Environ. Manage. 247, 580–587. doi: 10.1016/j.jenvman.2019.06.031
Smeets, E., Tabeau, A., Berkum, S., Moorad, J., van Meijl, H., Woltje, G., et al. (2014). The impact of the rebound effect of the use of first generation biofuels in the EU on greenhouse gas emissions: a critical review. Renew. Sustain. Energy Rev. 38, 393–403. doi: 10.1016/j.rser.2014.05.035
Smith, P., Davis, S. J., Creutzig, F., Fuss, S., Minx, J., Gabrielle, B., et al. (2016). Biophysical and economic limits to negative CO2 emissions. Nat. Clim. Chang. 6, 42–50.
Smolander, S., He, Q., Mogensen, D., Zhou, L., Bäck, J., Ruuskanen, T., et al. (2014). Comparing three vegetation monoterpene emission models to measured gas concentrations with a model of meteorology, air chemistry and chemical transport. Biogeosciences 11, 5425–5443. doi: 10.5194/bg-11-5425-2014
Sogachev, A., Kelly, M., and Leclerc, M. (2012). Consistent two-equation closure modelling for atmospheric research: buoyancy and vegetation implementations. Bound. Layer Meteor. 145, 307–327. doi: 10.1007/s10546-012-9726-5
Sogachev, A., Menzhulin, G., Heimann, M., and Lloyd, J. (2002). A simple three dimensional canopy - planetary boundary layer simulation model for scalar concentrations and fluxes. Tellus B 54, 784–819. doi: 10.1034/j.1600-0889.2002.201353.x
Sogachev, A., and Panferov, O. (2006). Modification of two-equation models to account for plant drag. Bound. Layer Meteorol. 121, 229–266. doi: 10.1007/s10546-006-9073-5
Soimakallio, S., Saikku, L., Valsta, L., and Pingoud, K. (2016). Climate Change Mitigation Challenge for Wood Utilization – The Case of Finland. Environ. Sci. Technol 50, 5127–5134. doi: 10.1021/acs.est.6b00122
Sommerhuber, P. F., Welling, J., and Krause, A. (2015). Substitution potentials of recycled HDPE and wood particles from post-consumer packaging waste in Wood–Plastic Composites. Waste Manage. 46, 76–85. doi: 10.1016/j.wasman.2015.09.011
Spracklen, D. V., Bonn, B., and Carslaw, K. S. (2008). Boreal forests, aerosols and the impacts on clouds and climate. Philos. Trans. R. Soc. A 366, 4613–4626.
Ter-Mikaelian, M., Colombo, S. J., and Chen, J. (2015). The Burning Question: Does Forest Bioenergy Reduce Carbon Emissions? A Review of Common Misconceptions about Forest Carbon Accounting. J. For. 113, 57–68. doi: 10.5849/jof.14-016
The National Forest Strategy 2025 (2019). Ministry of Agriculture and Forestry, Helsinki 2019. Available online at: http://julkaisut.valtioneuvosto.fi/bitstream/handle/10024/161386/MMM_7_2019_Mets%c3%a4strategia.pdf?sequence=4&isAllowed=y (accessed December 15, 2019).
Tomppo, E., Olsson, H., Ståhl, G., Nilsson, M., Hagner, O., and Katila, M. (2008). Combining national forest inventory field plots and remote sensing data for forest databases. Remote Sens. Environ. 112, 1982–1999. doi: 10.1016/j.rse.2007.03.032
Tunved, P., Hansson, H.-C., Kerminen, V.-M., Ström, J., Dal Maso, M., Lihavainen, H., et al. (2006). High natural aerosol loading over boreal forests. Science 312, 261–263. doi: 10.1126/science.1123052
Tuomi, M., Laiho, R., Repo, A., and Liski, J. (2011). Wood decomposition model for boreal forests. Ecol. Mod. 222, 709–718. doi: 10.1016/j.ecolmodel.2010.10.025
Twomey, S. (1977). The influence of pollution on the shortwave albedo of clouds. J. Atmos. Sci 34, 1149–1152. doi: 10.1175/1520-0469(1977)034<1149:tiopot>2.0.co;2
UNECE (2019). Forest Products Annual Market review 2018-2019. Available online at: http://www.unece.org/forests/areas-of-work/forestsfpm/outputs/forestsfpamr/forestsfpamr2019.html
Unger, N. (2014). Human land-use-driven reduction of forest volatiles cools global climate. Nat. Clim. Change 4, 907–910. doi: 10.1038/nclimate2347
Unger, N., Yue, X., and Harper, K. L. (2017). Aerosol climate change effects on land ecosystem services. Faraday Discuss. 200, 121–142. doi: 10.1039/c7fd00033b
Uppala, S. M., KÅllberg, P. W., Simmons, A. J., Andrae, U., Da Costa Bechtold, V., Fiorino, M., et al. (2005). The ERA-40 re-analysis. Q. J. R. Meteorol. Soc. 131, 2961–3012.
Wang, L., Cole, J. N., Bartlett, P., Verseghy, D., Derksen, C., Brown, R., et al. (2016). Investigating the spread in surface albedo for snow-covered forests in CMIP5 models. J. Geophys. Res. Atmos. 121, 1104–1119. doi: 10.1002/2015jd023824
Xausa, F., Paasonen, P., Makkonen, R., Arshinov, M., Ding, A., Denier Van Der Gon, H., et al. (2018). Advancing global aerosol simulations with size-segregated anthropogenic particle number emissions. Atmos. Chem. Phys. 18, 10039–10054. doi: 10.5194/acp-18-10039-2018
Yli-Pirilä, P., Copolovici, L., Kännaste, A., Noe, S., Blande, J. D., Mikkonen, S., et al. (2016). Herbivory by an outbreaking moth increases emissions of biogenic volatiles and leads to enhanced secondary organic aerosol formation capacity. Environ. Sci. Technol. 50, 11501–11510. doi: 10.1021/acs.est.6b02800
Zhou, L., Nieminen, T., Mogensen, D., Smolander, S., Rusanen, A., Kulmala, M., et al. (2014). SOSAA - a new model to simulate the concentrations of organic vapours, sulphuric acid and aerosols inside the ABL - Part 2: aerosol dynamics and one case study at a boreal forest site. Boreal Environ. Res. 19, 237–256.
Keywords: forest carbon sink, biogenic volatile organic compounds, substitution of fossil fuels, non-carbon effects, biophysical effects
Citation: Kalliokoski T, Bäck J, Boy M, Kulmala M, Kuusinen N, Mäkelä A, Minkkinen K, Minunno F, Paasonen P, Peltoniemi M, Taipale D, Valsta L, Vanhatalo A, Zhou L, Zhou P and Berninger F (2020) Mitigation Impact of Different Harvest Scenarios of Finnish Forests That Account for Albedo, Aerosols, and Trade-Offs of Carbon Sequestration and Avoided Emissions. Front. For. Glob. Change 3:562044. doi: 10.3389/ffgc.2020.562044
Received: 14 May 2020; Accepted: 07 September 2020;
Published: 06 October 2020.
Edited by:
Jose D. Fuentes, Pennsylvania State University (PSU), United StatesReviewed by:
Werner Alexander Kurz, Natural Resources Canada, CanadaCopyright © 2020 Kalliokoski, Bäck, Boy, Kulmala, Kuusinen, Mäkelä, Minkkinen, Minunno, Paasonen, Peltoniemi, Taipale, Valsta, Vanhatalo, Zhou, Zhou and Berninger. This is an open-access article distributed under the terms of the Creative Commons Attribution License (CC BY). The use, distribution or reproduction in other forums is permitted, provided the original author(s) and the copyright owner(s) are credited and that the original publication in this journal is cited, in accordance with accepted academic practice. No use, distribution or reproduction is permitted which does not comply with these terms.
*Correspondence: Tuomo Kalliokoski, dHVvbW8ua2FsbGlva29za2lAaGVsc2lua2kuZmk=
Disclaimer: All claims expressed in this article are solely those of the authors and do not necessarily represent those of their affiliated organizations, or those of the publisher, the editors and the reviewers. Any product that may be evaluated in this article or claim that may be made by its manufacturer is not guaranteed or endorsed by the publisher.
Research integrity at Frontiers
Learn more about the work of our research integrity team to safeguard the quality of each article we publish.