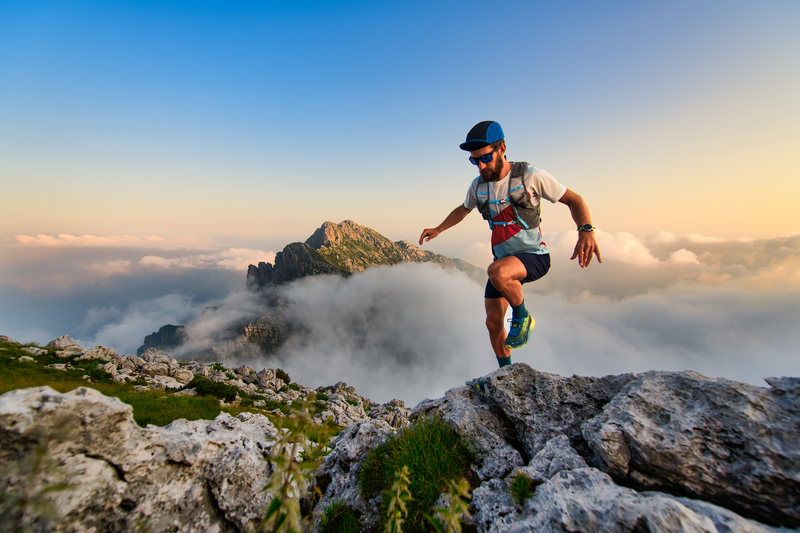
94% of researchers rate our articles as excellent or good
Learn more about the work of our research integrity team to safeguard the quality of each article we publish.
Find out more
ORIGINAL RESEARCH article
Front. For. Glob. Change , 24 July 2020
Sec. Tropical Forests
Volume 3 - 2020 | https://doi.org/10.3389/ffgc.2020.00080
This article is part of the Research Topic Tropical Montane Forests in a Changing Environment View all 10 articles
Predation plays a critical role in animal and plant survivorship, and can be highly sensitive to habitat loss and disturbance. Tropical montane forests in Southeast Asia are being modified rapidly by land-use change, and the consequences of this on predation likelihood are poorly understood. In Peninsular Malaysia, we conducted predation experiments at eight tropical montane forest sites along a disturbance gradient. We investigated whether (1) predation pressure in primary forests differs between different mountains; (2) predation probability is linked to habitat degradation; and (3) vegetation variables explain predation occurrence. At each forest site, we placed artificial nests with real and model quail eggs, dishes with real and artificial seeds of the cempedak (Artocarpus champeden), models resembling four-lined tree frogs (Polypedetes leucomystax) and models of the late instar caterpillar of the common Mormon (Papilio polytes) at points 100 m apart for three nights. Using Bayesian binomial simulations, we showed that predation likelihood in primary forests from different mountains can vary (e.g., probability of the difference in predation rate of artificial caterpillars between two primary forests was estimated at 82–100%). We also found that higher predation was not linked to habitat degradation for all artificial prey and seeds (e.g., comparing forests of varying degrees of disturbance from the same mountain, the probability that predation of an artificial caterpillar is lower at the primary forest was estimated at 2–20% only). Model selection and hierarchical partitioning showed that vegetation variables can explain predation occurrence, suggesting microhabitat characteristics may be influential. Conducting predation experiments by using artificial prey and seeds is useful for comparing predation likelihood at different sites, making ecological comparisons, and for informing conservation decisions. This novel approach of using multiple prey items also showed that predation for each can vary and thus caution against deploying a single prey type to draw broad inferences of predation in degraded systems.
Predation is a highly influential ecological process (Sih et al., 1985; Schmitz et al., 2010), affecting animal reproductive strategies (Martin, 1995; Fontaine and Martin, 2006), plant distribution (Bazzaz and Pickett, 1980; Chapman et al., 2016), vegetation recovery and forest succession (Doust, 2011). Predators and their associated ecosystem functions can be highly sensitive to habitat degradation due to anthropogenic pressures (Dobson et al., 2006; Schmitz et al., 2010). Generally, disturbed or degraded forests experience a higher incidence of avian nest predation than their pristine counterparts (Stephens et al., 2003; Vetter et al., 2013); caterpillar predation has been shown to be higher in forest fragments compared to continuous forest, although season and prey density have a great deal of influence (Posa et al., 2007; Ruiz-Guerra et al., 2012; Tvardikova and Novotny, 2012); and seed predation may be higher in forest fragments (Guariguata et al., 2002).
“High order effects” may be responsible for the higher predation in disturbed forests. For example, the absence of mega-predators may increase the populations of some medium- to small-size macropredators, which in turn could be detrimental to their prey species (Turner and Corlett, 1996). Another mechanism that increases predation in degraded forests are “negative edge effects” whereby the altered internal microclimate of forest near the edge may increase the density of disturbance-associated opportunistic predators (Laurance, 1991; Turner and Corlett, 1996).
However, the relationship between predation and habitat disturbance in tropical montane forests remains unclear. For example, studies of the edge effect on avian nest predation in montane forests have equivocal findings: Carlson and Hartman (2001) found no edge effect on nest predation rate in an Eastern Arc montane forest in Tanzania and Spanhove et al. (2014) reported from a montane forest in Kenya that nest predation increased from the edge toward the forest interior. Conversely, Sedláček et al. (2014) provided evidence for an edge effect on nest predation (i.e., higher predation) in a montane forest in the Bamenda-Bano Highlands, Cameroon.
Disturbance is a major driver of plant species diversity in both ecological and evolutionary time. However, little is understood about how this affects the incidence of seed predation in tropical montane forests (Chapman et al., 2016). Chinchilla (2009) reported that seed predation of four native tree species was not different between a primary tropical montane forest and its fragments in Mexico, and Myster (2015) found that seed predation in an undisturbed primary Ecuadorian forest was higher than a nearby secondary forest recovered from agriculture.
As hotspots of endemism and sources of major river systems, Southeast Asia's tropical montane forests have high conservation value (Peh et al., 2011). Despite this biological and geological importance, montane forests in Southeast Asia are threatened by increasing encroachment from agriculture, commercial forestry, and infrastructure development (Peh et al., 2011). Some case studies imply that degradation of these forests can have detrimental effects on predator-prey relationships (Goldsmith et al., 2007) and reduce population viability of range-restricted species (Sedláček et al., 2014). Therefore, investigating this critical ecological process in both pristine and disturbed forests in tropical mountains is key to our understanding of the current and future state of these habitats (Soh et al., 2019).
In this study, we carried out predation experiments in tropical montane forests in Peninsular Malaysia at a landscape scale and across a suite of sites of varying disturbance. We used four different prey items—artificial nests, artificial seeds, caterpillar models and frog models—along a disturbance gradient, from pristine forests to tea plantations. Based on this novel approach (i.e., using multiple prey types), we assessed whether predation probability in a given montane habitat type (e.g., pristine forest or rural area) differs between mountain ranges. We hypothesized that predation probability between mountain ranges may differ even within the same habitat type as predator distribution can be heterogenous (see Emmering and Schmidt, 2011). We also investigated whether predation probability consistently varies in different habitat types, and hypothesized that more disturbed land uses face greater predation. Lastly, we investigated whether predation can be explained by vegetation structure.
We surveyed a total of eight study sites across three locations (Cameron Highlands, Lojing Highlands, and Fraser's Hill) along the Titiwangsa mountain range in Peninsular Malaysia to represent a range of tropical montane habitats with varying degrees of human disturbance (Figure 1). These included primary forests (Pine, hereafter “Fraser primary”; Brinchang, “Cameron primary”; Yong Belar, “Lojing primary”), a primary forest edge (Bishop, “Fraser edge”), secondary forests (Forestry, “Cameron secondary”), a tea plantation (“Cameron tea”) and rural areas in Fraser's Hill (“Fraser rural”) and Cameron Highlands (“Cameron rural”). A summary of the habitat types, location, and background information of the eight sites is given in Table 1.
Figure 1. Satellite map of study sites of varying habitat disturbance levels within Cameron and Lojing Highlands (top panel) and Fraser's Hill (bottom right panel), Peninsular Malaysia. For site abbreviations and detailed descriptions, refer to Table 1.
Table 1. Summary of information on study sites for predation experiments from July to August 2016 including the names of the mountain ranges and sites and abbreviation used in the text; description of the habitat type; the geographical coordinates of the site, the median canopy cover of the sites; and the dates of the predation experiments.
We carried out the predation experiments from 30 July to 22 August 2016 (Table 1). Models were made using Sculpey III non-toxic modeling clay and painted with non-toxic paint to mimic real quail eggs, four-lined tree frogs (Polypedetes leucomystax), late instar caterpillars of the common Mormon (Papilio polytes) and cempedak (Artocarpus champeden) seeds. We used quail eggs because of their small size, which provided a close representation of shrub-nesting babbler species (see Sodhi et al., 2003). The chosen tree frog, butterfly, and plant species for our experiments were appropriate as they are commonly found in the montane forests of Peninsular Malaysia.
We set up 16 experimental stations along on a transect within each site 100 m apart. Each transect started randomly on an existing trail, at least 150 m from the edge of the forest. Each experimental station was a circular plot of 5 m radius off the trail, where we randomly installed our artificial prey items at least 2 m apart from each other (Supplementary Figure 1). For the egg experiments, we placed one real egg and one artificial egg in an artificial nest secured at a height of 1 m onto a shrub or tree sapling, as per Sodhi et al. (2003). We placed one real seed and one artificial seed on a petri dish for seed experiments. Petri dishes were placed directly on the forest floor near the buttress of trees to facilitate relocation (Sodhi et al., 2003).
For the caterpillar model experiments, we attached one artificial caterpillar onto a wooden stick, ~2 cm from the end and attached it at the height of 1 m using thin wire to a branch, as per Posa et al. (2007). Lastly, we placed one artificial frog on a cardboard square (8 cm2) for the frog model experiments, which were placed directly onto the forest floor. Following Sodhi et al. (2003), we adjusted the vegetation so that the percentage of cover by the vegetation directly above all prey items was about 50% when viewed at a height of 1.70 m.
We checked each experimental station after 3 days to determine any predation events. Nests were considered preyed upon if an egg was missing, broken, pecked, cracked or smashed, or if bite-marks were found on the artificial egg. Seeds were considered preyed upon if a seed was missing, or bite-marks were found on either real or artificial seed. Similarly, we considered predation to have occurred if a caterpillar or frog model was missing, or bite-marks were found. We examined the bite-marks imprinted on the artificial prey items or real seed to determine the potential predators (Sodhi et al., 2003; Posa et al., 2007). Predation by small mammals was determined by the presence of incisor marks (≤2 mm wide; i.e., evidence of gnawing); birds were identified by peck marks, long thin streaks or pinches; arthropods by small scratches, boreholes or bites; and reptiles by small, equally spaced teeth marks (Sodhi et al., 2003; Posa et al., 2007). Any prey item that could not be located or that was suspected to have been tampered with by human passers-by was omitted from analysis.
To determine if vegetation structure affects predation probability, we measured eight vegetation structure variables within each of the 16 experimental stations (i.e., circular plot of 5 m radius, each 100 m apart). These parameters included (1) aboveground biomass per ha; (2) canopy cover; (3) number of dead trees; (4) number of saplings; (5) ground cover; (6) leaf litter depth; (7) shrub cover; and (8) understorey volume.
To estimate the aboveground biomass, we measured the diameter at breast height (DBH) of all trees with DBH ≥ 2 cm within the experimental station; and used the allometric equation for tropical montane trees from Edwards and Grubb (1977). We used a densiometer to measure the canopy cover by averaging four readings from four cardinal directions. We counted the number of dead trees, and saplings (trees with DBH < 2 cm) within the experimental stations. In four cardinal directions 5 m from the center of each experimental station, we measured the average leaf litter depth using a ruler, and the average percentage of shrub cover (i.e., vegetation under 2 m in height) by visual estimation from two independent observers. At these four locations within each experimental station, we took photographs of the ground and overlayed these photographs with a grid of 48 squares. The number of squares with vegetation were tallied for each photograph and then used to obtain the average percentage of ground cover. Last, we also estimated understorey vegetation volume by erecting a 6-m telescopic pole marked at every 20 cm interval and counting the number of intervals the vegetation contacted the pole at four random positions (Soh et al., 2006).
Our study design, admittedly, could yield some potential biases or erroneous estimates of natural predation. First, our experiments did not consider specific prey-predator interactions. The traits of prey can affect the behavior of predators and predation occurrence (Sih et al., 2010; Sih, 2013). For example, seeds with caloric content are more frequently hoarded by rodents and thus less preyed upon, forming a mutualistic relationship with rodents (Wang et al., 2014). Second, like other studies with artificial predation, our results might not reflect directly natural predation, but instead, a predation risk. This is due to several limitations of using artificial prey and seeds: (1) both artificial and quail eggs lacked thermal cues, that attract reptilian predators (Thompson and Burhans, 2004), and parental activity, which can increase predation likelihood (Martin et al., 2000; Robinson et al., 2005); (2) the cardboard on which the frog models were placed may influence predator's recognition; (3) caterpillar and frog models lacked locomotion—an important signifying cue for many predators (Paluh et al., 2014); and (4) our use of relatively large cempedak seeds might not be representative of other tree species, as large seeds may have a lower predation likelihood due to low densities of large seed dispersers (Doust, 2011). Also, seeds partially damaged by predators may not affect viability as they may still survive and germinate (see Chapman et al., 2016). Nevertheless, since all experimental stations in this comparative study used the same artificial prey and seeds, the effect of the absence of natural markers was, therefore, controlled across all sites.
All statistical analyses were performed using R 3.2.2 (R Core Team, 2017). Chi-squared tests were used to determine if predation on each prey item category (e.g., seeds) was associated with a particular taxonomic group (e.g., mammals). To determine if the probability of predation differed between sites, we conducted Bayesian analysis using the “Bbinom” (Bayesian binomial simulation) function from the “wiqid” package (Meredith, 2017) to compare two sets of binomial data (presence and absence of predation). Admittedly, our sample size for each study site was relatively small (n = 16 for each prey type). Nevertheless, a Bayesian approach is suitable for analyzing small data sets for comparative studies (Hox et al., 2012). This approach simulates a sample (50,000 Markov Chain Monte Carlo [MCMC] simulations) from the posterior for a binomial likelihood (i.e., probability of predation of one site being lower than the other) with an informative prior. However, we used a uniform prior in our analyses instead, as we did not have any prior information about the predation probability in a Malaysian montane forest (see Tuyl et al., 2009). For each prey item, we compared binomial likelihood of predation between (1) different localities of pristine forest along the same mountain range; (2) different localities of rural area along the same mountain range; and (3) habitats of varying degree of anthropogenic disturbance within the same mountain.
We performed non-metric multidimensional scaling (NMDS) for each prey item to determine the relationship between predation events and vegetation structure. All variables were transformed to achieve normality if necessary, using Shapiro-Wilk tests of normality. Variables expressed in terms of percentage (i.e., canopy cover, shrub cover, and ground cover) were arcsine square-root transformed. Leaf litter depth and the number of trees with DBH < 2 cm strongly correlated with canopy cover (rs > 0.7) (Supplementary Table 1); these variables were therefore excluded from the analysis. NMDS was performed using the “metaNMDS” function from the “vegan” package (Oksanen et al., 2017).
We applied binary logistic regression and used MuMIn package (Barton, 2016) to run model selection based on Akaike Information Criterion (AIC; Akaike, 1973), comparing models for all possible parameter subsets in terms of parsimony and prediction. Due to the small sample size (number of samples/number of parameters < 40), we used AICc. The difference in the AICc values between the best model (one with the lowest AICc value) and other models (Δi) was calculated. Models were then ranked in order of increasing Δi. Finally, we used the hier.part package (Walsh and Nally, 2013) to run hierarchical partitioning regression analysis to determine the contribution of each variable toward predation.
A total of 124 artificial nests–each containing one artificial egg and one quail egg; 120 pairs of seeds–one artificial seed and one cempedak seed; 120 caterpillar models; and 121 frog models were used in this study. We observed that 27% of artificial nests, 43% of seed stations, 26% of caterpillar models, and 15% of frog models were preyed upon (Table 2).
Table 2. Number of artificial eggs, artificial seeds, caterpillar models, and frog models (excluding those tampered by humans) used in the predation experiments, and number of artificial prey items predated by birds, mammals, reptiles, and arthropods.
Avian predation was observed at artificial nests across all sites (Figure 2A). There was a significant association of artificial nest predation with avian predators (χ2 = 9.76; P = 0.02). Seed predation was significantly associated with mammalian predators (Figure 2B) (χ2 = 14.31; P < 0.01). Caterpillar models were preyed upon by all predator types, except mammals (Figure 2C; Table 2); but their predation was significantly associated with avian predators (χ2 = 8.53; P = 0.04). Lastly, frog models were preyed upon by all predator types, except arthropods (Figure 2D; Table 2), and there was no significant association of frog model predation with a particular predator type (χ2 = 3.56; P = 0.31).
Figure 2. Proportion of each predator type for (A) artificial nests; (B) artificial seeds (C) caterpillar models; and (D) frog models. Sites are clustered together according to their respective mountain range and presented in an order of increasing canopy cover (see Table 1). For abbreviations, refer to Table 1.
Our experiments broadly distinguished four groups of predators based on the characteristics of their bite marks. As many bite marks were unidentified, we did not have an adequate sample size to determine if there was a shift in predator taxa for each prey item or seed across the disturbance gradient. This phenomenon of a predator taxa shift from invertebrate to vertebrate coincided with land use intensity in one tropical lowland forest predation experiment (Boyle, 2012). Admittedly, the method of analyzing bite marks proved to be challenging: (1) some bite marks were not easily distinguishable, for example those of reptiles and non-rodent mammals; and (2) some invertebrate predators may leave inconspicuous or even no mark on clay models (Tvardikova and Novotny, 2012).
Incorporating camera trapping into predation experiments should improve the data quality for future investigations. This approach would enable a rapid assessment of whether predation is a conservation issue, for example if non-native predators are present (Pender et al., 2013). Future work should also estimate the richness and abundance of natural potential predators in order to control for any species-area relationship, as predator abundance could be correlated with occurrence of predation.
Our results showed that predation probability differs between different primary forests. The probability of a difference in artificial nest predation rate between Lojing primary and Cameron primary being greater than zero is low, estimated at 33% only (Supplementary Table 2). However, the probability that Lojing primary had a lower artificial nest predation rate than Fraser primary was estimated at 78% (Supplementary Table 2). Lojing primary had a lower artificial seed predation rate than Fraser primary and Cameron primary, estimated at a probability of 98 and 78%, respectively (but the probability of the difference between Cameron primary and Fraser primary was low, estimated at 8% only; Supplementary Table 2). Lojing primary also had a lower artificial caterpillar predation rate than Fraser primary and Cameron primary, estimated at a probability of 82 and 100%, respectively (conversely, the probability of the difference between Cameron primary and Fraser primary was low, estimated at only 3%; Supplementary Table 2). For artificial frogs, the probability of the predation difference between Lojing primary and Cameron primary being greater than zero is low, estimated at 66% only (similarly, that between Fraser primary and Cameron primary was also low, estimated at 3%; Supplementary Table 2). However, Lojing primary had a lower artificial frog predation rate than Fraser primary, estimated at 85% probability (Supplementary Table 2).
Similarly to observations in primary forests, rural areas also had different predation probability. Between Cameron rural and Fraser rural, the probability of the difference in artificial nest predation rates being greater than zero was estimated at 0% (i.e., their predation rates were the same; Supplementary Table 3). The predation rates of the artificial seeds and caterpillar models between these sites being greater than zero was low as well, estimated at 40 and 5%, respectively (Supplementary Table 3). However, the probability of the difference for the frog models was relatively high, estimated at 70% (Supplementary Table 3).
Our predation tests using different artificial prey and seeds therefore consistently reveal that the primary forests, even along the same mountain range, could have different predation likelihood. Likewise, predation likelihood could be different between the rural areas in separate locations. These observations fit the concept of site-dependent spatial heterogeneity in predation risk (see Rodenhouse et al., 1997). Differences in the local predator abundance and dynamics are possible mechanisms driving this (Emmering and Schmidt, 2011).
However, there are also some interesting patterns: predation at Lojing primary was constantly lower than Fraser primary for nests (probability = 78%), seeds (98%), caterpillars (82%) and frogs (85%), whereas predation between Fraser primary and Cameron primary did not differ across all prey types. Overall, our results indicate that predation likelihood may be site-specific, influenced by the structure of surrounding vegetation (see Seibold et al., 2013; for further discussion see section Hypothesis 3: Vegetation Structure Explains Predation Probability).
Our artificial avian nest experiment showed that there is no association between predation probability and habitat disturbance. Across the different land uses in Fraser's Hill, Fraser primary had a lower artificial nest predation rate than Fraser edge and Fraser rural with the probability estimated at 79 and 98%, respectively (Supplementary Table 4). In Cameron Highlands, Cameron primary had a lower artificial nest predation pressure than Cameron tea (probability that the difference is greater than zero = 91%). However, the probability that the artificial nest predation in Cameron primary was lower when compared to Cameron secondary and Cameron rural was estimated at only 30% each (Supplementary Table 4). Therefore, understorey bird nests in primary forest do not consistently show a lower predation pressure compared to nests in the more disturbed habitats.
Like the artificial avian nest, the artificial seeds did also not show a correlation between predation probability and disturbance intensity. In Fraser's Hill, the probability that Fraser primary had a lower artificial seed predation rate than Fraser edge and Fraser rural was low, estimated at 68 and 1%, respectively (Supplementary Table 5). Similarly, we estimated the probability that Cameron primary had a lower predation pressure than Cameron secondary, Cameron rural and Cameron tea at only 15, 10, and 5%, respectively (Supplementary Table 5). Hence, the seeds in primary forests may not have a lower predation pressure compared to seeds in more disturbed habitats.
Results of artificial avian nest and seeds experiments were also observed in the caterpillar models. In Cameron Highlands, the caterpillar models had a lower predation pressure in the Cameron primary compared to the Cameron secondary (probability that the difference being greater than zero = 98%), Cameron rural (probability = 97%) and Cameron tea (89%) (Supplementary Table 6). However, there is no clear evidence that the predation of caterpillar models in Fraser primary was lower than Fraser edge (probability that the difference being greater than zero = 29%) and Fraser rural (probability = 40%) (Supplementary Table 6). Hence, it remains equivocal that the caterpillars may have a lower predation pressure in primary forest than more disturbed habitats.
In agreement with other prey types, the predation probability of frog models was also not associated with habitat disturbance. In Cameron Highlands, there is no clear evidence that the frog models in primary forest had a lower predation pressure than those in secondary forest (probability that the difference being greater than zero = 2%), rural (probability = 20%) and tea (probability = 2%) (Supplementary Table 7). Likewise, in Fraser's Hill the predation of frog models in primary forest was not lower than in forest edge (probability of the difference being greater than zero = 30%) and Fraser rural (probability = 3%) (Supplementary Table 7). Showing similar results as other preys and seeds, the frogs in primary forest may not have a lower predation pressure compared to frogs in more disturbed habitats.
This study finds no conclusive evidence that predation likelihood is linked to habitat degradation. Our experiments using different artificial prey and seeds show that primary tropical montane forests may not have a lower predation probability than more disturbed systems. Conversely, the disturbed or degraded montane forests may not necessarily have increased predation. Hence, our observations contrasted with the general view that predation increases with disturbance intensity (e.g., Guariguata et al., 2002; Huhta et al., 2003; Stephens et al., 2003; Posa et al., 2007; Ruiz-Guerra et al., 2012; Tvardikova and Novotny, 2012; Vetter et al., 2013).
Our results, however, also yield two repeatable patterns: First, artificial nest predation at Fraser primary was consistently lower than its disturbed counterparts (Fraser edge, probability = 79%; and Fraser rural, 98%). Second, artificial caterpillar predation at Cameron primary was repeatedly lower than its disturbed counterparts (Cameron secondary, probability = 98%; Cameron rural, 97%; and Cameron tea, 89%). These patterns imply that the association of predation likelihood with habitat degradation may be context dependent, influenced by the interaction between prey type and locality; and they warrant further investigation. Varied responses of predator species in each area to degradation, as well as other complex dynamics which influence predators, could also be drivers of some of these patterns (see Laurance, 1991; Turner and Corlett, 1996; Schmitz et al., 2010; Doherty et al., 2015). With respect to statistical power, these clear predation patterns—supported by their relatively high probabilities—indicate that our sample size was sufficient.
We plotted an NMDS ordination of the experimental stations (with presence and absence of predation) and vegetation vectors, with the stress value of 0.12 for the final solution on 2 dimensions, for all artificial prey and seeds (Figure 3).
Figure 3. Non-metric multidimensional scaling plots showing predation across of different prey items–(A) artificial nests; (B) artificial seeds (C) caterpillar models; and (D) frog models—across all sites, with sites where predation occurred and did not occur indicated, and each variable included in the analysis plotted accordingly. AGB, aboveground biomass; CC, canopy cover; DT, dead trees; GC, ground cover; SC, shrub cover; and UV, understorey volume. Open circles are sites where predation occurred, and black circles where artificial prey models were left untouched.
The NMDS ordination of artificial nests showed a distinct cluster of experimental stations with predated nests broadly formed in both lower quadrants, in relation to the vegetation variables (Figure 3A). There was evidence of collinearity between shrub cover and understorey volume. Model selection revealed that only the aboveground biomass was included in the most parsimonious model (Δi = 0). The coefficient of aboveground biomass in this model estimated at −1.66 suggests that artificial nest predation was associated with lower aboveground biomass. Other vegetation variables such as canopy cover (coefficient = −0.61), dead trees (coefficient = 0.30) and ground cover (coefficient = 1.85) appeared in other robust models with Δi < 2 (Table 3), indicating that predation was lower at experimental stations with less canopy cover; and may be associated with more dead trees and greater ground cover. Hierarchical partitioning confirmed that aboveground biomass was the most important vegetation variable, independently explaining 51% of artificial nest predation (Figure 4A).
Table 3. Standardized binary logistic regression coefficients and AICc values for top models with Δi < 2.0 predicting predation events of (A) artificial nest; (B) artificial seed; (C) caterpillar model; and (D) frog model.
Figure 4. Hierarchical partitioning graphs, showing the independent effect (in terms of percentage) of each vegetation structure variable on the likelihood of predation occurring for (A) artificial nests; (B) artificial seeds; (C) caterpillar models; and (D) frog models. Scales differ between each graph. AGB, aboveground biomass; CC, canopy cover; DT, dead trees; GC, ground cover; and UV, understorey volume.
The ordination of artificial seed predation shows a distinct cluster of experimental stations, with predated seeds broadly occupying the lower and upper left quadrants in relation to vegetation variables (Figure 3B). Model selection revealed that only aboveground biomass and dead trees were included in the most parsimonious model (Δi = 0). The coefficients of aboveground biomass and dead trees in this model were estimated at 2.47 and 0.60, respectively, suggesting that artificial seed predation was associated with higher aboveground biomass and more dead trees. Ground cover (coefficient = 2.53) and understorey volume (coefficient = 0.55–0.62) appeared in some robust models with Δi <2 (Table 3), indicating that artificial seed predation may be associated with greater ground cover and understorey volume. Hierarchical partitioning showed that the aboveground biomass was the most important vegetation variable, independently accounting 42% of artificial seed predation (Figure 4B).
The ordination of caterpillar models shows a distinct cluster of experimental stations with predated seeds broadly occupying the upper right quadrant, in relation to the vegetation variables (Figure 3C). Model selection revealed that aboveground biomass, dead trees, ground cover, and understorey volume were included in the most parsimonious model (Δi = 0). The estimated coefficients of aboveground biomass (2.74), ground cover (7.38); and understorey volume (1.10) were all positive in this model. Dead trees were an exception to this (coefficient = −2.29). This suggests that caterpillar model predation was associated with higher aboveground biomass, ground cover, and understorey volume, with fewer dead trees. Canopy cover did not appear in any of the robust models with Δi <2 (Table 3). These indicate that the caterpillar model predation may not have any association with canopy cover. Hierarchical partitioning showed that the number of dead trees is the important vegetation variable, independently accounting for 33% of the artificial caterpillar predation outcome (Figure 4C); while understorey volume and aboveground biomass accounted for 25 and 23%, respectively.
Lastly, the ordination of frog models shows that the experimental stations with predated artificial frogs were distinctly clustered in the lower left quadrant (Figure 3D). Model selection revealed that only understorey volume (coefficient = 0.68) was include in the most parsimonious model (Δi = 0), suggesting that artificial frog predation was associated with higher understorey volume. Other vegetation variables such as aboveground biomass (coefficient = 1.70), canopy cover (coefficient = −0.93 to −1.63), dead trees (coefficient = −0.44), ground cover (coefficient = 3.21) and understorey volume (coefficient = 0.71 to 1.08) appeared in other robust models with Δi < 2 (Table 3), indicating that artificial frog predation was higher at experimental stations with less canopy cover and fewer dead trees; and may be associated with greater vegetation cover. Hierarchical partitioning showed that the understorey volume was the most important vegetation variable, independently explaining 70% of the artificial frog predation outcome (Figure 4D).
Different artificial prey and seeds demonstrated idiosyncratic vegetation characteristics associated with their predation, which may be explained by the microhabitat requirements of their predators during hunting (Martin, 1995; Seibold et al., 2013; Dagan and Izhaki, 2020). Model selection and hierarchical partitioning showed that vegetation cover may be the most influential variable for predation. Specifically, higher nest predation in tropical montane forest may be associated with lower aboveground biomass and canopy cover—a biotic environment which could increase the exposure and visibility of the nests to predators (Martin, 1995). On the other hand, higher predation of seeds, caterpillars and frogs may be associated with higher vegetation cover, which potentially provides predators with protection (Seibold et al., 2013; Dagan and Izhaki, 2020).
Our study showed that (1) predation probability differs between primary forests of different mountains, despite that they belong to the same mountain range; (2) there is no clear trend of predation probability along a habitat disturbance gradient; and (3) the predation probability of a prey item or seed may be influenced by the vegetation variables of its immediate surroundings. The use of multiple prey items clearly showed that predation risk for each can vary considerably and thus cautions against deploying a single prey type to draw a broad inference of predation in degraded systems. Investigating patterns using both microhabitat and the landscape scale broadened the inferences we could make about the drivers of predation in the study area. Future research should be directed toward understanding how vegetation structure can affect predator behaviors and their hunting efficiency in different montane habitats. Incorporating habitat fragmentation as a factor into the future study design will also help us to gauge the potential conservation value of human-modified landscape in a tropical mountain forest.
The data is already available online on the Knowledge Network for Biocomplexity. https://knb.ecoinformatics.org/#view/knb.1380.1.
KP and MS conceived the research. KM and MS carried out the field experiments. KM did the analyses and wrote the paper. All authors commented on the drafts.
This study was funded by the Institute of Life Sciences, University of Southampton, grant no. 511206105.
The authors declare that the research was conducted in the absence of any commercial or financial relationships that could be construed as a potential conflict of interest.
We thank the Economic Planning Unit and the Department of Wildlife and National Parks of Malaysia for supporting our project (permit number: UPE 40/200/19/3295). We would like to thank Marcus Lee for his tireless help during fieldwork. Caroline Murray and Blake Roberts also deserve many thanks for their assistance in making the artificial models.
The Supplementary Material for this article can be found online at: https://www.frontiersin.org/articles/10.3389/ffgc.2020.00080/full#supplementary-material
Akaike, H. (1973). Maximum likelihood identification of Gaussian autoregressive moving average models. Biometrika 60, 255–265. doi: 10.1093/biomet/60.2.255
Barton, K. (2016). MuMIn: Multi-Model Inference. Available online at: https://cran.r-project.org/web/packages/MuMIn/index.html (assessed September 24, 2019).
Bazzaz, F. A., and Pickett, S. T. A. (1980). Physiological ecology of tropical succession: a comparative review. Annu. Rev. Ecol. Syst. 11, 287–310. doi: 10.1146/annurev.es.11.110180.001443
Boyle, M. J. W. (2012). Quantifying predation pressure along a gradient of land use intensity in Sabah, Borneo. [M.Sc. thesis]. Imperial College London. Available online at: http://www.safeproject.net/wp-content/uploads/2012/11/boyle-thesis.pdf (assessed September 24, 2019).
Carlson, A., and Hartman, G. (2001). Tropical forest fragmentation and nest predation – an experimental study in an Eastern Arc montane forest, Tanzania. Biodivers. Conserv. 10, 1077–1085. doi: 10.1023/A:1016649731062
Chapman, H., Cordeiro, N. J., Dutton, P., Wenny, D., Kitamura, S., Kaplin, B., et al. (2016). Seed-dispersal ecology of tropical montane forests. J. Trop. Ecol. 32, 437–454. doi: 10.1017/S0266467416000389
Chinchilla, F. A. (2009). Seed predation by mammals in forest fragments in Monteverde, Costa Rica. Rev. Biol. Trop. 57, 865–877. doi: 10.15517/rbt.v57i3.5499
Dagan, U., and Izhaki, I. (2020). Vegetation structure governs nest predation in three types of conifer forest habitats. Eur. J. For. Res. doi: 10.1007/s10342-020-01279-3. [Epub ahead of print].
Dobson, A., Lodge, D., Alder, J., Cumming, G. S., Keymer, J., McGlade, J., et al. (2006). Habitat loss, trophic collapse, and the decline of ecosystem services. Ecology 87, 1915–1924. doi: 10.1890/0012-9658(2006)87[1915:HLTCAT]2.0.CO;2
Doherty, T. S., Dickman, C. R., Nimmo, D. G., and Ritchie, E. G. (2015). Multiple threats, or multiplying the threats? interactions between invasive predators and other ecological disturbances. Biol. Conserv. 190, 60–68. doi: 10.1016/j.biocon.2015.05.013
Doust, S. J. (2011). Seed removal and predation as factors affecting seed availability of tree species in degraded habitats and restoration plantings in rainforest areas of Queensland, Australia. Restor. Ecol. 19, 617–626. doi: 10.1111/j.1526-100X.2010.00681.x
Edwards, P. J., and Grubb, P. J. (1977). Studies of mineral cycling in a montane rain forest in New Guinea: I. the distribution of organic matter in the vegetation and soil. J. Ecol. 65:943. doi: 10.2307/2259387
Emmering, Q. C., and Schmidt, K. A. (2011). Nesting songbirds assess spatial heterogeneity of predatory chipmunks by eavesdropping on their vocalizations. J. Anim. Ecol. 80, 1305–1312. doi: 10.1111/j.1365-2656.2011.01869.x
Fontaine, J., and Martin, T. (2006). Parent birds assess nest predation risk and adjust their reproductive strategies. Ecol. Lett. 9, 428–434. doi: 10.1111/j.1461-0248.2006.00892.x
Goldsmith, S., Gillespie, H., and Weatherby, C. (2007). Restoration of Hawaiian montane wet forest: endemic longhorned beetles (Cerambycidae: Plagithmysus) in Koa (Fabaceae: Acacia koa) plantations and intact forest. Southwest. Nat. 52, 356–363. doi: 10.1894/0038-4909(2007)52[356:ROHMWF]2.0.CO;2
Guariguata, M. R., Claire, H. A.-L., and Jones, G. (2002). Tree seed fate in a logged and fragmented forest landscape, Northeastern Costa Rica. Biotropica 34, 405–415. doi: 10.1111/j.1744-7429.2002.tb00554.x
Hox, J. J. C. M., van de Schoot, R., and Matthijsse, S. (2012). How few countries will do? comparative survey analysis from a Bayesian perspective. Surv. Res. Methods 6, 87–93. doi: 10.18148/srm/2012.v6i2.5033
Huhta, E., Jäntti, A., Suorsa, P., Aho, T., Kuitunen, M., Nikula, A., et al. (2003). Habitat-related nest predation effect on the breeding success of the Eurasian treecreeper. Écoscience 10, 283–288. doi: 10.1080/11956860.2003.11682775
Laurance, W. F. (1991). Edge effects in tropical forest fragments: Application of a model for the design of nature reserves. Biol. Conserv. 57, 205–219. doi: 10.1016/0006-3207(91)90139-Z
Martin, T. (1995). Avian life history evolution in relation to nest and food. Ecol. Monogr. 65, 101–127. doi: 10.2307/2937160
Martin, T. E., Scott, J., and Menge, C. (2000). Nest predation increases with parental activity: separating nest site and parental activity effects. Proc. Biol. Sci. 267, 2287–2293. doi: 10.1098/rspb.2000.1281
Meredith, M. (2017). wiqid: Quick and Dirty Estimates for Wildlife Populations. Available online at: https://cran.r-project.org/web/packages/wiqid/index.html (assessed September 24, 2019).
Myster, R. W. (2015). Seed predation, pathogens and germination in primary vs. secondary cloud forest at Maquipucuna Reserve, Ecuador. J. Trop. Ecol. 31, 375–378. doi: 10.1017/S0266467415000127
Oksanen, J., Blanchet, F. G., Friendly, M., Kindt, R., Legendre, P., McGlinn, D., et al. (2017). vegan: Community Ecology Package. Available online at: https://cran.r-project.org/web/packages/vegan/index.html (accessed December 19, 2017).
Paluh, D. J., Hantak, M. M., and Saporito, R. A. (2014). A test of aposematism in the dendrobatid poison frog oophaga pumilio : the importance of movement in clay model experiments. J. Herpetol. 48, 249–254. doi: 10.1670/13-027
Peh, K. S., Soh, M. C., Sodhi, N. S., Laurence, W. F., Ong, D. J., and Clements, R. (2011). Up in the clouds: is sustainable use of tropical montane cloud forests possible in Malaysia? BioScience 61, 27–38. doi: 10.1525/bio.2011.61.1.8
Pender, R. J., Shiels, A. B., Bialic-Murphy, L., and Mosher, S. M. (2013). Large-scale rodent control reduces pre- and post-dispersal seed predation of the endangered Hawaiian lobeliad, Cyanea superba subsp. superba (Campanulaceae). Biol. Invasions 15, 213–223. doi: 10.1007/s10530-012-0280-3
Posa, M. R. C., Sodhi, N. S., and Koh, L. P. (2007). Predation on artificial nests and caterpillar models across a disturbance gradient in Subic Bay, Philippines. J. Trop. Ecol. 23, 27–33. doi: 10.1017/S0266467406003671
R Core Team (2017). R: A Language and Environment for Statistical Computing. Available online at: https://www.r-project.org/
Robinson, W. D., Styrsky, J. N., and Brawn, J. D. (2005). Are artificial bird nests effective surrogates for estimating predation on real bird nests? a test with tropical birds (son los nidos artificiales sustitutos efectivos para estimar la depredación de nidos verdaderos? Una prueba con Aves Tropicales). Auk 122, 843–852. doi: 10.1093/auk/122.3.843
Rodenhouse, N. L., Sherry, T. W., and Holmes, R. T. (1997). Site-dependent regulation of population size : a new synthesis. Ecology 78, 2025–2042. doi: 10.2307/2265942
Ruiz-Guerra, B., Renton, K., and Dirzo, R. (2012). Consequences of fragmentation of tropical moist forest for birds and their role in predation of herbivorous insects. Biotropica 44, 228–236. doi: 10.1111/j.1744-7429.2011.00795.x
Schmitz, O., Hawlena, D., and Trussel, G. (2010). Predator control of ecosystem nutrient dynamics. Ecol. Lett. 13, 1199–1209. doi: 10.1111/j.1461-0248.2010.01511.x
Sedláček, O., Mikeš, M., Albrecht, T., Horák, D., and Reif, J. (2014). Evidence for an edge effect on avian nest predation in fragmented afromontane forests in the Bamenda-Banso Highlands. Trop. Conserv. Sci. 7, 720–732. doi: 10.1177/194008291400700410
Seibold, S., Hempel, A., Piehl, S., Bässler, C., Brandl, R., Rösner, S., et al. (2013). Forest vegetation structure has more influence on predation risk of artificial ground nests than human activities. Basic Appl. Ecol. 14, 687–693. doi: 10.1016/j.baae.2013.09.003
Sih, A. (2013). Understanding variation in behavioural responses to human-induced rapid environmental change: a conceptual overview. Anim. Behav. 85, 1077–1088. doi: 10.1016/j.anbehav.2013.02.017
Sih, A., Bolnick, D. I., Luttbeg, B., Orrock, J. L., Peacor, S. D., Pintor, L. M., et al. (2010). Predator-prey naïveté, antipredator behavior, and the ecology of predator invasions. Oikos 119, 610–621. doi: 10.1111/j.1600-0706.2009.18039.x
Sih, A., Crowley, P., McPeek, M., Petranka, J., and Strohmeier, K. (1985). Predation, competition, and prey communities: a review of field experiments. Ann. Rev. Ecol. Evol. Syst. 16, 269–311. doi: 10.1146/annurev.es.16.110185.001413
Sodhi, N. S., Peh, K. S.-H., Ming Lee, T., Turner, I. M., Tan, H. T. W., Prawiradilaga, D. M., et al. (2003). Artificial nest and seed predation experiments on tropical southeast Asian islands. Biodivers. Conserv. 12, 2415–2433. doi: 10.1023/A:1025852214528
Soh, M. C. K., Mitchell, N. J., Ridley, A. R., Butler, C. W., Puan, C. L., and Peh, K. S.-H. (2019). Impacts of habitat degradation on tropical montane biodiversity and ecosystem services: a systematic map for identifying future research priorities. Front. For. Global Change 2:83. doi: 10.3389/ffgc.2019.00083
Soh, M. C. K., Sodhi, N. S., and Lim, S. L. H. (2006). High sensitivity of montane bird communities to habitat disturbance in Peninsular Malaysia. Biol. Conserv. 129, 149–166. doi: 10.1016/j.biocon.2005.10.030
Spanhove, T., Callens, T., Hallmann, C., Pellikka, P., and Lens, L. (2014). Nest predation in Afrotropical forest fragments shaped by inverse edge effects, timing of nest initiation and vegetation structure. J. Ornithol. 155, 411–420. doi: 10.1007/s10336-013-1021-9
Stephens, S., Koons, D., Rotella, J., and Willey, D. (2003). Effects of habitat fragmentation on avian nesting success: a review of the evidence at multiple spatial scales. Biol. Conserv. 115, 101–110. doi: 10.1016/S0006-3207(03)00098-3
Thompson, F. R., and Burhans, D. E. (2004). Differences in predators of artificial and real songbird nests: evidence of bias in artificial nest studies. Conserv. Biol. 18, 373–380. doi: 10.1111/j.1523-1739.2004.00167.x
Turner, I. M., and Corlett, R. T. (1996). The conservation value of small, isolated fragments of lowland tropical rain forest. Trends Ecol. Evol. 11, 330–333. doi: 10.1016/0169-5347(96)10046-X
Tuyl, F., Gerlach, R., and Mengersen, K. (2009). Posterior predictive arguments in favor of the Bayes-Laplace prior as the consensus prior for binomial and multinomial parameters. Bayesian Anal. 4, 151–158. doi: 10.1214/09-BA405
Tvardikova, K., and Novotny, V. (2012). Predation on exposed and leaf-rolling artificial caterpillars in tropical forests of Papua New Guinea. J. Trop. Ecol. 28, 331–341. doi: 10.1017/S0266467412000235
Vetter, D., Rücker, G., and Storch, I. (2013). A meta-analysis of tropical forest edge effects on bird nest predation risk: edge effects in avian nest predation. Biol. Conserv. 159, 382–395. doi: 10.1016/j.biocon.2012.12.023
Walsh, C., and Nally, R. M. (2013). Package “hier.part”: Hierarchical Partitioning. Available online at: https://cran.r-project.org/web/packages/hier.part/hier.part.pdf (assessed September 24, 2019).
Keywords: artificial prey, Southeast Asia, tropical cloud forest, habitat structure, statistical modeling
Citation: Murray K, Soh MCK, Puan CL, Abu Hashim AK, Abdul Patah P and Peh KS-H (2020) Predation on Multiple Prey Types Across a Disturbance Gradient in Tropical Montane Forests of Peninsular Malaysia. Front. For. Glob. Change 3:80. doi: 10.3389/ffgc.2020.00080
Received: 24 September 2019; Accepted: 09 June 2020;
Published: 24 July 2020.
Edited by:
Norma Salinas, Pontifical Catholic University of Peru, PeruReviewed by:
Matthew Brolly, University of Brighton, United KingdomCopyright © 2020 Murray, Soh, Puan, Abu Hashim, Abdul Patah and Peh. This is an open-access article distributed under the terms of the Creative Commons Attribution License (CC BY). The use, distribution or reproduction in other forums is permitted, provided the original author(s) and the copyright owner(s) are credited and that the original publication in this journal is cited, in accordance with accepted academic practice. No use, distribution or reproduction is permitted which does not comply with these terms.
*Correspondence: Kieran Murray, a2llcmFubXVycmF5OTVAZ21haWwuY29t; Kelvin S.-H. Peh, a2VsdmluLnBlaEBnbWFpbC5jb20=
Disclaimer: All claims expressed in this article are solely those of the authors and do not necessarily represent those of their affiliated organizations, or those of the publisher, the editors and the reviewers. Any product that may be evaluated in this article or claim that may be made by its manufacturer is not guaranteed or endorsed by the publisher.
Research integrity at Frontiers
Learn more about the work of our research integrity team to safeguard the quality of each article we publish.